An official website of the United States government
Official websites use .gov A .gov website belongs to an official government organization in the United States.
Secure .gov websites use HTTPS A lock ( Lock Locked padlock icon ) or https:// means you've safely connected to the .gov website. Share sensitive information only on official, secure websites.
- Publications
- Account settings
- Advanced Search
- Journal List

Effect of social media overload on college students’ academic performance under the COVID-19 quarantine
Qingfang zhang, xianghua yue.
- Author information
- Article notes
- Copyright and License information
Edited by: Athapol Ruangkanjanases, Chulalongkorn University, Thailand
Reviewed by: Yanliang Yu, Dongguan University of Technology, China; Muhammad Khalid Anser, Xi'an University of Architecture and Technology, China
*Correspondence: Xianghua Yue, [email protected]
This article was submitted to Organizational Psychology, a section of the journal Frontiers in Psychology
Received 2022 Mar 5; Accepted 2022 Aug 2; Collection date 2022.
This is an open-access article distributed under the terms of the Creative Commons Attribution License (CC BY). The use, distribution or reproduction in other forums is permitted, provided the original author(s) and the copyright owner(s) are credited and that the original publication in this journal is cited, in accordance with accepted academic practice. No use, distribution or reproduction is permitted which does not comply with these terms.
Features and relevant services of online social media have been attracting users during the COVID-19 pandemic. Previous studies have shown that college students tend to use social media more frequently than other groups. However, in being affected by social media overload, the social media use behaviors of many college students have been out of their control in terms of their capabilities or cognition. Based on the stressor–strain–outcome (SSO) model and the theory of compensatory internet use (TCIU), we developed a research model to study the causes of social media overload and its impact on college students’ academic performance during the COVID-19 pandemic. A total of 441 valid responses from college students through questionnaires in China are collected via purposive sampling and used in the data analysis. This study conducts PLS-SEM to analyze collected data, finding that boredom proneness is associated with overload (stress), which has a bearing on social media overload (strain) and the reduction in final performance (outcome). Through illustrating the psychological and behavioral conditions that hinder the academic performance of students, this study provides deeper insights into students’ uncontrollable use of social media. Moreover, with respect to the identified antecedents, this study aims to find solutions to mitigate the impact of social media overload resulting from boredom proneness on the academic performance of college students.
Keywords: boredom proneness, information overload, communication overload, system feature overload, academic performance
Introduction
The COVID-19 pandemic has dramatically hit the social operation in the world, resulting in a diversity of risks and challenges facing organizations (Cao; Said et al., 2021 ). In 2021, some regions of the Chinese mainland implemented lockdown policies to curb COVID-19. Boredom proneness during the lockdown period caused the improper use of social media, which may have affected the psychological health of individuals, especially those born between the mid-1990s and early 2010s ( Priporas et al., 2017 ). Thus, we took college students under quarantine and lockdown policies as the research samples to discuss whether college students were experiencing overload triggered by boredom proneness during quarantine. Affected by the COVID-19, many university cannot provide suitable studying environment, and even the class suspension, so the overload intention may come about when the psychological health of individuals. Boredom proneness is described as “the lack of interest in and the difficulty in focusing on current activities” ( Mikulas and Vodanovich, 1993 ; Fisher, 1998 ; Kass et al., 2010 ). Opinions given in this study contribute to deepening awareness and understanding of the excessive use of social media among college students ( Bright et al., 2015 ; Li, 2018 ; Salo et al., 2018 ; Turel et al., 2019 ). Most of the existing studies explore how to enhance classroom collaboration using social media and promote the use of social media in a higher education context ( Al-Rahmi et al., 2018 ) but neglect the correlation between boredom proneness, social media overload and the academic performance of students ( Cao and Sun, 2018 ). The ability to develop evidence-based interventions and solutions is compromised as existing research has largely overlooked the connection between boredom, social media use, and social media overload ( Cao and Sun, 2018 ).
The excessive use of social media may result in overload. We define social media overload according to three common dimensions: information overload, communication overload and system feature overload ( Bowker, 2008 ). Seldom has research investigated the antecedents of information, communication, and system feature overloads on social media ( Lee et al., 2016 ). Previous research has mainly concentrated on the negative correlation between students’ use of social media and student engagement ( Junco, 2015 ; Datu et al., 2018 ). For instance, multitasking behaviors provided by social media are correlated with student engagement ( Junco and Cotten, 2012 ; le Roux and Parry, 2017 ) and well-being ( Becker et al., 2013 ). Although the wide use of social media may cause stress for students and thus affect their academic performance ( Cao et al., 2018 ), seldom have studies used theoretical mechanisms to demonstrate how social media affects the academic performance of students. Giunchiglia et al. (2018) confirmed that the duration of usage social media apps negatively affected students’ academic performance. We aim to expand existing studies using the stressor–strain–outcome (SSO) model in combination with the theory of compensatory internet use ( Davis and Luthans, 1980 ) and to further discuss how boredom proneness causes the perception of social media overload (organism) and how the social media overload subsequently results in a reduction in academic performance (outcome).
The daily life of college students has changed enormously due to the outbreak of COVID-19 ( Khanra et al., 2021 ). College students are the major user group of social media, with a use level higher than average ( Maier et al., 2015 ; Turner, 2015 ). The enforcement of lockdown policies on the Chinese mainland gradually led to an issue regarding the use of social media among college students, resulting in a deviation from its original intended use ( Liu et al., 2021 ). Due to their intense usage of and limited external control over their Internet use, extensive free time, and flexible schedules, university students are more disposed than others to develop problematic social media use ( Turel and Qahri-Saremi, 2016 ). In this paper, we investigate the etiology and consequences of social media overload among university students, the phenomenon in which the extensive adoption and use of social media has exposed people to a massive amount of information and communication demands that may require energy and cognitive processing beyond their capabilities ( Lee et al., 2016 ). In addition, since the COVID-19 broke out in 2019, previous literature has failed to consider special context factors and cannot be used to demonstrate the reasons and psychological mechanisms for social media overload appearing in the lockdown period. Different from previous research, we first of all emphasize that the boredom proneness brought about by quarantine policies has led to social media overload among college students. Second, we investigate a situational state during the quarantine period, which has shaped a process stemming from inner feelings, which generates the social media overload and causes the decline in college students’ academic performance. We have found that social media overload results from the interaction of boredom proneness with three dimensions of overload (information overload, communication overload and system feature overload), thus ultimately affecting the academic performance of college students.
Literature review and theory development
Theory of compensatory internet use.
The TCIU is a contemporary theory that has been widely applied in social media as an extension of the uses and gratifications theory ( Elhai et al., 2017 , 2020 ; Tandon et al., 2020 ). The novelty of TCIU lies in its particular focus on psychopathology as a motivator of problematic internet or social media use ( Elhai et al., 2017 ). Information and communication overload can occur when people turn to social media in order to alleviate their boredom. Therefore, information overload and communication overload are conceptualized as strain factors. Strain can lead to various negative outcomes, such as dissatisfaction, emotional exhaustion, fatigue, or even the discontinued negative with academic performance ( Ragu-Nathan et al., 2008 ; Tarafdar et al., 2011 ; Maier et al., 2015 ). As stated by TCIU, individuals may overuse technologies (e.g., social media) to deal with or compensate for social needs that are perceived as lacking ( Wang et al., 2018 ) and negative emotions or stressors related to their living environment ( Wolniewicz et al., 2018 ). On the basis of TCIU, we consider that college students who are experiencing boredom proneness, which is a negative emotion ( Tandon et al., 2021 ), would increase their use of social media to cope with and compensate for it. We hold the same arguments as those of previous studies that individuals are more inclined to deal with perceived negative emotions ( Tandon et al., 2020 ) and unsatisfied requirements ( Wang et al., 2018 ; Wolniewicz et al., 2018 ) through social technologies, such as smart phones and social media. Thus, we consider TCIU an appropriate theory to establish the theoretical framework of our study. However, some scholars assert that TCIU has been focusing on the psychopathology and negative emotions, making it inadequate for providing theoretical support for social media use behaviors ( Wolniewicz et al., 2018 ). As a result, we contend that TCIU is not able to offer a comprehensive outlook for the study of the proposed associations, especially overload. Therefore, we complement TCIU with the SSO model to consider the associations between boredom proneness, overload and fatigue. In this paper, we investigate the etiology and consequences of social media overload among university students, the phenomenon in which the extensive adoption and use of social media has exposed people to a massive amount of information and communication demands that may require energy and cognitive processing beyond their capabilities ( Lee et al., 2016 ).
Our research model has been developed based on TCIU to explain the stress process combined with the SSO model ( Koeske and Koeske, 1993 ). The SSO model developed by Koeske and Koeske (1993) was originally applied in psychology studies to describe the stress process. On the grounds of this framework, stressors exert an influence on users, thus leading to their behavioral outcome. In the SSO model, stressors are defined as environmental stimuli that are, as believed by individuals, stimulating, troublesome or destructive. Common stressors include overload, conflict and intrusion ( Ayyagari et al., 2011 ; Bucher et al., 2013 ; Maier et al., 2015 ). Strain is an individual’s psychological response to stressors and may have a frustrating effect on an individual’s attention, physical condition and emotion ( Cheung and Tang, 2010 ). Studies of strains, such as emotional exhaustion and overload, are very widely conducted in relevant studies ( Choi et al., 2014 ; Zhang et al., 2016 ). In organizations, stressors are the conditions for factors generating stress, and strain is the psychological outcome of stress. Furthermore, stress is a modulator for the effect of perceived stressors on outcome variables ( Lee et al., 2016 ). Prior studies of stress contend that reduced organizational commitment, poor job performance and discontinuity of intention are the outcomes of stress ( Ragu-Nathan et al., 2008 ; Kim et al., 2012 ). In the past, the SSO model was commonly used to study a stress-related situation and its outcomes in the context of technology use ( Ragu-Nathan et al., 2008 ; Ayyagari et al., 2011 ; Dhir et al., 2018 ). For example, Cheung and Tang (2010) investigated how job stressors (including job characteristics and emotional dissonance) affect employees’ subjective health and work outcome ( Choi et al., 2014 ). Studies have shown that social stress related to customers has a positive effect on emotional exhaustion, which, in turn, has a negative effect on customer orientation and the performance of service restoration. These studies mainly discuss job stress in workplaces. As social media has been extensively used among college students, they are more likely to spend a lot of time on social media, resulting in the excessive use of social media related to technology stress and further bringing about negative outcomes ( Pavlou et al., 2007 ; Aladwani and Almarzouq, 2016 ). In the context of COVID-19, the excessive use of social media and the social media overload caused by boredom proneness among college students constitute stressors that affect individuals’ emotions and attitudes (e.g., overload, regret, or discontent) toward social media, and these stressors will further give rise to adverse outcomes such as a decline in academic performance ( Cao and Sun, 2018 ; Dhir et al., 2018 ; Nawaz et al., 2018 ; Yu et al., 2018 ). This is entirely in line with the major purpose of this study, that is, how stress-related factors trigger social media overload and thus a decline in academic performance (outcome). Consequently, we use the SSO model as the theoretical basis to discuss the negative effect of social media overload resulting from boredom proneness on the academic performance of college students.
Boredom proneness and overload
To be sure, social media is able to facilitate friendship development and maintenance, social contact well-being and knowledge exchange among college students, but it may bring about negative outcomes when it is used beyond individuals’ available resources and energy ( Karr-Wisniewski and Lu, 2010 ). In the context of social media, overload is the key factor for such negative outcomes ( Lee et al., 2016 ). The overload derives from the imbalance between the unexpected demands in the context and the limited handling ability of individuals ( Edwards and Cooper, 1990 ).
Overload, as a typical stressor, has been extensively examined in different research fields such as work overload ( Ayyagari et al., 2011 ), connection overload ( LaRose et al., 2014 ) and social overload ( Maier et al., 2012 ), for some people, this has resulted in overload, which refers to a mismatch between environmental requirements and an individual’s ability to cope. The topic of overload has been studied by many scholars, who argue that overload can be divided into information overload, communication overload and system feature overload ( Bowker, 2008 ). Information overload will appear when the information on social media is too much to be handled properly by an individual, especially when the information is presented in an excessively fast and discontinuous manner. Users reported feeling low on energy and unable to concentrate on important tasks after a period of heavy social media use ( Whelan et al., 2013 ). Communication overload refers to when individuals’ communication skills are challenged when too many communication demands are embedded in social media. System feature overload refers to when the features provided on social media exceed the users’ demands ( Thompson et al., 2005 ). To be specific, the younger generations, like college students, are the major participants in social activities on social media ( Kirschner and Karpinski, 2010 ), and have a strong desire for social contact. Since the interpersonal relationship in a social network is always reciprocal, individuals feel that they are obliged to meet the demands of online friends in the form of emotional or material support ( Maier et al., 2012 ). Individuals are likely to become exhausted in the face of an increasing number of social support requests if they are not able to satisfy such requests, and they may also feel uncomfortable regarding their too frequent responses to such social requests, thus leading to a perception of social contact burden ( Maier et al., 2015 ). Once the number of online social requests goes beyond the handling ability of users, a troublesome environmental stimulus will be generated, leading to a series of emotional and behavioral reactions ( Evans et al., 2000 ). This unwholesome state has been proven by experience to be a stressor that exerts a negative influence on one’s personal life ( Maier et al., 2015 ). Similar to the way that boredom proneness has been found to generate stress in social media users ( Whelan et al., 2013 ), we conceptualize boredom proneness as a stressor for the same population. For example, learners must pay attention to learning materials and other activities, such as familiarizing themselves with increasingly complex SNS system features, responding to demanding social support from friends, confronting the distractive advertising, etc. These overloads lead to negative emotional responses such as stress, frustration, and anxiety, which also occur in learning communities. When individuals feel threatened by the physical and psychological strain generated by SNSs, they have to prevent or reduce the adverse outcomes and restore emotional stability, thus threatening the sustainability of SNS’s massive benefits for people. Therefore, we consider information, communication and system feature overloads as stressors and three dimensions of overload related to the overuse of social media.
College students might have sought out other experiences to relieve boredom proneness during the quarantine and lockdown period, even though these experiences might have caused negative impact ( Bench and Lench, 2013 ). Boredom proneness is an undesirable feeling, so the human brain looks for external stimuli to avoid it. Social media provides almost endless experiences, which attract scores of people to pass time and seek out spiritual and emotional stimuli. However, excessive stimuli may be a bad thing. Prior studies of addiction have mentioned that boredom proneness can result in some adverse outcomes, such as social media overload. Social media become a kind of pathological pursuit for users to relieve their boredom proneness, thus resulting in the issue of overload ( Turel et al., 2014 , 2016 , 2019 ). Many prior studies have explained why people overuse social media, and boredom proneness is a significant influencing factor recognized by scholars; seeking out entertainment and killing time are powerful predictive factors for the use of social media platforms ( Quan-Haase and Young, 2010 ; Ku et al., 2013 ). Boredom proneness drives people to seek more stimulating activities in social media ( Bench and Lench, 2013 ; Alter, 2017 ), which then lead to information, communication and system feature overloads.
According to the logic of addiction studies described above, adverse outcomes will bring about information, communication and system feature overloads when people try to relieve their boredom proneness using social media. Thus, we propose the following hypotheses:
H1 : Boredom proneness is positively correlated with information overload. H2 : Boredom proneness is positively correlated with communication overload. H3 : Boredom proneness is positively correlated with system feature overload.
Antecedents of overload
We consider the overload examined in this study as psychological stress, which is an individual psychological state, and the behavioral outcomes that can be affected by cognitive bias ( Brooks and Califf, 2017 ; Mahmud et al., 2017 ; Steelman and Soror, 2017 ). In previous studies, overload has been extensively investigated as a special type of psychological tension caused by overloads ( Ahuja et al., 2007 ; Maier et al., 2015 ). In the social media context, an excessive amount of information and interaction may weaken user activation and make them feel tired ( Maier et al., 2015 ). Exhaustion refers to a state of extreme overload caused by the long-term and excessive consumption of spiritual resources ( Schaufeli, 1995 ; Ravindran et al., 2014 ). Moore (2000) investigate social networking service (SNS) exhaustion, which is a psychological reaction to stressors, as an outcome of social overload in the social networking environment. The investigation showed that workload is the strongest predictive factor of employee overload. In our context, overload refers to the disgust and potentially harmful and unconscious psychological reactions to stress conditions when using social media ( Maier et al., 2015 ). It represents the tiredness arising from social media use.
Information overload will occur if the information accessible to users on social media goes beyond their ability to handle it ( Zhang et al., 2016 ). Specifically, the information overload would have resulted if college students made responses that went beyond their management and handling abilities in order to eliminate boredom proneness during the quarantine and lockdown period. It can produce stress and negative emotions, so people cannot easily cope with requests from social media platforms, which is undesirable ( Cao and Sun, 2018 ). Plenty of information is generated on social media as the number of users and their level of activation increases ( Lee et al., 2016 ). As a result, college students need to be able to cope with the incessant information generated on social media, thus causing overload. The overabundance of information on social media may exceed the expected cognitive range of the human brain, making users feel overwhelmed and tired ( Lee et al., 2016 ). The massive amount of information on social media can quickly go beyond the cognitive range of humans, making people feel at a loss ( Wurman, 1990 ). Previous studies have shown that information overload is one of the major antecedents of social media overload in the social media context ( Lee et al., 2016 ; Zhang et al., 2016 ). Confronted with the massive amount of information, users may feel out of control ( Zhang et al., 2016 ) and may regret using social media ( Cao and Sun, 2018 ; Nawaz et al., 2018 ), thereby leading to social media overload ( Cao and Sun, 2018 ). Therefore, users may feel extremely tired on social media when they perceive information overload ( Gao et al., 2018 ). The overload will be evident when users feel that it is difficult to manage large amounts of information and communication from others ( Lee et al., 2016 ). Based on the above statements, we posit the follow hypothesis:
H4 : Information overload is positively correlated with social media overload.
Social media provides a diversity of features to facilitate communication ( Garrett and Danziger, 2007 ; Ou and Davison, 2011 ). Message and conversation requests from other users attracts the attention of users and excessively interferes with their behaviors, resulting in communication overload ( Karr-Wisniewski and Lu, 2010 ; Cao and Sun, 2018 ). As reported by Lee et al. (2016) , communication overload is a source of overload in the context of social media use. Users will perceive a communication overload if there are too many social requests to cope with and be satisfied on social media ( Karr-Wisniewski and Lu, 2010 ). After coping with the communication requests, individuals need take several minutes to recover the interrupted work or learning ( Karr-Wisniewski and Lu, 2010 ; Ou and Davison, 2011 ). Communication overload may repeatedly interrupt the daily learning tasks of college students ( McFarlane and Latorella, 2002 ; Cho et al., 2011 ), making them feel tired and giving rise to more severe spiritual or physical diseases ( Deutsch, 1961 ; Klapp, 1986 ). We, therefore, contend that college students often feel overload due to communication overload.
We develop the following hypothesis:
H5 : Communication overload has a positive impact on social media overload.
System feature overload will appear if the features of applications are not suitable for current tasks or the system features are too complex to complete tasks ( Thompson et al., 2005 ; Karr-Wisniewski and Lu, 2010 ; Zhang et al., 2016 ). In the social media context, system feature overload refers to social medial users’ perception of technology features. It can also be defined as a perception that features provided by social media exceed user demand ( Thompson et al., 2005 ; Zhang et al., 2016 ). If system features on social media often change and are too complex for users, system feature overload will appear and result in adverse outcomes, such as social media overload. For example, Facebook updates its system features and user interface almost once a week ( Fu et al., 2020 ), so users need to spend more time and attention to adapt to these frequent changes. System feature overload is particularly obvious when the design changes do not match user habits ( Ayyagari et al., 2011 ), especially when users have become accustomed to an appearance and find the system changes overwhelming. Users may also experience system feature overload, thus leading to the perception of social media overload ( Lee et al., 2016 ). Frequent unnecessary updates for feature interfaces will lead to social media overload ( Ayyagari et al., 2011 ; Ravindran et al., 2014 ). Hence, we contend that college students may be tired of social media use and feel overloaded when they realize that the cost required to learn and use system features on social media outweighs their benefits.
Based on the above statements, we make the following hypothesis:
H6 : System feature overload has a positive impact on social media overload.
Relationship between overload and academic performance
Indeed, the most popular social media applications are designed to encourage compulsive use ( Alter, 2017 ). Many people are unable to override their impulsive habitual use of social media ( Turel and Qahri-Saremi, 2016 ) and smartphones ( Soror et al., 2015 ). Social media users often do not get all the functionality they want from one platform and need to constantly switch between too many different (non-) technological alternatives, resulting in negative perceptions which Maier et al. (2015) . The relationship between social media use and academic performance has been a hot topic in the education field in recent years ( Liu et al., 2017 ). Meta-analysis has shown that the excessive use of social networking services has a significantly negative association with academic performance ( Aladwani and Almarzouq, 2016 ). It has also found that the compulsive use of social media leads to problematic learning outcomes. As a result, we consider the academic performance of students as an outcome in this study.
Overload is a complex physical and psychological state. Physical overload is clinically defined as “not [being] able to maintain the required or expected strength” ( González-Izal et al., 2012 ). Psychological overload is described as a state of overload that is related to stress and other intensive emotional experience ( Shen et al., 2006 ). Overload is a kind of subjective inner feeling that varies from person to person ( Nail and Winningham, 1995 ). Physical overload is more likely to appear in the mandatory environment related to physical labor but not in the context of social media use, so social media overload can be regarded as a form of psychological overload ( Zhang et al., 2016 ). Overload brings about a variety of outcomes that decrease students’ academic performance ( Junco, 2015 ). Academic performance means the extent to which students achieve their short-term or long-term educational goals. Prior studies have discussed all sorts of negative outcomes caused by social media use ( Tarafdar et al., 2015 ), and reduced academic performance is the most common one. Past studies seldom demonstrate that social media overload can lead to reduced academic performance among college students ( Ayyagari et al., 2011 ).
