Have a language expert improve your writing
Run a free plagiarism check in 10 minutes, generate accurate citations for free.
- Knowledge Base
Methodology
- Descriptive Research | Definition, Types, Methods & Examples

Descriptive Research | Definition, Types, Methods & Examples
Published on May 15, 2019 by Shona McCombes . Revised on June 22, 2023.
Descriptive research aims to accurately and systematically describe a population, situation or phenomenon. It can answer what , where , when and how questions , but not why questions.
A descriptive research design can use a wide variety of research methods to investigate one or more variables . Unlike in experimental research , the researcher does not control or manipulate any of the variables, but only observes and measures them.
Table of contents
When to use a descriptive research design, descriptive research methods, other interesting articles.
Descriptive research is an appropriate choice when the research aim is to identify characteristics, frequencies, trends, and categories.
It is useful when not much is known yet about the topic or problem. Before you can research why something happens, you need to understand how, when and where it happens.
Descriptive research question examples
- How has the Amsterdam housing market changed over the past 20 years?
- Do customers of company X prefer product X or product Y?
- What are the main genetic, behavioural and morphological differences between European wildcats and domestic cats?
- What are the most popular online news sources among under-18s?
- How prevalent is disease A in population B?
Prevent plagiarism. Run a free check.
Descriptive research is usually defined as a type of quantitative research , though qualitative research can also be used for descriptive purposes. The research design should be carefully developed to ensure that the results are valid and reliable .
Survey research allows you to gather large volumes of data that can be analyzed for frequencies, averages and patterns. Common uses of surveys include:
- Describing the demographics of a country or region
- Gauging public opinion on political and social topics
- Evaluating satisfaction with a company’s products or an organization’s services
Observations
Observations allow you to gather data on behaviours and phenomena without having to rely on the honesty and accuracy of respondents. This method is often used by psychological, social and market researchers to understand how people act in real-life situations.
Observation of physical entities and phenomena is also an important part of research in the natural sciences. Before you can develop testable hypotheses , models or theories, it’s necessary to observe and systematically describe the subject under investigation.
Case studies
A case study can be used to describe the characteristics of a specific subject (such as a person, group, event or organization). Instead of gathering a large volume of data to identify patterns across time or location, case studies gather detailed data to identify the characteristics of a narrowly defined subject.
Rather than aiming to describe generalizable facts, case studies often focus on unusual or interesting cases that challenge assumptions, add complexity, or reveal something new about a research problem .
If you want to know more about statistics , methodology , or research bias , make sure to check out some of our other articles with explanations and examples.
- Normal distribution
- Degrees of freedom
- Null hypothesis
- Discourse analysis
- Control groups
- Mixed methods research
- Non-probability sampling
- Quantitative research
- Ecological validity
Research bias
- Rosenthal effect
- Implicit bias
- Cognitive bias
- Selection bias
- Negativity bias
- Status quo bias
Cite this Scribbr article
If you want to cite this source, you can copy and paste the citation or click the “Cite this Scribbr article” button to automatically add the citation to our free Citation Generator.
McCombes, S. (2023, June 22). Descriptive Research | Definition, Types, Methods & Examples. Scribbr. Retrieved June 11, 2024, from https://www.scribbr.com/methodology/descriptive-research/
Is this article helpful?
Shona McCombes
Other students also liked, what is quantitative research | definition, uses & methods, correlational research | when & how to use, descriptive statistics | definitions, types, examples, "i thought ai proofreading was useless but..".
I've been using Scribbr for years now and I know it's a service that won't disappoint. It does a good job spotting mistakes”
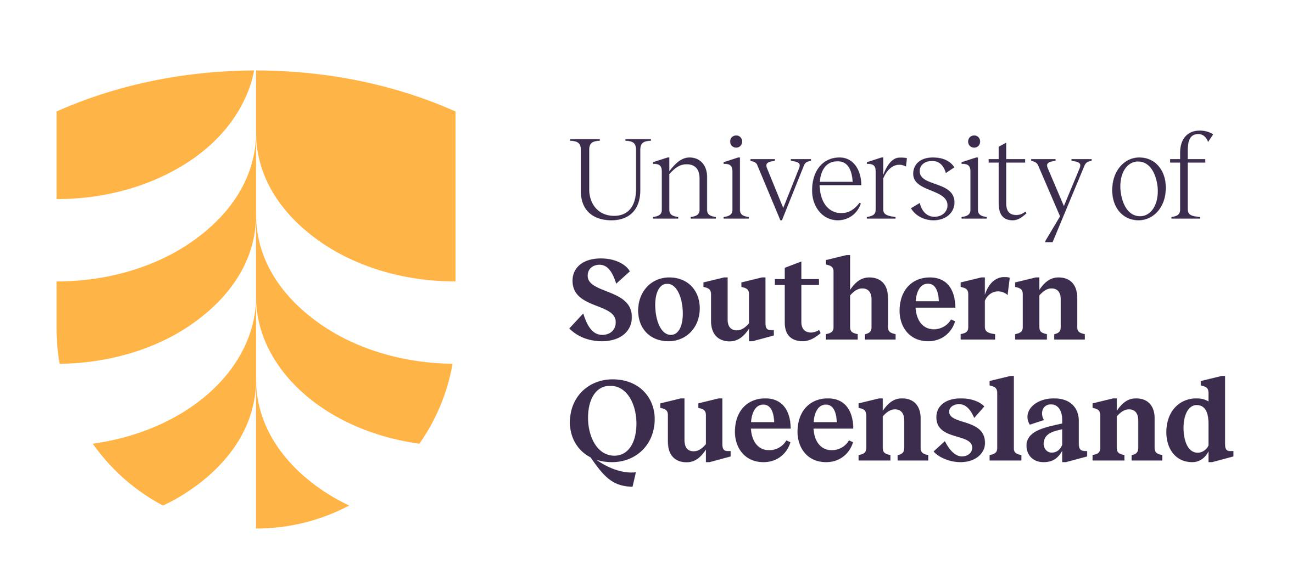
Want to create or adapt books like this? Learn more about how Pressbooks supports open publishing practices.
14 Quantitative analysis: Descriptive statistics
Numeric data collected in a research project can be analysed quantitatively using statistical tools in two different ways. Descriptive analysis refers to statistically describing, aggregating, and presenting the constructs of interest or associations between these constructs. Inferential analysis refers to the statistical testing of hypotheses (theory testing). In this chapter, we will examine statistical techniques used for descriptive analysis, and the next chapter will examine statistical techniques for inferential analysis. Much of today’s quantitative data analysis is conducted using software programs such as SPSS or SAS. Readers are advised to familiarise themselves with one of these programs for understanding the concepts described in this chapter.
Data preparation
In research projects, data may be collected from a variety of sources: postal surveys, interviews, pretest or posttest experimental data, observational data, and so forth. This data must be converted into a machine-readable, numeric format, such as in a spreadsheet or a text file, so that they can be analysed by computer programs like SPSS or SAS. Data preparation usually follows the following steps:
Data coding. Coding is the process of converting data into numeric format. A codebook should be created to guide the coding process. A codebook is a comprehensive document containing a detailed description of each variable in a research study, items or measures for that variable, the format of each item (numeric, text, etc.), the response scale for each item (i.e., whether it is measured on a nominal, ordinal, interval, or ratio scale, and whether this scale is a five-point, seven-point scale, etc.), and how to code each value into a numeric format. For instance, if we have a measurement item on a seven-point Likert scale with anchors ranging from ‘strongly disagree’ to ‘strongly agree’, we may code that item as 1 for strongly disagree, 4 for neutral, and 7 for strongly agree, with the intermediate anchors in between. Nominal data such as industry type can be coded in numeric form using a coding scheme such as: 1 for manufacturing, 2 for retailing, 3 for financial, 4 for healthcare, and so forth (of course, nominal data cannot be analysed statistically). Ratio scale data such as age, income, or test scores can be coded as entered by the respondent. Sometimes, data may need to be aggregated into a different form than the format used for data collection. For instance, if a survey measuring a construct such as ‘benefits of computers’ provided respondents with a checklist of benefits that they could select from, and respondents were encouraged to choose as many of those benefits as they wanted, then the total number of checked items could be used as an aggregate measure of benefits. Note that many other forms of data—such as interview transcripts—cannot be converted into a numeric format for statistical analysis. Codebooks are especially important for large complex studies involving many variables and measurement items, where the coding process is conducted by different people, to help the coding team code data in a consistent manner, and also to help others understand and interpret the coded data.
Data entry. Coded data can be entered into a spreadsheet, database, text file, or directly into a statistical program like SPSS. Most statistical programs provide a data editor for entering data. However, these programs store data in their own native format—e.g., SPSS stores data as .sav files—which makes it difficult to share that data with other statistical programs. Hence, it is often better to enter data into a spreadsheet or database where it can be reorganised as needed, shared across programs, and subsets of data can be extracted for analysis. Smaller data sets with less than 65,000 observations and 256 items can be stored in a spreadsheet created using a program such as Microsoft Excel, while larger datasets with millions of observations will require a database. Each observation can be entered as one row in the spreadsheet, and each measurement item can be represented as one column. Data should be checked for accuracy during and after entry via occasional spot checks on a set of items or observations. Furthermore, while entering data, the coder should watch out for obvious evidence of bad data, such as the respondent selecting the ‘strongly agree’ response to all items irrespective of content, including reverse-coded items. If so, such data can be entered but should be excluded from subsequent analysis.

Data transformation. Sometimes, it is necessary to transform data values before they can be meaningfully interpreted. For instance, reverse coded items—where items convey the opposite meaning of that of their underlying construct—should be reversed (e.g., in a 1-7 interval scale, 8 minus the observed value will reverse the value) before they can be compared or combined with items that are not reverse coded. Other kinds of transformations may include creating scale measures by adding individual scale items, creating a weighted index from a set of observed measures, and collapsing multiple values into fewer categories (e.g., collapsing incomes into income ranges).
Univariate analysis
Univariate analysis—or analysis of a single variable—refers to a set of statistical techniques that can describe the general properties of one variable. Univariate statistics include: frequency distribution, central tendency, and dispersion. The frequency distribution of a variable is a summary of the frequency—or percentages—of individual values or ranges of values for that variable. For instance, we can measure how many times a sample of respondents attend religious services—as a gauge of their ‘religiosity’—using a categorical scale: never, once per year, several times per year, about once a month, several times per month, several times per week, and an optional category for ‘did not answer’. If we count the number or percentage of observations within each category—except ‘did not answer’ which is really a missing value rather than a category—and display it in the form of a table, as shown in Figure 14.1, what we have is a frequency distribution. This distribution can also be depicted in the form of a bar chart, as shown on the right panel of Figure 14.1, with the horizontal axis representing each category of that variable and the vertical axis representing the frequency or percentage of observations within each category.
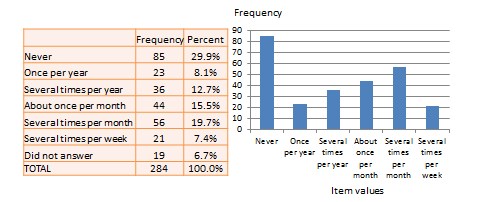
With very large samples, where observations are independent and random, the frequency distribution tends to follow a plot that looks like a bell-shaped curve—a smoothed bar chart of the frequency distribution—similar to that shown in Figure 14.2. Here most observations are clustered toward the centre of the range of values, with fewer and fewer observations clustered toward the extreme ends of the range. Such a curve is called a normal distribution .

Lastly, the mode is the most frequently occurring value in a distribution of values. In the previous example, the most frequently occurring value is 15, which is the mode of the above set of test scores. Note that any value that is estimated from a sample, such as mean, median, mode, or any of the later estimates are called a statistic .

Bivariate analysis
Bivariate analysis examines how two variables are related to one another. The most common bivariate statistic is the bivariate correlation —often, simply called ‘correlation’—which is a number between -1 and +1 denoting the strength of the relationship between two variables. Say that we wish to study how age is related to self-esteem in a sample of 20 respondents—i.e., as age increases, does self-esteem increase, decrease, or remain unchanged?. If self-esteem increases, then we have a positive correlation between the two variables, if self-esteem decreases, then we have a negative correlation, and if it remains the same, we have a zero correlation. To calculate the value of this correlation, consider the hypothetical dataset shown in Table 14.1.
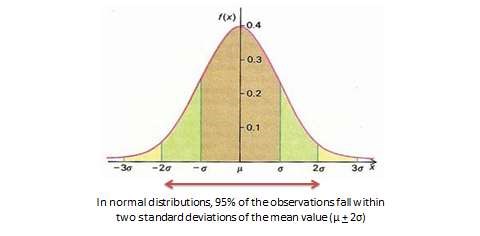
After computing bivariate correlation, researchers are often interested in knowing whether the correlation is significant (i.e., a real one) or caused by mere chance. Answering such a question would require testing the following hypothesis:
![Rendered by QuickLaTeX.com \[H_0:\quad r = 0 \]](https://usq.pressbooks.pub/app/uploads/quicklatex/quicklatex.com-74bb8e9e674477ba33a9eb751bfd254d_l3.png)
Social Science Research: Principles, Methods and Practices (Revised edition) Copyright © 2019 by Anol Bhattacherjee is licensed under a Creative Commons Attribution-NonCommercial-ShareAlike 4.0 International License , except where otherwise noted.
Share This Book
What Is Descriptive Analytics? A Complete Guide
When presented with new data, the first step a data analyst must take is always to understand what story it’s trying to tell. Data analytics is a complex beast, however, involving many different tools and analytical approaches. So which one should you use?
If you’re new to data and want to learn the basics, descriptive analytics is a good place to start. But what exactly is descriptive analytics, and how does it work? In this post, we’ll dive deep on the topic, answering all your questions, including:
- How does descriptive analytics work?
- How is descriptive analytics used?
- Advantages of descriptive analytics
- Disadvantages of descriptive analytics
- Descriptive analytics use cases
- Key takeaways
Ready to get the low-down on descriptive analytics? Let’s dive in.
1. How does descriptive analytics work?
Of all data analytics techniques , descriptive analytics is perhaps the most straightforward. It involves parsing (or breaking down) data and summarizing its main features and characteristics. In this way, descriptive analytics presents what has happened in the past without exploring why or how.
Because it is merely explanatory, descriptive analytics uses basic descriptive statistics. This includes measures of distribution (frequency or count), central tendency (mean, mode, and median), and variability (such as variance and standard deviation). Where relevant, it also measures the position of various data points, including the interquartile or percentile range.
Descriptive analytics often presents its findings using reports, pivot tables, and visualizations like histograms, line graphs, pie charts, and box and whisker plots . We won’t explore these further here, but you can learn more about descriptive statistics in this post .
2. How is descriptive analytics used?
Data analysts can use descriptive statistics to summarize more or less any type of data, although it helps to think of it as the first step in a more protracted process. That’s because while descriptive statistics may describe trends or patterns, it won’t dig deeper. For this, we need tools like diagnostic and predictive analytics. Nevertheless, descriptive analytics is exceptionally useful for introducing yourself to unknown data.
The following kinds of data can all be summarized using descriptive analytics:
- Financial statements
- Social media engagement
- Website traffic
- Scientific findings
- Weather reports
- Traffic data
The list goes on! Essentially, any data set can be summarized in one way or another, meaning descriptive analytics has an almost endless number of applications. We’ll explore these in more depth in section five. First, let’s look at some of the benefits and drawbacks of descriptive analytics.
3. Advantages of descriptive analytics
Although relatively simplistic as analytical approaches go, descriptive analytics nevertheless has many advantages. Descriptive analytics:
- Presents otherwise complex data in an easily digestible format.
- Provides a direct measure of the incidence of key data points.
- Is inexpensive and only requires basic mathematical skills to carry out.
- Is faster to carry out, especially with help from tools like Python or MS Excel.
- Relies on data that organizations already have access to, meaning there’s no need to source additional data.
- Looks at a complete population (rather than data sampling), making it considerably more accurate than inferential statistics .
But, of course, being so straightforward means descriptive analytics also has its limitations. Let’s explore some of these next.
4. Disadvantages of descriptive analytics
Okay, we’ve looked at the strengths of descriptive analytics—but where does it fall short? Some disadvantages of descriptive analytics include:
- You can summarize data sets you have access to, but these may not tell a complete story.
- You cannot use descriptive analytics to test a hypothesis or understand why data present the way they do.
- You cannot use descriptive analytics to predict what may happen in the future.
- You cannot generalize your findings to a broader population.
- Descriptive analytics tells you nothing about the data collection methodology, meaning the data set may include errors.
As you may suspect, although descriptive analytics are useful, it’s important not to overstretch their capabilities. Fortunately, we have diagnostic and predictive analytics to help fill in the gaps where descriptive analytics falls short.
5. Descriptive analytics use cases
Now we’ve covered the theory around descriptive analytics, how can it be used in the real world? While descriptive analytics only focuses on what has happened, not why, it remains a valuable first step in the broader data analytics process. Let’s take a look.
Tracking social media engagement
Social media is a key touchpoint along the sales journey. The ability to measure and present engagement metrics across a complex constellation of campaigns and social networks is, therefore, vital for determining the most successful approaches to digital marketing. Fortunately, marketing reports on social media engagement will include descriptive analytics by default. Clicks, likes, shares, detail expands, bounce rates, and so on are all measures of social media engagement that can be easily summarized using descriptive techniques.
For instance, perhaps a company is interested in knowing which social media account is driving the most traffic to their website. Using descriptive statistics, visualizations , and dashboards, they can easily compare information about different channels. Similarly, marketing teams can look at specific shareable content, perhaps comparing videos with blog posts, to see which results in the most clicks.
While none of this information draws direct conclusions (in that it doesn’t measure cause and effect) it’s still valuable. It helps teams to devise hypotheses or make informed guesses about where to invest their time and budget.
Streaming and e-commerce
Subscription streaming services like Spotify and Netflix, and e-commerce sites like Amazon and eBay all use descriptive analytics to identify trends. Descriptive measures help determine what’s currently most popular with users and buyers. Spotify , for example, uses descriptive analytics to learn which albums or artists subscribers are listening to. Meanwhile, Amazon uses descriptive analytics to compare customer purchases . In both cases, these insights inform their recommendation engines.
Netflix, meanwhile, takes this use of descriptive analytics even further. A highly data-driven company, Netflix uses descriptive analytics to see what genres and TV shows interest their subscribers most . These insights inform decision-making in areas from new content creation to marketing campaigns, and even which production companies they work with.
Learning management systems
From traditional education to corporate training, many organizations and schools now use online/offline hybrid learning. Learning management systems (or LMSs for those in the know!) are a ubiquitous part of this. LMS platforms track everything from user participation and attendance to test scores, and—in the case of e-learning courses—even how long it takes learners to complete. Summarizing this information, descriptive-analytical reports offer a high-level overview of what’s working and what’s not.
Using these data, teachers and training providers can track both individual and organization-level targets. They can analyze grade curves, or see which teaching resources are most popular. And while they won’t necessarily know why , it may be possible to infer from the data that videos, for example, are more popular than, say, written documents. Presenting this information is the first step towards improving course design and creating better learner outcomes.
6. Key takeaways
This post has offered a full introduction to descriptive analytics. We’ve learned that:
- Descriptive analytics is the simplest form of data analysis, and involves summarizing a data set’s main features and characteristics.
- Descriptive analytics relies on statistical measures of distribution, central tendency, and variability.
- It provides an overview of varied data types, from financial statements to surveys, website traffic, and scientific data.
- A key advantage of descriptive analytics is that it requires only basic math skills and allows you to present otherwise complex data in an easily digestible format.
- The main disadvantage of descriptive analytics is that it only summarizes data; it doesn’t draw conclusions or test hypotheses.
- We can use descriptive analytics to measure things like social media engagement, content curation, and learner outcomes.
To learn more about data analytics, or to try some test exercises, why not sign up for this free, 5-day data analytics short course ? You can also supplement your knowledge with the following introductory topics:
- Standard Error vs. Standard Deviation: What’s the Difference?
- What Is Data Visualization and Why Is It Important? A Complete Introduction
- The 7 Most Useful Data Analysis Methods and Techniques
- Business Essentials
- Leadership & Management
- Credential of Leadership, Impact, and Management in Business (CLIMB)
- Entrepreneurship & Innovation
- Digital Transformation
- Finance & Accounting
- Business in Society
- For Organizations
- Support Portal
- Media Coverage
- Founding Donors
- Leadership Team
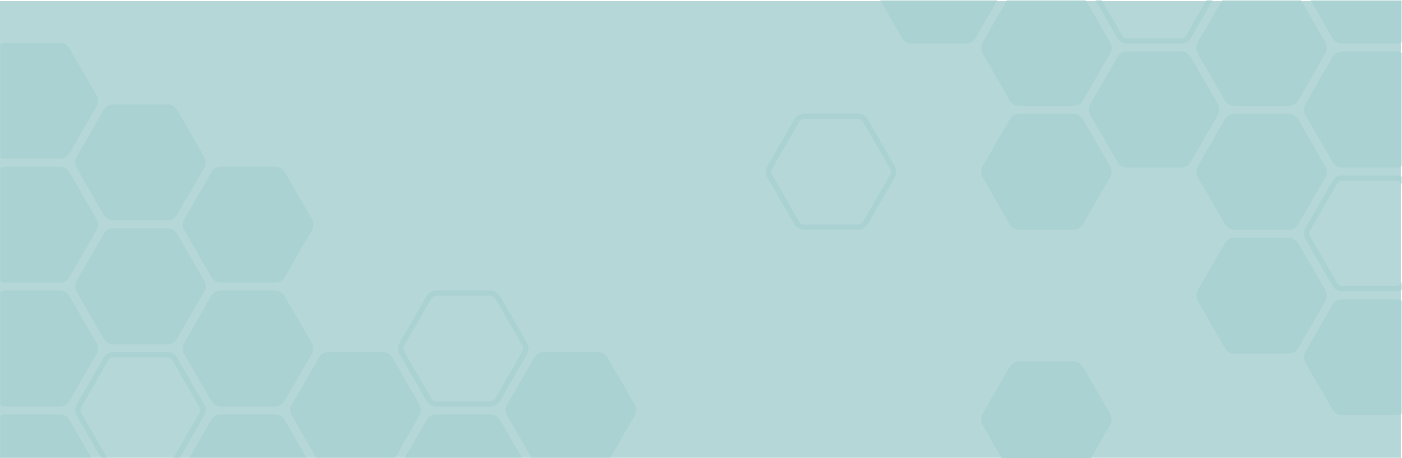
- Harvard Business School →
- HBS Online →
- Business Insights →
Business Insights
Harvard Business School Online's Business Insights Blog provides the career insights you need to achieve your goals and gain confidence in your business skills.
- Career Development
- Communication
- Decision-Making
- Earning Your MBA
- Negotiation
- News & Events
- Productivity
- Staff Spotlight
- Student Profiles
- Work-Life Balance
- AI Essentials for Business
- Alternative Investments
- Business Analytics
- Business Strategy
- Business and Climate Change
- Design Thinking and Innovation
- Digital Marketing Strategy
- Disruptive Strategy
- Economics for Managers
- Entrepreneurship Essentials
- Financial Accounting
- Global Business
- Launching Tech Ventures
- Leadership Principles
- Leadership, Ethics, and Corporate Accountability
- Leading Change and Organizational Renewal
- Leading with Finance
- Management Essentials
- Negotiation Mastery
- Organizational Leadership
- Power and Influence for Positive Impact
- Strategy Execution
- Sustainable Business Strategy
- Sustainable Investing
- Winning with Digital Platforms
What Is Descriptive Analytics? 5 Examples
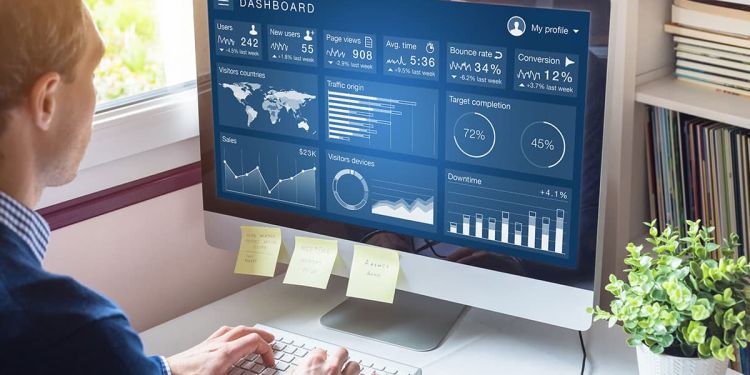
- 09 Nov 2021
Data analytics is a valuable tool for businesses aiming to increase revenue, improve products, and retain customers. According to research by global management consulting firm McKinsey & Company, companies that use data analytics are 23 times more likely to outperform competitors in terms of new customer acquisition than non-data-driven companies. They were also nine times more likely to surpass them in measures of customer loyalty and 19 times more likely to achieve above-average profitability.
Data analytics can be broken into four key types :
- Descriptive, which answers the question, “What happened?”
- Diagnostic , which answers the question, “Why did this happen?”
- Predictive , which answers the question, “What might happen in the future?”
- Prescriptive , which answers the question, “What should we do next?”
Each type of data analysis can help you reach specific goals and be used in tandem to create a full picture of data that informs your organization’s strategy formulation and decision-making.
Descriptive analytics can be leveraged on its own or act as a foundation for the other three analytics types. If you’re new to the field of business analytics, descriptive analytics is an accessible and rewarding place to start.
Access your free e-book today.
What Is Descriptive Analytics?
Descriptive analytics is the process of using current and historical data to identify trends and relationships. It’s sometimes called the simplest form of data analysis because it describes trends and relationships but doesn’t dig deeper.
Descriptive analytics is relatively accessible and likely something your organization uses daily. Basic statistical software, such as Microsoft Excel or data visualization tools , such as Google Charts and Tableau, can help parse data, identify trends and relationships between variables, and visually display information.
Descriptive analytics is especially useful for communicating change over time and uses trends as a springboard for further analysis to drive decision-making .
Here are five examples of descriptive analytics in action to apply at your organization.
Related: 5 Business Analytics Skills for Professionals
5 Examples of Descriptive Analytics
1. traffic and engagement reports.
One example of descriptive analytics is reporting. If your organization tracks engagement in the form of social media analytics or web traffic, you’re already using descriptive analytics.
These reports are created by taking raw data—generated when users interact with your website, advertisements, or social media content—and using it to compare current metrics to historical metrics and visualize trends.
For example, you may be responsible for reporting on which media channels drive the most traffic to the product page of your company’s website. Using descriptive analytics, you can analyze the page’s traffic data to determine the number of users from each source. You may decide to take it one step further and compare traffic source data to historical data from the same sources. This can enable you to update your team on movement; for instance, highlighting that traffic from paid advertisements increased 20 percent year over year.
The three other analytics types can then be used to determine why traffic from each source increased or decreased over time, if trends are predicted to continue, and what your team’s best course of action is moving forward.
2. Financial Statement Analysis
Another example of descriptive analytics that may be familiar to you is financial statement analysis. Financial statements are periodic reports that detail financial information about a business and, together, give a holistic view of a company’s financial health.
There are several types of financial statements, including the balance sheet , income statement , cash flow statement , and statement of shareholders’ equity. Each caters to a specific audience and conveys different information about a company’s finances.
Financial statement analysis can be done in three primary ways: vertical, horizontal, and ratio.
Vertical analysis involves reading a statement from top to bottom and comparing each item to those above and below it. This helps determine relationships between variables. For instance, if each line item is a percentage of the total, comparing them can provide insight into which are taking up larger and smaller percentages of the whole.
Horizontal analysis involves reading a statement from left to right and comparing each item to itself from a previous period. This type of analysis determines change over time.
Finally, ratio analysis involves comparing one section of a report to another based on their relationships to the whole. This directly compares items across periods, as well as your company’s ratios to the industry’s to gauge whether yours is over- or underperforming.
Each of these financial statement analysis methods are examples of descriptive analytics, as they provide information about trends and relationships between variables based on current and historical data.

3. Demand Trends
Descriptive analytics can also be used to identify trends in customer preference and behavior and make assumptions about the demand for specific products or services.
Streaming provider Netflix’s trend identification provides an excellent use case for descriptive analytics. Netflix’s team—which has a track record of being heavily data-driven—gathers data on users’ in-platform behavior. They analyze this data to determine which TV series and movies are trending at any given time and list trending titles in a section of the platform’s home screen.
Not only does this data allow Netflix users to see what’s popular—and thus, what they might enjoy watching—but it allows the Netflix team to know which types of media, themes, and actors are especially favored at a certain time. This can drive decision-making about future original content creation, contracts with existing production companies, marketing, and retargeting campaigns.
4. Aggregated Survey Results
Descriptive analytics is also useful in market research. When it comes time to glean insights from survey and focus group data, descriptive analytics can help identify relationships between variables and trends.
For instance, you may conduct a survey and identify that as respondents’ age increases, so does their likelihood to purchase your product. If you’ve conducted this survey multiple times over several years, descriptive analytics can tell you if this age-purchase correlation has always existed or if it was something that only occurred this year.
Insights like this can pave the way for diagnostic analytics to explain why certain factors are correlated. You can then leverage predictive and prescriptive analytics to plan future product improvements or marketing campaigns based on those trends.
Related: What Is Marketing Analytics?
5. Progress to Goals
Finally, descriptive analytics can be applied to track progress to goals. Reporting on progress toward key performance indicators (KPIs) can help your team understand if efforts are on track or if adjustments need to be made.
For example, if your organization aims to reach 500,000 monthly unique page views, you can use traffic data to communicate how you’re tracking toward it. Perhaps halfway through the month, you’re at 200,000 unique page views. This would be underperforming because you’d like to be halfway to your goal at that point—at 250,000 unique page views. This descriptive analysis of your team’s progress can allow further analysis to examine what can be done differently to improve traffic numbers and get back on track to hit your KPI.

Using Data to Identify Relationships and Trends
“Never before has so much data about so many different things been collected and stored every second of every day,” says Harvard Business School Professor Jan Hammond in the online course Business Analytics . “In this world of big data, data literacy —the ability to analyze, interpret, and even question data—is an increasingly valuable skill.”
Leveraging descriptive analytics to communicate change based on current and historical data and as a foundation for diagnostic, predictive, and prescriptive analytics has the potential to take you and your organization far.
Do you want to become a data-driven professional? Explore our eight-week Business Analytics course and our three-course Credential of Readiness (CORe) program to deepen your analytical skills and apply them to real-world business problems.

About the Author
- What is PESTLE?
- Entrepreneurs
- Permissions
- Privacy Policy
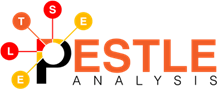
Descriptive Analysis: How-To, Types, Examples
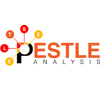
We review the basics of descriptive analysis, including what exactly it is, what benefits it has, how to do it, as well as some types and examples.
From diagnostic to predictive, there are many different types of data analysis . Perhaps the most straightforward of them is descriptive analysis, which seeks to describe or summarize past and present data, helping to create accessible data insights. In this short guide, we'll review the basics of descriptive analysis, including what exactly it is, what benefits it has, how to do it, as well as some types and examples.
What Is Descriptive Analysis?
Descriptive analysis, also known as descriptive analytics or descriptive statistics, is the process of using statistical techniques to describe or summarize a set of data. As one of the major types of data analysis, descriptive analysis is popular for its ability to generate accessible insights from otherwise uninterpreted data.
Unlike other types of data analysis, the descriptive analysis does not attempt to make predictions about the future. Instead, it draws insights solely from past data, by manipulating in ways that make it more meaningful.
Benefits of Descriptive Analysis
Descriptive analysis is all about trying to describe or summarize data. Although it doesn't make predictions about the future, it can still be extremely valuable in business environments . This is chiefly because descriptive analysis makes it easier to consume data, which can make it easier for analysts to act on.
Another benefit of descriptive analysis is that it can help to filter out less meaningful data. This is because the statistical techniques used within this type of analysis usually focus on the patterns in data, and not the outliers.
Types of Descriptive Analysis
According to CampusLabs.com , descriptive analysis can be categorized as one of four types. They are measures of frequency, central tendency, dispersion or variation, and position.
Measures of Frequency
In descriptive analysis, it's essential to know how frequently a certain event or response occurs. This is the purpose of measures of frequency, like a count or percent. For example, consider a survey where 1,000 participants are asked about their favourite ice cream flavor. A list of 1,000 responses would be difficult to consume, but the data can be made much more accessible by measuring how many times a certain flavor was selected.
Measures of Central Tendency
In descriptive analysis, it's also worth knowing the central (or average) event or response. Common measures of central tendency include the three averages — mean, median, and mode. As an example, consider a survey in which the height of 1,000 people is measured. In this case, the mean average would be a very helpful descriptive metric.
Measures of Dispersion
Sometimes, it may be worth knowing how data is distributed across a range. To illustrate this, consider the average height in a sample of two people. If both individuals are six feet tall, the average height is six feet. However, if one individual is five feet tall and the other is seven feet tall, the average height is still six feet. In order to measure this kind of distribution, measures of dispersion like range or standard deviation can be employed.
Measures of Position
Last of all, descriptive analysis can involve identifying the position of one event or response in relation to others. This is where measures like percentiles and quartiles can be used.

How to Do Descriptive Analysis
Like many types of data analysis, descriptive analysis can be quite open-ended. In other words, it's up to you what you want to look for in your analysis. With that said, the process of descriptive analysis usually consists of the same few steps.
- Collect data
The first step in any type of data analysis is to collect the data. This can be done in a variety of ways, but surveys and good old fashioned measurements are often used.
Another important step in descriptive and other types of data analysis is to clean the data. This is because data may be formatted in inaccessible ways, which will make it difficult to manipulate with statistics. Cleaning data may involve changing its textual format, categorizing it, and/or removing outliers.
- Apply methods
Finally, descriptive analysis involves applying the chosen statistical methods so as to draw the desired conclusions. What methods you choose will depend on the data you are dealing with and what you are looking to determine. If in doubt, review the four types of descriptive analysis methods explained above.
When to Do Descriptive Analysis
Descriptive analysis is often used when reviewing any past or present data. This is because raw data is difficult to consume and interpret, while the metrics offered by descriptive analysis are much more focused.
Descriptive analysis can also be conducted as the precursor to diagnostic or predictive analysis , providing insights into what has happened in the past before attempting to explain why it happened or predicting what will happen in the future.
Descriptive Analysis Example
As an example of descriptive analysis, consider an insurance company analyzing its customer base.
The insurance company may know certain traits about its customers, such as their gender, age, and nationality. To gain a better profile of their customers, the insurance company can apply descriptive analysis.
Measures of frequency can be used to identify how many customers are under a certain age; measures of central tendency can be used to identify who most of their customers are; measures of dispersion can be used to identify the variation in, for example, the age of their customers; finally, measures of position can be used to compare segments of customers based on specific traits.
Final Thoughts
Descriptive analysis is a popular type of data analysis. It's often conducted before diagnostic or predictive analysis, as it simply aims to describe and summarize past data.
To do so, descriptive analysis uses a variety of statistical techniques, including measures of frequency, central tendency, dispersion, and position. How exactly you conduct descriptive analysis will depend on what you are looking to find out, but the steps usually involve collecting, cleaning, and finally analyzing data.
In any case, this business analysis process is invaluable when working with data.
Image by Pexels
Integration of Cybersecurity in Market Analysis: How to Apply Different Methodologies to Identify Risks
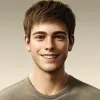
Streamlining Your Business Analysis with a Master's in Lean Manufacturing
Technological factors affecting business to include in pestle analysis.
Chapter 14 Quantitative Analysis Descriptive Statistics
Numeric data collected in a research project can be analyzed quantitatively using statistical tools in two different ways. Descriptive analysis refers to statistically describing, aggregating, and presenting the constructs of interest or associations between these constructs. Inferential analysis refers to the statistical testing of hypotheses (theory testing). In this chapter, we will examine statistical techniques used for descriptive analysis, and the next chapter will examine statistical techniques for inferential analysis. Much of today’s quantitative data analysis is conducted using software programs such as SPSS or SAS. Readers are advised to familiarize themselves with one of these programs for understanding the concepts described in this chapter.
Data Preparation
In research projects, data may be collected from a variety of sources: mail-in surveys, interviews, pretest or posttest experimental data, observational data, and so forth. This data must be converted into a machine -readable, numeric format, such as in a spreadsheet or a text file, so that they can be analyzed by computer programs like SPSS or SAS. Data preparation usually follows the following steps.
Data coding. Coding is the process of converting data into numeric format. A codebook should be created to guide the coding process. A codebook is a comprehensive document containing detailed description of each variable in a research study, items or measures for that variable, the format of each item (numeric, text, etc.), the response scale for each item (i.e., whether it is measured on a nominal, ordinal, interval, or ratio scale; whether such scale is a five-point, seven-point, or some other type of scale), and how to code each value into a numeric format. For instance, if we have a measurement item on a seven-point Likert scale with anchors ranging from “strongly disagree” to “strongly agree”, we may code that item as 1 for strongly disagree, 4 for neutral, and 7 for strongly agree, with the intermediate anchors in between. Nominal data such as industry type can be coded in numeric form using a coding scheme such as: 1 for manufacturing, 2 for retailing, 3 for financial, 4 for healthcare, and so forth (of course, nominal data cannot be analyzed statistically). Ratio scale data such as age, income, or test scores can be coded as entered by the respondent. Sometimes, data may need to be aggregated into a different form than the format used for data collection. For instance, for measuring a construct such as “benefits of computers,” if a survey provided respondents with a checklist of b enefits that they could select from (i.e., they could choose as many of those benefits as they wanted), then the total number of checked items can be used as an aggregate measure of benefits. Note that many other forms of data, such as interview transcripts, cannot be converted into a numeric format for statistical analysis. Coding is especially important for large complex studies involving many variables and measurement items, where the coding process is conducted by different people, to help the coding team code data in a consistent manner, and also to help others understand and interpret the coded data.
Data entry. Coded data can be entered into a spreadsheet, database, text file, or directly into a statistical program like SPSS. Most statistical programs provide a data editor for entering data. However, these programs store data in their own native format (e.g., SPSS stores data as .sav files), which makes it difficult to share that data with other statistical programs. Hence, it is often better to enter data into a spreadsheet or database, where they can be reorganized as needed, shared across programs, and subsets of data can be extracted for analysis. Smaller data sets with less than 65,000 observations and 256 items can be stored in a spreadsheet such as Microsoft Excel, while larger dataset with millions of observations will require a database. Each observation can be entered as one row in the spreadsheet and each measurement item can be represented as one column. The entered data should be frequently checked for accuracy, via occasional spot checks on a set of items or observations, during and after entry. Furthermore, while entering data, the coder should watch out for obvious evidence of bad data, such as the respondent selecting the “strongly agree” response to all items irrespective of content, including reverse-coded items. If so, such data can be entered but should be excluded from subsequent analysis.
Missing values. Missing data is an inevitable part of any empirical data set. Respondents may not answer certain questions if they are ambiguously worded or too sensitive. Such problems should be detected earlier during pretests and corrected before the main data collection process begins. During data entry, some statistical programs automatically treat blank entries as missing values, while others require a specific numeric value such as -1 or 999 to be entered to denote a missing value. During data analysis, the default mode of handling missing values in most software programs is to simply drop the entire observation containing even a single missing value, in a technique called listwise deletion . Such deletion can significantly shrink the sample size and make it extremely difficult to detect small effects. Hence, some software programs allow the option of replacing missing values with an estimated value via a process called imputation . For instance, if the missing value is one item in a multi-item scale, the imputed value may be the average of the respondent’s responses to remaining items on that scale. If the missing value belongs to a single-item scale, many researchers use the average of other respondent’s responses to that item as the imputed value. Such imputation may be biased if the missing value is of a systematic nature rather than a random nature. Two methods that can produce relatively unbiased estimates for imputation are the maximum likelihood procedures and multiple imputation methods, both of which are supported in popular software programs such as SPSS and SAS.
Data transformation. Sometimes, it is necessary to transform data values before they can be meaningfully interpreted. For instance, reverse coded items, where items convey the opposite meaning of that of their underlying construct, should be reversed (e.g., in a 1-7 interval scale, 8 minus the observed value will reverse the value) before they can be compared or combined with items that are not reverse coded. Other kinds of transformations may include creating scale measures by adding individual scale items, creating a weighted index from a set of observed measures, and collapsing multiple values into fewer categories (e.g., collapsing incomes into income ranges).
Univariate Analysis
Univariate analysis, or analysis of a single variable, refers to a set of statistical techniques that can describe the general properties of one variable. Univariate statistics include: (1) frequency distribution, (2) central tendency, and (3) dispersion. The frequency distribution of a variable is a summary of the frequency (or percentages) of individual values or ranges of values for that variable. For instance, we can measure how many times a sample of respondents attend religious services (as a measure of their “religiosity”) using a categorical scale: never, once per year, several times per year, about once a month, several times per month, several times per week, and an optional category for “did not answer.” If we count the number (or percentage) of observations within each category (except “did not answer” which is really a missing value rather than a category), and display it in the form of a table as shown in Figure 14.1, what we have is a frequency distribution. This distribution can also be depicted in the form of a bar chart, as shown on the right panel of Figure 14.1, with the horizontal axis representing each category of that variable and the vertical axis representing the frequency or percentage of observations within each category.
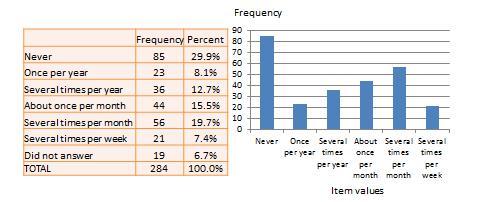
Figure 14.1. Frequency distribution of religiosity.
With very large samples where observations are independent and random, the frequency distribution tends to follow a plot that looked like a bell-shaped curve (a smoothed bar chart of the frequency distribution) similar to that shown in Figure 14.2, where most observations are clustered toward the center of the range of values, and fewer and fewer observations toward the extreme ends of the range. Such a curve is called a normal distribution.
Central tendency is an estimate of the center of a distribution of values. There are three major estimates of central tendency: mean, median, and mode. The arithmetic mean (often simply called the “mean”) is the simple average of all values in a given distribution. Consider a set of eight test scores: 15, 22, 21, 18, 36, 15, 25, 15. The arithmetic mean of these values is (15 + 20 + 21 + 20 + 36 + 15 + 25 + 15)/8 = 20.875. Other types of means include geometric mean (n th root of the product of n numbers in a distribution) and harmonic mean (the reciprocal of the arithmetic means of the reciprocal of each value in a distribution), but these means are not very popular for statistical analysis of social research data.
The second measure of central tendency, the median , is the middle value within a range of values in a distribution. This is computed by sorting all values in a distribution in increasing order and selecting the middle value. In case there are two middle values (if there is an even number of values in a distribution), the average of the two middle values represent the median. In the above example, the sorted values are: 15, 15, 15, 18, 22, 21, 25, 36. The two middle values are 18 and 22, and hence the median is (18 + 22)/2 = 20.
Lastly, the mode is the most frequently occurring value in a distribution of values. In the previous example, the most frequently occurring value is 15, which is the mode of the above set of test scores. Note that any value that is estimated from a sample, such as mean, median, mode, or any of the later estimates are called a statistic .
Dispersion refers to the way values are spread around the central tendency, for example, how tightly or how widely are the values clustered around the mean. Two common measures of dispersion are the range and standard deviation. The range is the difference between the highest and lowest values in a distribution. The range in our previous example is 36-15 = 21.
The range is particularly sensitive to the presence of outliers. For instance, if the highest value in the above distribution was 85 and the other vales remained the same, the range would be 85-15 = 70. Standard deviation , the second measure of dispersion, corrects for such outliers by using a formula that takes into account how close or how far each value from the distribution mean:
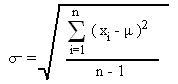
Figure 14.2. Normal distribution.
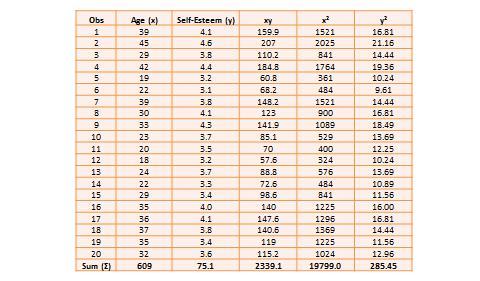
Table 14.1. Hypothetical data on age and self-esteem.
The two variables in this dataset are age (x) and self-esteem (y). Age is a ratio-scale variable, while self-esteem is an average score computed from a multi-item self-esteem scale measured using a 7-point Likert scale, ranging from “strongly disagree” to “strongly agree.” The histogram of each variable is shown on the left side of Figure 14.3. The formula for calculating bivariate correlation is:

