- Hawaii News
- National News
- International News
- Local Sports
- National Sports
- Letters to the Editor
- Entertainment
- Classifieds
- Print Ad Directory
- Browse notices
- Place a notice
- Statement of Values
- Terms of Service
- Submit News

- Today's Paper

Rural Hawai‘i faces major health challenges, UH report finds
Submitted by UH
WAILUKU–Hawai’i residents living in rural areas face significantly more health challenges than those who live in urban areas, according to a first-of-its-kind report by the University of Hawai’i Economic Research Organization (UHERO). Released Aug. 25, the report also found that the challenges are even greater for rural residents with disabilities and for those living in poverty.
The data was gathered in June 2023 through a survey of 1,571 residents, with more than 20% identifying as living in rural areas. The assessment used data from the UHERO Rapid Survey, a twice-yearly health survey of a general population cohort in Hawai’i that has been administered since 2022.
This is the first rural health report targeted specifically for Hawai’i that measures data at the individual level.
The full report can be found at https://uhero.hawaii.edu/rural-health-disparities-in-hawaii/.
Compared with those living in urban areas, residents in rural areas reported that their overall health was lower and had more days per week where their activities were restricted by their physical or mental health.
The report was produced by UHERO and funded by UH’s Rural Health Research and Policy Center (RHRPC) through a grant from the U.S. Department of Health and Human Services, Health Resources and Services Administration.
SIGNIFICANT FINDINGS:
¯ Residents in rural areas reported having a 43% lower odds of perceiving their overall health as excellent, very good or good compared to their counterparts in non-rural areas.
¯ Nearly 22% of respondents living in rural areas rated their health as fair or poor (21.7%, n=70) and fewer than 14% of non-rural respondents (14%, n=169) rated their health at this level.
¯ Rural residents reported an average of 3.8 days/week that their life activities were restricted by their physical health compared to 2.5 days in non-rural areas, and for mental health an average of 3.1 days/week versus 2.
¯ Having a disability and living in rural areas is associated with an additional 2 days/week of life activities restricted by physical health issues compared to people with disabilities in non-rural areas.
¯ Notably for Asians, high overall health (respondents who describe their overall health as excellent, very good or good) was less than 70% for rural residents, but more than 80% for non-rural residents.
¯ For those who live in rural households below the poverty line, less than 40% reported high overall health compared with more than 50% for non-rural residents.
¯ Native Hawaiians and Pacific Islanders (NHPI) made up a higher share of the rural sample (23.1%) compared to 14.7% among the people surveyed in non-rural areas, and NHPI reported 1.1 additional days per week that were affected by physical health issues compared with people who are not NHPI.
The report examines several definitions of rural areas. The results above are based on the report’s primary definition of rural areas, which are all zip codes in the state except for East O’ahu, Kāne’ohe in the east to Kapolei in the west, Līhu’e on Kaua’i, Kahului-Wailuku on Maui, and Hilo and Kailua-Kona on Hawai’i island.
The UH researchers noted that the report’s findings suggest that policy responses aimed at improving health outcomes and preventive healthcare utilization should include efforts to reduce disparities between rural and non-rural populations. The results also indicate that rural health policy should prioritize support for marginalized groups, especially individuals with disabilities and those with low incomes in rural regions.
“This report helps to tell the story of how health outcomes differ in rural areas compared to more urban areas, providing the evidence base for potential policy solutions to address these challenges,” said Aimee Grace, Principal Investigator of the Rural Health Research and Policy Center and Director of the UH System Office of Strategic Health Initiatives.
UHERO is housed in UH Mānoa’s College of Social Sciences and RHRPC is housed in the UH System Office of Strategic Health Initiatives.
Today's breaking news and more in your inbox
- Daily Newsletter
- Breaking News
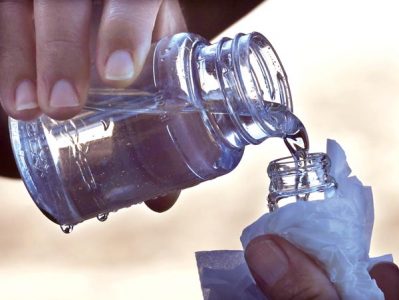
Brown Water Advisory issued from Waihe‘e to Kahului Harbor
Submitted by UH WAILUKU—Hawai‘i residents living in rural areas face significantly more health challenges ...
DOH and their partners announce Maui events for suicide prevention and awareness month in September
The Maui News WAILUKU—This year is the inaugural designation of September as “Suicide Prevention and ...
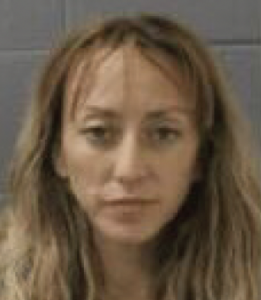
MPD asks for public’s assistance locating missing person
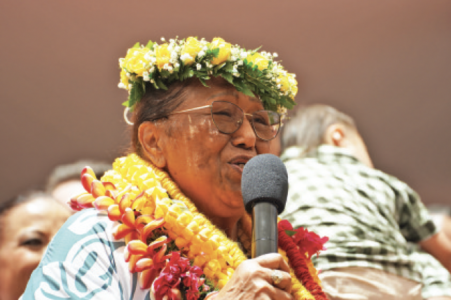
Carolee “Doll” Aricayos honored with 2024 Emma Farden Sharpe Hula Festival Legacy Award
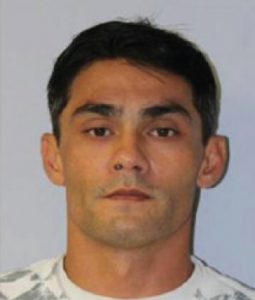
Robert Dumaran, suspect in IED case, has preliminary hearing continued to September
Only $99/year, subscribe today.
Securing data and preserving privacy in cloud IoT-based technologies an analysis of assessing threats and developing effective safeguard
- Open access
- Published: 27 August 2024
- Volume 57 , article number 269 , ( 2024 )
Cite this article
You have full access to this open access article
- Mayank Pathak 1 ,
- Kamta Nath Mishra 1 &
- Satya Prakash Singh 1
332 Accesses
6 Altmetric
Explore all metrics
The Internet of Things (IoT) is a powerful technology adopted in various industries. Applications of the IoT aim to enhance automation, productivity, and user comfort in a cloud and distributive computing environment. Cloud computing automatically stores and analyzes the large amounts of data generated by IoT-based applications. Cloud computing has become a crucial component of the information age through easier data administration and storage. Currently, government agencies, commercial enterprises, and end users are rapidly migrating their data to cloud environments. This may require end-user authentication, greater safety, and data recovery in the event of an attack. A few issues were discovered by authors after analysis and assessments of various aspects of the published research papers. The research analysis reveals that the existing methods need to be further improved to address the contemporary dangers related to data security and privacy. Based on the research reports, it can be stated that safe end-to-end data transmission in a cloud-IoT environment requires modifications and advancements in the design of reliable protocols. Upcoming technologies like blockchain, machine learning, fog, and edge computing mitigate data over the cloud to some level. The study provides a thorough analysis of security threats including their categorization, and potential countermeasures to safeguard our cloud-IoT data. Additionally, the authors have summarized cutting-edge approaches like machine learning and blockchain technologies being used in the data security privacy areas. Further, this paper discusses the existing problems related to data privacy and security in the cloud-IoT environment in today’s world and their possible future directions.
Explore related subjects
- Artificial Intelligence
Avoid common mistakes on your manuscript.
