Subscribe to the PwC Newsletter
Join the community, trending research, the ai scientist: towards fully automated open-ended scientific discovery.

This approach signifies the beginning of a new era in scientific discovery in machine learning: bringing the transformative benefits of AI agents to the entire research process of AI itself, and taking us closer to a world where endless affordable creativity and innovation can be unleashed on the world's most challenging problems.


Zero-Shot Surgical Tool Segmentation in Monocular Video Using Segment Anything Model 2
AngeLouCN/SAM-2_Surgical_Video • 3 Aug 2024
The Segment Anything Model 2 (SAM 2) is the latest generation foundation model for image and video segmentation.
LongWriter: Unleashing 10,000+ Word Generation from Long Context LLMs
By incorporating this dataset into model training, we successfully scale the output length of existing models to over 10, 000 words while maintaining output quality.
DeepSeek-Prover-V1.5: Harnessing Proof Assistant Feedback for Reinforcement Learning and Monte-Carlo Tree Search
We introduce DeepSeek-Prover-V1. 5, an open-source language model designed for theorem proving in Lean 4, which enhances DeepSeek-Prover-V1 by optimizing both training and inference processes.
Bilateral Reference for High-Resolution Dichotomous Image Segmentation
It comprises two essential components: the localization module (LM) and the reconstruction module (RM) with our proposed bilateral reference (BiRef).

MixTex: Unambiguous Recognition Should Not Rely Solely on Real Data

This paper introduces MixTex, an end-to-end LaTeX OCR model designed for low-bias multilingual recognition, along with its novel data collection method.

ControlNeXt: Powerful and Efficient Control for Image and Video Generation
In this paper, we propose ControlNeXt: a powerful and efficient method for controllable image and video generation.
Text-Driven Image Editing via Learnable Regions
Language has emerged as a natural interface for image editing.

LGRNet: Local-Global Reciprocal Network for Uterine Fibroid Segmentation in Ultrasound Videos
To this end, we collect and annotate the first ultrasound video dataset with 100 videos for uterine fibroid segmentation (UFUV).

OpenResearcher: Unleashing AI for Accelerated Scientific Research
gair-nlp/openresearcher • 13 Aug 2024
The rapid growth of scientific literature imposes significant challenges for researchers endeavoring to stay updated with the latest advancements in their fields and delve into new areas.
The Journal of Machine Learning Research
Volume 24, Issue 1
January 2023
- new algorithms with empirical, theoretical, psychological, or biological justification;
- experimental and/or theoretical studies yielding new insight into the design and behavior of learning in intelligent systems;
- accounts of applications of existing techniques that shed light on the strengths and weaknesses of the methods;
- formalization of new learning tasks (e.g., in the context of new applications) and of methods for assessing performance on those tasks;
- development of new analytical frameworks that advance theoretical studies of practical learning methods;
- computational models of data from natural learning systems at the behavioral or neural level; or
- extremely well-written surveys of existing work.
JMLR has a commitment to rigorous yet rapid reviewing. Final versions are published electronically (ISSN 1533-7928) immediately upon receipt. Printed volumes (ISSN: 1532-4435) are now published by Microtome Publishing and available for sale .
Subject Areas
Announcements.
ACM Updates Its Peer Review Policy
ACM is pleased to announce that its Publications Board has approved an updated Peer Review Policy . If you have any questions regarding the update, the associated FAQ addresses topics such as confidentiality, the use of large language models in the peer review process, conflicts of interest, and several other relevant concerns. If there are any issues that are not addressed in the FAQ, please contact ACM’s Director of Publications, Scott Delman .
New ACM Policy on Authorship ACM has a new Policy on Authorship , covering a range of key topics, including the use of generative AI tools. Please familiarize yourself with the new policy and the associated list of Frequently Asked Questions .
Most Frequent Affiliations
Most cited authors, latest issue.
- Volume 24, Issue 1 January 2023 ISSN: 1532-4435 EISSN: 1533-7928 View Table of Contents
The measure and mismeasure of fairness
Department of Statistics, Harvard University, Cambridge, MA
Department of Computer Science, Stanford University, Stanford, CA
Department of Applied Statistics, Social Science, and Humanities, New York University, New York, NY
Harvard Kennedy School, Harvard University, Cambridge, MA
PaLM: scaling language modeling with pathways

Recent Award Winners
Most popular, other acm journals.

Volume 2, Issue 2

Volume 3, Issue 2
April-June 2024

Volume 56, Issue 12
December 2024

Volume 5, Issue 2

Volume 36, Issue 2
Export Citations
- Please download or close your previous search result export first before starting a new bulk export. Preview is not available. By clicking download, a status dialog will open to start the export process. The process may take a few minutes but once it finishes a file will be downloadable from your browser. You may continue to browse the DL while the export process is in progress. Download
- Download citation
- Copy citation
We are preparing your search results for download ...
We will inform you here when the file is ready.
Your file of search results citations is now ready.
Your search export query has expired. Please try again.
Machine Learning
- Reports substantive results on a wide range of learning methods applied to various learning problems.
- Provides robust support through empirical studies, theoretical analysis, or comparison to psychological phenomena.
- Demonstrates how to apply learning methods to solve significant application problems.
- Improves how machine learning research is conducted.
- Prioritizes verifiable and replicable supporting evidence in all published papers.
- Hendrik Blockeel
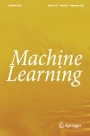
Latest issue
Volume 113, Issue 9
Latest articles
A cross-domain user association scheme based on graph attention networks with trajectory embedding.
- Zhenghao Yang
- Minhong Dong
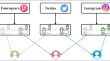
A class sensitivity feature guided T-type generative model for noisy label classification
- Hengjian Cui
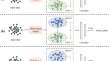
Weighting non-IID batches for out-of-distribution detection
- Zhilin Zhao
- Longbing Cao
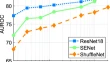
Learning an adaptive forwarding strategy for mobile wireless networks: resource usage vs. latency
- Victoria Manfredi
- Alicia P. Wolfe
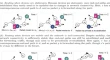
Empirical Bayes linked matrix decomposition
- Eric F. Lock
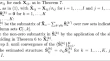
Journal updates
Cfp: discovery science 2023.
Submission Deadline: March 4, 2024
Guest Editors: Rita P. Ribeiro, Albert Bifet, Ana Carolina Lorena
CfP: IJCLR Learning and reasoning
Call for papers: conformal prediction and distribution-free uncertainty quantification.
Submission Deadline: January 7th, 2024
Guest Editors: Henrik Boström, Eyke Hüllermeier, Ulf Johansson, Khuong An Nguyen, Aaditya Ramdas
Call for Papers: Special Issue on Explainable AI for Secure Applications
Submissions Open: October 15, 2024 Submission Deadline: January 15, 2025
Guest Editors: Annalisa Appice, Giuseppeina Andresini, Przemysław Biecek, Christian Wressnegger
Journal information
- ACM Digital Library
- Current Contents/Engineering, Computing and Technology
- EI Compendex
- Google Scholar
- Japanese Science and Technology Agency (JST)
- Mathematical Reviews
- OCLC WorldCat Discovery Service
- Science Citation Index Expanded (SCIE)
- TD Net Discovery Service
- UGC-CARE List (India)
Rights and permissions
Editorial policies
© Springer Science+Business Media LLC, part of Springer Nature
- Find a journal
- Publish with us
- Track your research
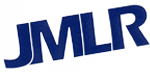
Frequently Asked Questions
JMLR Papers
Select a volume number to see its table of contents with links to the papers.
Volume 23 (January 2022 - Present)
Volume 22 (January 2021 - December 2021)
Volume 21 (January 2020 - December 2020)
Volume 20 (January 2019 - December 2019)
Volume 19 (August 2018 - December 2018)
Volume 18 (February 2017 - August 2018)
Volume 17 (January 2016 - January 2017)
Volume 16 (January 2015 - December 2015)
Volume 15 (January 2014 - December 2014)
Volume 14 (January 2013 - December 2013)
Volume 13 (January 2012 - December 2012)
Volume 12 (January 2011 - December 2011)
Volume 11 (January 2010 - December 2010)
Volume 10 (January 2009 - December 2009)
Volume 9 (January 2008 - December 2008)
Volume 8 (January 2007 - December 2007)
Volume 7 (January 2006 - December 2006)
Volume 6 (January 2005 - December 2005)
Volume 5 (December 2003 - December 2004)
Volume 4 (Apr 2003 - December 2003)
Volume 3 (Jul 2002 - Mar 2003)
Volume 2 (Oct 2001 - Mar 2002)
Volume 1 (Oct 2000 - Sep 2001)
Special Topics
Bayesian Optimization
Learning from Electronic Health Data (December 2016)
Gesture Recognition (May 2012 - present)
Large Scale Learning (Jul 2009 - present)
Mining and Learning with Graphs and Relations (February 2009 - present)
Grammar Induction, Representation of Language and Language Learning (Nov 2010 - Apr 2011)
Causality (Sep 2007 - May 2010)
Model Selection (Apr 2007 - Jul 2010)
Conference on Learning Theory 2005 (February 2007 - Jul 2007)
Machine Learning for Computer Security (December 2006)
Machine Learning and Large Scale Optimization (Jul 2006 - Oct 2006)
Approaches and Applications of Inductive Programming (February 2006 - Mar 2006)
Learning Theory (Jun 2004 - Aug 2004)
Special Issues
In Memory of Alexey Chervonenkis (Sep 2015)
Independent Components Analysis (December 2003)
Learning Theory (Oct 2003)
Inductive Logic Programming (Aug 2003)
Fusion of Domain Knowledge with Data for Decision Support (Jul 2003)
Variable and Feature Selection (Mar 2003)
Machine Learning Methods for Text and Images (February 2003)
Eighteenth International Conference on Machine Learning (ICML2001) (December 2002)
Computational Learning Theory (Nov 2002)
Shallow Parsing (Mar 2002)
Kernel Methods (December 2001)
. |
Machine Learning - Science topic

- Recruit researchers
- Join for free
- Login Email Tip: Most researchers use their institutional email address as their ResearchGate login Password Forgot password? Keep me logged in Log in or Continue with Google Welcome back! Please log in. Email · Hint Tip: Most researchers use their institutional email address as their ResearchGate login Password Forgot password? Keep me logged in Log in or Continue with Google No account? Sign up
Information
- Author Services
Initiatives
You are accessing a machine-readable page. In order to be human-readable, please install an RSS reader.
All articles published by MDPI are made immediately available worldwide under an open access license. No special permission is required to reuse all or part of the article published by MDPI, including figures and tables. For articles published under an open access Creative Common CC BY license, any part of the article may be reused without permission provided that the original article is clearly cited. For more information, please refer to https://www.mdpi.com/openaccess .
Feature papers represent the most advanced research with significant potential for high impact in the field. A Feature Paper should be a substantial original Article that involves several techniques or approaches, provides an outlook for future research directions and describes possible research applications.
Feature papers are submitted upon individual invitation or recommendation by the scientific editors and must receive positive feedback from the reviewers.
Editor’s Choice articles are based on recommendations by the scientific editors of MDPI journals from around the world. Editors select a small number of articles recently published in the journal that they believe will be particularly interesting to readers, or important in the respective research area. The aim is to provide a snapshot of some of the most exciting work published in the various research areas of the journal.
Original Submission Date Received: .
- Active Journals
- Find a Journal
- Proceedings Series
- For Authors
- For Reviewers
- For Editors
- For Librarians
- For Publishers
- For Societies
- For Conference Organizers
- Open Access Policy
- Institutional Open Access Program
- Special Issues Guidelines
- Editorial Process
- Research and Publication Ethics
- Article Processing Charges
- Testimonials
- Preprints.org
- SciProfiles
- Encyclopedia
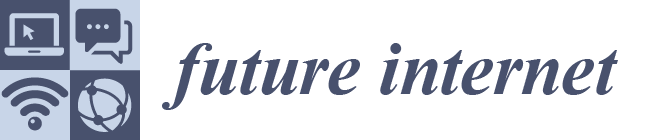
Article Menu