Figure 14.3. Histogram and correlation plot of age and self-esteem.
After computing bivariate correlation, researchers are often interested in knowing whether the correlation is significant (i.e., a real one) or caused by mere chance. Answering such a question would require testing the following hypothesis:
H 0 : r = 0
H 1 : r ≠ 0
H 0 is called the null hypotheses , and H 1 is called the alternative hypothesis (sometimes, also represented as H a ). Although they may seem like two hypotheses, H 0 and H 1 actually represent a single hypothesis since they are direct opposites of each other. We are interested in testing H 1 rather than H 0 . Also note that H 1 is a non-directional hypotheses since it does not specify whether r is greater than or less than zero. Directional hypotheses will be specified as H 0 : r ≤ 0; H 1 : r > 0 (if we are testing for a positive correlation). Significance testing of directional hypothesis is done using a one-tailed t-test, while that for non-directional hypothesis is done using a two-tailed t-test.
In statistical testing, the alternative hypothesis cannot be tested directly. Rather, it is tested indirectly by rejecting the null hypotheses with a certain level of probability. Statistical testing is always probabilistic, because we are never sure if our inferences, based on sample data, apply to the population, since our sample never equals the population. The probability that a statistical inference is caused pure chance is called the p-value . The p-value is compared with the significance level (α), which represents the maximum level of risk that we are willing to take that our inference is incorrect. For most statistical analysis, α is set to 0.05. A p-value less than α=0.05 indicates that we have enough statistical evidence to reject the null hypothesis, and thereby, indirectly accept the alternative hypothesis. If p>0.05, then we do not have adequate statistical evidence to reject the null hypothesis or accept the alternative hypothesis.
The easiest way to test for the above hypothesis is to look up critical values of r from statistical tables available in any standard text book on statistics or on the Internet (most software programs also perform significance testing). The critical value of r depends on our desired significance level (α = 0.05), the degrees of freedom (df), and whether the desired test is a one-tailed or two-tailed test. The degree of freedom is the number of values that can vary freely in any calculation of a statistic. In case of correlation, the df simply equals n – 2, or for the data in Table 14.1, df is 20 – 2 = 18. There are two different statistical tables for one-tailed and two -tailed test. In the two -tailed table, the critical value of r for α = 0.05 and df = 18 is 0.44. For our computed correlation of 0.79 to be significant, it must be larger than the critical value of 0.44 or less than -0.44. Since our computed value of 0.79 is greater than 0.44, we conclude that there is a significant correlation between age and self-esteem in our data set, or in other words, the odds are less than 5% that this correlation is a chance occurrence. Therefore, we can reject the null hypotheses that r ≤ 0, which is an indirect way of saying that the alternative hypothesis r > 0 is probably correct.
Most research studies involve more than two variables. If there are n variables, then we will have a total of n*(n-1)/2 possible correlations between these n variables. Such correlations are easily computed using a software program like SPSS, rather than manually using the formula for correlation (as we did in Table 14.1), and represented using a correlation matrix, as shown in Table 14.2. A correlation matrix is a matrix that lists the variable names along the first row and the first column, and depicts bivariate correlations between pairs of variables in the appropriate cell in the matrix. The values along the principal diagonal (from the top left to the bottom right corner) of this matrix are always 1, because any variable is always perfectly correlated with itself. Further, since correlations are non-directional, the correlation between variables V1 and V2 is the same as that between V2 and V1. Hence, the lower triangular matrix (values below the principal diagonal) is a mirror reflection of the upper triangular matrix (values above the principal diagonal), and therefore, we often list only the lower triangular matrix for simplicity. If the correlations involve variables measured using interval scales, then this specific type of correlations are called Pearson product moment correlations .
Another useful way of presenting bivariate data is cross-tabulation (often abbreviated to cross-tab, and sometimes called more formally as a contingency table). A cross-tab is a table that describes the frequency (or percentage) of all combinations of two or more nominal or categorical variables. As an example, let us assume that we have the following observations of gender and grade for a sample of 20 students, as shown in Figure 14.3. Gender is a nominal variable (male/female or M/F), and grade is a categorical variable with three levels (A, B, and C). A simple cross-tabulation of the data may display the joint distribution of gender and grades (i.e., how many students of each gender are in each grade category, as a raw frequency count or as a percentage) in a 2 x 3 matrix. This matrix will help us see if A, B, and C grades are equally distributed across male and female students. The cross-tab data in Table 14.3 shows that the distribution of A grades is biased heavily toward female students: in a sample of 10 male and 10 female students, five female students received the A grade compared to only one male students. In contrast, the distribution of C grades is biased toward male students: three male students received a C grade, compared to only one female student. However, the distribution of B grades was somewhat uniform, with six male students and five female students. The last row and the last column of this table are called marginal totals because they indicate the totals across each category and displayed along the margins of the table.
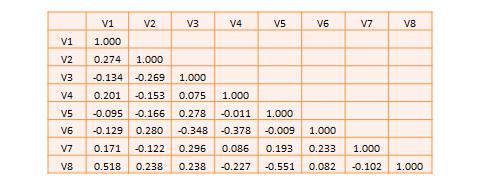
Table 14.2. A hypothetical correlation matrix for eight variables.
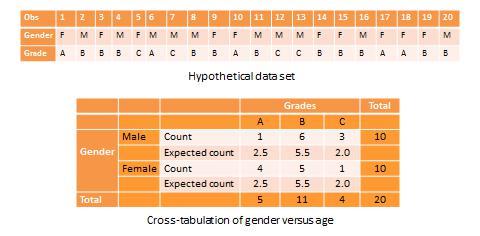
Table 14.3. Example of cross-tab analysis.
Although we can see a distinct pattern of grade distribution between male and female students in Table 14.3, is this pattern real or “statistically significant”? In other words, do the above frequency counts differ from that that may be expected from pure chance? To answer this question, we should compute the expected count of observation in each cell of the 2 x 3 cross-tab matrix. This is done by multiplying the marginal column total and the marginal row total for each cell and dividing it by the total number of observations. For example, for the male/A grade cell, expected count = 5 * 10 / 20 = 2.5. In other words, we were expecting 2.5 male students to receive an A grade, but in reality, only one student received the A grade. Whether this difference between expected and actual count is significant can be tested using a chi-square test . The chi-square statistic can be computed as the average difference between observed and expected counts across all cells. We can then compare this number to the critical value associated with a desired probability level (p < 0.05) and the degrees of freedom, which is simply (m-1)*(n-1), where m and n are the number of rows and columns respectively. In this example, df = (2 – 1) * (3 – 1) = 2. From standard chi-square tables in any statistics book, the critical chi-square value for p=0.05 and df=2 is 5.99. The computed chi -square value, based on our observed data, is 1.00, which is less than the critical value. Hence, we must conclude that the observed grade pattern is not statistically different from the pattern that can be expected by pure chance.
- Social Science Research: Principles, Methods, and Practices. Authored by : Anol Bhattacherjee. Provided by : University of South Florida. Located at : http://scholarcommons.usf.edu/oa_textbooks/3/ . License : CC BY-NC-SA: Attribution-NonCommercial-ShareAlike
Data Analysis in Quantitative Research
- Reference work entry
- First Online: 13 January 2019
- Cite this reference work entry
- Yong Moon Jung 2
1951 Accesses
2 Citations
Quantitative data analysis serves as part of an essential process of evidence-making in health and social sciences. It is adopted for any types of research question and design whether it is descriptive, explanatory, or causal. However, compared with qualitative counterpart, quantitative data analysis has less flexibility. Conducting quantitative data analysis requires a prerequisite understanding of the statistical knowledge and skills. It also requires rigor in the choice of appropriate analysis model and the interpretation of the analysis outcomes. Basically, the choice of appropriate analysis techniques is determined by the type of research question and the nature of the data. In addition, different analysis techniques require different assumptions of data. This chapter provides introductory guides for readers to assist them with their informed decision-making in choosing the correct analysis models. To this end, it begins with discussion of the levels of measure: nominal, ordinal, and scale. Some commonly used analysis techniques in univariate, bivariate, and multivariate data analysis are presented for practical examples. Example analysis outcomes are produced by the use of SPSS (Statistical Package for Social Sciences).
This is a preview of subscription content, log in via an institution to check access.
Access this chapter
- Available as PDF
- Read on any device
- Instant download
- Own it forever
- Available as EPUB and PDF
- Durable hardcover edition
- Dispatched in 3 to 5 business days
- Free shipping worldwide - see info
Tax calculation will be finalised at checkout
Purchases are for personal use only
Institutional subscriptions
Similar content being viewed by others
Data Analysis Techniques for Quantitative Study
Meta-Analytic Methods for Public Health Research
Armstrong JS. Significance tests harm progress in forecasting. Int J Forecast. 2007;23(2):321–7.
Article Google Scholar
Babbie E. The practice of social research. 14th ed. Belmont: Cengage Learning; 2016.
Google Scholar
Brockopp DY, Hastings-Tolsma MT. Fundamentals of nursing research. Boston: Jones & Bartlett; 2003.
Creswell JW. Research design: qualitative, quantitative, and mixed methods approaches. Thousand Oaks: Sage; 2014.
Fawcett J. The relationship of theory and research. Philadelphia: F. A. Davis; 1999.
Field A. Discovering statistics using IBM SPSS statistics. London: Sage; 2013.
Grove SK, Gray JR, Burns N. Understanding nursing research: building an evidence-based practice. 6th ed. St. Louis: Elsevier Saunders; 2015.
Hair JF, Black WC, Babin BJ, Anderson RE, Tatham RD. Multivariate data analysis. Upper Saddle River: Pearson Prentice Hall; 2006.
Katz MH. Multivariable analysis: a practical guide for clinicians. Cambridge: Cambridge University Press; 2006.
Book Google Scholar
McHugh ML. Scientific inquiry. J Specialists Pediatr Nurs. 2007; 8 (1):35–7. Volume 8, Issue 1, Version of Record online: 22 FEB 2007
Pallant J. SPSS survival manual: a step by step guide to data analysis using IBM SPSS. Sydney: Allen & Unwin; 2016.
Polit DF, Beck CT. Nursing research: principles and methods. Philadelphia: Lippincott Williams & Wilkins; 2004.
Trochim WMK, Donnelly JP. Research methods knowledge base. 3rd ed. Mason: Thomson Custom Publishing; 2007.
Tabachnick, B. G., & Fidell, L. S. (2013). Using multivariate statistics. Boston: Pearson Education.
Wells CS, Hin JM. Dealing with assumptions underlying statistical tests. Psychol Sch. 2007;44(5):495–502.
Download references
Author information
Authors and affiliations.
Centre for Business and Social Innovation, University of Technology Sydney, Ultimo, NSW, Australia
Yong Moon Jung
You can also search for this author in PubMed Google Scholar
Corresponding author
Correspondence to Yong Moon Jung .
Editor information
Editors and affiliations.
School of Science and Health, Western Sydney University, Penrith, NSW, Australia
Pranee Liamputtong
Rights and permissions
Reprints and permissions
Copyright information
© 2019 Springer Nature Singapore Pte Ltd.
About this entry
Cite this entry.
Jung, Y.M. (2019). Data Analysis in Quantitative Research. In: Liamputtong, P. (eds) Handbook of Research Methods in Health Social Sciences. Springer, Singapore. https://doi.org/10.1007/978-981-10-5251-4_109
Download citation
DOI : https://doi.org/10.1007/978-981-10-5251-4_109
Published : 13 January 2019
Publisher Name : Springer, Singapore
Print ISBN : 978-981-10-5250-7
Online ISBN : 978-981-10-5251-4
eBook Packages : Social Sciences Reference Module Humanities and Social Sciences Reference Module Business, Economics and Social Sciences
Share this entry
Anyone you share the following link with will be able to read this content:
Sorry, a shareable link is not currently available for this article.
Provided by the Springer Nature SharedIt content-sharing initiative
- Publish with us
Policies and ethics
- Find a journal
- Track your research

An official website of the United States government
The .gov means it’s official. Federal government websites often end in .gov or .mil. Before sharing sensitive information, make sure you’re on a federal government site.
The site is secure. The https:// ensures that you are connecting to the official website and that any information you provide is encrypted and transmitted securely.
- Publications
- Account settings
Preview improvements coming to the PMC website in October 2024. Learn More or Try it out now .
- Advanced Search
- Journal List
- Indian J Anaesth
- v.60(9); 2016 Sep
Basic statistical tools in research and data analysis
Zulfiqar ali.
Department of Anaesthesiology, Division of Neuroanaesthesiology, Sheri Kashmir Institute of Medical Sciences, Soura, Srinagar, Jammu and Kashmir, India
S Bala Bhaskar
1 Department of Anaesthesiology and Critical Care, Vijayanagar Institute of Medical Sciences, Bellary, Karnataka, India
Statistical methods involved in carrying out a study include planning, designing, collecting data, analysing, drawing meaningful interpretation and reporting of the research findings. The statistical analysis gives meaning to the meaningless numbers, thereby breathing life into a lifeless data. The results and inferences are precise only if proper statistical tests are used. This article will try to acquaint the reader with the basic research tools that are utilised while conducting various studies. The article covers a brief outline of the variables, an understanding of quantitative and qualitative variables and the measures of central tendency. An idea of the sample size estimation, power analysis and the statistical errors is given. Finally, there is a summary of parametric and non-parametric tests used for data analysis.
INTRODUCTION
Statistics is a branch of science that deals with the collection, organisation, analysis of data and drawing of inferences from the samples to the whole population.[ 1 ] This requires a proper design of the study, an appropriate selection of the study sample and choice of a suitable statistical test. An adequate knowledge of statistics is necessary for proper designing of an epidemiological study or a clinical trial. Improper statistical methods may result in erroneous conclusions which may lead to unethical practice.[ 2 ]
Variable is a characteristic that varies from one individual member of population to another individual.[ 3 ] Variables such as height and weight are measured by some type of scale, convey quantitative information and are called as quantitative variables. Sex and eye colour give qualitative information and are called as qualitative variables[ 3 ] [ Figure 1 ].

Classification of variables
Quantitative variables
Quantitative or numerical data are subdivided into discrete and continuous measurements. Discrete numerical data are recorded as a whole number such as 0, 1, 2, 3,… (integer), whereas continuous data can assume any value. Observations that can be counted constitute the discrete data and observations that can be measured constitute the continuous data. Examples of discrete data are number of episodes of respiratory arrests or the number of re-intubations in an intensive care unit. Similarly, examples of continuous data are the serial serum glucose levels, partial pressure of oxygen in arterial blood and the oesophageal temperature.
A hierarchical scale of increasing precision can be used for observing and recording the data which is based on categorical, ordinal, interval and ratio scales [ Figure 1 ].
Categorical or nominal variables are unordered. The data are merely classified into categories and cannot be arranged in any particular order. If only two categories exist (as in gender male and female), it is called as a dichotomous (or binary) data. The various causes of re-intubation in an intensive care unit due to upper airway obstruction, impaired clearance of secretions, hypoxemia, hypercapnia, pulmonary oedema and neurological impairment are examples of categorical variables.
Ordinal variables have a clear ordering between the variables. However, the ordered data may not have equal intervals. Examples are the American Society of Anesthesiologists status or Richmond agitation-sedation scale.
Interval variables are similar to an ordinal variable, except that the intervals between the values of the interval variable are equally spaced. A good example of an interval scale is the Fahrenheit degree scale used to measure temperature. With the Fahrenheit scale, the difference between 70° and 75° is equal to the difference between 80° and 85°: The units of measurement are equal throughout the full range of the scale.
Ratio scales are similar to interval scales, in that equal differences between scale values have equal quantitative meaning. However, ratio scales also have a true zero point, which gives them an additional property. For example, the system of centimetres is an example of a ratio scale. There is a true zero point and the value of 0 cm means a complete absence of length. The thyromental distance of 6 cm in an adult may be twice that of a child in whom it may be 3 cm.
STATISTICS: DESCRIPTIVE AND INFERENTIAL STATISTICS
Descriptive statistics[ 4 ] try to describe the relationship between variables in a sample or population. Descriptive statistics provide a summary of data in the form of mean, median and mode. Inferential statistics[ 4 ] use a random sample of data taken from a population to describe and make inferences about the whole population. It is valuable when it is not possible to examine each member of an entire population. The examples if descriptive and inferential statistics are illustrated in Table 1 .
Example of descriptive and inferential statistics

Descriptive statistics
The extent to which the observations cluster around a central location is described by the central tendency and the spread towards the extremes is described by the degree of dispersion.
Measures of central tendency
The measures of central tendency are mean, median and mode.[ 6 ] Mean (or the arithmetic average) is the sum of all the scores divided by the number of scores. Mean may be influenced profoundly by the extreme variables. For example, the average stay of organophosphorus poisoning patients in ICU may be influenced by a single patient who stays in ICU for around 5 months because of septicaemia. The extreme values are called outliers. The formula for the mean is

where x = each observation and n = number of observations. Median[ 6 ] is defined as the middle of a distribution in a ranked data (with half of the variables in the sample above and half below the median value) while mode is the most frequently occurring variable in a distribution. Range defines the spread, or variability, of a sample.[ 7 ] It is described by the minimum and maximum values of the variables. If we rank the data and after ranking, group the observations into percentiles, we can get better information of the pattern of spread of the variables. In percentiles, we rank the observations into 100 equal parts. We can then describe 25%, 50%, 75% or any other percentile amount. The median is the 50 th percentile. The interquartile range will be the observations in the middle 50% of the observations about the median (25 th -75 th percentile). Variance[ 7 ] is a measure of how spread out is the distribution. It gives an indication of how close an individual observation clusters about the mean value. The variance of a population is defined by the following formula:

where σ 2 is the population variance, X is the population mean, X i is the i th element from the population and N is the number of elements in the population. The variance of a sample is defined by slightly different formula:

where s 2 is the sample variance, x is the sample mean, x i is the i th element from the sample and n is the number of elements in the sample. The formula for the variance of a population has the value ‘ n ’ as the denominator. The expression ‘ n −1’ is known as the degrees of freedom and is one less than the number of parameters. Each observation is free to vary, except the last one which must be a defined value. The variance is measured in squared units. To make the interpretation of the data simple and to retain the basic unit of observation, the square root of variance is used. The square root of the variance is the standard deviation (SD).[ 8 ] The SD of a population is defined by the following formula:

where σ is the population SD, X is the population mean, X i is the i th element from the population and N is the number of elements in the population. The SD of a sample is defined by slightly different formula:

where s is the sample SD, x is the sample mean, x i is the i th element from the sample and n is the number of elements in the sample. An example for calculation of variation and SD is illustrated in Table 2 .
Example of mean, variance, standard deviation

Normal distribution or Gaussian distribution
Most of the biological variables usually cluster around a central value, with symmetrical positive and negative deviations about this point.[ 1 ] The standard normal distribution curve is a symmetrical bell-shaped. In a normal distribution curve, about 68% of the scores are within 1 SD of the mean. Around 95% of the scores are within 2 SDs of the mean and 99% within 3 SDs of the mean [ Figure 2 ].

Normal distribution curve
Skewed distribution
It is a distribution with an asymmetry of the variables about its mean. In a negatively skewed distribution [ Figure 3 ], the mass of the distribution is concentrated on the right of Figure 1 . In a positively skewed distribution [ Figure 3 ], the mass of the distribution is concentrated on the left of the figure leading to a longer right tail.

Curves showing negatively skewed and positively skewed distribution
Inferential statistics
In inferential statistics, data are analysed from a sample to make inferences in the larger collection of the population. The purpose is to answer or test the hypotheses. A hypothesis (plural hypotheses) is a proposed explanation for a phenomenon. Hypothesis tests are thus procedures for making rational decisions about the reality of observed effects.
Probability is the measure of the likelihood that an event will occur. Probability is quantified as a number between 0 and 1 (where 0 indicates impossibility and 1 indicates certainty).
In inferential statistics, the term ‘null hypothesis’ ( H 0 ‘ H-naught ,’ ‘ H-null ’) denotes that there is no relationship (difference) between the population variables in question.[ 9 ]
Alternative hypothesis ( H 1 and H a ) denotes that a statement between the variables is expected to be true.[ 9 ]
The P value (or the calculated probability) is the probability of the event occurring by chance if the null hypothesis is true. The P value is a numerical between 0 and 1 and is interpreted by researchers in deciding whether to reject or retain the null hypothesis [ Table 3 ].
P values with interpretation

If P value is less than the arbitrarily chosen value (known as α or the significance level), the null hypothesis (H0) is rejected [ Table 4 ]. However, if null hypotheses (H0) is incorrectly rejected, this is known as a Type I error.[ 11 ] Further details regarding alpha error, beta error and sample size calculation and factors influencing them are dealt with in another section of this issue by Das S et al .[ 12 ]
Illustration for null hypothesis

PARAMETRIC AND NON-PARAMETRIC TESTS
Numerical data (quantitative variables) that are normally distributed are analysed with parametric tests.[ 13 ]
Two most basic prerequisites for parametric statistical analysis are:
- The assumption of normality which specifies that the means of the sample group are normally distributed
- The assumption of equal variance which specifies that the variances of the samples and of their corresponding population are equal.
However, if the distribution of the sample is skewed towards one side or the distribution is unknown due to the small sample size, non-parametric[ 14 ] statistical techniques are used. Non-parametric tests are used to analyse ordinal and categorical data.
Parametric tests
The parametric tests assume that the data are on a quantitative (numerical) scale, with a normal distribution of the underlying population. The samples have the same variance (homogeneity of variances). The samples are randomly drawn from the population, and the observations within a group are independent of each other. The commonly used parametric tests are the Student's t -test, analysis of variance (ANOVA) and repeated measures ANOVA.
Student's t -test
Student's t -test is used to test the null hypothesis that there is no difference between the means of the two groups. It is used in three circumstances:

where X = sample mean, u = population mean and SE = standard error of mean

where X 1 − X 2 is the difference between the means of the two groups and SE denotes the standard error of the difference.
- To test if the population means estimated by two dependent samples differ significantly (the paired t -test). A usual setting for paired t -test is when measurements are made on the same subjects before and after a treatment.
The formula for paired t -test is:

where d is the mean difference and SE denotes the standard error of this difference.
The group variances can be compared using the F -test. The F -test is the ratio of variances (var l/var 2). If F differs significantly from 1.0, then it is concluded that the group variances differ significantly.
Analysis of variance
The Student's t -test cannot be used for comparison of three or more groups. The purpose of ANOVA is to test if there is any significant difference between the means of two or more groups.
In ANOVA, we study two variances – (a) between-group variability and (b) within-group variability. The within-group variability (error variance) is the variation that cannot be accounted for in the study design. It is based on random differences present in our samples.
However, the between-group (or effect variance) is the result of our treatment. These two estimates of variances are compared using the F-test.
A simplified formula for the F statistic is:

where MS b is the mean squares between the groups and MS w is the mean squares within groups.
Repeated measures analysis of variance
As with ANOVA, repeated measures ANOVA analyses the equality of means of three or more groups. However, a repeated measure ANOVA is used when all variables of a sample are measured under different conditions or at different points in time.
As the variables are measured from a sample at different points of time, the measurement of the dependent variable is repeated. Using a standard ANOVA in this case is not appropriate because it fails to model the correlation between the repeated measures: The data violate the ANOVA assumption of independence. Hence, in the measurement of repeated dependent variables, repeated measures ANOVA should be used.
Non-parametric tests
When the assumptions of normality are not met, and the sample means are not normally, distributed parametric tests can lead to erroneous results. Non-parametric tests (distribution-free test) are used in such situation as they do not require the normality assumption.[ 15 ] Non-parametric tests may fail to detect a significant difference when compared with a parametric test. That is, they usually have less power.
As is done for the parametric tests, the test statistic is compared with known values for the sampling distribution of that statistic and the null hypothesis is accepted or rejected. The types of non-parametric analysis techniques and the corresponding parametric analysis techniques are delineated in Table 5 .
Analogue of parametric and non-parametric tests

Median test for one sample: The sign test and Wilcoxon's signed rank test
The sign test and Wilcoxon's signed rank test are used for median tests of one sample. These tests examine whether one instance of sample data is greater or smaller than the median reference value.
This test examines the hypothesis about the median θ0 of a population. It tests the null hypothesis H0 = θ0. When the observed value (Xi) is greater than the reference value (θ0), it is marked as+. If the observed value is smaller than the reference value, it is marked as − sign. If the observed value is equal to the reference value (θ0), it is eliminated from the sample.
If the null hypothesis is true, there will be an equal number of + signs and − signs.
The sign test ignores the actual values of the data and only uses + or − signs. Therefore, it is useful when it is difficult to measure the values.
Wilcoxon's signed rank test
There is a major limitation of sign test as we lose the quantitative information of the given data and merely use the + or – signs. Wilcoxon's signed rank test not only examines the observed values in comparison with θ0 but also takes into consideration the relative sizes, adding more statistical power to the test. As in the sign test, if there is an observed value that is equal to the reference value θ0, this observed value is eliminated from the sample.
Wilcoxon's rank sum test ranks all data points in order, calculates the rank sum of each sample and compares the difference in the rank sums.
Mann-Whitney test
It is used to test the null hypothesis that two samples have the same median or, alternatively, whether observations in one sample tend to be larger than observations in the other.
Mann–Whitney test compares all data (xi) belonging to the X group and all data (yi) belonging to the Y group and calculates the probability of xi being greater than yi: P (xi > yi). The null hypothesis states that P (xi > yi) = P (xi < yi) =1/2 while the alternative hypothesis states that P (xi > yi) ≠1/2.
Kolmogorov-Smirnov test
The two-sample Kolmogorov-Smirnov (KS) test was designed as a generic method to test whether two random samples are drawn from the same distribution. The null hypothesis of the KS test is that both distributions are identical. The statistic of the KS test is a distance between the two empirical distributions, computed as the maximum absolute difference between their cumulative curves.
Kruskal-Wallis test
The Kruskal–Wallis test is a non-parametric test to analyse the variance.[ 14 ] It analyses if there is any difference in the median values of three or more independent samples. The data values are ranked in an increasing order, and the rank sums calculated followed by calculation of the test statistic.
Jonckheere test
In contrast to Kruskal–Wallis test, in Jonckheere test, there is an a priori ordering that gives it a more statistical power than the Kruskal–Wallis test.[ 14 ]
Friedman test
The Friedman test is a non-parametric test for testing the difference between several related samples. The Friedman test is an alternative for repeated measures ANOVAs which is used when the same parameter has been measured under different conditions on the same subjects.[ 13 ]
Tests to analyse the categorical data
Chi-square test, Fischer's exact test and McNemar's test are used to analyse the categorical or nominal variables. The Chi-square test compares the frequencies and tests whether the observed data differ significantly from that of the expected data if there were no differences between groups (i.e., the null hypothesis). It is calculated by the sum of the squared difference between observed ( O ) and the expected ( E ) data (or the deviation, d ) divided by the expected data by the following formula:

A Yates correction factor is used when the sample size is small. Fischer's exact test is used to determine if there are non-random associations between two categorical variables. It does not assume random sampling, and instead of referring a calculated statistic to a sampling distribution, it calculates an exact probability. McNemar's test is used for paired nominal data. It is applied to 2 × 2 table with paired-dependent samples. It is used to determine whether the row and column frequencies are equal (that is, whether there is ‘marginal homogeneity’). The null hypothesis is that the paired proportions are equal. The Mantel-Haenszel Chi-square test is a multivariate test as it analyses multiple grouping variables. It stratifies according to the nominated confounding variables and identifies any that affects the primary outcome variable. If the outcome variable is dichotomous, then logistic regression is used.
SOFTWARES AVAILABLE FOR STATISTICS, SAMPLE SIZE CALCULATION AND POWER ANALYSIS
Numerous statistical software systems are available currently. The commonly used software systems are Statistical Package for the Social Sciences (SPSS – manufactured by IBM corporation), Statistical Analysis System ((SAS – developed by SAS Institute North Carolina, United States of America), R (designed by Ross Ihaka and Robert Gentleman from R core team), Minitab (developed by Minitab Inc), Stata (developed by StataCorp) and the MS Excel (developed by Microsoft).
There are a number of web resources which are related to statistical power analyses. A few are:
- StatPages.net – provides links to a number of online power calculators
- G-Power – provides a downloadable power analysis program that runs under DOS
- Power analysis for ANOVA designs an interactive site that calculates power or sample size needed to attain a given power for one effect in a factorial ANOVA design
- SPSS makes a program called SamplePower. It gives an output of a complete report on the computer screen which can be cut and paste into another document.
It is important that a researcher knows the concepts of the basic statistical methods used for conduct of a research study. This will help to conduct an appropriately well-designed study leading to valid and reliable results. Inappropriate use of statistical techniques may lead to faulty conclusions, inducing errors and undermining the significance of the article. Bad statistics may lead to bad research, and bad research may lead to unethical practice. Hence, an adequate knowledge of statistics and the appropriate use of statistical tests are important. An appropriate knowledge about the basic statistical methods will go a long way in improving the research designs and producing quality medical research which can be utilised for formulating the evidence-based guidelines.
Financial support and sponsorship
Conflicts of interest.
There are no conflicts of interest.
Root out friction in every digital experience, super-charge conversion rates, and optimize digital self-service
Uncover insights from any interaction, deliver AI-powered agent coaching, and reduce cost to serve
Increase revenue and loyalty with real-time insights and recommendations delivered to teams on the ground
Know how your people feel and empower managers to improve employee engagement, productivity, and retention
Take action in the moments that matter most along the employee journey and drive bottom line growth
Whatever they’re are saying, wherever they’re saying it, know exactly what’s going on with your people
Get faster, richer insights with qual and quant tools that make powerful market research available to everyone
Run concept tests, pricing studies, prototyping + more with fast, powerful studies designed by UX research experts
Track your brand performance 24/7 and act quickly to respond to opportunities and challenges in your market
Explore the platform powering Experience Management
- Free Account
- For Digital
- For Customer Care
- For Human Resources
- For Researchers
- Financial Services
- All Industries
Popular Use Cases
- Customer Experience
- Employee Experience
- Net Promoter Score
- Voice of Customer
- Customer Success Hub
- Product Documentation
- Training & Certification
- XM Institute
- Popular Resources
- Customer Stories
- Artificial Intelligence
Market Research
- Partnerships
- Marketplace
The annual gathering of the experience leaders at the world’s iconic brands building breakthrough business results, live in Salt Lake City.
- English/AU & NZ
- Español/Europa
- Español/América Latina
- Português Brasileiro
- REQUEST DEMO
- Experience Management
- Descriptive Research
Try Qualtrics for free
Descriptive research: what it is and how to use it.
8 min read Understanding the who, what and where of a situation or target group is an essential part of effective research and making informed business decisions.
For example you might want to understand what percentage of CEOs have a bachelor’s degree or higher. Or you might want to understand what percentage of low income families receive government support – or what kind of support they receive.
Descriptive research is what will be used in these types of studies.
In this guide we’ll look through the main issues relating to descriptive research to give you a better understanding of what it is, and how and why you can use it.
Free eBook: 2024 global market research trends report
What is descriptive research?
Descriptive research is a research method used to try and determine the characteristics of a population or particular phenomenon.
Using descriptive research you can identify patterns in the characteristics of a group to essentially establish everything you need to understand apart from why something has happened.
Market researchers use descriptive research for a range of commercial purposes to guide key decisions.
For example you could use descriptive research to understand fashion trends in a given city when planning your clothing collection for the year. Using descriptive research you can conduct in depth analysis on the demographic makeup of your target area and use the data analysis to establish buying patterns.
Conducting descriptive research wouldn’t, however, tell you why shoppers are buying a particular type of fashion item.
Descriptive research design
Descriptive research design uses a range of both qualitative research and quantitative data (although quantitative research is the primary research method) to gather information to make accurate predictions about a particular problem or hypothesis.
As a survey method, descriptive research designs will help researchers identify characteristics in their target market or particular population.
These characteristics in the population sample can be identified, observed and measured to guide decisions.
Descriptive research characteristics
While there are a number of descriptive research methods you can deploy for data collection, descriptive research does have a number of predictable characteristics.
Here are a few of the things to consider:
Measure data trends with statistical outcomes
Descriptive research is often popular for survey research because it generates answers in a statistical form, which makes it easy for researchers to carry out a simple statistical analysis to interpret what the data is saying.
Descriptive research design is ideal for further research
Because the data collection for descriptive research produces statistical outcomes, it can also be used as secondary data for another research study.
Plus, the data collected from descriptive research can be subjected to other types of data analysis .
Uncontrolled variables
A key component of the descriptive research method is that it uses random variables that are not controlled by the researchers. This is because descriptive research aims to understand the natural behavior of the research subject.
It’s carried out in a natural environment
Descriptive research is often carried out in a natural environment. This is because researchers aim to gather data in a natural setting to avoid swaying respondents.
Data can be gathered using survey questions or online surveys.
For example, if you want to understand the fashion trends we mentioned earlier, you would set up a study in which a researcher observes people in the respondent’s natural environment to understand their habits and preferences.
Descriptive research allows for cross sectional study
Because of the nature of descriptive research design and the randomness of the sample group being observed, descriptive research is ideal for cross sectional studies – essentially the demographics of the group can vary widely and your aim is to gain insights from within the group.
This can be highly beneficial when you’re looking to understand the behaviors or preferences of a wider population.
Descriptive research advantages
There are many advantages to using descriptive research, some of them include:
Cost effectiveness
Because the elements needed for descriptive research design are not specific or highly targeted (and occur within the respondent’s natural environment) this type of study is relatively cheap to carry out.
Multiple types of data can be collected
A big advantage of this research type, is that you can use it to collect both quantitative and qualitative data. This means you can use the stats gathered to easily identify underlying patterns in your respondents’ behavior.
Descriptive research disadvantages
Potential reliability issues.
When conducting descriptive research it’s important that the initial survey questions are properly formulated.
If not, it could make the answers unreliable and risk the credibility of your study.
Potential limitations
As we’ve mentioned, descriptive research design is ideal for understanding the what, who or where of a situation or phenomenon.
However, it can’t help you understand the cause or effect of the behavior. This means you’ll need to conduct further research to get a more complete picture of a situation.
Descriptive research methods
Because descriptive research methods include a range of quantitative and qualitative research, there are several research methods you can use.
Use case studies
Case studies in descriptive research involve conducting in-depth and detailed studies in which researchers get a specific person or case to answer questions.
Case studies shouldn’t be used to generate results, rather it should be used to build or establish hypothesis that you can expand into further market research .
For example you could gather detailed data about a specific business phenomenon, and then use this deeper understanding of that specific case.
Use observational methods
This type of study uses qualitative observations to understand human behavior within a particular group.
By understanding how the different demographics respond within your sample you can identify patterns and trends.
As an observational method, descriptive research will not tell you the cause of any particular behaviors, but that could be established with further research.
Use survey research
Surveys are one of the most cost effective ways to gather descriptive data.
An online survey or questionnaire can be used in descriptive studies to gather quantitative information about a particular problem.
Survey research is ideal if you’re using descriptive research as your primary research.
Descriptive research examples
Descriptive research is used for a number of commercial purposes or when organizations need to understand the behaviors or opinions of a population.
One of the biggest examples of descriptive research that is used in every democratic country, is during elections.
Using descriptive research, researchers will use surveys to understand who voters are more likely to choose out of the parties or candidates available.
Using the data provided, researchers can analyze the data to understand what the election result will be.
In a commercial setting, retailers often use descriptive research to figure out trends in shopping and buying decisions.
By gathering information on the habits of shoppers, retailers can get a better understanding of the purchases being made.
Another example that is widely used around the world, is the national census that takes place to understand the population.
The research will provide a more accurate picture of a population’s demographic makeup and help to understand changes over time in areas like population age, health and education level.
Where Qualtrics helps with descriptive research
Whatever type of research you want to carry out, there’s a survey type that will work.
Qualtrics can help you determine the appropriate method and ensure you design a study that will deliver the insights you need.
Our experts can help you with your market research needs , ensuring you get the most out of Qualtrics market research software to design, launch and analyze your data to guide better, more accurate decisions for your organization.
Related resources
Market intelligence 10 min read, marketing insights 11 min read, ethnographic research 11 min read, qualitative vs quantitative research 13 min read, qualitative research questions 11 min read, qualitative research design 12 min read, primary vs secondary research 14 min read, request demo.
Ready to learn more about Qualtrics?