We contend that social media overload derives from individuals’ dependence on social media, particularly from college students’ excessive use of social media to eliminate boredom proneness during the COVID-19 lockdowns. This has been identified in recent research findings. For example, Islam et al. (2020) found that the increased use of social medial to explore new content (e.g., new information) during lockdowns may have brought about overload and have had a negative impact on academic performance. In the academic activities of college students, the focus on social media platforms may cause an explosion of psychological stress and tiredness, thus weakening their actual performance ( Ayyagari et al., 2011 ). Psychological efforts are required for a good performance in any task ( Boksem and Tops, 2008 ). The performance will decline when people feel tired ( Van der Linden and Eling, 2006 ). Social media overload may have a significantly negative impact on student attention during learning ( Caplan and High, 2006 ). As an example, other studies on American college students have stated that social media overload weakens actual academic performance ( Rosen et al., 2013a , b ; Junco, 2015 ). As reported, teachers commonly worry that social media overload will have a negative impact on the performance of students in various academic activities. Thus, we infer that social media overload has a negative effect on the academic performance of students because it distracts students’ attention from learning.
Based on the above statements, we posit the following hypothesis:
H7 : Social media overload has a negative impact on academic performance.
According to the above hypotheses, the research framework is shown in Figure 1 .
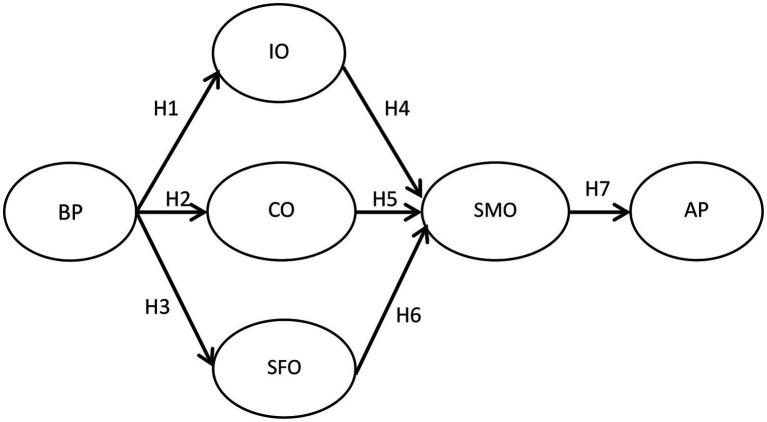
Research framework.
Data collection
We invited college students to engage in the survey through publishing questionnaires on i.chaoxing.com to collect data online. Every participant was informed that their answers would be kept confidential. Thanks to the suspension of offline courses during the lockdown of the city, data were collected for 2 weeks in September 2021. In order to enhance the sample representativeness, researchers select effective sample clusters based on their research purposes and issues. Thus, purposive sampling is adopted, and several conditions will be established during sampling so as to improve the representativeness of the research samples. First, mainland China, where the pandemic was most severe in the beginning, was selected as the main area for sampling, and the quarantine policy was the strictest. Thus, it is representative to a certain extent. Second, to understand the psychological characteristics of college students, it is necessary to focus on those who actually face boredom proneness. Third, while filling the questionnaire, all the samples were already isolated at home. In order to attract broader participation of college students, we offered a coupon valuing two dollars for each participant who completes the questionnaire. Students were required to provide their student number to avoid the repeat submission. All variables were adopted from prior literature and were measured on a five-point Likert scale with response choices ranging from “Strongly disagree (1)” to “Strongly agree (5)” In order to screen social media users from participants, we design a question: Are you a user of some social media? Only the questionnaires submitted by those who answered yes to this question are considered as the valid ones. A total of 500 students participated in this survey, and 15 of them were excluded from the sample pool because they do not use social media. Besides, additional 44 questionnaires were not completed. As a result, we finally obtained 441 valid samples.
When self-report questionnaires are used to collect data at the same time from the same participants, common method variance (CMV) may be a concern. A post hoc Harman one-factor analysis was used to test for CMV ( Podsakoff and Organ, 1986 ). The explained variance in one factor is 40.554%, which is smaller than the recommended threshold of 50%. Therefore, CMV is not problematic in this study to test the research model, a survey instrument was developed with each construct measured using multiple items ( Harman, 1976 ). Most items were adapted from existing measures in the related literature with confirmed content validity and reliability, and then modified to fit our research context. Boredom proneness was measured by four items adapted from Vodanovich et al. (2005) . Information overload were measured by four items adapted from Karr-Wisniewski and Lu (2010) . Communication overload were measured by four items adapted from Karr-Wisniewski and Lu (2010) . System feature overload was measured by three items adapted from ( Lee et al., 2016 ). Social media overload was measured using Maier et al. (2015) instrument (four items). Academic performance uses the scales (four items) developed by Welbourne et al. (1998) . All items were measured with a five-point Likert scale (1, totally disagree; 5, totally agree).
Evaluation of measurement model
Data analysis was divided into two stages: the reliability and validity of the measurement model were evaluated in the first stage, and the structural model was examined in the second stage to conduct the examination of the research hypotheses ( Hair et al., 2009 ). In this study, the latent variable structural equation models (SMEs) of SmartPLS3.0 and SPSS 25 were adopted as the analysis method. Currently, academics generally agree with the approach of Anderson and Gerbing (1984) . That is, CFA should report Standardized Factor Loading, Multivariate Correlation Squared, Composite Reliability, and Average Variance Extracted for all variables, and only after these metrics pass the test can structural models be evaluated. Currently, academics generally agree with the approach of Anderson and Gerbing (1984) . That is, CFA should report Standardized Factor Loading, Multivariate Correlation Squared, Composite Reliability, and Average Variance Extracted for all variables, and only after these metrics pass the test can structural models be evaluated. Table 1 shows the average number, factor loading, reliability, and average variance extracted (AVE) value of each construct in this study. The composite reliability (CR) of each construct in this study ranged from 0.870 to 0.954, and every Cronbach’s alpha (α) was higher than 0.7, indicating a high reliability for the constructs in this study. AVE ranged from 0.791 to 0.897, which was also greater than 0.500, indicating a good convergent validity for the constructs in this study. Table 2 shows the correlation coefficient matrix for each construct in this study. The square root of the AVE for each construct was greater than the correlation coefficient for the dimensions ( Chin, 1998 ), indicating a good discriminant validity for the constructs in this study. Besides, Henseler et al. (2016) have proposed that the heterotrait-monotrait ratio (HTMT) of correlations based on the multitrait-multimethod matrix could be adopted as a method to determine discriminant validity. Table 3 shows that the HTMT values for the constructs are all lower than 0.9, showing a good discriminant validity for the constructs in this study. The above analysis showed a good construct validity for this study.
Summary of study measures and factor loadings of CFA and SEM.
SD, standard deviation; CR, composite reliability; AVE, average variance explained.
Matrix of construct correlation coefficients.
The diagonal line is the square root of the average variance extracted (AVE) of each dimension.
Heterotrait-monotrait (HTMT).
Data analysis and results
Smart PLS 3.0 was adopted for the structural model analysis in this study. The value of the standardized root mean square residual (SRMR) can be applied to evaluate the fit of the research model, which is between 0 and 1: the closer it is to 0, the better the fit is. However, the saturated model of the SRMR assumes that the number of paths in the structural model is the same as the number of related constructs in the measurement model, and the estimated model is calculated in terms of the sample dataset itself and the rows. When the SRMR of the saturated model and the estimated model is less than 0.08, it indicates a good fit for the model Hu and Bentler (1999) . The value of the normed fit index (NFI) is between 0 and 1, where the closer it is to 1, the better the fit is, and a value of NFI greater than 0.8 means an acceptable fit.
According to the results calculated by Smart PLS, the value of the SRMR for the saturated model in this study is 0.056, and the value of the SRMR for the estimated model is 0.064, both of which are less than 0.080. The value of NFI is 0.842, which meets the requirements for fit. There is thus a good model fit for this study.
After the evaluation and measurement results were found to be satisfactory, we evaluated the structural model, and examined the hypothesis through the percentage of variance and the significance of structural path. Figure 2 shows the test results of the PLS analysis containing control variables. Boredom Proneness was positively correlated with information overload ( β = 0.456, p < 0.001), communication overload ( β = 0.525, p < 0.001) and system feature overload ( β = 0.392, p < 0.001), thus supporting H1, H2, and H3. Information overload ( β = 0.253, p < 0.01) and communication overload ( β = 0.324, p < 0.001) had a significant positive correlation with the sense of Social Media Overload, thus supporting H4 and 5. Social media overload ( β = −0.239, p < 0.001) was positively correlated with independent academic performance, thus supporting H7. But system feature overload ( β = 0.018, p = 0.124) is not significant for social media overload, so H6 is not supported.
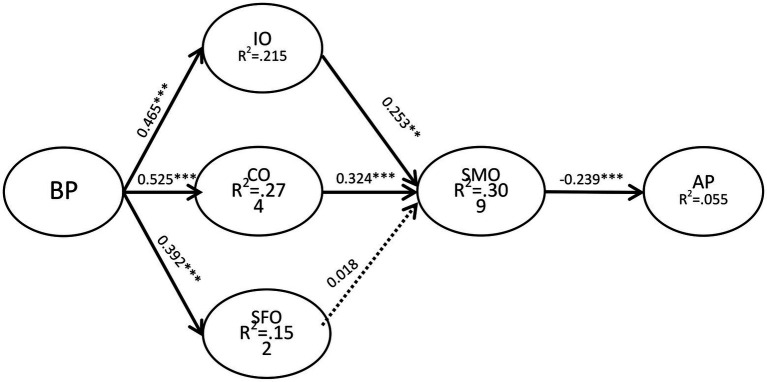
SEM analysis of the research model.
This study reveals that boredom proneness is correlated with social media overload can lead to reduced academic performance among college students. Our analysis of data collected from 441 college students confirms that boredom proneness is positively correlated with information, communication and system feature overloads as well as social media overload and it weaken students’ academic performance.
College students tend to be negatively susceptible to social media because of their flexible timetable, lots of free time and lack of self-control, especially when bored during the quarantine and lockdown period. Recent studies have observed the harmful effects of social media overload ( Lutz et al., 2014 ; Bright et al., 2015 ; Maier et al., 2015 ; Dhir et al., 2018 ), but none of them have given a theoretical explanation on how and why the social media overload leads to reduced performance. This study aims to discuss the impact of social media overload on college students’ academic performance through combining TCIU with the SSO model.
Theoretical significance
As an expansion of prior studies on the SSO model, this study finds that the boredom proneness which appeared among college students during COVID-19 interacted with its resulting social media overload, which further has caused a decline in students’ academic performance. This study makes multiple theoretical contributions. First of all, we combine TCIU with the SSO model to theoretically understand antecedents and outcomes of social media overload. Our study confirms that the use of the SSO model allows us to better understand the validity of digital technologies and education phenomena. Although prior literature has investigated social media overload through the SSO model and stress–strain–outcome model ( Luqman et al., 2017 ; Loh et al., 2021 ), we maintain that the SSO model can better illustrate this complex human behavior.
Second, our research findings show that boredom proneness is positively correlated with overload. In terms of practical implications, the findings from this study can be used as justification for developing targeted interventions that enhance cognitive control abilities so as to avoid social media overload and ultimately impaired performance. Current studies of antecedents of social media overload either place emphasis on demographics and usage characteristics ( Laumer et al., 2014 ) or underline system and information characteristics ( Cho et al., 2011 ; Lee et al., 2016 ). On the contrary, this study verifies the effectiveness of implicit stimuli in explaining social media overload, which means that boredom proneness may finally cause the social media overload that adversely affects college students’ academic performance. Our results show that information overload and communication overload significantly herald social media overload. In essence, social media overload is a transient phenomenon, and it takes a period of time for users to reach the overload state. The inability to control social media use and override impulsive judgments will finally lead to overload. Thus, we conclude that the problematic use of technologies will result in an imbalance between cognitive processes ( Soror et al., 2015 ; Turel et al., 2016 ). This study also finds that social media overload has a negative impact on academic performance. Although most of the prior literature uses social media overload as a mechanism to explain problematic social media use ( LaRose et al., 2003 , 2014 ), our study proves that social media overload will eventually lead to a decline in students’ academic performance, which is an extension of current knowledge.
However, system feature overload has no effect on social media overload, which is contrary to our expectations. Some scholars have indicated that individuals are more willing to use diversified system features to form a relatively special work style and are more tolerant of the corresponding increase in cognitive load and interference caused by system features. In addition, some prior studies also reveal that individuals may be accustomed to continuous and high system function overload after forming an intimate relationship with their mobile devices in life ( Yoo, 2010 ; Bødker et al., 2014 ). As argued in previous studies, system feature overload will reduce as the age lowers ( Zhang et al., 2016 ). A possible explanation is that college students are more interested in social network services, which makes them not susceptible to system feature overload.
Third, by discussing the dark side of social media use among college students, this study has enriched the research on cyber psychology and is beneficial for the development of research on technology stress. In the context of the COVID-19 quarantine, studies on social media overload among college students are urgently needed and are important to offer new insights. College students are a major user group of social media, and the focus of relevant studies is placed on how this group actively engages in social media but seldom on how they wrongly use social media ( Priporas et al., 2017 ; Throuvala et al., 2019 ). Our findings dispel the stereotype that college students can completely take control of social media use on their own, and it foregrounds this generation’s vulnerability in terms of social media use, especially during the pandemic ( Turner, 2015 ). As stated by TCIU, social media have been major recreational tools for college students during the COVID-19 quarantine period. This study attests that overload will result in social media overload from the perspective of users and underlines the potential defects of using social media in college students’ learning processes.
Practical significance
To achieve our goal, we followed the stress-based behavioral theory proposed in the SSO model ( Davis and Luthans, 1980 ). In this process, this study facilitates research on general explanations for the relationship between social media and college students’ academic performance and gives more detailed and specific explanations for the causality. As expected, boredom proneness results in the overload.
This study has the following practical significances. First of all, parents, educators and the public should raise their awareness of the dark side of social media, especially boredom proneness. Parents and educators need to encourage young social media users (e.g., college students and teenagers) to bravely confront their boredom proneness, motivate them to avoid or get rid of negative boredom and support the positive use of social media as motivation.
Second, our study also offers valuable insights for developers of social media systems. It is well-known that social media developers build in features to distract users from alternate tasks in order to increase fixation with their own service. We found that these overloads lead to social media overload among students, and ultimately negatively affects their academic performance. Other studies report overloaded users are more likely stop using the service altogether. Thus, it makes business sense for social media providers to incorporate features in their service which assist the user in avoiding overload.
Third, although this study does not support the influence of system feature overload on social media overload, we still recommend that social media providers facilitate a smooth transition by announcing the changed schedule and scope in advance to reduce discomfort. Besides, social media providers can also provide user guides to introduce new features to users. It is even suggested that SNS providers allow users to design their own SNS accounts instead of developing complex accounts and then integrating embedded features into these accounts.
Research limitations and research prospects
There are some limitations to this study. First of all, data were collected at four universities in a particular country, i.e., China. The research results may be different if other age groups, cultural backgrounds and pandemic quarantine stages are considered. To eliminate this limitation, we suggest that other scholars include a similar research model, other age groups with different cultural backgrounds and all the stages of the pandemic in their studies. Second, this is a cross-sectional study that collects data from online groups, so there is the issue of method bias (e.g., selection and response bias), and possible changes to given relationships within a period of time cannot be reflected. To address this limitation and considering the continuity of the pandemic, we suggest using a longitudinal method and/or qualitative method to further explore psychological health and the effects of social media use. Third, overload is considered to be a harmful psychological state in this study, and social media overload is considered as the behavioral outcome of the information overload on social media. However, previous research on the dark side of social media use reveals that psychological illnesses also include other aspects, such as anxiety, depression and tiredness ( Dhir et al., 2018 ; Evers et al., 2020 ), as well as sleep problems ( Dhir et al., 2019 ; Malik et al., 2020 ; Tandon et al., 2020 ). Consequently, we advocate for other scholars to expand the findings of this study by taking other relevant issues into account. Likewise, scholars can also explore how to improve individuals’ psychological well-being and mental health by making use of social media during the pandemic. Finally, gender has been an important influencing factor for studies of college students behaviors, because male students may have a different grasp of social media overload from female students. As a result, this study suggests to consider comparisons between males and females to offer richer and more valuable significance to the development of theoretical models.
Data availability statement
The raw data supporting the conclusions of this article will be made available by the authors, without undue reservation.
Ethics statement
The studies involving human participants were reviewed and approved by Academic Committee of Fujian University of Technology. The patients/participants provided their written informed consent to participate in this study.
Author contributions
All authors listed have made a substantial, direct, and intellectual contribution to the work and approved it for publication.
Fujian Social Science Planning youth project. Research on the development mechanism of agricultural e-commerce industry in Fujian Province from the perspective of big data (FJ2020C062).
Conflict of interest
The authors declare that the research was conducted in the absence of any commercial or financial relationships that could be construed as a potential conflict of interest.
Publisher’s note
All claims expressed in this article are solely those of the authors and do not necessarily represent those of their affiliated organizations, or those of the publisher, the editors and the reviewers. Any product that may be evaluated in this article, or claim that may be made by its manufacturer, is not guaranteed or endorsed by the publisher.