- Subscribe SciFeed
- Recommended Articles
- Google Scholar
- on Google Scholar
- Table of Contents
Find support for a specific problem in the support section of our website.
Please let us know what you think of our products and services.
Visit our dedicated information section to learn more about MDPI.
JSmol Viewer
Machine learning: models, challenges, and research directions.
1. Introduction
- Brief discussion of data pre-processing;
- Detailed classification of supervised, semi-supervised, unsupervised, and reinforcement learning models;
- Study of known optimization techniques;
- Challenges of machine learning in the field of cybersecurity.
2. Related Work and Research Methodology
Reference | Year | Study Highlights | Coverage of Data Pre-Processing and Hyperparameter Tuning | Coverage of Machine Learning | ||||
---|---|---|---|---|---|---|---|---|
Data Pre-Processing | Hyperparameter Tuning Approach | Supervised Learning | Unsupervised Learning | Semi-Supervised Learning | Reinforcement Learning | |||
[ ] | 2021 | Describes the known deep learning models, their principles, and characteristics. | ✓ | ✓ | ||||
[ ] | 2019 | Focuses on limited machine learning techniques on only software-defined networking. | ✓ | ✓ | ✓ | ✓ | ||
[ ] | 2022 | Investigates the known issues in the field of system designs that can be solved using machine learning techniques. | ✓ | ✓ | ✓ | |||
[ ] | 2021 | Presents a detailed description of a few supervised models and their optimization techniques. | ✓ | ✓ | ||||
[ ] | 2021 | Provides an overview of semi-supervised machine learning techniques with their existing algorithms. | ✓ | |||||
[ ] | 2022 | Provides the state of the art, challenges, and limitations of supervised models in the field of maritime risk analysis. | ✓ | |||||
[ ] | 2022 | Reviews hardware architecture of reinforcement learning algorithms. | ✓ | |||||
[ ] | 2022 | Presents the existing algorithm for wireless sensor networks and describes the existing challenges of using such techniques. | ✓ | |||||
[ ] | 2016 | Describes most of the known supervised algorithms for classification problems. | ✓ | |||||
[ ] | 2019 | Provides a description of known supervised and unsupervised models. | ✓ | ✓ | ||||
[ ] | 2021 | Discusses supervised and unsupervised deep learning models for intrusion detection systems. | ✓ | ✓ | ||||
[ ] | 2021 | Surveys existing supervised and unsupervised techniques in smart grid. | ✓ | ✓ | ||||
[ ] | 2021 | Explains known algorithms for image classifications. | ✓ | ✓ | ✓ | |||
[ ] | 2022 | Illustrates the unsupervised deep learning models and summarizes their challenges. | ✓ | |||||
[ ] | 2023 | Discusses techniques for energy usage in future | ✓ | ✓ | ✓ | ✓ | ||
[ ] | 2020 | Reviews various ML techniques in the security of the Internet of Things. | ✓ | ✓ | ✓ | ✓ | ||
[ ] | 2020 | Proposes a taxonomy of machine learning techniques in the security of Internet of Things. | ✓ | ✓ | ✓ | ✓ | ||
[ ] | 2019 | Surveys the taxonomy of machine learning models in intrusion detection systems. | ✓ | ✓ | ✓ | |||
[ ] | 2022 | Gives ML techniques in industrial control systems. | ✓ | ✓ | ✓ | ✓ | ✓ | |
[ ] | 2022 | Proposes the taxonomy of intrusion detection systems for supervised models. | ✓ |
3. Machine Learning Models
3.1. supervised learning, 3.2. semi-supervised learning, 3.3. unsupervised learning, 3.4. reinforcement learning, 4. machine learning processes, 4.1. data pre-processing, 4.2. tuning approaches, 4.3. evaluation metrics, 4.3.1. evaluation metrics for supervised learning, 4.3.2. evaluation metrics for unsupervised learning models, 4.3.3. evaluation metrics for semi-supervised learning models, 4.3.4. evaluation metrics for reinforcement learning models, 5. challenges and future directions, 6. conclusions, author contributions, data availability statement, conflicts of interest.
- Sarker, I.H. Machine Learning: Algorithms, real-world applications and research directions. SN Comput. Sci. 2021 , 2 , 160. [ Google Scholar ] [ CrossRef ]
- Vinuesa, R.; Azizpour, H.; Leite, I.; Balaam, M.; Dignum, V.; Domisch, S.; Felländer, A.; Langhans, S.D.; Tegmark, M.; Nerini, F.F. The role of artificial intelligence in achieving the sustainable development goals. Nat. Commun. 2020 , 11 , 233. [ Google Scholar ] [ CrossRef ] [ PubMed ]
- Ullah, Z.; Al-Turjman, F.; Mostarda, L.; Gagliardi, R. Applications of artificial intelligence and machine learning in smart cities. Comput. Commun. 2020 , 154 , 313–323. [ Google Scholar ] [ CrossRef ]
- Ozcanli, A.K.; Yaprakdal, F.; Baysal, M. Deep learning methods and applications for electrical power systems: A comprehensive review. Int. J. Energy Res. 2020 , 44 , 7136–7157. [ Google Scholar ] [ CrossRef ]
- Zhao, S.; Blaabjerg, F.; Wang, H. An Overview of Artificial Intelligence Applications for Power Electronics. IEEE Trans. Power Electron. 2021 , 36 , 4633–4658. [ Google Scholar ] [ CrossRef ]
- Mamun, A.A.; Sohel, M.; Mohammad, N.; Sunny, M.S.H.; Dipta, D.R.; Hossain, E. A Comprehensive Review of the Load Fore-casting Techniques Using Single and Hybrid Predictive Models. IEEE Access 2020 , 8 , 134911–134939. [ Google Scholar ] [ CrossRef ]
- Massaoudi, M.; Darwish, A.; Refaat, S.S.; Abu-Rub, H.; Toliyat, H.A. UHF Partial Discharge Localization in Gas-Insulated Switch-gears: Gradient Boosting Based Approach. In Proceedings of the 2020 IEEE Kansas Power and Energy Conference (KPEC), Manhattan, KS, USA, 13–14 July 2020; pp. 1–5. [ Google Scholar ]
- Ali, S.S.; Choi, B.J. State-of-the-Art Artificial Intelligence Techniques for Distributed Smart Grids: A Review. Electronics 2020 , 9 , 1030. [ Google Scholar ] [ CrossRef ]
- Yin, L.; Gao, Q.; Zhao, L.; Zhang, B.; Wang, T.; Li, S.; Liu, H. A review of machine learning for new generation smart dispatch in power systems. Eng. Appl. Artif. Intell. 2020 , 88 , 103372. [ Google Scholar ] [ CrossRef ]
- Peng, S.; Sun, S.; Yao, Y.-D. A Survey of Modulation Classification Using Deep Learning: Signal Representation and Data Prepro-cessing. In IEEE Transactions on Neural Networks and Learning Systems ; IEEE: New York, NY, USA, 2021. [ Google Scholar ]
- Arjoune, Y.; Kaabouch, N. A Comprehensive Survey on Spectrum Sensing in Cognitive Radio Networks: Recent Advances, New Challenges, and Future Research Directions. Sensors 2019 , 19 , 126. [ Google Scholar ] [ CrossRef ]
- Meng, T.; Jing, X.; Yan, Z.; Pedrycz, W. A survey on machine learning for data fusion. Inf. Fusion 2020 , 57 , 115–129. [ Google Scholar ] [ CrossRef ]
- Carvalho, D.V.; Pereira, E.M.; Cardoso, J.S. Machine Learning Interpretability: A Survey on Methods and Metrics. Electronics 2019 , 8 , 832. [ Google Scholar ] [ CrossRef ]
- Khoei, T.T.; Ismail, S.; Kaabouch, N. Boosting-based Models with Tree-structured Parzen Estimator Optimization to Detect Intrusion Attacks on Smart Grid. In Proceedings of the 2021 IEEE 12th Annual Ubiquitous Computing, Electronics & Mobile Communication Conference (UEMCON), New York, NY, USA, 1–4 December 2021; pp. 165–170. [ Google Scholar ] [ CrossRef ]
- Hutter, F.; Lücke, J.; Schmidt-Thieme, L. Beyond manual tuning of hyperparameters. KI-Künstliche Intell. 2015 , 29 , 329–337. [ Google Scholar ] [ CrossRef ]
- Khoei, T.T.; Aissou, G.; Hu, W.C.; Kaabouch, N. Ensemble Learning Methods for Anomaly Intrusion Detection System in Smart Grid. In Proceedings of the IEEE International Conference on Electro Information Technology (EIT), Mt. Pleasant, MI, USA, 14–15 May 2021; pp. 129–135. [ Google Scholar ] [ CrossRef ]
- Waubert de Puiseau, C.; Meyes, R.; Meisen, T. On reliability of reinforcement learning based production scheduling systems: A comparative survey. J. Intell. Manuf. 2022 , 33 , 911–927. [ Google Scholar ] [ CrossRef ]
- Moos, J.; Hansel, K.; Abdulsamad, H.; Stark, S.; Clever, D.; Peters, J. Robust Reinforcement Learning: A Review of Foundations and Recent Advances. Mach. Learn. Knowl. Extr. 2022 , 4 , 276–315. [ Google Scholar ] [ CrossRef ]
- Latif, S.; Cuayáhuitl, H.; Pervez, F.; Shamshad, F.; Ali, H.S.; Cambria, E. A survey on deep reinforcement learning for audio-based applications. Artif. Intell. Rev. 2022 , 56 , 2193–2240. [ Google Scholar ] [ CrossRef ]
- Passah, A.; Kandar, D. A lightweight deep learning model for classification of synthetic aperture radar images. Ecol. Inform. 2023 , 77 , 102228. [ Google Scholar ] [ CrossRef ]
- Verbraeken, J.; Wolting, M.; Katzy, J.; Kloppenburg, J.; Verbelen, T.; Rellermeyer, J.S. A survey on distributed machine learning. ACM Comput. Surv. 2020 , 53 , 1–33. [ Google Scholar ] [ CrossRef ]
- Dargan, S.; Kumar, M.; Ayyagari, M.R.; Kumar, G. A survey of deep learning and its applications: A new paradigm to machine learning. Arch. Comput. Methods Eng. 2020 , 27 , 1071–1092. [ Google Scholar ] [ CrossRef ]
- Pitropakis, N.; Panaousis, E.; Giannetsos, T.; Anastasiadis, E.; Loukas, G. A taxonomy and survey of attacks against machine learning. Comput. Sci. Rev. 2019 , 34 , 100199. [ Google Scholar ] [ CrossRef ]
- Wu, X.; Xiao, L.; Sun, Y.; Zhang, J.; Ma, T.; He, L. A survey of human-in-the-loop for machine learning. Futur. Gener. Comput. Syst. 2022 , 135 , 364–381. [ Google Scholar ] [ CrossRef ]
- Wang, Q.; Ma, Y.; Zhao, K.; Tian, Y. A comprehensive survey of loss functions in machine learning. Ann. Data Sci. 2022 , 9 , 187–212. [ Google Scholar ] [ CrossRef ]
- Choi, H.; Park, S. A Survey of Machine Learning-Based System Performance Optimization Techniques. Appl. Sci. 2021 , 11 , 3235. [ Google Scholar ] [ CrossRef ]
- Rawson, A.; Brito, M. A survey of the opportunities and challenges of supervised machine learning in maritime risk analysis. Transp. Rev. 2022 , 43 , 108–130. [ Google Scholar ] [ CrossRef ]
- Ahmad, R.; Wazirali, R.; Abu-Ain, T. Machine Learning for Wireless Sensor Networks Security: An Overview of Challenges and Issues. Sensors 2022 , 22 , 4730. [ Google Scholar ] [ CrossRef ] [ PubMed ]
- Singh, A.; Thakur, N.; Sharma, A. A review of supervised machine learning algorithms. In Proceedings of the 2016 3rd International Conference on Computing for Sustainable Global Development (INDIACom), New Delhi, India, 16–18 March 2016; pp. 1310–1315. [ Google Scholar ]
- Abdallah, E.E.; Eleisah, W.; Otoom, A.F. Intrusion Detection Systems using Supervised Machine Learning Techniques: A survey. Procedia Comput. Sci. 2022 , 201 , 205–212. [ Google Scholar ] [ CrossRef ]
- Dike, H.U.; Zhou, Y.; Deveerasetty, K.K.; Wu, Q. Unsupervised Learning Based On Artificial Neural Network: A Review. In Proceedings of the 2018 IEEE International Conference on Cyborg and Bionic Systems (CBS), 25–27 October 2018; pp. 322–327. [ Google Scholar ]
- van Engelen, J.E.; Hoos, H.H. A survey on semi-supervised learning. Mach. Learn. 2020 , 109 , 373–440. [ Google Scholar ] [ CrossRef ]
- Rothmann, M.; Porrmann, M. A Survey of Domain-Specific Architectures for Reinforcement Learning. IEEE Access 2022 , 10 , 13753–13767. [ Google Scholar ] [ CrossRef ]
- Dong, S.; Wang, P.; Abbas, K. A survey on deep learning and its applications. Comput. Sci. Rev. 2020 , 40 , 100379. [ Google Scholar ] [ CrossRef ]
- Ray, S. A Quick Review of Machine Learning Algorithms. In Proceedings of the 2019 International Conference on Machine Learning, Big Data, Cloud and Parallel Computing (COMITCon), Faridabad, India, 14–16 February 2019; pp. 35–39. [ Google Scholar ]
- Lansky, J.; Ali, S.; Mohammadi, M.; Majeed, M.K.; Karim, S.H.T.; Rashidi, S.; Hosseinzadeh, M.; Rahmani, A.M. Deep Learning-Based Intrusion Detection Systems: A Systematic Review. IEEE Access 2021 , 9 , 101574–101599. [ Google Scholar ] [ CrossRef ]
- Massaoudi, M.; Abu-Rub, H.; Refaat, S.S.; Chihi, I.; Oueslati, F.S. Deep Learning in Smart Grid Technology: A Review of Recent Advancements and Future Prospects. IEEE Access 2021 , 9 , 54558–54578. [ Google Scholar ] [ CrossRef ]
- Liu, H.; Lang, B. Machine Learning and Deep Learning Methods for Intrusion Detection Systems: A Survey. Appl. Sci. 2019 , 9 , 4396. [ Google Scholar ] [ CrossRef ]
- Wu, N.; Xie, Y. A survey of machine learning for computer architecture and systems. ACM Comput. Surv. 2022 , 55 , 1–39. [ Google Scholar ] [ CrossRef ]
- Schmarje, L.; Santarossa, M.; Schröder, S.-M.; Koch, R. A Survey on Semi-, Self- and Unsupervised Learning for Image Classification. IEEE Access 2021 , 9 , 82146–82168. [ Google Scholar ] [ CrossRef ]
- Xie, J.; Yu, F.R.; Huang, T.; Xie, R.; Liu, J.; Wang, C.; Liu, Y. A Survey of Machine Learning Techniques Applied to Software Defined Networking (SDN): Research Issues and Challenges. In IEEE Communications Surveys & Tutorials ; IEEE: New York, NY, USA, 2019; Volume 21, pp. 393–430. [ Google Scholar ]
- Yao, Z.; Lum, Y.; Johnston, A.; Mejia-Mendoza, L.M.; Zhou, X.; Wen, Y.; Aspuru-Guzik, A.; Sargent, E.H.; Seh, Z.W. Machine learning for a sustainable energy future. Nat. Rev. Mater. 2023 , 8 , 202–215. [ Google Scholar ] [ CrossRef ]
- Al-Garadi, M.A.; Mohamed, A.; Al-Ali, A.K.; Du, X.; Ali, I.; Guizani, M. A Survey of Machine and Deep Learning Methods for Internet of Things (IoT) Security. In IEEE Communications Surveys & Tutorials ; IEEE: New York, NY, USA, 2020; Volume 22, pp. 1646–1685. [ Google Scholar ]
- Messaoud, S.; Bradai, A.; Bukhari, S.H.R.; Quang, P.T.A.; Ahmed, O.B.; Atri, M. A survey on machine learning in internet of things: Algorithms, strategies, and applications. Internet Things 2020 , 12 , 100314. [ Google Scholar ] [ CrossRef ]
- Umer, M.A.; Junejo, K.N.; Jilani, M.T.; Mathur, A.P. Machine learning for intrusion detection in industrial control systems: Ap-plications, challenges, and recommendations. Int. J. Crit. Infrastruct. Prot. 2022 , 38 , 100516. [ Google Scholar ] [ CrossRef ]
- Von Rueden, L.; Mayer, S.; Garcke, J.; Bauckhage, C.; Schuecker, J. Informed machine learning–towards a taxonomy of explicit integration of knowledge into machine learning. Learning 2019 , 18 , 19–20. [ Google Scholar ]
- Waring, J.; Lindvall, C.; Umeton, R. Automated machine learning: Review of the state-of-the-art and opportunities for healthcare. Artif. Intell. Med. 2020 , 104 , 101822. [ Google Scholar ] [ CrossRef ]
- Wang, H.; Lv, L.; Li, X.; Li, H.; Leng, J.; Zhang, Y.; Thomson, V.; Liu, G.; Wen, X.; Luo, G. A safety management approach for Industry 5.0′ s human-centered manufacturing based on digital twin. J. Manuf. Syst. 2023 , 66 , 1–12. [ Google Scholar ] [ CrossRef ]
- Reuther, A.; Michaleas, P.; Jones, M.; Gadepally, V.; Samsi, S.; Kepner, J. Survey and Benchmarking of Machine Learning Accelerators. In Proceedings of the 2019 IEEE High Performance Extreme Computing Conference (HPEC), Waltham, MA USA, 24–26 September 2019; pp. 1–9. [ Google Scholar ]
- Kaur, B.; Dadkhah, S.; Shoeleh, F.; Neto, E.C.P.; Xiong, P.; Iqbal, S.; Lamontagne, P.; Ray, S.; Ghorbani, A.A. Internet of Things (IoT) security dataset evolution: Challenges and future directions. Internet Things 2023 , 22 , 100780. [ Google Scholar ] [ CrossRef ]
- Paullada, A.; Raji, I.D.; Bender, E.M.; Denton, E.; Hanna, A. Data and its (dis)contents: A survey of dataset development and use in machine learning research. Patterns 2021 , 2 , 100336. [ Google Scholar ] [ CrossRef ] [ PubMed ]
- Slimane, H.O.; Benouadah, S.; Khoei, T.T.; Kaabouch, N. A Light Boosting-based ML Model for Detecting Deceptive Jamming Attacks on UAVs. In Proceedings of the 2022 IEEE 12th Annual Computing and Communication Workshop and Conference (CCWC), Las Vegas, NV, USA, 26–29 January 2022; pp. 328–333. [ Google Scholar ]
- Manesh, M.R.; Kenney, J.; Hu, W.C.; Devabhaktuni, V.K.; Kaabouch, N. Detection of GPS spoofing attacks on unmanned aerial systems. In Proceedings of the 16th IEEE Annual Consumer Communications & Networking Conference (CCNC), Las Vegas, NV, USA, 11–14 January 2019; pp. 1–6. [ Google Scholar ]
- Sharifani, K.; Amini, M. Machine Learning and Deep Learning: A Review of Methods and Applications. World Inf. Technol. Eng. J. 2023 , 10 , 3897–3904. [ Google Scholar ]
- Obaid, H.S.; Dheyab, S.A.; Sabry, S.S. The Impact of Data Pre-Processing Techniques and Dimensionality Reduction on the Ac-curacy of Machine Learning. In Proceedings of the 2019 9th Annual Information Technology, Electromechanical Engineering and Microelectronics Conference (IEMECON), Jaipur, India, 13–15 March 2019; pp. 279–283. [ Google Scholar ]
- Liu, B.; Ding, M.; Shaham, S.; Rahayu, W.; Lin, Z. When machine learning meets privacy: A survey and outlook. ACM Comput. Surv. (CSUR) 2021 , 54 , 1–36. [ Google Scholar ] [ CrossRef ]
- Singh, S.; Gupta, P. Comparative study ID3, cart and C4. 5 decision tree algorithm: A survey. Int. J. Adv. Inf. Sci. Technol. (IJAIST) 2014 , 27 , 97–103. [ Google Scholar ]
- Zhang, M.-L.; Zhou, Z.-H. ML-KNN: A lazy learning approach to multi-label learning. Pattern Recognit. 2007 , 40 , 2038–2048. [ Google Scholar ] [ CrossRef ]
- Musavi, M.T.; Ahmed, W.; Chan, K.H.; Faris, K.B.; Hummels, D.M. On the training of radial basis function classifiers. Neural Netw. 1992 , 5 , 595–603. [ Google Scholar ] [ CrossRef ]
- Zhou, J.; Gandomi, A.H.; Chen, F.; Holzinger, A. Evaluating the Quality of Machine Learning Explanations: A Survey on Methods and Metrics. Electronics 2021 , 10 , 593. [ Google Scholar ] [ CrossRef ]
- Jiang, T.; Fang, H.; Wang, H. Blockchain-Based Internet of Vehicles: Distributed Network Architecture and Performance Analy-sis. IEEE Internet Things J. 2019 , 6 , 4640–4649. [ Google Scholar ] [ CrossRef ]
- Jia, W.; Dai, D.; Xiao, X.; Wu, H. ARNOR: Attention regularization based noise reduction for distant supervision relation classifi-cation. In Proceedings of the 57th Annual Meeting of the Association for Computational Linguistics, Florence, Italy, 28 July–2 August 2019; pp. 1399–1408. [ Google Scholar ]
- Abiodun, O.I.; Omolara, A.E.; Dada, K.V.; Mohamed, N.A.; Arshad, H. State-of-the-art in artificial neural network applications: A survey. Heliyon 2018 , 4 , e00938. [ Google Scholar ] [ CrossRef ]
- Izeboudjen, N.; Larbes, C.; Farah, A. A new classification approach for neural networks hardware: From standards chips to embedded systems on chip. Artif. Intell. Rev. 2014 , 41 , 491–534. [ Google Scholar ] [ CrossRef ]
- Wang, D.; He, H.; Liu, D. Intelligent Optimal Control With Critic Learning for a Nonlinear Overhead Crane System. IEEE Trans. Ind. Informatics 2018 , 14 , 2932–2940. [ Google Scholar ] [ CrossRef ]
- Wang, S.-C. Artificial Neural Network. In Interdisciplinary Computing in Java Programming ; Springer: Berlin/Heidelberg, Germany, 2003; pp. 81–100. [ Google Scholar ]
- Albawi, S.; Mohammed, T.A.; Al-Zawi, S. Understanding of a convolutional neural network. In Proceedings of the International Conference on Engineering and Technology (ICET), Antalya, Turkey, 21–23 August 2017. [ Google Scholar ]
- Khoei, T.T.; Slimane, H.O.; Kaabouch, N. Cyber-Security of Smart Grids: Attacks, Detection, Countermeasure Techniques, and Future Directions. Commun. Netw. 2022 , 14 , 119–170. [ Google Scholar ] [ CrossRef ]
- Gunturi, S.K.; Sarkar, D. Ensemble machine learning models for the detection of energy theft. Electr. Power Syst. Res. 2021 , 192 , 106904. [ Google Scholar ] [ CrossRef ]
- Chafii, M.; Bader, F.; Palicot, J. Enhancing coverage in narrow band-IoT using machine learning. In Proceedings of the 2018 IEEE Wireless Communications and Networking Conference (WCNC), Barcelona, Spain, 15–18 April 2018; pp. 1–6. [ Google Scholar ]
- Bithas, P.S.; Michailidis, E.T.; Nomikos, N.; Vouyioukas, D.; Kanatas, A.G. A Survey on Machine-Learning Techniques for UAV-Based Communications. Sensors 2019 , 19 , 5170. [ Google Scholar ] [ CrossRef ] [ PubMed ]
- Benos, L.; Tagarakis, A.C.; Dolias, G.; Berruto, R.; Kateris, D.; Bochtis, D. Machine Learning in Agriculture: A Comprehensive Updated Review. Sensors 2021 , 21 , 3758. [ Google Scholar ] [ CrossRef ]
- Wagle, P.P.; Rani, S.; Kowligi, S.B.; Suman, B.H.; Pramodh, B.; Kumar, P.; Raghavan, S.; Shastry, K.A.; Sanjay, H.A.; Kumar, M.; et al. Machine Learning-Based Ensemble Network Security System. In Recent Advances in Artificial Intelligence and Data Engineering ; Springer: Berlin/Heidelberg, Germany, 2022; pp. 3–15. [ Google Scholar ]
- Sutton, C.D. Classification and regression trees, bagging, and boosting. Handb. Stat. 2005 , 24 , 303–329. [ Google Scholar ]
- Zaadnoordijk, L.; Besold, T.R.T.; Cusack, R. Lessons from infant learning for unsupervised machine learning. Nat. Mach. Intell. 2022 , 4 , 510–520. [ Google Scholar ] [ CrossRef ]
- Khoei, T.T.; Kaabouch, N. A Comparative Analysis of Supervised and Unsupervised Models for Detecting Attacks on the Intrusion Detection Systems. Information 2023 , 14 , 103. [ Google Scholar ] [ CrossRef ]
- Kumar, P.; Gupta, G.P.; Tripathi, R. An ensemble learning and fog-cloud architecture-driven cyber-attack detection framework for IoMT networks. Comput. Commun. 2021 , 166 , 110–124. [ Google Scholar ] [ CrossRef ]
- Hady, M.; Abdel, A.M.F.; Schwenker, F. Semi-supervised learning. In Handbook on Neural Information Processing ; Springer: Berlin/Heidelberg, Germany, 2013. [ Google Scholar ]
- Elsken, T.; Metzen, J.H.; Hutter, F. Neural architecture search: A survey. J. Mach. Learn. Res. 2019 , 20 , 1–21. [ Google Scholar ]
- Luo, Y.; Zhu, J.; Li, M.; Ren, Y.; Zhang, B. Smooth neighbors on teacher graphs for semi-supervised learning. In Proceedings of the IEEE Conference on Computer Vision and Pattern Recognition, Lake City, UT, USA, 18–22 June 2018; pp. 8896–8905. [ Google Scholar ]
- Park, S.; Park, J.; Shin, S.; Moon, I. Adversarial dropout for supervised and semi-supervised learning. In Proceedings of the Thirty-Second AAAI Conference on Artificial Intelligence, New Orleans, LA, USA, 2–7 February 2018; pp. 3917–3924. [ Google Scholar ]
- Khoei, T.T.; Kaabouch, N. ACapsule Q-learning based reinforcement model for intrusion detection system on smart grid. In Proceedings of the IEEE International Conference on Electro Information Technology (eIT), Romeoville, IL, USA, 18–20 May 2023; pp. 333–339. [ Google Scholar ]
- Polydoros, A.S.; Nalpantidis, L. Survey of model-based reinforcement learning: Applications on robotics. J. Intell. Robot. Syst. 2017 , 86 , 153–173. [ Google Scholar ] [ CrossRef ]
- Degris, T.; Pilarski, P.M.; Sutton, R.S. Model-Free reinforcement learning with continuous action in practice. In Proceedings of the 2012 American Control Conference (ACC), Montreal, QC, Canada, 27–29 June 2012; pp. 2177–2182. [ Google Scholar ] [ CrossRef ]
- Cao, D.; Hu, W.; Zhao, J.; Zhang, G.; Zhang, B.; Liu, Z.; Chen, Z.; Blaabjerg, F. Reinforcement learning and its applications in modern power and energy systems: A review. J. Mod. Power Syst. Clean Energy 2020 , 8 , 1029–1042. [ Google Scholar ] [ CrossRef ]
- Zhang, J.M.; Harman, M.; Ma, L.; Liu, Y. Machine Learning Testing: Survey, Landscapes and Horizons. In IEEE Transactions on Software Engineering ; IEEE: New York, NY, USA, 2022; Volume 48, pp. 1–36. [ Google Scholar ]
- Salahdine, F.; Kaabouch, N. Security threats, detection, and countermeasures for physical layer in cognitive radio networks: A survey. Phys. Commun. 2020 , 39 , 101001. [ Google Scholar ] [ CrossRef ]
- Ramírez, J.; Yu, W.; Perrusquía, A. Model-free reinforcement learning from expert demonstrations: A survey. Artif. Intell. Rev. 2022 , 55 , 3213–3241. [ Google Scholar ] [ CrossRef ]
- Yang, L.; Shami, A. On hyperparameter optimization of machine learning algorithms: Theory and practice. Neurocomputing 2020 , 415 , 295–316. [ Google Scholar ] [ CrossRef ]
- Dev, K.; Maddikunta, P.K.R.; Gadekallu, T.R.; Bhattacharya, S.; Hegde, P.; Singh, S. Energy Optimization for Green Communication in IoT Using Harris Hawks Optimization. In IEEE Transactions on Green Communications and Networking ; IEEE: New York, NY, USA, 2022; Volume 6, pp. 685–694. [ Google Scholar ]
- Khodadadi, N.; Snasel, V.; Mirjalili, S. Dynamic Arithmetic Optimization Algorithm for Truss Optimization Under Natural Fre-quency Constraints. IEEE Access 2022 , 10 , 16188–16208. [ Google Scholar ] [ CrossRef ]
- Cummins, C.; Wasti, B.; Guo, J.; Cui, B.; Ansel, J.; Gomez, S.; Jain, S.; Liu, J.; Teytaud, O.; Steinerm, B.; et al. CompilerGym: Robust, Performant Compiler Optimization Environments for AI Research. In Proceedings of the 2022 IEEE/ACM In-ternational Symposium on Code Generation and Optimization (CGO), Seoul, Republic of Korea, 2–6 April 2022; pp. 92–105. [ Google Scholar ]
- Zhang, W.; Gu, X.; Tang, L.; Yin, Y.; Liu, D.; Zhang, Y. Application of machine learning, deep learning and optimization algo-rithms in geoengineering and geoscience: Comprehensive review and future challenge. Gondwana Res. 2022 , 109 , 1–17. [ Google Scholar ] [ CrossRef ]
- Mittal, S.; Vaishay, S. A survey of techniques for optimizing deep learning on GPUs. J. Syst. Arch. 2019 , 99 , 101635. [ Google Scholar ] [ CrossRef ]
- Zhang, Q.; Yang, L.T.; Chen, Z.; Li, P. A survey on deep learning for big data. Inf. Fusion 2018 , 42 , 146–157. [ Google Scholar ] [ CrossRef ]
- Oyelade, O.N.; Ezugwu, A.E.-S.; Mohamed, T.I.A.; Abualigah, L. Ebola Optimization Search Algorithm: A New Nature-Inspired Metaheuristic Optimization Algorithm. IEEE Access 2022 , 10 , 16150–16177. [ Google Scholar ] [ CrossRef ]
- Blank, J.; Deb, K. Pymoo: Multi-Objective Optimization in Python. IEEE Access 2020 , 8 , 89497–89509. [ Google Scholar ] [ CrossRef ]
- Qiao, K.; Yu, K.; Qu, B.; Liang, J.; Song, H.; Yue, C. An Evolutionary Multitasking Optimization Framework for Constrained Multi-objective Optimization Problems. IEEE Trans. Evol. Comput. 2022 , 26 , 263–277. [ Google Scholar ] [ CrossRef ]
- Riaz, M.; Ahmad, S.; Hussain, I.; Naeem, M.; Mihet-Popa, L. Probabilistic Optimization Techniques in Smart Power System. Energies 2022 , 15 , 825. [ Google Scholar ] [ CrossRef ]
- Yu, T.; Zhu, H. Hyper-parameter optimization: A review of algorithms and applications. arXiv 2020 , arXiv:2003.05689. [ Google Scholar ]
- Yang, X.; Song, Z.; King, I.; Xu, Z. A Survey on deep semi-supervised learning. arXiv 2021 , arXiv:2103.00550. [ Google Scholar ] [ CrossRef ]
- Gibson, B.R.; Rogers, T.T.; Zhu, X. Human semi-supervised learning. Top. Cogn. Sci. 2013 , 5 , 132–172. [ Google Scholar ] [ CrossRef ]
- Nguyen, T.T.; Nguyen, N.D.; Nahavandi, S. Deep Reinforcement Learning for Multiagent Systems: A Review of Challenges, Solutions, and Applications. IEEE Trans. Cybern. 2020 , 50 , 3826–3839. [ Google Scholar ] [ CrossRef ]
- Canese, L.; Cardarilli, G.C.; Di Nunzio, L.; Fazzolari, R.; Giardino, D.; Re, M.; Spanò, S. Multi-Agent Reinforcement Learning: A Review of Challenges and Applications. Appl. Sci. 2021 , 11 , 4948. [ Google Scholar ] [ CrossRef ]
- Du, W.; Ding, S. A survey on multi-agent deep reinforcement learning: From the perspective of challenges and applications. Artif. Intell. Rev. 2020 , 54 , 3215–3238. [ Google Scholar ] [ CrossRef ]
- Salwan, D.; Kant, S.; Pareek, H.; Sharma, R. Challenges with reinforcement learning in prosthesis. Mater. Today Proc. 2022 , 49 , 3133–3136. [ Google Scholar ] [ CrossRef ]
- Narkhede, M.S.; Chatterji, S.; Ghosh, S. Trends and challenges in optimization techniques for operation and control of Mi-crogrid—A review. In Proceedings of the 2012 1st International Conference on Power and Energy in NERIST (ICPEN), Nirjuli, India, 28–29 December 2012; pp. 1–7. [ Google Scholar ]
- Khoei, T.T.; Ismail, S.; Kaabouch, N. Dynamic Selection Techniques for Detecting GPS Spoofing Attacks on UAVs. Sensors 2022 , 22 , 662. [ Google Scholar ] [ CrossRef ]
- Khoei, T.T.; Ismail, S.; Al Shamaileh, K.; Devabhaktuni, V.K.; Kaabouch, N. Impact of Dataset and Model Parameters on Machine Learning Performance for the Detection of GPS Spoofing Attacks on Unmanned Aerial Vehicles. Appl. Sci. 2022 , 13 , 383. [ Google Scholar ] [ CrossRef ]
- Khoei, T.T.; Kaabouch, N. Densely Connected Neural Networks for Detecting Denial of Service Attacks on Smart Grid Network. In Proceedings of the IEEE 13th Annual Ubiquitous Computing, Electronics & Mobile Communication Conference (UEMCON), New York, NY, USA, 26–29 October 2022; pp. 0207–0211. [ Google Scholar ]
- Khan, A.; Khan, S.H.; Saif, M.; Batool, A.; Sohail, A.; Khan, M.W. A Survey of Deep Learning Techniques for the Analysis of COVID-19 and their usability for Detecting Omicron. J. Exp. Theor. Artif. Intell. 2023 , 1–43. [ Google Scholar ] [ CrossRef ]
- Gopinath, M.; Sethuraman, S.C. A comprehensive survey on deep learning based malware detection techniques. Comput. Sci. Rev. 2023 , 47 , 100529. [ Google Scholar ]
- Gheisari, M.; Ebrahimzadeh, F.; Rahimi, M.; Moazzamigodarzi, M.; Liu, Y.; Pramanik, P.K.D.; Heravi, M.A.; Mehbodniya, A.; Ghaderzadeh, M.; Feylizadeh, M.R.; et al. Deep learning: Applications, architectures, models, tools, and frameworks: A com-prehensive survey. In CAAI Transactions on Intelligence Technology ; IET: Stevenage, UK, 2023. [ Google Scholar ]
- Morgan, D.; Jacobs, R. Opportunities and challenges for machine learning in materials science. Annu. Rev. Mater. Res. 2020 , 50 , 71–103. [ Google Scholar ] [ CrossRef ]
- Phoon, K.K.; Zhang, W. Future of machine learning in geotechnics. Georisk Assess. Manag. Risk Eng. Syst. Geohazards 2023 , 17 , 7–22. [ Google Scholar ] [ CrossRef ]
- Krishnam, N.P.; Ashraf, M.S.; Rajagopal, B.R.; Vats, P.; Chakravarthy, D.S.K.; Rafi, S.M. Analysis of Current Trends, Advances and Challenges of Machine Learning (Ml) and Knowledge Extraction: From Ml to Explainable AI. Ind. Qualif.-Stitute Adm. Manag. UK 2022 , 58 , 54–62. [ Google Scholar ]
- Li, Z.; Yoon, J.; Zhang, R.; Rajabipour, F.; Srubar, W.V., III; Dabo, I.; Radlińska, A. Machine learning in concrete science: Applications, challenges, and best practices. NPJ Comput. Mater. 2022 , 8 , 127. [ Google Scholar ] [ CrossRef ]
- Houssein, E.H.; Abohashima, Z.; Elhoseny, M.; Mohamed, W.M. Machine learning in the quantum realm: The state-of-the-art, challenges, and future vision. Expert Syst. Appl. 2022 , 194 , 116512. [ Google Scholar ] [ CrossRef ]
- Khan, T.; Tian, W.; Zhou, G.; Ilager, S.; Gong, M.; Buyya, R. Machine learning (ML)-centric resource management in cloud computing: A review and future directions. J. Netw. Comput. Appl. 2022 , 204 , 103405. [ Google Scholar ] [ CrossRef ]
- Esterhuizen, J.A.; Goldsmith, B.R.; Linic, S. Interpretable machine learning for knowledge generation in heterogeneous catalysis. Nat. Catal. 2022 , 5 , 175–184. [ Google Scholar ] [ CrossRef ]
- Bharadiya, J.P. Leveraging Machine Learning for Enhanced Business Intelligence. Int. J. Comput. Sci. Technol. 2023 , 7 , 1–19. [ Google Scholar ]
- Talaei Khoei, T.; Ould Slimane, H.; Kaabouch, N. Deep learning: Systematic review, models, challenges, and research directions. In Neural Computing and Applications ; Springer: Berlin/Heidelberg, Germany, 2023; pp. 1–22. [ Google Scholar ]
- Ben Amor, S.; Belaid, F.; Benkraiem, R.; Ramdani, B.; Guesmi, K. Multi-criteria classification, sorting, and clustering: A bibliometric review and research agenda. Ann. Oper. Res. 2023 , 325 , 771–793. [ Google Scholar ] [ CrossRef ]
- Valdez, F.; Melin, P. A review on quantum computing and deep learning algorithms and their applications. Soft Comput. 2023 , 27 , 13217–13236. [ Google Scholar ] [ CrossRef ]
- Fihri, W.F.; Arjoune, Y.; Hassan El Ghazi, H.; Kaabouch, N.; Abou El Majd, A.B. A particle swarm optimization based algorithm for primary user emulation attack detection. In Proceedings of the 2018 IEEE 8th Annual Computing and Communication Workshop and Conference (CCWC), Las Vegas, NV, USA, 8–10 January 2018; pp. 823–827. [ Google Scholar ]
Click here to enlarge figure
Classification Category | Characteristics | Advantages | Disadvantages |
---|---|---|---|
Bayesian- Based | |||
Tree- based | |||
Instance- based | |||
Regularization-based | |||
Neural network-based | |||
Ensemble-based |
Classification Category | Characteristics | Advantage | Disadvantage |
---|---|---|---|
Inductive- Based | Generates a model that can create predictions for any sample in the input space | The predictions of new samples are independent of old samples | The same model can be used in training and predicting new data samples |
Transductive- based | Predictive strengths are limited to objects that are processed during the training steps | No difference between the training and testing steps | No distinction between the transductive algorithms in a supervised manner |
Classification Category | Characteristics | Advantages | Disadvantages |
---|---|---|---|
Cluster-based | Divides uncategorized data into similar groups; | ||
Dimensionality reduction-based | Decreases the number of features in the given dataset; | ||
Neural network-based | Inspiration of human brains. |
Classification Category | Characteristics | Advantage | Disadvantage |
---|---|---|---|
Model-based | Optimal actions are learned via a model | ||
Model free-based | No transition of a probability distribution or reward associated with the Markov decision process |
Data Preprocessing Steps | Methodology | Technique | Highlights |
---|---|---|---|
Data transformation | Standardization and normalization | Unit vector normalization | Extract the given data, and convert them to a usable format |
Max abs scalar | |||
Quantile transformer scalar | |||
Robust scalar Min-max scaling | |||
Power transformer scalar | |||
Unit vector normalization | |||
Standard scalar | |||
Data cleaning | Missing value imputation | Complete case analysis | Loss of efficiency, strong bias, and complications in handling data. |
Frequent category imputation | |||
Mean/median imputation | |||
Mode imputation | |||
End of tail imputation | |||
Nearest neighbor imputation | |||
Iterative imputation | |||
Hot and cold deck imputation | |||
Exploration imputation | |||
Interpolation imputation | |||
Regression-based imputation | |||
Noise treatment | Data polishing | ||
Noise filters | |||
Data reduction/ increasing | Feature selection | Wrapper | Decrease or increase the number of samples or features that are not important in the process of training |
Filter | |||
Embedded | |||
Feature extraction | Principle component analysis | ||
Linear discriminative analysis | |||
Independent component analysis | |||
Partial least square | |||
Multifactor dimensionality reduction | |||
Nonlinear dimensionality reduction | |||
Autoencoder | |||
Tensor decomposition | |||
Instance generation | Condensation algorithms | ||
Edition algorithms | |||
Hybrid algorithms | |||
Discretization | Discretization-based | Chi-squared discretization | Loss of information, simplicity, readability, and faster learning process |
Efficient discretization | |||
Imbalanced learning | Under-sampling | Random under-sampling | Presents true evaluation results |
Tomek links | |||
Condensed nearest neighbor | |||
Edited nearest neighbor | |||
Near-miss under-sampling | |||
Oversampling | Random oversampling | ||
Synthetic minority oversampling technique | |||
Adaptive synthetic | |||
Borderline-synthetic minority oversampling technique |
Hyperparameter Methods | Strengths | Limitations |
---|---|---|
Grid search | ||
Random search | ||
Genetic algorithm | ||
Gradient-based techniques | ||
Bayesian optimization-Gaussian process | ||
Particle swarm optimization | ||
Bayesian optimization-tree structure parzen estimator | ||
Hyperband | ||
Bayesian optimization-SMAC | ||
Population-based |
Category | Metric Name |
---|---|
Supervised Learning | |
Unsupervised Learning | |
Semi-Supervised Learning | |
Reinforcement Learning |
Challenges | Descriptions |
---|---|
Interpretability and Explain-ability | |
Bias and Fairness | |
Adversarial Robustness | |
Privacy and Security | |
Reinforcement Learning | |
Quantum Computing | |
Multi-Criteria Models |
The statements, opinions and data contained in all publications are solely those of the individual author(s) and contributor(s) and not of MDPI and/or the editor(s). MDPI and/or the editor(s) disclaim responsibility for any injury to people or property resulting from any ideas, methods, instructions or products referred to in the content. |
Share and Cite
Talaei Khoei, T.; Kaabouch, N. Machine Learning: Models, Challenges, and Research Directions. Future Internet 2023 , 15 , 332. https://doi.org/10.3390/fi15100332
Talaei Khoei T, Kaabouch N. Machine Learning: Models, Challenges, and Research Directions. Future Internet . 2023; 15(10):332. https://doi.org/10.3390/fi15100332
Talaei Khoei, Tala, and Naima Kaabouch. 2023. "Machine Learning: Models, Challenges, and Research Directions" Future Internet 15, no. 10: 332. https://doi.org/10.3390/fi15100332
Article Metrics
Article access statistics, further information, mdpi initiatives, follow mdpi.
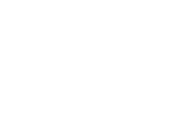
Subscribe to receive issue release notifications and newsletters from MDPI journals