1 Introduction
The speedy development of technology and Internet of Things (IoT)-based devices in organizations and enterprises give rise to progressive increases in various types of data. IoT has become a vital part of human life and it can be sensed in our day-to-day activities. It was said by Kumar et al. ( 2019 ) that IoT is a revolutionary approach that has changed numerous aspects of human life. It makes our lives easy and secure by handling various applications of smart city societies including pollution control , smart transportation , smart industries , smart home security systems , smart water supply , and many more systems. The small amount of data accumulates and gives rise to Big Data which is stored, processed, and analyzed by a set of technologies. Big Data is a large volume of data generated by IoT sensors , servers , social media , and medical equipment, etc . Cloud computing is internet-based computing that enables inexpensive, reliable, easy, simple, and convenient accessibility to the resource (Albugmi et al. 2016 ). Cloud computing provides service, and reduces infrastructure maintenance overheads. Apart from this it also provides better performance to the end users and flexibility for storing data over the cloud. However, storing highly confidential Big Data obtained from IoT devices , medical data , and server data over the cloud may pose threats to attackers. Therefore, data security is a most important concern when a large or bulk of confidential data is to be stored in the cloud (Sumithra and Parameswari 2022 ).
Cyber attacks target IoT devices that impact stakeholders, and they may damage physical systems, m-health, and economic systems severely. Earlier events show that IoT devices hold numerous vulnerabilities. Many manufacturers struggle to protect IoT devices from vulnerabilities (Schiller et al. 2022a ). Cloud computing integrates distributed computing, grid computing, and utility computing to establish a shared virtual resource pool (Sun 2019 ). There are privacy and security issues in these cases because the owners have no control over the information and tasks carried out on the platform. Various privacy protection methods have been introduced such as encryption , access control , cryptography , and digital signature but they are not strong enough, as a result, attackers easily break through the security wall and harm the data over the cloud.
The authors of the research papers reviewed various methods and suggested some measures and directions to protect the data in cloud computing and edge computing environments (Ravi Kumar et al. 2018 ; Zhang et al. 2018 ). Through this study, the author found that data privacy , data remoteness , data leakage , and data segregation are crucial problems that may exist. The survey paper (Hong-Yen and Jiankun 2019 ) addressed modern privacy and preserving models to focus on numerous privacy-interrelated frameworks to be implemented in practice.
As a contribution, the current paper aims to accomplish the following objectives:
To examine existing security frameworks , standards , and techniques that incorporate different standards across multiple areas of cloud-IoT technologies.
To explore and discuss open-ended challenges in a Cloud-IoT-based environment concerning securities and privacy.
To present and discuss the classification of challenges in Cloud-IoT environments after evaluating the performance of existing literature. It also provides solutions for the identified open-ended challenges and addresses future security concerns related to Cloud-IoT technologies.
The following are the Research Questions ( RQs ) that the researchers tried to investigate through the current research paper:
RQ 1 : To Investigate how IoT , Big Data , and Cloud computing technologies are interconnected, and how security can be a major concern when data is stored in a cloud environment.
RQ 2 : What are the security objectives for the data security and privacy domain?
RQ 3 : What are the privacy concerns for end-users in cloud-IoT-based environments?
RQ 4 : What is the role of edge computing in enhancing privacy in a cloud-IoT environment?
RQ 5 : What are vulnerabilities that exist in the cloud-IoT infrastructure?
RQ 6 : What are the current research trends and areas of focus?
RQ 7 : What are Advancements in security threat detection and avoidance ?
RQ 8 : How machine learning can be a useful tool in detecting vulnerabilities within a cloud-IoT environment?
RQ 9 : How can blockchain technology be an effective measure of data security and privacies?
RQ 10 : What are Current Issues in Data Security and Privacy?
Regarding the remaining portion of the document, Sect. 2 describes the methodology of this research work. Section 3 discusses the characteristics of a research paper and explains how the current paper differs from others. Section 4 talks about security goals in the Cloud-IoT environment. Section 5 discusses the taxonomy related to Cloud-IoT environment which includes Big Data and IoT along with its applications in various domains. Section 6 is a comprehensive study of various attacks in the Cloud-IoT environment. Section 7 (A) explores the study of various research trends through Table 1 . Section 7 (B) describes attack vectors and mitigation strategies through Table 2 . Section 8 presents an in-depth analysis of digital forensics. Section 9 talks about the machine learning and Blockchain technologies-based approaches used for threat detection and recovery. Section 10 covers the current challenges in data security and privacy, and it provides a brief description of possible solutions listed in Table 3 . Further, this section highlights the research gaps identified by the author in Table 4 . Conclusions and future research work are discussed in Sect. 11 .
2 Methodology: a systematic approach
The methodology is the systematic approach that is used by the author to conduct research, analyze the data, and frame conclusions. The methodology section covers the boundary area of methods and approaches that are followed by the author to write the current research paper. The methodology of this research paper is as follows: To examine IoT security challenges and threats author searched numerous kinds of literature on IoT security. For this keyword IoT, Cloud-IoT security was used for standard survey papers that were published in reputed journals like IEEE, Elsevier, Springer, and many more. After completing this task, the author examined numerous techniques and methodologies presented in those survey papers critically analyzed the facts, and algorithms, and selected a set of relevant topics that is important from a security perspective, provided with the help of the author’s individual experience in the sphere of security. In addition to this, the author introduced various standard approaches that are recognized in the sphere of security for protection against threats. At last, the author utilized Internet-based search techniques to find the most appropriate security products. The Methodology of the current paper is divided into three standard stages as follows.
Phase 1 Identification of the study area, formulation of the research questions, sampling, and establishing the primary search approach or standards.
Phase 2 Using the search strategy or criterion about existing literature, carrying out keyword searches, Boolean searches involving the combination of keywords and phrases with the operators “AND”, and “OR”, and database searches, assessing the results, and formulating selection criteria.
Phase 3 Finding and evaluating approved literature, articles, papers, websites, and web documents by the chosen primary research topic.
Figure 1 shows the distribution of references over the year. The figure portrays which year the researcher’s paper was selected to prepare the current paper. The author selected the previous research paper from year 2016 to 2024, a recently published paper. The author reviewed the paper which includes published journal and conference papers. The author searched, examined, and analyzed the paper was included in the references section of the manuscript.
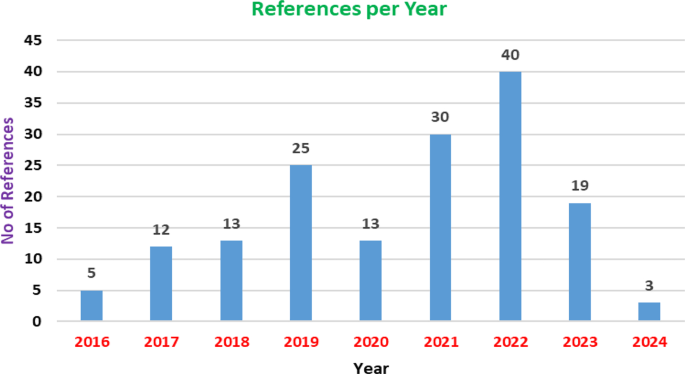
Reference timeline
2.1 Inclusion/exclusion criteria
The inclusion and exclusion criteria aim to identify the research studies that correspond with the questions under investigation. The primary studies were identified using the inclusion criteria that we are presenting. The exclusion criteria were left out since they represent the negative version of the inclusion criteria that were specified.
Inclusion Criteria
IC 1 : Publications released on and after the year 2016.
IC 2 : Publications that have been published in peer-reviewed journals, conferences, workshops, etc.
IC 3 : English-language articles published.
IC 4 : Articles that are required to contain an abstract and title.
IC 5 : Publication that specifically addresses the subject topic such as data security, privacies encryption decryption, machine learning, blockchain, or research problems.