- Ahuja M. K., Chudoba K. M., Kacmar C. J., McKnight D. H., George J. F. (2007). IT road warriors: balancing work–family conflict, job autonomy, and work overload to mitigate turnover intentions. MIS Q. 31, 1–17. doi: 10.2307/25148778 [ DOI ] [ Google Scholar ]
- Aladwani A. M., Almarzouq M. (2016). Understanding compulsive social media use: the premise of complementing self-conceptions mismatch with technology. Comput. Hum. Behav. 60, 575–581. doi: 10.1016/j.chb.2016.02.098 [ DOI ] [ Google Scholar ]
- Al-Rahmi W. M., Alias N., Othman M. S., Marin V. I., Tur G. (2018). A model of factors affecting learning performance through the use of social media in Malaysian higher education. Comput. Educ. 121, 59–72. doi: 10.1016/j.compedu.2018.02.010 [ DOI ] [ Google Scholar ]
- Alter A. (2017). Irresistible: The Rise of Addictive Technology and the Business of Keeping Us Hooked. Penguin, New York, NY. [ Google Scholar ]
- Anderson J. C., Gerbing D. W. (1984). The effect of sampling error on convergence, improper solutions, and goodness-of-fit indices for maximum likelihood confirmatory factor analysis. Psychometrika 49, 155–173. doi: 10.1007/BF02294170 [ DOI ] [ Google Scholar ]
- Ayyagari R., Grover V., Purvis R. (2011). Technostress: technological antecedents and implications. MIS Q. 35, 831–858. doi: 10.2307/41409963 [ DOI ] [ Google Scholar ]
- Becker M. W., Alzahabi R., Hopwood C. J. (2013). Media multitasking is associated with symptoms of depression and social anxiety. Cyberpsychol. Behav. Soc. Netw. 16, 132–135. doi: 10.1089/cyber.2012.0291, PMID: [ DOI ] [ PubMed ] [ Google Scholar ]
- Bench S. W., Lench H. C. (2013). On the function of boredom. Behav. Sci. 3, 459–472. doi: 10.3390/bs3030459, PMID: [ DOI ] [ PMC free article ] [ PubMed ] [ Google Scholar ]
- Bødker M., Gimpel G., Hedman J. (2014). Time-out/time-in: the dynamics of everyday experiential computing devices. Inf. Syst. J. 24, 143–166. doi: 10.1111/isj.12002 [ DOI ] [ Google Scholar ]
- Boksem M. A., Tops M. (2008). Mental fatigue: costs and benefits. Brain Res. Rev. 59, 125–139. doi: 10.1016/j.brainresrev.2008.07.001 [ DOI ] [ PubMed ] [ Google Scholar ]
- Bowker G. (2008). Structures of Participation in Digital Culture. New York, NY: Social Science Research Council. [ Google Scholar ]
- Bright L. F., Kleiser S. B., Grau S. L. (2015). Too much Facebook? An exploratory examination of social media fatigue. Comput. Hum. Behav. 44, 148–155. doi: 10.1016/j.chb.2014.11.048 [ DOI ] [ Google Scholar ]
- Brooks S., Califf C. (2017). Social media-induced technostress: its impact on the job performance of it professionals and the moderating role of job characteristics. Comput. Netw. 114, 143–153. doi: 10.1016/j.comnet.2016.08.020 [ DOI ] [ Google Scholar ]
- Bucher E., Fieseler C., Suphan A. (2013). The stress potential of social media in the workplace. Inf. Commun. Soc. 16, 1639–1667. doi: 10.1080/1369118X.2012.710245 [ DOI ] [ Google Scholar ]
- Cao X., Masood A., Luqman A., Ali A. (2018). Excessive use of mobile social networking sites and poor academic performance: antecedents and consequences from stressor-strain-outcome perspective. Comput. Hum. Behav. 85, 163–174. doi: 10.1016/j.chb.2018.03.023 [ DOI ] [ Google Scholar ]
- Cao X., Sun J. (2018). Exploring the effect of overload on the discontinuous intention of social media users: An SOR perspective. Comput. Hum. Behav. 81, 10–18. doi: 10.1016/j.chb.2017.11.035 [ DOI ] [ Google Scholar ]
- Caplan S. E., High A. C. (2006). Beyond excessive use: the interaction between cognitive and behavioral symptoms of problematic Internet use. Commun. Res. Rep. 23, 265–271. doi: 10.1080/08824090600962516 [ DOI ] [ Google Scholar ]
- Cheung F., Tang C. (2010). The influence of emotional dissonance on subjective health and job satisfaction: testing the stress–strain–outcome model. J. Appl. Soc. Psychol. 40, 3192–3217. doi: 10.1111/j.1559-1816.2010.00697.x [ DOI ] [ Google Scholar ]
- Chin W. W. (1998). Commentary: issues and opinion on structural equation modeling. MIS Q. 22, 7–16. [ Google Scholar ]
- Cho J., Ramgolam D. I., Schaefer K. M., Sandlin A. N. (2011). The rate and delay in overload: an investigation of communication overload and channel synchronicity on identification and job satisfaction. J. Appl. Commun. Res. 39, 38–54. doi: 10.1080/00909882.2010.536847 [ DOI ] [ Google Scholar ]
- Choi C. H., Kim T. T., Lee G., Lee S. K. (2014). Testing the stressor–strain–outcome model of customer-related social stressors in predicting emotional exhaustion, customer orientation and service recovery performance. Int. J. Hosp. Manag. 36, 272–285. doi: 10.1016/j.ijhm.2012.09.009 [ DOI ] [ Google Scholar ]
- Datu J. A. D., Yang W., Valdez J. P. M., Chu S. K. W. (2018). Is Facebook involvement associated with academic engagement among Filipino university students? A cross-sectional study. Comput. Educ. 125, 246–253. doi: 10.1016/j.compedu.2018.06.010 [ DOI ] [ Google Scholar ]
- Davis T. R., Luthans F. (1980). A social learning approach to organizational behavior. Acad. Manag. Rev. 5, 281–290. doi: 10.2307/257438 [ DOI ] [ Google Scholar ]
- Deutsch K. W. (1961). On social communication and the metropolis. Daedalus 90, 99–110. [ Google Scholar ]
- Dhir A., Kaur P., Chen S., Pallesen S. (2019). Antecedents and consequences of social media fatigue. Int. J. Inf. Manag. 48, 193–202. doi: 10.1016/j.ijinfomgt.2019.05.021 [ DOI ] [ Google Scholar ]
- Dhir A., Kaur P., Rajala R. (2018). Why do young people tag photos on social networking sites? Explaining user intentions. Int. J. Inf. Manag. 38, 117–127. doi: 10.1016/j.ijinfomgt.2017.07.004 [ DOI ] [ Google Scholar ]
- Edwards J. R., Cooper C. L. (1990). The person–environment fit approach to stress: recurring problems and some suggested solutions. J. Organ. Behav. 11, 293–307. doi: 10.1002/job.4030110405 [ DOI ] [ Google Scholar ]
- Elhai J. D., Hall B. J., Levine J. C., Dvorak R. D. (2017). Types of smartphone usage and relations with problematic smartphone behaviors: the role of content consumption vs. social smartphone use. Cyberpsychology 11. doi: 10.5817/CP2017-2-3 [ DOI ] [ Google Scholar ]
- Elhai J. D., Yang H., Fang J., Bai X., Hall B. J. (2020). Depression and anxiety symptoms are related to problematic smartphone use severity in Chinese young adults: fear of missing out as a mediator. Addict. Behav. 101:105962. doi: 10.1016/j.addbeh.2019.04.020, PMID: [ DOI ] [ PubMed ] [ Google Scholar ]
- Evans G. W., Rhee E., Forbes C., Allen K. M., Lepore S. J. (2000). The meaning and efficacy of social withdrawal as a strategy for coping with chronic residential crowding. J. Environ. Psychol. 20, 335–342. doi: 10.1006/jevp.1999.0174 [ DOI ] [ Google Scholar ]
- Evers K., Chen S., Rothmann S., Dhir A., Pallesen S. (2020). Investigating the relation among disturbed sleep due to social media use, school burnout, and academic performance. J. Adolesc. 84, 156–164. doi: 10.1016/j.adolescence.2020.08.011, PMID: [ DOI ] [ PubMed ] [ Google Scholar ]
- Fisher C. D. (1998). Effects of external and internal interruptions on boredom at work: two studies. J. Organ. Behav. 19, 503–522. doi: [ DOI ] [ Google Scholar ]
- Fu S., Li H., Liu Y., Pirkkalainen H., Salo M. (2020). Social media overload, exhaustion, and use discontinuance: examining the effects of information overload, system feature overload, and social overload. Inf. Process. Manag. 57:102307. doi: 10.1016/j.ipm.2020.102307 [ DOI ] [ Google Scholar ]
- Gao W., Liu Z., Guo Q., Li X. (2018). The dark side of ubiquitous connectivity in smartphone-based SNS: an integrated model from information perspective. Comput. Hum. Behav. 84, 185–193. doi: 10.1016/j.chb.2018.02.023 [ DOI ] [ Google Scholar ]
- Garrett R. K., Danziger J. N. (2007). IM = Interruption management? Instant messaging and disruption in the workplace. J. Comput.-Mediat. Commun. 13, 23–42. doi: 10.1111/j.1083-6101.2007.00384.x [ DOI ] [ Google Scholar ]
- Giunchiglia F., Zeni M., Gobbi E., Bignotti E., Bison I. (2018). Mobile social media usage and academic performance. Comput. Hum. Behav. 82, 177–185. [ Google Scholar ]
- González-Izal M., Malanda A., Gorostiaga E., Izquierdo M. (2012). Electromyographic models to assess muscle fatigue. J. Electromyogr. Kinesiol. 22, 501–512. doi: 10.1016/j.jelekin.2012.02.019, PMID: [ DOI ] [ PubMed ] [ Google Scholar ]
- Hair J. F., Black W. C., Babin B. J., Anderson R. E., Tatham R. L. (2009). Análise Multivariada de Dados. Porto Alegre: Bookman Editora. [ Google Scholar ]
- Harman H. H. (1976). Modern factor analysis: University of Chicago press. [ Google Scholar ]
- Henseler J., Hubona G., Ray P. A. (2016). Using PLS path modeling in new technology research: updated guidelines. Industr. Manag. Data Syst. 116, 2–20., PMID: 20380915 [ Google Scholar ]
- Hu L. T., Bentler P. M. (1999). Cutoff criteria for fit indexes in covariance structure analysis: conventional criteria versus new alternatives. Struct. Equat. Model. 6, 1–55. [ Google Scholar ]
- Islam A. N., Laato S., Talukder S., Sutinen E. (2020). Misinformation sharing and social media fatigue during COVID-19: an affordance and cognitive load perspective. Technol. Forecast. Soc. Chang. 159:120201. doi: 10.1016/j.techfore.2020.120201, PMID: [ DOI ] [ PMC free article ] [ PubMed ] [ Google Scholar ]
- Junco R. (2015). Student class standing, Facebook use, and academic performance. J. Appl. Dev. Psychol. 36, 18–29. doi: 10.1016/j.appdev.2014.11.001 [ DOI ] [ Google Scholar ]
- Junco R., Cotten S. R. (2012). No A 4 U: the relationship between multitasking and academic performance. Comput. Educ. 59, 505–514. doi: 10.1016/j.compedu.2011.12.023 [ DOI ] [ Google Scholar ]
- Karr-Wisniewski P., Lu Y. (2010). When more is too much: operationalizing technology overload and exploring its impact on knowledge worker productivity. Comput. Hum. Behav. 26, 1061–1072. doi: 10.1016/j.chb.2010.03.008 [ DOI ] [ Google Scholar ]
- Kass S. J., Beede K. E., Vodanovich S. J. (2010). Self-report measures of distractibility as correlates of simulated driving performance. Accid. Anal. Prev. 42, 874–880. doi: 10.1016/j.aap.2009.04.012, PMID: [ DOI ] [ PubMed ] [ Google Scholar ]
- Khanra S., Dhir A., Kaur P., Joseph R. P. (2021). Factors influencing the adoption postponement of mobile payment services in the hospitality sector during a pandemic. J. Hosp. Tour. Manag. 46, 26–39. doi: 10.1016/j.jhtm.2020.11.004 [ DOI ] [ Google Scholar ]
- Kim T. T., Paek S., Choi C. H., Lee G. (2012). Frontline service employees’ customer-related social stressors, emotional exhaustion, and service recovery performance: customer orientation as a moderator. Serv. Bus. 6, 503–526. doi: 10.1007/s11628-012-0164-8 [ DOI ] [ Google Scholar ]
- Kirschner P. A., Karpinski A. C. (2010). Facebook® and academic performance. Comput. Hum. Behav. 26, 1237–1245. doi: 10.1016/j.chb.2010.03.024 [ DOI ] [ Google Scholar ]
- Klapp O. E. (1986). Overload and Boredom: Essays on the Quality of Life in the Information Society: Greenwood Publishing Group Inc. Westport, CT. [ Google Scholar ]
- Koeske G. F., Koeske R. D. (1993). A preliminary test of a stress–strain–outcome model for reconceptualizing the burnout phenomenon. J. Soc. Serv. Res. 17, 107–135. doi: 10.1300/J079v17n03_06 [ DOI ] [ Google Scholar ]
- Ku Y.-C., Chu T.-H., Tseng C.-H. (2013). Gratifications for using CMC technologies: a comparison among SNS, IM, and e-mail. Comput. Hum. Behav. 29, 226–234. doi: 10.1016/j.chb.2012.08.009 [ DOI ] [ Google Scholar ]
- LaRose R., Connolly R., Lee H., Li K., Hales K. D. (2014). Connection overload? A cross cultural study of the consequences of social media connection. Inf. Syst. Manag. 31, 59–73. doi: 10.1080/10580530.2014.854097 [ DOI ] [ Google Scholar ]
- LaRose R., Lin C. A., Eastin M. S. (2003). Unregulated internet usage: addiction, habit, or deficient self-regulation? Media Psychol. 5, 225–253. doi: 10.1207/S1532785XMEP0503_01 [ DOI ] [ Google Scholar ]
- Laumer S., Maier C., Eckhardt A., Weitzel T. (2014). Why are they grumbling about my new system? Theoretical foundation and empirical evidence of employee grumbling as a user resistance behavior. Paper Presented at the International Conference on Information Systems 2014, Auckland.
- le Roux D. B., Parry D. A. (2017). In-lecture media use and academic performance: does subject area matter? Comput. Hum. Behav. 77, 86–94. doi: 10.1016/j.chb.2017.08.030 [ DOI ] [ Google Scholar ]
- Lee A. R., Son S.-M., Kim K. K. (2016). Information and communication technology overload and social networking service fatigue: A stress perspective. Comput. Hum. Behav. 55, 51–61. doi: 10.1016/j.chb.2015.08.011 [ DOI ] [ Google Scholar ]
- Li Y. (2018). Upward social comparison and depression in social network settings: the roles of envy and self-efficacy. Internet Res. 29, 46–59. doi: 10.1108/IntR-09-2017-0358 [ DOI ] [ Google Scholar ]
- Liu D., Kirschner P. A., Karpinski A. C. (2017). A meta-analysis of the relationship of academic performance and social network site use among adolescents and young adults. Comput. Hum. Behav. 77, 148–157. doi: 10.1016/j.chb.2017.08.039 [ DOI ] [ Google Scholar ]
- Liu H., Liu W., Yoganathan V., Osburg V.-S. (2021). COVID-19 information overload and generation Z's social media discontinuance intention during the pandemic lockdown. Technol. Forecast. Soc. Chang. 166:120600. doi: 10.1016/j.techfore.2021.120600, PMID: [ DOI ] [ PMC free article ] [ PubMed ] [ Google Scholar ]
- Loh X.-K., Lee V.-H., Loh X.-M., Tan G. W.-H., Ooi K.-B., Dwivedi Y. K. (2021). The dark side of mobile learning via social media: how bad can it get? Inf. Syst. Front. 1–18. doi: 10.1007/s10796-021-10202-z [ DOI ] [ PMC free article ] [ PubMed ] [ Google Scholar ]
- Luqman A., Cao X., Ali A., Masood A., Yu L. (2017). Empirical investigation of Facebook discontinues usage intentions based on SOR paradigm. Comput. Hum. Behav. 70, 544–555. doi: 10.1016/j.chb.2017.01.020 [ DOI ] [ Google Scholar ]
- Lutz C., Ranzini G., Meckel M. (2014). Stress 2.0: social media overload among Swiss teenagers. Stud. Media. Commun. 12, 1–22. doi: 10.1108/S2050-206020140000008001 [ DOI ] [ Google Scholar ]
- Mahmud I., Ramayah T., Kurnia S. (2017). To use or not to use: modelling end user grumbling as user resistance in pre-implementation stage of enterprise resource planning system. Inf. Syst. 69, 164–179. doi: 10.1016/j.is.2017.05.005 [ DOI ] [ Google Scholar ]
- Maier C., Laumer S., Eckhardt A., Weitzel T. (2012). “When social networking turns to social overload: explaining the stress, emotional exhaustion, and quitting behavior from social network sites’ users.” European Conference on Information Systems Proceedings, p. 71.
- Maier C., Laumer S., Weinert C., Weitzel T. (2015). The effects of technostress and switching stress on discontinued use of social networking services: a study of Facebook use. Inf. Syst. J. 25, 275–308. doi: 10.1111/isj.12068 [ DOI ] [ Google Scholar ]
- Malik A., Dhir A., Kaur P., Johri A. (2020). Correlates of social media fatigue and academic performance decrement: a large cross-sectional study. Inf. Technol. People 34, 557–580. doi: 10.1108/ITP-06-2019-0289 [ DOI ] [ Google Scholar ]
- McFarlane D. C., Latorella K. A. (2002). The scope and importance of human interruption in human–computer interaction design. HCI Intern. 17, 1–61. doi: 10.1207/S15327051HCI1701_1 [ DOI ] [ Google Scholar ]
- Mikulas W. L., Vodanovich S. J. (1993). The essence of boredom. Psychol. Rec. 43:3. [ Google Scholar ]
- Moore J. E. (2000). One road to turnover: An examination of work exhaustion in technology professionals. MIS Q. 24, 141–168. doi: 10.2307/3250982 [ DOI ] [ Google Scholar ]
- Nail L. M., Winningham M. L. (1995). Fatigue and weakness in cancer patients: the symptom experience. Paper presented at the Seminars in Oncology Nursing. [ DOI ] [ PubMed ]
- Nawaz M. A., Shah Z., Nawaz A., Asmi F., Hassan Z., Raza J. (2018). Overload and exhaustion: classifying SNS discontinuance intentions. Cogent Psychol. 5:1515584. doi: 10.1080/23311908.2018.1515584 [ DOI ] [ Google Scholar ]
- Ou C. X., Davison R. M. (2011). Interactive or interruptive? Instant messaging at work. Decis. Support. Syst. 52, 61–72. doi: 10.1016/j.dss.2011.05.004 [ DOI ] [ Google Scholar ]
- Pavlou P. A., Liang H., Xue Y. (2007). Understanding and mitigating uncertainty in online exchange relationships: a principal-agent perspective. MIS Q. 31, 105–136. doi: 10.2307/25148783 [ DOI ] [ Google Scholar ]
- Podsakoff P. M., Organ D. W. (1986). Self-reports in organizational research: problems and prospects. J. Manag. 12, 531–544. doi: 10.1177/014920638601200408 [ DOI ] [ Google Scholar ]
- Priporas C.-V., Stylos N., Fotiadis A. K. (2017). Generation Z consumers' expectations of interactions in smart retailing: a future agenda. Comput. Hum. Behav. 77, 374–381. doi: 10.1016/j.chb.2017.01.058 [ DOI ] [ Google Scholar ]
- Quan-Haase A., Young A. L. (2010). Uses and gratifications of social media: a comparison of Facebook and instant messaging. Bull. Sci. Technol. Soc. 30, 350–361. doi: 10.1177/0270467610380009 [ DOI ] [ Google Scholar ]
- Ragu-Nathan T., Tarafdar M., Ragu-Nathan B. S., Tu Q. (2008). The consequences of technostress for end users in organizations: conceptual development and empirical validation. Inf. Syst. Res. 19, 417–433. doi: 10.1287/isre.1070.0165 [ DOI ] [ Google Scholar ]
- Ravindran T., Yeow Kuan A. C., Hoe Lian D. G. (2014). Antecedents and effects of social network fatigue. J. Assoc. Inf. Sci. Technol. 65, 2306–2320. doi: 10.1002/asi.23122 [ DOI ] [ Google Scholar ]
- Rosen L. D., Carrier L. M., Cheever N. A. (2013a). Facebook and texting made me do it: media-induced task-switching while studying. Comput. Hum. Behav. 29, 948–958. doi: 10.1016/j.chb.2012.12.001 [ DOI ] [ Google Scholar ]
- Rosen L. D., Whaling K., Rab S., Carrier L. M., Cheever N. A. (2013b). Is Facebook creating “iDisorders”? The link between clinical symptoms of psychiatric disorders and technology use, attitudes and anxiety. Comput. Hum. Behav. 29, 1243–1254. doi: 10.1016/j.chb.2012.11.012 [ DOI ] [ Google Scholar ]
- Said H., Ali L., Ali F., Chen X. (2021). COVID-19 and unpaid leave: impacts of psychological contract breach on organizational distrust and turnover intention: mediating role of emotional exhaustion. Tour. Manag. Perspect. 39:100854. doi: 10.1016/j.tmp.2021.100854 [ DOI ] [ PMC free article ] [ PubMed ] [ Google Scholar ]
- Salo J., Mäntymäki M., Islam A. N. (2018). The dark side of social media–and Fifty Shades of Grey introduction to the special issue: the dark side of social media. Internet Res. 28, 1166–1168. doi: 10.1108/IntR-10-2018-442 [ DOI ] [ Google Scholar ]
- Schaufeli W. (1995). The Maslach burnout inventory-general survey: a self-report questionnaire to assess burnout at the workplace. Paper presented at the extending the burnout construct: Reflecting changing career paths, symposium, APA/NIOSH conference, work, stress and health '95. [ Google Scholar ]
- Shen J., Barbera J., Shapiro C. M. (2006). Distinguishing sleepiness and fatigue: focus on definition and measurement. Sleep Med. Rev. 10, 63–76. doi: 10.1016/j.smrv.2005.05.004, PMID: [ DOI ] [ PubMed ] [ Google Scholar ]
- Soror A. A., Hammer B. I., Steelman Z. R., Davis F. D., Limayem M. M. (2015). Good habits gone bad: explaining negative consequences associated with the use of mobile phones from a dual-systems perspective. Inf. Syst. J. 25, 403–427. doi: 10.1111/isj.12065 [ DOI ] [ Google Scholar ]
- Steelman Z. R., Soror A. A. (2017). Why do you keep doing that? The biasing effects of mental states on IT continued usage intentions. Comput. Hum. Behav. 73, 209–223. doi: 10.1016/j.chb.2017.03.027 [ DOI ] [ Google Scholar ]
- Tandon A., Dhir A., Almugren I., AlNemer G. N., Mäntymäki M. (2021). Fear of missing out (FoMO) among social media users: a systematic literature review, synthesis and framework for future research. Internet Res. 31, 782–821. doi: 10.1108/INTR-11-2019-0455 [ DOI ] [ Google Scholar ]
- Tandon A., Kaur P., Dhir A., Mäntymäki M. (2020). Sleepless due to social media? Investigating problematic sleep due to social media and social media sleep hygiene. Comput. Hum. Behav. 113:106487. doi: 10.1016/j.chb.2020.106487 [ DOI ] [ Google Scholar ]
- Tarafdar M., Pullins E. B., Ragu-Nathan T. (2015). Technostress: negative effect on performance and possible mitigations. Inf. Syst. J. 25, 103–132. doi: 10.1111/isj.12042 [ DOI ] [ Google Scholar ]
- Tarafdar M., Tu Q., Ragu-Nathan T. S., Ragu-Nathan B. S. (2011). Crossing to the dark side: examining creators, outcomes, and inhibitors of technostress. Commun. Assoc. Comput. Mach. 54, 113–120. doi: 10.1145/1995376.1995403 [ DOI ] [ Google Scholar ]
- Thompson D. V., Hamilton R. W., Rust R. T. (2005). Feature fatigue: when product capabilities become too much of a good thing. J. Mark. Res. 42, 431–442. doi: 10.1509/jmkr.2005.42.4.431 [ DOI ] [ Google Scholar ]
- Throuvala M. A., Griffiths M. D., Rennoldson M., Kuss D. J. (2019). Motivational processes and dysfunctional mechanisms of social media use among adolescents: A qualitative focus group study. Comput. Hum. Behav. 93, 164–175. doi: 10.1016/j.chb.2018.12.012 [ DOI ] [ Google Scholar ]
- Turel O., He Q., Xue G., Xiao L., Bechara A. (2014). Examination of neural systems sub-serving Facebook “addiction”. Psychol. Rep. 115, 675–695. doi: 10.2466/18.PR0.115c31z8, PMID: [ DOI ] [ PubMed ] [ Google Scholar ]
- Turel O., Matt C., Trenz M., Cheung C. M., D’Arcy J., Qahri-Saremi H., et al. (2019). Panel report: the dark side of the digitization of the individual. Internet Res. 29, 274–288. doi: 10.1108/INTR-04-2019-541 [ DOI ] [ Google Scholar ]
- Turel O., Qahri-Saremi H. (2016). Problematic use of social networking sites: antecedents and consequence from a dual-system theory perspective. J. Manag. Inf. Syst. 33, 1087–1116. doi: 10.1080/07421222.2016.1267529 [ DOI ] [ Google Scholar ]
- Turel O., Romashkin A., Morrison K. M. (2016). Health outcomes of information system use lifestyles among adolescents: videogame addiction, sleep curtailment and cardio-metabolic deficiencies. PLoS One 11:e0154764. doi: 10.1371/journal.pone.0154764, PMID: [ DOI ] [ PMC free article ] [ PubMed ] [ Google Scholar ]
- Turner A. (2015). Generation Z: technology and social interest. J. Individ. Psychol. 71, 103–113. doi: 10.1353/jip.2015.0021 [ DOI ] [ Google Scholar ]
- Van der Linden D., Eling P. (2006). Mental fatigue disturbs local processing more than global processing. Psychol. Res. 70, 395–402. doi: 10.1007/s00426-005-0228-7, PMID: [ DOI ] [ PubMed ] [ Google Scholar ]
- Vodanovich S. J., Wallace J. C., Kass S. J. (2005). A confirmatory approach to the factor structure of the boredom proneness scale: evidence for a two-factor short form. J. Pers. Assess. 85, 295–303. doi: 10.1207/s15327752jpa8503_05, PMID: [ DOI ] [ PubMed ] [ Google Scholar ]
- Wang P., Xie X., Wang X., Wang X., Zhao F., Chu X., et al. (2018). The need to belong and adolescent authentic self-presentation on SNSs: A moderated mediation model involving FoMO and perceived social support. Personal. Individ. Differ. 128, 133–138. doi: 10.1016/j.paid.2018.02.035 [ DOI ] [ Google Scholar ]
- Welbourne T. M., Johnson D. E., Erez A. (1998). The role-based performance scale: validity analysis of a theory-based measure. Acad. Manag. J. 41, 540–555. [ Google Scholar ]
- Whelan E., Teigland R. (2013). Transactive memory systems as a collective filter for mitigating information overload in digitally enabled organizational groups. Inf. Organ. 23, 177–197. [ Google Scholar ]
- Wolniewicz C. A., Tiamiyu M. F., Weeks J. W., Elhai J. D. (2018). Problematic smartphone use and relations with negative affect, fear of missing out, and fear of negative and positive evaluation. Psychiatry Res. 262, 618–623. doi: 10.1016/j.psychres.2017.09.058, PMID: [ DOI ] [ PubMed ] [ Google Scholar ]
- Wurman R. S. (1990). Information Anxiety: What to Do When Information Doesn’t Tell you What You Need to Know. New York, NY: Bantam. [ Google Scholar ]
- Yoo Y. (2010). Computing in everyday life: a call for research on experiential computing. MIS Q. 34, 213–231. doi: 10.2307/20721425 [ DOI ] [ Google Scholar ]
- Yu L., Cao X., Liu Z., Wang J. (2018). Excessive social media use at work: exploring the effects of social media overload on job performance. Inf. Technol. People 31, 1091–1112. doi: 10.1108/ITP-10-2016-0237 [ DOI ] [ Google Scholar ]
- Zhang S., Zhao L., Lu Y., Yang J. (2016). Do you get tired of socializing? An empirical explanation of discontinuous usage behaviour in social network services. Inf. Manag. 53, 904–914. doi: 10.1016/j.im.2016.03.006 [ DOI ] [ Google Scholar ]
Associated Data
This section collects any data citations, data availability statements, or supplementary materials included in this article.