Bridging the Gap: Overcome these 7 flaws in descriptive research design
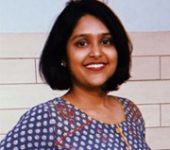
Descriptive research design is a powerful tool used by scientists and researchers to gather information about a particular group or phenomenon. This type of research provides a detailed and accurate picture of the characteristics and behaviors of a particular population or subject. By observing and collecting data on a given topic, descriptive research helps researchers gain a deeper understanding of a specific issue and provides valuable insights that can inform future studies.
In this blog, we will explore the definition, characteristics, and common flaws in descriptive research design, and provide tips on how to avoid these pitfalls to produce high-quality results. Whether you are a seasoned researcher or a student just starting, understanding the fundamentals of descriptive research design is essential to conducting successful scientific studies.
Table of Contents
What Is Descriptive Research Design?
The descriptive research design involves observing and collecting data on a given topic without attempting to infer cause-and-effect relationships. The goal of descriptive research is to provide a comprehensive and accurate picture of the population or phenomenon being studied and to describe the relationships, patterns, and trends that exist within the data.
Descriptive research methods can include surveys, observational studies , and case studies, and the data collected can be qualitative or quantitative . The findings from descriptive research provide valuable insights and inform future research, but do not establish cause-and-effect relationships.
Importance of Descriptive Research in Scientific Studies
1. understanding of a population or phenomenon.
Descriptive research provides a comprehensive picture of the characteristics and behaviors of a particular population or phenomenon, allowing researchers to gain a deeper understanding of the topic.
2. Baseline Information
The information gathered through descriptive research can serve as a baseline for future research and provide a foundation for further studies.
3. Informative Data
Descriptive research can provide valuable information and insights into a particular topic, which can inform future research, policy decisions, and programs.
4. Sampling Validation
Descriptive research can be used to validate sampling methods and to help researchers determine the best approach for their study.
5. Cost Effective
Descriptive research is often less expensive and less time-consuming than other research methods , making it a cost-effective way to gather information about a particular population or phenomenon.
6. Easy to Replicate
Descriptive research is straightforward to replicate, making it a reliable way to gather and compare information from multiple sources.
Key Characteristics of Descriptive Research Design
The primary purpose of descriptive research is to describe the characteristics, behaviors, and attributes of a particular population or phenomenon.
2. Participants and Sampling
Descriptive research studies a particular population or sample that is representative of the larger population being studied. Furthermore, sampling methods can include convenience, stratified, or random sampling.
3. Data Collection Techniques
Descriptive research typically involves the collection of both qualitative and quantitative data through methods such as surveys, observational studies, case studies, or focus groups.
4. Data Analysis
Descriptive research data is analyzed to identify patterns, relationships, and trends within the data. Statistical techniques , such as frequency distributions and descriptive statistics, are commonly used to summarize and describe the data.
5. Focus on Description
Descriptive research is focused on describing and summarizing the characteristics of a particular population or phenomenon. It does not make causal inferences.
6. Non-Experimental
Descriptive research is non-experimental, meaning that the researcher does not manipulate variables or control conditions. The researcher simply observes and collects data on the population or phenomenon being studied.
When Can a Researcher Conduct Descriptive Research?
A researcher can conduct descriptive research in the following situations:
- To better understand a particular population or phenomenon
- To describe the relationships between variables
- To describe patterns and trends
- To validate sampling methods and determine the best approach for a study
- To compare data from multiple sources.
Types of Descriptive Research Design
1. survey research.
Surveys are a type of descriptive research that involves collecting data through self-administered or interviewer-administered questionnaires. Additionally, they can be administered in-person, by mail, or online, and can collect both qualitative and quantitative data.
2. Observational Research
Observational research involves observing and collecting data on a particular population or phenomenon without manipulating variables or controlling conditions. It can be conducted in naturalistic settings or controlled laboratory settings.
3. Case Study Research
Case study research is a type of descriptive research that focuses on a single individual, group, or event. It involves collecting detailed information on the subject through a variety of methods, including interviews, observations, and examination of documents.
4. Focus Group Research
Focus group research involves bringing together a small group of people to discuss a particular topic or product. Furthermore, the group is usually moderated by a researcher and the discussion is recorded for later analysis.
5. Ethnographic Research
Ethnographic research involves conducting detailed observations of a particular culture or community. It is often used to gain a deep understanding of the beliefs, behaviors, and practices of a particular group.
Advantages of Descriptive Research Design
1. provides a comprehensive understanding.
Descriptive research provides a comprehensive picture of the characteristics, behaviors, and attributes of a particular population or phenomenon, which can be useful in informing future research and policy decisions.
2. Non-invasive
Descriptive research is non-invasive and does not manipulate variables or control conditions, making it a suitable method for sensitive or ethical concerns.
3. Flexibility
Descriptive research allows for a wide range of data collection methods , including surveys, observational studies, case studies, and focus groups, making it a flexible and versatile research method.
4. Cost-effective
Descriptive research is often less expensive and less time-consuming than other research methods. Moreover, it gives a cost-effective option to many researchers.
5. Easy to Replicate
Descriptive research is easy to replicate, making it a reliable way to gather and compare information from multiple sources.
6. Informs Future Research
The insights gained from a descriptive research can inform future research and inform policy decisions and programs.
Disadvantages of Descriptive Research Design
1. limited scope.
Descriptive research only provides a snapshot of the current situation and cannot establish cause-and-effect relationships.
2. Dependence on Existing Data
Descriptive research relies on existing data, which may not always be comprehensive or accurate.
3. Lack of Control
Researchers have no control over the variables in descriptive research, which can limit the conclusions that can be drawn.
The researcher’s own biases and preconceptions can influence the interpretation of the data.
5. Lack of Generalizability
Descriptive research findings may not be applicable to other populations or situations.
6. Lack of Depth
Descriptive research provides a surface-level understanding of a phenomenon, rather than a deep understanding.
7. Time-consuming
Descriptive research often requires a large amount of data collection and analysis, which can be time-consuming and resource-intensive.
7 Ways to Avoid Common Flaws While Designing Descriptive Research
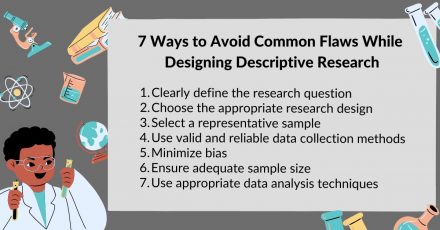
1. Clearly define the research question
A clearly defined research question is the foundation of any research study, and it is important to ensure that the question is both specific and relevant to the topic being studied.
2. Choose the appropriate research design
Choosing the appropriate research design for a study is crucial to the success of the study. Moreover, researchers should choose a design that best fits the research question and the type of data needed to answer it.
3. Select a representative sample
Selecting a representative sample is important to ensure that the findings of the study are generalizable to the population being studied. Researchers should use a sampling method that provides a random and representative sample of the population.
4. Use valid and reliable data collection methods
Using valid and reliable data collection methods is important to ensure that the data collected is accurate and can be used to answer the research question. Researchers should choose methods that are appropriate for the study and that can be administered consistently and systematically.
5. Minimize bias
Bias can significantly impact the validity and reliability of research findings. Furthermore, it is important to minimize bias in all aspects of the study, from the selection of participants to the analysis of data.
6. Ensure adequate sample size
An adequate sample size is important to ensure that the results of the study are statistically significant and can be generalized to the population being studied.
7. Use appropriate data analysis techniques
The appropriate data analysis technique depends on the type of data collected and the research question being asked. Researchers should choose techniques that are appropriate for the data and the question being asked.
Have you worked on descriptive research designs? How was your experience creating a descriptive design? What challenges did you face? Do write to us or leave a comment below and share your insights on descriptive research designs!

extremely very educative
Indeed very educative and useful. Well explained. Thank you
Simple,easy to understand
Rate this article Cancel Reply
Your email address will not be published.
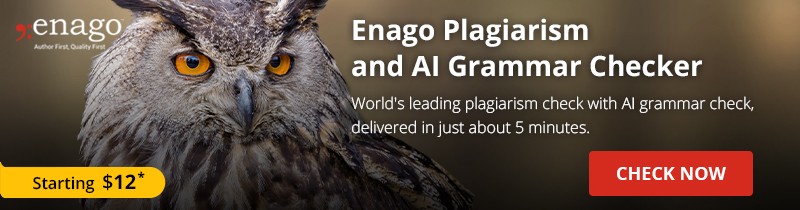
Enago Academy's Most Popular Articles
![analysis of data descriptive research What is Academic Integrity and How to Uphold it [FREE CHECKLIST]](https://www.enago.com/academy/wp-content/uploads/2024/05/FeatureImages-59-210x136.png)
Ensuring Academic Integrity and Transparency in Academic Research: A comprehensive checklist for researchers
Academic integrity is the foundation upon which the credibility and value of scientific findings are…
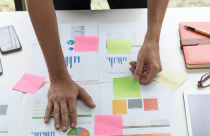
- Publishing Research
- Reporting Research
How to Optimize Your Research Process: A step-by-step guide
For researchers across disciplines, the path to uncovering novel findings and insights is often filled…
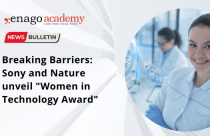
- Industry News
- Trending Now
Breaking Barriers: Sony and Nature unveil “Women in Technology Award”
Sony Group Corporation and the prestigious scientific journal Nature have collaborated to launch the inaugural…
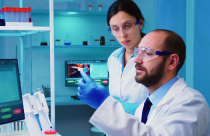
Achieving Research Excellence: Checklist for good research practices
Academia is built on the foundation of trustworthy and high-quality research, supported by the pillars…
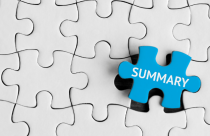
- Promoting Research
Plain Language Summary — Communicating your research to bridge the academic-lay gap
Science can be complex, but does that mean it should not be accessible to the…
Choosing the Right Analytical Approach: Thematic analysis vs. content analysis for…
Comparing Cross Sectional and Longitudinal Studies: 5 steps for choosing the right…
Research Recommendations – Guiding policy-makers for evidence-based decision making

Sign-up to read more
Subscribe for free to get unrestricted access to all our resources on research writing and academic publishing including:
- 2000+ blog articles
- 50+ Webinars
- 10+ Expert podcasts
- 50+ Infographics
- 10+ Checklists
- Research Guides
We hate spam too. We promise to protect your privacy and never spam you.
I am looking for Editing/ Proofreading services for my manuscript Tentative date of next journal submission:
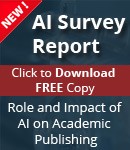
As a researcher, what do you consider most when choosing an image manipulation detector?
- Skip to main content
- Skip to primary sidebar
- Skip to footer
- QuestionPro

- Solutions Industries Gaming Automotive Sports and events Education Government Travel & Hospitality Financial Services Healthcare Cannabis Technology Use Case NPS+ Communities Audience Contactless surveys Mobile LivePolls Member Experience GDPR Positive People Science 360 Feedback Surveys
- Resources Blog eBooks Survey Templates Case Studies Training Help center

Home Market Research
Data Analysis in Research: Types & Methods
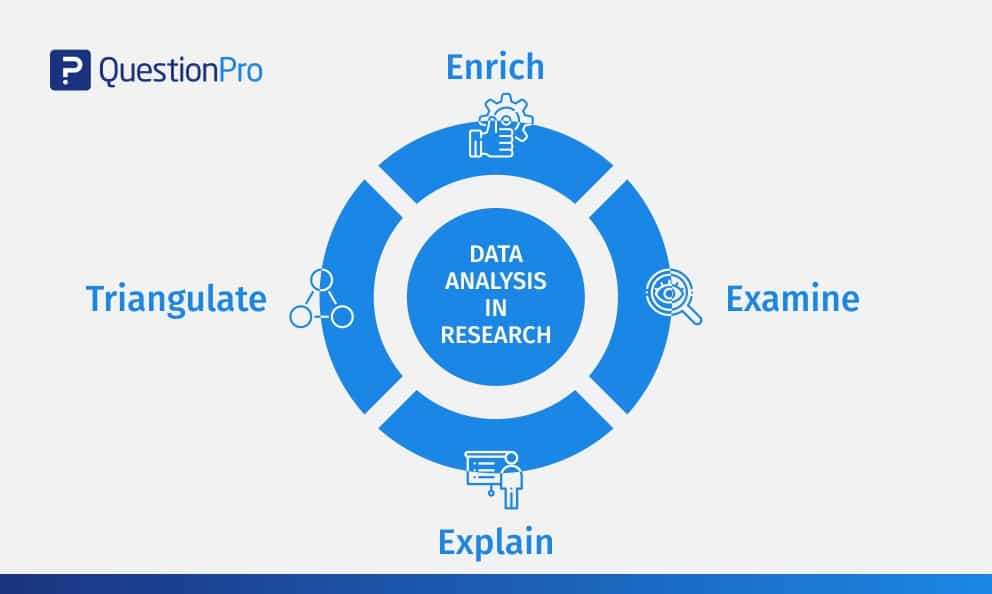
Content Index
Why analyze data in research?
Types of data in research, finding patterns in the qualitative data, methods used for data analysis in qualitative research, preparing data for analysis, methods used for data analysis in quantitative research, considerations in research data analysis, what is data analysis in research.
Definition of research in data analysis: According to LeCompte and Schensul, research data analysis is a process used by researchers to reduce data to a story and interpret it to derive insights. The data analysis process helps reduce a large chunk of data into smaller fragments, which makes sense.
Three essential things occur during the data analysis process — the first is data organization . Summarization and categorization together contribute to becoming the second known method used for data reduction. It helps find patterns and themes in the data for easy identification and linking. The third and last way is data analysis – researchers do it in both top-down and bottom-up fashion.
LEARN ABOUT: Research Process Steps
On the other hand, Marshall and Rossman describe data analysis as a messy, ambiguous, and time-consuming but creative and fascinating process through which a mass of collected data is brought to order, structure and meaning.
We can say that “the data analysis and data interpretation is a process representing the application of deductive and inductive logic to the research and data analysis.”
Researchers rely heavily on data as they have a story to tell or research problems to solve. It starts with a question, and data is nothing but an answer to that question. But, what if there is no question to ask? Well! It is possible to explore data even without a problem – we call it ‘Data Mining’, which often reveals some interesting patterns within the data that are worth exploring.
Irrelevant to the type of data researchers explore, their mission and audiences’ vision guide them to find the patterns to shape the story they want to tell. One of the essential things expected from researchers while analyzing data is to stay open and remain unbiased toward unexpected patterns, expressions, and results. Remember, sometimes, data analysis tells the most unforeseen yet exciting stories that were not expected when initiating data analysis. Therefore, rely on the data you have at hand and enjoy the journey of exploratory research.
Create a Free Account
Every kind of data has a rare quality of describing things after assigning a specific value to it. For analysis, you need to organize these values, processed and presented in a given context, to make it useful. Data can be in different forms; here are the primary data types.
- Qualitative data: When the data presented has words and descriptions, then we call it qualitative data . Although you can observe this data, it is subjective and harder to analyze data in research, especially for comparison. Example: Quality data represents everything describing taste, experience, texture, or an opinion that is considered quality data. This type of data is usually collected through focus groups, personal qualitative interviews , qualitative observation or using open-ended questions in surveys.
- Quantitative data: Any data expressed in numbers of numerical figures are called quantitative data . This type of data can be distinguished into categories, grouped, measured, calculated, or ranked. Example: questions such as age, rank, cost, length, weight, scores, etc. everything comes under this type of data. You can present such data in graphical format, charts, or apply statistical analysis methods to this data. The (Outcomes Measurement Systems) OMS questionnaires in surveys are a significant source of collecting numeric data.
- Categorical data: It is data presented in groups. However, an item included in the categorical data cannot belong to more than one group. Example: A person responding to a survey by telling his living style, marital status, smoking habit, or drinking habit comes under the categorical data. A chi-square test is a standard method used to analyze this data.
Learn More : Examples of Qualitative Data in Education

Data analysis in qualitative research
Data analysis and qualitative data research work a little differently from the numerical data as the quality data is made up of words, descriptions, images, objects, and sometimes symbols. Getting insight from such complicated information is a complicated process. Hence it is typically used for exploratory research and data analysis .
Although there are several ways to find patterns in the textual information, a word-based method is the most relied and widely used global technique for research and data analysis. Notably, the data analysis process in qualitative research is manual. Here the researchers usually read the available data and find repetitive or commonly used words.
For example, while studying data collected from African countries to understand the most pressing issues people face, researchers might find “food” and “hunger” are the most commonly used words and will highlight them for further analysis.
LEARN ABOUT: Level of Analysis
The keyword context is another widely used word-based technique. In this method, the researcher tries to understand the concept by analyzing the context in which the participants use a particular keyword.
For example , researchers conducting research and data analysis for studying the concept of ‘diabetes’ amongst respondents might analyze the context of when and how the respondent has used or referred to the word ‘diabetes.’
The scrutiny-based technique is also one of the highly recommended text analysis methods used to identify a quality data pattern. Compare and contrast is the widely used method under this technique to differentiate how a specific text is similar or different from each other.
For example: To find out the “importance of resident doctor in a company,” the collected data is divided into people who think it is necessary to hire a resident doctor and those who think it is unnecessary. Compare and contrast is the best method that can be used to analyze the polls having single-answer questions types .
Metaphors can be used to reduce the data pile and find patterns in it so that it becomes easier to connect data with theory.
Variable Partitioning is another technique used to split variables so that researchers can find more coherent descriptions and explanations from the enormous data.
LEARN ABOUT: Qualitative Research Questions and Questionnaires
There are several techniques to analyze the data in qualitative research, but here are some commonly used methods,
- Content Analysis: It is widely accepted and the most frequently employed technique for data analysis in research methodology. It can be used to analyze the documented information from text, images, and sometimes from the physical items. It depends on the research questions to predict when and where to use this method.
- Narrative Analysis: This method is used to analyze content gathered from various sources such as personal interviews, field observation, and surveys . The majority of times, stories, or opinions shared by people are focused on finding answers to the research questions.
- Discourse Analysis: Similar to narrative analysis, discourse analysis is used to analyze the interactions with people. Nevertheless, this particular method considers the social context under which or within which the communication between the researcher and respondent takes place. In addition to that, discourse analysis also focuses on the lifestyle and day-to-day environment while deriving any conclusion.
- Grounded Theory: When you want to explain why a particular phenomenon happened, then using grounded theory for analyzing quality data is the best resort. Grounded theory is applied to study data about the host of similar cases occurring in different settings. When researchers are using this method, they might alter explanations or produce new ones until they arrive at some conclusion.
LEARN ABOUT: 12 Best Tools for Researchers
Data analysis in quantitative research
The first stage in research and data analysis is to make it for the analysis so that the nominal data can be converted into something meaningful. Data preparation consists of the below phases.
Phase I: Data Validation
Data validation is done to understand if the collected data sample is per the pre-set standards, or it is a biased data sample again divided into four different stages
- Fraud: To ensure an actual human being records each response to the survey or the questionnaire
- Screening: To make sure each participant or respondent is selected or chosen in compliance with the research criteria
- Procedure: To ensure ethical standards were maintained while collecting the data sample
- Completeness: To ensure that the respondent has answered all the questions in an online survey. Else, the interviewer had asked all the questions devised in the questionnaire.
Phase II: Data Editing
More often, an extensive research data sample comes loaded with errors. Respondents sometimes fill in some fields incorrectly or sometimes skip them accidentally. Data editing is a process wherein the researchers have to confirm that the provided data is free of such errors. They need to conduct necessary checks and outlier checks to edit the raw edit and make it ready for analysis.
Phase III: Data Coding
Out of all three, this is the most critical phase of data preparation associated with grouping and assigning values to the survey responses . If a survey is completed with a 1000 sample size, the researcher will create an age bracket to distinguish the respondents based on their age. Thus, it becomes easier to analyze small data buckets rather than deal with the massive data pile.
LEARN ABOUT: Steps in Qualitative Research
After the data is prepared for analysis, researchers are open to using different research and data analysis methods to derive meaningful insights. For sure, statistical analysis plans are the most favored to analyze numerical data. In statistical analysis, distinguishing between categorical data and numerical data is essential, as categorical data involves distinct categories or labels, while numerical data consists of measurable quantities. The method is again classified into two groups. First, ‘Descriptive Statistics’ used to describe data. Second, ‘Inferential statistics’ that helps in comparing the data .
Descriptive statistics
This method is used to describe the basic features of versatile types of data in research. It presents the data in such a meaningful way that pattern in the data starts making sense. Nevertheless, the descriptive analysis does not go beyond making conclusions. The conclusions are again based on the hypothesis researchers have formulated so far. Here are a few major types of descriptive analysis methods.
Measures of Frequency
- Count, Percent, Frequency
- It is used to denote home often a particular event occurs.
- Researchers use it when they want to showcase how often a response is given.
Measures of Central Tendency
- Mean, Median, Mode
- The method is widely used to demonstrate distribution by various points.
- Researchers use this method when they want to showcase the most commonly or averagely indicated response.
Measures of Dispersion or Variation
- Range, Variance, Standard deviation
- Here the field equals high/low points.
- Variance standard deviation = difference between the observed score and mean
- It is used to identify the spread of scores by stating intervals.
- Researchers use this method to showcase data spread out. It helps them identify the depth until which the data is spread out that it directly affects the mean.
Measures of Position
- Percentile ranks, Quartile ranks
- It relies on standardized scores helping researchers to identify the relationship between different scores.
- It is often used when researchers want to compare scores with the average count.
For quantitative research use of descriptive analysis often give absolute numbers, but the in-depth analysis is never sufficient to demonstrate the rationale behind those numbers. Nevertheless, it is necessary to think of the best method for research and data analysis suiting your survey questionnaire and what story researchers want to tell. For example, the mean is the best way to demonstrate the students’ average scores in schools. It is better to rely on the descriptive statistics when the researchers intend to keep the research or outcome limited to the provided sample without generalizing it. For example, when you want to compare average voting done in two different cities, differential statistics are enough.
Descriptive analysis is also called a ‘univariate analysis’ since it is commonly used to analyze a single variable.
Inferential statistics
Inferential statistics are used to make predictions about a larger population after research and data analysis of the representing population’s collected sample. For example, you can ask some odd 100 audiences at a movie theater if they like the movie they are watching. Researchers then use inferential statistics on the collected sample to reason that about 80-90% of people like the movie.
Here are two significant areas of inferential statistics.
- Estimating parameters: It takes statistics from the sample research data and demonstrates something about the population parameter.
- Hypothesis test: I t’s about sampling research data to answer the survey research questions. For example, researchers might be interested to understand if the new shade of lipstick recently launched is good or not, or if the multivitamin capsules help children to perform better at games.
These are sophisticated analysis methods used to showcase the relationship between different variables instead of describing a single variable. It is often used when researchers want something beyond absolute numbers to understand the relationship between variables.
Here are some of the commonly used methods for data analysis in research.
- Correlation: When researchers are not conducting experimental research or quasi-experimental research wherein the researchers are interested to understand the relationship between two or more variables, they opt for correlational research methods.
- Cross-tabulation: Also called contingency tables, cross-tabulation is used to analyze the relationship between multiple variables. Suppose provided data has age and gender categories presented in rows and columns. A two-dimensional cross-tabulation helps for seamless data analysis and research by showing the number of males and females in each age category.
- Regression analysis: For understanding the strong relationship between two variables, researchers do not look beyond the primary and commonly used regression analysis method, which is also a type of predictive analysis used. In this method, you have an essential factor called the dependent variable. You also have multiple independent variables in regression analysis. You undertake efforts to find out the impact of independent variables on the dependent variable. The values of both independent and dependent variables are assumed as being ascertained in an error-free random manner.
- Frequency tables: The statistical procedure is used for testing the degree to which two or more vary or differ in an experiment. A considerable degree of variation means research findings were significant. In many contexts, ANOVA testing and variance analysis are similar.
- Analysis of variance: The statistical procedure is used for testing the degree to which two or more vary or differ in an experiment. A considerable degree of variation means research findings were significant. In many contexts, ANOVA testing and variance analysis are similar.
- Researchers must have the necessary research skills to analyze and manipulation the data , Getting trained to demonstrate a high standard of research practice. Ideally, researchers must possess more than a basic understanding of the rationale of selecting one statistical method over the other to obtain better data insights.
- Usually, research and data analytics projects differ by scientific discipline; therefore, getting statistical advice at the beginning of analysis helps design a survey questionnaire, select data collection methods , and choose samples.
LEARN ABOUT: Best Data Collection Tools
- The primary aim of data research and analysis is to derive ultimate insights that are unbiased. Any mistake in or keeping a biased mind to collect data, selecting an analysis method, or choosing audience sample il to draw a biased inference.
- Irrelevant to the sophistication used in research data and analysis is enough to rectify the poorly defined objective outcome measurements. It does not matter if the design is at fault or intentions are not clear, but lack of clarity might mislead readers, so avoid the practice.
- The motive behind data analysis in research is to present accurate and reliable data. As far as possible, avoid statistical errors, and find a way to deal with everyday challenges like outliers, missing data, data altering, data mining , or developing graphical representation.
LEARN MORE: Descriptive Research vs Correlational Research The sheer amount of data generated daily is frightening. Especially when data analysis has taken center stage. in 2018. In last year, the total data supply amounted to 2.8 trillion gigabytes. Hence, it is clear that the enterprises willing to survive in the hypercompetitive world must possess an excellent capability to analyze complex research data, derive actionable insights, and adapt to the new market needs.
LEARN ABOUT: Average Order Value
QuestionPro is an online survey platform that empowers organizations in data analysis and research and provides them a medium to collect data by creating appealing surveys.
MORE LIKE THIS
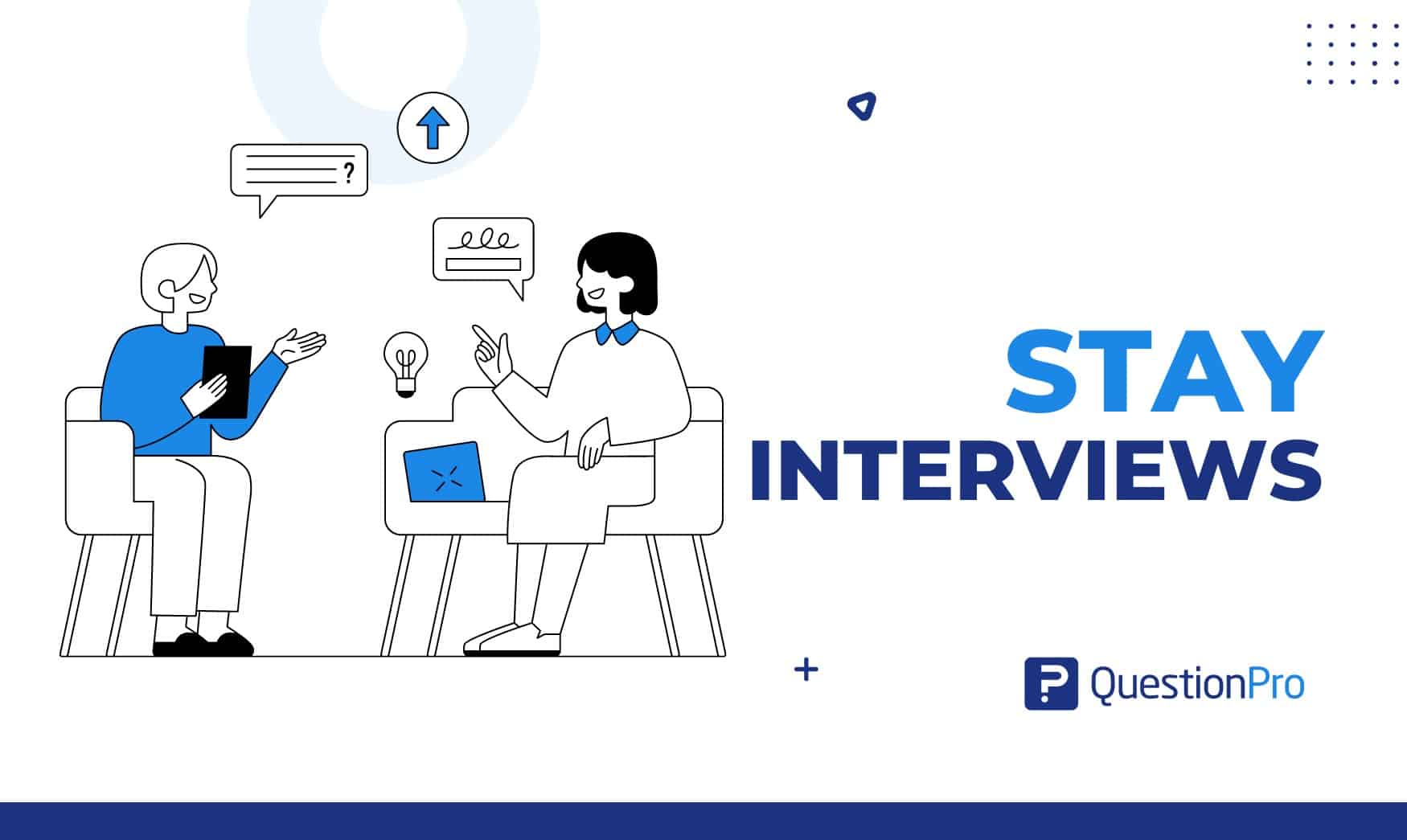
Stay Interviews: What Is It, How to Conduct, 15 Questions
Jun 11, 2024
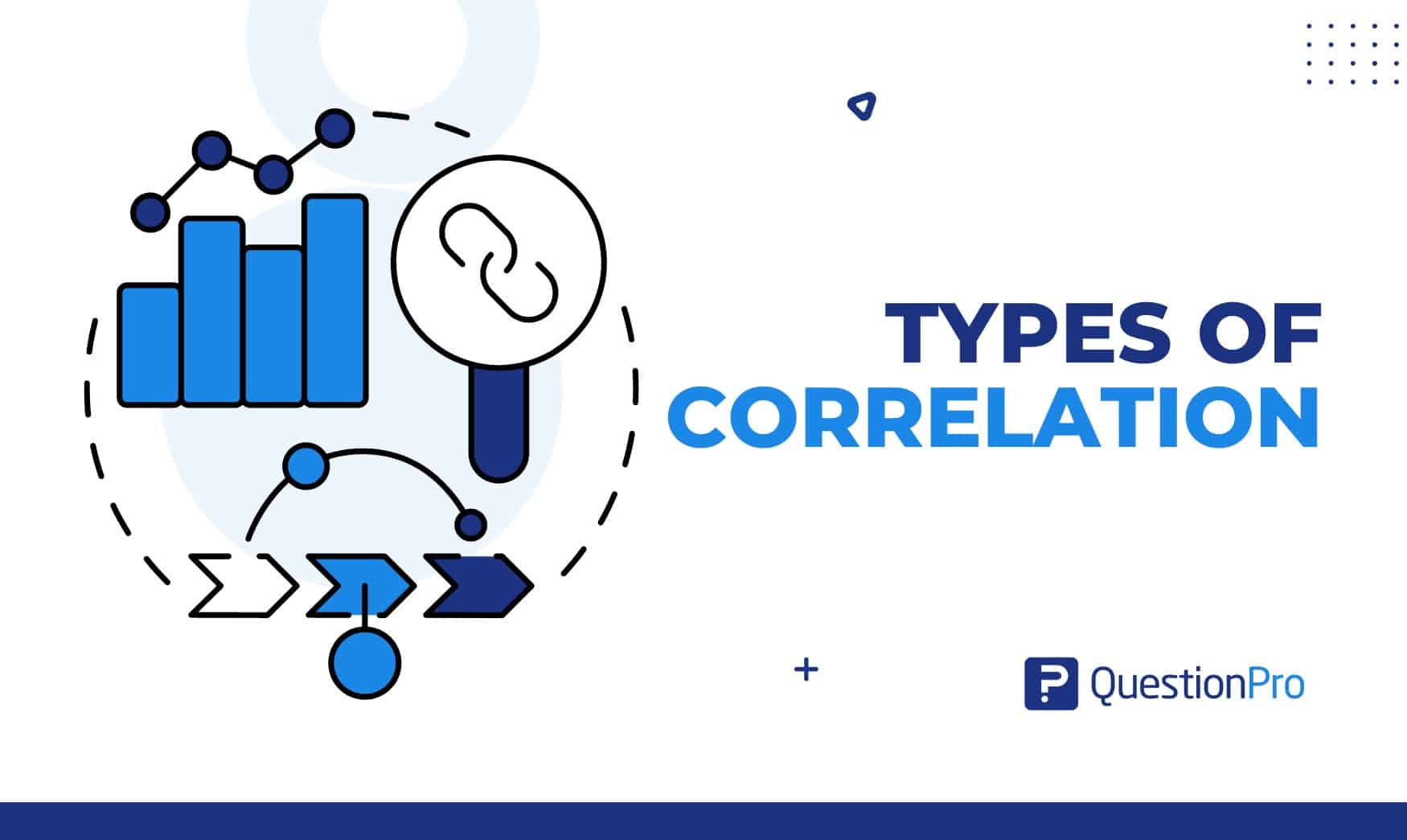
Exploring Types of Correlation for Patterns and Relationship
Jun 10, 2024
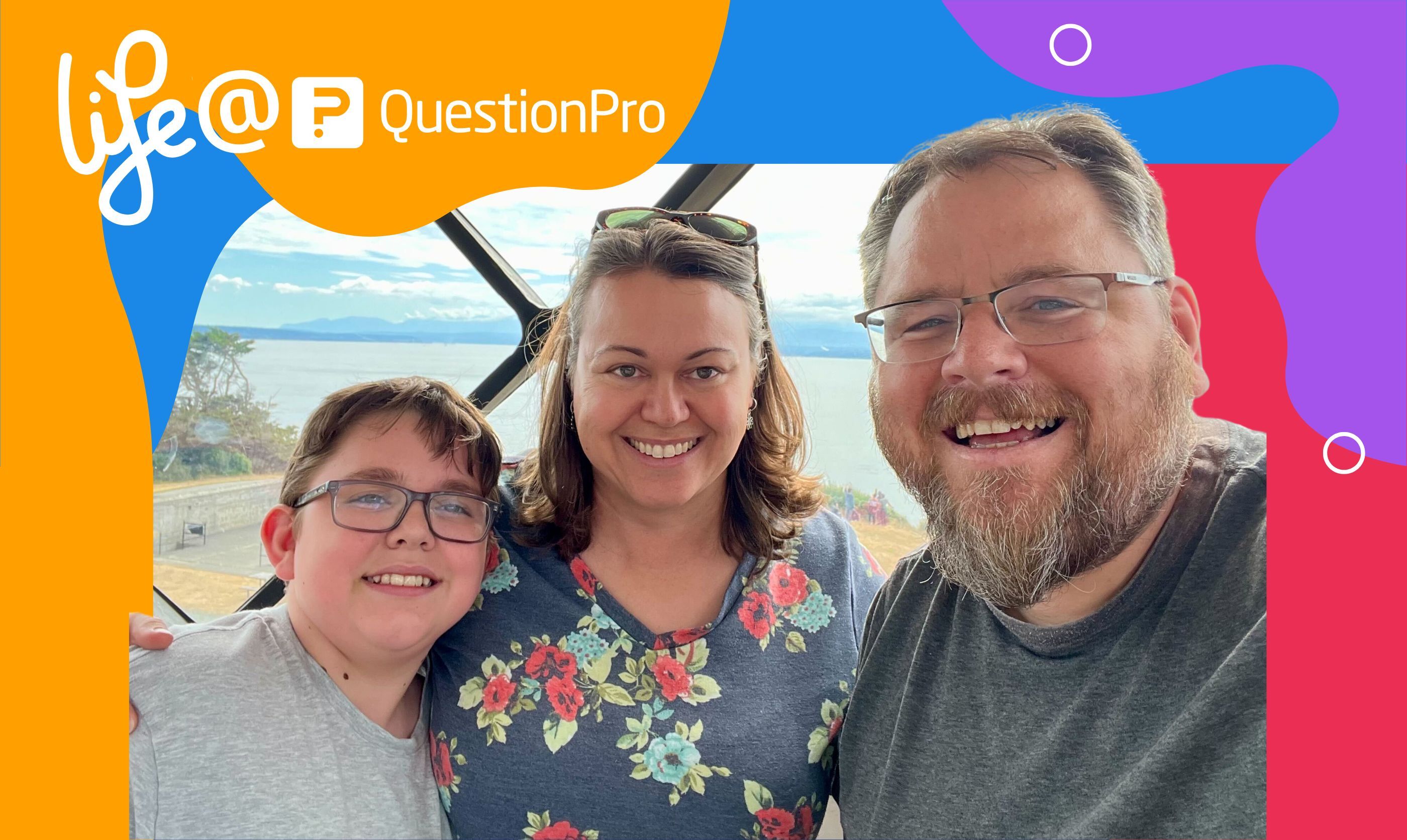
Life@QuestionPro: The Journey of Kristie Lawrence
Jun 7, 2024
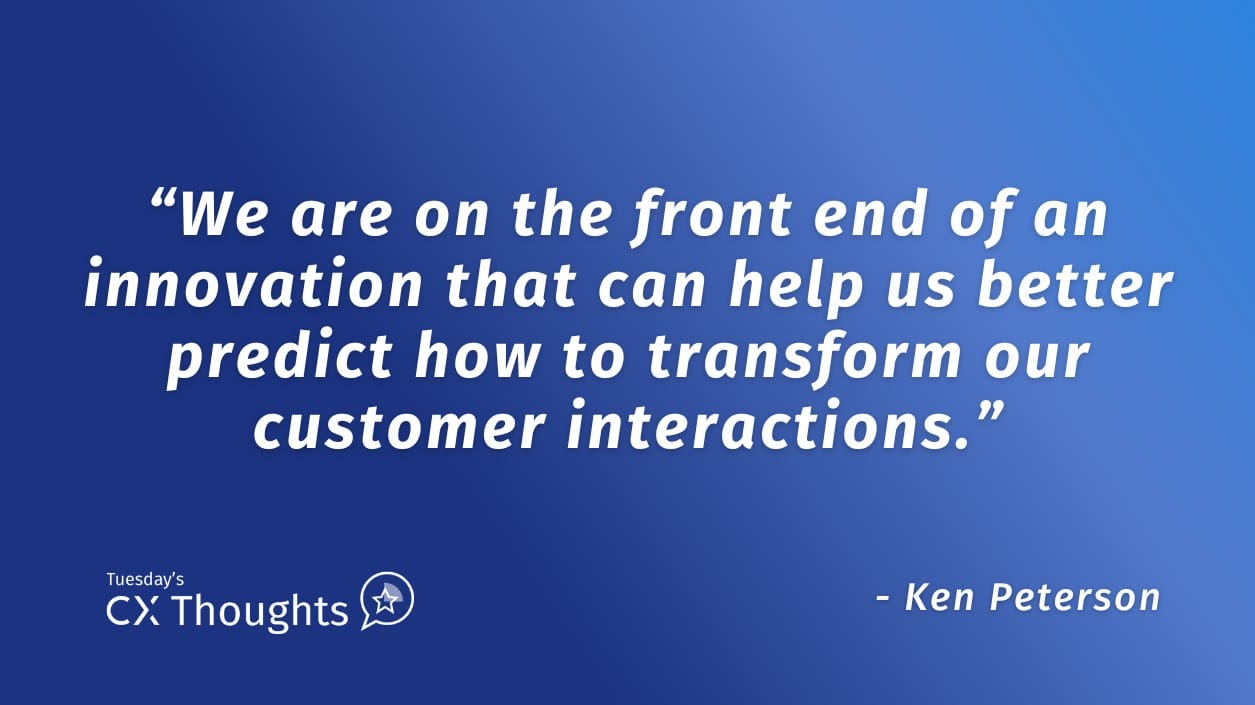
How Can I Help You? — Tuesday CX Thoughts
Jun 5, 2024
Other categories
- Academic Research
- Artificial Intelligence
- Assessments
- Brand Awareness
- Case Studies
- Communities
- Consumer Insights
- Customer effort score
- Customer Engagement
- Customer Experience
- Customer Loyalty
- Customer Research
- Customer Satisfaction
- Employee Benefits
- Employee Engagement
- Employee Retention
- Friday Five
- General Data Protection Regulation
- Insights Hub
- Life@QuestionPro
- Market Research
- Mobile diaries
- Mobile Surveys
- New Features
- Online Communities
- Question Types
- Questionnaire
- QuestionPro Products
- Release Notes
- Research Tools and Apps
- Revenue at Risk
- Survey Templates
- Training Tips
- Uncategorized
- Video Learning Series
- What’s Coming Up
- Workforce Intelligence
Child Care and Early Education Research Connections
Descriptive research studies.
Descriptive research is a type of research that is used to describe the characteristics of a population. It collects data that are used to answer a wide range of what, when, and how questions pertaining to a particular population or group. For example, descriptive studies might be used to answer questions such as: What percentage of Head Start teachers have a bachelor's degree or higher? What is the average reading ability of 5-year-olds when they first enter kindergarten? What kinds of math activities are used in early childhood programs? When do children first receive regular child care from someone other than their parents? When are children with developmental disabilities first diagnosed and when do they first receive services? What factors do programs consider when making decisions about the type of assessments that will be used to assess the skills of the children in their programs? How do the types of services children receive from their early childhood program change as children age?
Descriptive research does not answer questions about why a certain phenomenon occurs or what the causes are. Answers to such questions are best obtained from randomized and quasi-experimental studies . However, data from descriptive studies can be used to examine the relationships (correlations) among variables. While the findings from correlational analyses are not evidence of causality, they can help to distinguish variables that may be important in explaining a phenomenon from those that are not. Thus, descriptive research is often used to generate hypotheses that should be tested using more rigorous designs.
A variety of data collection methods may be used alone or in combination to answer the types of questions guiding descriptive research. Some of the more common methods include surveys, interviews, observations, case studies, and portfolios. The data collected through these methods can be either quantitative or qualitative. Quantitative data are typically analyzed and presenting using descriptive statistics . Using quantitative data, researchers may describe the characteristics of a sample or population in terms of percentages (e.g., percentage of population that belong to different racial/ethnic groups, percentage of low-income families that receive different government services) or averages (e.g., average household income, average scores of reading, mathematics and language assessments). Quantitative data, such as narrative data collected as part of a case study, may be used to organize, classify, and used to identify patterns of behaviors, attitudes, and other characteristics of groups.
Descriptive studies have an important role in early care and education research. Studies such as the National Survey of Early Care and Education and the National Household Education Surveys Program have greatly increased our knowledge of the supply of and demand for child care in the U.S. The Head Start Family and Child Experiences Survey and the Early Childhood Longitudinal Study Program have provided researchers, policy makers and practitioners with rich information about school readiness skills of children in the U.S.
Each of the methods used to collect descriptive data have their own strengths and limitations. The following are some of the strengths and limitations of descriptive research studies in general.
Study participants are questioned or observed in a natural setting (e.g., their homes, child care or educational settings).
Study data can be used to identify the prevalence of particular problems and the need for new or additional services to address these problems.
Descriptive research may identify areas in need of additional research and relationships between variables that require future study. Descriptive research is often referred to as "hypothesis generating research."
Depending on the data collection method used, descriptive studies can generate rich datasets on large and diverse samples.
Limitations:
Descriptive studies cannot be used to establish cause and effect relationships.
Respondents may not be truthful when answering survey questions or may give socially desirable responses.
The choice and wording of questions on a questionnaire may influence the descriptive findings.
Depending on the type and size of sample, the findings may not be generalizable or produce an accurate description of the population of interest.
Qualitative vs Quantitative Research Methods & Data Analysis
Saul Mcleod, PhD
Editor-in-Chief for Simply Psychology
BSc (Hons) Psychology, MRes, PhD, University of Manchester
Saul Mcleod, PhD., is a qualified psychology teacher with over 18 years of experience in further and higher education. He has been published in peer-reviewed journals, including the Journal of Clinical Psychology.
Learn about our Editorial Process
Olivia Guy-Evans, MSc
Associate Editor for Simply Psychology
BSc (Hons) Psychology, MSc Psychology of Education
Olivia Guy-Evans is a writer and associate editor for Simply Psychology. She has previously worked in healthcare and educational sectors.
On This Page:
What is the difference between quantitative and qualitative?
The main difference between quantitative and qualitative research is the type of data they collect and analyze.
Quantitative research collects numerical data and analyzes it using statistical methods. The aim is to produce objective, empirical data that can be measured and expressed in numerical terms. Quantitative research is often used to test hypotheses, identify patterns, and make predictions.
Qualitative research , on the other hand, collects non-numerical data such as words, images, and sounds. The focus is on exploring subjective experiences, opinions, and attitudes, often through observation and interviews.
Qualitative research aims to produce rich and detailed descriptions of the phenomenon being studied, and to uncover new insights and meanings.
Quantitative data is information about quantities, and therefore numbers, and qualitative data is descriptive, and regards phenomenon which can be observed but not measured, such as language.
What Is Qualitative Research?
Qualitative research is the process of collecting, analyzing, and interpreting non-numerical data, such as language. Qualitative research can be used to understand how an individual subjectively perceives and gives meaning to their social reality.
Qualitative data is non-numerical data, such as text, video, photographs, or audio recordings. This type of data can be collected using diary accounts or in-depth interviews and analyzed using grounded theory or thematic analysis.
Qualitative research is multimethod in focus, involving an interpretive, naturalistic approach to its subject matter. This means that qualitative researchers study things in their natural settings, attempting to make sense of, or interpret, phenomena in terms of the meanings people bring to them. Denzin and Lincoln (1994, p. 2)
Interest in qualitative data came about as the result of the dissatisfaction of some psychologists (e.g., Carl Rogers) with the scientific study of psychologists such as behaviorists (e.g., Skinner ).
Since psychologists study people, the traditional approach to science is not seen as an appropriate way of carrying out research since it fails to capture the totality of human experience and the essence of being human. Exploring participants’ experiences is known as a phenomenological approach (re: Humanism ).
Qualitative research is primarily concerned with meaning, subjectivity, and lived experience. The goal is to understand the quality and texture of people’s experiences, how they make sense of them, and the implications for their lives.
Qualitative research aims to understand the social reality of individuals, groups, and cultures as nearly as possible as participants feel or live it. Thus, people and groups are studied in their natural setting.
Some examples of qualitative research questions are provided, such as what an experience feels like, how people talk about something, how they make sense of an experience, and how events unfold for people.
Research following a qualitative approach is exploratory and seeks to explain ‘how’ and ‘why’ a particular phenomenon, or behavior, operates as it does in a particular context. It can be used to generate hypotheses and theories from the data.
Qualitative Methods
There are different types of qualitative research methods, including diary accounts, in-depth interviews , documents, focus groups , case study research , and ethnography.
The results of qualitative methods provide a deep understanding of how people perceive their social realities and in consequence, how they act within the social world.
The researcher has several methods for collecting empirical materials, ranging from the interview to direct observation, to the analysis of artifacts, documents, and cultural records, to the use of visual materials or personal experience. Denzin and Lincoln (1994, p. 14)
Here are some examples of qualitative data:
Interview transcripts : Verbatim records of what participants said during an interview or focus group. They allow researchers to identify common themes and patterns, and draw conclusions based on the data. Interview transcripts can also be useful in providing direct quotes and examples to support research findings.
Observations : The researcher typically takes detailed notes on what they observe, including any contextual information, nonverbal cues, or other relevant details. The resulting observational data can be analyzed to gain insights into social phenomena, such as human behavior, social interactions, and cultural practices.
Unstructured interviews : generate qualitative data through the use of open questions. This allows the respondent to talk in some depth, choosing their own words. This helps the researcher develop a real sense of a person’s understanding of a situation.
Diaries or journals : Written accounts of personal experiences or reflections.
Notice that qualitative data could be much more than just words or text. Photographs, videos, sound recordings, and so on, can be considered qualitative data. Visual data can be used to understand behaviors, environments, and social interactions.
Qualitative Data Analysis
Qualitative research is endlessly creative and interpretive. The researcher does not just leave the field with mountains of empirical data and then easily write up his or her findings.
Qualitative interpretations are constructed, and various techniques can be used to make sense of the data, such as content analysis, grounded theory (Glaser & Strauss, 1967), thematic analysis (Braun & Clarke, 2006), or discourse analysis.
For example, thematic analysis is a qualitative approach that involves identifying implicit or explicit ideas within the data. Themes will often emerge once the data has been coded .
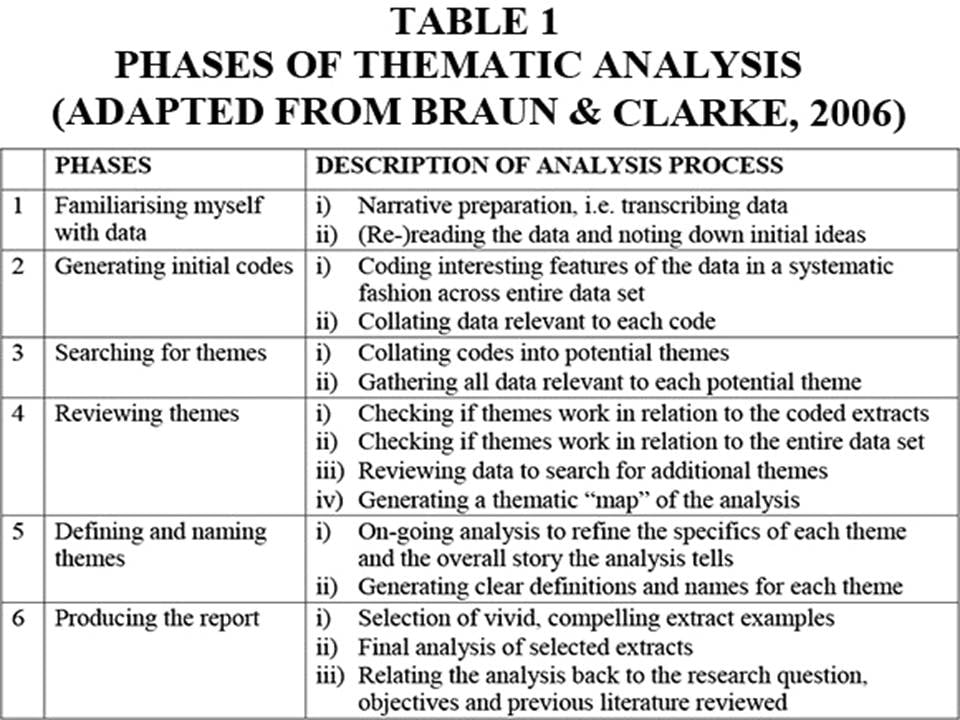
Key Features
- Events can be understood adequately only if they are seen in context. Therefore, a qualitative researcher immerses her/himself in the field, in natural surroundings. The contexts of inquiry are not contrived; they are natural. Nothing is predefined or taken for granted.
- Qualitative researchers want those who are studied to speak for themselves, to provide their perspectives in words and other actions. Therefore, qualitative research is an interactive process in which the persons studied teach the researcher about their lives.
- The qualitative researcher is an integral part of the data; without the active participation of the researcher, no data exists.
- The study’s design evolves during the research and can be adjusted or changed as it progresses. For the qualitative researcher, there is no single reality. It is subjective and exists only in reference to the observer.
- The theory is data-driven and emerges as part of the research process, evolving from the data as they are collected.
Limitations of Qualitative Research
- Because of the time and costs involved, qualitative designs do not generally draw samples from large-scale data sets.
- The problem of adequate validity or reliability is a major criticism. Because of the subjective nature of qualitative data and its origin in single contexts, it is difficult to apply conventional standards of reliability and validity. For example, because of the central role played by the researcher in the generation of data, it is not possible to replicate qualitative studies.
- Also, contexts, situations, events, conditions, and interactions cannot be replicated to any extent, nor can generalizations be made to a wider context than the one studied with confidence.
- The time required for data collection, analysis, and interpretation is lengthy. Analysis of qualitative data is difficult, and expert knowledge of an area is necessary to interpret qualitative data. Great care must be taken when doing so, for example, looking for mental illness symptoms.
Advantages of Qualitative Research
- Because of close researcher involvement, the researcher gains an insider’s view of the field. This allows the researcher to find issues that are often missed (such as subtleties and complexities) by the scientific, more positivistic inquiries.
- Qualitative descriptions can be important in suggesting possible relationships, causes, effects, and dynamic processes.
- Qualitative analysis allows for ambiguities/contradictions in the data, which reflect social reality (Denscombe, 2010).
- Qualitative research uses a descriptive, narrative style; this research might be of particular benefit to the practitioner as she or he could turn to qualitative reports to examine forms of knowledge that might otherwise be unavailable, thereby gaining new insight.
What Is Quantitative Research?
Quantitative research involves the process of objectively collecting and analyzing numerical data to describe, predict, or control variables of interest.
The goals of quantitative research are to test causal relationships between variables , make predictions, and generalize results to wider populations.
Quantitative researchers aim to establish general laws of behavior and phenomenon across different settings/contexts. Research is used to test a theory and ultimately support or reject it.
Quantitative Methods
Experiments typically yield quantitative data, as they are concerned with measuring things. However, other research methods, such as controlled observations and questionnaires , can produce both quantitative information.
For example, a rating scale or closed questions on a questionnaire would generate quantitative data as these produce either numerical data or data that can be put into categories (e.g., “yes,” “no” answers).
Experimental methods limit how research participants react to and express appropriate social behavior.
Findings are, therefore, likely to be context-bound and simply a reflection of the assumptions that the researcher brings to the investigation.
There are numerous examples of quantitative data in psychological research, including mental health. Here are a few examples:
Another example is the Experience in Close Relationships Scale (ECR), a self-report questionnaire widely used to assess adult attachment styles .
The ECR provides quantitative data that can be used to assess attachment styles and predict relationship outcomes.
Neuroimaging data : Neuroimaging techniques, such as MRI and fMRI, provide quantitative data on brain structure and function.
This data can be analyzed to identify brain regions involved in specific mental processes or disorders.
For example, the Beck Depression Inventory (BDI) is a clinician-administered questionnaire widely used to assess the severity of depressive symptoms in individuals.
The BDI consists of 21 questions, each scored on a scale of 0 to 3, with higher scores indicating more severe depressive symptoms.
Quantitative Data Analysis
Statistics help us turn quantitative data into useful information to help with decision-making. We can use statistics to summarize our data, describing patterns, relationships, and connections. Statistics can be descriptive or inferential.
Descriptive statistics help us to summarize our data. In contrast, inferential statistics are used to identify statistically significant differences between groups of data (such as intervention and control groups in a randomized control study).
- Quantitative researchers try to control extraneous variables by conducting their studies in the lab.
- The research aims for objectivity (i.e., without bias) and is separated from the data.
- The design of the study is determined before it begins.
- For the quantitative researcher, the reality is objective, exists separately from the researcher, and can be seen by anyone.
- Research is used to test a theory and ultimately support or reject it.
Limitations of Quantitative Research
- Context: Quantitative experiments do not take place in natural settings. In addition, they do not allow participants to explain their choices or the meaning of the questions they may have for those participants (Carr, 1994).
- Researcher expertise: Poor knowledge of the application of statistical analysis may negatively affect analysis and subsequent interpretation (Black, 1999).
- Variability of data quantity: Large sample sizes are needed for more accurate analysis. Small-scale quantitative studies may be less reliable because of the low quantity of data (Denscombe, 2010). This also affects the ability to generalize study findings to wider populations.
- Confirmation bias: The researcher might miss observing phenomena because of focus on theory or hypothesis testing rather than on the theory of hypothesis generation.
Advantages of Quantitative Research
- Scientific objectivity: Quantitative data can be interpreted with statistical analysis, and since statistics are based on the principles of mathematics, the quantitative approach is viewed as scientifically objective and rational (Carr, 1994; Denscombe, 2010).
- Useful for testing and validating already constructed theories.
- Rapid analysis: Sophisticated software removes much of the need for prolonged data analysis, especially with large volumes of data involved (Antonius, 2003).
- Replication: Quantitative data is based on measured values and can be checked by others because numerical data is less open to ambiguities of interpretation.
- Hypotheses can also be tested because of statistical analysis (Antonius, 2003).
Antonius, R. (2003). Interpreting quantitative data with SPSS . Sage.
Black, T. R. (1999). Doing quantitative research in the social sciences: An integrated approach to research design, measurement and statistics . Sage.
Braun, V. & Clarke, V. (2006). Using thematic analysis in psychology . Qualitative Research in Psychology , 3, 77–101.
Carr, L. T. (1994). The strengths and weaknesses of quantitative and qualitative research : what method for nursing? Journal of advanced nursing, 20(4) , 716-721.
Denscombe, M. (2010). The Good Research Guide: for small-scale social research. McGraw Hill.
Denzin, N., & Lincoln. Y. (1994). Handbook of Qualitative Research. Thousand Oaks, CA, US: Sage Publications Inc.
Glaser, B. G., Strauss, A. L., & Strutzel, E. (1968). The discovery of grounded theory; strategies for qualitative research. Nursing research, 17(4) , 364.
Minichiello, V. (1990). In-Depth Interviewing: Researching People. Longman Cheshire.
Punch, K. (1998). Introduction to Social Research: Quantitative and Qualitative Approaches. London: Sage
Further Information
- Designing qualitative research
- Methods of data collection and analysis
- Introduction to quantitative and qualitative research
- Checklists for improving rigour in qualitative research: a case of the tail wagging the dog?
- Qualitative research in health care: Analysing qualitative data
- Qualitative data analysis: the framework approach
- Using the framework method for the analysis of
- Qualitative data in multi-disciplinary health research
- Content Analysis
- Grounded Theory
- Thematic Analysis