IC 6 : Research with subject-specific keywords included.
IC 7 : Systematic reviews, theoretical analysis, and empirical study.
Exclusion Criteria
EX 1 : Articles that are loosely connected to the research question or do not answer it.
EX 2 : Articles whose complete text cannot be accessed.
EX 3 : Articles not available in English.
EX 4 : Articles that were released almost ten years ago.
EX 5 : Studies with poor ratings or serious methodological errors.
EX 6 : Articles that don’t explicitly address privacy and data security concerns is excluded.
EX 7 : To prevent prejudice from incorporating the same study more than once, remove duplicate publications.
2.2 Algorithms, tools, and techniques Implemented
Alogrithm 1 : RSA (Rivest-Shamir-Adleman), ECC (Elliptic Curve Cryptography), and AES (Advanced Encryption Standard) are a few examples of the particular encryption techniques used in the studies.
Alogrithm 2 : Determine which machine learning techniques—such as anomaly detection methods (e.g., k-means clustering, isolation forests)—are utilized to detect data breaches or to ensure data security.
Tool 1 : To manage and organize references, use programs like Scispace, Citation Gecko, and Open Knowledge Map.
Tool 2 : To create visual representations of the data in MS Excel (graph).
Tool 3 : To check Grammar and Spelling Grammarly software tools are used.
Tool 4 : To draw the picture tools such as Paint, Smart Draw, and Origin-Lab are used.
Tool 5 : iThenticate is used for Plagiarism detection.
Techniques for Search
Technique 1 : Use Boolean operators and specified keywords to search IEEE Xplore, Scopus, and Google Scholar. The following query is an example: “data security” AND “privacies” AND (encryption OR data protection) AND “2016–2024”.
3 Advancing IoT, Big Data, and cloud integration: novelty in current research
In the rapidly evolving landscape of technology, the convergence of the IoT, Big Data, and Cloud computing stands at the forefront of innovation. Each domain, when studied individually, offers significant advancements and benefits. However, the integration of these technologies opens up unprecedented possibilities, presenting both opportunities and challenges. This research work provides the novel aspects of combining IoT , Big Data , and Cloud computing . Further, the paper highlights the transformative impact on various industries and emerging security concerns. This study aims to uncover new insights and propose solutions to ensure the safe and efficient deployment of integrated systems by exploring how these technologies interact. The major contributions of the current research paper are as follows:
Integration of IoT, Big Data, and Cloud Computing : The paper examines the combined effects and security threats of integrating IoT, Big Data, and Cloud computing.
Role Analysis : It offers an in-depth analysis of how IoT, Big Data, and Cloud storage work together.
Data Flow : The paper explores the process where data generated from IoT devices becomes Big Data and is subsequently stored in the Cloud.
Security Threats : It highlights the potential security threats during the transmission and storage of data.
Proposed Protections : The authors propose standard approaches to protect against potential attacks that could compromise the data.
Digital Forensics : The paper discusses digital forensics as a method to preserve and analyze digital data post-attack, aiding in tracing the attacker’s footprint and identifying patterns and trends.
Recent Data Security Technologies : In this research work, the authors addressed new technologies that have the potential to significantly reduce threats in cloud-IoT environments.
Research Focus : Authors determine the researcher’s field of expertise methodically.
4 Security goals in Cloud-IoT environments: a comprehensive overview
Security in Cloud-IoT environments is paramount due to the interconnected nature of devices and the vast amount of sensitive data they generate and process. Ensuring the confidentiality, integrity, and availability of data and services has become a major challenge as cloud computing and IoT devices become more integrated into everyday life and vital infrastructure. In an ever-changing digital ecosystem, this comprehensive overview seeks to explore the major security objectives, difficulties, and tactics that are crucial for protecting Cloud-IoT environments.
Figure 2 shows security objectives in a cloud environment. To guarantee the confidentiality, integrity, availability, and general security of data, applications, and resources hosted in the cloud, security objectives for a cloud environment are essential. These goals assist businesses in defining their security objectives and directing the application of suitable security solutions. For the confidentiality, integrity, and availability of data and services hosted in the cloud, security objectives for the environment are crucial. These goals aid organizations in developing a framework for putting security measures in place and in defining their security objectives. To respond to changing threats and keep a solid security posture in the cloud, it is essential to regularly assess and update security goals and procedures.
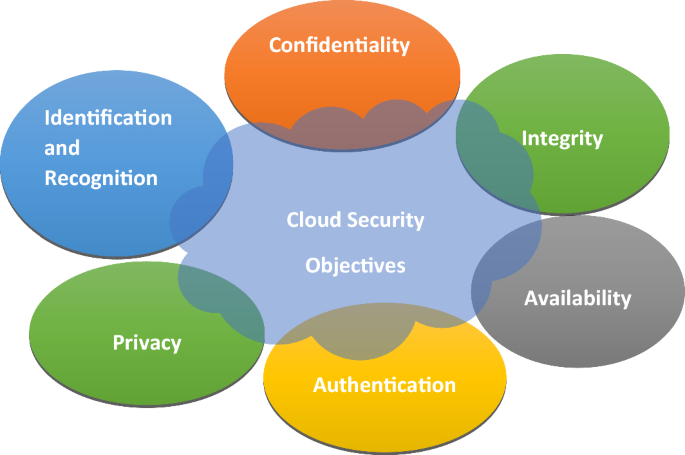
Security objective in cloud environment
Confidentiality Confidentiality refers to safeguarding or protecting critical data from unauthorized access. The information will only be revealed or accessible to those persons who are authorized (Schiller et al. 2022a ).
Identification and Recognition Identification is a unique way to provide attributes to users or devices to differentiate from other users. Recognition is related to the validation of the claimed identity. When a user gives a password, it matches with the saved password and identifies an individual (Schiller et al. 2022a ).
Privac: To safeguard the privacy of individual data, security measures are implemented. It also ensures that data must be responsibly handled. It involves protecting personnel information (Schiller et al. 2022a ).
Authentication: The authentication measures procedure involves confirming the identities of individuals and protecting against unauthorized access. It involves the user providing a username and password (Schiller et al. 2022a ).
Availability Availability refers to the accessibility and usage of data when required by an authenticated person. It involves maintaining availability includes protecting against denial of service, downtime, and disruptions that can hamper the availability of data (Schiller et al. 2022a ).
Integrity Integrity ensures that data should be consistent, accurate, and unchangeable throughout its lifecycle. It also ensures the trustworthiness of the data (Schiller et al. 2022a ).
Case studies that demonstrate how these security goals are implemented in practice are described below:
Estonia’s e-Residency Program: e-Residents receive a government-issued digital ID that is stored on a blockchain. This ID allows them to securely sign documents, access Estonian e-services, and run a business remotely.
MediLedger in Pharmaceutical Supply Chain: MediLedger uses blockchain, a decentralized ledger, to ensure data integrity and transparency.
Civic’s blockchain-based identity verification: It allows users to create and verify digital identities. Further, Enigma uses secure multi-party computation ( sMPC ) on the blockchain to ensure that data can be shared and analyzed without being exposed.
5 Taxonomy of Cloud-IoT environment
In the rapidly growing landscape of the Cloud-IoT environment, understanding the taxonomy is significant for navigating the complexities of connected devices and realizing their full potential in the swift diversification of the Cloud-IoT ecosystem.
5.1 The relationship between IoT, Big Data, and cloud computing
There is a strong synergistic relationship between Cloud Computing, Big Data, and the IoT, with each technology augmenting the other’s capabilities. IoT enables data collection which is uploaded to the cloud for storage and processing. These bulk data are accumulated in the cloud and form a large volume of data known as Big Data. Big Data tools and techniques are applied to these bulk data for processing and scrutiny of data on the cloud. Real-time monitoring and analysis are made possible by the convergence of cloud computing, Big Data, and IoT. This makes it possible to respond and act quickly, which optimizes processes, boosts productivity, and enhances user experiences.