Data Availability Statement
- View on publisher site
- PDF (685.3 KB)
- Collections
Similar articles
Cited by other articles, links to ncbi databases.
- Download .nbib .nbib
- Format: AMA APA MLA NLM
Add to Collections
CONCEPTUAL ANALYSIS article
The effect of social media on the development of students’ affective variables.
- 1 Science and Technology Department, Nanjing University of Posts and Telecommunications, Nanjing, China
- 2 School of Marxism, Hohai University, Nanjing, Jiangsu, China
- 3 Government Enterprise Customer Center, China Mobile Group Jiangsu Co., Ltd., Nanjing, China
The use of social media is incomparably on the rise among students, influenced by the globalized forms of communication and the post-pandemic rush to use multiple social media platforms for education in different fields of study. Though social media has created tremendous chances for sharing ideas and emotions, the kind of social support it provides might fail to meet students’ emotional needs, or the alleged positive effects might be short-lasting. In recent years, several studies have been conducted to explore the potential effects of social media on students’ affective traits, such as stress, anxiety, depression, and so on. The present paper reviews the findings of the exemplary published works of research to shed light on the positive and negative potential effects of the massive use of social media on students’ emotional well-being. This review can be insightful for teachers who tend to take the potential psychological effects of social media for granted. They may want to know more about the actual effects of the over-reliance on and the excessive (and actually obsessive) use of social media on students’ developing certain images of self and certain emotions which are not necessarily positive. There will be implications for pre- and in-service teacher training and professional development programs and all those involved in student affairs.
Introduction
Social media has turned into an essential element of individuals’ lives including students in today’s world of communication. Its use is growing significantly more than ever before especially in the post-pandemic era, marked by a great revolution happening to the educational systems. Recent investigations of using social media show that approximately 3 billion individuals worldwide are now communicating via social media ( Iwamoto and Chun, 2020 ). This growing population of social media users is spending more and more time on social network groupings, as facts and figures show that individuals spend 2 h a day, on average, on a variety of social media applications, exchanging pictures and messages, updating status, tweeting, favoring, and commenting on many updated socially shared information ( Abbott, 2017 ).
Researchers have begun to investigate the psychological effects of using social media on students’ lives. Chukwuere and Chukwuere (2017) maintained that social media platforms can be considered the most important source of changing individuals’ mood, because when someone is passively using a social media platform seemingly with no special purpose, s/he can finally feel that his/her mood has changed as a function of the nature of content overviewed. Therefore, positive and negative moods can easily be transferred among the population using social media networks ( Chukwuere and Chukwuere, 2017 ). This may become increasingly important as students are seen to be using social media platforms more than before and social networking is becoming an integral aspect of their lives. As described by Iwamoto and Chun (2020) , when students are affected by social media posts, especially due to the increasing reliance on social media use in life, they may be encouraged to begin comparing themselves to others or develop great unrealistic expectations of themselves or others, which can have several affective consequences.
Considering the increasing influence of social media on education, the present paper aims to focus on the affective variables such as depression, stress, and anxiety, and how social media can possibly increase or decrease these emotions in student life. The exemplary works of research on this topic in recent years will be reviewed here, hoping to shed light on the positive and negative effects of these ever-growing influential platforms on the psychology of students.
Significance of the study
Though social media, as the name suggests, is expected to keep people connected, probably this social connection is only superficial, and not adequately deep and meaningful to help individuals feel emotionally attached to others. The psychological effects of social media on student life need to be studied in more depth to see whether social media really acts as a social support for students and whether students can use social media to cope with negative emotions and develop positive feelings or not. In other words, knowledge of the potential effects of the growing use of social media on students’ emotional well-being can bridge the gap between the alleged promises of social media and what it actually has to offer to students in terms of self-concept, self-respect, social role, and coping strategies (for stress, anxiety, etc.).
Exemplary general literature on psychological effects of social media
Before getting down to the effects of social media on students’ emotional well-being, some exemplary works of research in recent years on the topic among general populations are reviewed. For one, Aalbers et al. (2018) reported that individuals who spent more time passively working with social media suffered from more intense levels of hopelessness, loneliness, depression, and perceived inferiority. For another, Tang et al. (2013) observed that the procedures of sharing information, commenting, showing likes and dislikes, posting messages, and doing other common activities on social media are correlated with higher stress. Similarly, Ley et al. (2014) described that people who spend 2 h, on average, on social media applications will face many tragic news, posts, and stories which can raise the total intensity of their stress. This stress-provoking effect of social media has been also pinpointed by Weng and Menczer (2015) , who contended that social media becomes a main source of stress because people often share all kinds of posts, comments, and stories ranging from politics and economics, to personal and social affairs. According to Iwamoto and Chun (2020) , anxiety and depression are the negative emotions that an individual may develop when some source of stress is present. In other words, when social media sources become stress-inducing, there are high chances that anxiety and depression also develop.
Charoensukmongkol (2018) reckoned that the mental health and well-being of the global population can be at a great risk through the uncontrolled massive use of social media. These researchers also showed that social media sources can exert negative affective impacts on teenagers, as they can induce more envy and social comparison. According to Fleck and Johnson-Migalski (2015) , though social media, at first, plays the role of a stress-coping strategy, when individuals continue to see stressful conditions (probably experienced and shared by others in media), they begin to develop stress through the passage of time. Chukwuere and Chukwuere (2017) maintained that social media platforms continue to be the major source of changing mood among general populations. For example, someone might be passively using a social media sphere, and s/he may finally find him/herself with a changed mood depending on the nature of the content faced. Then, this good or bad mood is easily shared with others in a flash through the social media. Finally, as Alahmar (2016) described, social media exposes people especially the young generation to new exciting activities and events that may attract them and keep them engaged in different media contexts for hours just passing their time. It usually leads to reduced productivity, reduced academic achievement, and addiction to constant media use ( Alahmar, 2016 ).
The number of studies on the potential psychological effects of social media on people in general is higher than those selectively addressed here. For further insights into this issue, some other suggested works of research include Chang (2012) , Sriwilai and Charoensukmongkol (2016) , and Zareen et al. (2016) . Now, we move to the studies that more specifically explored the effects of social media on students’ affective states.
Review of the affective influences of social media on students
Vygotsky’s mediational theory (see Fernyhough, 2008 ) can be regarded as a main theoretical background for the support of social media on learners’ affective states. Based on this theory, social media can play the role of a mediational means between learners and the real environment. Learners’ understanding of this environment can be mediated by the image shaped via social media. This image can be either close to or different from the reality. In the case of the former, learners can develop their self-image and self-esteem. In the case of the latter, learners might develop unrealistic expectations of themselves by comparing themselves to others. As it will be reviewed below among the affective variables increased or decreased in students under the influence of the massive use of social media are anxiety, stress, depression, distress, rumination, and self-esteem. These effects have been explored more among school students in the age range of 13–18 than university students (above 18), but some studies were investigated among college students as well. Exemplary works of research on these affective variables are reviewed here.
In a cross-sectional study, O’Dea and Campbell (2011) explored the impact of online interactions of social networks on the psychological distress of adolescent students. These researchers found a negative correlation between the time spent on social networking and mental distress. Dumitrache et al. (2012) explored the relations between depression and the identity associated with the use of the popular social media, the Facebook. This study showed significant associations between depression and the number of identity-related information pieces shared on this social network. Neira and Barber (2014) explored the relationship between students’ social media use and depressed mood at teenage. No significant correlation was found between these two variables. In the same year, Tsitsika et al. (2014) explored the associations between excessive use of social media and internalizing emotions. These researchers found a positive correlation between more than 2-h a day use of social media and anxiety and depression.
Hanprathet et al. (2015) reported a statistically significant positive correlation between addiction to Facebook and depression among about a thousand high school students in wealthy populations of Thailand and warned against this psychological threat. Sampasa-Kanyinga and Lewis (2015) examined the relationship between social media use and psychological distress. These researchers found that the use of social media for more than 2 h a day was correlated with a higher intensity of psychological distress. Banjanin et al. (2015) tested the relationship between too much use of social networking and depression, yet found no statistically significant correlation between these two variables. Frison and Eggermont (2016) examined the relationships between different forms of Facebook use, perceived social support of social media, and male and female students’ depressed mood. These researchers found a positive association between the passive use of the Facebook and depression and also between the active use of the social media and depression. Furthermore, the perceived social support of the social media was found to mediate this association. Besides, gender was found as the other factor to mediate this relationship.
Vernon et al. (2017) explored change in negative investment in social networking in relation to change in depression and externalizing behavior. These researchers found that increased investment in social media predicted higher depression in adolescent students, which was a function of the effect of higher levels of disrupted sleep. Barry et al. (2017) explored the associations between the use of social media by adolescents and their psychosocial adjustment. Social media activity showed to be positively and moderately associated with depression and anxiety. Another investigation was focused on secondary school students in China conducted by Li et al. (2017) . The findings showed a mediating role of insomnia on the significant correlation between depression and addiction to social media. In the same year, Yan et al. (2017) aimed to explore the time spent on social networks and its correlation with anxiety among middle school students. They found a significant positive correlation between more than 2-h use of social networks and the intensity of anxiety.
Also in China, Wang et al. (2018) showed that addiction to social networking sites was correlated positively with depression, and this correlation was mediated by rumination. These researchers also found that this mediating effect was moderated by self-esteem. It means that the effect of addiction on depression was compounded by low self-esteem through rumination. In another work of research, Drouin et al. (2018) showed that though social media is expected to act as a form of social support for the majority of university students, it can adversely affect students’ mental well-being, especially for those who already have high levels of anxiety and depression. In their research, the social media resources were found to be stress-inducing for half of the participants, all university students. The higher education population was also studied by Iwamoto and Chun (2020) . These researchers investigated the emotional effects of social media in higher education and found that the socially supportive role of social media was overshadowed in the long run in university students’ lives and, instead, fed into their perceived depression, anxiety, and stress.
Keles et al. (2020) provided a systematic review of the effect of social media on young and teenage students’ depression, psychological distress, and anxiety. They found that depression acted as the most frequent affective variable measured. The most salient risk factors of psychological distress, anxiety, and depression based on the systematic review were activities such as repeated checking for messages, personal investment, the time spent on social media, and problematic or addictive use. Similarly, Mathewson (2020) investigated the effect of using social media on college students’ mental health. The participants stated the experience of anxiety, depression, and suicidality (thoughts of suicide or attempts to suicide). The findings showed that the types and frequency of using social media and the students’ perceived mental health were significantly correlated with each other.
The body of research on the effect of social media on students’ affective and emotional states has led to mixed results. The existing literature shows that there are some positive and some negative affective impacts. Yet, it seems that the latter is pre-dominant. Mathewson (2020) attributed these divergent positive and negative effects to the different theoretical frameworks adopted in different studies and also the different contexts (different countries with whole different educational systems). According to Fredrickson’s broaden-and-build theory of positive emotions ( Fredrickson, 2001 ), the mental repertoires of learners can be built and broadened by how they feel. For instance, some external stimuli might provoke negative emotions such as anxiety and depression in learners. Having experienced these negative emotions, students might repeatedly check their messages on social media or get addicted to them. As a result, their cognitive repertoire and mental capacity might become limited and they might lose their concentration during their learning process. On the other hand, it should be noted that by feeling positive, learners might take full advantage of the affordances of the social media and; thus, be able to follow their learning goals strategically. This point should be highlighted that the link between the use of social media and affective states is bi-directional. Therefore, strategic use of social media or its addictive use by students can direct them toward either positive experiences like enjoyment or negative ones such as anxiety and depression. Also, these mixed positive and negative effects are similar to the findings of several other relevant studies on general populations’ psychological and emotional health. A number of studies (with general research populations not necessarily students) showed that social networks have facilitated the way of staying in touch with family and friends living far away as well as an increased social support ( Zhang, 2017 ). Given the positive and negative emotional effects of social media, social media can either scaffold the emotional repertoire of students, which can develop positive emotions in learners, or induce negative provokers in them, based on which learners might feel negative emotions such as anxiety and depression. However, admittedly, social media has also generated a domain that encourages the act of comparing lives, and striving for approval; therefore, it establishes and internalizes unrealistic perceptions ( Virden et al., 2014 ; Radovic et al., 2017 ).
It should be mentioned that the susceptibility of affective variables to social media should be interpreted from a dynamic lens. This means that the ecology of the social media can make changes in the emotional experiences of learners. More specifically, students’ affective variables might self-organize into different states under the influence of social media. As for the positive correlation found in many studies between the use of social media and such negative effects as anxiety, depression, and stress, it can be hypothesized that this correlation is induced by the continuous comparison the individual makes and the perception that others are doing better than him/her influenced by the posts that appear on social media. Using social media can play a major role in university students’ psychological well-being than expected. Though most of these studies were correlational, and correlation is not the same as causation, as the studies show that the number of participants experiencing these negative emotions under the influence of social media is significantly high, more extensive research is highly suggested to explore causal effects ( Mathewson, 2020 ).
As the review of exemplary studies showed, some believed that social media increased comparisons that students made between themselves and others. This finding ratifies the relevance of the Interpretation Comparison Model ( Stapel and Koomen, 2000 ; Stapel, 2007 ) and Festinger’s (1954) Social Comparison Theory. Concerning the negative effects of social media on students’ psychology, it can be argued that individuals may fail to understand that the content presented in social media is usually changed to only represent the attractive aspects of people’s lives, showing an unrealistic image of things. We can add that this argument also supports the relevance of the Social Comparison Theory and the Interpretation Comparison Model ( Stapel and Koomen, 2000 ; Stapel, 2007 ), because social media sets standards that students think they should compare themselves with. A constant observation of how other students or peers are showing their instances of achievement leads to higher self-evaluation ( Stapel and Koomen, 2000 ). It is conjectured that the ubiquitous role of social media in student life establishes unrealistic expectations and promotes continuous comparison as also pinpointed in the Interpretation Comparison Model ( Stapel and Koomen, 2000 ; Stapel, 2007 ).
Implications of the study
The use of social media is ever increasing among students, both at school and university, which is partly because of the promises of technological advances in communication services and partly because of the increased use of social networks for educational purposes in recent years after the pandemic. This consistent use of social media is not expected to leave students’ psychological, affective and emotional states untouched. Thus, it is necessary to know how the growing usage of social networks is associated with students’ affective health on different aspects. Therefore, we found it useful to summarize the research findings in recent years in this respect. If those somehow in charge of student affairs in educational settings are aware of the potential positive or negative effects of social media usage on students, they can better understand the complexities of students’ needs and are better capable of meeting them.
Psychological counseling programs can be initiated at schools or universities to check upon the latest state of students’ mental and emotional health influenced by the pervasive use of social media. The counselors can be made aware of the potential adverse effects of social networking and can adapt the content of their inquiries accordingly. Knowledge of the potential reasons for student anxiety, depression, and stress can help school or university counselors to find individualized coping strategies when they diagnose any symptom of distress in students influenced by an excessive use of social networking.
Admittedly, it is neither possible to discard the use of social media in today’s academic life, nor to keep students’ use of social networks fully controlled. Certainly, the educational space in today’s world cannot do without the social media, which has turned into an integral part of everybody’s life. Yet, probably students need to be instructed on how to take advantage of the media and to be the least affected negatively by its occasional superficial and unrepresentative content. Compensatory programs might be needed at schools or universities to encourage students to avoid making unrealistic and impartial comparisons of themselves and the flamboyant images of others displayed on social media. Students can be taught to develop self-appreciation and self-care while continuing to use the media to their benefit.
The teachers’ role as well as the curriculum developers’ role are becoming more important than ever, as they can significantly help to moderate the adverse effects of the pervasive social media use on students’ mental and emotional health. The kind of groupings formed for instructional purposes, for example, in social media can be done with greater care by teachers to make sure that the members of the groups are homogeneous and the tasks and activities shared in the groups are quite relevant and realistic. The teachers cannot always be in a full control of students’ use of social media, and the other fact is that students do not always and only use social media for educational purposes. They spend more time on social media for communicating with friends or strangers or possibly they just passively receive the content produced out of any educational scope just for entertainment. This uncontrolled and unrealistic content may give them a false image of life events and can threaten their mental and emotional health. Thus, teachers can try to make students aware of the potential hazards of investing too much of their time on following pages or people that publish false and misleading information about their personal or social identities. As students, logically expected, spend more time with their teachers than counselors, they may be better and more receptive to the advice given by the former than the latter.
Teachers may not be in full control of their students’ use of social media, but they have always played an active role in motivating or demotivating students to take particular measures in their academic lives. If teachers are informed of the recent research findings about the potential effects of massively using social media on students, they may find ways to reduce students’ distraction or confusion in class due to the excessive or over-reliant use of these networks. Educators may more often be mesmerized by the promises of technology-, computer- and mobile-assisted learning. They may tend to encourage the use of social media hoping to benefit students’ social and interpersonal skills, self-confidence, stress-managing and the like. Yet, they may be unaware of the potential adverse effects on students’ emotional well-being and, thus, may find the review of the recent relevant research findings insightful. Also, teachers can mediate between learners and social media to manipulate the time learners spend on social media. Research has mainly indicated that students’ emotional experiences are mainly dependent on teachers’ pedagogical approach. They should refrain learners from excessive use of, or overreliance on, social media. Raising learners’ awareness of this fact that individuals should develop their own path of development for learning, and not build their development based on unrealistic comparison of their competences with those of others, can help them consider positive values for their activities on social media and, thus, experience positive emotions.
At higher education, students’ needs are more life-like. For example, their employment-seeking spirits might lead them to create accounts in many social networks, hoping for a better future. However, membership in many of these networks may end in the mere waste of the time that could otherwise be spent on actual on-campus cooperative projects. Universities can provide more on-campus resources both for research and work experience purposes from which the students can benefit more than the cyberspace that can be tricky on many occasions. Two main theories underlying some negative emotions like boredom and anxiety are over-stimulation and under-stimulation. Thus, what learners feel out of their involvement in social media might be directed toward negative emotions due to the stimulating environment of social media. This stimulating environment makes learners rely too much, and spend too much time, on social media or use them obsessively. As a result, they might feel anxious or depressed. Given the ubiquity of social media, these negative emotions can be replaced with positive emotions if learners become aware of the psychological effects of social media. Regarding the affordances of social media for learners, they can take advantage of the potential affordances of these media such as improving their literacy, broadening their communication skills, or enhancing their distance learning opportunities.
A review of the research findings on the relationship between social media and students’ affective traits revealed both positive and negative findings. Yet, the instances of the latter were more salient and the negative psychological symptoms such as depression, anxiety, and stress have been far from negligible. These findings were discussed in relation to some more relevant theories such as the social comparison theory, which predicted that most of the potential issues with the young generation’s excessive use of social media were induced by the unfair comparisons they made between their own lives and the unrealistic portrayal of others’ on social media. Teachers, education policymakers, curriculum developers, and all those in charge of the student affairs at schools and universities should be made aware of the psychological effects of the pervasive use of social media on students, and the potential threats.
It should be reminded that the alleged socially supportive and communicative promises of the prevalent use of social networking in student life might not be fully realized in practice. Students may lose self-appreciation and gratitude when they compare their current state of life with the snapshots of others’ or peers’. A depressed or stressed-out mood can follow. Students at schools or universities need to learn self-worth to resist the adverse effects of the superficial support they receive from social media. Along this way, they should be assisted by the family and those in charge at schools or universities, most importantly the teachers. As already suggested, counseling programs might help with raising students’ awareness of the potential psychological threats of social media to their health. Considering the ubiquity of social media in everybody’ life including student life worldwide, it seems that more coping and compensatory strategies should be contrived to moderate the adverse psychological effects of the pervasive use of social media on students. Also, the affective influences of social media should not be generalized but they need to be interpreted from an ecological or contextual perspective. This means that learners might have different emotions at different times or different contexts while being involved in social media. More specifically, given the stative approach to learners’ emotions, what learners emotionally experience in their application of social media can be bound to their intra-personal and interpersonal experiences. This means that the same learner at different time points might go through different emotions Also, learners’ emotional states as a result of their engagement in social media cannot be necessarily generalized to all learners in a class.