Related Articles
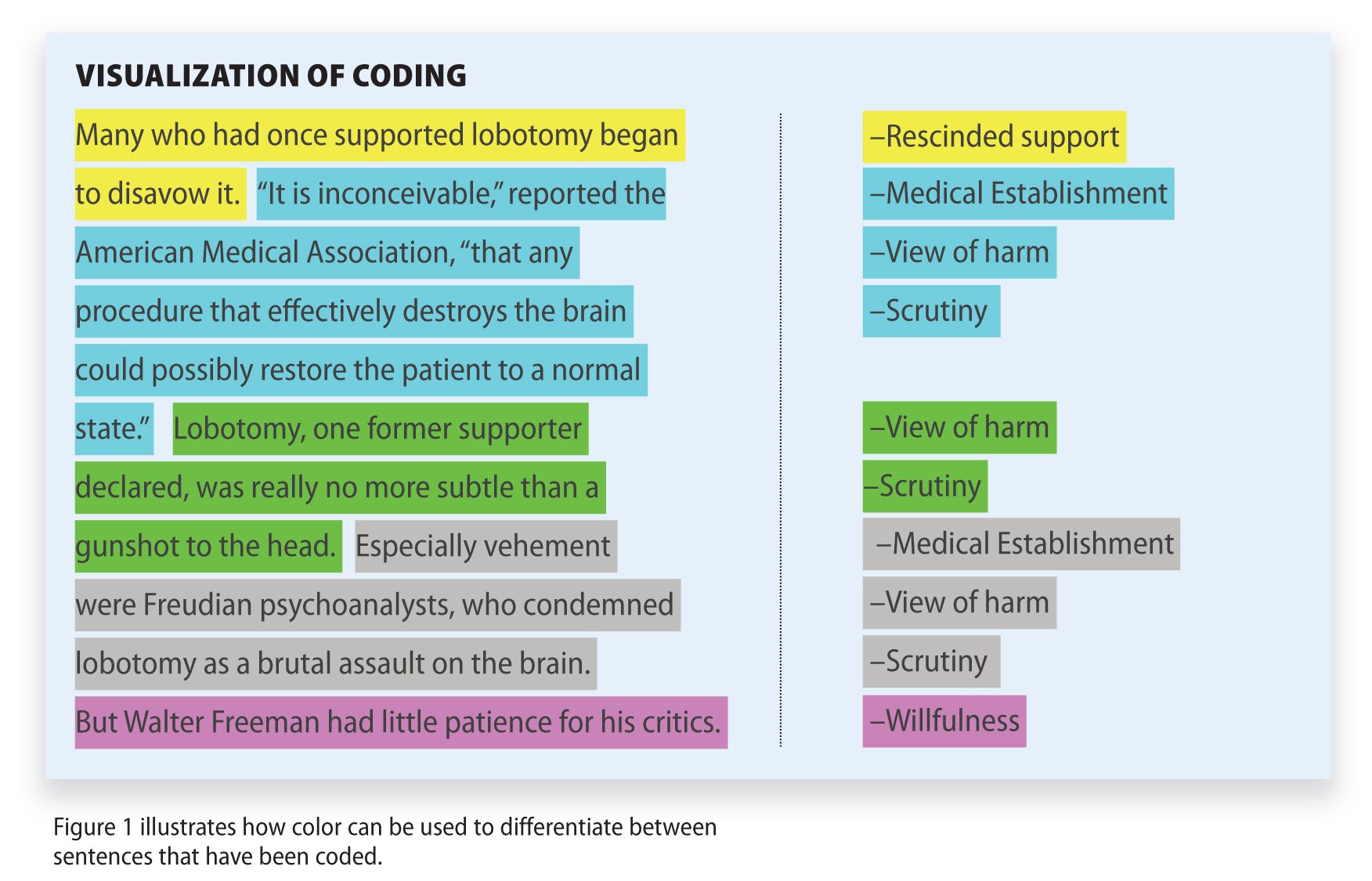
Research Methodology
Qualitative Data Coding
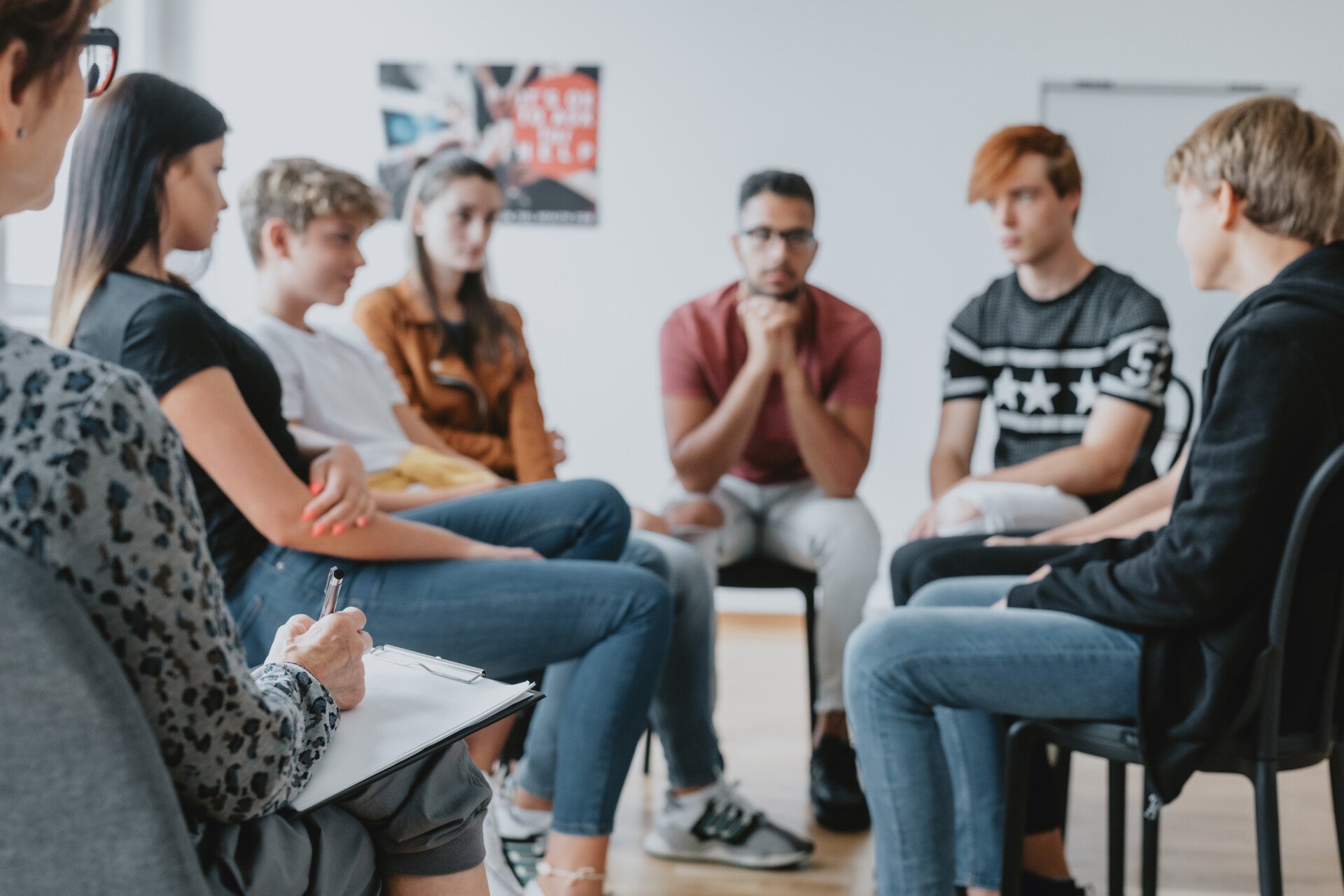
What Is a Focus Group?
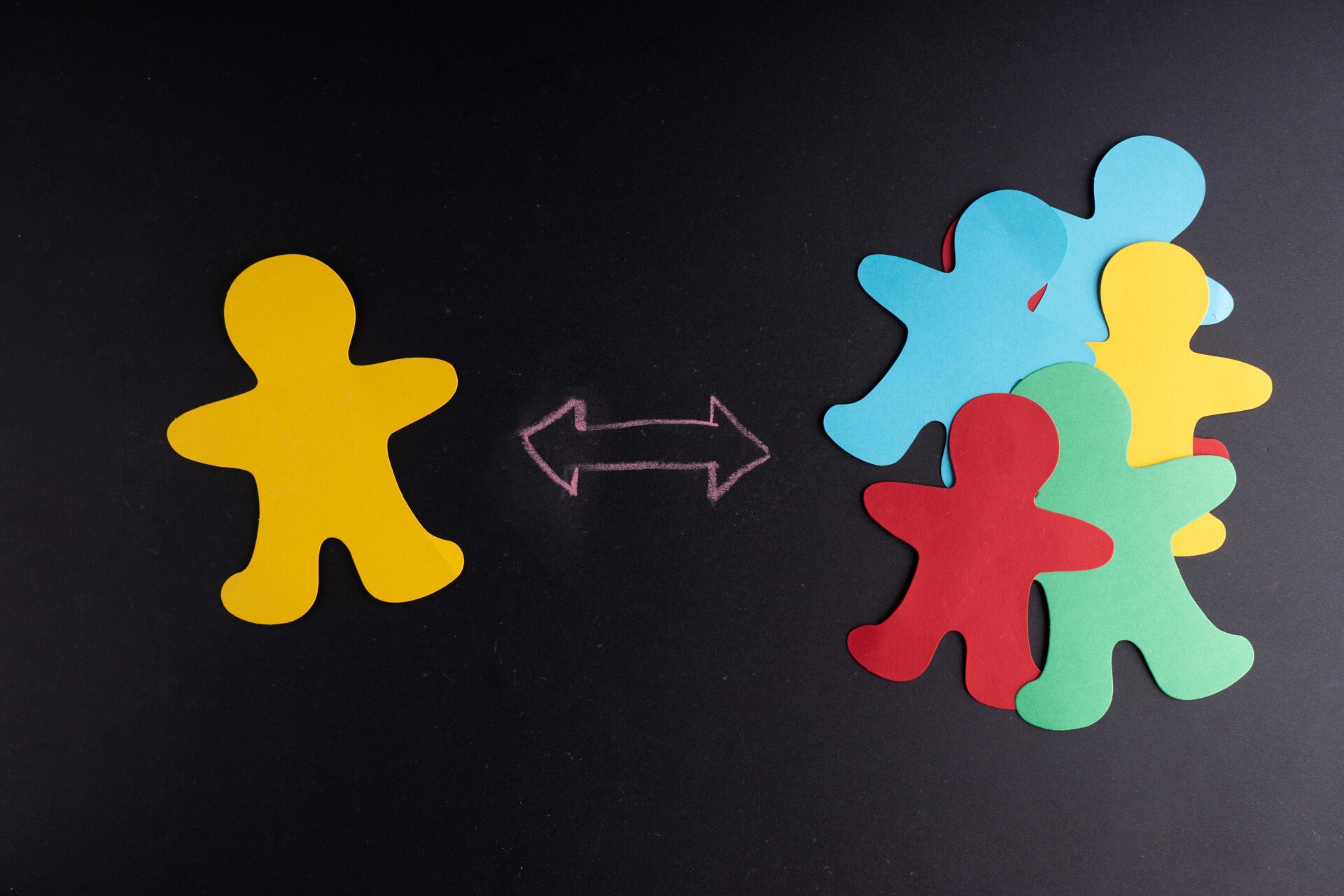
Cross-Cultural Research Methodology In Psychology
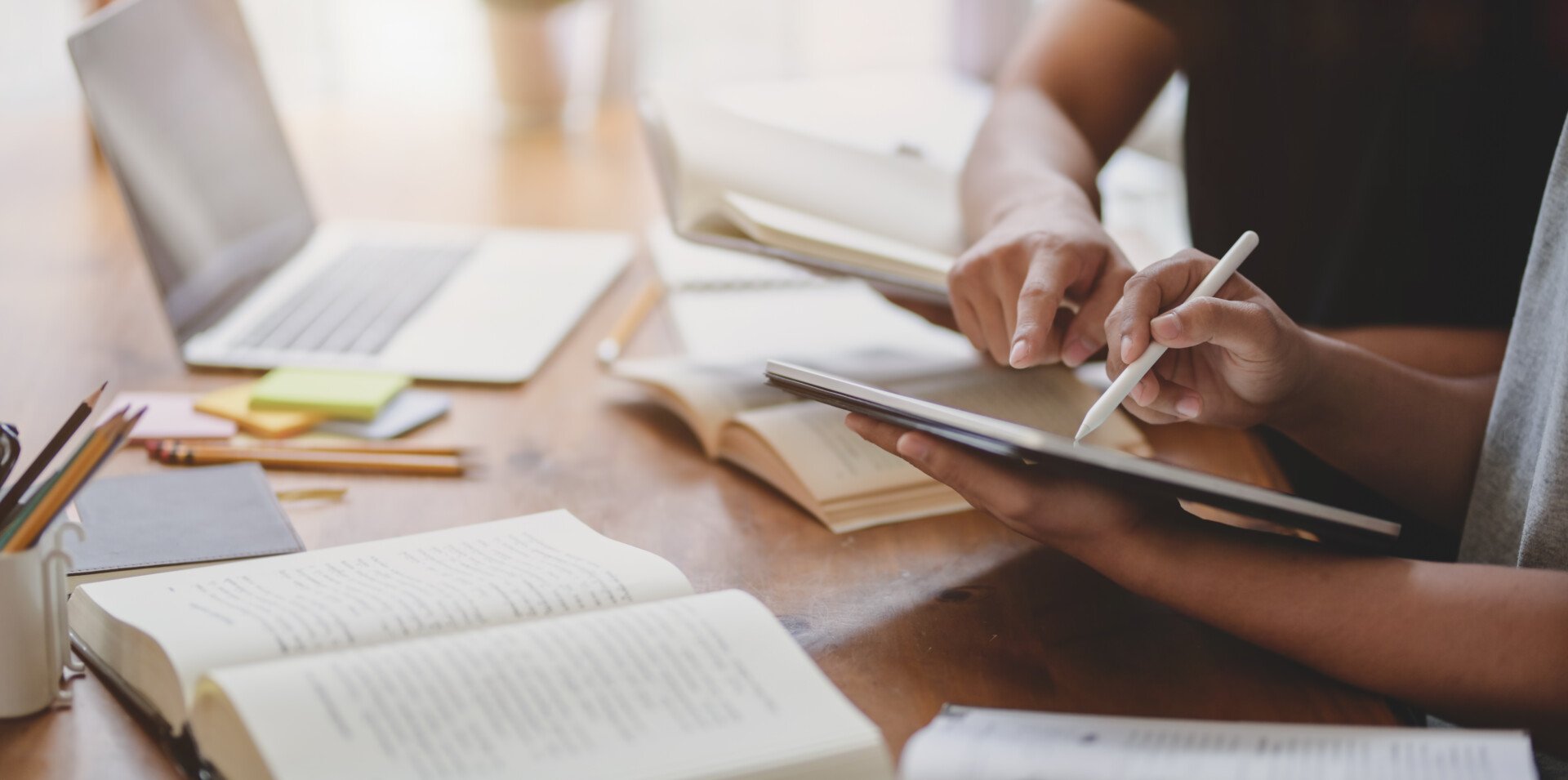
What Is Internal Validity In Research?
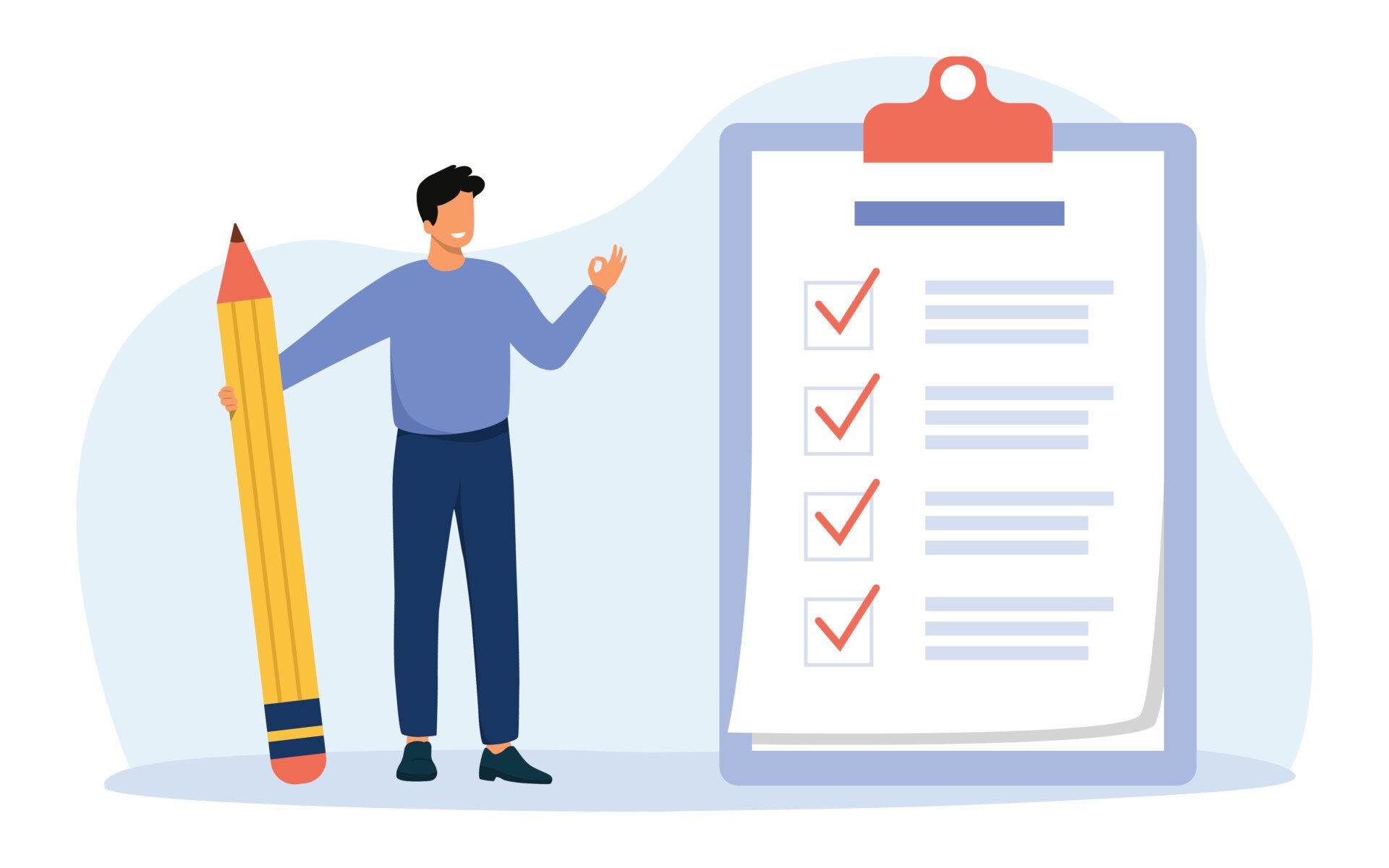
Research Methodology , Statistics
What Is Face Validity In Research? Importance & How To Measure
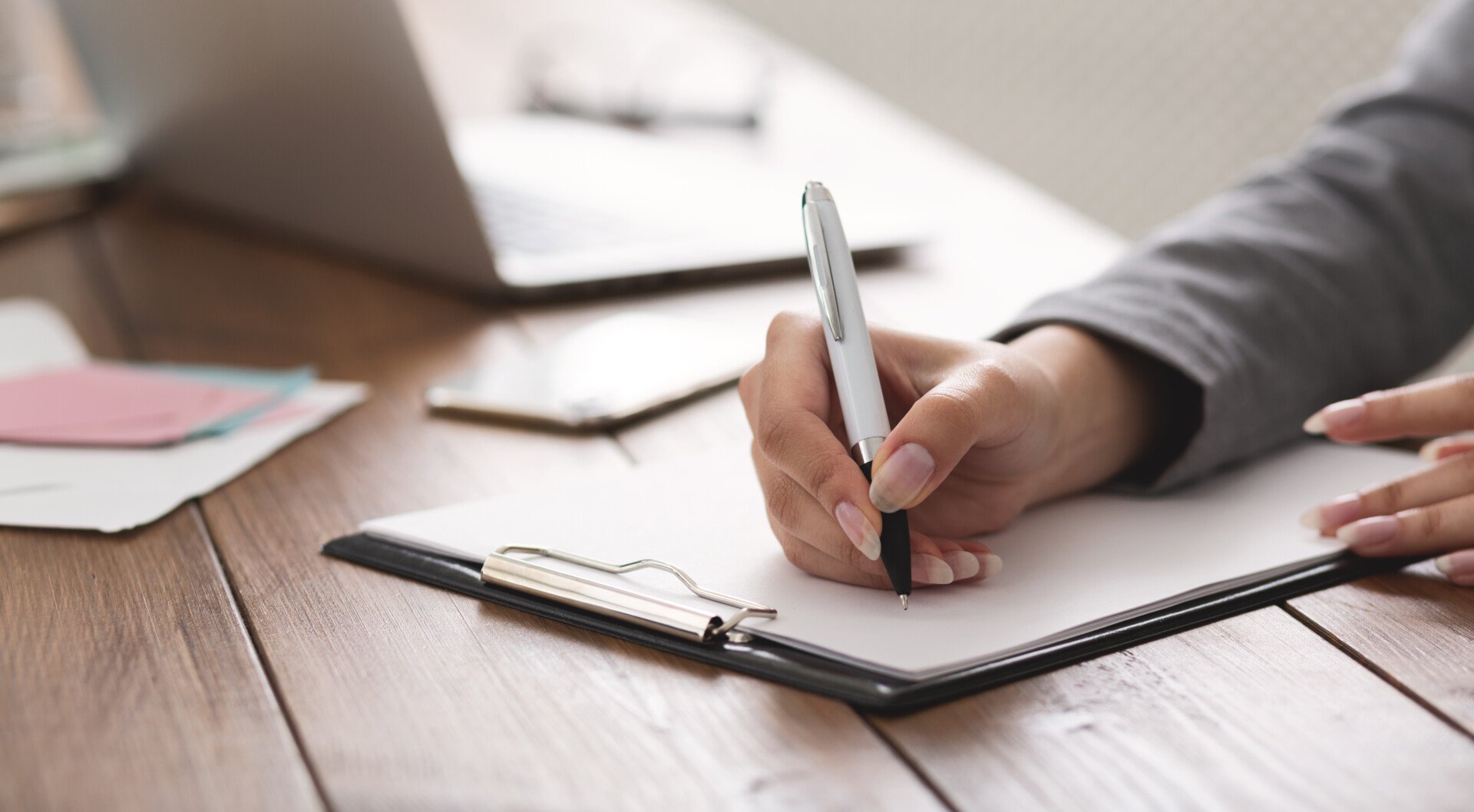
Criterion Validity: Definition & Examples
Qualitative vs. quantitative data in research: what's the difference?
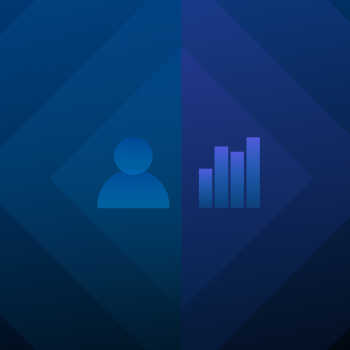
If you're reading this, you likely already know the importance of data analysis. And you already know it can be incredibly complex.
At its simplest, research and it's data can be broken down into two different categories: quantitative and qualitative. But what's the difference between each? And when should you use them? And how can you use them together?
Understanding the differences between qualitative and quantitative data is key to any research project. Knowing both approaches can help you in understanding your data better—and ultimately understand your customers better. Quick takeaways:
Quantitative research uses objective, numerical data to answer questions like "what" and "how often." Conversely, qualitative research seeks to answer questions like "why" and "how," focusing on subjective experiences to understand motivations and reasons.
Quantitative data is collected through methods like surveys and experiments and analyzed statistically to identify patterns. Qualitative data is gathered through interviews or observations and analyzed by categorizing information to understand themes and insights.
Effective data analysis combines quantitative data for measurable insights with qualitative data for contextual depth.
What is quantitative data?
Qualitative and quantitative data differ in their approach and the type of data they collect.
Quantitative data refers to any information that can be quantified — that is, numbers. If it can be counted or measured, and given a numerical value, it's quantitative in nature. Think of it as a measuring stick.
Quantitative variables can tell you "how many," "how much," or "how often."
Some examples of quantitative data :
How many people attended last week's webinar?
How much revenue did our company make last year?
How often does a customer rage click on this app?
To analyze these research questions and make sense of this quantitative data, you’d normally use a form of statistical analysis —collecting, evaluating, and presenting large amounts of data to discover patterns and trends. Quantitative data is conducive to this type of analysis because it’s numeric and easier to analyze mathematically.
Computers now rule statistical analytics, even though traditional methods have been used for years. But today’s data volumes make statistics more valuable and useful than ever. When you think of statistical analysis now, you think of powerful computers and algorithms that fuel many of the software tools you use today.
Popular quantitative data collection methods are surveys, experiments, polls, and more.
Quantitative Data 101: What is quantitative data?
Take a deeper dive into what quantitative data is, how it works, how to analyze it, collect it, use it, and more.
Learn more about quantitative data →
What is qualitative data?
Unlike quantitative data, qualitative data is descriptive, expressed in terms of language rather than numerical values.
Qualitative data analysis describes information and cannot be measured or counted. It refers to the words or labels used to describe certain characteristics or traits.
You would turn to qualitative data to answer the "why?" or "how?" questions. It is often used to investigate open-ended studies, allowing participants (or customers) to show their true feelings and actions without guidance.
Some examples of qualitative data:
Why do people prefer using one product over another?
How do customers feel about their customer service experience?
What do people think about a new feature in the app?
Think of qualitative data as the type of data you'd get if you were to ask someone why they did something. Popular data collection methods are in-depth interviews, focus groups, or observation.
Start growing with data and Fullstory.
Request your personalized demo of the Fullstory behavioral data platform.
What are the differences between qualitative vs. quantitative data?
When it comes to conducting data research, you’ll need different collection, hypotheses and analysis methods, so it’s important to understand the key differences between quantitative and qualitative data:
Quantitative data is numbers-based, countable, or measurable. Qualitative data is interpretation-based, descriptive, and relating to language.
Quantitative data tells us how many, how much, or how often in calculations. Qualitative data can help us to understand why, how, or what happened behind certain behaviors .
Quantitative data is fixed and universal. Qualitative data is subjective and unique.
Quantitative research methods are measuring and counting. Qualitative research methods are interviewing and observing.
Quantitative data is analyzed using statistical analysis. Qualitative data is analyzed by grouping the data into categories and themes.
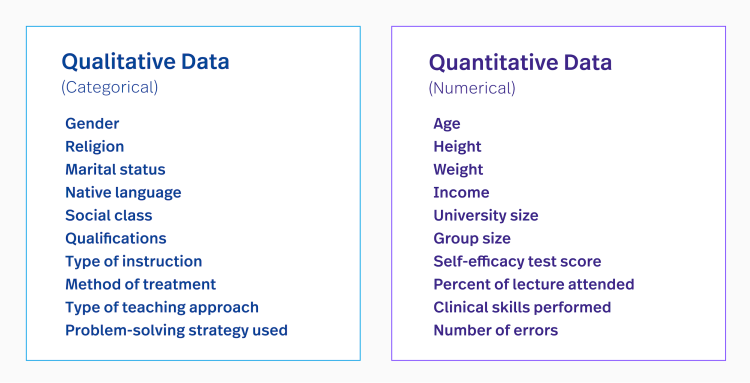
As you can see, both provide immense value for any data collection and are key to truly finding answers and patterns.
More examples of quantitative and qualitative data
You’ve most likely run into quantitative and qualitative data today, alone. For the visual learner, here are some examples of both quantitative and qualitative data:
Quantitative data example
The customer has clicked on the button 13 times.
The engineer has resolved 34 support tickets today.
The team has completed 7 upgrades this month.
14 cartons of eggs were purchased this month.
Qualitative data example
My manager has curly brown hair and blue eyes.
My coworker is funny, loud, and a good listener.
The customer has a very friendly face and a contagious laugh.
The eggs were delicious.
The fundamental difference is that one type of data answers primal basics and one answers descriptively.
What does this mean for data quality and analysis? If you just analyzed quantitative data, you’d be missing core reasons behind what makes a data collection meaningful. You need both in order to truly learn from data—and truly learn from your customers.
What are the advantages and disadvantages of each?
Both types of data has their own pros and cons.
Advantages of quantitative data
It’s relatively quick and easy to collect and it’s easier to draw conclusions from.
When you collect quantitative data, the type of results will tell you which statistical tests are appropriate to use.
As a result, interpreting your data and presenting those findings is straightforward and less open to error and subjectivity.
Another advantage is that you can replicate it. Replicating a study is possible because your data collection is measurable and tangible for further applications.
Disadvantages of quantitative data
Quantitative data doesn’t always tell you the full story (no matter what the perspective).
With choppy information, it can be inconclusive.
Quantitative research can be limited, which can lead to overlooking broader themes and relationships.
By focusing solely on numbers, there is a risk of missing larger focus information that can be beneficial.
Advantages of qualitative data
Qualitative data offers rich, in-depth insights and allows you to explore context.
It’s great for exploratory purposes.
Qualitative research delivers a predictive element for continuous data.
Disadvantages of qualitative data
It’s not a statistically representative form of data collection because it relies upon the experience of the host (who can lose data).
It can also require multiple data sessions, which can lead to misleading conclusions.
The takeaway is that it’s tough to conduct a successful data analysis without both. They both have their advantages and disadvantages and, in a way, they complement each other.
Now, of course, in order to analyze both types of data, information has to be collected first.
Let's get into the research.
Quantitative and qualitative research
The core difference between qualitative and quantitative research lies in their focus and methods of data collection and analysis. This distinction guides researchers in choosing an appropriate approach based on their specific research needs.
Using mixed methods of both can also help provide insights form combined qualitative and quantitative data.
Best practices of each help to look at the information under a broader lens to get a unique perspective. Using both methods is helpful because they collect rich and reliable data, which can be further tested and replicated.
What is quantitative research?
Quantitative research is based on the collection and interpretation of numeric data. It's all about the numbers and focuses on measuring (using inferential statistics ) and generalizing results. Quantitative research seeks to collect numerical data that can be transformed into usable statistics.
It relies on measurable data to formulate facts and uncover patterns in research. By employing statistical methods to analyze the data, it provides a broad overview that can be generalized to larger populations.
In terms of digital experience data, it puts everything in terms of numbers (or discrete data )—like the number of users clicking a button, bounce rates , time on site, and more.
Some examples of quantitative research:
What is the amount of money invested into this service?
What is the average number of times a button was dead clicked ?
How many customers are actually clicking this button?
Essentially, quantitative research is an easy way to see what’s going on at a 20,000-foot view.
Each data set (or customer action, if we’re still talking digital experience) has a numerical value associated with it and is quantifiable information that can be used for calculating statistical analysis so that decisions can be made.
You can use statistical operations to discover feedback patterns (with any representative sample size) in the data under examination. The results can be used to make predictions , find averages, test causes and effects, and generalize results to larger measurable data pools.
Unlike qualitative methodology, quantitative research offers more objective findings as they are based on more reliable numeric data.
Quantitative data collection methods
A survey is one of the most common research methods with quantitative data that involves questioning a large group of people. Questions are usually closed-ended and are the same for all participants. An unclear questionnaire can lead to distorted research outcomes.
Similar to surveys, polls yield quantitative data. That is, you poll a number of people and apply a numeric value to how many people responded with each answer.
Experiments
An experiment is another common method that usually involves a control group and an experimental group . The experiment is controlled and the conditions can be manipulated accordingly. You can examine any type of records involved if they pertain to the experiment, so the data is extensive.
What is qualitative research?
Qualitative research does not simply help to collect data. It gives a chance to understand the trends and meanings of natural actions. It’s flexible and iterative.
Qualitative research focuses on the qualities of users—the actions that drive the numbers. It's descriptive research. The qualitative approach is subjective, too.
It focuses on describing an action, rather than measuring it.
Some examples of qualitative research:
The sunflowers had a fresh smell that filled the office.
All the bagels with bites taken out of them had cream cheese.
The man had blonde hair with a blue hat.
Qualitative research utilizes interviews, focus groups, and observations to gather in-depth insights.
This approach shines when the research objective calls for exploring ideas or uncovering deep insights rather than quantifying elements.
Qualitative data collection methods
An interview is the most common qualitative research method. This method involves personal interaction (either in real life or virtually) with a participant. It’s mostly used for exploring attitudes and opinions regarding certain issues.
Interviews are very popular methods for collecting data in product design .
Focus groups
Data analysis by focus group is another method where participants are guided by a host to collect data. Within a group (either in person or online), each member shares their opinion and experiences on a specific topic, allowing researchers to gather perspectives and deepen their understanding of the subject matter.
Digital Leadership Webinar: Accelerating Growth with Quantitative Data and Analytics
Learn how the best-of-the-best are connecting quantitative data and experience to accelerate growth.
So which type of data is better for data analysis?
So how do you determine which type is better for data analysis ?
Quantitative data is structured and accountable. This type of data is formatted in a way so it can be organized, arranged, and searchable. Think about this data as numbers and values found in spreadsheets—after all, you would trust an Excel formula.
Qualitative data is considered unstructured. This type of data is formatted (and known for) being subjective, individualized, and personalized. Anything goes. Because of this, qualitative data is inferior if it’s the only data in the study. However, it’s still valuable.
Because quantitative data is more concrete, it’s generally preferred for data analysis. Numbers don’t lie. But for complete statistical analysis, using both qualitative and quantitative yields the best results.
At Fullstory, we understand the importance of data, which is why we created a behavioral data platform that analyzes customer data for better insights. Our platform delivers a complete, retroactive view of how people interact with your site or app—and analyzes every point of user interaction so you can scale.
Unlock business-critical data with Fullstory
A perfect digital customer experience is often the difference between company growth and failure. And the first step toward building that experience is quantifying who your customers are, what they want, and how to provide them what they need.
Access to product analytics is the most efficient and reliable way to collect valuable quantitative data about funnel analysis, customer journey maps , user segments, and more.
But creating a perfect digital experience means you need organized and digestible quantitative data—but also access to qualitative data. Understanding the why is just as important as the what itself.
Fullstory's DXI platform combines the quantitative insights of product analytics with picture-perfect session replay for complete context that helps you answer questions, understand issues, and uncover customer opportunities.
Start a free 14-day trial to see how Fullstory can help you combine your most invaluable quantitative and qualitative insights and eliminate blind spots.
About the author
Our team of experts is committed to introducing people to important topics surrounding analytics, digital experience intelligence, product development, and more.
Related posts
Quantitative data is used for calculations or obtaining numerical results. Learn about the different types of quantitative data uses cases and more.
Discover how data discovery transforms raw data into actionable insights for informed decisions, improved strategies, and better customer experiences.
Learn the 3 key benefits democratized data can achieve, and 3 of the most pertinent dangers of keeping data (and teams) siloed.
Learn the essentials of behavioral data and its transformative impact on customer experience. Our comprehensive guide provides the tools and knowledge to harness this power effectively.
Discover how Fullstory leverages AI to turn raw data into actionable insights, transforming user experiences and driving business growth.
Discover how just-in-time data, explained by Lane Greer, enhances customer insights and decision-making beyond real-time analytics.
- Top Courses
- Online Degrees
- Find your New Career
- Join for Free
What Does a Data Analyst Do? Your 2024 Career Guide
A data analyst gathers, cleans, and studies data sets to help solve problems. Here's how you can start on a path to become one.
![analysis of data descriptive research [Featured Image] A data scientist works on a desktop computer in an office.](https://d3njjcbhbojbot.cloudfront.net/api/utilities/v1/imageproxy/https://images.ctfassets.net/wp1lcwdav1p1/1zPto5S1K55iCLiSWdlmKB/cc15e59dd1c8f3465e32f50e41d2a00f/GettyImages-1387361694.jpg?w=1500&h=680&q=60&fit=fill&f=faces&fm=jpg&fl=progressive&auto=format%2Ccompress&dpr=1&w=1000)
A data analyst collects, cleans, and interprets data sets in order to answer a question or solve a problem. They work in many industries, including business, finance, criminal justice, science, medicine, and government.
What kind of customers should a business target in its next ad campaign? What age group is most vulnerable to a particular disease? What patterns in behavior are connected to financial fraud?
These are the types of questions you might be pressed to answer as a data analyst. Read on to find out more about what a data analyst is, what skills you'll need, and how you can start on a path to becoming one.
Start advancing your data analysis skills today
Explore a career path as a data analyst with the Google Data Analytics Professional Certificate . Learn key analytical skills like data cleaning, analysis, and visualization, as well as tools like spreadsheets, SQL, R programming, and Tableau.
What is data analysis?
Data analysis is the process of gleaning insights from data to inform better business decisions. The process of analyzing data typically moves through five iterative phases:
Identify the data you want to analyze
Collect the data
Clean the data in preparation for analysis
Analyze the data
Interpret the results of the analysis
Data analysis can take different forms, depending on the question you’re trying to answer. You can read more about the types of data analysis here. Briefly, descriptive analysis tells us what happened, diagnostic analysis tells us why it happened, predictive analytics forms projections about the future, and prescriptive analysis creates actionable advice on what actions to take.
Watch the video below for an introduction to data analytics and preview the Google course:
Data analyst tasks and responsibilities
A data analyst is a person whose job is to gather and interpret data in order to solve a specific problem. The role includes plenty of time spent with data but entails communicating findings too.
Here’s what many data analysts do on a day-to-day basis:
Gather data: Analysts often collect data themselves. This could include conducting surveys, tracking visitor characteristics on a company website, or buying datasets from data collection specialists.
Clean data: Raw data might contain duplicates, errors, or outliers. Cleaning the data means maintaining the quality of data in a spreadsheet or through a programming language so that your interpretations won’t be wrong or skewed.
Model data: This entails creating and designing the structures of a database. You might choose what types of data to store and collect, establish how data categories are related to each other, and work through how the data actually appears.
Interpret data: Interpreting data will involve finding patterns or trends in data that could answer the question at hand.
Present: Communicating the results of your findings will be a key part of your job. You do this by putting together visualizations like charts and graphs, writing reports, and presenting information to interested parties.
What tools do data analysts use?
During the process of data analysis, analysts often use a wide variety of tools to make their work more accurate and efficient. Some of the most common tools in the data analytics industry include:
Microsoft Excel
Google Sheets
R or Python
Microsoft Power BI
Jupyter Notebooks
Data analyst salary and job outlook
The average base salary for a data analyst in the US is $69,517 in December 2021, according to Glassdoor. This can vary depending on your seniority, where in the US you’re located, and other factors.
Data analysts are in high demand. The World Economic Forum listed it as number two in growing jobs in the US [ 1 ]. The Bureau of Labor Statistics also reports related occupations as having extremely high growth rates.
From 2020 to 2030, operations research analyst positions are expected to grow by 25 percent, market research analysts by 22 percent, and mathematicians and statisticians by 33 percent. That’s a lot higher than the total employment growth rate of 7.7 percent.
Data analyst vs. data scientist: What’s the difference?
Data analysts and data scientists both work with data, but what they do with it differs. Data analysts typically work with existing data to solve defined business problems. Data scientists build new algorithms and models to make predictions about the future. Learn more about the difference between data scientists and data analysts .
Types of data analysts
As advancing technology has rapidly expanded the types and amount of information we can collect, knowing how to gather, sort, and analyze data has become a crucial part of almost any industry. You’ll find data analysts in the criminal justice, fashion, food, technology, business, environment, and public sectors—among many others.
People who perform data analysis might have other titles, such as:
Medical and health care analyst
Market research analyst
Business analyst
Business intelligence analyst
Operations research analyst
Intelligence analyst
Interested in business intelligence? Gain skills in data modeling and data visualization:
How to become a data analyst
There’s more than one path toward a career as a data analyst. Whether you’re just graduating from school or looking to switch careers, the first step is often assessing what transferable skills you have and building the new skills you’ll need in this new role.
Data analyst technical skills
Database tools: Microsoft Excel and SQL should be mainstays in any data analyst’s toolbox. While Excel is ubiquitous across industries, SQL can handle larger sets of data and is widely regarded as a necessity for data analysis.
Programming languages: Learning a statistical programming language like Python or R will let you handle large sets of data and perform complex equations. Though Python and R are among the most common, it’s a good idea to look at several job descriptions of a position you’re interested in to determine which language will be most useful to your industry.
Data visualization: Presenting your findings in a clear and compelling way is crucial to being a successful data analyst. Knowing how best to present information through charts and graphs will make sure colleagues, employers, and stakeholders will understand your work. Tableau, Jupyter Notebook, and Excel are among the many tools used to create visuals.
Statistics and math: Knowing the concepts behind what data tools are actually doing will help you tremendously in your work. Having a solid grasp of statistics and math will help you determine which tools are best to use to solve a particular problem, help you catch errors in your data, and have a better understanding of the results.
If that seems like a lot, don’t worry—there are plenty of courses that will walk you through the basics of the technical skills you need as a data analyst. This IBM Data Analyst Professional Certificate course on Coursera can be a good place to start.
Data analyst workplace skills
Problem solving: A data analyst needs to have a good understanding of the question being asked and the problem that needs to be solved. They also should be able to find patterns or trends that might reveal a story. Having critical thinking skills will allow you to focus on the right types of data, recognize the most revealing methods of analysis, and catch gaps in your work.
Communication: Being able to get your ideas across to other people will be crucial to your work as a data analyst. Strong written and speaking skills to communicate with colleagues and other stakeholders are good assets to have as a data analyst.
Industry knowledge: Knowing about the industry you work in—health care, business, finance, or otherwise—will give you an advantage in your work and in job applications. If you’re trying to break into a specific industry, take some time to pay attention to the news in your industry or read a book on the subject. This can familiarize you with the industry’s main issues and trends.
Learn more: 7 In-Demand Data Analyst Skills to Get Hired
Resources to start your data analyst career
We've curated a collection of resources to help you decide whether becoming a data analyst is right for you—including figuring out what skills you'll need to learn and courses you can take to pursue this career.
Paths to becoming a data analyst
Acquiring these skills is the first step to becoming a data analyst. Here are a few routes you can take to get them that are flexible enough to fit in around your life.
Professional certificate: Entry-level professional certificate programs usually require no previous experience in the field. They can teach you basic skills like SQL or statistics while giving you the chance to create projects for your portfolio and provide real-time feedback on your work. Several professional certificate programs on Coursera do just that.
Bachelor's degree: The Bureau of Labor Statistics recommends a bachelor’s degree for jobs that involve data analysis. If you’re considering getting a degree to become a data analyst, focusing your coursework in statistics, math, or computer science can give you a head start with potential employers. Many online bachelor’s degrees have flexible scheduling so you can fit a degree in around your priorities.
Self-study: If you want a path that doesn’t include formal training, it’s possible to learn the skills necessary for data analysis on your own. Get started with this data analytics reading list for beginners . Once you’re ready to start building a portfolio , here are some ideas for data analytics projects .
For more on how to become a data analyst (with or without a degree), check out our step-by-step guide .
Data analyst career advancement
Being a data analyst can also open doors to other careers. Many who start as data analysts go on to work as data scientists . Like analysts, data scientists use statistics, math, and computer science to analyze data. A scientist, however, might use advanced techniques to build models and other tools to provide insights into future trends.
Start advancing your data analyst skills today
If you’re ready to start exploring a career as a data analyst, build job-ready skills in less than six months with the Google Data Analytics Professional Certificate on Coursera. Learn how to clean, organize, analyze, visualize, and present data from data professionals at Google.
If you're ready to build on your existing data science skills to qualify for in-demand job titles like junior data scientist and data science analyst, consider the Google Advanced Data Analytics Professional Certificate .
Frequently asked questions (FAQ)
Is a data analyst a good job .
Data analysts tend to be in demand and well paid. If you enjoy solving problems, working with numbers, and thinking analytically, a career as a data analyst could be a good fit for you.
What should I study to become a data analyst?
Most entry-level data analyst positions require at least a bachelor’s degree. Fields of study might include data analysis, mathematics, finance, economics, or computer science. Earning a master’s degree in data analysis, data science, or business analytics might open new, higher-paying job opportunities.
Read more: What Degree Do I Need to Become a Data Analyst?
Does data analysis require coding?
You might not be required to code as part of your day-to-day requirements as a data analyst. However, knowing how to write some basic Python or R , as well as how to write queries in SQL (Structured Query Language) can help you clean, analyze, and visualize data.
How do I get a job as a data analyst with no experience?
Sometimes even junior data analyst job listings ask for previous experience. Luckily, it’s possible to gain experience working with data even if you’ve never had a job as an analyst. Degree programs, certification courses, and online classes often include hands-on data projects. If you’re learning on your own, you can find free data sets on the internet that you can work with to start getting experience (and building your portfolio).
How long does it take to become a data analyst?
The amount of time it takes to develop the skills you need to get a job as a data analyst will depend on what you already know, your strategy for learning new skills, and the type of role you’re applying for. But it might not take as long as you think. It’s possible to learn the skills you need for an entry-level role as a data analyst in approximately 64 hours of learning, according to Coursera’s 2021 Global Skills Report . It’s possible to earn your Google Data Analytics or IBM Data Analyst Professional Certificate in less than six months.
Article sources
World Economic Forum. " The Future of Jobs Report 2020 , http://www3.weforum.org/docs/WEF_Future_of_Jobs_2020.pdf." Accessed April 1, 2024.
Keep reading
Coursera staff.
Editorial Team
Coursera’s editorial team is comprised of highly experienced professional editors, writers, and fact...
This content has been made available for informational purposes only. Learners are advised to conduct additional research to ensure that courses and other credentials pursued meet their personal, professional, and financial goals.