Figure 3 illustrates the relationship between the IoT devices that are placed at remote locations. Data is generated from IoT devices which are stored and analysed on the cloud using Big Data tools. Finally, after processing data on the cloud decision is made. IoT, Big Data, and cloud computing work together to create a potent trio that propels efficiency and innovation in a wide range of sectors, including manufacturing, agriculture, smart cities, and healthcare.
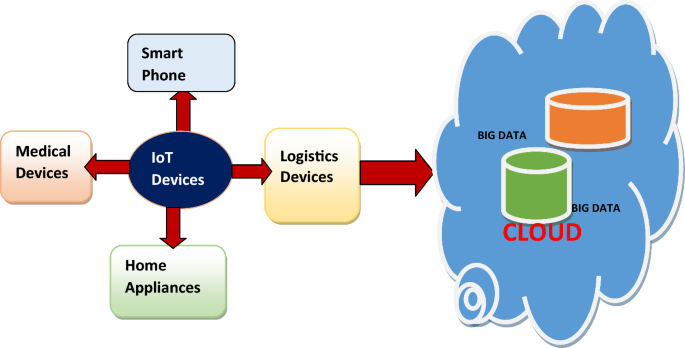
Relationship between IoT, Big Data, and cloud computing
5.1.1 Understanding the dynamics of Big Data
Big Data in a few years come out as an ideal that has provided an enormous amount of data and provided a chance to enhance and refine decision-making applications. Big Data offers great value and has been considered as being a driving force behind economic growth and technological innovation (Dutkiewicz et al. 2022 ). Machines and humans both contribute to data through online records, closed-circuit television streaming, and other means. Social media and smartphones create enormous amounts of data every minute (Ram Mohan et al. 2018 ). Big Data is a large amount of data that is fast and complex. These data are not easy to process using conventional methods. Today Giant Companies substantial portion of the value advanced from data generated by the company which is continually examined to produce better and advanced products. A prime example of Big Data is the New York Stock Exchange, which creates one terabyte of fresh trade data daily. Big Data characteristics are defined by the 4 V’s i.e. Volume, Variety, Velocity, and Veracity which is shown in the figure below. Big Data involves three main actions integration, managing, and analysis.
Figure 4 A and B illustrate the essential 4 V’s i.e. Variety, Volume Velocity, and Veracity of Big Data through 4 blocks. Volume block represents the size of data that grows exponentially such as Peta byte, Exa byte, etc. It represents how much information is present. The volume of data is increasing exponentially. Velocity block shows that data is streaming into the server for analysis and the outcome is only useful if the delay is short. It is used to portray how fast information can be available. Data must be generated quickly and should also be processed rapidly. For example, a healthcare monitoring system in which sensors record the activities that occur in our body and if an abnormal situation occurs needs a quick reaction. Variety blocks represent, a variety of data and various formats, types, and structures of data that exist such as sensor data, PDF, photo, video, social media data, time series, etc. The veracity block ensures that data should be consistent, relevant, and complete in itself. Hence, the error can be minimized accurate results can be produced and decisions can be taken through analysis of the result.
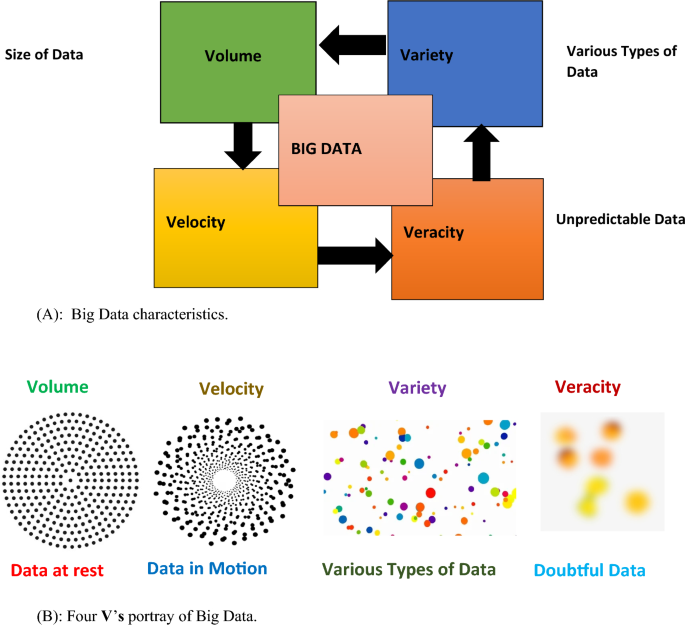
A Big Data characteristics. B Four V’s portray of Big Data
Apart from its several advantages Big Data faces security challenges as well such as attackers can damage or steal information where a large volume of data is stored such as cloud and fog. An attacker can steal data and he/she can attempt to study and analyze data and thereafter can change the outcome of the result accordingly. Therefore special protection and privacy of data such as cryptographic defense mechanisms should be provided so that data can be kept safe and secure (Kaaniche and Laurent 2017 ). The healthcare industry is one of the most promising areas where Big Data may be used to effect change. Large-scale medical data holds great promise for bettering patient outcomes, anticipating epidemics, gaining insightful knowledge, preventing avoidable diseases, lowering healthcare costs, and enhancing overall quality of life. To address security and privacy threats in healthcare, the author has provided some suggested strategies and approaches that have been documented in the literature, while also outlining their drawbacks (Abouelmehdi et al. 2018 ).
5.1.2 Connecting the world: the evolution and impact of the Internet of Things
The development of the Internet of Things has revolutionized the Internet market around the world. The Internet of Things is a device that when connected to the Internet transmits, receives, and stores data over the cloud. The Internet of Things is embedded with several devices such as sensors, physical devices, and software to control the devices. IoT can be device can include anything that contains a UID (Unique Identification Number) that can be used to in identify uniquely over the internet. IoT devices have several benefits such as high efficiency, providing more business opportunities, high productivity, increased mobility, and many more. Apart from the above-mentioned benefits, IoT devices can also be deployed to monitor tool execution and find and diagnose the issues before any major break happens in the functioning of the device, also in addition it reduces maintenance costs and thereby increases the throughput. IoT devices can able to gather large volumes of data beyond any human can think of it. As the world is developing data is considered to be an oil for the development of any country, so to cope with new challenges IoT devices should also be made smarter than traditional devices which can able to guide and make decisions. To achieve such objectives IoT devices should be accompanied by machine learning and artificial intelligence technology to enhance the performance of the device and to make sense of collected data.
Figure 5 illustrates the key components of IoT devices. The components are the building blocks of IoT which is shown in the diagram. These “DGCAU” components collectively facilitate the working of the IoT devices. Each component is significant in terms of productivity, data collection, monitoring, and connectivity. In Fig. 5 ‘D’ stands for IoT Device. IoT devices are those which are such as medical equipment, smart meters, home security systems, smart lights, etc. which are used to collect data. The second ‘G’ stands for Gateway which is similar to a centralized hub that is used to interconnect IoT devices and sensors to the cloud. Advanced gateway facilitates data flow in both directions between IoT devices and the cloud. ‘C’ indicates the cloud aids in the storage of data and simultaneously analyzing data. Rapid processing and strong control mechanisms enable cloud-enabled IoT devices to minimize the risk of attack. User identities and data are protected by strict authentication methods, encryption tools, and biometric authentication in Internet of Things devices. ‘A’ signifies the Analysis of data that was stored in the cloud to determine the outcome. Analysis tool studies large amounts of data and produces useful information, which is helpful in decision-making. The last component ‘U’ represents the user interface or UI module that facilitates the user to administer the IoT device with which they are interacting. it is generally a graphical user interface that includes a display screen, mouse, keyboard, etc.