As the majority of studies on the psychological effects of social media on student life have been conducted on school students than in higher education, it seems it is too soon to make any conclusive remark on this population exclusively. Probably, in future, further studies of the psychological complexities of students at higher education and a better knowledge of their needs can pave the way for making more insightful conclusions about the effects of social media on their affective states.
Suggestions for further research
The majority of studies on the potential effects of social media usage on students’ psychological well-being are either quantitative or qualitative in type, each with many limitations. Presumably, mixed approaches in near future can better provide a comprehensive assessment of these potential associations. Moreover, most studies on this topic have been cross-sectional in type. There is a significant dearth of longitudinal investigation on the effect of social media on developing positive or negative emotions in students. This seems to be essential as different affective factors such as anxiety, stress, self-esteem, and the like have a developmental nature. Traditional research methods with single-shot designs for data collection fail to capture the nuances of changes in these affective variables. It can be expected that more longitudinal studies in future can show how the continuous use of social media can affect the fluctuations of any of these affective variables during the different academic courses students pass at school or university.
As already raised in some works of research reviewed, the different patterns of impacts of social media on student life depend largely on the educational context. Thus, the same research designs with the same academic grade students and even the same age groups can lead to different findings concerning the effects of social media on student psychology in different countries. In other words, the potential positive and negative effects of popular social media like Facebook, Snapchat, Twitter, etc., on students’ affective conditions can differ across different educational settings in different host countries. Thus, significantly more research is needed in different contexts and cultures to compare the results.
There is also a need for further research on the higher education students and how their affective conditions are positively and negatively affected by the prevalent use of social media. University students’ psychological needs might be different from other academic grades and, thus, the patterns of changes that the overall use of social networking can create in their emotions can be also different. Their main reasons for using social media might be different from school students as well, which need to be investigated more thoroughly. The sorts of interventions needed to moderate the potential negative effects of social networking on them can be different too, all requiring a new line of research in education domain.
Finally, there are hopes that considering the ever-increasing popularity of social networking in education, the potential psychological effects of social media on teachers be explored as well. Though teacher psychology has only recently been considered for research, the literature has provided profound insights into teachers developing stress, motivation, self-esteem, and many other emotions. In today’s world driven by global communications in the cyberspace, teachers like everyone else are affecting and being affected by social networking. The comparison theory can hold true for teachers too. Thus, similar threats (of social media) to self-esteem and self-worth can be there for teachers too besides students, which are worth investigating qualitatively and quantitatively.
Probably a new line of research can be initiated to explore the co-development of teacher and learner psychological traits under the influence of social media use in longitudinal studies. These will certainly entail sophisticated research methods to be capable of unraveling the nuances of variation in these traits and their mutual effects, for example, stress, motivation, and self-esteem. If these are incorporated within mixed-approach works of research, more comprehensive and better insightful findings can be expected to emerge. Correlational studies need to be followed by causal studies in educational settings. As many conditions of the educational settings do not allow for having control groups or randomization, probably, experimental studies do not help with this. Innovative research methods, case studies or else, can be used to further explore the causal relations among the different features of social media use and the development of different affective variables in teachers or learners. Examples of such innovative research methods can be process tracing, qualitative comparative analysis, and longitudinal latent factor modeling (for a more comprehensive view, see Hiver and Al-Hoorie, 2019 ).
Author contributions
Both authors listed have made a substantial, direct, and intellectual contribution to the work, and approved it for publication.
This study was sponsored by Wuxi Philosophy and Social Sciences bidding project—“Special Project for Safeguarding the Rights and Interests of Workers in the New Form of Employment” (Grant No. WXSK22-GH-13). This study was sponsored by the Key Project of Party Building and Ideological and Political Education Research of Nanjing University of Posts and Telecommunications—“Research on the Guidance and Countermeasures of Network Public Opinion in Colleges and Universities in the Modern Times” (Grant No. XC 2021002).
Conflict of interest
Author XX was employed by China Mobile Group Jiangsu Co., Ltd.
The remaining author declares that the research was conducted in the absence of any commercial or financial relationships that could be construed as a potential conflict of interest.
Publisher’s note
All claims expressed in this article are solely those of the authors and do not necessarily represent those of their affiliated organizations, or those of the publisher, the editors and the reviewers. Any product that may be evaluated in this article, or claim that may be made by its manufacturer, is not guaranteed or endorsed by the publisher.
Aalbers, G., McNally, R. J., Heeren, A., de Wit, S., and Fried, E. I. (2018). Social media and depression symptoms: A network perspective. J. Exp. Psychol. Gen. 148, 1454–1462. doi: 10.1037/xge0000528
PubMed Abstract | CrossRef Full Text | Google Scholar
Abbott, J. (2017). Introduction: Assessing the social and political impact of the internet and new social media in Asia. J. Contemp. Asia 43, 579–590. doi: 10.1080/00472336.2013.785698
CrossRef Full Text | Google Scholar
Alahmar, A. T. (2016). The impact of social media on the academic performance of second year medical students at College of Medicine, University of Babylon, Iraq. J. Med. Allied Sci. 6, 77–83. doi: 10.5455/jmas.236927
Banjanin, N., Banjanin, N., Dimitrijevic, I., and Pantic, I. (2015). Relationship between internet use and depression: Focus on physiological mood oscillations, social networking and online addictive behavior. Comp. Hum. Behav. 43, 308–312. doi: 10.1016/j.chb.2014.11.013
Barry, C. T., Sidoti, C. L., Briggs, S. M., Reiter, S. R., and Lindsey, R. A. (2017). Adolescent social media use and mental health from adolescent and parent perspectives. J. Adolesc. 61, 1–11. doi: 10.1016/j.adolescence.2017.08.005
Chang, Y. (2012). The relationship between maladaptive perfectionism with burnout: Testing mediating effect of emotion-focused coping. Pers. Individ. Differ. 53, 635–639. doi: 10.1016/j.paid.2012.05.002
Charoensukmongkol, P. (2018). The impact of social media on social comparison and envy in teenagers: The moderating role of the parent comparing children and in-group competition among friends. J. Child Fam. Stud. 27, 69–79. doi: 10.1007/s10826-017-0872-8
Chukwuere, J. E., and Chukwuere, P. C. (2017). The impact of social media on social lifestyle: A case study of university female students. Gender Behav. 15, 9966–9981.
Google Scholar
Drouin, M., Reining, L., Flanagan, M., Carpenter, M., and Toscos, T. (2018). College students in distress: Can social media be a source of social support? Coll. Stud. J. 52, 494–504.
Dumitrache, S. D., Mitrofan, L., and Petrov, Z. (2012). Self-image and depressive tendencies among adolescent Facebook users. Rev. Psihol. 58, 285–295.
PubMed Abstract | Google Scholar
Fernyhough, C. (2008). Getting Vygotskian about theory of mind: Mediation, dialogue, and the development of social understanding. Dev. Rev. 28, 225–262. doi: 10.1016/j.dr.2007.03.001
Festinger, L. (1954). A Theory of social comparison processes. Hum. Relat. 7, 117–140. doi: 10.1177/001872675400700202
Fleck, J., and Johnson-Migalski, L. (2015). The impact of social media on personal and professional lives: An Adlerian perspective. J. Individ. Psychol. 71, 135–142. doi: 10.1353/jip.2015.0013
Fredrickson, B. L. (2001). The role of positive emotions in positive psychology: The broaden-and-build theory of positive emotions. Am. Psychol. 56, 218–226. doi: 10.1037/0003-066X.56.3.218
Frison, E., and Eggermont, S. (2016). Exploring the relationships between different types of Facebook use, perceived online social support, and adolescents’ depressed mood. Soc. Sci. Compu. Rev. 34, 153–171. doi: 10.1177/0894439314567449
Hanprathet, N., Manwong, M., Khumsri, J., Yingyeun, R., and Phanasathit, M. (2015). Facebook addiction and its relationship with mental health among Thai high school students. J. Med. Assoc. Thailand 98, S81–S90.
Hiver, P., and Al-Hoorie, A. H. (2019). Research Methods for Complexity Theory in Applied Linguistics. Bristol: Multilingual Matters. doi: 10.21832/HIVER5747
Iwamoto, D., and Chun, H. (2020). The emotional impact of social media in higher education. Int. J. High. Educ. 9, 239–247. doi: 10.5430/ijhe.v9n2p239
Keles, B., McCrae, N., and Grealish, A. (2020). A systematic review: The influence of social media on depression, anxiety and psychological distress in adolescents. Int. J. Adolesc. Youth 25, 79–93. doi: 10.1080/02673843.2019.1590851
Ley, B., Ogonowski, C., Hess, J., Reichling, T., Wan, L., and Wulf, V. (2014). Impacts of new technologies on media usage and social behavior in domestic environments. Behav. Inform. Technol. 33, 815–828. doi: 10.1080/0144929X.2013.832383
Li, J.-B., Lau, J. T. F., Mo, P. K. H., Su, X.-F., Tang, J., Qin, Z.-G., et al. (2017). Insomnia partially mediated the association between problematic Internet use and depression among secondary school students in China. J. Behav. Addict. 6, 554–563. doi: 10.1556/2006.6.2017.085
Mathewson, M. (2020). The impact of social media usage on students’ mental health. J. Stud. Affairs 29, 146–160.
Neira, B. C. J., and Barber, B. L. (2014). Social networking site use: Linked to adolescents’ social self-concept, self-esteem, and depressed mood. Aus. J. Psychol. 66, 56–64. doi: 10.1111/ajpy.12034
O’Dea, B., and Campbell, A. (2011). Online social networking amongst teens: Friend or foe? Ann. Rev. CyberTher. Telemed. 9, 108–112.
Radovic, A., Gmelin, T., Stein, B. D., and Miller, E. (2017). Depressed adolescents positive and negative use of social media. J. Adolesc. 55, 5–15. doi: 10.1016/j.adolescence.2016.12.002
Sampasa-Kanyinga, H., and Lewis, R. F. (2015). Frequent use of social networking sites is associated with poor psychological functioning among children and adolescents. Cyberpsychol. Behav. Soc. Network. 18, 380–385. doi: 10.1089/cyber.2015.0055
Sriwilai, K., and Charoensukmongkol, P. (2016). Face it, don’t Facebook it: Impacts of social media addiction on mindfulness, coping strategies and the consequence on emotional exhaustion. Stress Health 32, 427–434. doi: 10.1002/smi.2637
Stapel, D. A. (2007). “In the mind of the beholder: The interpretation comparison model of accessibility effects,” in Assimilation and Contrast in Social Psychology , eds D. A. Stapel and J. Suls (London: Psychology Press), 143–164.
Stapel, D. A., and Koomen, W. (2000). Distinctiveness of others, mutability of selves: Their impact on self-evaluations. J. Pers. Soc. Psychol. 79, 1068–1087. doi: 10.1037//0022-3514.79.6.1068
Tang, F., Wang, X., and Norman, C. S. (2013). An investigation of the impact of media capabilities and extraversion on social presence and user satisfaction. Behav. Inform. Technol. 32, 1060–1073. doi: 10.1080/0144929X.2013.830335
Tsitsika, A. K., Tzavela, E. C., Janikian, M., Ólafsson, K., Iordache, A., Schoenmakers, T. M., et al. (2014). Online social networking in adolescence: Patterns of use in six European countries and links with psychosocial functioning. J. Adolesc. Health 55, 141–147. doi: 10.1016/j.jadohealth.2013.11.010
Vernon, L., Modecki, K. L., and Barber, B. L. (2017). Tracking effects of problematic social networking on adolescent psychopathology: The mediating role of sleep disruptions. J. Clin. Child Adolesc. Psychol. 46, 269–283. doi: 10.1080/15374416.2016.1188702
Virden, A., Trujillo, A., and Predeger, E. (2014). Young adult females’ perceptions of high-risk social media behaviors: A focus-group approach. J. Commun. Health Nurs. 31, 133–144. doi: 10.1080/07370016.2014.926677
Wang, P., Wang, X., Wu, Y., Xie, X., Wang, X., Zhao, F., et al. (2018). Social networking sites addiction and adolescent depression: A moderated mediation model of rumination and self-esteem. Pers. Individ. Differ. 127, 162–167. doi: 10.1016/j.paid.2018.02.008
Weng, L., and Menczer, F. (2015). Topicality and impact in social media: Diverse messages, focused messengers. PLoS One 10:e0118410. doi: 10.1371/journal.pone.0118410
Yan, H., Zhang, R., Oniffrey, T. M., Chen, G., Wang, Y., Wu, Y., et al. (2017). Associations among screen time and unhealthy behaviors, academic performance, and well-being in Chinese adolescents. Int. J. Environ. Res. Public Health 14:596. doi: 10.3390/ijerph14060596
Zareen, N., Karim, N., and Khan, U. A. (2016). Psycho-emotional impact of social media emojis. ISRA Med. J. 8, 257–262.
Zhang, R. (2017). The stress-buffering effect of self-disclosure on Facebook: An examination of stressful life events, social support, and mental health among college students. Comp. Hum. Behav. 75, 527–537. doi: 10.1016/j.chb.2017.05.043
Keywords : affective variables, education, emotions, social media, post-pandemic, emotional needs
Citation: Chen M and Xiao X (2022) The effect of social media on the development of students’ affective variables. Front. Psychol. 13:1010766. doi: 10.3389/fpsyg.2022.1010766
Received: 03 August 2022; Accepted: 25 August 2022; Published: 15 September 2022.
Reviewed by:
Copyright © 2022 Chen and Xiao. This is an open-access article distributed under the terms of the Creative Commons Attribution License (CC BY) . The use, distribution or reproduction in other forums is permitted, provided the original author(s) and the copyright owner(s) are credited and that the original publication in this journal is cited, in accordance with accepted academic practice. No use, distribution or reproduction is permitted which does not comply with these terms.
*Correspondence: Miao Chen, [email protected] ; Xin Xiao, [email protected]
Disclaimer: All claims expressed in this article are solely those of the authors and do not necessarily represent those of their affiliated organizations, or those of the publisher, the editors and the reviewers. Any product that may be evaluated in this article or claim that may be made by its manufacturer is not guaranteed or endorsed by the publisher.
- Open access
- Published: 21 November 2024
Fostering college students’ mental well-being: the impact of social networking site utilization on emotion management and regulation
- Yuehua Han 1 &
- Zhifen Xu 1
BMC Psychology volume 12 , Article number: 681 ( 2024 ) Cite this article
Metrics details
With the widespread proliferation of the Internet, social networking sites have increasingly become integrated into the daily lives of university students, leading to a growing reliance on these platforms. Several studies have suggested that this emotional dependence on social networking sites stems from unmet psychological needs. Meanwhile, social rejection has been identified as a prevalent phenomenon that exacerbates the deficiency of individual psychological needs. However, existing research on aspect-level sentiment analysis among college students within social networking sites faces challenges such as inadequate feature extraction, ineffective handling of data noise, and the neglect of complex interactions in multimodal data. To address these issues, this paper introduces a novel approach, the Multi-Granular View Dynamic Fusion Model (MVDFM), developed from both coarse-grained and fine-grained perspectives. MVDFM extracts multi-granular view features from textual and visual content, incorporating a dynamic gating self-attention mechanism. Additionally, it proposes a three-view decomposition higher-order pooling mechanism for a two-stage dynamic fusion of these features. Experimental results demonstrate the model’s effectiveness, achieving accuracy and F1 values of 78.78% and 74.48% on the Twitter-2015 dataset, and 73.89% and 72.47% on the Twitter-2017 dataset, respectively. This efficient supervision enables the extraction of deep semantic information from multimodal data generated by college students on social networking sites. The model adeptly mines pertinent information related to target aspect-based words, enhancing the efficacy of aspect-level emotion prediction. Furthermore, it facilitates an effective exploration of the intricate interplay between social rejection, monitoring on social networking sites, the fear of missing out, and dependence on social networking sites, ultimately aiding university students in regulating their emotional management.
Peer Review reports
Introduction
While social networking sites function as technological mediators, and communication studies address technical concerns, the domain of psychology delves into the concealed psychological needs underlying this technological efficacy and the influencing mechanisms. The user base of social networking sites continues to burgeon annually, with site functionalities iterating to align with evolving user demands. Traditional instant messaging and basic personal information no longer suffice for contemporary users. The increasing sharing of personal information provides a more detailed picture of individuals, fostering a proclivity among users to seek more information, potentially leading to social networking site dependence.
Diverging from the common perception of “Internet addiction,” social networking dependence not only emphasizes usage duration but also underscores the interplay between an individual’s goal attainment abilities and their capacity to create, gather, process, and disseminate information on social networks [ 1 ]. Numerous studies have corroborated that social networking site addiction heightens levels of depression and anxiety, diminishes academic performance, and diminishes the overall psychological well-being of students [ 2 ]. Specifically tailored as an interactive platform for college students, social networking sites facilitate the expansion of social circles and the augmentation of opportunities for interpersonal engagement. When mitigating social networking site dependence, judicious use of these platforms can foster the psychological well-being of college students, aiding in stress alleviation, bolstering their sense of self-identity, and fortifying social support. Furthermore, the diverse content and abundant information on social networking sites cater to college students’ curiosity and thirst for knowledge. By perusing and assimilating such information, college students can enrich their knowledge base and elevate their cognitive proficiency, thereby enhancing their emotional management capabilities.
However, the utilization of social networking sites inevitably brings about some adverse consequences. Comments and visual content on these platforms can evoke potent emotional responses from college students. Excessive reliance on social networking sites may result in a distancing of real-life relationships and a decline in face-to-face communication skills. Additionally, negative information, such as unfounded criticism or attacks, occasionally surfaces on social networking sites, and prolonged exposure to such environments may impose stress on the mental health of college students, impacting their emotional management.
To foster the cultivation of resilient mental states among college students confronted with negative information on social networking sites, scholars in the research community have advocated for aspect-level sentiment analysis. This analytical approach aims to discern the sentiment tendencies of individual aspectual entities within textual expressions [ 3 ]. In contrast to conventional sentiment analysis tasks, this fine-grained sentiment analysis provides more nuanced and targeted insights, aligning closely with the intricacies of genuine emotional cognition, thereby garnering substantial attention from academia. However, prevailing sentiment classification methods predominantly rely on textual data, neglecting the valuable auxiliary information that multimodal data, such as images and videos, can furnish.
In recent years, propelled by advancements in multimedia technology, the content consumed by college students on social networking sites has embraced a multimodal trajectory. Beyond mere textual comments or tweets, users now incorporate data with diverse modalities, including images, to enrich their expressive viewpoints [ 4 ]. These interconnected multimodal data [ 5 ] possess untapped potential for intuitive expression and the conveyance of opinionated information. Consequently, integrating information from text-related images becomes imperative to fortify the robustness of aspect-level sentiment analysis models. It necessitates the dynamic emphasis of focal elements in each feature vector based on distinct target aspect words, thereby augmenting the quality of view feature generation. In response, this paper introduces the Multi-Granularity View Dynamic Fusion Model (MVDFM) with the ensuing key contributions:
From both coarse-grained and fine-grained perspectives, vectorization and coding of text and image data on social networks are executed to comprehensively capture data features and enhance the information representation of the model.
Extraction of multi-granularity view features from text and images is achieved, complemented by the design of a dynamic gating self-attention mechanism for noise reduction at the fine-granularity level, ensuring the meticulous quality of feature extraction.
To exploit the complementarity and coherence across multiple views at distinct granularities, a Triple-View Factorized High-order pooling (TFH) mechanism is introduced. This mechanism facilitates a two-stage dynamic fusion of multi-granularity view features, culminating in the derivation of the final sentiment polarity of the target aspect word.
Section 2 provides an overview of the current landscape in social networking site dependency and aspect-level sentiment analysis. The construction of the MVDFM is detailed in Sect. 3. Section 4 is dedicated to elucidating experimental results, scrutinizing the scheme’s performance, conducting a comparative analysis with classical schemes, and presenting ablation experiments that dissect the role of each module in the model. Furthermore, the section delves into exploring the interplay between social exclusion, social networking site monitoring, the fear of missing out, and social networking site dependency under affective prediction.
Related works
Social networking site dependency.
On social networking sites, college students curate personal profiles that enables the linkage of their individual pages with those of friends. These friends, granted access to profiles, home pages, and other information, can engage in instantaneous messaging at their convenience. In certain communication studies, a proclivity exists to differentiate between social networking sites and social media. Nonetheless, within the realms of psychology and select communication research, there persists a belief that a distinct demarcation between the two is unnecessary. Unlike their early singular functionalities, contemporary popular domestic social networking sites now exhibit attributes akin to social media platforms. For instance, on WeChat, the circle of friends facilitates the sharing of web links, while QQ permits the perusal of copious information within a user’s space. Simultaneously, social networking sites have assimilated various social attributes. Platforms like microblogging enable reciprocal attention for viewing homepages and instant messaging. In addressing deleterious habits associated with social networking sites, the research community employs terminology such as social networking site addiction and problematic social networking site use. Notably, social networking site dependence is emerging as a focal point within this discourse.
Literature [ 6 ] has outlined internet dependence, segmenting it into five subtypes. Social networking site dependence, an expansive concept, has been elucidated through various perspectives. Some studies extend it from the foundation of media and technology dependence. Initially, scholars introduced the overarching concept of technology dependence into the realm of social networking site dependence. Certain experts posit that technology dependence serves as a pivotal determinant influencing behavioral habits in using social networking sites. Conversely, other studies build upon the framework of psychological trust, contending that dependence is fundamentally a psychological state. Dependence is further categorized into material dependence, primarily associated with substance abuse, and psychological dependence. Literature [ 7 ] defines social networking site dependence as an incapacity to regulate one’s usage of these platforms due to a deficiency in self-regulation within the context of psychological dependence.