- Statistics & Predictive Analytics
Harness your Data for Better Decision Making
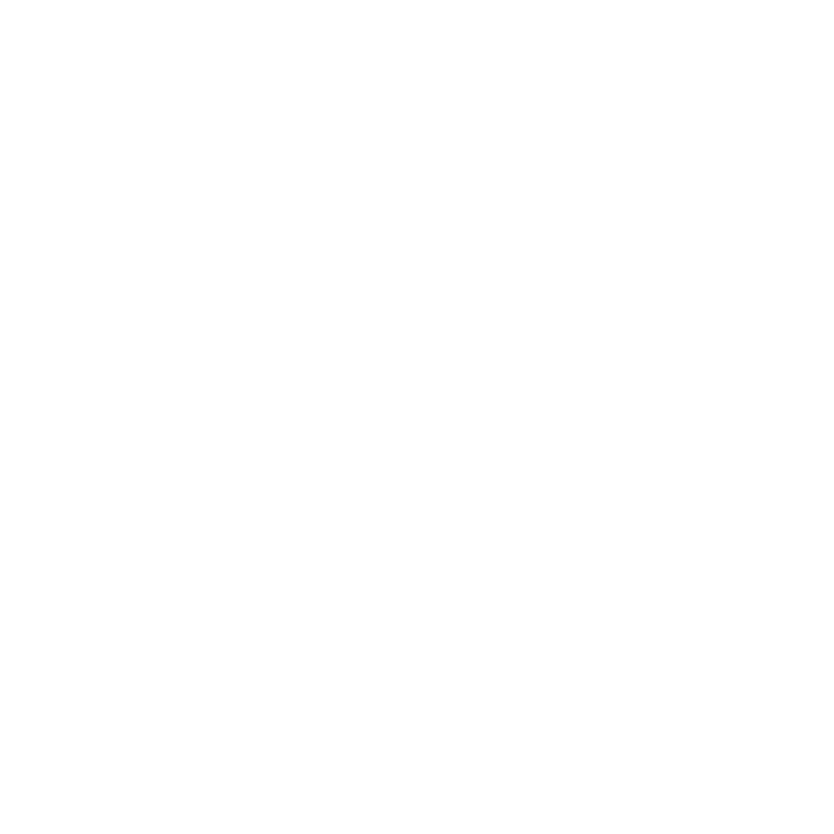
- Random Forests
SOLUTION TOPICS
Data exploration, descriptive statistics, advanced predictive analytics, quality tools, design of experiments, reliability, time series, monte carlo simulation, our statistical analysis solutions .
With Minitab’s software, you can easily make data-driven decisions. Our market-leading, flexible, and user-friendly statistical software helps you explore and investigate your data. You can discover insights, predict outcomes, and improve your results.
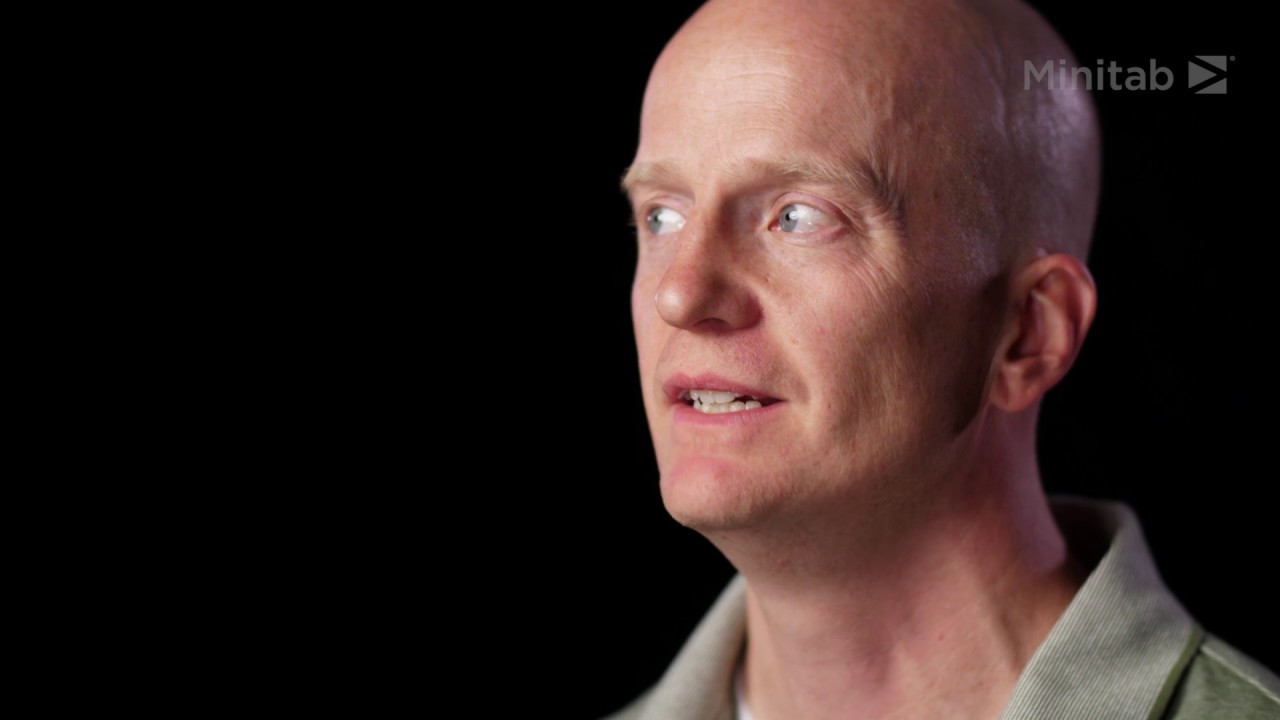
As the market leader in statistical software, Minitab prides itself on providing powerful solutions for everyone, no matter how early or advanced they are on their analytics journey.
Data exploration is an early step in data analysis, typically to uncover patterns, characteristics, and points of interest. By using interactive visualizations, users can quickly explore their data and identify areas or patterns to dive into for further analysis. Minitab’s proprietary Graph Builder was built to accelerate and facilitate data exploration with an interactive and easy-to-browse gallery that lets you seamlessly switch from one graph to the next, using the same data and without re-running your analysis. Instantly, visualize and explore your data.
Minitab’s state-of-the-art graph builder can be used for data exploration and for learning new statistical visualizations that will make your analysis clearer and more powerful.
Descriptive statistics, which describes the properties of sample and population data, is used in our daily personal and business lives. Descriptive statistics is a way to organize, represent, and describe a collection of data, using tables, graphs, and summary measures, including popular parameters such as mean (average), median, or standard deviation. Descriptive statistics also are powerful tools to measure things like frequency, dispersion, and measure of position (like percentile or quartile ranking). Minitab provides all the descriptive statistical tools you need to analyze your data and differentiate yourself by providing additional context and statistics around your daily work.
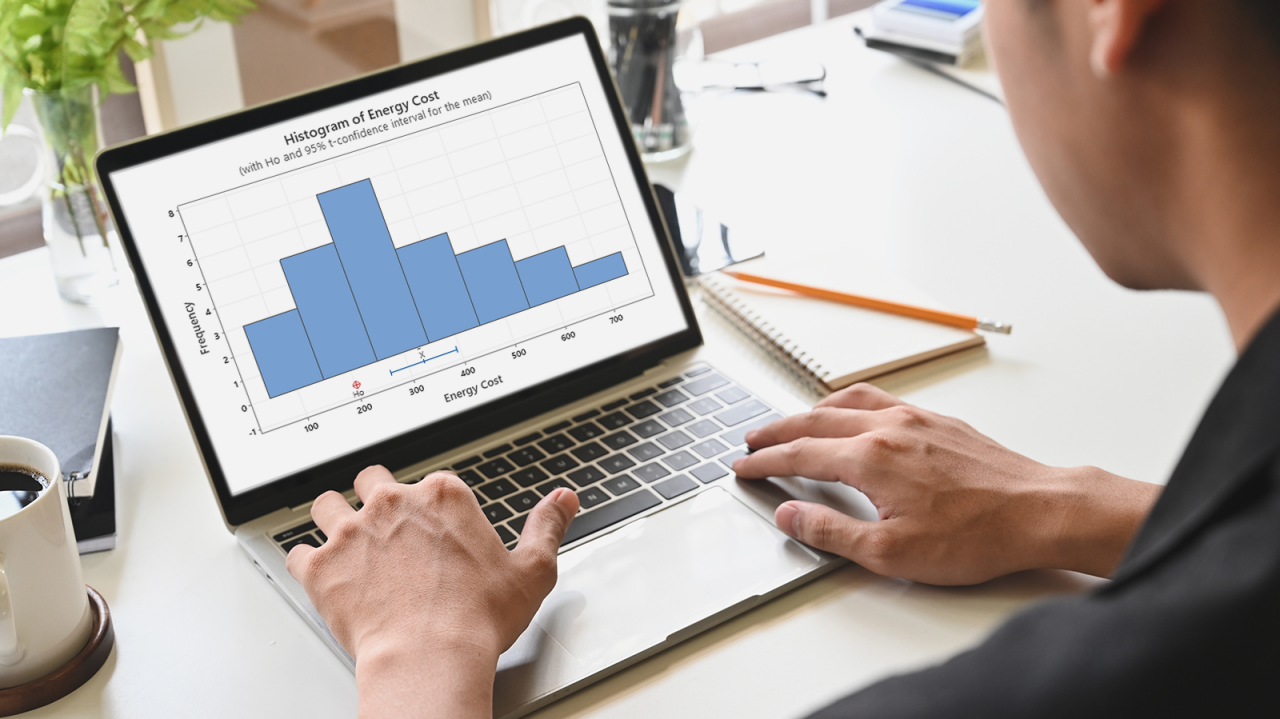
Predictive analytics is a category of data analytics aimed at making predictions about future outcomes, based on historical data and analytics techniques. Minitab provides a variety of statistical techniques (including data mining, machine learning, and predictive modeling) to understand future occurrences. Regression modeling is one of the most popular tools around, and often many professionals’ first foray into predictive analytics.
For more advanced statisticians, we offer data science tools and a more advanced predictive analytics module .
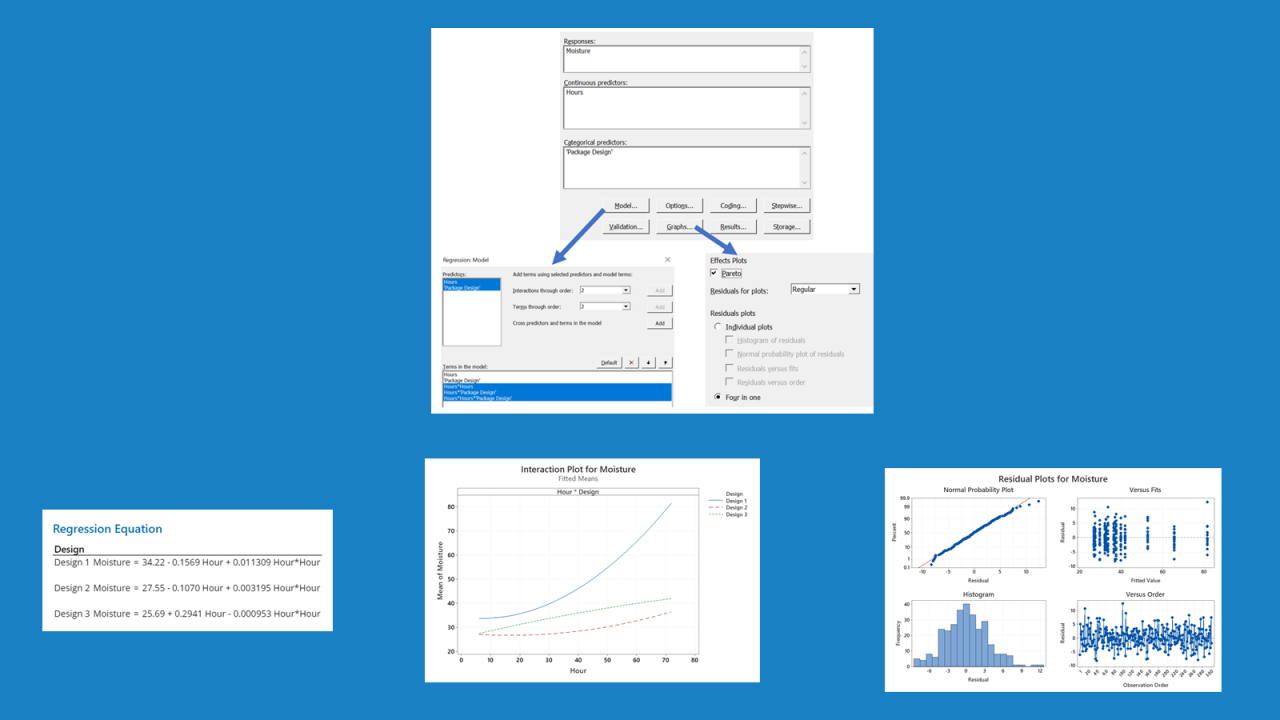
Read about building, verifying and visualizing a regression model in Minitab Statistical Software.
If you’re serious about leveraging predictive analytics to solve everyday challenges, then boost your analytical power with best-in-class, accurate machine learning algorithms! They provide deeper insights into your data. In addition to providing traditional statistical methods, we’ve made more advanced methods like tree-based methods more accessible to everyone.
Take your regression skills out of this world with MARS ® (Multivariate Adaptive Regression Splines) or advance your skills by using our tree-based methods like Classification and Regression Trees, better known as CART ® , Random Forests ® , and gradient boosting, better known as TreeNet ® . Not sure what method to choose? Easily confirm you're using the best predictive model to answer your question with Automated Machine Learning (Auto-ML). Perfect for those who are new to predictive analytics and need recommendations, and experts looking for a second opinion.
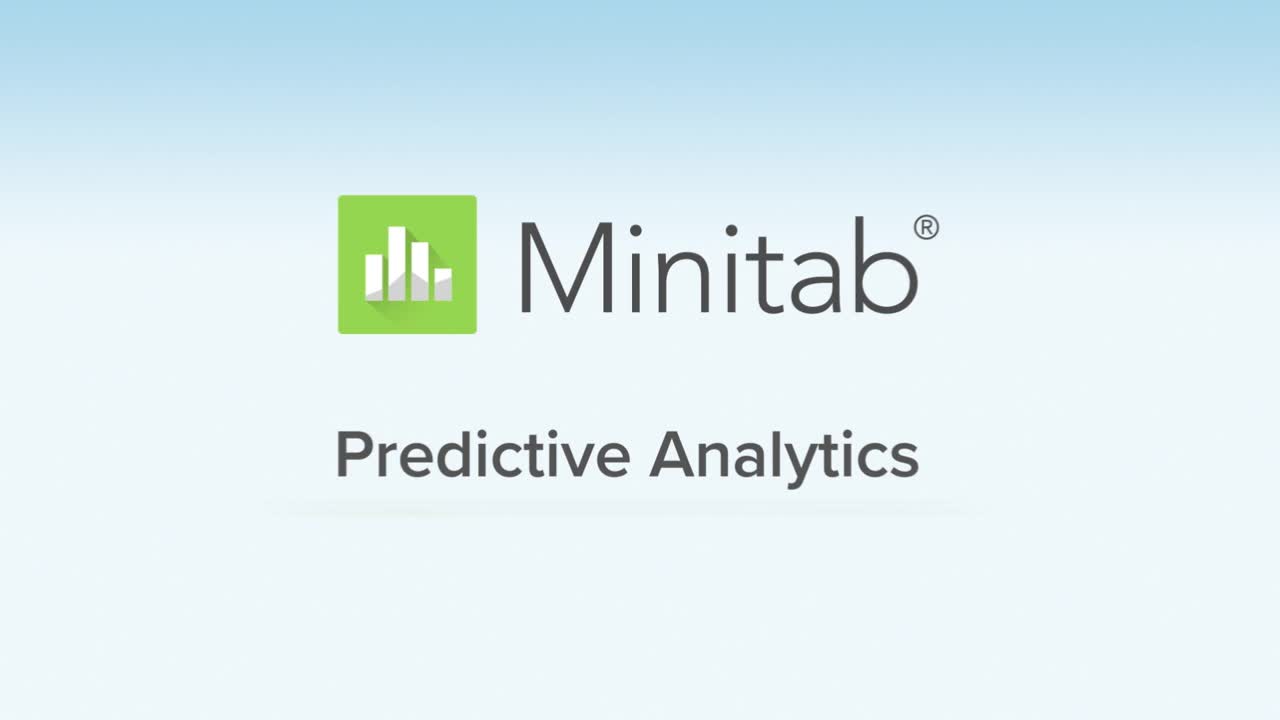
Minitab is the market leader in quality tools because of the vast array of powerful tools we offer, the ease of using them, and the training and education we provide to help organizations continuously improve. Minitab sets the standard for quality analytics tools, such as Statistical Process Control , including Control Charts and Capability Analysis , Measurement System Analysis . In addition to statistical methods typically used in quality improvement, Minitab also offers Brainstorming tools and FMEAs.
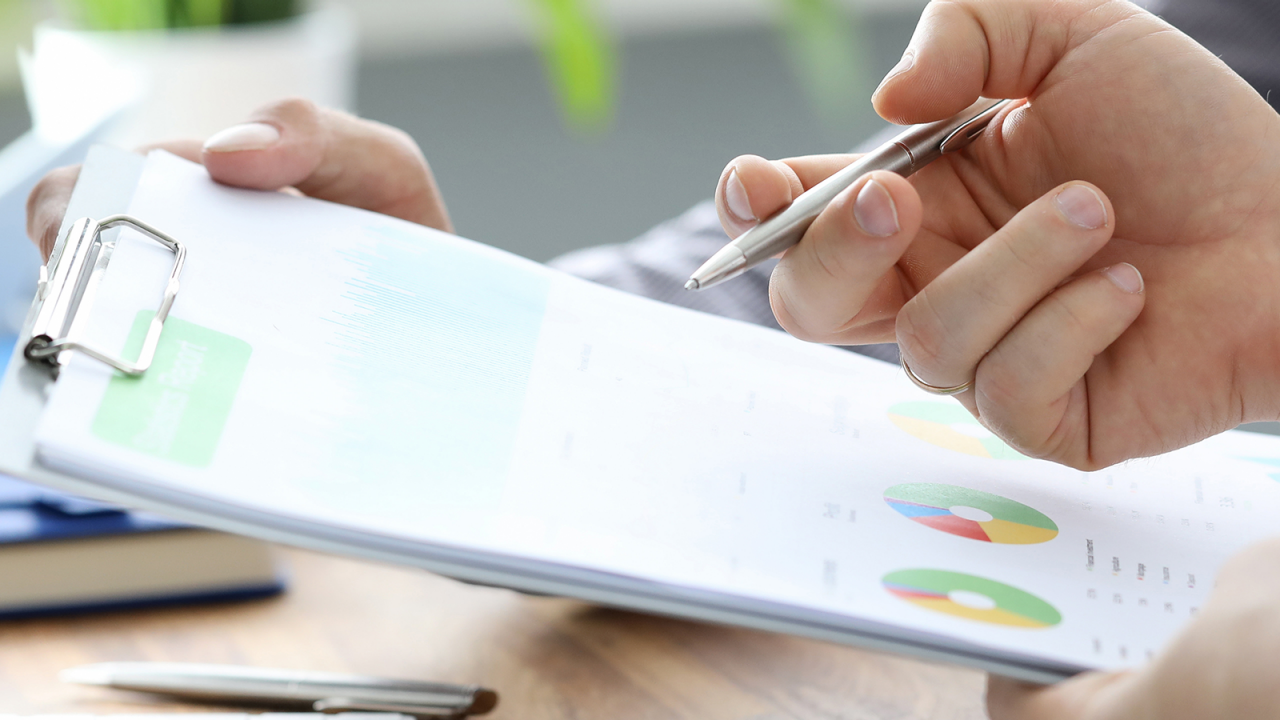
You probably know Design of Experiments (DOE) are the most efficient way to optimize and improve your process. But many of us find DOE intimidating, especially if it's not a tool we use often. How do you select an appropriate design, and ensure you've got the right number of factors and levels? And after you've gathered your data, how do you pick the right model for your analysis? One way to get started with DOE is the Assistant in Minitab Statistical Software.
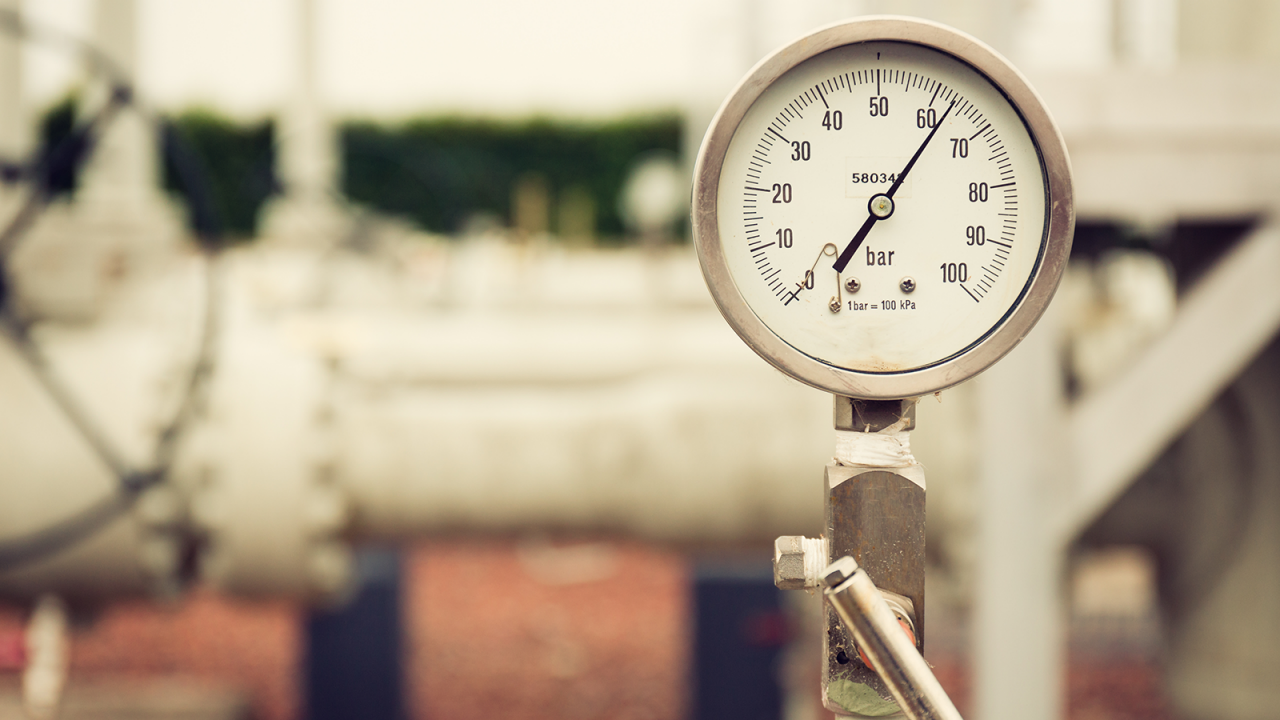
In cases like equipment failure, the original equipment manufacturers (OEM) would be at fault. These OEMS must then quickly find the root cause and determine the risk for other vehicles still operating in field so they can predict how many additional vehicles will return with the same problem or continue driving with no problems at all (also called survivors). Eventually if the risk is high enough, a vehicle recall in that market could be necessary. Learn how one company predicted and prevented product failure.
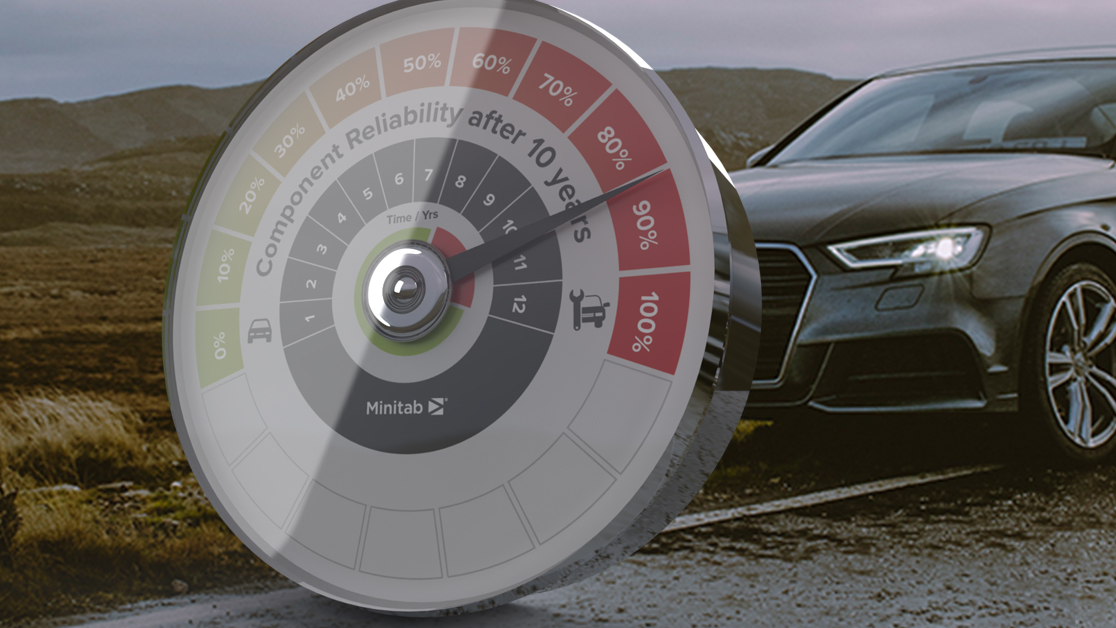
Modeling time series analysis requires different methods and comparisons of models. Always check accuracy measurements to see which model is minimizing the errors. It is also good practice to predict forecasts on data you currently have collected to confirm the best fitting model, before you make forecasts on unobserved data. Learn more in this blog.
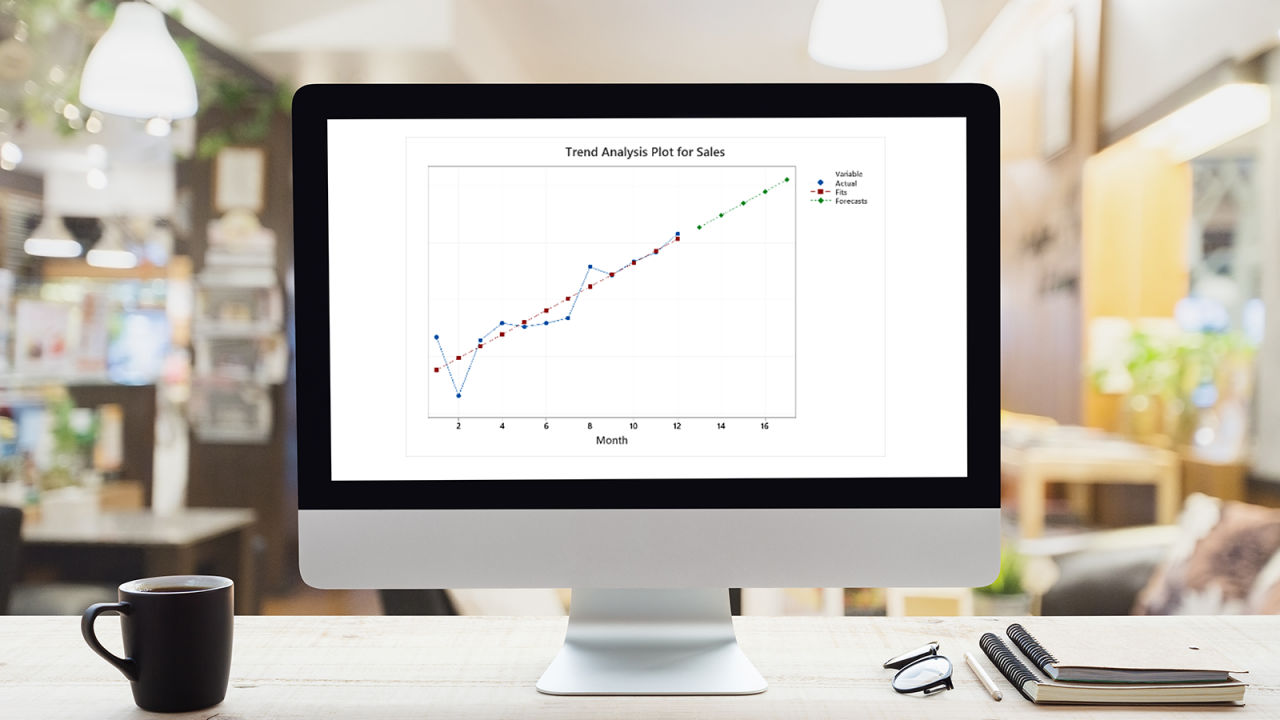
Monte Carlo simulations have come a long way since they were initially applied in the 1940s, when scientists working on the atomic bomb calculated the probabilities of one fissioning uranium atom causing a fission reaction in another. Today, using Minitab’s solutions, you can simulate the range of possible outcomes to aid in decision-making, forecast financial results or estimate project timelines, understand the variability in a process or system, or manage risk by understanding cost/benefit relationships.
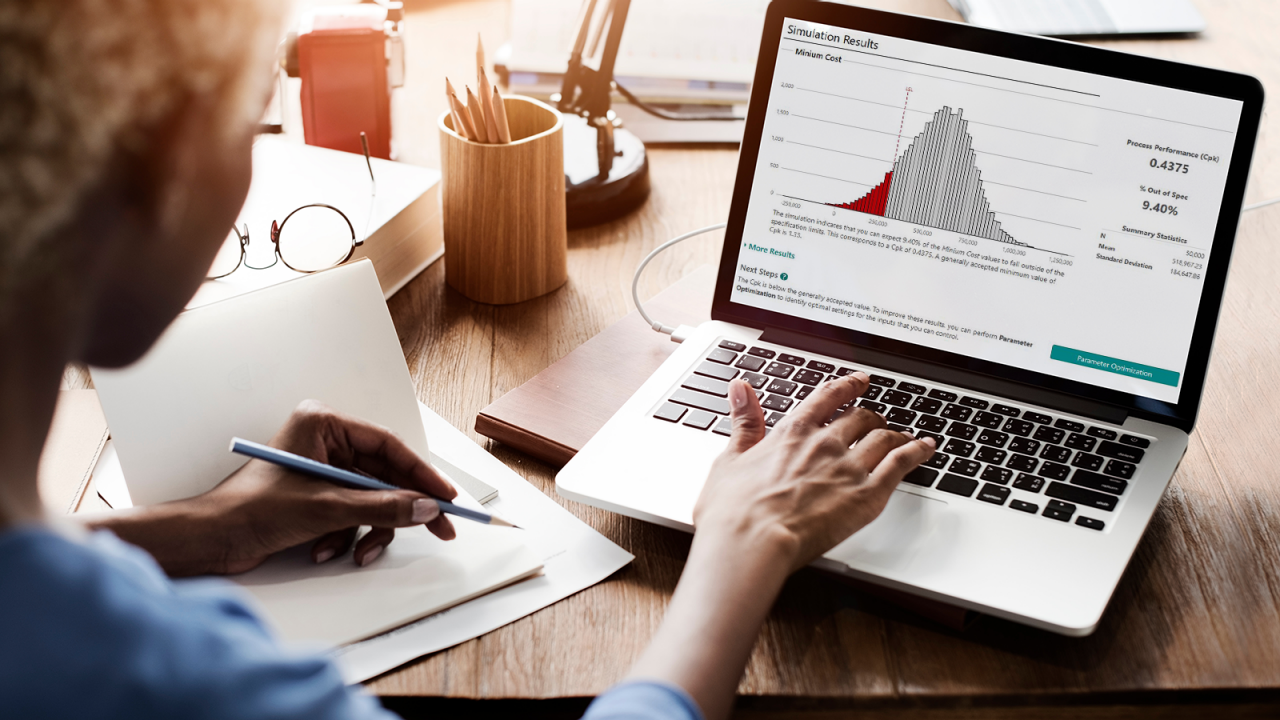
Powerful, Market-Leading, and Easy-to-Use Statistical Software
Knowing how to analyze data can be overwhelming. The good news is MinitabStatistical Software can analyze your data for you, so you can focus on what’s most important: Making great data-informed decisions.
Only Minitab provides comprehensive visualizations and analytics with the platform flexibility you need. Whether it’s an exploratory investigation into your data, an in-depth analysis, or a dashboard full of insights to share with your team, Minitab has the statistical methods you need to turn data into knowledge.
DID YOU KNOW?
90% of the Fortune 100 and 70% of the Fortune 500 use Minitab Statistical Software.
OUR CUSTOMERS
“With Minitab, you can do a wide variety of statistical [analysis] with seamless effort. Also, it offers a good documentation of its functionalities, including the theory behind it.”
Romario G. Consultant
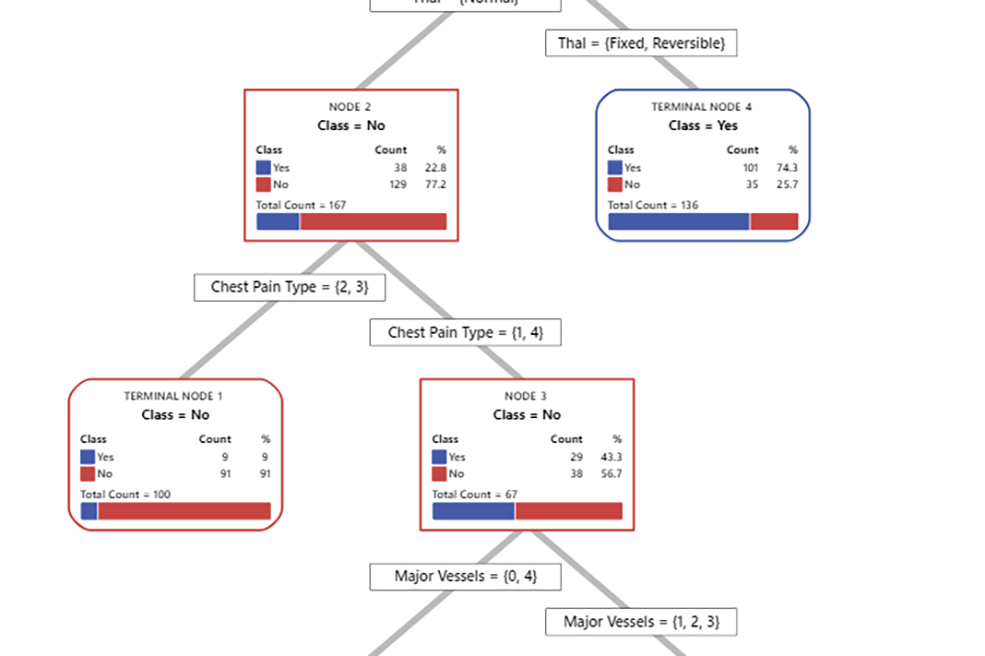
"The tools built into Minitab make it easy for even a novice statistician to get meaningful results. Help topics and examples are plentiful with the option to explore the theory behind the recommended tests through supporting topics.”
Travis B. Quality Engineer
- Trust Center
© 2024 Minitab, LLC. All Rights Reserved.
- Terms of Use
- Privacy Notice
- Cookie Settings
You are now leaving minitab.com.
Click Continue to proceed to:
- Open access
- Published: 03 December 2022
Negative emotions experienced by healthcare staff following medication administration errors: a descriptive study using text-mining and content analysis of incident data
- Sanu Mahat 1 ,
- Anne Marie Rafferty 2 ,
- Katri Vehviläinen-Julkunen 3 , 4 &
- Marja Härkänen 1
BMC Health Services Research volume 22 , Article number: 1474 ( 2022 ) Cite this article
4482 Accesses
4 Citations
73 Altmetric
Metrics details
Medication errors regardless of the degree of patient harm can have a negative emotional impact on the healthcare staff involved. The potential for self-victimization of healthcare staff following medication errors can add to the moral distress of healthcare staff. The stigma associated with errors and their disclosure often haunts healthcare professionals, leading them to question their own professional competence. This paper investigates the negative emotions expressed by healthcare staff in their reported medication administration error incidents along with the immediate responses they received from their seniors and colleagues after the incident.
This is a retrospective study using a qualitative descriptive design and text mining. This study includes free-text descriptions of medication administration error incidents ( n = 72,390) reported to National Reporting & Learning System in 2016 from England and Wales. Text-mining by SAS text miner and content analysis was used to analyse the data.
Analysis of data led to the extraction of 93 initial codes and two categories i.e., 1) negative emotions expressed by healthcare staff which included 4 sub-categories of feelings: (i) fear; (ii) disturbed; (iii) sadness; (iv) guilt and 2) Immediate response from seniors and colleagues which included 2 sub-categories: (i) Reassurance and support and (ii) Guidance on what to do after an error.
Negative emotions expressed by healthcare staff when reporting medication errors could be a catalyst for learning and system change. However, negative emotions when internalized as fear, guilt, or self-blame, could have a negative impact on the mental health of individuals concerned, reporting culture, and opportunities for learning from the error. Findings from this study, hence, call for future research to investigate the impact of negative emotions on healthcare staff well-being and identify ways to mitigate these in practice.
Peer Review reports
Medication Errors (MEs) are recognized by the World Health Organization as the leading cause of injury and avoidable harm in healthcare, costing approximately 42 billion dollars annually, which is nearly 1% of total global health expenditure [ 1 ]. The safety of patients is at the forefront of the healthcare system; however, healthcare staff can also be traumatized by the aftermath of MEs. Although the healthcare mantra is “first do no harm”, healthcare professionals involved in adverse events can feel guilt, shame, anger, fear, and anxiety [ 2 ]. They are often neglected with only a few coping strategies and support systems available to help them [ 3 ]. Negative consequences of an adverse event can reach far beyond the “first victim” i.e., the patient. Thus, affecting healthcare staff psychologically making them “second victims” [ 4 ]. The term “second victim” was first coined by Dr. Albert Wu to explain the emotions of a young resident who committed an error and had experienced ridicule, shame, and lack of support, from his peers [ 2 ]. Although this concept was first applied to physicians, other healthcare staff, including nurses, also experience similar emotions. Scott et al. [ 5 ] described the term second victim as “a healthcare provider involved in an unanticipated adverse patient event, medical error and/or a patient-related injury who has become victimized in the sense that the provider is traumatized by the event. Frequently, second victims feel personally responsible for the unexpected patient outcomes and experience as though they have failed their patient, feeling doubts about their clinical skills and knowledge base”[ 5 ].
The use of the term second victim has been criticized recently [ 6 , 7 ] arguing that it might act as a way in which healthcare providers can evade responsibility and accountability and it might be offensive to affected patients and families [ 6 ]. Laying accountability at the door of an individual, ignoring the wider organizational ramifications of accountability in terms of the conditions which trigger errors in the first place, can let the organization off the hook. Even though the use of the term “victim” may sound spurious and uncomfortable to many healthcare professionals, patients, and families, it is indubitably an advantage in reinforcing the seriousness and urgency of the problem among policymakers and healthcare managers [ 8 ]. Wu et al.[ 8 ] have suggested the importance of the use of the term second victim as it is notable and denotes urgency. These assumptions regarding the use of the term second victim are inherent in both positions. Therefore, our research is designed to take this debate one step further by analyzing the consequences of errors in terms of emotional response and lived experiences of healthcare staff.
Regardless of the degree of patient harm, the mere thought of potential patient injury caused by ME is sufficient to induce the feelings of fear, distress, anger, anxiety, guilt and remorse in healthcare staff [ 9 , 10 , 11 ]. Although evidence suggests multiple system-based causes of MEs, the error-maker still tends to blame themselves i.e., they should have functioned proficiently [ 11 ]. If the seriousness of these issues remains unaddressed, it can negatively affect healthcare workers’ personal and professional well-being causing depression, burnout, Post Traumatic Stress Disorder (PTSD), and even suicidal thoughts [ 4 , 12 , 13 ]. Error prevention has therefore been a focus of major attention for healthcare organizations for years but the impact of MEs on the healthcare professional involved has received less attention. A more nuanced and textured exploration of the impact of the problem upon healthcare workers is required if preventative strategies are to be effective [ 11 ].
Previous studies have shown that often MEs causing harm are reported whereas near misses are often under-reported [ 14 ]. This underestimates the number of healthcare staff going through negative experiences [ 15 ]. Fear of legal consequences, blame, losing patients’ trust, and punishment have been recognized as barriers to ME reporting[ 16 ] leading healthcare staff to suffer in silence, sometimes struggling alone in isolation and burdened with a sense of shame [ 9 ]. Therefore, a system is needed to mitigate these barriers and create a “just culture guide” which helps healthcare managers to treat staff involved in adverse events fairly, support open and fair culture and maximize learning from errors [ 17 ]. However, it is apparent that irrespective of organizational effort in promoting a just and no-blame culture, the stigma persists with respect to speaking up about errors [ 18 ].
Patient safety incident reporting has become a common practice, but little is known about the feelings of those who commit or witness incidents. Despite the recent debate regarding the use of the term second victim, we are adopting this terminology throughout our research to analyse the consequences of MEs in terms of psychological responses from healthcare staff. Previous research into second victims has mainly been carried out in a single setting, but this study uses reported incidents at a national level drawing from a range of settings. Also, no previous studies, as far as we are aware, have focused only on Medication Administration Errors (MAEs). To our knowledge, none of these studies have used free-text descriptions of reported medication incidents to review the feelings and emotional responses associated with reporting nor text mining as an innovative method for such analysis.
The aim of this study was to investigate negative emotions expressed by healthcare staff in their reported MAE incidents along with the immediate responses they received from their seniors and colleagues after the incident.
Study design and setting
A retrospective study using qualitative descriptive method and text-mining with an inductive content analysis of the incident data related to Medication Administration (MA) reported in England and Wales was done.
Description of the data
The data consists of MA incidents ( n = 72,390) retrieved from the National reporting and Learning System (NRLS) database based on inclusion criteria: (1) incidents reported to have occurred in England and Wales between 1 January and 31 December 2016, (2) medication incident, (3) administration/supply of medicine from a clinical area, and (4) acute National Health Services (NHS) trust (either specialist or non-specialist). The data included incident reports from all levels of healthcare staff ranging from student nurses to senior-level health professionals who were involved in and who have witnessed the MAE incidents.
Data were acquired from NHS England and NHS Improvement. NRLS is largely voluntary and is the only database that includes all types of patient safety incidents. This study used free-text descriptions of the incidents i.e., healthcare staffs’ descriptions of “what has happened?” or “when the incident occurred?” during the medication process.
Data analysis
First, negative emotional expressions associated with MAEs were defined using the literature and dictionaries (Oxford Learners’ Dictionary, Merriam-Websters’ Dictionary, and Cambridge Dictionary) to define synonyms of the negative emotional expressions (Table 1 ). Second, those expressions were searched from the free-text descriptions of the incidents which were specifically related to MA. For that, The SAS® Enterprise Miner 13.2 and its Text Miner tool were used. Multiple steps were followed for data analysis as described in Fig. 1 . SAS® Text Miner automatically processes the data using ‘text parsing’ i.e., converting unstructured text into a structured form. Text parsing includes tokenization (breaking text into words/terms), stemming (which chops off the end of words reducing words to their stem or root forms), and part-of text tagging (for each word, the algorithm decides whether it is a noun, verb, adjective, adverb, preposition and so on). ‘Text filtering’ was then used to reduce the total number of parsed terms and check the spellings. The English language was chosen for parsing and filtering the text. Using an interactive filter viewer, negative emotional expressions described in the free text were identified and the number of each expression was collected (See Supplementary file 1 ). For the next phase of the analysis, the most common expressions were chosen which are bolded in online-only material 2 (See Supplementary file 2 ).
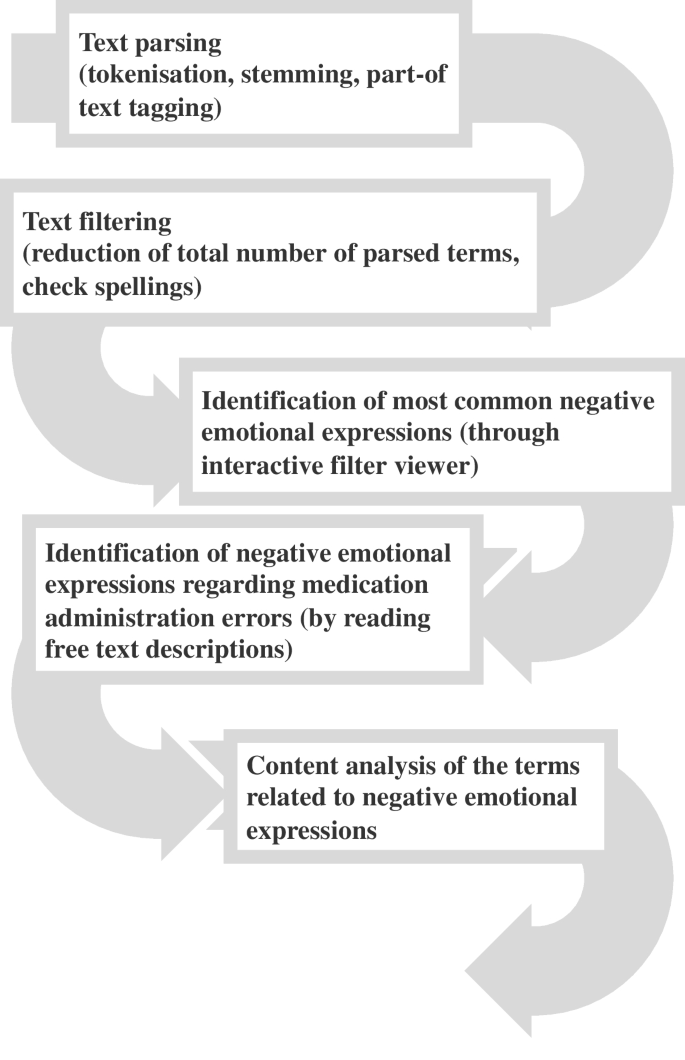
Analysis process of medication administration incident reports’ free text descriptions
Expressions chosen for analysis were used as a search term in an interactive filter viewer. All the descriptions of the incidents that included those expressions (a total of 1861 incident reports) were collected and read through repeatedly. In the first phase of this analysis, the aim was to define who had experienced the emotional feeling. Most of negative emotions were expressed by patients or relatives (See Supplementary file 1 ). Those descriptions of incidents that included negative emotions expressed by healthcare staff and which were expressed in relation to MAEs ( n = 93) were then selected for further analysis.
Content analysis was used to analyze the data. The lead author followed an inductive content analysis where the researchers carefully read, organized, and integrated and formed categories, concepts, and themes by comparing the similarities and differences between the coded data [ 19 ]. The lead author read through the data repeatedly and during this process, identified the main theme which is: Emotional expressions of healthcare staff after MAEs. The data were organized into main themes and sub-themes. After the preliminary classification, a co-coder [the last author of this paper] participated in the analysis and read the classification structure and the related data independently. Once thematic saturation was achieved, both researchers analyzed the entire data corpus according to standard thematic analysis techniques [ 20 ]. All authors contributed to the final form of the analysis. Finally, direct quotes were used to support the findings.
Negative emotional expressions of healthcare staff after MAEs
We found 15 different types of negative emotional expressions used including worry, anxiety, annoyance, agitation, stress, unhappiness, distress, concern, anger, upset, shock, sorry, fault, depression, and frustration. These 15 different types of emotions were expressed 1,861 times in the incident reports (See Supplementary file 1 ).
Among those emotional expressions, 12 were exhibited by the healthcare staff and were mentioned 154 times. Only eight of those 12 expressions: worry, upset, agitation, faulty, sorry, concerned, stressed, and distress were expressed by healthcare staff in direct relation to MAEs, the frequency of expression here was 93 times. The data extraction process in presented as a flowchart in Fig. 2 .
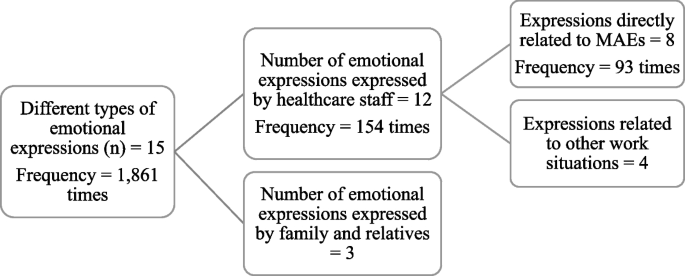
Typology and frequency of emotional expressions
The key emotions revealed were further classified into four categories: (1) feeling of fear, (2) feeling of upset, (3) feeling of sadness, and (4) feeling of guilt (Table 2 ).
Feeling of fear
Healthcare staff described their feeling of fear regarding MAEs using four different synonyms i.e., distressed, concerned, stressed, and worried. Staff mentioned how fearful they were when they discovered their mistakes. Distress was revealed in three of the incident reports as expressions of fear of healthcare staff. Usually, MAE incidents were reported either by the error-makers themselves or by those witnessing their errors. One of the staff described the fear felt by her colleague (staff nurse) by reporting how distressed he was after he administered a medication through wrong route (intravenous instead of oral):
“ I was assessing a patient on Ward X when a staff nurse approached me extremely distressed and agitated. He then ran into the utility without explaining what the problem was. I followed him…nurses were present who proceeded to explain that the nurse who approached me had given a patient 2mls of Oramorph [liquid morphine that has to be given orally] intravenously …"
Healthcare staff also expressed the extreme pressure which acted as an important contextual trigger, driving intense the feelings of fear. Another emotion linked to fear was “concerned” which was expressed in 23 cases by healthcare staff after making an error. One of the healthcare staff reported an error (prescribed wrong strength), which the staff realized two hours later and became concerned about it:
" Prescribed TTA (to take away) of ‘Augmentin [Amoxicillin Clavulanate] Duo’125/31 8 ml TDS [three times a day]. As written, this would be a drug error-there is no 125/31 strength of …This was my error, which I realized and became concerned about 2 hours later …"
Stress was expressed in three cases by healthcare staff while reporting the incident; however, this emotion was expressed by staff not as their feelings after MAEs, but as the reason underlying MAEs. These kinds of explanations were found in many incident reports where healthcare staff accepted the error but eventually pointed towards other hidden causes behind the error:
" Gave Clexane [Enoxaparin] 60 mg to wrong patient. Ward extremely busy- heavy workload and was very stressed due to workload …"
Being “worried” was another expression of fear reported in 11 incident reports by healthcare staff. They were found to be worried about several situations such as the health of patient, degree of harm caused by error, associated legal procedures, and their professional career. One staff nurse was worried about the patients’ condition as he did not administer insulin dosage to one of his patients:
" Staff nurse came to me at the end of the shift and stated that he thought that the patients’ insulin was prescribed prn [whenever necessary] and had not given any…I explained he needed to inform the nurse in charge…he was very sincere and worried that he had not given this insulin …"
Feeling disturbed
The feeling of being disturbed was expressed using two synonyms: upset and agitated. They addressed themselves as being upset in 24 incident reports following MAEs committed either by themselves or by their fellow staff. Healthcare staff reported the error made by fellow staff member and described the emotion of his/her colleague as:
" Nurse called me was very upset to explain that she had given wrong treatment to patient …"
Even near miss situations have caused healthcare staff to get emotionally disturbed. Even after apologizing with patient and family, healthcare staff felt upset thinking that if they were not aware of the near miss situation in time, patients’ condition would have been severe:
" SN asked me to do a syringe driver with her for a palliative patient…on drawing up the ketamine driver, myself and SN made a drug error in which we drew 5 times more ketamine than the required dose…The family and patient have been informed of the drug error we made and we gave our sincere apology for our faults…both myself and SN are very upset with the near miss situation and aware that things could have gone very differently …"
Healthcare staff expressed being agitated in two reports after discovering that they had committed MAEs, except in some situations, where staff though agitated denied their mistake by underestimating the severity of the error they made:
" Patient was discharged off the system by the nurse without confirming with medical team/pharmacy that patient was ready to go… Patient left without anti-sickness medication which the team had told her she could have…Nurse was evidently agitated that the incident was being reported and did not understand that she should check with the team before authorizing …"
Some reports revealed extreme negative emotions associated with feelings of upset such as being devastated and questioning one’s own professional competence. The use of such intense and traumatic language can reflect how much the healthcare staff were impacted and even emotionally wrecked after MAE. One healthcare staff after accidentally administering wrong dosage to the patient, reported that the error was entirely his/her own fault:
" Pt px 120 mg on gentamicin on EOMA, I accidentally gave 210 mg in error. This was entirely my fault …The checker confirmed what I had done. I am so devastated about this and really upset I’d made such a mistake…today was just hectic and I lost concentration ..."
Feeling of sadness
Healthcare staff expressed their feeling of sadness at being sorry for the mistake they had made; it was one of the most common negative emotional expressions expressed in 13 cases. Most staff used this to express a sense of remorse after the error. After missing a dose of insulin for a patient, one healthcare staff expressed his/her sadness by stating that he/she is sorry about the incident:
" I am sorry to say that I missed one dose of insulin (at 22.30…) for one of my patients …"
Along with the feeling of sadness, one healthcare staff also mentioned about learning from the error and how he/she have accepted that she was wrong to assume things:
" I was sitting at the desk, staff nurse handed me a tray with intravenous antibiotics and said, here is one because I had given her patient drug chart, I assume it was patients’ medication. I did not take the drug chart with me to the patient and afterwards when staff nurse came with patients’ drug, I realized I have given the wrong drug. I was very upset as I have never done anything in this form before. I always take the drug chart with me to the patient. I am deeply sorry, and this is a massive learning curve for me, I hold my hand up it was wrong to assume this ."
Healthcare staff who had mentioned learning from the error was quite common in many incident reports. However, there were few cases where the staff did not understand the seriousness of the error she has caused:
"… I spoke to the student nurse about the seriousness of her actions, she said sorry; however, I did not feel she understood the seriousness of what she did …"
Feeling of guilt
In 14 incident reporting cases, healthcare staff were aware of their mistakes and the consequences they might have. They expressed their guilt and identified themselves as being at fault and blaming themselves.
" IV flucloxacillin drawn up and checked by myself and staff nurse…administered drug however in error name band/ allergy band not checked. Realized immediately after administration that I had gone to the wrong patient and given the incorrect medication…conversation with senior staff nurse about error. Explained that the error was my fault completely…patient does not appear to have come to any harm …"
However, this emotion was not just expressed following the error, but also as another reason for error attribution. For example, in the report below, a staff member made an error, and blamed herself and phone reception for being muffled:
" I had to hand over two diabetic patients to the 5–8 pm. I rang Ward sister and confirmed this again later. However, patient was not reallocated, and insulin omitted…Ward sister apologized for yesterday missed patient…she said the reception to her phone was muffled and that it was her fault …"
Immediate response from seniors and colleagues
Some of the healthcare staff while reporting their feelings behind MAE incidents also discussed regarding the immediate responses they received from their seniors and colleagues. Healthcare staff explained how their seniors and colleagues responded after they were informed about MAEs. These responses are categorized into two sub-categories: (1) Reassurance and support and (2) Guidance on what to do after an error.
Reassurance and support
In three incident reports, healthcare staff mentioned about the reassurance and positive support they received from their seniors and colleagues after the disclosure of MAEs, about how they tried to handle the situation very calmly without getting angry. This helped them to cope effectively without undue stress and burden. A nurse mentioned that she reassured one of her colleagues who was very disturbed after she gave the wrong medication to her patient:
" Staff nurse by mistake gave the patient wrong medication…. misread the information by being interrupted by a patient and member of staff…. I reassured the staff nurse as she was very upset …"
Even a little support and reassurance and few kind words during the time of MAEs can help the healthcare staff to cope up with the situation effectively. As one member remarked:
" Medication error – digoxin prescribed in two doses (125mcg and 62.5mcg) did not realize and administered…Immediately alerted sister in-charge of ward and contacted doctor. Doctor did not come to the ward but was happy that observations had been recorded…and told us not to worry …"
Guidance on what to do after error
In 11 incident reports, healthcare staff mentioned about receiving advice from their seniors and colleagues regarding the right thing to do after making an error. They have been guided to observe the situation of the patient to ensure that no serious harm would be caused to them:
"… Administered the oramorph in an unlabeled syringe which was in the same tray as a 10ml flush…I discussed the situation with the medical registrar on call who advised me to monitor observations regularly …" "… I spoke to the nurse in charge after the error from the following shift who said that I should speak to the ward manager at the earliest opportunity which I did …"
Furthermore, in cases where healthcare staff neglected to document the incident, a colleague intervened to guide the staff member to follow the protocol. As one staff member described:
"… I discussed the incident with a colleague shortly afterwards. However, I neglected to escalate and correctly document the incident…The aforementioned colleague has since approached me to discuss the incident, further to this I approached and discussed the incident with my ward manager …"
Our study identified four categories of negative emotions expressed in incident reports: feelings of fear, disturbed, sadness, and guilt with various sub-categories. In addition, this study also captured the immediate responses received by healthcare staff after they informed their seniors and colleagues about MAEs including the reassurance, support, and guidance on what to do after an error. Incident reporting by healthcare staff in this study indicated that unintentional harm caused due to MAEs and even near misses can affect the healthcare staff involved in error emotionally, increasing their risk of becoming the second victim of MAEs, confirming previous research [ 9 , 21 ].
A major finding of this study was the negative emotions experienced by healthcare staff after MAEs. Healthcare staff in this study expressed their fear while reporting incidents by using negative emotions such as stressed, distressed, concerned, and worried. They not only blamed themselves for these mistakes, but also considered other additional explanations which, they perceived as causing the error. These kinds of emotions can be related to staff members’ narration of fear and anxiety for patients’ well-being and for their own professional careers [ 22 ]. Similarly, feelings of being disturbed expressed as being upset and agitated were widely mentioned in incident reports. Identical reasons such as realization of the error and thoughts of the possible seriousness of the error and associated issues lay behind emotions. Further, feeling of sadness expressed as being sorry for the mistake made was another most common emotional expression. Also, healthcare staff felt a deep burden of responsibility for their actions. Feelings of being guilty or at fault is one of the risk factors for healthcare staff for becoming the second victim of MEs. It can also cause loss of self-esteem and inculcate a sense of failure and hopelessness. In a similar study by Treiber & Jones [ 22 ], nurses, upon committing even minor errors, expressed raw and painful emotions, regardless of the degree of harm. Nurses can often recall the details of the error and what they felt at that time [ 22 ]. While the lack of any apparent linkage between emotional response and degree of patient harm might appear counter intuitive, one possible explanation might be that healthcare professionals are not well enough supported by their organizations to cope with any form of negative experience. Thus, those affected might develop strong negative emotion [ 23 ].
Making an error might also have serious consequences for disrupting the personal and professional lives of staff, causing personal and moral distress, and affecting the quality and safety of patient care [ 23 ]. It is crucial to pay attention to these emotional expressions as incidents that are sensitive and make an impact, are often remembered, and reflected in the attempt to prevent recurrence. On the other hand, these incidents can unintentionally impose a mental burden on healthcare staff making them second victim [ 2 ]. Our findings confirms that MAEs can generate negative feelings in healthcare staff associated with it, which can endure long beyond the immediate effect.
Research has confirmed a direct relationship between nurse staffing and missed patient care [ 24 , 25 ], revealing poor nurse staffing as a risk factor for MEs along with other organizational factors such as poor working conditions, distractions, and high workload [ 26 ]. Similarly, in this study, reporters mentioned their own actions as a trigger for MAEs along with the above-mentioned factors whereas some reporters explained organizational and environmental conditions and context surrounding the error as reasons to reduce blame. In the absence of support, self-blame seems to assume greater prominence. This can have long-term repercussions for maintaining emotional health and well-being, a major failure of workforce strategy, especially during the pandemic situations.
The current study also found other healthcare authorities responding in several ways after being informed about MAEs. Sometimes, staff may not know what to do after MEs, they might panic and lose control. Thus, adequate support from colleagues and seniors sensitive to these issues may prevent the error-makers from translating further into second victimhood of MEs. How the organization and related individuals responds is clearly linked to the emotional impact the error can have on the healthcare staff who made the error. Appropriate support and guidance from seniors and colleagues have been found to alleviate the suffering, while lack of support has increased their psychological burden [ 27 ]. Some of the healthcare professionals in our study also opted for consulting with their seniors: doctors, colleagues, and mentors after MAEs and reported about how they have received guidance and suggestions, which helped them to cope effectively. Emotional support plays a vital role in restoring faith and confidence among healthcare professionals in patient safety. Support from co-workers and healthcare institution helps the error-makers to retain a sense of control [ 2 ]. Reassurance from seniors and colleagues can also strengthen healthcare staff’s self-esteem and facilitate the correct reporting of MAEs. As is well known, only a fraction of incidents are reported thus deterring the improvement of patient safety with barriers identified as time pressure, fear of the consequences [ 28 ], poor institutional support, lack of feedback, a blame culture, and inadequate training [ 15 ]. Yet, we can still improve patient safety by identifying these barriers. Moreover, while some staff members perhaps too readily assumed responsibility for errors, as reflected in the prominence of self-blame, others demonstrated reluctance, which could be linked to fear of the consequences of MAEs. Furthermore, little is known about the dynamics and consequences of reporting-what prompts some to report and others not to do so. We demonstrate that the emotional expression of staff can be extremely distressing and negatively impact health and well-being of healthcare staff.
Implications for practice
Our findings indicate that immediate negative feelings experienced by healthcare staff after making MAEs can have long-lasting impacts that stretch far beyond the event itself thus potentially traumatizing them and inducing ruminative thoughts, which trigger the memory. The short, medium, and long-term consequences of errors are unknown as yet but could contribute to burnout and other factors associated with intention to leave the profession. Indeed, a negative memory that will stay with them forever, if not handled accurately. They could potentially become second victims of an error, if unable to confront and deal with negative feelings associated with the error. One source of challenge could be stigma related to this making it difficult to continue to work after MAE. Our findings suggest appropriate guidance and support from fellow staff members could help healthcare staff to handle the situation effectively. Therefore, it should be paramount to tailor appropriate support from persons in-charge and colleagues and to promote an open culture where it is understood. Errors can impair mental health of those who are involved, hence, the system triggers surrounding such errors need to be understood and prevented. In addition, more detailed information about these emotions after incidents and their long-term consequences on emotional well-being should be studied in future.
Implications for research
The negative feelings expressed by healthcare staff after MAEs identified in this study could provide the basis for designing an intervention study to support emotionally affected staff in healthcare institutions. It could be helpful to design a support program which recognizes the importance of expressed emotion and its consequences for internationalizing a sense of self blame and victimhood and the long-term repercussions this might have for the mental health and well-being of the health workforce.
Strengths and Limitations
As far as we are aware, this is the first-time text-mining and content analysis have been used to identify negative emotions reported by healthcare staffs’ MAEs, derived from free text in a large national database. A text-mining approach was used for identifying reports that included emotional expressions, as manual data analysis would have been almost impossible for such a big data set and this approach has been recognized to be time-effective in analysing big-data regarding medication incidents (Härkänen et al., 2019). Further, the emotional expressions identified in this study are relatively rare. These descriptive data of emotional expressions nevertheless cast light on the issues related to MAEs. Furthermore, the researchers adhered to the Standards for Reporting Qualitative Research (SRQR) checklist (see the list in Supplementary file 3 ).
However, while analyzing the free-text descriptions, we may have missed some important expressions as this was a pilot methodology we were testing, subjective decisions were made. Similarly, it was very difficult to combine the synonyms of the word used to express the negative emotions which can give rise to ambiguities. For example, in many cases, one single word could either be a verb, or noun or an adjective i.e., words can have different implication [ 29 ]. On the contrary, this study sheds some light upon how important it is to write incident report and to identify the negative emotions of staff, to prevent further consequences from occurring, encourage reporting and put support mechanisms in place. Patient safety incident data is likely to contain some limitations, more specifically, reporting error and bias which will affect the number, type and temporality of reported incidents and data interpretation [ 30 ]. Since reporting is largely voluntary, there are some potential limitations of NRLS being a reliable indicator of exact number of incidents. Nevertheless, increasing number of incidents may reflect an improved reporting culture. Further, the methodology did not allow for the identification of any positive emotions that might have been expressed by healthcare staff when reporting MAE incidents, as only free-text descriptions which included negative emotions were analyzed .From the free text-descriptions, most of the reports were found to be from nurses, however, staff-specific generalizability and scope is limited due to lack of staff type identification in NRLS data i.e., ST01 [ 31 ]. This makes it difficult to precisely quantify the impact and potential benefits of this research.
A wide range of negative emotions was expressed by healthcare staff after reported MA incidents. However, the associated psychological trauma and low mood expressed by healthcare staff represent significant negative impacts underlying reported negative emotions. It is more likely that MAE incidents are under-reported, therefore problems could be much higher in terms of prevalence and magnitude. There was tremendous variation in reports of healthcare staff encountering with MAEs; some reacted in extremely negative ways, whereas the majority expressed little about their feelings. Although many of the incident reporters did not express their feelings in their reports, there is also the possibility of them being affected by the aftermath of MAEs. Several actions were taken by healthcare staff to help cope with the error: which included, seeking guidance, reassuring, and supporting each other. This calls for further efforts from healthcare organizations to support healthcare staff as a matter of routine when encouraging reporting. Though we do know little about the long-term consequences, from what we see in our data, the scarring effect could potentially be considerable. Therefore, support programs need to be co-designed but incentivize to reward reporting without imposing an emotional burden on already overburdened staff. This is vital for error reporting, safety, and ultimately prevention to flourish in the long run. First and foremost, the system needs to promote psychological safety for its users, which our research currently demonstrates.
Availability of data and materials
Data supporting the findings of this study are made available from NRLS/NHS Improvement. However, restrictions apply to the availability of these data. For this current study, these data were used under license, therefore, are not publicly available. Data are however available if contacted to authors (MH, AMR, SM) upon reasonable request and with permission from NRLS/NHS Improvement.
Abbreviations
Medication Error
Medication Administration Error
Medication Administration
National Reporting and Learning System
National Health Services
Standards for Reporting Qualitative Research
World Health Organization. WHO launches global effort to halve medication-related errors in 5 years [Internet]. 2017 [Cited 2021 Jun 21]. Available from: https://www.who.int/news/item/29-03-2017-who-launches-global-effort-to-halve-medication-related-errors-in-5-years .
Wu AW. Medical error: The second victim. BMJ. 2000;18(7237):726–7. 320.
Article Google Scholar
Wu AW, Steckelberg RC. Medical error, incident investigation and the second victim: Doing better but feeling worse? BMJ Qual Saf. 2012;21(4):267–70.
Article PubMed Google Scholar
Busch IM, Moretti F, Purgato M, Barbui C, Wu AW, Rimondini M. Dealing with Adverse Events: A Meta-analysis on Second Victims’ Coping Strategies. J Patient Saf. 2020;16(2):E51–60.
Scott SD, Hirschinger LE, Cox KR, McCoig M, Hahn-Cover K, Epperly KM, et al. Caring for our own: Deploying a systemwide second victim rapid response team. Jt Comm J Qual Patient Saf. 2010 May;36(5)(1):233–40.
PubMed Google Scholar
Clarkson MD, Haskell H, Hemmelgarn C, Skolnik PJ. Abandon the term “second victim.” BMJ. 2019;27(364):l1233.
Tumelty ME. The second victim: A contested term? J Patient Saf. 2021;17(8):E1488–93.
Wu AW, Shapiro J, Harrison R, Scott SD, Connors C, Kenney L, et al. The Impact of Adverse Events on Clinicians: What’s in a Name? J Patient Saf. 2020;16(1):65–72.
Article PubMed CAS Google Scholar
Seys D, Wu AW, Gerven E, Van, Vleugels A, Euwema M, Panella M, et al. Health Care Professionals as Second Victims after Adverse Events: A Systematic Review. Eval Heal Prof. 2013;36(2):135–62.
Helo S, Moulton CE. Complications: acknowledging, managing, and coping with human error. Transl Androl Urol. 2017;6(6):773–82.
Article PubMed PubMed Central Google Scholar
Jones JH, Treiber LA. More Than 1 Million Potential Second Victims: How Many Could Nursing Education Prevent? Nurse Educ. 2018;43(3):154–7.
Headley M. Are second victims getting the help they need [Internet]. Vol. 15, Patient Safety & Quality Healthcare. 2018 [Cited 2022 May 17]. p. 12–6. Available from: https://www.psqh.com/analysis/are-second-victims-getting-the-help-they-need/ .
Stehman CR, Testo Z. Burnout DO. Suicide : Physician Loss in Emergency Medicine, Part I. West J Emerg Med. 2019;20:485–94.
Cottell M, Wätterbjörk I, Hälleberg Nyman M. Medication-related incidents at 19 hospitals: A retrospective register study using incident reports. Nurs Open. 2020;7(5):1526–35.
Hartnell N, MacKinnon N, Sketris I, Fleming M. Identifying, understanding and overcoming barriers to medication error reporting in hospitals: A focus group study. BMJ Qual Saf. 2012 May;21(5):361–8.
Mahdaviazad H, Askarian M, Kardeh B. Medical Error Reporting: Status Quo and Perceived Barriers in an Orthopedic Center in Iran. Int J Prev Med. 2020;11:14. https://doi.org/10.4103/ijpvm.IJPVM_235_18 .
NHS England. NHS Just Culture Guide [Internet]. 2018 [Cited 2022 May 17]. Available from: https://www.england.nhs.uk/patient-safety/a-just-culture-guide/ .
Edrees HH, Wu AW. Does One Size Fit All? Assessing the Need for Organizational Second Victim Support Programs. J Patient Saf. 2021;17(3):e247–54.
Kyngäs H. Inductive content analysis. In: The application of content analysis in nursing science research. New York (NY): Springer, Cham; 2020. p. 13–21. https://doi.org/10.1007/978-3-030-30199-6_2 .
Saunders B, Sim J, Tom K, Baker S, Waterfield J, Bartlam B, et al. Saturation in qualitative research: exploring its conceptualization and operationalization. Qual Quant. 2018;52(4):1893–907.
Ullström S, Sachs MA, Hansson J, Øvretveit J, Brommels M. Suffering in silence: A qualitative study of second victims of adverse events. BMJ Qual Saf. 2014;23(4):325–31.
Treiber LA, Jones JH. Devastatingly human: An analysis of registered nurses’ medication error accounts. Qual Health Res. 2010;20(10):1327–42.
Harrison R, Lawton R, Perlo J, Gardner P, Armitage G, Shapiro J. Emotion and Coping in the Aftermath of Medical Error: A Cross-Country Exploration. J Patient Saf. 2015;11(1):28–35.
Ball JE, Griffiths P, Rafferty AM, Lindqvist R, Murrells T, Tishelman C. A cross-sectional study of ‘care left undone’ on nursing shifts in hospitals. J Adv Nurs. 2016;72(9):2086–97.
Griffiths P, Recio-Saucedo A, Dall’Ora C, Briggs J, Maruotti A, Meredith P, et al. The association between nurse staffing and omissions in nursing care: A systematic review. J Adv Nurs. 2018;74:1474–87.
Sessions LC, Nemeth LS, Catchpole K, Kelechi TJ. Nurses’ perceptions of high-alert medication administration safety: A qualitative descriptive study. J Adv Nurs. 2019;75(12):3654–67.
Lee W, Pyo J, Jang SG, Choi JE, Ock M. Experiences and responses of second victims of patient safety incidents in Korea: A qualitative study. BMC Health Serv Res. 2019;19(1):1–12.
Mahajan RP. Critical incident reporting and learning. Br J Anaesth [Internet]. 2010;105(1):69–75. Available from: https://doi.org/10.1093/bja/aeq133 .
Härkänen M, Paananen J, Murrells T, Rafferty AM, Franklin BD. Identifying risks areas related to medication administrations - Text mining analysis using free-text descriptions of incident reports. BMC Health Serv Res. 2019;19(1):1–9.
NHS Improvement. NRLS official statistics publications: data quality statement [Internet]. 2018. Available from: https://improvement.nhs.uk/documents/2549/NRLS_Guidance_notes_March_2018.pdf .
NHS England. Patient Safety Alert: improving medication error incident reporting and learning (supporting information) [Internet]. Patient Safety Alert: Stage 3 (directive). 2014. Available from: https://www.england.nhs.uk/2014/03/improving-medication-error-incident-reporting-and-learning/ .
Download references
Acknowledgements
The authors want to thank the NHS England and the NHS Improvement Patient safety team for helping the authors through the data acquisition process and refining the data extraction.
This study has been partially supported from the grant received from Sairaanhoitajien Koulultussäätiö and from early-stage researcher position from the University of Eastern Finland for the first author.
Author information
Authors and affiliations.
Department of Nursing Science, University of Eastern Finland, Yliopistonranta 1c, Kuopio, Finland
Sanu Mahat & Marja Härkänen
King’s College London: Florence Nightingale Faculty of Nursing, Midwifery and Palliative Care, James Clerk Maxwell Building, 57 Waterloo Road, SE1 8WA, London, UK
Anne Marie Rafferty
Department of Nursing Science, University of Eastern Finland, Kuopio, Yliopistonranta 1, 70210, Finland
Katri Vehviläinen-Julkunen
Kuopio University Hospital, Puijonlaaksontie 2, 70210, Kuopio, Finland
You can also search for this author in PubMed Google Scholar
Contributions
SM conducted the analysis, but all authors (SM, AMR, KV-J, and MH) participated in interpretation of data and in drafting and revising the manuscript critically and gave final approval of the version to be submitted.
Corresponding author
Correspondence to Sanu Mahat .
Ethics declarations
Ethics approval and consent to participate.
Data sharing agreement (Ref: 063.DSA.17) between NHS Improvement and King’s College London dated 22.08.2019 allowed us to use this data. As the data used for this study were voluntarily and anonymously submitted incident reports data (a register study), the need for seeking informed consent from the incident reporters was waived from the ethics committee. The King’s College London ethics committee (LRS-17/18-5150) gave ethical approval for this study in October 2017. Incident data used for this study did not comprise any personal or professional identifiers. Therefore, the anonymity and confidentiality of the data and the persons involved were fully ensured. Further, data handling was made confidential and ethical guidelines were followed.
Consent for publication
Not applicable.
Competing interests
No competing interests have been declared by authors.
Additional information
Publisher’s note.
Springer Nature remains neutral with regard to jurisdictional claims in published maps and institutional affiliations.
Supplementary Information
Additional file 1: supplementary file 1..
Number of incident reports with negative emotional expressions and description about the healthcare staffs’ feeling. Supplementary file 2. Number of negative emotional expressions related specifically to medication administration incident reports ( n =72,390). Supplementary file 3. SRQR checklist for reporting qualitative studies.
Rights and permissions
Open Access This article is licensed under a Creative Commons Attribution 4.0 International License, which permits use, sharing, adaptation, distribution and reproduction in any medium or format, as long as you give appropriate credit to the original author(s) and the source, provide a link to the Creative Commons licence, and indicate if changes were made. The images or other third party material in this article are included in the article's Creative Commons licence, unless indicated otherwise in a credit line to the material. If material is not included in the article's Creative Commons licence and your intended use is not permitted by statutory regulation or exceeds the permitted use, you will need to obtain permission directly from the copyright holder. To view a copy of this licence, visit http://creativecommons.org/licenses/by/4.0/ . The Creative Commons Public Domain Dedication waiver ( http://creativecommons.org/publicdomain/zero/1.0/ ) applies to the data made available in this article, unless otherwise stated in a credit line to the data.
Reprints and permissions
About this article
Cite this article.
Mahat, S., Rafferty, A.M., Vehviläinen-Julkunen, K. et al. Negative emotions experienced by healthcare staff following medication administration errors: a descriptive study using text-mining and content analysis of incident data. BMC Health Serv Res 22 , 1474 (2022). https://doi.org/10.1186/s12913-022-08818-1
Download citation
Received : 29 June 2022
Accepted : 09 November 2022
Published : 03 December 2022
DOI : https://doi.org/10.1186/s12913-022-08818-1
Share this article
Anyone you share the following link with will be able to read this content:
Sorry, a shareable link is not currently available for this article.
Provided by the Springer Nature SharedIt content-sharing initiative
- Incident report
- Medication error
- Negative emotions
- Second victim
- Healthcare staff
- Text-mining
- Content analysis
BMC Health Services Research
ISSN: 1472-6963
- General enquiries: [email protected]
- Open access
- Published: 02 June 2024
Neuroleptic malignant syndrome and serotonin syndrome: a comparative bibliometric analysis
- Waleed M. Sweileh 1
Orphanet Journal of Rare Diseases volume 19 , Article number: 221 ( 2024 ) Cite this article
277 Accesses
1 Altmetric
Metrics details
This study aimed to analyze and map scientific literature on Neuroleptic Malignant Syndrome (NMS) and Serotonin Syndrome (SS) from prestigious, internationally indexed journals. The objective was to identify key topics, impactful articles, prominent journals, research output, growth patterns, hotspots, and leading countries in the field, providing valuable insights for scholars, medical students, and international funding agencies.
A systematic search strategy was implemented in the PubMed MeSH database using specific keywords for NMS and SS. The search was conducted in the Scopus database, renowned for its extensive coverage of scholarly publications. Inclusion criteria comprised articles published from 1950 to December 31st, 2022, restricted to journal research and review articles written in English. Data were analyzed using Microsoft Excel for descriptive analysis, and VOSviewer was employed for bibliometric mapping.
The search yielded 1150 articles on NMS and 587 on SS, with the majority being case reports. Growth patterns revealed a surge in NMS research between 1981 and 1991, while SS research increased notably between 1993 and 1997. Active countries and journals differed between NMS and SS, with psychiatry journals predominating for NMS and pharmacology/toxicology journals for SS. Authorship analysis indicated higher multi-authored articles for NMS. Top impactful articles focused on review articles and pathogenic mechanisms. Research hotspots included antipsychotics and catatonia for NMS, while SS highlighted drug interactions and specific medications like linezolid and tramadol.
Conclusions
NMS and SS represent rare but life-threatening conditions, requiring detailed clinical and scientific understanding. Differential diagnosis and management necessitate caution in prescribing medications affecting central serotonin or dopamine systems, with awareness of potential drug interactions. International diagnostic tools and genetic screening tests may aid in safe diagnosis and prevention. Reporting rare cases and utilizing bibliometric analysis enhance knowledge dissemination and research exploration in the field of rare drug-induced medical conditions.
Introduction
Neuroleptic malignant syndrome (NMS) and serotonin syndrome (SS) are drug-induced, potentially life-threatening conditions that are infrequently encountered in medical practice, necessitating prompt intervention [ 1 , 2 , 3 , 4 ]. Neuroleptic Malignant Syndrome is characterized by a decrease in dopamine activity in the brain, often associated with the use of dopamine antagonists, primarily neuroleptic or antipsychotic medications [ 5 , 6 ]. While the exact pathophysiology of NMS remains incompletely understood, it is believed to involve dopamine dysregulation in the basal ganglia and hypothalamus. This dysregulation, particularly the blockade of dopamine receptors, especially D2 receptors, leads to a state of dopamine deficiency, manifesting in symptoms such as muscle rigidity, hyperthermia, and autonomic instability. Furthermore, withdrawal from dopamine agonists, such as L-Dopa, can also precipitate NMS in susceptible individuals. Serotonin Syndrome is characterized by an excess of serotonin (5-HT) in the central nervous system, typically stemming from the use of serotonergic medications or substances that elevate serotonin levels [ 7 , 8 ]. These drugs encompass antidepressants, notably selective serotonin reuptake inhibitors (SSRIs), opioids, specific psychedelics, serotonin agonists, and herbal supplements. The pathophysiology of SS revolves around the excessive stimulation of serotonin receptors, particularly the 5-HT2A receptors. This heightened stimulation precipitates a spectrum of symptoms, ranging from agitation, confusion, hyperthermia, muscle rigidity, to autonomic dysfunction. The severity of SS can vary widely, from mild manifestations to life-threatening conditions, contingent upon the extent of serotonin excess and individual susceptibility factors.