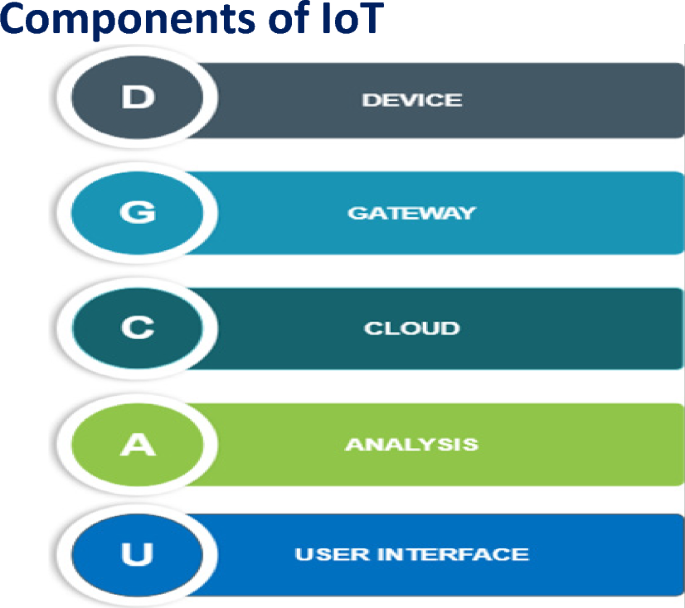
Key components of the Internet of Things
Figure 6 shows the various applications of the IoT which are technology paradigms used to interconnect the devices with the Internet, collect data, share data, transmit data, and act upon data. IoT has enormous application in day-to-day life therefore enabling us to perform our work widely and conveniently . Smart Lighting IoT can be used to operate the light remotely through a smartphone. Transportation IoT is used to track vehicles and goods in real-time. IoT finds application in health which enables doctors to monitor the patients remotely. In Logistics IoT helps to keep track of goods and vehicle devices. IoT is useful for smart framing because IoT sensors can monitor, measure, and track soil moisture, nutrients needed for crop fertilization, and irrigation needs. IoT devices used in retail monitor the department’s real-time inventory level and stock and forward orders when a product is discovered to be out of stock. With features like motion sensors, doorbell cameras, and video surveillance , smart home security systems employ the Internet of Things to monitor and secure houses. IoT is used by smart grids to increase the effectiveness and dependability of electricity delivery. Water quality indicators like pH, turbidity, chlorine levels, and pollutants are continuously monitored by IoT sensors. Smart meters with IoT capabilities allow for real-time monitoring of utility consumption. IoT equipment on autonomous vehicles processes sensor data in real time. This entails reading and assessing the environment to make deft choices regarding safety, navigation, and vehicle control. Wearable gadgets gather information on activities, health, and other topics before sending it for analysis to smartphones or the cloud for processing.
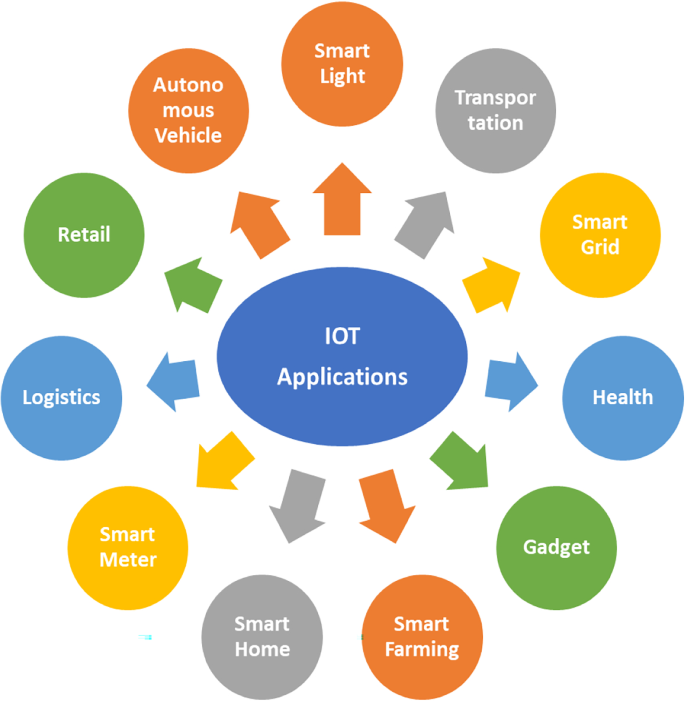
Internet of Things applications
Apart from the benefits of IoT devices in day-to-day life, IoT devices suffer security threats as well. The rapid growth of IoT devices has revolutionized how we interact with technology. As the number of IoT devices increases the security concern also increases simultaneously. The author addresses the issue of sharing sensitive data securely for designated recipients in the context of the Blockchain Internet of Things (B-IoT) (Yin et al. 2022 ). The author has scrutinized the security flaws in computer systems based on cloud, blockchain, IoT, and fog computing (Mishra et al. 2022 ; Yao 2022 ; Abdulkader 2022 ). Security challenges and threats in IoT and cloud environments addressed by various authors are presented in the papers (Pandey et al. 2023 ; Ray and Dutta 2020 ; Bedi et al. 2021 ). Cloud Computing and IoT Using Attribute-Based Encryption approaches are developed by authors found to be very effective in the security domain (Mihailescu et al. 2022 ; Henze et al. 2017 ). The author presents D-CAM, a solution for achieving distributed configuration, authorization, and management across borders between IoT networks (Simsek 2023 ). The study presented by the author is a novel handshake protocol for the broker-based publish/subscribe paradigm in the Internet of Things that offers key exchange-based authentication, authorization, and access control (Shin and Kwon 2020 ; Stergiou et al. 2018 ).The goal of a systematic literature review (SLR) paper is to examine the body of research on cloud computing security, risks, and difficulties that are presented by authors (Wang 2021 ). The primary issue in the cloud environment has been confirmed to be data access, despite the security measures being deemed dependable (Javid et al. 2020 ; Gai et al. 2021 ; Shukla 2022 ). We suggested an effective data access control method that uses optimal homomorphic encryption (HE) to get around this issue (Gnana Sophia et al. 2023 ). The paper highlights the edge computing security and privacy requirements (Yahuza et al. 2020 ). Multiple encryption techniques are presented by the authors which are significant in protecting privacy and data security (Sharma et al. 2019 ; Silva et al. 2018 ; Bertino 2016 ). The author proposes a distributed machine learning-oriented data integrity verification scheme (DML-DIV) to ensure the integrity of training data (Zhao and Jiang 2020 ). The researcher introduced an identity-based (ID-based) RDIC protocol including security against a malicious cloud server which is presented in the paper (Yu et al. 2017 ; Sookhak et al. 2018 ). The authors studied various security challenges concerning IoT devices, Big Data generated by IoT devices, and cloud and presented them in the paper (Akmal et al. 2021 ; Awaysheh et al. 2022 ; Tang 2020 ; Shi 2018 ).