- Conferences
- Last updated November 18, 2021
- In AI Origins & Evolution
Top Machine Learning Research Papers Released In 2021
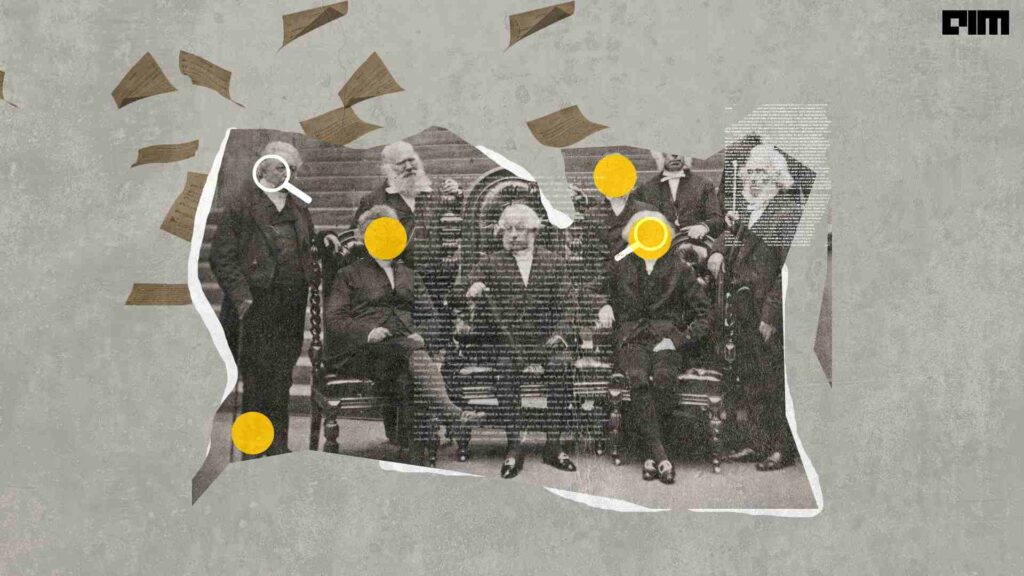
- Published on November 18, 2021
- by Dr. Nivash Jeevanandam
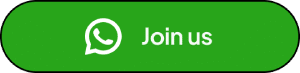
Advances in machine learning and deep learning research are reshaping our technology. Machine learning and deep learning have accomplished various astounding feats this year in 2021, and key research articles have resulted in technical advances used by billions of people. The research in this sector is advancing at a breakneck pace and assisting you to keep up. Here is a collection of the most important recent scientific study papers.
Rebooting ACGAN: Auxiliary Classifier GANs with Stable Training
The authors of this work examined why ACGAN training becomes unstable as the number of classes in the dataset grows. The researchers revealed that the unstable training occurs due to a gradient explosion problem caused by the unboundedness of the input feature vectors and the classifier’s poor classification capabilities during the early training stage. The researchers presented the Data-to-Data Cross-Entropy loss (D2D-CE) and the Rebooted Auxiliary Classifier Generative Adversarial Network to alleviate the instability and reinforce ACGAN (ReACGAN). Additionally, extensive tests of ReACGAN demonstrate that it is resistant to hyperparameter selection and is compatible with a variety of architectures and differentiable augmentations.
This article is ranked #1 on CIFAR-10 for Conditional Image Generation.
For the research paper, read here .
For code, see here .