Additionally, literature [ 8 ] posits that social media dependence revolves around an individual’s perception of the pivotal role social media plays in achieving daily life goals. It is also considered a manifestation of addictive behavior, emphasizing the psychological state associated with using social networking sites. Social media dependence is delineated as a dual manifestation, involving both psychological and behavioral dependence, stemming from a lack of self-regulation within the context of psychological dependence [ 9 ]. Concurrently, the utilization of social networking sites leaves an imprint on college students’ self-perception. The interactive platforms within social networks facilitate an understanding of peers’ life statuses and garner evaluations and feedback about oneself from others [ 10 ]. This interactive process enables college students to enhance their self-awareness through social comparison and attain opportunities for self-improvement. Nevertheless, it is acknowledged that this interaction may also wield a negative impact on college students’ self-perception.
Other research scholars have explored how news recommendation systems with values and diversity perception affect readers’ news choices [ 11 ], focusing on the impact of misinformation generated by generative AI on user information processing [ 12 ], and how people identify misinformation in health advice provided by AI [ 13 ]. These provide a rich background and theoretical support for the study of the effects of social networking site use on college students’ mental health and emotional management. They reveal the effects of algorithms, information recommendation and misinformation on user psychology and behavior, and provide guidance for formulating effective intervention strategies.
In summary, the impact of social networking site use on college students’ mental health and emotion management regulation is intricate. While it can, to a certain extent, mitigate loneliness, enhance the sense of social support, and augment emotion management skills, an excessive dependence on social networking sites may engender adverse effects. Consequently, college students are advised to adhere to the principle of moderation, enjoying the convenience and amusement offered by social networking sites while remaining vigilant about potential risks. In light of this, scholars in the research community advocate for the implementation of affective predictive analytics to proactively manage and regulate college students’ emotions on social networking sites, fostering a trajectory toward healthy psychological development.
- Sentiment analysis
Amidst the advancement of deep learning techniques, a plethora of studies has embraced the integration of deep neural networks to autonomously extract feature representation information pertaining to aspectual words for aspect-level sentiment analysis. Long Short-Term Memory (LSTM) [ 14 ], a venerable model in natural language processing, renowned for its robust sequence modeling capabilities and is frequently employed to tackle aspect-level sentiment analysis challenges. Literature [ 15 ] introduces the Target-Dependent Long Short-Term Memory (TDLSTM) model, an enhancement of LSTM. This model utilizes LSTM for feature extraction on aspectual words, their preceding, and succeeding texts, enabling the prediction of aspect-level sentiment. While LSTM demonstrates commendable performance, its limitation lies in extracting sequential features without the ability to selectively emphasize pertinent viewpoint information related to aspectual words. This lacuna is adeptly addressed by the attention mechanism [ 16 ], which calculates weights to gauge the significance of different words in the text. The attention mechanism has emerged as a ubiquitous model in aspect-level sentiment analysis.
Building upon LSTM networks to discern the latent semantics of context and aspectual words, literature [ 17 ] devises an Interactive Attention Network (IAN) rooted in the attention mechanism. IAN accurately predicts sentiment polarity by directing attention towards the words most germane to aspectual words. In a bid to fortify the interrelations between aspectual words and their corresponding viewpoint information, literature [ 18 ] introduces a novel model leveraging Graph Convolutional Network (GCN) [ 19 ]. GCN is utilized to model the syntactic dependency tree of a text, thereby mining dependencies between syntactic information and words. This approach aims to mitigate issues of misalignment between aspects and irrelevant viewpoint words.
While the aforementioned methods have significantly propelled the progress of aspectual sentiment analysis, they predominantly focus on the examination of textual modal data, neglecting the informative contributions of other multimodal sources such as pictures and videos. These visual elements, increasingly prevalent in user-generated content, hold significant reference value for discerning the sentiment polarity of aspectual words. Addressing this gap, literature [ 20 ] pioneers the domain of multimodal aspect-level sentiment analysis by introducing the Multi-Interactive Memory Network (MIEN). MIEN employs a multi-hopping attention mechanism to model the intricate interactions among aspect words, text, and images, predicting the sentiment polarity of predefined aspect categories. Subsequent research, as exemplified by literature [ 21 ], delves into the interplay between target aspect words and text and image data. Utilizing the attention mechanism, local features pertaining to aspect words in textual and visual representations are mined, and the fused features contribute to improved predictions. The literature [ 22 ] focuses on emotion-driven distress identification and cause extraction within multimodal online posts. On social networking sites, university students frequently express their emotions and difficulties through various forms such as text, images, and videos. The method proposed in this paper standardizes the analysis of these multimodal data, enabling more accurate recognition of students’ emotional distress and extraction of the causes underlying their difficulties. Meanwhile, literature [ 23 ] explores the application of computational intelligence in natural language processing and sentiment analysis. Sentiment analysis is a crucial technique for understanding the emotions and opinions expressed in text, which is essential for analyzing the speech and emotional states of university students on social networking sites. Additionally, literature [ 24 ] introduces a context- and knowledge-enriched Transformer framework for recognizing emotions in conversations. Conversations among university students on social networking sites often contain rich contextual information and emotional exchanges, and the method proposed in this paper allows for more precise identification of emotions within these conversations.
Building upon this foundation, researchers have endeavored to enhance model performance by elevating the quality of feature generation. Literature [ 25 ] devises the Entity-Sensitive Attention and Fusion Network (ESAFN), leveraging the attention mechanism to capture dynamic relationships between aspect words and text/image data. This approach aims to enhance the quality of visual features by mitigating noise in the image data. Literature [ 26 ] introduces the Hierarchical Interactive Multimodal Transformer model (HIMT), featuring an auxiliary reconstruction module to diminish semantic disparities between images and text, thereby improving multimodal feature fusion. Meanwhile, literature [ 27 ] proposes the Cross-Modal Multitask Transformer (CIMT), employing auxiliary task control models like named entity recognition and image aspectual emotion prediction to generate aspect-word-aware textual and visual representations. A cross-modal interaction module is introduced for the final feature fusion to predict the sentiment polarity of target aspect words.
Despite the diversity of approaches, common challenges persist, particularly in insufficient feature extraction and the neglect of noise in text and image modalities unrelated to target aspect words. Consequently, for the task of aspect word sentiment prediction, further research is warranted to expand feature extraction, ensuring comprehensive information representation, and to address noise reduction in relevant text and image data, thereby preserving the quality of feature representation.
Methodology
The proposed method for multimodal aspect-level sentiment analysis, as delineated in this manuscript, encompasses key processes such as the encoding of multimodal data, dynamic extraction of multi-granular view features, fusion of multigranular view features, culminating in multimodal aspect-level sentiment prediction. The schematic representation of the model structure are illustrated in Fig. 1 . During multimodal data encoding, we leverage the text pre-training model, BERT, and the image pre-training model, chosen to encode text, aspect words, and images at varying granularity levels. This preparation is crucial for generating subsequent multigranular view features.
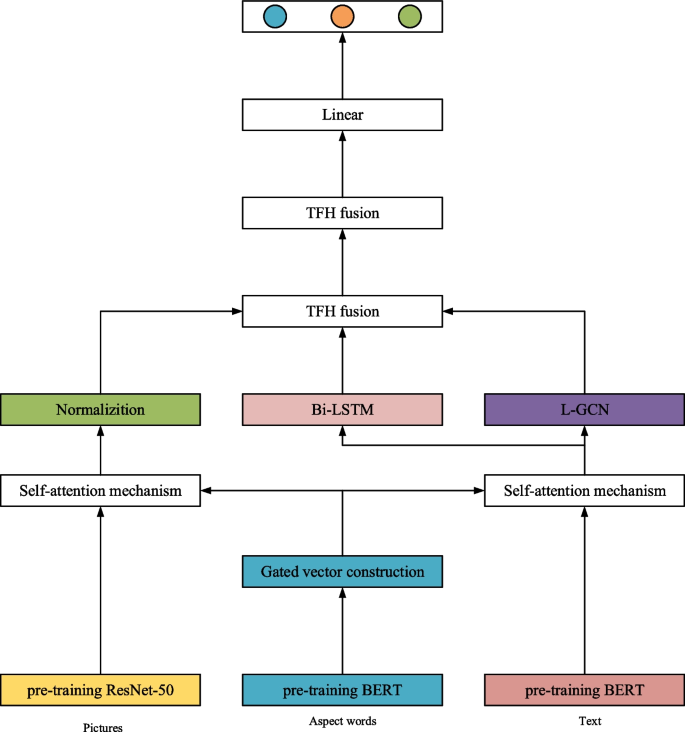
Model framework
The multi-modal aspect-level sentiment analysis method proposed in this paper, which is based on dynamic fusion of multi-grained views, primarily encompasses processes such as multi-modal data encoding, dynamic extraction of multi-grained view features, fusion of multi-grained view features, and ultimately, multi-modal aspect-level sentiment prediction. The specific model structure is illustrated in Fig. 1 . During the multi-modal data encoding phase, pre-trained text model BERT and image pre-trained models are selected to encode text, aspect terms, and images at different granularity levels, respectively, laying a solid foundation for the subsequent generation of multi-grained view features. Notably, the dynamic extraction module of multi-grained view features incorporates the core component of the MVDFM architecture—the dynamic gated self-attention mechanism. This mechanism dynamically adjusts the importance of features at different granularity levels based on contextual information and task requirements, effectively capturing crucial information. Specifically, it utilizes gating units to weight coarse-grained and fine-grained features, enabling the model to adaptively focus on the most critical feature views for sentiment analysis.
Furthermore, the MVDFM architecture encompasses an innovative triple-view decomposition high-order pooling mechanism. This mechanism targets the fused multi-grained view features and processes global views, regional views, as well as sequential and syntactic views through three independent branches, achieving high-order abstraction and detailed decomposition of features. Each branch employs advanced high-order pooling techniques to deeply explore the complex relationships between features and extract more representative high-order features. These high-order features are then integrated, further enhancing the model’s expressive power and generalization ability. Lastly, during the dimensionality reduction of the fused features, the model ingeniously utilizes activation functions to map high-dimensional features into a low-dimensional space while preserving critical sentiment information. Through this process, the model generates a prediction probability vector that accurately reflects the distribution probabilities of aspect terms across different sentiment categories, thereby achieving precise classification of aspect-level sentiments.

Multimodal data encoding
To fully harness the potential of multimodal data and comprehensively extract the rich information within, this study adopts a strategy of encoding images and text based on extraction granularity. For images, we leverage deep learning models pre-trained on large datasets to extract both global and regional features. Global features represent the overall image content, while regional features focus on specific parts of the image that are likely to contain sentiment-related information. To mitigate noise, we apply techniques such as data augmentation and regularization during model training, enhancing the model’s ability to generalize and filter out irrelevant information. Specifically, the text pre-training model, BERT, and the image pre-training model, ResNet50, are employed for the encoding of text, aspect words, and images, respectively. In the case of text and aspect words, a preprocessing step involves converting them to “[CLS] + text+[SEP]” and “[CLS] + aspect word+[SEP]” before inputting them into the BERT model for encoding. As for images, they undergo resizing to 224 × 224 pixels and are converted to the RGB three-channel format before being subjected to feature extraction using ResNet50.
Based on the above description, five information representations will eventually be derived. The text vectorization at sentence granularity output by the [CLS] tag of the BERT model is denoted as \(H_{s}^{0} \in {{\mathbb{R}}^{1 \times 768}}\) . The image vectorization at image granularity output by the last layer of ResNet50 is denoted as \(H_{i}^{0} \in {{\mathbb{R}}^{1 \times 768}}\) . The text vectorization at word granularity output by BERT is denoted as \(H_{s}^{{}} \in {{\mathbb{R}}^{n^\prime \times 768}}\) and the aspectual word vectorization is denoted as \({H_a} \in {{\mathbb{R}}^{r^\prime \times 768}}\) . The image vectorization at region granularity output by the penultimate layer of ResNet50 is denoted as \({H_i} \in {{\mathbb{R}}^{49 \times 768}}\) where \(n^\prime\) and \(r^\prime\) denote the encoded text and aspectual word sequence lengths,49 and the number of subregions into which an image is divided.
Feature extraction
To extract global view features for both text and image, this paper amalgamates the vectorized representation of text at the granularity of sentence vectors and the vectorized representation of the overall image granularity. This fusion incorporates aspect word feature information, including contextual context information, for both text and image.
Firstly, the context subsequence \(\left\{h_s^{k+1},h_s^{k+2},\dots,h_s^{k+r}\right\}\) is extracted as the aspect word feature matrix with contextual information based on the pre-recorded start position and the length of the aspect word, and secondly, it is subjected to average pooling operation to generate \(H_{{a^\prime}}^{0}\) . The feature vector generated by adding \(H_{s}^{0}\) and \(H_{{a^\prime}}^{0}\) vectors through the self-attention mechanism is used as the text global view feature \(View_{s}^{{Glo}}\) , and the feature vector generated by adding \(H_{i}^{0}\) and \(H_{a^\prime}^{0}\) through the self-attention mechanism is used as the image global view feature \(View_{i}^{{Glo}}\) , and the specific procedure is as follows.
Where r refers to the aspect word text length.
The fine-grained level view features that offer a richer expression of information, but concurrently, they tend to incorporate considerable noise unrelated to the sentiment expression itself. This redundant information is absolute noise. Furthermore, in instances where a single sample contains multiple aspect words with varying sentiment polarities, the information pertinent to aspect word A may be perceived as noise relative to aspect word B. To accurately extract viewpoint information related to the target aspect word, it is imperative to undertake noise reduction processing in the early stages of feature extraction to enhance the feature representation of both text and image. Accordingly, this paper introduces the dynamic gating self-attention mechanism, a module primarily grounded in the self-attention mechanism, enhanced with a specialized improvement in the gating mechanism, as illustrated in Fig. 2 .
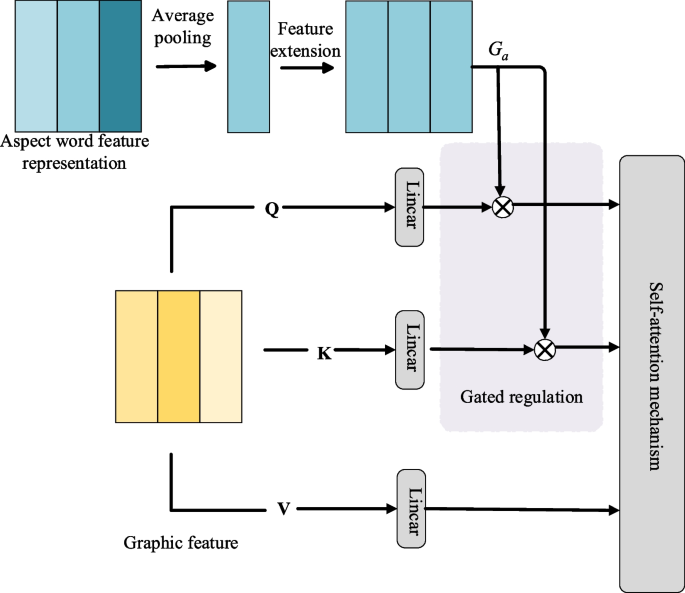
Dynamic gated self-attention mechanism
This module first utilizes the aspect word feature representation \({H_a}\) to construct the gating regulation vector \({G_a}\) , and the specific process is shown in Eq. ( 4 ):
Where \(\sigma ( \cdot )\) denotes the Sigmoid activation function, \({W_G}\) and \({b_G}\) are the trainable parameters, and \(~expand( \cdot )\) refers to the lengthwise extended feature vector. Secondly, the gating adjustment vector \({G_a}\) is embedded into the multi-head self-attention mechanism by Hadamard dot-product, which participates in the generation of \(H_{s}^{{{k_j}}}\) , \(H_{i}^{{{k_j}}}\) , and \(H_{s}^{{{Q_j}}}\) , \(H_{i}^{{{Q_j}}}\) in each attention head. The specific process is as in Eqs. ( 5 ) and ( 6 ).
Among them, \((1+G_{a}^{j})\) retains the features that have not been activated by the gating vector to enhance the activated features, and \(\circ\) is the Hadammard dot product. The features \(\tilde {H}_{s}^{{{k_j}}}\) , \(\tilde {H}_{i}^{{{k_j}}}\) , and \(\tilde {H}_{s}^{{{Q_j}}}\) , \(\tilde {H}_{i}^{{{Q_j}}}\) that have been adjusted by the gating vector will then be involved in the weighting process between Key and Query of the self-attention mechanism, at which time the calculated weights are dynamically adjusted. When assigning weights to the Value features, attention can be strategically directed towards pertinent text words or image regions. Specifically, higher weights are assigned to information associated with the target aspect words, while lower weights are allocated to information relatively unrelated to the target aspect words. This approach ensures that weights are tailored to different features based on their association with distinct aspect words. The information linked to the target aspect word is bestowed with a higher weight value, while information unrelated to the target aspect word is assigned a lower weight value. This dynamic weighting scheme facilitates effective noise reduction corresponding to different target aspect words. Finally, the text and image feature representations \({H_{sa}}\) and \({H_{ia}}\) which are sensitive to the aspect words are generated. The specific process is shown in Eqs. ( 7 , 8 , 9 , 10 ).
Where \(W_{{sa}}^{h}\) and \(W_{{ia}}^{h}\) are trainable parameters and h denotes the number of heads of the multi-head attention mechanism.
The multi-granularity view feature fusion layer introduces a Triple-view Factorized High-order pooling (TFH) mechanism designed for this model. The TFH mechanism employs a two-phase form to fuse the upper-layer text global view features \(View_{s}^{{Glo}}\) , image global view features \(View_{i}^{{Glo}}\) , text order view features \(Vie{w^{Seq}}\) , text syntax view features \(Vie{w^{Sny}}\) , and image region view features to exploit the complementarity and consistency between different view features at different granularities and enhance the information representation capability. region view feature \(Vie{w^{\operatorname{Re}g}}\) are fused to systematically investigate the complementarity and consistency across diverse view features at various granularities. This fusion process is aimed at enhancing the information representation capability of the merged features.
This paper initiates the design of TFB as the foundational stacking block for layer assembly to create the TFH, allowing for the extraction of deeper information. In contrast to coarse-grained view features, fine-grained view features encapsulate more nuanced local information. Consequently, these features are interactively fused, enabling the model to discern interactions among different local features in both images and text. To enhance the overall feature fusion process, this paper adopts a two-phase structure: first, achieving fusion interaction among fine-grained view features, followed by the fusion between coarse-grained views, culminating in the generation of the final fused features. The TFBs adeptly capture interactions between different features while preserving correlations among distinct modal features, serving as an effective fusion mechanism.
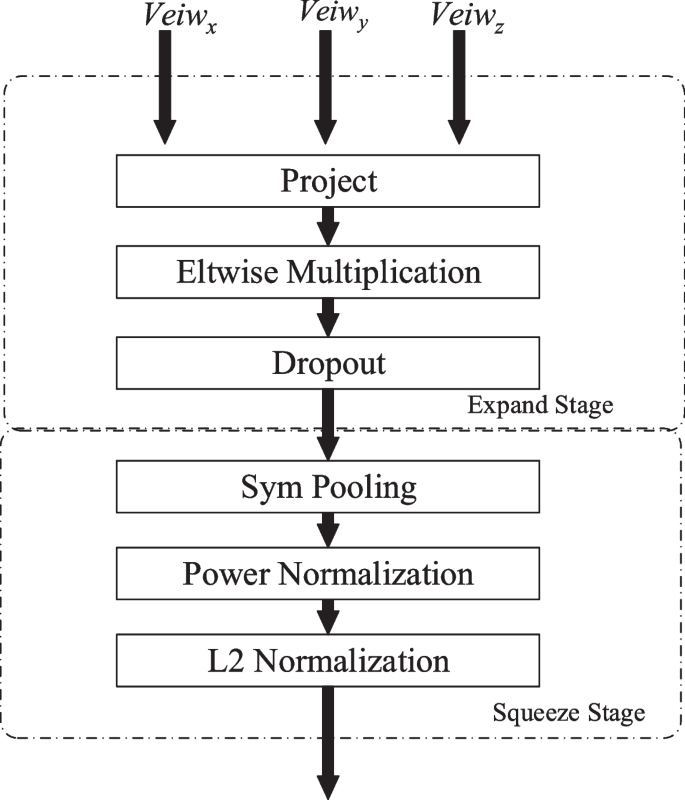
Three view decomposition bilinear pooling mechanism
Illustrated in Fig. 3 , each TFB comprises two phases: feature expansion and feature compression. In the feature expansion phase, a projection layer is employed to project inputs into a consistent space and dimension. The Hadamard dot product operation integrates features, exploring their correlations, with a discarding layer to prevent overfitting. In the feature compression phase, the mechanism utilizes summation and pooling aggregation for the integrated information, incorporating Power normalization and L2 regularization to guard against local convergence in the model.
Multimodal aspect-level sentiment prediction
The output layer aims to normalize the final fused feature output from the THF mechanism using a Softmax classifier, resulting in the probability values for each aspect word sentiment category. The sentiment associated with the maximum probability value is considered the model’s predicted result. The specific process is outlined as follows:
Where \(\hat {y}\) is the final sentiment prediction distribution, \({W_p}\) is the trainable parameters, and \({b_p}\) is the bias.
Acknowledging the ambiguity in defining neutral emotions and the potential unreliability of label values, this paper introduces Label Smoothing Regularization (LSR). LSR employs smoothed values instead of exclusively hot labels, providing the labels with a certain fault-tolerant space to enhance the model’s generalization performance. The generation process for smooth labels is articulated in Eq. ( 12 ).