5.2 Navigating the cloud: exploring the world of cloud computing
Cloud Computing refers to Internet-based computing, where shared resources data, software, and information are to the customer and devices on demand. The term “cloud” used to appear on the Internet. Huge memory space and inexpensive, high-performance computing are made possible by the cloud computing paradigm. Users can get cost savings and productivity benefits to manage projects and develop collaborations by moving their local data management system to cloud storage and utilizing cloud-based services. Information and knowledge extraction is greatly aided by computing infrastructure, particularly cloud computing. The services for cloud computing are provided using the network, generally the Internet. The characteristics of cloud computing include broad network access, on-demand service, rapid elasticity, and many more. With the help of the cloud, numerous services are accessible to clients. Broadly there are three types of services offered that enable the client to use software, platform, and infrastructure. Several types of cloud can be subscribed to by anyone as per the requirement of an individual or any organization. These include private cloud, public cloud, and hybrid cloud. Private cloud solely owned by any business houses. In this type of cloud infrastructure software is preserved on a private network and hardware and software entirely belong to the organization. Public clouds are commonly cloud services that are allotted to various subscribers. Third-party owned and operated the cloud resource.
The public cloud is mostly used for online office applications, testing, development, etc. A hybrid cloud is a combination of public and private clouds, which is implemented by a couple of interrelated organizations. Common types of cloud services are presented through the 3-layer architecture of Cloud Services in Fig. 7 and each one is discussed.
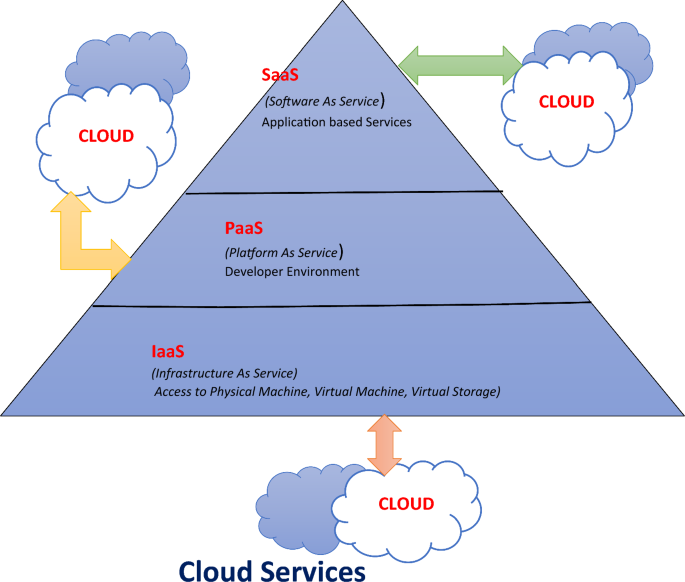
3-Layer architecture of cloud services
Figure 7 exhibits the different types of cloud and services provided by the cloud. The figure conveys the three-layer architecture of the cloud. IaaS makes virtualized computing resources available via the internet, enabling customers to pay-as-you-go access and manage the essential parts of the infrastructure. These resources often include storage, networking, virtual machines, and other things. Platform as a Service (PaaS) is a cloud computing architecture that offers developers a platform and environment to create, deploy, and manage applications. PaaS provides a variety of tools and services that speed up and improve the efficiency of the application development process. A cloud computing approach called Software as a Service (SaaS) allows users to access software programs online. SaaS has many benefits, including affordability, scalability, and accessibility.
Because crucial data is processed and stored on the cloud, for instance in Internet of Things applications, it also poses security and privacy issues (Alouffi et al. 2021 ; Hamzah Amlak and Kraidi Al-Saedi 2023 ; Yu et al. 2022 ). Cloud security is an important area where authors have tried to find the best possible solution through their research they have highlighted the challenges of possible solutions to the problem through finding and investigation in the paper (Gupta et al. 2022 ; Chaowei et al. 2017 ; Wang et al. 2021 ).
To ensure the integrity of data kept in the cloud, the author’s study proposes an effective public auditing technique that makes use of Third third-party auditor (TPA) (Reddy 2018 ; Hiremath and Kunte 2017 ; Yan and Gui 2021 ). The author proposes an efficient certificate-based data integrity auditing protocol for cloud-assisted WBANs (wireless body area networks (Li and Zhang 2022 ). The author proposed a secure architecture by associating DNA cryptography, HMAC, and a third-party auditor to provide security and privacy (Kumar 2021 ; Duan et al. 2019 ). Adversaries are always coming up with new ways to get access to users’ devices and data through developing technologies like the cloud, edge, and IoT. The author discussed various attacks along with security solutions (Pawlicki et al. 2023 ). The paper highlights the research challenges and directions concerning cyber security to build a comprehensive security model for Electronic health records (Chenthara et al. 2019 ; Hou et al. 2020 ; Ishaq et al. 2021 ; Jusak et al. 2022 ). The author mentioned the research and analysis of privacy-preserving data mining (PPDM) and classified using various approaches for data modification in the research paper (Binjubeir et al. 2020 ).
Even with all the benefits mentioned, there are security and privacy issues while using cloud computing (Nanda et al. 2020 ; Himeur et al. 2022 ). The issue of data security and privacy for Big Data is complicated by the use of cloud computing for Big Data management, storage, and applications. Since cloud services are typically offered on a common infrastructure, there is always potential for new attacks, both internal and external, such as password theft or application programming interface (API) flaws. The author has proposed a software architecture model by using approaches like hardware security extensions (Intel SGX) and homomorphic encryption. To improve data security in large data cloud environments and defend against threats, a virtualization design and related tactics are suggested by the author. The TID (Token Identification) model developed by the author provides security to the data. The user has various access rights as a client. The authentication access token establishes a connection with the user account after the user logs into the cloud network. The researchers have developed the Remote Data Checking (RDC) technique, which uses the sampling technique to evaluate the integrity of data that is outsourced across remote servers. Authors developed the techniques for remote data auditing that are very beneficial in ensuring the integrity and dependability of the data that is outsourced. Data, auditing, monitoring, and output these elements are all included in the DAMO taxonomy. The author in his paper offers a unique security-by-design framework for the implementation of BD (Big Data) frameworks via cloud computing (Big Cloud) (Ye et al. 2021 ). Various data security issues in the Big Data cloud computing environment are addressed by the authors in his paper. Various methods for safeguarding privacy and data security in public clouds are covered in the article (Jain et al. 2016 ). A multi-cloud architecture with privacy and data security enabled is suggested by the author. To increase user security on SNg (Social Networking) by utilizing techniques that can give data about BD technology (Big Data) greater privacy. This approach is described by the author in the paper along with various metrics and usage-related outcomes. The author examines financial risk analysis and related regulatory studies using blockchain and Big Data technologies. A secure cloud environment can be achieved by using a hybrid cryptographic system (HCS), which combines the advantages of symmetric and asymmetric encryption.
Figure 8 shows a hierarchical structure created to handle and process data and applications efficiently depending on how close they are to the user or the source of the data. “Hierarchical edge computing” refers to the interplay between these three layers, cloud, fog, and edge. The Cloud Layer is a centralized data processing center that provides abundant computing and storage capacity for handling and storing enormous volumes of data as well as running sophisticated applications. The growths of the Internet and its associated ideas, such as edge computing, cloud computing, and the Internet of Things, have had a permanent impact. The cloud layer is a highly scalable data center that is perfect for managing large-scale applications and services because they can extend horizontally to manage increased workloads.