Dense Unsupervised Learning for Video Segmentation
The authors presented a straightforward and computationally fast unsupervised strategy for learning dense spacetime representations from unlabeled films in this study. The approach demonstrates rapid convergence of training and a high degree of data efficiency. Furthermore, the researchers obtain VOS accuracy superior to previous results despite employing a fraction of the previously necessary training data. The researchers acknowledge that the research findings may be utilised maliciously, such as for unlawful surveillance, and that they are excited to investigate how this skill might be used to better learn a broader spectrum of invariances by exploiting larger temporal windows in movies with complex (ego-)motion, which is more prone to disocclusions.
This study is ranked #1 on DAVIS 2017 for Unsupervised Video Object Segmentation (val).
Temporally-Consistent Surface Reconstruction using Metrically-Consistent Atlases
The authors offer an atlas-based technique for producing unsupervised temporally consistent surface reconstructions by requiring a point on the canonical shape representation to translate to metrically consistent 3D locations on the reconstructed surfaces. Finally, the researchers envisage a plethora of potential applications for the method. For example, by substituting an image-based loss for the Chamfer distance, one may apply the method to RGB video sequences, which the researchers feel will spur development in video-based 3D reconstruction.
This article is ranked #1 on ANIM in the category of Surface Reconstruction.
EdgeFlow: Achieving Practical Interactive Segmentation with Edge-Guided Flow
The researchers propose a revolutionary interactive architecture called EdgeFlow that uses user interaction data without resorting to post-processing or iterative optimisation. The suggested technique achieves state-of-the-art performance on common benchmarks due to its coarse-to-fine network design. Additionally, the researchers create an effective interactive segmentation tool that enables the user to improve the segmentation result through flexible options incrementally.
This paper is ranked #1 on Interactive Segmentation on PASCAL VOC
Learning Transferable Visual Models From Natural Language Supervision
The authors of this work examined whether it is possible to transfer the success of task-agnostic web-scale pre-training in natural language processing to another domain. The findings indicate that adopting this formula resulted in the emergence of similar behaviours in the field of computer vision, and the authors examine the social ramifications of this line of research. CLIP models learn to accomplish a range of tasks during pre-training to optimise their training objective. Using natural language prompting, CLIP can then use this task learning to enable zero-shot transfer to many existing datasets. When applied at a large scale, this technique can compete with task-specific supervised models, while there is still much space for improvement.
This research is ranked #1 on Zero-Shot Transfer Image Classification on SUN
CoAtNet: Marrying Convolution and Attention for All Data Sizes
The researchers in this article conduct a thorough examination of the features of convolutions and transformers, resulting in a principled approach for combining them into a new family of models dubbed CoAtNet. Extensive experiments demonstrate that CoAtNet combines the advantages of ConvNets and Transformers, achieving state-of-the-art performance across a range of data sizes and compute budgets. Take note that this article is currently concentrating on ImageNet classification for model construction. However, the researchers believe their approach is relevant to a broader range of applications, such as object detection and semantic segmentation.
This paper is ranked #1 on Image Classification on ImageNet (using extra training data).
SwinIR: Image Restoration Using Swin Transformer
The authors of this article suggest the SwinIR image restoration model, which is based on the Swin Transformer . The model comprises three modules: shallow feature extraction, deep feature extraction, and human-recognition reconstruction. For deep feature extraction, the researchers employ a stack of residual Swin Transformer blocks (RSTB), each formed of Swin Transformer layers, a convolution layer, and a residual connection.
This research article is ranked #1 on Image Super-Resolution on Manga109 – 4x upscaling.
📣 Want to advertise in AIM? Book here

Dr. Nivash Jeevanandam
- Classification , Deep Learning , Generative Adversarial Network , gradient boosting , Machine Learning , RGB , supervised learning , Unsupervised Learning

Companies like OpenAI, Google, Mistral, and Anthropic have publicly stated that they red team their systems and use external contractors, including jailbreakers, to undertake pentesting.