Where \(y^\prime\left(k\vert x\right)\) denotes the newly generated smoothed label distribution of the sample x with label value k, \(y(k|x)\) is the original label distribution of the sample x with label value k for the smoothing parameter value \(\varepsilon\) is 0.2, \(u(k)\) denotes the prior distribution of the label k In this paper, we set it to be \(1/{\text{C}}\) . The KL dispersion is used to measure it as shown in Eq. ( 13 ):
The final loss function consists of the cross-entropy loss and the \({L_{lsr}}\) , \({L_2}\) regularization terms as in Eq. ( 14 ):
Where in is the coefficient of the \({L_2}\) regularization term and C is the number of categories of the label.
Experiments and analysis
This paper evaluates the model’s performance using two publicly available datasets: Twitter-2015 and Twitter-2017. Each dataset encompasses three sentiment polarities—positive, neutral, and negative—for every given aspect word. The inclusion of emotion-embedded data is valuable for assessing the model’s efficacy in regulating college students’ mental health and emotion management. The samples are partitioned into training, validation, and test sets in a 3:1:1 ratio. Model training is executed on the training set, with parameter adjustments based on experimental results from the validation set. The final performance evaluation is carried out on the test set.
The experiments were conducted using Windows 10 operating system, using PyCharmn2019.3 as the compilation software, based on the PyTorch deep learning framework, written in python3.8, and the models were trained and tested in an experimental environment of NVIDIAGeForceRTX3060 with 12GB of video memory.
Evaluation indicators
To optimize the model performance, this study employed the Adam optimizer for parameter updates. Following several experiments, the optimal parameter combinations for the model on the Twitter-2015 and Twitter-2017 datasets were determined. The specific experimental parameter settings are detailed in Table 1 .
In this paper, the model’s effectiveness is assessed using accuracy and F1 value as evaluation metrics for the sentiment analysis task. Accuracy represents the overall performance of the model on all samples. The F1 value, a harmonized mean of accuracy and recall, serves to balance the two metrics. A higher F1 value indicates strong performance in both accuracy and recall. Notations include TP for true positives, TN for true negatives, FP for false positives, and FN for false negatives. The calculations for accuracy and F1 value are presented below:
Model comparison under different datasets
In the model comparison, the selected models for comparison include the image unimodal model ResNet-Aspect [ 28 ], the graphic multimodal model ResNet-BERT, CIME [ 27 ], and HIMT [ 16 ]. The conclusive experimental results are presented in Fig. 4 .
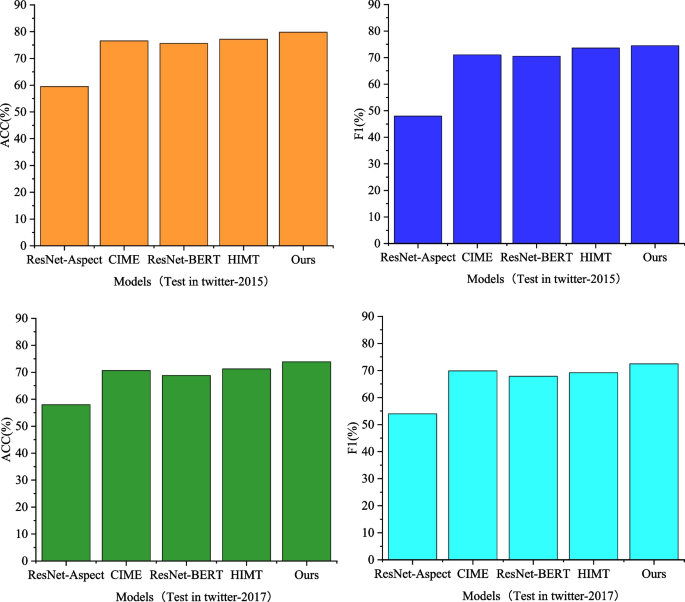
Comparison of model performance on different data sets
In our model comparison, we selected the following baseline models: the image-only model ResNet-Aspect [ 21 ], and the multimodal image-text models ResNet-BERT [ 22 ], CIME [ 20 ], and HIMT [ 19 ]. The final experimental results, presented in Fig. 4 , reveal that the image-only model ResNet-Aspect performed the worst among all models, achieving an accuracy and F1 score of 59.47% and 47.98% respectively on the Twitter2015 dataset. This suggests that the information related to aspect terms contained in images is limited, and text contains more valuable and exploitable content than images, serving as a crucial source of information for aspect-level sentiment analysis.
For text-only models, current research has shown that the pre-trained model BERT exhibits excellent representation capabilities for text content and can effectively learn context-related information, thereby enhancing model performance. Therefore, we also chose BERT to encode the text and aspect terms in our proposed model, MVDFM.
Furthermore, compared to BERT, ResNet-BERT achieved improved model performance, indicating that the incorporation of image data is beneficial for aspect-level sentiment analysis. Images enrich text information and can provide supplementary or supportive roles to text, thereby enhancing the effectiveness of sentiment classification.
Among all multimodal image-text models, CIME and HIMT significantly outperformed ResNet-BERT, which directly concatenates text and image features. This is because these multimodal models consider the matching relationship between aspect terms and multimodal data, and can reasonably design network architectures tailored to the characteristics of multimodal data to model the interaction between text and images, thereby improving the accuracy of aspect-level sentiment prediction.
Finally, our proposed model, MVDFM, achieved the best experimental results on both the Twitter-2015 and Twitter2017 datasets. Compared to the current state-of-the-art model HIMT, MVDFM improved accuracy by 0.55% and 1.67%, and F1 score by 0.88% and 2.45% respectively. On the Twitter2017 dataset, MVDFM achieved an accuracy and F1 score of 73.89% and 72.47% respectively. These results demonstrate that the overall design of the MVDFM model has significant advantages compared to other multimodal models. It can effectively utilize information from relevant text or images to predict sentiment polarity for different aspect terms, achieving good prediction performance.
Importantly, while the experiments primarily evaluated the performance of the models, future research should explore the practical applications of these models in mental health or emotion management. Empirical studies or discussions directly targeting these areas would further enhance the impact of our research. For example, by analyzing frequently occurring sad or negative graphic-text content, potential mental health risks can be promptly identified, enabling early interventions.
Ablation experiments
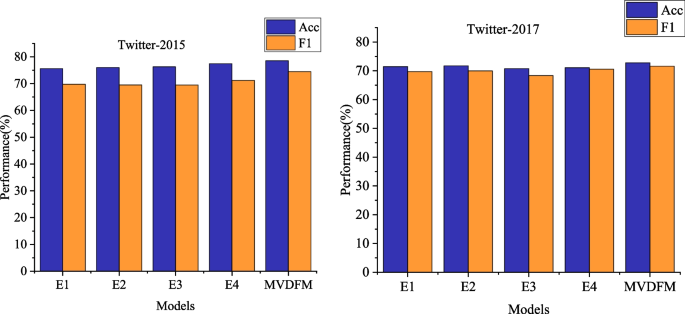
Results of ablation experiment
To further investigate the effectiveness of each component in the MVDFM model and its impact on overall performance, four sets of ablation experiments are designed. The experiments utilize the same two datasets, Twitter-2015 and Twitter-2017, and maintain consistent parameter settings with the full model. The specific ablation models are outlined as follows:
E1: Remove the dynamic self-attention mechanism from the original model and directly employ feature splicing to fuse aspect word information with images and text.
E2: Eliminate the text global view features and image global view features from the original model, retaining only the image region view features, text sequence view features, and text syntax view features for aspect-level sentiment prediction.
E3: Exclude the text-image region view features, text-phrase order view features, and text-syntax view features from the original model, preserving only the global view features and the image global view features for aspect-level sentiment prediction.
E4: Omit the TFH fusion mechanism from the original model and directly fuse the five view features using average pooling and feature splicing.
Figure 5 illustrates that the full model outperforms the ablated models in terms of performance. First, the removal of either coarse-grained or fine-grained level view features leads to a significant decrease in model performance, particularly evident in the Twitter-2015 dataset. The accuracy decrease can be around 3%. Analyzing data from different levels and perspectives allows for the expression of diverse features, providing varied information. The combination of these features facilitates mutual complementation and enhancement, enabling a more comprehensive extraction of effective information. This, in turn, expands the amount of information provided by the data, addressing challenges related to short text and insufficient data in the dataset.
Second, the complete model exhibits an accuracy improvement of 2.41% and 2.02% on the two Twitter datasets compared to the model without the dynamic gating self-attention mechanism. This suggests that the inclusion of the gating mechanism dynamically adjusts the computation of attentional weights, prioritizing information related to aspectual words. Consequently, the gating mechanism addresses redundant information and noise in the data.
Finally, the removal of the TFH mechanism has a noticeable impact on the model performance compared to the complete model. On the two Twitter datasets, accuracy is enhanced by 1.26% and 1.70%, respectively. This indicates that the TFH mechanism effectively taps into complex interactions among different features, ensuring comprehensive integration and further improving sentiment classification effectiveness.
In summary, MVDFM exhibits inherent flexibility by extracting multi-grained view features from text and images through both coarse-grained and fine-grained perspectives. When dealing with large datasets, the model can adjust the granularity of feature extraction according to needs, thereby optimizing the use of computational resources while maintaining performance. Secondly, the design of the dynamic gated self-attention mechanism enables the model to dynamically focus on key information in the input data, reducing the processing of non-key information and thus improving computational efficiency. This mechanism is particularly important when handling real-time sentiment analysis tasks, as it allows the model to quickly respond and process new data inputs. Furthermore, the triple-view decomposition high-order pooling mechanism proposed by MVDFM further enhances the model’s computational efficiency through two-stage dynamic fusion of multi-grained view features. This mechanism not only helps capture complex relationships in the input data but also reduces computational load through decomposition and pooling operations, thereby alleviating potential computational bottlenecks.
Analysis of college students’ mental health and emotion management
To comprehensively and accurately capture the emotional features of text and image data on social networking platforms, we adopt a combined coarse-grained and fine-grained perspective for vectorized encoding. Coarse-grained analysis focuses on grasping overall emotional trends, such as the general distribution of positive, negative, or neutral sentiments. In contrast, fine-grained analysis delves into the details of text and image elements, identifying subtle cues like specific emotional vocabulary, emojis, and color schemes. This dual strategy significantly enhances the model’s information expression capabilities, laying a solid foundation for subsequent emotional analysis. Furthermore, we extract multi-grained view features from text and images and innovatively design a dynamic gated self-attention mechanism. This mechanism effectively filters out noise information, such as irrelevant vocabulary or blurred image areas, at the fine-grained level, ensuring high-quality and accurate feature extraction. This step is crucial for precisely identifying the complex emotions expressed by university students in graphic-text comments, providing a reliable basis for subsequent mental health assessments. To deeply explore the complementarity and consistency among different granularity views, we propose a view decomposition high-order pooling mechanism. This mechanism integrates multi-grained view features through a two-stage dynamic fusion strategy, ultimately outputting the emotional polarity of target aspect terms. This method not only improves the performance of graphic-text emotion prediction but, more importantly, it accurately analyzes the emotional information contained in the graphic-text content posted by university students on social networking sites, predicting their emotional states and indirectly reflecting their mental health conditions. For example, by analyzing frequently appearing sad or negative graphic-text content, potential mental health risks can be promptly identified, enabling early interventions.

Emotional network analysis under affective prediction in social networking sites
Enhancing the performance of graphic emotion prediction allows for effective analysis of emotional information embedded in the text and images of college students when they make graphic comments on social networking sites. This predictive capability aids in understanding and detecting their mental health status. Specifically:
Detecting Psychological Distress: Text and image content on social networking sites serve as indicators of the emotional state of college students. Frequent posting of sad or negative texts and images over time may signal psychological stress or emotional problems. Emotion prediction can help identify potential issues and enable timely intervention and support.
Self-Regulation of Emotions: Graphic emotion prediction assists college students in understanding and regulating their emotions. Analyzing their text and image content allows students to recognize mood changes and emotional states. This self-awareness facilitates better emotional management, including the use of positive psychological suggestions, emotional catharsis, or seeking professional psychological counseling.
During the experimental exploration presented in Fig. 6 , it was observed that social networking site dependence in college students falls into different qualitative potential categories and is influenced by factors such as social rejection, social networking site monitoring, and the fear of missing out. Additionally, social rejection is positively related to social website monitoring and fear of missing out. Social networking site monitoring mediates between social exclusion and social networking site dependence, while misplaced fear mediates between social exclusion and social networking site dependence. Furthermore, a chain mediation effect is observed, with social networking site monitoring and misplaced fear acting as mediators between social exclusion and social networking site dependence.
Interactions on social networking sites play a crucial role in enabling college students to form communities of support and mutual aid. Through communication and sharing with others who share similar experiences or feelings, students can garner emotional support and empathy, contributing to their mental health and emotional regulation. However, it is essential to recognize that graphic emotion prediction is not flawless. Factors such as individual language expression ability and cultural background can impact the accuracy of prediction results. Therefore, when employing this technique, it is crucial to consider various factors and integrate it with other mental health assessment methods to ensure accuracy and reliability.
In the field of law, particularly in litigation, contract review, legal research, and other areas, a vast amount of textual and image data requires analysis. Vectorizing and encoding text and image data from social networks through both coarse-grained and fine-grained perspectives can significantly enhance the digital processing capabilities of legal texts. This not only facilitates rapid retrieval and classification of legal documents but also enables automatic extraction of key information through machine learning algorithms, such as critical obligations in contract clauses and authenticity assessments of evidentiary materials, thereby enhancing the efficiency and accuracy of legal practice. Furthermore, this paper extracts multi-grained view features from text and images and designs a dynamic gated self-attention mechanism, which means that in the legal field, it becomes possible to more precisely identify and analyze key information in legal documents. The proposed Triple-view Factorized High-order Pooling (TFH) mechanism holds broad application prospects in the process of legal decision-making. By fusing multi-view information across different granularities, the model can more comprehensively understand the complexity of legal issues and provide more accurate legal advice. Combining these innovations, the prospects for Legal Master (LL.M.) graduates become broader and more diversified. On the one hand, with the continuous development of artificial intelligence and big data technologies, LL.M. graduates will have the opportunity to master more advanced digital tools and methods, enhancing their competitiveness and professional expertise in legal practice. On the other hand, LL.M. graduates can also leverage these technologies to conduct interdisciplinary research, such as in the cross-fields of law and computer science, law and data science, contributing to the development and innovation of the legal industry.
Moreover, with the advent of globalization and the Internet era, cross-border legal affairs are increasing, placing higher demands on legal talents with international perspectives and cross-cultural communication abilities. Therefore, during their training, LL.M. students should focus on international education and the accumulation of practical experience to adapt to the demands of the global legal market.
This paper introduces a dynamic fusion model for multimodal aspect-level sentiment analysis, particularly focusing on graphic information from social networking sites. The model considers multimodal data from coarse-grained and fine-grained perspectives, extending various text and image view features. This expansion enhances the information content in multimodal data, ensuring more robust feature extraction for improved sentiment prediction, even in scenarios with limited multimodal data samples. To filter out noise unrelated to the target aspect word, a dynamic gating self-attention mechanism is proposed. This mechanism dynamically adjusts weight assignments for each word or region based on target aspect word information, effectively reducing noise in the data. Furthermore, a three-view decomposition higher-order pooling mechanism is employed for final feature fusion. This mechanism captures complex interactions among features from different viewpoints, achieving effective fusion through multiple expansion and compression stages. Experimental results demonstrate that the proposed model outperforms current baseline methods on the Twitter-2015 and Twitter-2017 datasets. The analysis indicates a strong correlation between graphical sentiment prediction and college students’ mental health and emotion management regulation on social networking sites. The technique presented in this paper not only helps identify potential psychological problems in college students, providing timely intervention and support, but also assists students in better understanding and regulating their emotions, thereby promoting their mental health and emotion management skills.
Data availability
No datasets were generated or analysed during the current study.
Loriaux S. Kant on Social Justice: poverty, dependence, and Depersonification[J]. South J Philos. 2023;61(1):233–56.
Article Google Scholar
Kreya M, Wok S. Social Media Addiction and Its Influence on Mental Health among University Students in Cambodia: Beyond Cultivation Theory[C]//2nd Kuala Lumpur International Multidisciplinary Academic Conference (KLIMAC2020). 2021.
Murtadha A, Pan S, Wen B et al. BERT-ASC: Auxiliary-Sentence Construction for Implicit Aspect Learning in sentiment Analysis[J]. 2022. https://doi.org/10.48550/arXiv.2203.11702
Rizal A, Soemarno S, Mulawarman AD, et al. Tourism marketing strategy to increase tourist visit to Bawean Island, Gresik, East Java[J]. Am J Tour Manag. 2015;4(3):54–60.
Google Scholar
Boehm KM, Khosravi P, Vanguri R, et al. Harnessing multimodal data integration to advance precision oncology[J]. Nat Rev Cancer. 2022;22(2):114–26.
Article PubMed Google Scholar
Priego-Parra BA, Triana-Romero A, Pinto-Gálvez SM, et al. Anxiety, depression, attitudes, and internet addiction during the initial phase of the 2019 coronavirus disease (COVID-19) epidemic: a cross-sectional study in México[J]. MedRxiv. 2020: 2020.05. 10.20095844.
Liu Z, Lin X, Wang X, et al. Self-regulation deficiency in predicting problematic use of mobile social networking apps: the role of media dependency[J]. Decis Sci. 2022;53(5):827–55.
Tsoy D, Tirasawasdichai T, Kurpayanidi KI. Role of social media in shaping public risk perception during COVID-19 pandemic: a theoretical review[J]. Int J Manage Sci Bus Adm. 2021;7(2):35–41.
Henzel V, Håkansson A. Hooked on virtual social life. Problematic social media use and associations with mental distress and addictive disorders[J]. PLoS ONE. 2021;16(4):e0248406.
Article PubMed PubMed Central Google Scholar
Cataldo I, Lepri B, Neoh MJY, et al. Social media usage and development of psychiatric disorders in childhood and adolescence: a review[J]. Front Psychiatry. 2021;11:508595.
Shin D, Zhou S. A Value and Diversity-Aware News Recommendation systems: can algorithmic gatekeeping nudge readers to View Diverse News?[J]. Journalism & Mass Communication Quarterly; 2024. p. 10776990241246680.
Shin D, Koerber A, Lim JS. Impact of misinformation from generative AI on user information processing: how people understand misinformation from generative AI[J]. New Media & Society; 2024. p. 14614448241234040.
Shin D, Jitkajornwanich K, Lim JS et al. Debiasing misinformation: how do people diagnose health recommendations from AI?[J]. Online Information Review, 2024 (ahead-of-print).
Ma N, Yin H, Wang K. Prediction of the remaining useful life of supercapacitors at different temperatures based on improved long short-term memory[J]. Energies. 2023;16(14):5240.
Al Mehedi MA, Amur A, Metcalf J, et al. Predicting the performance of green stormwater infrastructure using multivariate long short-term memory (LSTM) neural network[J]. J Hydrol. 2023;625:130076.
Guo MH, Xu TX, Liu JJ, et al. Attention mechanisms in computer vision: a survey[J]. Comput Visual Media. 2022;8(3):331–68.
Zheng W, Zhang S, Yang C, et al. Lightweight multilayer interactive attention network for aspect-based sentiment analysis[J]. Connection Sci. 2023;35(1):2189119.
Bhatti UA, Tang H, Wu G, et al. Deep learning with graph convolutional networks: an overview and latest applications in computational intelligence. Int J Intelligent Syst. 2023;2023:1–28.
Zhao Q, Yang F, An D, et al. Modeling structured dependency tree with Graph Convolutional Networks for aspect-level sentiment Classification[J]. Sensors. 2024;24(2):418.
Xu N, Mao W, Chen G. Multi-interactive memory network for aspect based multimodal sentiment analysis. Proceed AAAI Conference Artificial Intelligence. 2019;33(01):371–8.
Singh G, Ghosh S, Verma A, et al. Standardizing Distress Analysis: Emotion-Driven Distress Identification and Cause Extraction (DICE) in Multimodal Online Posts[C]//Proceedings of the 2023 Conference on Empirical Methods in Natural Language Processing. 2023:4517–4532.
Ghosh S, Ekbal A, Bhattacharyya P. Natural language processing and sentiment analysis: perspectives from computational intelligence[M]//Computational Intelligence Applications for Text and Sentiment Data Analysis. Academic; 2023. pp. 17–47.
Ghosh S, Varshney D, Ekbal A, et al. Context and knowledge enriched transformer framework for emotion recognition in conversations[C]//2021 International joint conference on neural networks (IJCNN). IEEE, 2021: 1–8.
Zhan Z, Xu B. Analyzing review sentiments and product images by parallel deep nets for personalized recommendation[J]. Inf Process Manag. 2023;60(1):103166.
Fang X, Liu D, Zhou P, et al. Hierarchical local-global transformer for temporal sentence grounding[J]. IEEE Transactions on Multimedia; 2023.
Wang R, Jo W, Zhao D, et al. Husformer: a multi-modal transformer for multi-modal human state recognition[J]. IEEE Transactions on Cognitive and Developmental Systems; 2024.
Irfan M, Ayub N, Ahmed QA, et al. AQSA: aspect-based quality sentiment analysis for multi-labeling with Improved ResNet. Hybrid Algorithm[J] Electron. 2023;12(6):1298.
Hoang DH, Tran AK, Dang DNM, et al. RBBA: ResNet-BERT-Bahdanau Attention for Image Caption Generator[C]//2023 14th International Conference on Information and Communication Technology Convergence (ICTC). IEEE; 2023. p. 430–5.
Download references
Acknowledgements
The author thanks the anonymous reviewers whose comments and suggestions helped to improve the manuscript.
The work received no funding.
Author information
Authors and affiliations.