Both NMS and SS exhibit shared clinical manifestations, including hyperthermia, hypertension, hypersalivation, diaphoresis, and altered mental status [ 4 ], with instances of coexistence reported in some patients [ 9 ]. However, they diverge in their etiologies and clinical presentations. For instance, individuals with NMS typically display hyporeflexia, normal pupil size, and normal bowel sounds, contrasting with SS patients who often present with hyperreflexia, dilated pupils, and hyperactive bowel activity [ 10 ]. NMS is typified by lead-pipe muscle rigidity, whereas SS manifests with increased muscle tone, particularly in the lower extremities [ 11 , 12 ]. Given these distinctions, treatment strategies for NMS and SS diverge based on their underlying causes [ 2 ]. The mechanisms driving these syndromes differ significantly; while NMS involves diminished dopamine activity in the brain, SS is characterized by elevated serotonin levels [ 13 ]. Dopamine antagonists, such as neuroleptics or antipsychotics, are commonly implicated in NMS [ 14 , 15 , 16 ], although other triggers like withdrawal from dopamine agonists, like L-Dopa, can also induce NMS [ 17 , 18 ]. Conversely, SS can result from various drug classes, including antidepressants, opioids, psychedelics, serotonin agonists, and certain herbs [ 7 , 19 , 20 , 21 , 22 , 23 ]. Consequently, distinct medications are employed for their management; benzodiazepines and serotonin antagonists are standard therapy for SS, whereas dopaminergic agents and dantrolene are preferred for NMS [ 10 ]. While the incidence of NMS remains low, particularly among patients receiving newer generation antipsychotics [ 24 , 25 ], recent studies on SS incidence are lacking. However, a 1999 study reported an incidence of 0.4 cases per 1000 patient-months with nefazodone [ 26 ], while SS incidence reaches 14–16% in cases of selective serotonin reuptake inhibitor (SSRI) overdose [ 27 ].
Research context and objectives
The landscape of psychiatric pharmacotherapy has evolved over time, witnessing a surge in the number of approved drugs and the introduction of novel classes into clinical practice [ 28 , 29 , 30 , 31 ]. This trend is particularly notable in the treatment of depression and schizophrenia, where the absence of universally safe and effective drugs persists [ 32 , 33 , 34 , 35 , 36 ]. Additionally, off-label utilization of antidepressants and antipsychotics has been observed among patients with dementia and other neuro-cognitive disorders [ 37 , 38 , 39 , 40 , 41 ], contributing to an upward trajectory in psychiatric drug consumption [ 42 , 43 ]. The risk of SS is linked to any medication or herb augmenting the central serotonergic pathway, necessitating vigilant monitoring by healthcare professionals due to the potential for adverse effects, whether as a primary mechanism or side effect [ 20 ]. A concerning trend of unsupported polypharmacy in psychiatric medications has also emerged [ 44 ], along with significant prescribing of antidepressants and antipsychotics to dementia patients without documented indications of depression or psychosis [ 45 , 46 ], mirroring similar trends among individuals with intellectual disabilities [ 47 ]. The escalating demand for psychiatric therapy raises apprehensions regarding the likelihood of adverse medication effects [ 48 ], exacerbated by increased prescribing rates, polypharmacy, and off-label usage, which heighten the incidence of drug-induced toxicities, including NMS and SS. Analyzing published literature on drug-induced NMS and SS provides valuable insights into these rare yet severe toxicities, aligning with the pressing global public health burden of depression, schizophrenia, and related conditions, accentuated by the fatal toxicities associated with specific psychiatric medications. This scientific literature on NMS and SS is ripe for analysis and mapping to delineate current research hotspots [ 49 , 50 , 51 , 52 , 53 , 54 , 55 ], addressing the gap in the literature. Accordingly, the present study aims to analyze and map scientific research on NMS and SS published in prestigious, internationally indexed journals. Through this analysis, the study seeks to identify key topics, impactful articles, prominent journals, research output, growth patterns, hotspots, and leading countries in the field, providing valuable insights for scholars, medical students, and international funding agencies to discern research trajectories, bibliographic trends, and knowledge structures pertaining to NMS and SS. Ultimately, this endeavor aims to invigorate scholarly discourse and inform clinical practice in the field.
Database and keywords
In this study, we employed a systematic search strategy to extract relevant scientific literature on NMS and SS from the PubMed MeSH database. Specifically, we utilized the following keywords:
Malignant neuroleptic syndrome: “malignant neuroleptic syndrome”.
Serotonin syndrome: “serotonin syndrome” or “serotonin toxicity”.
To ensure comprehensive coverage, we conducted our search in Scopus, a prestigious scientific database owned by Elsevier, which has previously been utilized for analyzing research in psychiatry [ 56 , 57 ]. Scopus is renowned for its extensive coverage, encompassing a vast array of scholarly publications in the field. Notably, Scopus encompasses over 95% of the content included in other databases such as PubMed and Web of Science, rendering it an ideal platform for our study [ 58 ].
Inclusion and exclusion criteria
We restricted our search to articles published from 1950 to December 31st, 2022, and focused exclusively on journal research and review articles written in English. Excluded from our analysis were editorials, notes, letters, and conference abstracts. Additionally, articles pertaining to non-human subjects were excluded, ensuring the relevance of our findings. We meticulously reviewed the titles and abstracts of over 100 articles to eliminate irrelevant publications, such as those mentioning NMS or SS only marginally, thereby refining the scope of our analysis.
Our search strategy yielded results indicative of its validity, as evidenced by the prominent presence of leading scientists and journals in the fields of psychiatry and pharmacology. This reaffirmed the robustness of our search criteria and the relevance of the retrieved literature to our study objectives.
Data management, analysis, and mapping
The dataset comprising the retrieved articles was subjected to descriptive analysis using Microsoft Excel. Subsequently, we employed VOSviewer, a freely available online tool, for bibliometric mapping purposes [ 59 ]. VOSviewer maps offer researchers a visual tool for exploring bibliometric data, revealing patterns, relationships, and trends within a dataset. Interpretation of these maps involves understanding several key elements. Firstly, node size indicates the prominence or frequency of an item, with larger nodes representing more significant themes or influential publications. Secondly, node color categorizes items into clusters, with similar colors indicating thematic groupings. Thirdly, the thickness of connecting lines between nodes signifies the strength of associations, with thicker lines indicating stronger connections. Lastly, the distance between nodes reflects the similarity or dissimilarity between items, with closer nodes indicating stronger relationships. Overall, VOSviewer maps provide a comprehensive visual overview of bibliometric data, enabling researchers to identify clusters, influential publications, and emerging trends within their field of study by considering the interplay between node size, color, line thickness, and spatial relationships. Within the descriptive analysis, we presented lists of active countries and journals, alongside a linear graph illustrating the growth of publications over time. In the keyword visualization map generated using VOSviewer, node size corresponded to the frequency of occurrence of each keyword, enabling visual identification of prominent themes. Similarly, in the journal visualization map, node size reflected the normalized citation count received by each journal, providing insights into publication impact within the field.
Number of publications
The search strategy yielded a total of 1150 articles on NMS and 587 on SS. Among the articles on NMS, 791 (68.8%) were case reports, while 384 (65.4%) of the articles on SS took the form of case reports.
Growth of publications
The earliest scientific publication on NMS dates back to 1973 [ 60 ], while publications on SS emerged in 1979 [ 61 ]. Research on NMS experienced a notable surge between 1981 and 1991, followed by a fluctuating decline. Conversely, research on SS saw a steep increase between 1993 and 1997, followed by a fluctuating rise. Figure 1 illustrates the growth trends of research on NMS and SS.
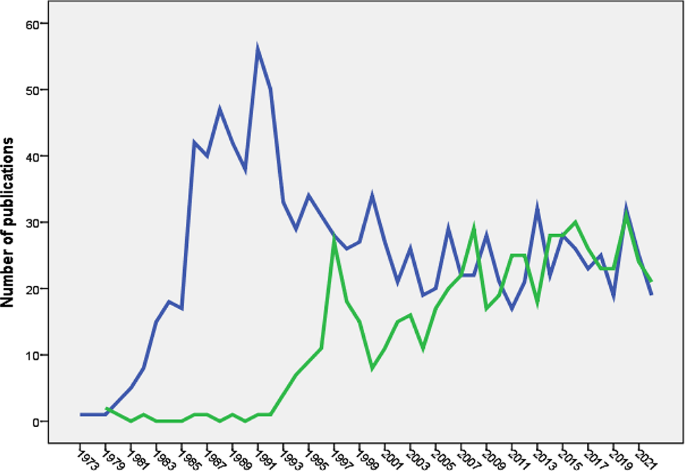
Annual growth of publications of NMS (blue line) and SS (green line). The Figure was created by SPSS program
Active countries and journals
Table 1 outlines the top five countries contributing articles on NMS and SS. Japan ranked second in NMS publications but fifth in SS publications. Table 2 presents the top five active journals for both NMS and SS, with NMS publications primarily within psychiatry journals and SS publications within pharmacology/toxicology journals.
Authorship analysis
Articles on NMS involved 3820 authors (mean = 3.1 authors per article), with 89 (7.3%) single-authored and 171 (14.1%) multi-authored articles. Similarly, articles on SS included 2105 authors (mean = 3.0 authors per article), with 102 (16.0%) single-authored and 41 (7.1%) multi-authored articles.
Most impactful articles
The top five impactful articles on NMS comprised mainly review articles and a research article focusing on the pathogenic role of dopamine antagonists [ 62 ]. For SS, the top five impactful articles included review articles and research articles discussing the Hunter diagnostic criteria [ 63 ] and the role of monoamine oxidase inhibitors (MAO-I) and opioid analgesics in serotonin toxicity [ 64 ].
Research hotspots
Research hotspots were identified by mapping author keywords with a minimum occurrence of five times (Figs. 2 and 3 ). Notable hotspots for SS included antidepressants, SSRIs, tramadol, linezolid, cyproheptadine, and drug interactions. For NMS, hotspots included antipsychotics (various drug names), catatonia, and rhabdomyolysis.
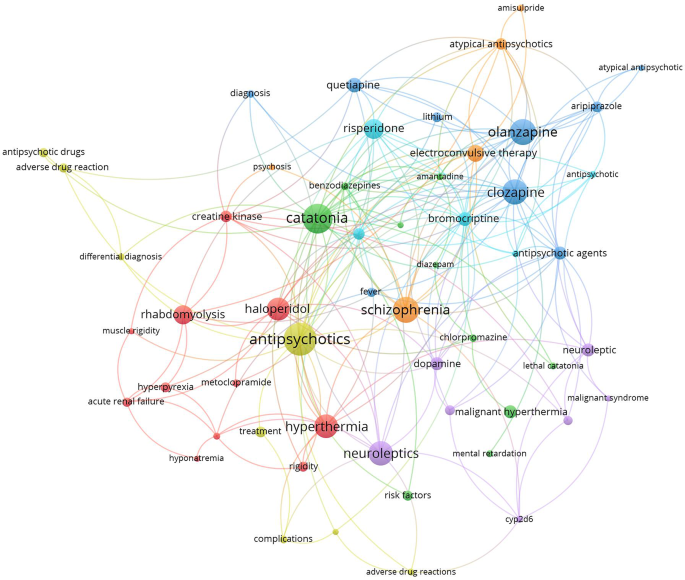
Network visualization map of author keywords with minimum occurrences of five times. Large nodes represent research hotspots on NMS. The term NMS was not shown to make other keywords more visible
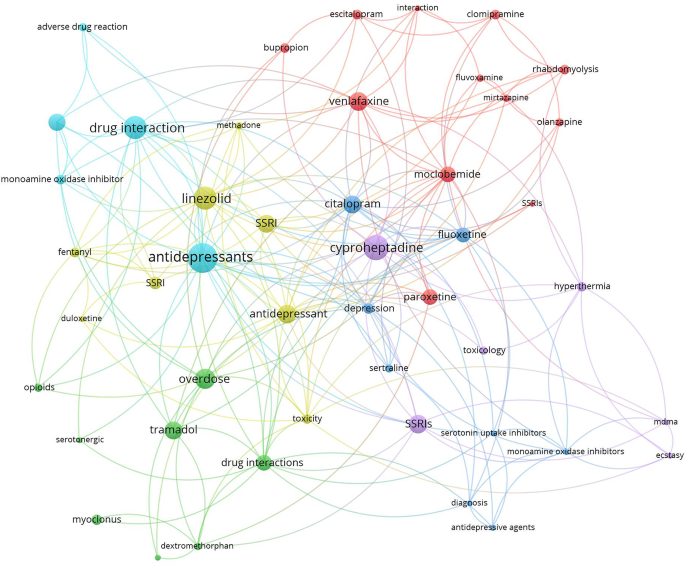
Network visualization map of author keywords with minimum occurrences of five times. Large nodes represent research hotspots on SS. The term SS was not shown to make other keywords more visible
Journal citation analysis
The top 15 active journals in publishing articles on NMS and SS were mapped (Figs. 4 and 5 ). Notably, articles on NMS published in the American Journal of Psychiatry and the Journal of Clinical Psychiatry received the highest number of citations per article. Similarly, articles on SS published in Clinical Toxicology and the Annals of Pharmacotherapy garnered the most citations per article.
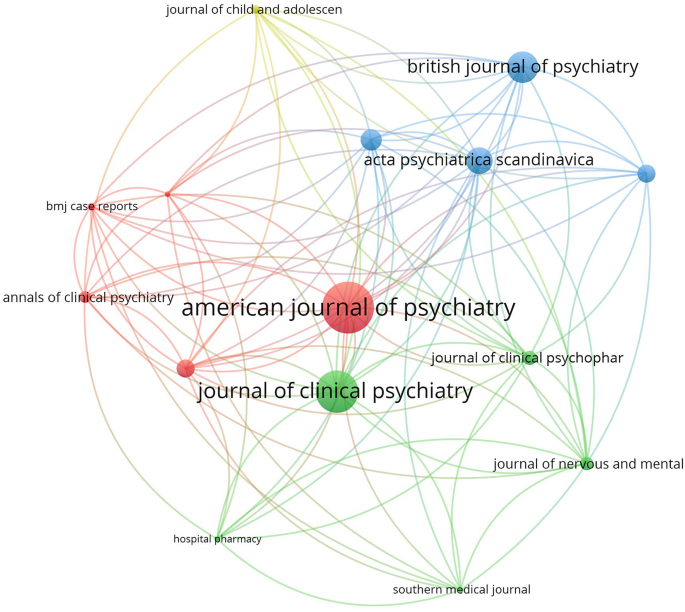
Network visualization map of the top 15 journals in the field of NMS. Large node sized indicates higher normalized citation count
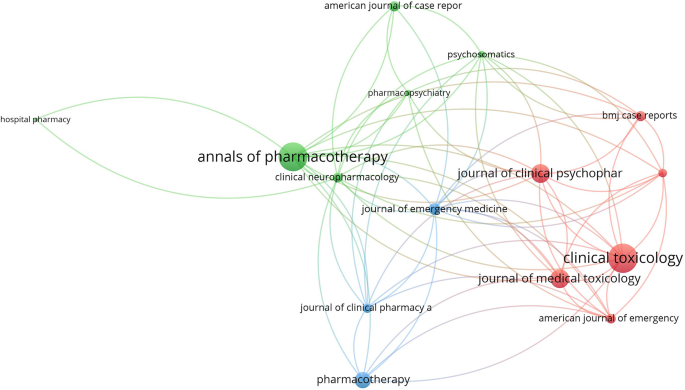
Network visualization map of the top 15 journals in the field of SS. Large node sized indicates higher normalized citation count
Geographic mapping
The geographic distribution of research publications on NMS and SS was illustrated on a worldwide map (Fig. 6 ), with the majority of contributions originating from the US. Several countries in specific regions showed minimal to no research output on either NMS or SS.
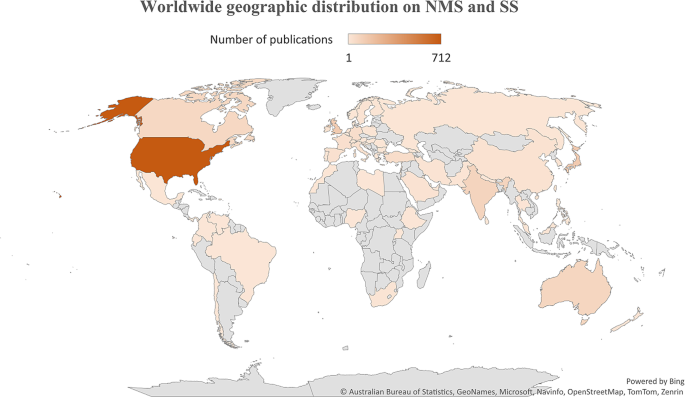
Worldwide distribution of research publications on NMS and SS. Figure was created by Microsoft Excel
Molecular genetics
The retrieved literature on NMS has 20 articles that discussed the potential link between NMS and certain genetics. Ten articles discussed the potential linkage between Cytochrome 2D6 and potential risk for NMS [ 65 , 66 , 67 , 68 , 69 , 70 , 71 , 72 , 73 , 74 ]. Five articles discussed the potential linkage between dopamine receptor 2 gene polymorphism and NMS [ 75 , 76 , 77 , 78 , 79 ]. Four articles discussed the linkage between ryanodine receptor gene mutations and susceptibility to NMS [ 80 , 81 , 82 , 83 ]. No association was found between NMS and serotonin receptor gene variation [ 84 ]. The literature on SS has few articles that discussed the genetic predisposition of patients to SS such as the 5-HT receptor gene or the CYP 2D6 gene polymorphism [ 85 , 86 ].
Drug interactions
Serious drug-drug interactions leading to NMS were mentioned in a limited number of articles and involved the administration of two dopamine antagonists [ 87 ] or two atypical antipsychotic drugs [ 88 ]. However, there were many articles discussing potential SS caused by drug-drug interactions, which included SSRI–methylene blue [ 89 ], SSRI–metoclopramide [ 89 ], sertraline–phenelzine [ 90 ], anti-depressants–opioids [ 91 ], citalopram-fentanyl [ 92 ], a combination of two anti-depressants [ 93 ], SSRI-linezolid [ 94 , 95 , 96 , 97 , 98 , 99 , 100 , 101 , 102 ], sertraline–phenelzine [ 90 ], citalopram-buspirone [ 103 ], venlafaxine-tranylcypromine [ 104 ], and many others [ 92 , 105 , 106 , 107 , 108 , 109 ].
Non-psychiatric causative agents
The retrieved literature on SS, showed that several drugs and drug classes not related to antidepressants can induce SS. Examples of such drugs included Linezolid, CNS stimulants (amphetamine), hallucinogens (LSD), opioids (fentanyl), ondansetron, sumatriptan, and certain herbs (St. John’s wort), metoclopramide, ritonavir, and others [ 5 , 20 , 110 , 111 ]. The retrieved literature on NMS showed that drug-induced NMS is limited to antipsychotics and withdrawal of dopamine agonists [ 112 , 113 , 114 ].
Diagnostic criteria
For NMS, there were 30 articles that discussed issues related to diagnosis. In 2011, an international panel tried to develop NMS diagnostic criteria [ 115 , 116 ]. The neutrophil-lymphocyte ratio was suggested by certain researchers as a diagnostic test for NMS [ 117 , 118 ]. The differential diagnosis for NMS compared to SS and catatonia was also published [ 13 , 118 , 119 ]. For SS, there were 17 articles that discussed issues related to diagnosis of SS. The Hunter diagnostic criteria was one of these articles [ 63 ]. Other articles discussed controversies and the importance of differential diagnosis in SS [ 120 ].
The current study analyzed and compared the scientific literature on two rare drug-induced conditions with certain overlapping clinical features. Both syndromes are mainly caused by medications used in psychiatry, such as those for schizophrenia and depression. The name “NMS” implies that the syndrome is correlated with the use of neuroleptic medications, while the name “SS” implies that it is correlated with any medication or herb that raises serotonin centrally.
The analysis showed that the volume of research publications on NMS was larger and started earlier than research publications on SS. The NMS is associated with the use of dopamine antagonists (neuroleptics). The history of using old-generation antipsychotics for the treatment of schizophrenia dates back to the 1950s [ 121 , 122 , 123 , 124 , 125 ]. On the other hand, the introduction of the SSRI drug class, the main causative agent of SS, dates back to the late 1980s [ 126 ]. The difference in the history of introduction into clinical practice explains the differences between SS and NMS in growth patterns. The difference in the volume of literature between the two syndromes could be due to diagnostic uncertainty [ 127 ] for NMS versus SS, the seriousness of medical complications, or debate regarding whether an atypical antipsychotic drug class causes NMS in a similar way to conventional antipsychotics [ 13 , 30 , 128 , 129 , 130 ]. The current study showed that the number of research publications on NMS started to decline after 1991 but the number of publications on SS started to increase after 1997. The introduction of atypical antipsychotics with lesser dopaminergic side effects than conventional antipsychotics decreased the incidence of NMS and therefore decreased the number of publications with time. On the other hand, the increased number of SS publications after 1997 could be explained by the many reported drug interactions at serotonin level leading to more cases of SS with time.
The current study showed that journals in the field of psychiatry ranked highest in publishing articles on NMS, while those in the field of pharmacology/toxicology ranked highest in publishing articles on SS. The reason for this difference is difficult to explain. However, NMS is primarily limited to schizophrenia patients taking antipsychotic drugs, while SS might occur in normal people taking SSRIs for depression or any other condition. Furthermore, the potentially large numbers of drug- or drug-herb interactions make the SS interesting to pharmacology/toxicology journals [ 22 ]. Actually, SS has been termed “serotonin toxicity” implying relatedness to toxicology [ 131 ].
The findings of the current study regarding active countries were not surprising. The English-speaking countries, the US, the UK, Australia, and Canada showed leading roles in many scientific disciplines and ranked first in several studies that analyzed research activity [ 132 , 133 , 134 , 135 ]. This is due to advancements in technology, medicine, clinical practice, and research funding in high-income countries relative to other countries. However, there are also reasons related to the nature of journals indexed in Scopus. The vast majority of Scopus-indexed journals publish articles in English, and the vast majority of the journals are issued by publishers and institutions based in the US, Europe, or Australia. Therefore, Scopus might be biased toward scholars in English-speaking countries. The finding that research articles on NMS tend to be multi-authored while those on SS are not is not easy to explain. However, it is possible that cases of NMS tend to involve a larger medical team due to the nature of complications that might involve renal and blood complications. Furthermore, the treatment of NMS requires medications and follow-up. All this makes the number of authors in a case study of NMS higher than those involved in SS cases [ 13 , 136 , 137 ].
Of the retrieved articles on SS and NMS, the research article “The hunter serotonin toxicity criteria: Simple and accurate diagnostic decision rules for serotonin toxicity” [ 63 ] received the highest number of citations excluding the review articles. The diagnosis of SS is based on the clinical symptoms and the medical history of the patient. Harvey Sternbach introduced the first diagnostic criteria for SS in 1991 and the Hunter Diagnostic Criteria tool was introduced in 2003 [ 63 , 138 ].
Mapping the retrieved literature on NMS showed that rhabdomyolysis and catatonia constituted distinct research hotspots in addition to those related to antipsychotic medications and schizophrenia. However, mapping the author keywords of SS research publications showed that linezolid, drug interactions, and tramadol constituted research hotspots in addition to antidepressants and SSRIs. Rhabdomyolysis has been reported as a consequence of NMS even among children and adolescents [ 139 , 140 ]. However, reports of rhabdomyolysis among patients taking antipsychotics were published, suggesting that rhabdomyolysis could be a side effect of antipsychotics even in the absence of NMS [ 139 , 140 ]. Catatonia is, as NMS, a consequence of neuroleptic drugs, and there is an overlap in clinical features between the symptoms of catatonia and those of NMS, which makes the distinction between them difficult [ 141 ]. Linezolid is an antibiotic that was originally designed to be used as an anti-depressant by virtue of its MAO enzyme inhibition property [ 142 ]. This explains the many cases of SS induced by drug interactions with Linezolid [ 141 ]. The relatively higher number of research articles on drug/herb interactions leading to SS is attributed to the presence of many and different drug classes that affect and increase serotonergic pathways in the brain [ 90 , 105 , 109 , 143 ]. The scientific controversy about the potential ability of tramadol to cause SS received a high number of citations. Current scientific evidence supports the ability of tramadol to cause SS due to its molecular pharmacological effects on both the opioid and serotonergic systems [ 105 , 107 , 144 , 145 , 146 , 147 ]. Cyproheptadine was also a research hotspot in the field of SS. Cyproheptadine has anti-histaminic, anticholinergic, and anti-serotonergic properties and that is why it has been used to counter the symptoms of SS [ 148 , 149 , 150 ].
The current study showed that SS has a wide range of possible drug/herb interactions due to the many drugs that affect the serotonin system. Of particular interest is the one with opioid analgesics, since they are commonly used in hospital settings. Opioids, including fentanyl and even dextromethorphan in cough syrups, were reported to increase serotonin levels, and therefore caution should be practiced when given to patients with SSRIs in their medical records [ 19 , 22 , 109 , 151 ].
Limitations
Limitations arise in this study from various factors. Firstly, the reliance on the Scopus database for literature retrieval could potentially limit the inclusivity of articles from low- and middle-income countries. Although Scopus offers extensive coverage, the possibility exists that some relevant journals from these regions may not be indexed, thereby leading to a potential underestimation of publications from certain geographic areas. Secondly, despite efforts to employ a comprehensive search strategy, the use of a title-abstract search method might have resulted in the retrieval of some false-positive results. While validation tests were conducted to mitigate this issue, the possibility of false positives cannot be entirely ruled out. Thirdly, the analysis focused solely on articles published in English-language journals, which could introduce a language bias and limit the generalizability of findings. This exclusion of literature published in other languages may have led to the omission of relevant data from non-English sources. Lastly, diagnostic uncertainty poses a challenge in distinguishing between neuroleptic malignant syndrome (NMS) and serotonin syndrome (SS) due to overlapping clinical features and the absence of definitive diagnostic tests. Misdiagnosis or underreporting of cases may have occurred, potentially impacting the accuracy of the literature analysis and conclusions drawn from it.
In conclusion, NMS and SS represent rare but potentially life-threatening conditions associated with drug-induced dysregulation of dopamine and serotonin systems, respectively. The study analyzed and compared the scientific literature on these syndromes, revealing distinct growth patterns, research hotspots, and publication trends. The findings underscored the evolving landscape of psychiatric pharmacotherapy and the complexities involved in diagnosing and managing NMS and SS. While NMS research exhibited a longer history and a decline in publications over time, SS research witnessed a notable increase in publications, reflecting advancements in pharmacological understanding and the recognition of SS as a significant clinical entity. Identified research hotspots provided valuable insights into emerging areas of interest, including drug interactions, molecular genetics, and diagnostic criteria. Understanding these trends is essential for informing clinical practice, guiding future research endeavors, and promoting collaboration among scholars and healthcare professionals. Despite the study’s contributions, several limitations warrant consideration, including database restrictions, potential publication bias, and diagnostic uncertainties. Addressing these limitations through expanded literature search strategies, international collaboration, and improved diagnostic tools is crucial for advancing knowledge and enhancing patient care in the field of rare drug-induced syndromes. Moving forward, efforts to develop standardized diagnostic criteria, genetic screening tools, and international reporting mechanisms for NMS and SS are warranted. Additionally, continued bibliometric analysis and mapping of literature on rare medical conditions can facilitate ongoing research and contribute to the dissemination of knowledge across global healthcare communities.
Data availability
All data present in this article can be retrieved from Scopus using keywords listed in the methodology.
Abbreviations
- Neuroleptic malignant syndrome
Serotonin Syndrome/ Serotonin toxicity
Darracq MA, Comment. Neuroleptic malignant syndrome versus serotonin syndrome: the search for a diagnostic tool. Ann Pharmacother. 2012;46(4):611–2. https://doi.org/10.1345/aph.1P787a . author reply 3.
Article PubMed Google Scholar
Sokoro AA, Zivot J, Ariano RE. Neuroleptic malignant syndrome versus serotonin syndrome: the search for a diagnostic tool. Ann Pharmacother. 2011;45(9):e50. https://doi.org/10.1345/aph.1P787 .
Fernández M, Lago L, Alonso MG, Guede A, Benavente JL, Olivares JM. Serotonin syndrome versus neuroleptic malignant syndrome: a case report. Actas Esp Psiquiatr. 2018;46(2):68–74.
PubMed Google Scholar
Dosi R, Ambaliya A, Joshi H, Patell R. Serotonin syndrome versus neuroleptic malignant syndrome: a challenging clinical quandary. BMJ Case Rep. 2014. https://doi.org/10.1136/bcr-2014-204154 . 2014.
Article PubMed PubMed Central Google Scholar
Tormoehlen LM, Rusyniak DE. Neuroleptic malignant syndrome and serotonin syndrome. Handb Clin Neurol. 2018;157:663–75. https://doi.org/10.1016/b978-0-444-64074-1.00039-2 .
Ware MR, Feller DB, Hall KL. Neuroleptic malignant syndrome: diagnosis and management. Prim Care Companion CNS Disord. 2018;20(1). https://doi.org/10.4088/PCC.17r02185 .
Maitland S, Baker M. Serotonin syndrome. Drug Ther Bull. 2022;60(6):88–91. https://doi.org/10.1136/dtb.2021.000032 .
Mikkelsen N, Damkier P, Pedersen SA. Serotonin syndrome-A focused review. Basic Clin Pharmacol Toxicol. 2023;133(2):124–9. https://doi.org/10.1111/bcpt.13912 .
Article CAS PubMed Google Scholar
Prakash S, Lodha D, Rawat KS. Coexistence of serotonin syndrome and neuroleptic malignant syndrome: does it exist? BMJ Case Rep. 2021;14(8). https://doi.org/10.1136/bcr-2021-241578 .
Katus LE, Frucht SJ. Management of Serotonin Syndrome and neuroleptic malignant syndrome. Curr Treat Options Neurol. 2016;18(9):39. https://doi.org/10.1007/s11940-016-0423-4 .
Hadad E, Weinbroum AA, Ben-Abraham R. Drug-induced hyperthermia and muscle rigidity: a practical approach. Eur J Emerg Med. 2003;10(2):149–54. https://doi.org/10.1097/00063110-200306000-00018 .
Haddad PM, Dursun SM. Neurological complications of psychiatric drugs: clinical features and management. Hum Psychopharmacol. 2008;23(Suppl 1):15–26. https://doi.org/10.1002/hup.918 .
Perry PJ, Wilborn CA. Serotonin syndrome vs neuroleptic malignant syndrome: a contrast of causes, diagnoses, and management. Ann Clin Psychiatry. 2012;24(2):155–62.
Pileggi DJ, Cook AM. Neuroleptic malignant syndrome. Ann Pharmacother. 2016;50(11):973–81. https://doi.org/10.1177/1060028016657553 .
Hasan S, Buckley P. Novel antipsychotics and the neuroleptic malignant syndrome: a review and critique. Am J Psychiatry. 1998;155(8):1113–6. https://doi.org/10.1176/ajp.155.8.1113 .
Belvederi Murri M, Guaglianone A, Bugliani M, Calcagno P, Respino M, Serafini G, et al. Second-generation antipsychotics and neuroleptic malignant syndrome: systematic review and case report analysis. Drugs R D. 2015;15(1):45–62. https://doi.org/10.1007/s40268-014-0078-0 .
Article CAS PubMed PubMed Central Google Scholar
Mizuno Y, Takubo H, Mizuta E, Kuno S. Malignant syndrome in Parkinson’s disease: concept and review of the literature. Parkinsonism Relat Disord. 2003;9(Suppl 1). https://doi.org/10.1016/s1353-8020(02)00125-6 . :S3-9.
Rainer C, Scheinost NA, Lefeber EJ. Neuroleptic malignant syndrome. When levodopa withdrawal is the cause. Postgrad Med. 1991;89(5):175–8. https://doi.org/10.1080/00325481.1991.11700900 .
Baldo BA, Rose MA. The anaesthetist, opioid analgesic drugs, and serotonin toxicity: a mechanistic and clinical review. Br J Anaesth. 2020;124(1):44–62. https://doi.org/10.1016/j.bja.2019.08.010 .
Bartlett D. Drug-Induced Serotonin Syndrome. Crit Care Nurse. 2017;37(1):49–54. https://doi.org/10.4037/ccn2017169 .
Hu Z, Yang X, Ho PC, Chan SY, Heng PW, Chan E, et al. Herb-drug interactions: a literature review. Drugs. 2005;65(9):1239–82. https://doi.org/10.2165/00003495-200565090-00005 .
Baldo BA. Opioid analgesic drugs and serotonin toxicity (syndrome): mechanisms, animal models, and links to clinical effects. Arch Toxicol. 2018;92(8):2457–73. https://doi.org/10.1007/s00204-018-2244-6 .
Scotton WJ, Hill LJ, Williams AC, Barnes NM. Serotonin syndrome: pathophysiology, clinical features, management, and potential future directions. Int J Tryptophan Res. 2019;12:1178646919873925. https://doi.org/10.1177/1178646919873925 .
Velamoor VR. Neuroleptic malignant syndrome. Recognition, prevention and management. Drug Saf. 1998;19(1):73–82. https://doi.org/10.2165/00002018-199819010-00006 .
Berman BD. Neuroleptic malignant syndrome: a review for neurohospitalists. Neurohospitalist. 2011;1(1):41–7. https://doi.org/10.1177/1941875210386491 .
Mackay FJ, Dunn NR, Mann RD. Antidepressants and the serotonin syndrome in general practice. Br J Gen Pract. 1999;49(448):871–4.
CAS PubMed PubMed Central Google Scholar
Isbister GK, Bowe SJ, Dawson A, Whyte IM. Relative toxicity of selective serotonin reuptake inhibitors (SSRIs) in overdose. J Toxicol Clin Toxicol. 2004;42(3):277–85. https://doi.org/10.1081/clt-120037428 .
Adell A. Brain NMDA receptors in Schizophrenia and Depression. Biomolecules. 2020;10(6). https://doi.org/10.3390/biom10060947 .
Laszlovszky I, Barabássy Á, Németh G, Cariprazine. A broad-spectrum antipsychotic for the treatment of Schizophrenia: Pharmacology, Efficacy, and Safety. Adv Ther. 2021;38(7):3652–73. https://doi.org/10.1007/s12325-021-01797-5 .
Orzelska-Górka J, Mikulska J, Wiszniewska A, Biała G. New atypical antipsychotics in the treatment of Schizophrenia and Depression. Int J Mol Sci. 2022;23(18). https://doi.org/10.3390/ijms231810624 .
Marwaha S, Palmer E, Suppes T, Cons E, Young AH, Upthegrove R. Novel and emerging treatments for major depression. Lancet. 2023;401(10371):141–53. https://doi.org/10.1016/s0140-6736(22)02080-3 .
Preda A, Shapiro BB. A safety evaluation of aripiprazole in the treatment of schizophrenia. Expert Opin Drug Saf. 2020;19(12):1529–38. https://doi.org/10.1080/14740338.2020.1832990 .
Solmi M, Murru A, Pacchiarotti I, Undurraga J, Veronese N, Fornaro M, et al. Safety, tolerability, and risks associated with first- and second-generation antipsychotics: a state-of-the-art clinical review. Ther Clin Risk Manag. 2017;13:757–77. https://doi.org/10.2147/tcrm.S117321 .
Cheniaux E, Nardi AE. Evaluating the efficacy and safety of antidepressants in patients with bipolar disorder. Expert Opin Drug Saf. 2019;18(10):893–913. https://doi.org/10.1080/14740338.2019.1651291 .
Sommer BR, Fenn H, Pompei P, DeBattista C, Lembke A, Wang P, et al. Safety of antidepressants in the elderly. Expert Opin Drug Saf. 2003;2(4):367–83. https://doi.org/10.1517/14740338.2.4.367 .
Carvalho AF, Sharma MS, Brunoni AR, Vieta E, Fava GA. The Safety, Tolerability and Risks Associated with the use of newer generation antidepressant drugs: a critical review of the literature. Psychother Psychosom. 2016;85(5):270–88. https://doi.org/10.1159/000447034 .
Yunusa I, Rashid N, Demos GN, Mahadik BS, Abler VC, Rajagopalan K. Comparative outcomes of commonly used off-label atypical antipsychotics in the treatment of dementia-related psychosis: a Network Meta-analysis. Adv Ther. 2022;39(5):1993–2008. https://doi.org/10.1007/s12325-022-02075-8 .
Scheltema Beduin A, de Haan L. Off-label second generation antipsychotics for impulse regulation disorders: a review. Psychopharmacol Bull. 2010;43(3):45–81.
Hefner G, Wolff J, Toto S, Reißner P, Klimke A. Off-label use of antidepressants, antipsychotics, and mood-stabilizers in psychiatry. J Neural Transm (Vienna). 2022;129(11):1353–65. https://doi.org/10.1007/s00702-022-02542-0 .
Haw C, Stubbs J. Off-label use of antipsychotics: are we mad? Expert Opin Drug Saf. 2007;6(5):533–45. https://doi.org/10.1517/14740338.6.5.533 .
Maher AR, Theodore G. Summary of the comparative effectiveness review on off-label use of atypical antipsychotics. J Manag Care Pharm. 2012;18(5 Suppl B):S1–20. https://doi.org/10.18553/jmcp.2012.18.s5-b.1 .
González-López MDC, Díaz-Calvo V, Ruíz-González C, Nievas-Soriano BJ, Rebollo-Lavado B, Parrón-Carreño T. Consumption of Psychiatric drugs in Primary Care during the COVID-19 pandemic. Int J Environ Res Public Health. 2022;19(8). https://doi.org/10.3390/ijerph19084782 .
Heald AH, Stedman M, Farman S, Khine C, Davies M, De Hert M, et al. Links between the amount of antipsychotic medication prescribed per population at general practice level, local demographic factors and medication selection. BMC Psychiatry. 2020;20(1):528. https://doi.org/10.1186/s12888-020-02915-3 .
Mojtabai R, Olfson M. National trends in psychotropic medication polypharmacy in office-based psychiatry. Arch Gen Psychiatry. 2010;67(1):26–36. https://doi.org/10.1001/archgenpsychiatry.2009.175 .
Drummond N, McCleary L, Freiheit E, Molnar F, Dalziel W, Cohen C, et al. Antidepressant and antipsychotic prescribing in primary care for people with dementia. Can Fam Physician. 2018;64(11):e488–97.
PubMed PubMed Central Google Scholar
Luo H, Lau WCY, Chai Y, Torre CO, Howard R, Liu KY, et al. Rates of antipsychotic drug prescribing among people living with Dementia during the COVID-19 pandemic. JAMA Psychiatry. 2023;80(3):211–9. https://doi.org/10.1001/jamapsychiatry.2022.4448 .
Henderson A, McSkimming P, Kinnear D, McCowan C, McIntosh A, Allan L, et al. Changes over a decade in psychotropic prescribing for people with intellectual disabilities: prospective cohort study. BMJ Open. 2020;10(9):e036862. https://doi.org/10.1136/bmjopen-2020-036862 .
Rogers K, Spring B. Mental Health professionals are in high demand as the pandemic enters a Second Year. CNBC; 2022.
Liu Q, He H, Yang J, Feng X, Zhao F, Lyu J. Changes in the global burden of depression from 1990 to 2017: findings from the Global Burden of Disease study. J Psychiatr Res. 2020;126:134–40. https://doi.org/10.1016/j.jpsychires.2019.08.002 .
Woody CA, Ferrari AJ, Siskind DJ, Whiteford HA, Harris MG. A systematic review and meta-regression of the prevalence and incidence of perinatal depression. J Affect Disord. 2017;219:86–92. https://doi.org/10.1016/j.jad.2017.05.003 .
Chong HY, Teoh SL, Wu DB, Kotirum S, Chiou CF, Chaiyakunapruk N. Global economic burden of schizophrenia: a systematic review. Neuropsychiatr Dis Treat. 2016;12:357–73. https://doi.org/10.2147/ndt.S96649 .
Charlson FJ, Ferrari AJ, Santomauro DF, Diminic S, Stockings E, Scott JG, et al. Global Epidemiology and burden of Schizophrenia: findings from the global burden of Disease Study 2016. Schizophr Bull. 2018;44(6):1195–203. https://doi.org/10.1093/schbul/sby058 .
Global, regional, and, national, burden, of, 12, mental, disorders, in, 204, countries, and, territories, 1990–2019, a, systematic, analysis, for, the, Global, Burden, of, Disease, Study, 2019. Lancet Psychiatry. 2022;9(2):137–50. https://doi.org/10.1016/s2215-0366(21)00395-3 .
Walker ER, McGee RE, Druss BG. Mortality in mental disorders and global disease burden implications: a systematic review and meta-analysis. JAMA Psychiatry. 2015;72(4):334–41. https://doi.org/10.1001/jamapsychiatry.2014.2502 .
Hany M, Rehman B, Azhar Y, Chapman J. Schizophrenia. StatPearls. Treasure Island (FL): StatPearls Publishing Copyright © 2023. StatPearls Publishing LLC.; 2023.
Sweileh WM. Analysis of global research output on diabetes depression and suicide. Ann Gen Psychiatry. 2018;17:44. https://doi.org/10.1186/s12991-018-0214-2 .
Sweileh WM. Contribution of researchers in the arab region to peer-reviewed literature on mental health and well-being of university students. Int J Ment Health Syst. 2021;15(1):50. https://doi.org/10.1186/s13033-021-00477-9 .
Falagas ME, Pitsouni EI, Malietzis GA, Pappas G. Comparison of PubMed, Scopus, web of Science, and Google Scholar: strengths and weaknesses. FASEB Journal: Official Publication Federation Am Soc Experimental Biology. 2008;22(2):338–42. https://doi.org/10.1096/fj.07-9492LSF .
Article CAS Google Scholar
van Eck NJ, Waltman L. Software survey: VOSviewer, a computer program for bibliometric mapping. Scientometrics. 2010;84(2):523–38. https://doi.org/10.1007/s11192-009-0146-3 .
Meltzer HY. Rigidity, hyperpyrexia and coma following fluphenazine enanthate. Psychopharmacologia. 1973;29(4):337–46. https://doi.org/10.1007/bf00429281 .
Silbergeld EK, Hruska RE. Lisuride and LSD: dopaminergic and serotonergic interactions in the serotonin syndrome. Psychopharmacology. 1979;65(3):233–7. https://doi.org/10.1007/bf00492209 .
Henderson VW, Wooten GF. Neuroleptic malignant syndrome: a pathogenetic role for dopamine receptor blockade? Neurology. 1981;31(2):132–7. https://doi.org/10.1212/wnl.31.2.132 .
Dunkley EJ, Isbister GK, Sibbritt D, Dawson AH, Whyte IM. The Hunter Serotonin Toxicity Criteria: simple and accurate diagnostic decision rules for serotonin toxicity. QJM. 2003;96(9):635–42. https://doi.org/10.1093/qjmed/hcg109 .
Gillman PK. Monoamine oxidase inhibitors, opioid analgesics and serotonin toxicity. Br J Anaesth. 2005;95(4):434–41. https://doi.org/10.1093/bja/aei210 .
Ueno S, Otani K, Kaneko S, Koshiro K, Kondoh K, Kotani Y, et al. Cytochrome P-450 2D6 gene polymorphism is not associated with neuroleptic malignant syndrome. Biol Psychiatry. 1996;40(1):72–4. https://doi.org/10.1016/0006-3223(95)00427-0 .
Ochi S, Kawasoe K, Abe M, Fukuhara R, Sonobe K, Kawabe K, et al. A case study: neuroleptic malignant syndrome with risperidone and CYP2D6 gene variation. Gen Hosp Psychiatry. 2011;33(6):640. https://doi.org/10.1016/j.genhosppsych.2011.03.003 . .e1-2.
Article Google Scholar
Kato D, Kawanishi C, Kishida I, Furuno T, Matsumura T, Hasegawa H, et al. CYP2D6 gene deletion allele in patients with neuroleptic malignant syndrome: preliminary report. Psychiatry Clin Neurosci. 2005;59(4):504–7. https://doi.org/10.1111/j.1440-1819.2005.01405.x .
Iwahashi K, Yoshihara E, Nakamura K, Ameno K, Watanabe M, Tsuneoka Y, et al. CYP2D6 HhaI genotype and the neuroleptic malignant syndrome. Neuropsychobiology. 1999;39(1):33–7. https://doi.org/10.1159/000026557 .
Iwahashi K, Nakamura K, Suwaki H, Tsuneoka Y, Ichikawa Y. CYP2D6 Hhal genotype and the neuroleptic malignant syndrome (NMS). Clin Chim Acta. 1997;265(1):143–4. https://doi.org/10.1016/s0009-8981(97)00113-7 .
Kato D, Kawanishi C, Kishida I, Furuno T, Suzuki K, Onishi H, et al. Effects of CYP2D6 polymorphisms on neuroleptic malignant syndrome. Eur J Clin Pharmacol. 2007;63(11):991–6. https://doi.org/10.1007/s00228-007-0355-8 .
Kawanishi C, Shimoda Y, Fujimaki J, Onishi H, Suzuki K, Hanihara T, et al. Mutation involving cytochrome P450IID6 in two Japanese patients with neuroleptic malignant syndrome. J Neurol Sci. 1998;160(1):102–4. https://doi.org/10.1016/s0022-510x(98)00238-x .
Kawanishi C, Hanihara T, Maruyama Y, Matsumura T, Onishi H, Inoue K, et al. Neuroleptic malignant syndrome and hydroxylase gene mutations: no association with CYP2D6A or CYP2D6B. Psychiatr Genet. 1997;7(3):127–9. https://doi.org/10.1097/00041444-199723000-00007 .
Butwicka A, Krystyna S, Retka W, Wolańczyk T. Neuroleptic malignant syndrome in an adolescent with CYP2D6 deficiency. Eur J Pediatr. 2014;173(12):1639–42. https://doi.org/10.1007/s00431-013-2208-z .
Zivković M, Mihaljević-Peles A, Sagud M, Silić A, Mihanović M. The role of CYP2D6 and TaqI a polymorphisms in malignant neuroleptic syndrome: two case reports with three episodes. Psychiatr Danub. 2010;22(1):112–6.
Mihara K, Kondo T, Suzuki A, Yasui-Furukori N, Ono S, Sano A, et al. Relationship between functional dopamine D2 and D3 receptors gene polymorphisms and neuroleptic malignant syndrome. Am J Med Genet B Neuropsychiatr Genet. 2003;117b(1):57–60. https://doi.org/10.1002/ajmg.b.10025 .
Kishida I, Kawanishi C, Furuno T, Kato D, Ishigami T, Kosaka K. Association in Japanese patients between neuroleptic malignant syndrome and functional polymorphisms of the dopamine D(2) receptor gene. Mol Psychiatry. 2004;9(3):293–8. https://doi.org/10.1038/sj.mp.4001422 .
Kishida I, Kawanishi C, Furuno T, Matsumura T, Hasegawa H, Sugiyama N, et al. Lack of association in Japanese patients between neuroleptic malignant syndrome and the TaqI a polymorphism of the dopamine D2 receptor gene. Psychiatr Genet. 2003;13(1):55–7. https://doi.org/10.1097/00041444-200303000-00010 .
Ram A, Cao Q, Keck PE Jr., Pope HG Jr., Otani K, Addonizio G, et al. Structural change in dopamine D2 receptor gene in a patient with neuroleptic malignant syndrome. Am J Med Genet. 1995;60(3):228–30. https://doi.org/10.1002/ajmg.1320600311 .
Akihito Suzuki MD, Tsuyoshi Kondo PD,, MDPD, Koichi Otani MD,, PD, Kazuo Mihara MD,, PD, Norio, Yasui-Furukori MD,, PD, Sano A,, MDPD et al. Association of the TaqI A Polymorphism of the Dopamine D2 Receptor Gene With Predisposition to Neuroleptic Malignant Syndrome. 2001;158(10):1714-6. https://doi.org/10.1176/appi.ajp.158.10.1714 .
Matsusue A, Hara K, Kageura M, Kashiwagi M, Lu W, Ishigami A, et al. Genetic analysis of ryanodine receptor 1 gene and carnitine palmitoyltransferase II gene: an autopsy case of neuroleptic malignant syndrome related to vegetamin. Leg Med (Tokyo). 2009;11(Suppl 1):S570–2. https://doi.org/10.1016/j.legalmed.2009.01.074 .
Miyatake R, Iwahashi K, Matsushita M, Nakamura K, Suwaki H. No association between the neuroleptic malignant syndrome and mutations in the RYR1 gene associated malignant hyperthermia. J Neurol Sci. 1996;143(1–2):161–5. https://doi.org/10.1016/s0022-510x(96)00015-9 .
Sato T, Nishio H, Iwata M, Kentotsuboi, Tamura A, Miyazaki T, et al. Postmortem molecular screening for mutations in ryanodine receptor type 1 (RYR1) gene in psychiatric patients suspected of having died of neuroleptic malignant syndrome. Forensic Sci Int. 2010;194(1–3):77–9. https://doi.org/10.1016/j.forsciint.2009.10.014 .
Russell T, Riazi S, Kraeva N, Steel AC, Hawryluck LA. Ecstacy-induced delayed rhabdomyolysis and neuroleptic malignant syndrome in a patient with a novel variant in the ryanodine receptor type 1 gene. Anaesthesia. 2012;67(9):1021–4. https://doi.org/10.1111/j.1365-2044.2012.07226.x .
Kawanishi C, Hanihara T, Shimoda Y, Suzuki K, Sugiyama N, Onishi H, et al. Lack of association between neuroleptic malignant syndrome and polymorphisms in the 5-HT1A and 5-HT2A receptor genes. Am J Psychiatry. 1998;155(9):1275–7. https://doi.org/10.1176/ajp.155.9.1275 .
Kaneda Y, Kawamura I, Fujii A, Ohmori T. Serotonin syndrome - ‘potential’ role of the CYP2D6 genetic polymorphism in asians. Int J Neuropsychopharmacol. 2002;5(1):105–6. https://doi.org/10.1017/s1461145701002723 .
Calmy KIL, Ambrosioni A, Assouline J, Daali B, Fathi Y. Serotonin syndrome following drug-drug interactions and CYP2D6 and CYP2C19 genetic polymorphisms in an HIV-infected patient. Aids. 2012;26(18):2417–8. https://doi.org/10.1097/QAD.0b013e32835a11ba .
Agarwal P, Omoruyi A, Perai KG, MacDaid K, Burton A. Neuroleptic malignant syndrome (NMS) on clozapine with a potential atypical Interaction with Paliperidone. Case Rep Psychiatry. 2021;2021:5584104. https://doi.org/10.1155/2021/5584104 .
Mazhar F, Akram S, Haider N, Ahmed R. Overlapping of Serotonin Syndrome with neuroleptic malignant syndrome due to Linezolid-Fluoxetine and Olanzapine-Metoclopramide interactions: a Case Report of two serious adverse drug effects caused by Medication Reconciliation failure on Hospital Admission. Case Rep Med. 2016;2016:7128909. https://doi.org/10.1155/2016/7128909 .
Gillman PK. CNS toxicity involving methylene blue: the exemplar for understanding and predicting drug interactions that precipitate serotonin toxicity. J Psychopharmacol. 2011;25(3):429–36. https://doi.org/10.1177/0269881109359098 .
Graber MA, Hoehns TB, Perry PJ. Sertraline-phenelzine drug interaction: a serotonin syndrome reaction. Ann Pharmacother. 1994;28(6):732–5. https://doi.org/10.1177/106002809402800610 .
Gnanadesigan N, Espinoza RT, Smith R, Israel M, Reuben DB. Interaction of serotonergic antidepressants and opioid analgesics: is serotonin syndrome going undetected? J Am Med Dir Assoc. 2005;6(4):265–9. https://doi.org/10.1016/j.jamda.2005.04.012 .
Ailawadhi S, Sung KW, Carlson LA, Baer MR. Serotonin syndrome caused by interaction between citalopram and fentanyl. J Clin Pharm Ther. 2007;32(2):199–202. https://doi.org/10.1111/j.1365-2710.2007.00813.x .
Chan BS, Graudins A, Whyte IM, Dawson AH, Braitberg G, Duggin GG. Serotonin syndrome resulting from drug interactions. Med J Aust. 1998;169(10):523–5. https://doi.org/10.5694/j.1326-5377.1998.tb123399.x .
Clark DB, Andrus MR, Byrd DC. Drug interactions between linezolid and selective serotonin reuptake inhibitors: case report involving sertraline and review of the literature. Pharmacotherapy. 2006;26(2):269–76. https://doi.org/10.1592/phco.26.2.269 .
Bai AD, McKenna S, Wise H, Loeb M, Gill SS. Association of Linezolid with Risk of Serotonin Syndrome in patients receiving antidepressants. JAMA Netw Open. 2022;5(12):e2247426. https://doi.org/10.1001/jamanetworkopen.2022.47426 .
Karkow DC, Kauer JF, Ernst EJ. Incidence of Serotonin Syndrome with Combined Use of Linezolid and Serotonin Reuptake inhibitors compared with Linezolid Monotherapy. J Clin Psychopharmacol. 2017;37(5):518–23. https://doi.org/10.1097/jcp.0000000000000751 .
Gupta V, Karnik ND, Deshpande R, Patil MA. Linezolid-induced serotonin syndrome. BMJ Case Rep. 2013;2013. https://doi.org/10.1136/bcr-2012-008199 .
Mitwally H, Saad MO, Alkhiyami D, Fahmi AM, Mahmoud S, Hmoud EA, et al. Risk of serotonin syndrome in acutely ill patients receiving linezolid and opioids concomitantly: a retrospective cohort study. IJID Reg. 2022;5:137–40. https://doi.org/10.1016/j.ijregi.2022.09.008 .
Huang V, Gortney JS. Risk of serotonin syndrome with concomitant administration of linezolid and serotonin agonists. Pharmacotherapy. 2006;26(12):1784–93. https://doi.org/10.1592/phco.26.12.1784 .
Gatti M, Raschi E, De Ponti F. Serotonin syndrome by drug interactions with linezolid: clues from pharmacovigilance-pharmacokinetic/pharmacodynamic analysis. Eur J Clin Pharmacol. 2021;77(2):233–9. https://doi.org/10.1007/s00228-020-02990-1 .
Masbough F, Roshanzamiri S, Rahimi M, Sahraei Z, Evini PET. Serotonin syndrome due to concomitant use of linezolid and methadone. Clin Case Rep. 2022;10(11):e6341. https://doi.org/10.1002/ccr3.6341 .
Essakow J, Jin L, Marupudi N, Wattier R, McQuillen P, Franzon D. Serotonin Syndrome in an infant Associated with Linezolid and Opioid Use. J Pediatr Pharmacol Ther. 2022;27(6):564–8. https://doi.org/10.5863/1551-6776-27.6.564 .
Spigset O, Adielsson G. Combined serotonin syndrome and hyponatraemia caused by a citalopram-buspirone interaction. Int Clin Psychopharmacol. 1997;12(1):61–3. https://doi.org/10.1097/00004850-199701000-00010 .
Brubacher JR, Hoffman RS, Lurin MJ. Serotonin syndrome from venlafaxine-tranylcypromine interaction. Vet Hum Toxicol. 1996;38(5):358–61.
CAS PubMed Google Scholar
Nelson EM, Philbrick AM. Avoiding serotonin syndrome: the nature of the interaction between tramadol and selective serotonin reuptake inhibitors. Ann Pharmacother. 2012;46(12):1712–6. https://doi.org/10.1345/aph.1Q748 .
Aboukarr A, Giudice M. Interaction between Monoamine oxidase B inhibitors and selective serotonin reuptake inhibitors. Can J Hosp Pharm. 2018;71(3):196–207.
Gray R, Moore Iii A, Berry F, Afroze F, Cherukupalli D. Serotonin Syndrome after PACU Administration of Tramadol and Meperidine. Turk J Anaesthesiol Reanim. 2022;50(4):309–11. https://doi.org/10.5152/tjar.2022.21355 .
Mateo-Carrasco H, Muñoz-Aguilera EM, García-Torrecillas JM, Abu Al-Robb H. Serotonin syndrome probably triggered by a morphine-phenelzine interaction. Pharmacotherapy. 2015;35(6):e102–5. https://doi.org/10.1002/phar.1581 .
Declercq PL, Eraldi JP, Beuzelin M, Gélinotte S, Marchalot A, Bougerol F, et al. Severe serotonin syndrome caused by an interaction between an antidepressant and a cough syrup. Therapie. 2021;76(3):249–52. https://doi.org/10.1016/j.therap.2020.02.020 .
Foong AL, Grindrod KA, Patel T, Kellar J. Demystifying serotonin syndrome (or serotonin toxicity). Can Fam Physician. 2018;64(10):720–7.
Sun-Edelstein C, Tepper SJ, Shapiro RE. Drug-induced serotonin syndrome: a review. Expert Opin Drug Saf. 2008;7(5):587–96. https://doi.org/10.1517/14740338.7.5.587 .
Chan TC, Evans SD, Clark RF. Drug-induced hyperthermia. Crit Care Clin. 1997;13(4):785–808. https://doi.org/10.1016/s0749-0704(05)70369-9 .
Horseman M, Panahi L, Udeani G, Tenpas AS, Verduzco R Jr., Patel PH, et al. Drug-Induced Hyperth Rev Cureus. 2022;14(7):e27278. https://doi.org/10.7759/cureus.27278 .
Savvidou A, Jennions E, Wikström S, Olsson-Engman M, Sofou K, Darin N. Drug-induced hyperthermia with rhabdomyolysis in CLN3 disease. Eur J Paediatr Neurol. 2022;39:74–8. https://doi.org/10.1016/j.ejpn.2022.06.007 .
Gurrera RJ, Caroff SN, Cohen A, Carroll BT, DeRoos F, Francis A, et al. An international consensus study of neuroleptic malignant syndrome diagnostic criteria using the Delphi method. J Clin Psychiatry. 2011;72(9):1222–8. https://doi.org/10.4088/JCP.10m06438 .
Gurrera RJ, Mortillaro G, Velamoor V, Caroff SN. A validation study of the International Consensus Diagnostic Criteria for neuroleptic malignant syndrome. J Clin Psychopharmacol. 2017;37(1):67–71. https://doi.org/10.1097/jcp.0000000000000640 .
Karamustafalioglu N, Kalelioglu T, Celikel G, Genc A, Emul M. Clinical utility of neutrophil-lymphocyte ratio in the diagnosis of neuroleptic malignant syndrome. Nord J Psychiatry. 2019;73(4–5):288–92. https://doi.org/10.1080/08039488.2019.1623315 .
Kalelioglu T, Celikel G, Balaban OD, Karamustafalioglu N, Penberthy JK. Can Neutrophil-Lymphocyte ratio be a useful Criterion for neuroleptic malignant syndrome in the absence of Leukocytosis? Iran J Psychiatry. 2021;16(3):370–3. https://doi.org/10.18502/ijps.v16i3.6264 .
Védie C, Poinso F, Hemmi F, Rivet B. Major symptoms and differential diagnosis of neuroleptic malignant syndrome: three case reports. Eur Psychiatry. 2000;15(5):334–7. https://doi.org/10.1016/s0924-9338(00)00403-x .
Uddin MF, Alweis R, Shah SR, Lateef N, Shahnawaz W, Ochani RK, et al. Controversies in serotonin syndrome diagnosis and management: a review. J Clin Diagn Res. 2017;11(9):Oe05–7. https://doi.org/10.7860/jcdr/2017/29473.10696 .
Jašović-Gašić M, Vuković O, Pantović M, Cvetić T, Marić-Bojović N. Antipsychotics–history of development and field of indication, new wine–old glassess. Psychiatr Danub. 2012;24(Suppl 3):S342–4.
Danilov DS. [A current view on the history of atypical antipsychotics]. Zh Nevrol Psikhiatr Im S S Korsakova. 2017;117(5):85–93. https://doi.org/10.17116/jnevro20171175185-93 .
De Risio A, Lang AP. History and therapeutic rationale of long acting antipsychotics. Curr Clin Pharmacol. 2014;9(1):39–52. https://doi.org/10.2174/15748847113089990057 .
Shen WW. A history of antipsychotic drug development. Compr Psychiatry. 1999;40(6):407–14. https://doi.org/10.1016/s0010-440x(99)90082-2 .
Ramachandraiah CT, Subramaniam N, Tancer M. The story of antipsychotics: past and present. Indian J Psychiatry. 2009;51(4):324–6. https://doi.org/10.4103/0019-5545.58304 .
Perez-Caballero L, Torres-Sanchez S, Bravo L, Mico JA, Berrocoso E. Fluoxetine: a case history of its discovery and preclinical development. Expert Opin Drug Discov. 2014;9(5):567–78. https://doi.org/10.1517/17460441.2014.907790 .
Margetić B, Aukst-Margetić B. Neuroleptic malignant syndrome and its controversies. Pharmacoepidemiol Drug Saf. 2010;19(5):429–35. https://doi.org/10.1002/pds.1937 .
Saha KB, Bo L, Zhao S, Xia J, Sampson S, Zaman RU. Chlorpromazine versus atypical antipsychotic drugs for schizophrenia. Cochrane Database Syst Rev. 2016;4(4):Cd010631. https://doi.org/10.1002/14651858.CD010631.pub2 .
Divac N, Prostran M, Jakovcevski I, Cerovac N. Second-generation antipsychotics and extrapyramidal adverse effects. Biomed Res Int. 2014;2014:656370. https://doi.org/10.1155/2014/656370 .
Meltzer HY. Update on typical and atypical antipsychotic drugs. Annu Rev Med. 2013;64:393–406. https://doi.org/10.1146/annurev-med-050911-161504 .
Talton CW. Serotonin Syndrome/Serotonin toxicity. Fed Pract. 2020;37(10):452–9. https://doi.org/10.12788/fp.0042 .
Sweileh WM, Sawalha AF, Al-Jabi SW, Zyoud SH, Shraim NY, Abu-Taha AS. A bibliometric analysis of literature on malaria vector resistance: (1996–2015). Globalization Health. 2016;12(1):76. https://doi.org/10.1186/s12992-016-0214-4 .
Zyoud SH, Shakhshir M, Abushanab AS, Al-Jabi SW, Koni A, Shahwan M, et al. Mapping the global research landscape on nutrition and the gut microbiota: visualization and bibliometric analysis. World J Gastroenterol. 2022;28(25):2981–93. https://doi.org/10.3748/wjg.v28.i25.2981 .
Sweileh WM. Bibliometric analysis of peer-reviewed literature on climate change and human health with an emphasis on infectious diseases. Globalization Health. 2020;16(1):44. https://doi.org/10.1186/s12992-020-00576-1 .
Sweileh WM. Global research trends of World Health Organization’s top eight emerging pathogens. Globalization Health. 2017;13(1):9. https://doi.org/10.1186/s12992-017-0233-9 .
Bilanakis N, Peritogiannis V, Kalampokis G. Infections as complications of neuroleptic malignant syndrome. World J Biol Psychiatry. 2009;10(4 Pt 3):973–6. https://doi.org/10.1080/15622970801935578 .
Taniguchi N, Tanii H, Nishikawa T, Miyamae Y, Shinozaki K, Inoue Y, et al. Classification system of complications in neuroleptic malignant syndrome. Methods Find Exp Clin Pharmacol. 1997;19(3):193–9.
Hegerl U, Bottlender R, Gallinat J, Kuss HJ, Ackenheil M, Möller HJ. The serotonin syndrome scale: first results on validity. Eur Arch Psychiatry Clin Neurosci. 1998;248(2):96–103. https://doi.org/10.1007/s004060050024 .
Modi S, Dharaiya D, Schultz L, Varelas P. Neuroleptic malignant syndrome: complications, outcomes, and Mortality. Neurocrit Care. 2016;24(1):97–103. https://doi.org/10.1007/s12028-015-0162-5 .
Park JI, Park TW. Rhabdomyolysis and Neuroleptic Malignant Syndrome Associated with very low-dose antipsychotics in children and adolescent. Clin Psychopharmacol Neurosci. 2019;17(3):450–2. https://doi.org/10.9758/cpn.2019.17.3.450 .
Desai S, Hirachan T, Toma A, Gerolemou A. Malignant Catatonia Versus neuroleptic malignant syndrome. Cureus. 2021;13(6):e15818. https://doi.org/10.7759/cureus.15818 .
Shouan A, Kumar R, Lal V, Grover S. Linezolid-induced serotonin syndrome. Ind Psychiatry J. 2020;29(2):345–8. https://doi.org/10.4103/ipj.ipj_37_19 .
Keltner N, Harris CP. Serotonin syndrome: a case of fatal SSRI/MAOI interaction. Perspect Psychiatr Care. 1994;30(4):26–31. https://doi.org/10.1111/j.1744-6163.1994.tb00446.x .
Park SH, Wackernah RC, Stimmel GL. Serotonin syndrome: is it a reason to avoid the use of tramadol with antidepressants? J Pharm Pract. 2014;27(1):71–8. https://doi.org/10.1177/0897190013504957 .
Beakley BD, Kaye AM, Kaye AD, Tramadol. Pharmacology, Side effects, and Serotonin Syndrome: a review. Pain Physician. 2015;18(4):395–400.
Hassamal S, Miotto K, Dale W, Danovitch I. Tramadol: understanding the risk of serotonin syndrome and seizures. Am J Med. 2018;131(11):1382. e1-.e6 https://doi.org/10.1016/j.amjmed.2018.04.025 .
Ruiz de Villa A, Jones T, Lleshi A, Macahuachi M, Lamar K, Bazikian Y. Serotonin toxicity precipitated by Tramadol in the setting of polypharmacy: a case of Serotonin Syndrome. Cureus. 2021;13(11):e20059. https://doi.org/10.7759/cureus.20059 .
Graudins A, Stearman A, Chan B. Treatment of the serotonin syndrome with cyproheptadine. J Emerg Med. 1998;16(4):615–9. https://doi.org/10.1016/s0736-4679(98)00057-2 .
Deardorff OG, Khan T, Kulkarni G, Doisy R, Loehr C. Serotonin Syndrome: Prophylactic Treatment with Cyproheptadine. Prim Care Companion CNS Disord. 2016;18(4). https://doi.org/10.4088/PCC.16br01966 .
Lappin RI, Auchincloss EL. Treatment of the serotonin syndrome with cyproheptadine. N Engl J Med. 1994;331(15):1021–2. https://doi.org/10.1056/nejm199410133311514 .
Baldo BA. Toxicities of opioid analgesics: respiratory depression, histamine release, hemodynamic changes, hypersensitivity, serotonin toxicity. Arch Toxicol. 2021;95(8):2627–42. https://doi.org/10.1007/s00204-021-03068-2 .
Download references
Acknowledgements
Not applicable.
Author information
Authors and affiliations.
Department of Biomedical Sciences, Faculty of Medicine and Health Sciences, An-Najah National University, Nablus, Palestine
Waleed M. Sweileh
You can also search for this author in PubMed Google Scholar
Contributions
W.S initiated the idea, did the analysis, writing, and submission.
Corresponding author
Correspondence to Waleed M. Sweileh .
Ethics declarations
Ethics approval and consent to participate, consent for publication, competing interests.
The authors declare that he has no financial or non-financial competing interests.
Additional information
Publisher’s note.
Springer Nature remains neutral with regard to jurisdictional claims in published maps and institutional affiliations.
Rights and permissions
Open Access This article is licensed under a Creative Commons Attribution 4.0 International License, which permits use, sharing, adaptation, distribution and reproduction in any medium or format, as long as you give appropriate credit to the original author(s) and the source, provide a link to the Creative Commons licence, and indicate if changes were made. The images or other third party material in this article are included in the article’s Creative Commons licence, unless indicated otherwise in a credit line to the material. If material is not included in the article’s Creative Commons licence and your intended use is not permitted by statutory regulation or exceeds the permitted use, you will need to obtain permission directly from the copyright holder. To view a copy of this licence, visit http://creativecommons.org/licenses/by/4.0/ . The Creative Commons Public Domain Dedication waiver ( http://creativecommons.org/publicdomain/zero/1.0/ ) applies to the data made available in this article, unless otherwise stated in a credit line to the data.
Reprints and permissions
About this article
Cite this article.
Sweileh, W.M. Neuroleptic malignant syndrome and serotonin syndrome: a comparative bibliometric analysis. Orphanet J Rare Dis 19 , 221 (2024). https://doi.org/10.1186/s13023-024-03227-5
Download citation
Received : 20 April 2023
Accepted : 27 May 2024
Published : 02 June 2024
DOI : https://doi.org/10.1186/s13023-024-03227-5
Share this article
Anyone you share the following link with will be able to read this content:
Sorry, a shareable link is not currently available for this article.
Provided by the Springer Nature SharedIt content-sharing initiative
- Serotonin syndrome
- Research publications, comparative analysis
Orphanet Journal of Rare Diseases
ISSN: 1750-1172
- Submission enquiries: Access here and click Contact Us
- General enquiries: [email protected]