Top Editorial Picks
Redis 8 Launches with AI Capabilities, Expands Developer Access Siddharth Jindal
Salesforce Launches Two New AI Sales Agents: Einstein SDR and Einstein Sales Coach Siddharth Jindal
Chevron Invests $1 Billion in Bengaluru for Largest Global Innovation Hub Vidyashree Srinivas
Revrag AI Raises $600K to Transform B2B Sales with AI Agents Pritam Bordoloi
Bill Gates Turns to Computer Vision to Eradicate Malaria Siddharth Jindal
Subscribe to The Belamy: Our Weekly Newsletter
Biggest ai stories, delivered to your inbox every week., "> "> flagship events.
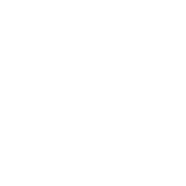
Discover how Cypher 2024 expands to the USA, bridging AI innovation gaps and tackling the challenges of enterprise AI adoption
© Analytics India Magazine Pvt Ltd & AIM Media House LLC 2024
- Terms of use
- Privacy Policy

Thank you for visiting nature.com. You are using a browser version with limited support for CSS. To obtain the best experience, we recommend you use a more up to date browser (or turn off compatibility mode in Internet Explorer). In the meantime, to ensure continued support, we are displaying the site without styles and JavaScript.
- View all journals
- Explore content
- About the journal
- Publish with us
- Sign up for alerts
- Open access
- Published: 21 August 2024
A comparison between machine and deep learning models on high stationarity data
- Domenico Santoro ORCID: orcid.org/0000-0001-9505-0673 1 na1 ,
- Tiziana Ciano 2 , 3 na1 &
- Massimiliano Ferrara 3 , 4 na1
Scientific Reports volume 14 , Article number: 19409 ( 2024 ) Cite this article
Metrics details
- Computational science
Advances in sensor, computing, and communication technologies are enabling big data analytics by providing time series data. However, conventional models struggle to identify sequence features and forecast accuracy. This paper investigates time series features and shows that some machine learning algorithms can outperform deep learning models. In particular, the problem analyzed concerned predicting the number of vehicles passing through an Italian tollbooth in 2021. The dataset, composed of 8766 rows and 6 columns relating to additional tollbooths, proved to have high stationarity and was treated through machine learning methods such as support vector machine, random forest, and eXtreme gradient boosting (XGBoost), as well as deep learning through recurrent neural networks with long short-term memory (RNN-LSTM) cells. From the comparison of these models, the prediction through the XGBoost algorithm outperforms competing algorithms, particularly in terms of MAE and MSE. The result highlights how a shallower algorithm than a neural network is, in this case, able to obtain a better adaptation to the time series instead of a much deeper model that tends to develop a smoother prediction.
Similar content being viewed by others
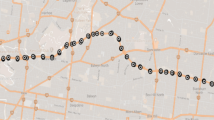
Development and evaluation of bidirectional LSTM freeway traffic forecasting models using simulation data
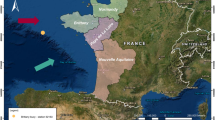
Harnessing LSTM and XGBoost algorithms for storm prediction
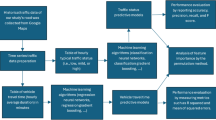
Predicting vehicle travel time on city streets for trip preplanning and predicting heavy traffic for proactive control of street congestion
Introduction.
Recent advances in sensor, computing, and communication technologies are primary sources that are rich in providing time series data. Some technical evidence in this direction also arises in decision sciences and economics, particularly in mathematical finance. These advances transform how complex real-world systems are monitored and controlled 1 , 2 . Time series forecasting is one of the most critical aspects of big data analytics. However, conventional time series forecasting models cannot effectively identify appropriate sequence features, often leading to a lack of forecast accuracy. Time series are generated chronologically and have high dimensionality and temporal dependence. High dimensionality allows for more information about the behavior of the series, but generally, for analysis, it is crucial to consider each time point as one dimension. Instead, temporal dependencies mean that even two numerically identical points can belong to different classes or predict different behaviors. Time series can be divided into single-variable time series and multi-variable time series, secondary to the notice of the number of sampling variables at a given point in time. These combined characteristics make accurate time series prediction very difficult. Time series are statistical recordings of stochastic processes over time, focusing on discrete, equally spaced observations. They have temporal dependence, where the distribution of an observation depends on previous values and are typically analyzed over all non-negative integers. “Stationarity” is a crucial concept in time series, indicating that a series’ behavior remains constant over time, despite variations. Stationary series have a well-understood theory and are fundamental to studying time series, although many non-stationary ones are related. Stationarity is an invariant property that means statistical characteristics of a time series remain consistent over time. While it may not be plausible over long periods, it is often assumed in statistical analysis of time series over shorter intervals. There are two definitions of stationarity: weak stationarity, which only considers the covariance of a process, and strict stationarity, which assumes distributions remain invariant over time. Numerous approaches to the prediction of temporal series have been proposed in the literature, including the autoregressive approach 3 , 4 , the autoregressive approach of integrated mobile media 5 , 6 , the support vector machine approach 7 , 8 , and neural network-based approaches 9 , 10 , 11 . Various hybrid approaches have been proposed 12 , 13 , 14 , 15 . Deep learning is a new approach that combines non-linear neural networks to obtain a multi-dimensional representation of original input 16 , 17 . It can learn the functionalities of input data, improving accuracy in non-linear and non-static datasets. The use of neural networks in predicting time series has become increasingly frequent thanks to the ever-increasing computational capacity and advanced techniques. Specifically, neural networks based on long short-term memory (LSTM) architecture have become state-of-the-art in the prediction literature thanks to the memory effect. For example, Varnousfaderan and Shibab 18 use different types of LSTM-based networks to predict bird movement for flight planning to minimize collisions, highlighting how the ability to learn long-order dependence in sequence prediction problems allows for very accurate predictions. Sen et al. 19 use neural networks in financial markets to predict asset prices and build an efficient portfolio, demonstrating how LSTM cells are optimal even in the presence of financial data. Zdravković et al. 20 compare different types of LSTM neural networks to predict the fluid temperature in the district heating systems (DHS) supply line, demonstrating how, after an accurate transformation of the dataset, these neural networks can obtain very high prediction accuracy values. Baesmat et al. 21 develop a hybrid approach for prediction in power system operations by combining neural networks with Artificial Bee Colony (ABC) algorithms, thanks to which they can improve network learning procedures and obtain superior results compared to classical models. At the same time, Baesmat and Shiri 22 demonstrate that a curve-fitting approach can outperform the previous neural network-based method. Or Wen and Li 23 , that improve the predictive capabilities of the LSTM through the Attention mechanism in a particular model called LSTM-attention-LSTM based on encoder-decoder architecture, demonstrating how the latter is more accurate than many vanilla models in the prediction task.
In this paper, we will deeply investigate some time series features, considering some endogenous mathematical aspects that arose from the observations related to a class of big data from a certain library. We will show that implementing some machine learning (ML) algorithms will be more effective concerning a more robust model, as LSTM is usually determined with this issue. For example, Abbasimher et al. 24 propose using an XGBoost regressor on two renewable energy consumption datasets through a two-stage forecasting framework and comparing this algorithm with the main deep learning models. From this analysis, the authors highlight how the XGBoost regressor outperforms its competitors. Alipour and Charandabi 25 use the XGBoost classifier in combination with NLP models to improve price movement prediction, demonstrating how this combination is optimal. Ghasemi and Naser 26 use some ML algorithms such as XGBoost and random forest to predict compressive strength properties for 3D printed concrete mixes, highlighting how these two algorithms obtain excellent results and allow the identification of the most significant features. Qiu and Wang 27 use the K-Means algorithm to perform customer segmentation of customers in the credit card industry, demonstrating how non-complex clustering algorithms can produce excellent results. Additional ML methods, such as Compressed Sensing, are used to study wireless communications in Industrial Internet-of-Things (IIoT) devices 28 , 29 , or Bayesian Learning-based algorithms for channel estimation 30 .
In several cases, however, the XGBoost algorithm has been directly compared with LSTM-based neural networks for the prediction task. For example, Frifra et al. 31 propose a comparison between LSTM and XGBoost to predict storm characteristics and occurrence in Western France, highlighting how, in their case, XGBoost is more accurate than LSTM networks. Hu et al. 32 compare the XGBoost algorithm with RNN-LSTM for predicting wind waves, which require more usability than numerical methods inspired by land physics. From the comparison, it is clear that XGBoost generally performs better than RNN-LSTM. Tehranian 33 compares different ML algorithms, such as random forest, XGBoost, probit, and neural networks, in predicting economic recessions by exploiting macroeconomic indicators and market sentiment, highlighting how ML algorithms are the most accurate. Fan et al. 34 analyzing cooling load predictions by comparing ML algorithms with neural networks, highlighting how non-linear models obtain lower performance than XGBoost, although requiring more time for computation. Or Wei et al. 35 , which compare different models in the prediction of a heating load of a residential district, from which it is clear that the ML models, such as XGBoost and SVR, are the fastest (in training time) and obtain excellent results on a par with those obtained by the LSTM network. Furthermore, the propensity for ML algorithms that require fewer hyperparameters is also significant.
In many cases, it is evident that the non-linearity of neural network-based models underperforms the model’s potential since they often deal with data characterized by stationarity. Some main limitations concern the impossibility of increasing the accuracy beyond a certain threshold. Others, instead, have to do with intrinsic characteristics of the time series. In the latter case, much data is derived from recordings of physical/natural phenomena or related to repeated human activities that appear stationary. So far, many authors have preferred to resort to dataset manipulations to eliminate stationarity, for example, by applying restrictions (where possible) or working with decompositions of the latter to obtain higher accuracy values of DL models. However, it is clear that models characterized by a lower complexity are more accurate in the prediction phase than competitors in this type of time series. The main contributions of this paper are:
The analysis of the vehicle flows dataset from some Italian tollbooths that highlight highly stationary characteristics;
The comparison between RNN-LSTM, XGBoost, SVM, and random forest in the prediction task based on the previous dataset through the best hyperparameters’ combination;
The explainability analysis of the best performing algorithm, XGBoost, through the SHAP framework to highlight which are the most significant features.
Road-map of the paper
This article is structured as follows: Sect. “ Machine and deep learning algorithms ” introduces the machine and deep learning algorithms/models to compare for prediction; Section “ Data description ” presents the data used from tollbooths and the main characteristics; “ Comparison between models ” reports the comparisons between the main hyperparameter combinations of the different algorithms in the prediction task, based on the dataset used and analyzes the explainability of the XGBoost model in terms of feature importance; finally, Sect. “ Conclusions ” concludes the paper with an overview of the work done, some final remarks and its limitations.
Machine and deep learning algorithms
Nowadays, the algorithms and techniques for time series prediction are increasingly “deep” and performing. However, a task of the same type can be performed with different methods, which leads to results that, in most cases, achieve better accuracy with more information. For example, a DL model widely used for the prediction task is neural networks. An artificial neural network (ANN) is a computational model inspired by the human brain, which comprises artificial neurons 36 that perform computations within them. The key feature of ANNs is the ability to learn, i.e. adapting the network parameters to specific data. A first specific type of ANN is the feedforward neural network (FNN), where connections move in a one-way sequence from one node to the next, like in the Perceptron 37 case. On the other hand, ANNs that can be equipped with feedback connections in which training requires different time instants are called recurrent neural networks (RNNs). The unfolding in time process for training makes these types of networks ideal for data sequences. To train an RNN, considering feedback connections, a particular version of the Backpropagation 38 algorithm is used: the backpropagation through time (BPTT), in which the gradients are computed at each time step.
Neural networks suffer from a problem related to the gradient of the loss function to be computed, which leads to the explosion or vanishing of the gradient and can lead to the interruption of training. To prevent this problem, a particular architecture was introduced: the Long-short term memory (LSTM) 39 . This unit uses specific control gates to “decide” which information should be forwarded to the next level. Specifically, the LSTM cell is made up of an input gate , an output gate , and a forget gate . Considering an input \(X_t\) and the previous hidden state \(S_{t-1}\) , a new state \(S_t\) can be described as:
where \(\sigma \) is the sigmoid activation function, i the identify gate, f the forget gate, o the output gate, C the cell state, U the input weight matrix, W the recurrent weight matrix, b the bias, and \(\odot \) represents the Hadamard product. RNN-LSTM represents the newest and most widespread architectures for time series forecasting.
On the other hand, ML algorithms used for prediction are generally more explainable than those of DL’s competitors. A first type of proposed model is support vector machines (SVMs) 40 , initially used for classification, has been extended for the regression task. Specifically, SVM finds the optimum separating hyperplane (OSH) between two classes, and its main objective is to maximize the margin between classes of training samples 41 . Its extension, support vector regression (SVR), also called \(\epsilon \) -SVR, minimize the loss function 42 , 43
under the following constraints:
where w is the weight vector, C is a regularization term, \(\zeta _i, \zeta _i^*\) are slack variables related to prediction error, b is the bias term, \(\phi \) is a map function over the feature space, \(y_i\) is the coefficient vector, and \(\epsilon \) is the error parameter user-defined. In this way, the \(\epsilon \) -SVR finds the linear function that deviates at most \(\epsilon \) from the coefficient vector 43 . To improve the separability of the input data, it is possible to apply a kernel function that adds non-linearity and transports them into a higher dimensional space. An example is represented by the radial basis function (RBF) between two points, \(K(x_1, x_2)\) , defined as:
where \(\sigma \) is an hyperparameter.
A different approach from the previous one is to use decision Trees to carry out classification or regression tasks, as in the random forest 44 (RF) case, an ensemble method that uses many trees. These are generated randomly through a training phase on a random sample with a replacement of the training set ( bagging ) and a restriction of the features. Through this mechanism, random forest is also used to identify the most important features by minimizing the out-of-bag error (OOB), i.e. the error on values not considered in the sampling process. Furthermore, no form of pruning is applied to the trees 45 .
A further evolution in the use of trees is eXtreme Gradient Boosting (XGBoost) 46 , an iterative algorithm implemented in a boosting library. The main algorithm implemented for learning is the sequential creation of regression trees, the classification and regression tree (CART) 47 . The potential of using decision trees lies in dividing alternatives’ space into different subsets based on a measure, a process which, repeated recursively, allows classification rules to be obtained. XGBoost training generates sequential trees to minimize prediction errors 48 , 49 . Specifically, the objective function to minimize can be divided into two components 50 , the error function \(L(\cdot )\) and a regularization term \(\Omega (\cdot )\) :
where \(L(y_i, {\hat{y}}_i)\) is the loss function for i -th tree with prediction \({\hat{y}}_i\) . Instead, the \(\Omega (f)\) is composed:
where T is the number of leaves whose weight is represented by \(\omega \) , \(\gamma \) is a learning rate used for pruning, and \(\lambda \) in a regularization parameter. Identifying the optimal branch of the tree in this algorithm occurs through a greedy method, with which the candidate with the highest probability is searched for and continues on that path. Unlike LSTM, XGBoost enjoys much higher explainability due to the classifier’s “simplicity” of the decisions obtainable at each level and its high generalizability and computational speed. A popular framework to further improve the explainability of this algorithm (and, in general, machine learning algorithms) is SHapley Additive exPlanations (SHAP) 51 . Specifically, SHAP allows explaining each feature’s contribution to the model used. This framework is based on a Game Theory approach that measures each player’s contribution in a cooperative game, the Shapley value. For a feature \(x_j\) , the Shapley value is given by 52 :
where p is the number of features in total feature set Y , \(X \subseteq Y\setminus \{j\}\) is the set of features combinations without the j -th, and f ( X ), f ( Y ) are the model prediction in different feature sets. A variant for tree-based algorithms is TreeSHAP 53 , which is computationally less expensive than the basic framework.
Data description
The prediction tests with LSTM and XGBoost were carried out on a dataset relating to the number of vehicles passing through 5 Italian tollbooths on different days. Sequential numbering indicates the “interest” for each of them, linked, for example, to geographical factors. In this sense, Tollbooth 1 is of greater interest than Tollbooth 5 and is the subject of the prediction task. Specifically, the dataset used represents a restriction of the originally collected data, which included a series of additional variables linked to climatic conditions and extended over a longer period. The original dataset, weighing over 250 MB, was reduced to the current version (around 100 MB), containing the hourly data of the vehicles passing through the tollbooths from 1/1/2021 to 12/31/2021 (in US format). For more information related to the Data, see the Acknowledgment at the end of the present work. The dataset comprises 8766 rows and 6 features related to the registration time and the 5 most relevant tollbooths, as shown in Table 1 . Figure 1 contains a plot of the different tollbooths, differentiated by color, while Table 2 presents some statistics of this dataset.
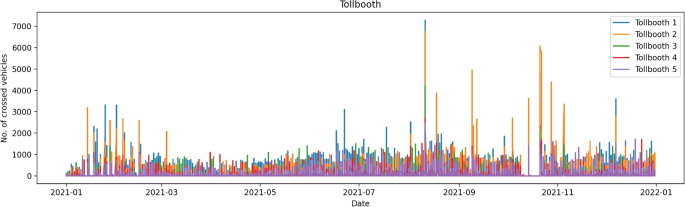
Dataset features plot indexed by hours.
Graphically, it is evident how the different time series are characterized by stationarity, in which many hours are characterized by the passage of no vehicles, especially at night, followed by hours of heavy traffic. We have performed, with the statsmodels Python module, the Augmented Dickey-Fuller (ADF) 54 and Kwiatkowski-Phillips-Schmidt-Shin (KPSS) 55 tests to prove it, as shown also in Table 2 . Particularly, the ADF tests the null hypothesis \(H_0\) , which is that the series presents a unit root against an alternative hypothesis \(H_1\) , which is the absence of unit roots. On the other hand, KPSS tests a null hypothesis \(H_0\) of trend-stationarity of the series against an alternative hypothesis \(H_1\) of the presence of a unit root. At a 95% level, the ADF test on the different features demonstrates the stationarity of the latter since the null hypothesis \(H_0\) can be rejected. Similarly, from the KPSS test, it is clear that at a level of 95%, the null hypothesis \(H_0\) can be rejected, highlighting non-stationarity. This contrast between the two tests indicates how the considered time series are difference-stationary processes . To highlight stationarity through the KPSS test, a new series can be built by differencing between different time-step observations, as shown in Fig. 2 , bringing the results of the two tests into a common agreement.
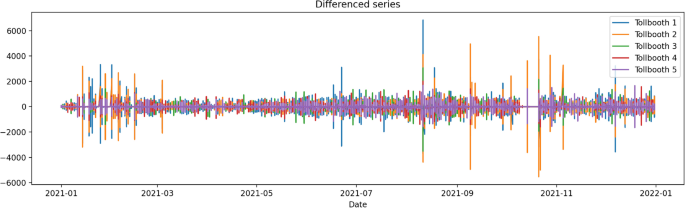
Differenced series.
Comparison between models
We want to test the predictive capabilities of SVM, Random Forest, XGBoost, and RNN-LSTM on the Tollbooth 1 feature. However, unlike Machine Learning models, RNN-LSTM needs to reshape the dataset size by considering a sliding window to “look back”, which is why several attempts have been made with a maximum window of 24 hours in the past, from which the dimensionality tensor has a maximum size equal to (7865, 24, 4). All analyses were performed through Python , and the scaler used in all cases is the StandardScaler . We have set the LSTM network structure with a maximum of 5 input layers with several neurons from 1 to 30, and 1 output layer with 1 neuron only given the one output feature. Given the data type, adding excessive complexity to the network was inappropriate. Table 3 shows the remaining hyperparameters, which control the learning process. On the other hand, from the ML algorithm side, also Table 3 shows the hyperparameters for XGBoost, \(\epsilon \) -SVR, and Random Forest. Particularly, to best adapt them to the dataset type, a GridSearchCV was applied to select the best combination of hyperparameters. For XGBoost and Random Forest, several tests were carried out by modifying the max depth of the trees and number of estimators, while for \(\epsilon \) -SVR, the substantial change concerns the type of kernel used. The dataset was divided into a training set (80%) and a test set (20%). The size of the test set is different because the RNN-LSTM considers a 3D tensor to be the size of the training set, reducing the number of observations allocated to the test set. In contrast, the other machine learning algorithms consider a 2D array. Table 4 compares the different RNN-LSTM and the machine learning algorithms in terms of mean absolute error (MAE), mean squared error (MSE), root mean squared error (RMSE), and \(R^2\) of the prediction. These metrics are calculated as:
where \(y_i\) represents the observed data, \({\hat{y}}_i\) is the predicted ones, and \({\bar{y}}\) is the average value of each features. Greater attention is paid to the MAE and MSE, where the lower values indicate a better model performance in the prediction phase. Specifically, for the LSTM, there is a description of layers and neurons per layer that minimizes the MAE at the best sliding window value obtained (in most cases, equal to 24). The notation used to describe LSTM networks is \(LSTM_{layers:\{neurons \; per \; layer\}}\) , for XGBoost is \(XGBoost_{max\_depth}\) , for SVM is \(SVM_{kernel;C;\epsilon }\) , and for random forest is \(RF_{n\_estimators}\) . For example, an LSTM network with 3 layers and 1 neuron in the first layer, 10 in the second, and 1 in the last layer will be indicated as \(LSTM_{3:\{1,10,1\}}\) . Table 4 presents, in bold, the best values relating to the metrics considered. Specifically, the combination of hyperparameters has been identified for each model to obtain more performing metrics through Cross-Validation. However, further values are reported to show how the accuracy is drastically reduced with minimal variations in the hyperparameters. A first piece of evidence from the MAE and MSE values from the LSTM network is that a relatively simple model (consisting of 2 layers and 3 neurons in total) obtains the best results compared to evolutions with multiple states and neurons. Information of this type pushes us to test the prediction with less complex models, from which we see how XGBoost obtains the best performance among all the models considered, almost on par with random forest. XGBoost’s advantage over the latter is boosting, but the use of Decision Trees allows, in both cases, the building of very high-performance models. Further evidence of the prevalence of “simple” models compared to more complex ones can be observed from the \(\epsilon \) -SVR. In this model, using a linear kernel produces a model with the lowest MAE compared to an RBF-type kernel. The latter allows the addition of non-linearity to the model, which, as highlighted for RNN-LSTM networks, does not bring any advantage to this data type. The high stationarity of the data makes it difficult to add non-linearity to extract more information, from which a more explainable and branched algorithm like XGBoost manages to outperform a complex model like LSTM. Figure 3 shows an example of prediction on the 200-hour test set of the best models ( \(LSTM_{2:\{2,1\}}\) , \(\hbox {XGBoost}_6\) , \(\hbox {SVR}_{lin;0.01;0.1}\) , and \(\hbox {RF}_{{10}}\) ). Specifically, even graphically, we can see that the prediction with LSTM tends to be less stationary and smoother while maintaining the prediction around a trend. At the same time, XGBoost optimally adapts the detrended predicted series to the original one, which is the best choice for a prediction with this type of time series. A similar behavior is adopted by Random Forest (which still uses Decision Trees) but achieves lower performance than XGBoost.
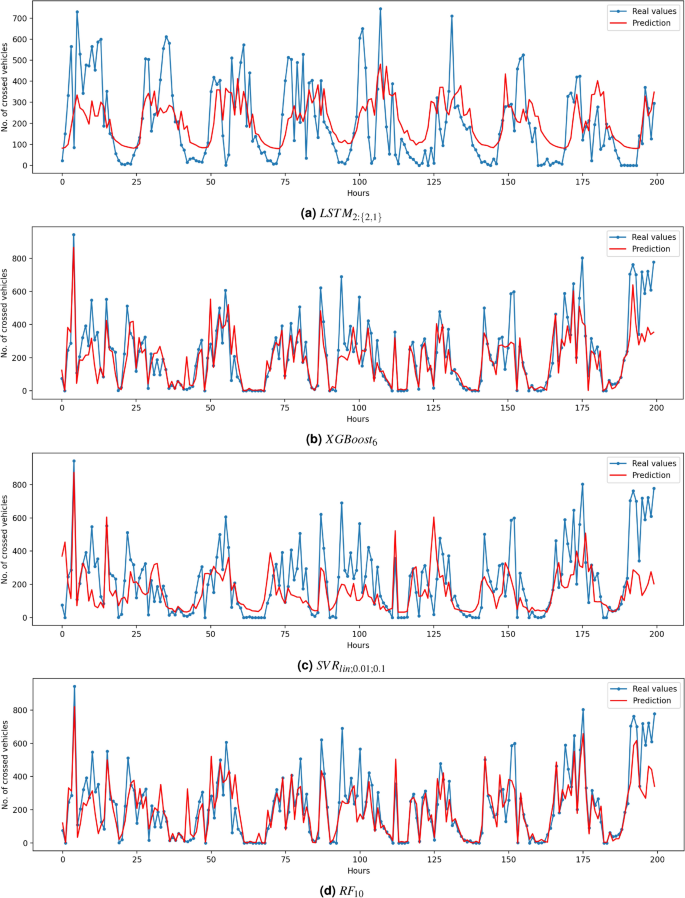
Comparison between different models.
Depending on the results, it may be interesting to transfer the characteristics of this specific model to other domains. Transfer learning (TL) allows the transfer of information from a source domain to a target domain, such as information on instances, parameters, and feature characteristics 56 . In this case, the TL can be used on the influx of vehicles at motorway tollbooths for which there is a lot of missing data due to malfunctions. Although having the same data distribution is difficult, it is still possible to benefit from very accurate predictive models.
Going into explainability in detail, the SHAP framework allows us to study the importance of the different features in the prediction phase. In this case, the idea is to use it on the XGBoost algorithm, which has outperformed its competitors. Considering Tollbooth 1 as the target feature, as shown in Fig. 4 b, the most important feature that affects the prediction is Tollbooth 3 linked to the highest Shapley value, followed by Tollbooth 4 . The distribution can explain this relationship over time of vehicles that passed through Tollbooths 2 to 5. Assuming that Tollbooth 1 is the one of greatest interest to travelers and absorbs the greatest number of vehicles that pass through at different times of the day, different types of users use the remaining toll booths. In this case, Tollbooth 3 has a distribution of vehicles very similar to Tollbooth 1 at different times of the day, albeit with a much smaller number of vehicles, which is why it is the feature that most influence the model. Instead, Tollbooth 2 , despite having a very high average number of vehicles passed through (on a par with Tollbooth 1 ), has a different temporal distribution, which makes it a feature characterized by minimal importance and almost on a par with Tollbooth 5 . The summary plot, however, present in Fig. 4 a, allows us to illustrate different Shapley values as the instances vary, considering the increase in feature values depending on the color intensity of each point. Specifically, the high values achieved by the different features that impact the model correspond to increasingly higher Shapley values and, consequently, higher predicted values (in terms of vehicles passed through). Although not the most important, the Tollbooth 2 feature reaches higher values (regarding vehicles passed through), pushing towards an increase in the Shapley value. This analysis through the SHAP framework shows that the dataset used, although characterized by few features, has optimal characteristics since no feature has a zero magnitude. Therefore, all the features impact the final model, although some in a limited way compared to others.
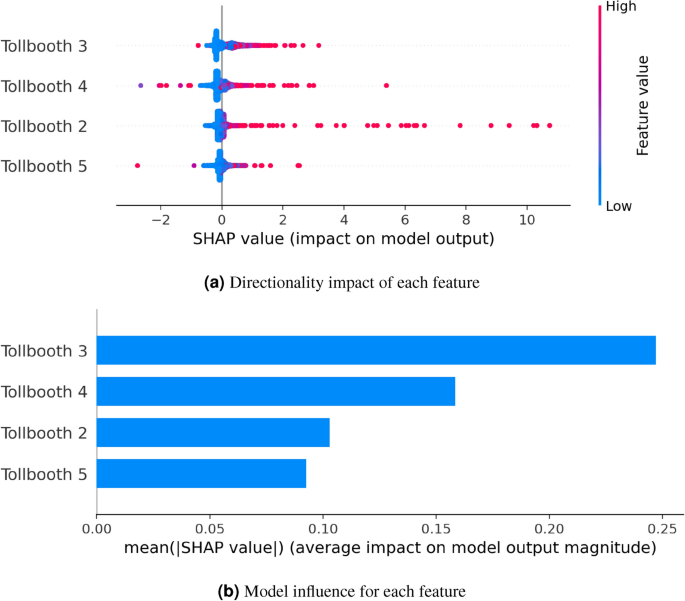
Feature importance summary using SHAP.
Conclusions
Time series prediction represents a fundamental task in many sectors. However, the presence of stationary data is still challenging, especially if the prediction is carried out using deep learning techniques. This work considers data from motorway tollbooths characterized by high stationarity. Here, a series of comparisons were made between machine and deep learning algorithms. Specifically, RNN-LSTM, XGBoost, \(\epsilon \) -SVR, and Random Forest.
The results highlight how XGBoost outperformed the algorithms for prediction on data with these characteristics, obtaining the best results in terms of MAE, MSE, RMSE, and \(R^2\) is clear how the Deep Learning models tend to neutralize the excessive number of peaks in the time series considered, producing a smoother prediction but not corresponding to reality. Using machine learning algorithms such as XGBoost is preferable to more complex models.
The advantage of this result is the possibility of using a computationally less expensive algorithm on this highly stationary data since XGBoost does not require the use of a large number of parameters like an LSTM neural network. Furthermore, using a CART-based algorithm like XGBoost allows us to benefit from a certain degree of explainability of what contributed to the model’s performance. However, using a machine learning algorithm can be seen as a limitation since, in a historical moment in which deep learning models achieved extraordinary performance in many areas, this demonstrates the ineffectiveness of neural networks on data with extreme characteristics such as high stationarity. A further limitation concerns the explainability of the phenomenon since it is possible to identify which are the most essential features. Still, due to the strong peaks in the data, it remains challenging to understand which are the most significant patterns that can be used for prediction.
Data availability
The datasets generated and/or analyzed during the current study are not publicly available since they belong in full to the MONTUR Project still under development (see Acknowledgments), but are available from the corresponding author on reasonable request.
Cheng, C. et al. Time series forecasting for nonlinear and non-stationary processes: A review and comparative study. IIE Trans. 47 , 1053–1071 (2015).
Google Scholar
Schober, P. et al. Stochastic computing design and implementation of a sound source localization system. IEEE J. Emerg. Sel. Top. Circuits Syst. 13 , 295–311. https://doi.org/10.1109/JETCAS.2023.3243604 (2023).
ADS Google Scholar
Akaike, H. Fitting autoregreesive models for prediction. In Selected Papers of Hirotugu Akaike (ed. Akaike, H.) 131–135 (Springer, 1969).
Hurvich, C. M. & Tsai, C.-L. Regression and time series model selection in small samples. Biometrika 76 , 297–307 (1989).
MathSciNet Google Scholar
Box, G. E. & Pierce, D. A. Distribution of residual autocorrelations in autoregressive-integrated moving average time series models. J. Am. Stat. Assoc. 65 , 1509–1526 (1970).
Williams, B. M., Durvasula, P. K. & Brown, D. E. Urban freeway traffic flow prediction: Application of seasonal autoregressive integrated moving average and exponential smoothing models. Transp. Res. Rec. 1644 , 132–141 (1998).
Cao, L.-J. & Tay, F. E. H. Support vector machine with adaptive parameters in financial time series forecasting. IEEE Trans. Neural Netw. 14 , 1506–1518 (2003).
CAS PubMed Google Scholar
Müller, K.-R. et al. Predicting time series with support vector machines. In International conference on artificial neural networks , 999–1004 (Springer, 1997).
Zhang, G. P. & Berardi, V. L. Time series forecasting with neural network ensembles: An application for exchange rate prediction. J. Oper. Res. Soc. 52 , 652–664 (2001).
Noel, M. M. & Pandian, B. J. Control of a nonlinear liquid level system using a new artificial neural network based reinforcement learning approach. Appl. Soft Comput. 23 , 444–451 (2014).
Chen, Y., Yang, B. & Dong, J. Time-series prediction using a local linear wavelet neural network. Neurocomputing 69 , 449–465 (2006).
Zhang, G. P. Time series forecasting using a hybrid arima and neural network model. Neurocomputing 50 , 159–175 (2003).
Jain, A. & Kumar, A. M. Hybrid neural network models for hydrologic time series forecasting. Appl. Soft Comput. 7 , 585–592 (2007).
Aladag, C. H., Egrioglu, E. & Kadilar, C. Forecasting nonlinear time series with a hybrid methodology. Appl. Math. Lett. 22 , 1467–1470 (2009).
Maguire, L. P., Roche, B., McGinnity, T. M. & McDaid, L. Predicting a chaotic time series using a fuzzy neural network. Inf. Sci. 112 , 125–136 (1998).
LeCun, Y., Bengio, Y. & Hinton, G. Deep learning. Nature 521 , 436–444 (2015).
ADS CAS PubMed Google Scholar
Schmidhuber, J. Deep learning in neural networks: An overview. Neural Netw. 61 , 85–117 (2015).
PubMed Google Scholar
Varnousfaderani, E. S. & Shihab, S. A. M. Bird movement prediction using long short-term memory networks to prevent bird strikes with low altitude aircraft. AIAA Aviat. 2023 Forum https://doi.org/10.2514/6.2023-4531.c1 (2023).
Sen, J., Dutta, A. & Mehtab, S. Stock portfolio optimization using a deep learning lstm model. 2021 IEEE Mysore Sub Section International Conference (MysuruCon) 263–271, https://doi.org/10.1109/MysuruCon52639.2021.9641662 (2021).
Zdravković, M., Ćirić, I. & Ignjatović, M. Explainable heat demand forecasting for the novel control strategies of district heating systems. Annu. Rev. Control. 53 , 405–413. https://doi.org/10.1016/j.arcontrol.2022.03.009 (2022).
Baesmat, K. H., Masoudipour, I. & Samet, H. Improving the performance of short-term load forecast using a hybrid artificial neural network and artificial bee colony algorithm amélioration des performances de la prévision de la charge à court terme à l’aide d’un réseau neuronal artificiel hybride et d’un algorithme de colonies d’abeilles artificielles. IEEE Can. J. Electr. Comput. Eng. 44 , 275–282. https://doi.org/10.1109/ICJECE.2021.3056125 (2021).
Baesmat, H. K. & Shiri, A. A new combined method for future energy forecasting in electrical networks. Int. Trans. Electr. Energy Syst. 29 , e2749. https://doi.org/10.1002/etep.2749 (2019) ( E2749 ITEES-17-0407.R4 ).
Wen, X. & Li, W. Time series prediction based on lstm-attention-lstm model. IEEE Access 11 , 48322–48331. https://doi.org/10.1109/ACCESS.2023.3276628 (2023).
Abbasimehr, H., Paki, R. & Bahrini, A. A novel xgboost-based featurization approach to forecast renewable energy consumption with deep learning models. Sustain. Comput. Inform. Syst. 38 , 100863. https://doi.org/10.1016/j.suscom.2023.100863 (2023).
Alipour, P. & Esmaeilpour Charandabi, S. The impact of tweet sentiments on the return of cryptocurrencies: Rule-based vs. machine learning approaches. Eur. J. Bus. Manag. Res. 9 , 1–5. https://doi.org/10.24018/ejbmr.2024.9.1.2180 (2024).
Ghasemi, A. & Naser, M. Tailoring 3d printed concrete through explainable artificial intelligence. Structures 56 , 104850. https://doi.org/10.1016/j.istruc.2023.07.040 (2023).
Qiu, Y. & Wang, J. A machine learning approach to credit card customer segmentation for economic stability. In Proc. of the 4th International Conference on Economic Management and Big Data Applications, ICEMBDA 2023, October 27–29, 2023, Tianjin, China [SPACE] https://doi.org/10.4108/eai.27-10-2023.2342007 (2024).
Wang, H. et al. Machine learning-enabled mimo-fbmc communication channel parameter estimation in iiot: A distributed cs approach. Digit. Commun. Netw. 9 , 306–312. https://doi.org/10.1016/j.dcan.2022.10.012 (2023).
CAS Google Scholar
Wang, H., Xu, L., Yan, Z. & Gulliver, T. A. Low-complexity mimo-fbmc sparse channel parameter estimation for industrial big data communications. IEEE Trans. Ind. Inf. 17 , 3422–3430. https://doi.org/10.1109/TII.2020.2995598 (2021).
Wang, H. et al. Sparse Bayesian learning based channel estimation in fbmc/oqam industrial iot networks. Comput. Commun. 176 , 40–45. https://doi.org/10.1016/j.comcom.2021.05.020 (2021).
Frifra, A., Maanan, M., Maanan, M. & Rhinane, H. Harnessing lstm and xgboost algorithms for storm prediction. Sci. Rep. https://doi.org/10.1038/s41598-024-62182-0 (2024).
PubMed PubMed Central Google Scholar
Hu, H., van der Westhuysen, A. J., Chu, P. & Fujisaki-Manome, A. Predicting lake erie wave heights and periods using xgboost and lstm. Ocean Model. 164 , 101832. https://doi.org/10.1016/j.ocemod.2021.101832 (2021).
Tehranian, K. Can machine learning catch economic recessions using economic and market sentiments? http://arxiv.org/abs/2308.16200v1 (2023).
Fan, C., Xiao, F. & Zhao, Y. A short-term building cooling load prediction method using deep learning algorithms. Appl. Energy 195 , 222–233. https://doi.org/10.1016/j.apenergy.2017.03.064 (2017).
Wei, Z. et al. Prediction of residential district heating load based on machine learning: A case study. Energy 231 , 120950. https://doi.org/10.1016/j.energy.2021.120950 (2021).
McCullock, W. S. & Pitts, W. H. A logical calculus of the ideas immanent in nervous activity. Bull. Math. Biophys. 5 , 115–133 (1943).
Rosenblatt, F. The percepron: A probabilistic model for information storage and organization in the brain. Psychol. Rev. 65 , 386 (1958).
Rumelhart, D. E., Hinton, G. E. & Williams, R. J. Learning representation by back-propagation errors. Nature https://doi.org/10.1038/323533a0 (1986).
Hochreiter, S. & Schmidhuber, J. Long short-term memory. Neural Comput. 9 , 1735–1780. https://doi.org/10.1162/neco.1997.9.8.1735 (1997).
Vapnik, V. N. The Nature of Statistical Learning Theory (Springer, 1995).
Ciano, T. & Ferrara, M. Karush-kuhn-tucker conditions and lagrangian approach for improving machine learning techniques: A survey and new developments. Atti della Accademia Peloritana dei Pericolanti - Classe di Scienze Fisiche, Matematiche e Naturali 102 , 1. https://doi.org/10.1478/AAPP.1021A1 (2024).
Sabzekar, M. & Hasheminejad, S. M. H. Robust regression using support vector regressions. Chaos Solitons Fractals 144 , 110738. https://doi.org/10.1016/j.chaos.2021.110738 (2021).
Klopfenstein, Q. & Vaiter, S. Linear support vector regression with linear constraints. Mach. Learn. 110 , 1939–1974. https://doi.org/10.1007/s10994-021-06018-2 (2021).
Breiman, L. Random forests. Mach. Learn. 45 , 5–32. https://doi.org/10.1023/A:1010933404324 (2001).
Biau, G. Analysis of a random forests model. J. Mach. Learn. Res. 13 , 1063–1095 (2012).
Chen, T. & Guestrin, C. Xgboost: A scalable tree boosting system. In Proceedings of the 22nd ACM SIGKDD International Conference on Knowledge Discovery and Data Mining , KDD ’16, 785–794, https://doi.org/10.1145/2939672.2939785 (Association for Computing Machinery, 2016).
Breiman, L., Friedman, J., Olshen, R. & Stone, C. J. Classification and Regression Trees (Chapman and Hall/CRC, 1984).
Li, S. & Zhang, X. Research on orthopedic auxiliary classification and prediction model based on xgboost algorithm. Neural Comput. Appl. 32 , 1971–1979. https://doi.org/10.1007/s00521-019-04378-4 (2020).
Mohril, R. S., Solanki, B. S., Kulkarni, M. S. & Lad, B. K. Xgboost based residual life prediction in the presence of human error in maintenance. Neural Comput. Appl. 35 , 3025–3039. https://doi.org/10.1007/s00521-022-07216-2 (2022).
Mustapha, I. B., Abdulkareem, Z., Abdulkareem, M. & Ganiyu, A. Predictive modeling of physical and mechanical properties of pervious concrete using xgboost. Neural Comput. Appl. https://doi.org/10.1007/s00521-024-09553-w (2024).
Lundberg, S. M. & Lee, S.-I. A unified approach to interpreting model predictions. In Proc. of the 31st International Conference on Neural Information Processing Systems 4768–4777 (2017).
Li, Z. Extracting spatial effects from machine learning model using local interpretation method: An example of shap and xgboost. Comput. Environ. Urban Syst. 96 , 101845. https://doi.org/10.1016/j.compenvurbsys.2022.101845 (2022).
Lundberg, S. M., Erion, G. G. & Lee, S.-I. Consistent individualized feature attribution for tree ensembles. http://arxiv.org/abs/1802.03888 (2018).
Dickey, D. A. & Fuller, W. A. Distribution of the estimators for autoregressive time series with a unit root. J. Am. Stat. Assoc. 74 , 427–431. https://doi.org/10.2307/2286348 (1979).
Kwiatkowski, D., Phillips, P. C., Schmidt, P. & Shin, Y. Testing the null hypothesis of stationarity against the alternative of a unit root: How sure are we that economic time series have a unit root?. J. Econom. 54 , 159–178. https://doi.org/10.1016/0304-4076(92)90104-Y (1992).
Liu, W., Liu, W. D. & Gu, J. Predictive model for water absorption in sublayers using a joint distribution adaption based xgboost transfer learning method. J. Petrol. Sci. Eng. 188 , 106937. https://doi.org/10.1016/j.petrol.2020.106937 (2020).
Download references
Acknowledgements
The authors acknowledge the University of Aosta Valley, in particular the Department of Economics and Political Sciences by the CT-TEM UNIVDA - Centro Transfrontaliero sul Turismo e l’Economia di Montagna and their Director. Prof. Marco Alderighi for their support through the MONTUR Project. A part of Data testing was developed by using “Real Time Series” extrapolated by the mentioned project and will be adopted as the basis for future work. The present work defines the crucial and pivotal structural elements of the Decision Support Systems will be developed into the MONTUR Project. The Authors thank equally the Decisions LAB - Department of Law, Economics and Human Sciences - University Mediterranea of Reggio Calabria for its support to the present research. Funded by European Union- Next Generation EU, Component M4C2, Investment 1.1., Progetti di Ricerca di Rilevante Interesse Nazionale (PRIN) - Notice 1409, 14/09/2022- BANDO PRIN 2022 PNRR. Project title: “Climate risk and uncertainty: environmental sustainability and asset pricing”. Project code “P20225MJW8”, CUP: E53D23016470001.
Author information
These authors contributed equally: Domenico Santoro, Tiziana Ciano and Massimiliano Ferrara.
Authors and Affiliations
Department of Economics, Management and Territory, University of Foggia, 71121, Foggia, FG, Italy
Domenico Santoro
Department of Economics and Political Sciences, University of Aosta Valley, 11100, Aosta, AO, Italy
Tiziana Ciano
Department of Law, Economics and Human Sciences & Decisions_Lab, University “Mediterranea” of Reggio Calabria, 89125, Reggio Calabria, RC, Italy
Tiziana Ciano & Massimiliano Ferrara
Department of Management and Technology, ICRIOS - The Invernizzi Centre for Research in Innovation, Organization, Strategy and Entrepreneurship, Bocconi University, 20136, Milan, MI, Italy
Massimiliano Ferrara
You can also search for this author in PubMed Google Scholar
Contributions
M.F. and T.C. conceptualization, M.F. and T.C. data acquisition, M.F. and T.C. conceived the experiment(s), D.S. conducted the experiment(s), D.S., T.C., and M.F. analyzed the results and selected the models, M.F. project administration. All authors reviewed the manuscript.
Corresponding author
Correspondence to Massimiliano Ferrara .
Ethics declarations
Competing interests.
The authors declare no competing interests.
Additional information
Publisher's note.
Springer Nature remains neutral with regard to jurisdictional claims in published maps and institutional affiliations.
Rights and permissions
Open Access This article is licensed under a Creative Commons Attribution-NonCommercial-NoDerivatives 4.0 International License, which permits any non-commercial use, sharing, distribution and reproduction in any medium or format, as long as you give appropriate credit to the original author(s) and the source, provide a link to the Creative Commons licence, and indicate if you modified the licensed material. You do not have permission under this licence to share adapted material derived from this article or parts of it. The images or other third party material in this article are included in the article’s Creative Commons licence, unless indicated otherwise in a credit line to the material. If material is not included in the article’s Creative Commons licence and your intended use is not permitted by statutory regulation or exceeds the permitted use, you will need to obtain permission directly from the copyright holder. To view a copy of this licence, visit http://creativecommons.org/licenses/by-nc-nd/4.0/ .
Reprints and permissions
About this article
Cite this article.
Santoro, D., Ciano, T. & Ferrara, M. A comparison between machine and deep learning models on high stationarity data. Sci Rep 14 , 19409 (2024). https://doi.org/10.1038/s41598-024-70341-6
Download citation
Received : 15 April 2024
Accepted : 14 August 2024
Published : 21 August 2024
DOI : https://doi.org/10.1038/s41598-024-70341-6
Share this article
Anyone you share the following link with will be able to read this content:
Sorry, a shareable link is not currently available for this article.
Provided by the Springer Nature SharedIt content-sharing initiative
By submitting a comment you agree to abide by our Terms and Community Guidelines . If you find something abusive or that does not comply with our terms or guidelines please flag it as inappropriate.
Quick links
- Explore articles by subject
- Guide to authors
- Editorial policies
Sign up for the Nature Briefing: AI and Robotics newsletter — what matters in AI and robotics research, free to your inbox weekly.