School of Business, Xinyang Normal University, Xinyang, Henan, 464000, China
Yuehua Han & Zhifen Xu
You can also search for this author in PubMed Google Scholar
Contributions
Yuehua Han was responsible for study conception, design and interpretation of results. Zhifen Xu was responsible for data collection, analysis and the project administration. Yuehua Han and Zhifen Xu were responsible for draft manuscript preparation. All authors reviewed the results and approved the final version of the manuscript.
Corresponding author
Correspondence to Yuehua Han .
Ethics declarations
Ethics approval and consent to participate.
This article does not contain any studies with human participants performed by any of the authors.
Consent for publication
Not applicable.
Competing interests
The authors declare no competing interests.
Additional information
Publisher’s note.
Springer Nature remains neutral with regard to jurisdictional claims in published maps and institutional affiliations.
Rights and permissions
Open Access This article is licensed under a Creative Commons Attribution 4.0 International License, which permits use, sharing, adaptation, distribution and reproduction in any medium or format, as long as you give appropriate credit to the original author(s) and the source, provide a link to the Creative Commons licence, and indicate if changes were made. The images or other third party material in this article are included in the article's Creative Commons licence, unless indicated otherwise in a credit line to the material. If material is not included in the article's Creative Commons licence and your intended use is not permitted by statutory regulation or exceeds the permitted use, you will need to obtain permission directly from the copyright holder. To view a copy of this licence, visit http://creativecommons.org/licenses/by/4.0/ .
Reprints and permissions
About this article
Cite this article.
Han, Y., Xu, Z. Fostering college students’ mental well-being: the impact of social networking site utilization on emotion management and regulation. BMC Psychol 12 , 681 (2024). https://doi.org/10.1186/s40359-024-02186-7
Download citation
Received : 22 February 2024
Accepted : 11 November 2024
Published : 21 November 2024
DOI : https://doi.org/10.1186/s40359-024-02186-7
Share this article
Anyone you share the following link with will be able to read this content:
Sorry, a shareable link is not currently available for this article.
Provided by the Springer Nature SharedIt content-sharing initiative
- Social networking site
- Mental health
- Emotion management
- Multimodal data
BMC Psychology
ISSN: 2050-7283
- General enquiries: [email protected]
Advertisement
Social Media Addiction and Its Impact on College Students' Academic Performance: The Mediating Role of Stress
- Regular Article
- Published: 01 November 2021
- Volume 32 , pages 81–90, ( 2023 )
Cite this article
- Lei Zhao ORCID: orcid.org/0000-0002-7337-3065 1 , 2
8633 Accesses
21 Citations
1 Altmetric
Explore all metrics
Social media use can bring negative effects to college students, such as social media addiction (SMA) and decline in academic performance. SMA may increase the perceived stress level of college students, and stress has a negative impact on academic performance, but this potential mediating role of stress has not been verified in existing studies. In this paper, a research model was developed to investigate the antecedent variables of SMA, and the relationship between SMA, stress and academic performance. With the data of 372 Chinese college students (mean age 21.3, 42.5% males), Partial Least Squares, Structural Equation Model was adopted to evaluate measurement model and structural model. The results show that use intensity is an important predictor of SMA, and both SMA and stress have a negative impact on college students’ academic performance. In addition, we further confirmed that stress plays a mediating role in the relationship between SMA and college students’ academic performance.
This is a preview of subscription content, log in via an institution to check access.
Access this article
Subscribe and save.
- Get 10 units per month
- Download Article/Chapter or eBook
- 1 Unit = 1 Article or 1 Chapter
- Cancel anytime
Price includes VAT (Russian Federation)
Instant access to the full article PDF.
Rent this article via DeepDyve
Institutional subscriptions
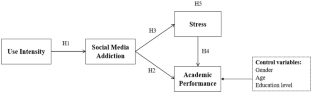
Similar content being viewed by others
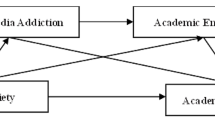
Social media addiction and academic engagement as serial mediators between social anxiety and academic performance among college students
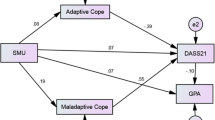
Psychological distress, social media use, and academic performance of medical students: the mediating role of coping style
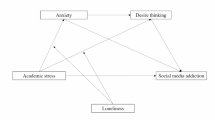
From academia to addiction: understanding the mechanism behind how academic stress fuels social media addiction in PhD students
Al-Yafi, K., El-Masri, M., & Tsai, R. (2018). The effects of using social network sites on academic performance: The case of Qatar. Journal of Enterprise Information Management , 31 (3), 446–462. https://doi.org/10.1108/JEIM-08-2017-0118 .
Andreassen, C. S., & Pallesen, S. (2014). Social network site addiction—an overview. Current Pharmaceutical Design, 20 , 1–9.
Article Google Scholar
Andreassen, C. S., Pallesen, S., & Griffiths, M. D. (2017). The relationship between addictive use of social media, narcissism, and self-esteem: Findings from a large national survey. Addictive Behaviors, 64 , 287–293. https://doi.org/10.1016/j.addbeh.2016.03.006
Andreassen, C. S., Torsheim, T., Brunborg, G. S., & Pallesen, S. (2012). Development of a facebook addiction scale. Psychological Reports, 110 (2), 501–517.
Argyris, Y. E., & Xu, J. (2016). Enhancing self-efficacy for career development in Facebook. Computers in Human Behavior, 55 (2), 921–931. https://doi.org/10.1016/j.chb.2015.10.023
Arnett, J. J. (2000). Emerging adulthood: A theory of development from the late teens through the twenties. American Psychologist, 55 (5), 469–480. https://doi.org/10.1016/j.chb.2015.10.023
Bano, S., Cisheng, W., Khan, A., & Khan, N. A. (2019). WhatsApp use and student’s psychological well-being: Role of social capital and social integration. Children and Youth Services Review, 103 , 200–208. https://doi.org/10.1016/j.childyouth.2019.06.002
Barclay, D., Higgins, C., & Thompson, R. (1995). The partial least squares (PLS) approach to causal modeling: Personal computer adoption and use as an illustration. Technology Studies, 2 (2), 285–309.
Google Scholar
Bijari, B., Javadinia, S. A., Erfanian, M., Abedini, M. R., & Abassi, A. (2013). The impact of virtual social networks on students’ academic achievement in Birjand University of Medical Sciences in East Iran. Procedia Social and Behavioural Sciences, 83 , 103–106. https://doi.org/10.1016/j.sbspro.2013.06.020
Błachnio, A., Przepiorka, A., & Pantic, I. (2016). Association between facebook addiction, self-esteem and life satisfaction: A cross-sectional study. Computers in Human Behavior, 55 , 701–705. https://doi.org/10.1016/j.chb.2015.10.026
Brailovskaia, J., Rohmann, E., Bierhoff, H. W., Schillack, H., & Margraf, J. (2019). The relationship between daily stress, social support and Facebook addiction disorder. Psychiatry Research, 276 , 167–174. https://doi.org/10.1016/j.psychres.2019.05.014
Brailovskaia, J., Schillack, H., & Margraf, J. (2018). Facebook addiction disorder in Germany. Cyberpsychology, Behavior, and Social Networking, 21 (7), 450–456. https://doi.org/10.1089/cyber.2018.0140
Buil, I., Martínez, E., & Matute, J. (2016). From internal brand management to organizational citizenship behaviors: Evidence from frontline employees in the hotel industry. Tourism Management, 57 (12), 256–271. https://doi.org/10.1016/j.tourman.2016.06.009
Busalim, A. H., Masrom, M., & Zakaria, W. N. B. W. (2019). The impact of Facebook addiction and self-esteem on students’ academic performance: A multi-group analysis. Computers & Education, 142 , 103651. https://doi.org/10.1016/j.compedu.2019.103651
Carolus, A., Binder, J. F., Muench, R., Schmidt, C., Schneider, F., & Buglass, S. L. (2019). Smartphones as digital companions: Characterizing the relationship between users and their phones. New Media & Society, 21 , 914–938. https://doi.org/10.1177/1461444818817074
China Internet Network Information Center (CNNIC), 2020. The 44th Statistical Report on Internet Development in China (in Chinese). Retrieved August 28, 2020, from http://www.cac.gov.cn/2020-08/30/c_1124938750.htm
Cohen, S., Kamarck, T., & Mermelstein, R. (1983). A global measure of perceived stress. Journal of Health and Social Behavior, 24 (4), 385–396. https://doi.org/10.2307/2136404
Dendle, C., Baulch, J., Pellicano, R., Hay, M., Lichtwark, I., Ayoub, S., & Horne, K. (2018). Medical student psychological distress and academic performance. Medical Teacher . https://doi.org/10.4236/ce.2018.99099
Doleck, T., & Lajoie, S. (2018). Social networking and academic performance: A review. Education and Information Technologies, 23 (1), 435–465. https://doi.org/10.1007/s10639-017-9612-3
Griffths, M. D., Kuss, D. J., & Demetrovics, Z. (2014). Social networking addictions: An overview of preliminary findings. Behavioral Addictions Criteria, Evidence, and Treatment , 119–141. https://doi.org/10.1016/B978-0-12-407724-9.00006-9 .
Hair, J. F. J., Hult, G. T. M., Ringle, C., & Sarstedt, M. (2016). A primer on partial least squares structural equation modeling (PLS-SEM) . Sage Publication.
Hair, J. F., Risher, J. J., Sarstedt, M., & Ringle, C. M. (2019). When to use and how to report the results of pls-sem. European Business Review, 31 (1), 2–24. https://doi.org/10.1108/EBR-11-2018-0203
Hayes, A. F. (2009). Beyond Baron and Kenny: Statistical mediation analysis in the new millennium. Communication Monographs, 76 (4), 408–420. https://doi.org/10.1080/03637750903310360
Hormes, J. M., Kearns, B., & Timko, C. A. (2014). Craving Facebook? Behavioral addiction to online social networking and its association with emotion regulation deficits. Addiction, 109 (12), 2079–2088. https://doi.org/10.1111/add.12713
Hsiao, K. L., Shu, Y., & Huang, T. C. (2017). Exploring the effect of compulsive social app usage on technostress and academic performance: Perspectives from personality traits. Telematics and Informatics, 34 , 679–690. https://doi.org/10.1016/j.tele.2016.11.001
Jie, T., Yizhen, Y., Yukai, D., Ying, M., Dongying, Z., & Jiaji, W. (2014). Addictive behaviors prevalence of internet addiction and its association with stressful life events and psychological symptoms among adolescent internet users. Addictive Behaviors, 39 (3), 744–747. https://doi.org/10.1016/j.adolescence.2016.07.012
Juana, I., Serafín, B., Jesús, L., et al. (2020). Effectiveness of music therapy and progressive muscle relaxation in reducing stress before exams and improving academic performance in nursing students: A randomized trial. Nurse Education Today, 84 , 104217. https://doi.org/10.1016/j.nedt.2019.104217
Kimberly, Y. (2009). Facebook addiction disorder . The Center for Online Addiction.
Lau, W. W. F. (2017). Effects of social media usage and social media multitasking on the academic performance of university students. Computers in Human Behavior, 68 (3), 286–291. https://doi.org/10.1016/j.chb.2016.11.043
Lazarus, R. S., & Folkman, S. (1984). Stress, appraisal, and coping . Springer.
Lee, W. W. S. (2017). Relationships among grit, academic performance, perceived academic failure, and stress in associate degree students. Journal of Adolescence, 60 , 148–152. https://doi.org/10.1016/j.adolescence.2017.08.006
Leung, L. (2007). Stressful life events, motives for Internet use, and social support among digital kids. CyberPsychology & Behavior, 10 (2), 204–214. https://doi.org/10.1089/cpb.2006.9967
Liang, C., Gu, D., Tao, F., Jain, H. K., Zhao, Y., & Ding, B. (2017). Influence of mechanism of patient-accessible hospital information system implementation on doctor-patient relationships: A service fairness perspective. Information & Management, 54 (1), 57–72.
Lim, K., & Jeoung, Y. S. (2010). Understanding major factors in taking Internet based lectures for the national college entrance exam according to academic performance by case studies. The Journal of the Korea Contents Association, 10 (12), 477–491. https://doi.org/10.5392/JKCA.2010.10.12.477
Lim, Y., & Nam, S.-J. (2016). Time spent on the Internet by multicultural adolescents in Korea. Asia Pacific Journal of Education, 37 (1), 55–68. https://doi.org/10.1080/02188791.2016.1169994
Lin, C. L., Jin, Y. Q., Zhao, Q., Yu, S. W., & Su, Y. S. (2021). Factors influence students’ switching behavior to online learning under covid-19 pandemic: A push-pull-mooring model perspective. The Asia-Pacific Education Researcher, 30 (3), 229–245. https://doi.org/10.1007/s40299-021-00570-0
Lovibond, P. F., & Lovibond, S. H. (1995). The structure of negative emotional states: Comparison of the depression anxiety stress scales (DASS) with the Beck depression and anxiety inventories. Behaviour Research and Therapy, 33 (3), 335–343. https://doi.org/10.1016/j.jad.2015.12.004
Marino, C., Gini, G., Vieno, A., & Spada, M. M. (2018). A comprehensive meta-analysis on problematic Facebook use. Computers in Human Behavior, 83 , 262–277. https://doi.org/10.1016/j.chb.2018.02.009
Masood, A., Luqman, A., Feng, Y., & Ali, A. (2020). Adverse consequences of excessive social networking site use on academic performance: Explaining underlying mechanism from stress perspective. Computers in Human Behavior . https://doi.org/10.1016/j.chb.2020.106476
McDaniel, B. (2015). ‘Technoference’: Everyday intrusions and inter-ruptions of technology in couple and family relationships. In C. Bruess Içinde (Ed.), Family communication in the age of digital and social media. Peter Lang Publishing.
Nayak, J. K. (2018). Relationship among smartphone usage, addiction, academic performance and the moderating role of gender: A study of higher education students in India. Computers and Education, 123 (5), 164–173. https://doi.org/10.1016/j.compedu.2018.05.007
O'Brien, L. (2012). Six ways to use social media in education. Retrieved from https://cit.duke.edu/blog/2012/04/six-ways-to-use-social-media-in-education/
Orosz, G., Istvan, T., & Beata, B. (2016). Four facets of Facebook intensity—the development of the multidimensional facebook intensity scale. Personality and Individual Differences, 100 , 95–104. https://doi.org/10.1016/j.paid.2015.11.038
Oye, N. D., Adam, M. H., & Nor Zairah, A. R. (2012). Model of perceived influence of academic performance using social networking. International Journal of Computers and Technology, 2 (2), 24–29. https://doi.org/10.24297/ijct.v2i1.2612
Pang, H. (2018). How does time spent on WeChat bolster subjective well-being through social integration and social capital? Telematics and Informatics, 25 , 2147–2156. https://doi.org/10.1016/j.tele.2018.07.015
Paul, J. A., Baker, H. M., & Cochran, J. D. (2012). Effect of online social networking on student academic performance. Computers in Human Behavior, 28 (6), 2117–2127. https://doi.org/10.1016/j.chb.2012.06.016
Peng, Y., & Li, J. (2021). The effect of customer education on service innovation satisfaction: The mediating role of customer participation. Journal of Hospitality and Tourism Management, 47 (5), 326–334. https://doi.org/10.1016/j.jhtm.2020.12.014
Phu, B., & Gow, A. J. (2019). Facebook use and its association with subjective happiness and loneliness. Computers in Human Behavior, 92 , 151–159. https://doi.org/10.1016/j.chb.2018.11.020
Podsakoff, P. M., MacKenzie, S. B., Lee, J.-Y., & Podsakoff, N. P. (2003). Common method biases in behavioral research: A critical review of the literature and recommended remedies. Journal of Applied Psychology , 88 (5), 879–903. https://doi.org/10.1037/0021-9010.88.5.879 .
Prato, C. A., & Yucha, C. B. (2013). Biofeedback-assisted relaxation training to decrease test anxiety in nursing students. Nursing Education Perspectives, 34 (2), 76–81. https://doi.org/10.1097/00024776-201303000-00003
Rouis, S. (2012). Impact of cognitive absorption on facebook on students’ achievement. Cyberpsychology, Behavior, and Social Networking, 15 (6), 296–303. https://doi.org/10.1089/cyber.2011.0390
Ryan, T., Chester, A., Reece, J., & Xenos, S. (2014). The uses and abuses of facebook: A review of facebook addiction. Journal of Behavioral Addictions, 3 (3), 133–148. https://doi.org/10.1556/JBA.3.2014.016
Sandra, K., Dawans, B. V., Heinrichs, M., & Fuchs, R. (2013). Does the level of physical exercise affect physiological and psychological responses to psychosocial stress in women. Psychology of Sport and Exercise, 14 , 266–274. https://doi.org/10.1016/j.psychsport.2012.11.003
Shaw, M., & Black, D. W. (2008). Internet addiction . Springer.
Shi, C., Yu, L., Wang, N., Cheng, B., & Cao, X. (2020). Effects of social media overload on academic performance: A stressor–strain–outcome perspective. Asian Journal of Communication, 30 (2), 1–19. https://doi.org/10.1080/01292986.2020.1748073
Statista (2020). Number of social media users worldwide from 2010 to 2021 (in billions). Retrieved from https://www.statista.com/statistics/278414/number-of-worldwide-social-network-users/
Tang, J. H., Chen, M. C., Yang, C. Y., Chung, T. Y., & Lee, Y. A. (2016). Personality traits, interpersonal relationships, online social support, and Facebook addiction. Telematics and Informatics, 33 (1), 102–108. https://doi.org/10.1016/j.tele.2015.06.003
Wang, J. L., Wang, H. Z., Gaskin, J., & Wang, L. H. (2015). The role of stress and motivation in problematic smartphone use among college students. Computers in Human Behavior, 53 , 181–188. https://doi.org/10.1016/j.chb.2015.07.005
Wartberg, L., Kriston, L., & Thomasius, R. (2020). Internet gaming disorder and problematic social media use in a representative sample of German adolescents: Prevalence estimates, comorbid depressive symptoms and related psychosocial aspects. Computers in Human Behavior, 103 , 31–36. https://doi.org/10.1016/j.chb.2019.09.014
Xu, X. A., Xue, K. N., Wang, L. L., Gursoy, D., & Song, Z. B. (2021). Effects of customer-to-customer social interactions in virtual travel communities on brand attachment: the mediating role of social well-being. Tourism Management Perspectives, 38 , 100790. https://doi.org/10.1016/j.tmp.2021.100790
Zhao, L. (2021). The impact of social media use types and social media addiction on subjective well-being of college students: A comparative analysis of addicted and non-addicted students. Computers in Human Behavior Reports, 4 (2), 100122. https://doi.org/10.1016/j.chbr.2021.100122
Zhao, L., Liang, C., & Gu, D. (2021). Mobile social media use and trailing parents’ life satisfaction: Social capital and social integration perspective. International Journal of Aging and Human Development, 92 (3), 383–405. https://doi.org/10.1177/0091415020905549
Download references
Acknowledgements
This study is supported by the Planning Subject for the 14th Five-year Plan of National Education Sciences (Grant No. EIA210425).
Author information
Authors and affiliations.
School of Management, Hefei University of Technology, Hefei, 230009, Anhui, China
Wendian College, Anhui University, Hefei, 230601, Anhui, China
You can also search for this author in PubMed Google Scholar
Corresponding author
Correspondence to Lei Zhao .
Ethics declarations
Conflict of interest.
The authors declared no potential conflict of interest with respect to the research, authorship, and/or publication of this article.
Additional information
Publisher's note.
Springer Nature remains neutral with regard to jurisdictional claims in published maps and institutional affiliations.
Rights and permissions
Reprints and permissions
About this article
Zhao, L. Social Media Addiction and Its Impact on College Students' Academic Performance: The Mediating Role of Stress. Asia-Pacific Edu Res 32 , 81–90 (2023). https://doi.org/10.1007/s40299-021-00635-0
Download citation
Accepted : 21 October 2021
Published : 01 November 2021
Issue Date : February 2023
DOI : https://doi.org/10.1007/s40299-021-00635-0
Share this article
Anyone you share the following link with will be able to read this content:
Sorry, a shareable link is not currently available for this article.
Provided by the Springer Nature SharedIt content-sharing initiative
- Use intensity
- Social media addiction
- Academic performance
- Find a journal
- Publish with us
- Track your research

IMAGES
VIDEO
COMMENTS
This research paper explores the effects of social media on college students' academic performance, mental health, and productivity. The study includes a sample size of 57 college...
Social media has a strong influence on the everyday lives of college students. A particular question of advanced research interest is whether social media also play a role when attending class.
By combining a review of existing literature with surveys and interviews, we seek to gain a comprehensive understanding of how social media influences students' time management, academic...
Based on the stressor–strain–outcome (SSO) model and the theory of compensatory internet use (TCIU), we developed a research model to study the causes of social media overload and its impact on college students’ academic performance during the COVID-19 pandemic.
The current study explores the students’ behavioral intention to use social media and actual social media use in higher education, specifically the perception of their academic...
These researchers investigated the emotional effects of social media in higher education and found that the socially supportive role of social media was overshadowed in the long run in university students’ lives and, instead, fed into their perceived depression, anxiety, and stress.
Survey responses were collected from 659 undergraduate and graduate students to determine the relationship between social media usage and overall academic performance, as well as explore if this relationship is moderated by attention (regulation of time/study environment) and motivation (effort regulation).
To address the research gap, the present research made an attempt to investigate the crucial role of social media in affecting students’ psychological (PWB) and subjective well-being (SWB) by testing the mediating role of self-esteem and online social support and the moderation effect of cyberbullying.
With the widespread proliferation of the Internet, social networking sites have increasingly become integrated into the daily lives of university students, leading to a growing reliance on these platforms. Several studies have suggested that this emotional dependence on social networking sites stems from unmet psychological needs. Meanwhile, social rejection has been identified as a prevalent ...
Firstly, the results show the negative effect of social media use on college students’ academic performance, strongly emphasizing the importance for college students to reduce the negative impact of SMA on their academic performance.