Official websites use .gov
A .gov website belongs to an official government organization in the United States.
Secure .gov websites use HTTPS
A lock ( ) or https:// means you've safely connected to the .gov website. Share sensitive information only on official, secure websites.

National Health and Nutrition Examination Survey
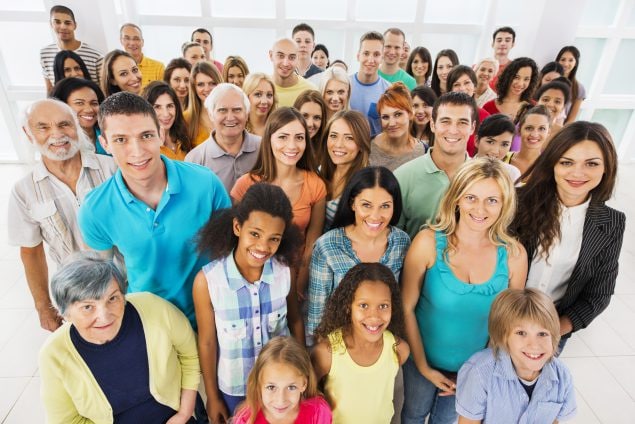
If you were selected, learn more about participating
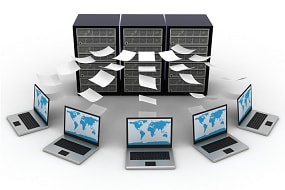
Access data, documentation & response rates
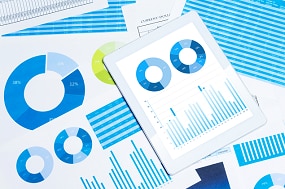
View health and nutrition reports & CDC Growth Charts
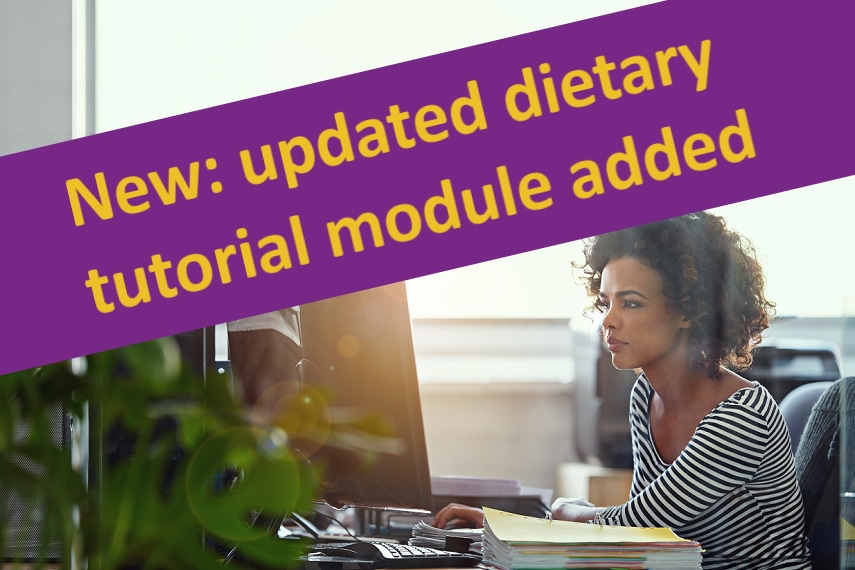
Review step-by-step guidance on using NHANES data
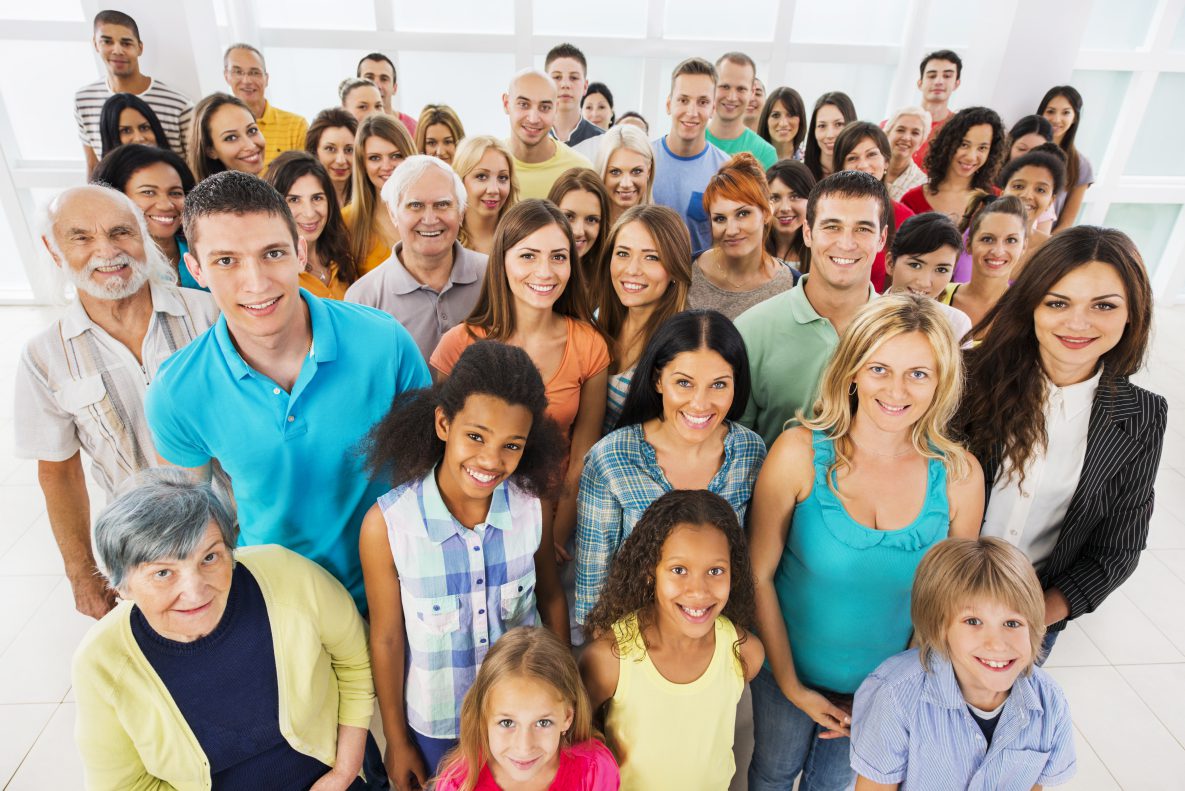
Publications
- Plan and Operations of the National Health and Nutrition Examination Survey, August 2021–August 2023
Data Release
- Perfluoroalkyl and Polyfluoroalkyl Substances (P_PFAS 2017-March 2020)
- NHANES Longitudinal Study
- Growth Charts
- Surveys and Data Collection Systems
- Research Data Center
Exit Notification / Disclaimer Policy
- The Centers for Disease Control and Prevention (CDC) cannot attest to the accuracy of a non-federal website.
- Linking to a non-federal website does not constitute an endorsement by CDC or any of its employees of the sponsors or the information and products presented on the website.
- You will be subject to the destination website's privacy policy when you follow the link.
- CDC is not responsible for Section 508 compliance (accessibility) on other federal or private website.