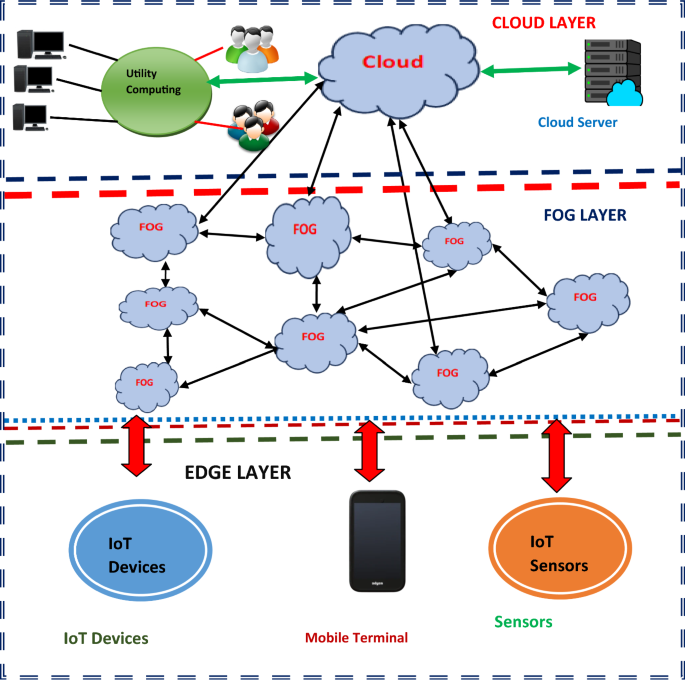
Hierarchical edge computing
The fog Layer is an intermediate layer after the cloud layer which spreads and distributes processing responsibilities among several local servers or devices, which can be very useful for IoT applications with many data sources. Fog computing is ideal for latency-sensitive applications that demand quick responses. Virtual components called cloudlets are employed in fog computing. Fog computing has emerged as a promising paradigm in overcoming the growing challenges (e.g., low latency, location awareness, and geographic distribution) arising from many real-world IoT applications, by extending the cloud to the network edge. To facilitate data offloading and computation, these virtual computers offer a micro data centre close to mobile devices (Lu et al. 2020 ). Fog computing offers new insights into the extension of cloud computing systems by procuring services to the edges of the network. It shortens the time it takes for data to go to the cloud and back by processing it closer to the source. The edge layer, which is frequently located adjacent to IoT medical devices themselves (Muzammal et al. 2018 ), is the one that is nearest to the data source or end users. A promising paradigm that expands on cloud computing capabilities is edge computing. It processes data instantly, allowing for extremely quick replies devices, sensor devices, and industrial machinery, mobile terminals are examples of edge devices that can function autonomously and make decisions in the present without relying on a central cloud infrastructure (Ghaffar et al. 2020 ; Jiang et al. 2016 ). Big Data applications are a risk for cyber security assaults, as these attacks directly affect applications utilized across several sectors, such as Big Data analytics. The authors presented a novel data encryption approach, which is known as Dynamic Data Encryption Strategy (D2ES) to protect and safeguard the data which proves promising in cloud computing. Encrypted data can be obtained by cryptography methods, enabling secure communication links within the networking system. Researchers suggested the blockchain-based Shamir threshold cryptography solution for IIoT (Industrial Internet of Things) data protection. An improved data security in mobile edge computing, the Fine-Grained Access Control mechanism (FGAC) is suggested to guarantee data security during data access (Ahmed et al. 2021 ).To analyze and investigate the data reduction at the fog level, researchers attempted to create a model. This researcher has successfully applied methods including artificial intelligence, principal component analysis (PCA), and the Naïve Bayesian classifier for data reduction.
6 Exploring the complex landscape of Cloud-IoT threats: an in-depth analysis
Security concerns are growing along with the integration of Cloud Computing and the IoT. Numerous dangers and vulnerabilities that might compromise the availability, confidentiality, and integrity of data and services are brought about by the junction of these two technologies. We examine the subtleties, possible effects, and vital necessity of strong security measures to protect against changing hazards in interconnected environments as we delve into the complex nature of Cloud-IoT security concerns in this analysis.
Figure 9 illustrates the numerous types of attacks that can take place in the cloud. These Attacks can harm the cloud service provider as well as cloud customers. The attacker is an individual who attempts to use a cloud infrastructure, platform, or service’s vulnerabilities or flaws for nefarious reasons in the world of cloud computing. Because they frequently house significant data and offer computational resources that may be used for a variety of purposes, such as launching cyber-attacks, stealing confidential information, or causing disruption, cloud systems are very alluring targets for attackers. For different purposes, including data theft, service interruption, or resource exploitation, attackers target cloud environments. To breach cloud systems, attackers use a range of methods and tactics. These attack methods can include insider threats, sniffer attacks password change SQL-Ingestion, Eavesdropping, malware, distributed denial-of-service (DDoS) attacks, phishing, and more (Basit et al. 2021 ; Ullah et al. 2019 ; Jahromi et al. 2021 ).
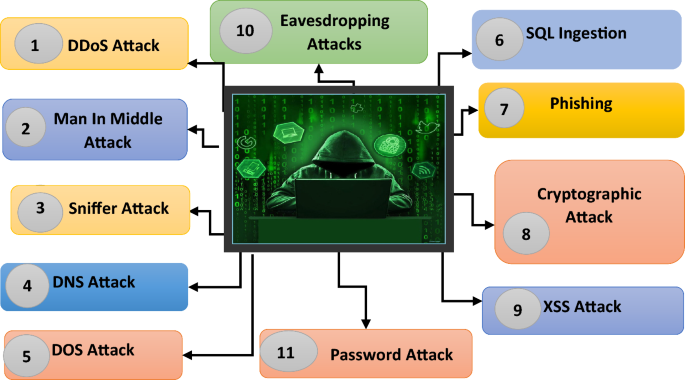
Threats in cloud computing environment
DDOS Attack A distributed denial-of-service attack aims to disrupt regular network operations by flooding the network with traffic. Denial-of-service attacks aim to prevent end users from accessing the network.
Man-in-Middle Attack In a man-in-middle attack, the attacker generally modifies the conversation between the two parties. In a man-in-the-middle attack, attackers generally eavesdrop on sensitive information and alter the conversation. The integrity and security of sensitive data are seriously threatened by MitM attacks.
Sniffer Attack It is an attack in which an unauthorized person intercepts and gains control over network traffic. The goal is to capture and examine the data when it passes over the network.
DNS Attack The domain name system attacks the domain name system, which is responsible for converting human name readable to IP address. DNS attacks have the potential to affect the DNS infrastructure’s availability, integrity, and confidentiality, which could cause interruptions to internet services.
DOS Attack A Denial of Service (DoS) assault involves the exploitation of a single source, typically a compromised device or computer, to overwhelm a target’s resources and cause a loss of service.
SQL Ingestion In SQL (Structure Query Language Ingestion), attackers ingest harmful code inside the parameters of a web application. The main goal of attackers is to manipulate SQL databases. In this type of attack, the attacker gains the advantage of bad input, which enables the attacker to execute the SQL command.
Phishing Attack In Phishing attackers use some trick to expose delicate information, for example, username, personal information, password, and credit card details. Phishing attacks sometimes use the personas of reliable companies, banks, or websites to trick people into doing things that could jeopardize their security.
Cryptographic Attacks Cryptography is important to ensure confidentiality and integrity and authenticate the user. The attacker exploits vulnerability or weakness in the existing system. Attackers compromise the security of cryptographic systems.
XSS Attacks Cross-site scripting (XSS) is one of the serious attacks that occur when vulnerable code which is in the form of a script is injected into the web page of the user. The objective of the attacker is to steal sensitive information about the user by running the scripting code in the user’s browse.
Eavesdropping Attacks Eavesdropping is a kind of attack in which attacker unauthorized person tries to listen to or sniff the conversation between two people and steal information. In this type of attack, the attacker even manipulates the information.
Password Change Request Interception Attack The assailant attempts to intercept legitimate users’ password changes. Interception of this kind could happen during a browser-server conversation.
7 Exploring research trends and areas of focus
As technology continues to evolve at a rapid pace, researchers and academics are continually exploring new trends and areas of focus within their respective fields. To keep ahead of new difficulties, seize opportunities, and encourage innovation, this investigation is essential. We explore the current research trends and areas of attention in a variety of disciplines in this overview, offering insight into the cutting-edge subjects that are influencing the direction of technological and scientific advancement. After scrutinizing the number of published research papers we came across various domains in which researchers have worked and proposed various security frameworks.