IEEE Account
- Change Username/Password
- Update Address
Purchase Details
- Payment Options
- Order History
- View Purchased Documents
Profile Information
- Communications Preferences
- Profession and Education
- Technical Interests
- US & Canada: +1 800 678 4333
- Worldwide: +1 732 981 0060
- Contact & Support
- About IEEE Xplore
- Accessibility
- Terms of Use
- Nondiscrimination Policy
- Privacy & Opting Out of Cookies
A not-for-profit organization, IEEE is the world's largest technical professional organization dedicated to advancing technology for the benefit of humanity. © Copyright 2024 IEEE - All rights reserved. Use of this web site signifies your agreement to the terms and conditions.
arXiv's Accessibility Forum starts next month!
Help | Advanced Search
Computer Science > Machine Learning
Title: extraction of research objectives, machine learning model names, and dataset names from academic papers and analysis of their interrelationships using llm and network analysis.
Abstract: Machine learning is widely utilized across various industries. Identifying the appropriate machine learning models and datasets for specific tasks is crucial for the effective industrial application of machine learning. However, this requires expertise in both machine learning and the relevant domain, leading to a high learning cost. Therefore, research focused on extracting combinations of tasks, machine learning models, and datasets from academic papers is critically important, as it can facilitate the automatic recommendation of suitable methods. Conventional information extraction methods from academic papers have been limited to identifying machine learning models and other entities as named entities. To address this issue, this study proposes a methodology extracting tasks, machine learning methods, and dataset names from scientific papers and analyzing the relationships between these information by using LLM, embedding model, and network clustering. The proposed method's expression extraction performance, when using Llama3, achieves an F-score exceeding 0.8 across various categories, confirming its practical utility. Benchmarking results on financial domain papers have demonstrated the effectiveness of this method, providing insights into the use of the latest datasets, including those related to ESG (Environmental, Social, and Governance) data.
Comments: | 10 pages, 8 figures |
Subjects: | Machine Learning (cs.LG); Artificial Intelligence (cs.AI); Computation and Language (cs.CL) |
Cite as: | [cs.LG] |
(or [cs.LG] for this version) | |
Focus to learn more arXiv-issued DOI via DataCite (pending registration) |
Submission history
Access paper:.
- HTML (experimental)
- Other Formats