IMAGES
VIDEO
COMMENTS
Descriptive Analytics. Definition: Descriptive analytics focused on describing or summarizing raw data and making it interpretable. This type of analytics provides insight into what has happened in the past. It involves the analysis of historical data to identify patterns, trends, and insights. Descriptive analytics often uses visualization ...
Reflect on the QCI research scenario and the associated data set discussed in Chap. 10.1007/978-981-15-2537-7_4. Consider the following questions that Maree might wish to address with respect to decision accuracy and speed scores: ... Analysis Descriptive Statistics Descriptive Statistics then select the reports and plots that you want to see ...
A data set is a collection of responses or observations from a sample or entire population. In quantitative research, after collecting data, the first step of statistical analysis is to describe characteristics of the responses, such as the average of one variable (e.g., age), or the relation between two variables (e.g., age and creativity).
Descriptive research methods. Descriptive research is usually defined as a type of quantitative research, though qualitative research can also be used for descriptive purposes. The research design should be carefully developed to ensure that the results are valid and reliable.. Surveys. Survey research allows you to gather large volumes of data that can be analyzed for frequencies, averages ...
16. Approaching Descriptive Analysis: Summary Descriptive analysis is a valuable research tool. It can contribute to a wide range of studies, both descriptive and causal in nature. When approaching descriptive work, researchers should endeavor to first recognize a phenomenon of interest.
Numeric data collected in a research project can be analysed quantitatively using statistical tools in two different ways. Descriptive analysis refers to statistically describing, aggregating, and presenting the constructs of interest or associations between these constructs.Inferential analysis refers to the statistical testing of hypotheses (theory testing).
Descriptive analytics is the simplest form of data analysis, and involves summarizing a data set's main features and characteristics. Descriptive analytics relies on statistical measures of distribution, central tendency, and variability. It provides an overview of varied data types, from financial statements to surveys, website traffic, and ...
5 Examples of Descriptive Analytics. 1. Traffic and Engagement Reports. One example of descriptive analytics is reporting. If your organization tracks engagement in the form of social media analytics or web traffic, you're already using descriptive analytics. These reports are created by taking raw data—generated when users interact with ...
Performance Measurement and Management. Descriptive techniques often include constructing tables of means and quantiles, measures of dispersion such as variance or standard deviation, and cross-tabulations or "crosstabs" that can be used to examine many disparate hypotheses. Those hypotheses are often about observed differences across subgroups.
In descriptive analysis, it's also worth knowing the central (or average) event or response. Common measures of central tendency include the three averages — mean, median, and mode. As an example, consider a survey in which the height of 1,000 people is measured. In this case, the mean average would be a very helpful descriptive metric.
Descriptive analysis is a sort of data research that aids in describing, demonstrating, or helpfully summarizing data points so those patterns may develop that satisfy all of the conditions of the data. It is the technique of identifying patterns and links by utilizing recent and historical data. Because it identifies patterns and associations ...
Numeric data collected in a research project can be analyzed quantitatively using statistical tools in two different ways. Descriptive analysis refers to statistically describing, aggregating, and presenting the constructs of interest or associations between these constructs. Inferential analysis refers to the statistical testing of hypotheses ...
Quantitative data analysis serves as part of an essential process of evidence-making in health and social sciences. It is adopted for any types of research question and design whether it is descriptive, explanatory, or causal. However, compared with qualitative counterpart, quantitative data analysis has less flexibility.
Descriptive statistics try to describe the relationship between variables in a sample or population. Descriptive statistics provide a summary of data in the form of mean, median and mode. Inferential statistics use a random sample of data taken from a population to describe and make inferences about the whole population. It is valuable when it ...
Descriptive research design. Descriptive research design uses a range of both qualitative research and quantitative data (although quantitative research is the primary research method) to gather information to make accurate predictions about a particular problem or hypothesis. As a survey method, descriptive research designs will help ...
Descriptive research typically involves the collection of both qualitative and quantitative data through methods such as surveys, observational studies, case studies, or focus groups. 4. Data Analysis. Descriptive research data is analyzed to identify patterns, relationships, and trends within the data.
Definition of research in data analysis: According to LeCompte and Schensul, research data analysis is a process used by researchers to reduce data to a story and interpret it to derive insights. ... Descriptive analysis is also called a 'univariate analysis' since it is commonly used to analyze a single variable. Inferential statistics.
Qualitative research collects data qualitatively, and the method of analysis is also primarily qualitative. This often involves an inductive exploration of the data to identify recurring themes, patterns, or concepts and then describing and interpreting those categories. Of course, in qualitative research, the data collected qualitatively can ...
Descriptive statistics, such as means and percentages, describe data obtained from empirical observations and measurements, whereas inferen tial statistics are used to make infer ences or draw conclusions about a population, given the data were actu ally obtained for a sample. This article briefly discusses common descriptive data analysis ...
A particularly important part of the thematic analysis process involves coding the data. A code is simply a short, descriptive word or phrase that assigns meaning to the data related to the researcher's analytic interests. ... On the basis of Rocco (2010), Storberg-Walker's (2012) amended list on qualitative data analysis in research papers ...
Descriptive research is a type of research that is used to describe the characteristics of a population. It collects data that are used to answer a wide range of what, when, and how questions pertaining to a particular population or group. For example, descriptive studies might be used to answer questions such as: What percentage of Head Start ...
Quantitative data is information about quantities, and therefore numbers, and qualitative data is descriptive, and regards phenomenon which can be observed but not measured, such as language. ... Qualitative Data Analysis. Qualitative research is endlessly creative and interpretive. The researcher does not just leave the field with mountains of ...
Unlike quantitative data, qualitative data is descriptive, expressed in terms of language rather than numerical values. Qualitative data analysis describes information and cannot be measured or counted. It refers to the words or labels used to describe certain characteristics or traits. You would turn to qualitative data to answer the "why?" or ...
Clean the data in preparation for analysis. Analyze the data. Interpret the results of the analysis. Data analysis can take different forms, depending on the question you're trying to answer. You can read more about the types of data analysis here. Briefly, descriptive analysis tells us what happened, diagnostic analysis tells us why it ...
Descriptive statistics, which describes the properties of sample and population data, is used in our daily personal and business lives. Descriptive statistics is a way to organize, represent, and describe a collection of data, using tables, graphs, and summary measures, including popular parameters such as mean (average), median, or standard deviation.
Text-mining by SAS text miner and content analysis was used to analyse the data. Analysis of data led to the extraction of 93 initial codes and two categories i.e., 1) negative emotions expressed by healthcare staff which included 4 sub-categories of feelings: (i) fear; (ii) disturbed; (iii) sadness; (iv) guilt and 2) Immediate response from ...
Data were analyzed using Microsoft Excel for descriptive analysis, and VOSviewer was employed for bibliometric mapping. The search yielded 1150 articles on NMS and 587 on SS, with the majority being case reports. Growth patterns revealed a surge in NMS research between 1981 and 1991, while SS research increased notably between 1993 and 1997.
The National Health and Nutrition Examination Survey (NHANES) is a program that monitors the health and nutritional status of the U.S. population.