References & Citations
- Google Scholar
- Semantic Scholar
BibTeX formatted citation

Bibliographic and Citation Tools
Code, data and media associated with this article, recommenders and search tools.
- Institution
arXivLabs: experimental projects with community collaborators
arXivLabs is a framework that allows collaborators to develop and share new arXiv features directly on our website.
Both individuals and organizations that work with arXivLabs have embraced and accepted our values of openness, community, excellence, and user data privacy. arXiv is committed to these values and only works with partners that adhere to them.
Have an idea for a project that will add value for arXiv's community? Learn more about arXivLabs .
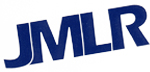
Frequently Asked Questions
JMLR Papers
Select a volume number to see its table of contents with links to the papers.
Volume 25 (January 2024 - Present)
Volume 24 (January 2023 - December 2023)
Volume 23 (January 2022 - December 2022)
Volume 22 (January 2021 - December 2021)
Volume 21 (January 2020 - December 2020)
Volume 20 (January 2019 - December 2019)
Volume 19 (August 2018 - December 2018)
Volume 18 (February 2017 - August 2018)
Volume 17 (January 2016 - January 2017)
Volume 16 (January 2015 - December 2015)
Volume 15 (January 2014 - December 2014)
Volume 14 (January 2013 - December 2013)
Volume 13 (January 2012 - December 2012)
Volume 12 (January 2011 - December 2011)
Volume 11 (January 2010 - December 2010)
Volume 10 (January 2009 - December 2009)
Volume 9 (January 2008 - December 2008)
Volume 8 (January 2007 - December 2007)
Volume 7 (January 2006 - December 2006)
Volume 6 (January 2005 - December 2005)
Volume 5 (December 2003 - December 2004)
Volume 4 (Apr 2003 - December 2003)
Volume 3 (Jul 2002 - Mar 2003)
Volume 2 (Oct 2001 - Mar 2002)
Volume 1 (Oct 2000 - Sep 2001)
Special Topics
Bayesian Optimization
Learning from Electronic Health Data (December 2016)
Gesture Recognition (May 2012 - present)
Large Scale Learning (Jul 2009 - present)
Mining and Learning with Graphs and Relations (February 2009 - present)
Grammar Induction, Representation of Language and Language Learning (Nov 2010 - Apr 2011)
Causality (Sep 2007 - May 2010)
Model Selection (Apr 2007 - Jul 2010)
Conference on Learning Theory 2005 (February 2007 - Jul 2007)
Machine Learning for Computer Security (December 2006)
Machine Learning and Large Scale Optimization (Jul 2006 - Oct 2006)
Approaches and Applications of Inductive Programming (February 2006 - Mar 2006)
Learning Theory (Jun 2004 - Aug 2004)
Special Issues
In Memory of Alexey Chervonenkis (Sep 2015)
Independent Components Analysis (December 2003)
Learning Theory (Oct 2003)
Inductive Logic Programming (Aug 2003)
Fusion of Domain Knowledge with Data for Decision Support (Jul 2003)
Variable and Feature Selection (Mar 2003)
Machine Learning Methods for Text and Images (February 2003)
Eighteenth International Conference on Machine Learning (ICML2001) (December 2002)
Computational Learning Theory (Nov 2002)
Shallow Parsing (Mar 2002)
Kernel Methods (December 2001)
. |

IMAGES
COMMENTS
Benjamin Recht. Article No.: 20, Pages 724-750. This paper provides elementary analyses of the regret and generalization of minimum-norm interpolating classifiers (MNIC). The MNIC is the function of smallest Reproducing Kernel Hilbert Space norm that perfectly interpolates a label pattern on a finite ...
The AI Scientist: Towards Fully Automated Open-Ended Scientific Discovery. sakanaai/ai-scientist • • 12 Aug 2024 This approach signifies the beginning of a new era in scientific discovery in machine learning: bringing the transformative benefits of AI agents to the entire research process of AI itself, and taking us closer to a world where endless affordable creativity and innovation can ...
MushroomRL: Simplifying Reinforcement Learning Research Carlo D'Eramo, Davide Tateo, Andrea Bonarini, Marcello Restelli, Jan Peters; (131):1−5, 2021. (Machine Learning Open Source Software Paper) Locally Differentially-Private Randomized Response for Discrete Distribution Learning
Explore the latest research papers on machine learning, including topics on falsifiable, replicable, and reprocible empirical ML research.
Machine learning is the ability of a machine to improve its performance based on previous results. Machine learning methods enable computers to learn without being explicitly programmed and have ...
The Journal of Machine Learning Research (JMLR) provides an international forum for the electronic and paper publication of high-quality scholarly articles in all areas of machine learning.JMLR seeks previously unpublished papers that contain:new algorithms with empirical, theoretical, psychological, or biological justification; experimental and/or theoretical studies yielding new insight into ...
(Machine Learning Open Source Software Paper) Torchhd: An Open Source Python Library to Support Research on Hyperdimensional Computing and Vector Symbolic Architectures Mike Heddes, Igor Nunes, Pere Vergés, Denis Kleyko, Danny Abraham, Tony Givargis, Alexandru Nicolau, Alexander Veidenbaum; (255):1−10, 2023.
The Journal of Machine Learning Research (JMLR), established in 2000, provides an international forum for the electronic and paper publication of high-quality scholarly articles in all areas of machine learning. All published papers are freely available online. JMLR has a commitment to rigorous yet rapid reviewing. Final versions are published ...
To discuss the applicability of machine learning-based solutions in various real-world application domains. To highlight and summarize the potential research directions within the scope of our study for intelligent data analysis and services. The rest of the paper is organized as follows.
Weather and climate predicted accurately — without using a supercomputer. A cutting-edge global model of the atmosphere combines machine learning with a numerical model based on the laws of ...
Machine Learning is an international forum focusing on computational approaches to learning. ... Improves how machine learning research is conducted. Prioritizes verifiable and replicable supporting evidence in all published papers. Editor-in-Chief. Hendrik Blockeel; Journal Impact Factor 4.3 (2023)
JMLR Papers. Select a volume number to see its table of contents with links to the papers. Volume 23 (January 2022 - Present) . Volume 22 (January 2021 - December 2021) . Volume 21 (January 2020 - December 2020) . Volume 20 (January 2019 - December 2019) . Volume 19 (August 2018 - December 2018) . Volume 18 (February 2017 - August 2018) . Volume 17 (January 2016 - January 2017)
Machine learning, especially its subfield of Deep Learning, had many amazing advances in the recent years, and important research papers may lead to breakthroughs in technology that get used by billio ns of people. The research in this field is developing very quickly and to help our readers monitor the progress we present the list of most important recent scientific papers published since 2014.
Machine Learning with Applications (MLWA) is a peer reviewed, open access journal focused on research related to machine learning.The journal encompasses all aspects of research and development in ML, including but not limited to data mining, computer vision, natural language processing (NLP), intelligent systems, neural networks, AI-based software engineering, bioinformatics and their ...
Since ML appeared in the 1990s, all published documents (i.e., journal papers, reviews, conference papers, preprints, code repositories and more) related to this field from 1990 to 2020 have been selected, and specifically, within the search fields, the following keywords were used: "machine learning" OR "machine learning-based approach" OR ...
Explore the latest full-text research PDFs, articles, conference papers, preprints and more on MACHINE LEARNING. Find methods information, sources, references or conduct a literature review on ...
Machine learning techniques have emerged as a transformative force, revolutionizing various application domains, particularly cybersecurity. The development of optimal machine learning applications requires the integration of multiple processes, such as data pre-processing, model selection, and parameter optimization. While existing surveys have shed light on these techniques, they have mainly ...
Journal of Machine Learning Research. JMLR Volume 21. A Low Complexity Algorithm with O (√T) Regret and O (1) Constraint Violations for Online Convex Optimization with Long Term Constraints. Hao Yu, Michael J. Neely; (1):1−24, 2020. [ abs ] [ pdf ] [ bib ] A Statistical Learning Approach to Modal Regression.
A Pipeline for Data-Driven Learning of Topological Features with Applications to Protein Stability Prediction. Amish Mishra, Francis Motta. Comments: 13 figures, 23 pages (without appendix and references) Subjects: Machine Learning (stat.ML); Machine Learning (cs.LG); Data Analysis, Statistics and Probability (physics.data-an)
Top Machine Learning Research Papers Released In 2021. Advances in the machine and deep learning in 2021 could lead to new technologies utilised by billions of people worldwide. Share. Published on November 18, 2021. by Dr. Nivash Jeevanandam. Advances in machine learning and deep learning research are reshaping our technology.
Today, intelligent systems that offer artificial intelligence capabilities often rely on machine learning. Machine learning describes the capacity of systems to learn from problem-specific training data to automate the process of analytical model building and solve associated tasks. Deep learning is a machine learning concept based on artificial neural networks. For many applications, deep ...
Machine Learning. Abstract: In machine learning, a computer first learns to perform a task by studying a training set of examples. The computer then performs the same task with data it hasn't encountered before. This article presents a brief overview of machine-learning technologies, with a concrete case study from code analysis.
Advances in sensor, computing, and communication technologies are enabling big data analytics by providing time series data. However, conventional models struggle to identify sequence features and ...
The main objective of this paper is to highlight the research directions and explain the main roles of current Artificial Intelligence (AI)/Machine Learning (ML) frameworks and available cloud infrastructures in building end-to-end ML lifecycle management for healthcare systems and sensitive biomedical data. We identify and explore the versatility of many genuine techniques from distributed ...
Therefore, research focused on extracting combinations of tasks, machine learning models, and datasets from academic papers is critically important, as it can facilitate the automatic recommendation of suitable methods. Conventional information extraction methods from academic papers have been limited to identifying machine learning models and ...
ML is a process of learning models of relationships from data, and the supervised learning uses regression and classification techniques to predict the target by the other variables. The term task in mrl3 means a prediction problem, the term learners—the ML algorithms, including decision trees, support vector machines, neural networks, and more.
This paper presents a procedure for determining the initial design parameters using analytical calculation method for a PMSM, followed by developing machine learning algorithms (XGBoost, random forest, and artificial neural networks) with the available benchmarking data and compare their performance to determine the motor design parameters.
JMLR Papers. Select a volume number to see its table of contents with links to the papers. Volume 25 (January 2024 - Present) . Volume 24 (January 2023 - December 2023) . Volume 23 (January 2022 - December 2022) . Volume 22 (January 2021 - December 2021) . Volume 21 (January 2020 - December 2020) . Volume 20 (January 2019 - December 2019) ...