Table 1 represents the research work and focus of various researchers in field security. From the table above it can be concluded that researcher have focused on Cloud Computing and their finding are more concentrated on Cloud security and the Internet of Things. The researcher primarily focused on the development of security algorithms to protect the data from being damaged or corrupted by cyber attackers. Through study, it was found that researchers have developed innovative techniques by making use of machine learning techniques, and blockchain technology to safeguard data developed for the Internet of Things.
Cryptography is another eminent way to protect our data. Researchers have created algorithms to encrypt and decrypt data prominently so that data can be safely transmitted over the network. A method like PSEBVC: Provably Secure ECC and Biometric Based Authentication Framework is developed by the author as a countermeasure for attacks.
In the digital landscape, the risks of cyber-attacks are growing enormously which is becoming a challenge for both organizations and individuals. A comprehensive examination of attack vectors and mitigation strategies is essential for understanding and effectively countering these attacks (Wylde et al. 2022a , b ). Through an analysis of numerous attack pathways and related mitigation techniques including artificial intelligence-based solutions discussed in paper (Al Hamid et al. 2017 ; Abed and Anupam 2022 ). This research paper aims to offer important insights on how to enhance security and defend against cyber threats in a constantly changing security environment. The objective of this analysis is to provide individuals and organizations with the necessary knowledge and tools to improve their digital security and minimize risks in the constantly changing threat landscape. To do this, each attack mechanism is thoroughly examined, and appropriate remedies are explored through Table 2 .
Table 2 is a complete description of the investigation of the several research papers related to security threats that exist, various categories of attackers that occur on the cloud, and countermeasures that can be taken to prevent attacks summarized in the Table by the author. The table shows how attacks affect the data and what standard approaches were developed by researchers to protect data.
8 Unveiling the intricacies of digital forensics in Cloud-IoT environments
Digital Forensics is a branch of forensic science that concentrates on recovery of data, analysis of data and exhibit the digital evidence that is found on electronic devices. The IoT Forensics can be identified as part of Digital Forensics. The objective of IoT Forensics is to explore digital information in an authorized manner. IoT forensics data can be accumulated through IoT devices, sensors, networks, and cloud. There are some differences between security, IoT, and forensics. The protection against physical and logical security threats is provided by IoT security adopts multiple methods to protect from threats and minimize attacks (Unal et al. 2018 ). Forensics examines the data present in the devices and recreates the happenings by utilizing investigative methods to preserve and analyze digital data. Post-mortem examinations are the main focus of forensics i.e. discovering shortcomings that emerged from the event. Forensic experts obtain digital proof throughout the actual event with the help of standard approaches used in forensic analyses of physical proofs of electronic data to determine and reframe the events by storing and analysis of digital information using different methods of investigation. Some authors have presented detailed studies to investigate the forensic issues in cloud computing and provide possible solutions, and guidelines, including existing case studies (Morioka and Sharbaf 2016 ; Al-Dhaqm et al. 2021 ). The paper offers an enhanced blockchain-based IoT digital forensics architecture that builds the Blockchain’s Merkle tree using the fuzzy hash in addition to the traditional hash for authentication (Mahrous et al. 2021 ). Authors Almutairi and Moulahi ( 2023 ) trained models locally using federated learning on data stored on the IoT devices using a dataset created to simulate attacks in the IoT environment. In order to make the blockchain lightweight, the authors next carried out aggregation via blockchain by gathering the parameters from the IoT gateway (Almutairi and Moulahi 2023 ).
The IoT has revolutionized various sectors through seamless device interactions, yet it has introduced significant security and privacy challenges. Traditional security measures often fall short due to IoT’s distinct characteristics like heterogeneity and resource limitations. Danish Javed et al. ( 2024a ) explored the synergy of quantum computing, federated learning, and 6G networks to bolster IoT security. Quantum computing enhanced encryption, while federated learning preserved data privacy by keeping training data on local devices. Leveraging 6G’s high-speed, low-latency capabilities allows for secure, real-time data processing among IoT devices. The study also reviewed recent advancements, proposed a framework for integrating these technologies, and discussed future directions for IoT security. Recent innovations in network communication have revolutionized the industrial sector with automatic communication through the Industrial Internet of Things (IIoT) . Despite its benefits, the increased connectivity and use of low-power devices in IIoT heighten vulnerability to attacks, and its diverse nature complicates centralized threat detection. To tackle this, authors Javed et al. ( 2023 ) proposed a fog-based Augmented Intelligence (IA) defense mechanism that uses GRU and BiLSTM deep learning classifiers for anomaly detection and secure communication. This framework (Cu-GRU-BiLSTM), which achieved up to 99.91% accuracy, surpassed existing threat detection methods, proving its effectiveness for securing IIoT environments (Javeed et al. 2023 ).
Further, the hybrid approach proposed by Danish Javed et al. ( 2024b ) enhances intrusion detection in federated learning (FL) for IoT by addressing existing limitations. Here, CNNs identify local intrusion patterns by extracting spatial features, while BiLSTM captures sequential patterns and temporal dependencies. Using a zero-trust model, data stays on local devices, and only the learned weights are shared with the centralized FL server. The server then combines updates to improve the global model’s accuracy. Tests on CICIDS2017 and Edge-IIoTset datasets show this method outperforms centralized and federated deep learning-based IDS.
9 Advancements in security threat detection and avoidance
With the constant advancement in sophistication of cyber attacks, enterprises, and individuals alike are obliged to use innovative methods and technologies to detect, prevent, and mitigate potential security breaches. Threat detection is seeing tremendous breakthroughs, enabling defenders to keep one step ahead of malicious actors. These advancements include machine learning algorithms and behavior analysis methodologies. This ongoing change emphasizes how crucial it is to take preventative action to protect sensitive data and maintain digital trust in an environment where dangers are becoming more complicated.
9.1 Harnessing the power of machine learning
Machine learning is a subset of artificial intelligence (AI) that focuses on developing models and algorithms that enable computers to learn from data and make decisions or predictions without having to be explicitly programmed to do so. As a result, machine learning algorithms are beneficial when dealing with vast amounts of data since, after being trained on the data (Ali et al. 2020 ), the trained model uses its learning experience to present precise outcomes on new data. Data generated by IoT devices may suffer from threats (Safaei Yaraziz et al. 2023 ). Today Machine Learning proves to be one of the strongest tools to identify threats and maintain the integrity of data in transmission. The foundation of machine learning is the algorithms that are used to train the models. The first step in using machine learning to address a problem is gathering data. Next come tasks like data preparation, data analysis, training, testing, and eventually deploying the model for real-world application. Two types of ML problems can be solved by supervised machine learning algorithms: regression and classification. Classification is used to solve problems with binary target variables ( yes / no ), while Regression ML algorithms are used to address problems of similar nature when the target variable is continuous. A phishing attack has become one of the most prominent attacks faced by internet users, and governments. The attacker(s) transmits URL(s) to the intended victims via text messaging, social networking, or spam messages. They do this by mimicking the behavior of authentic websites when creating website pages. Malware attack during data in transit is a common type of attacks that can manipulate the data and damage the data. To prevent such attacks ML model can be one of the tools to identify such attacks and prevent them to such extents. Machine Learning algorithms have been used to build several intrusion detection systems, improving the systems’ ability to identify threats and enabling uninterrupted business operations (Pathak et al. 2023 ). Despite many benefits that SDN(Software-Defined Networking) offers such as offer nimble and adaptable network growth, malicious attacks that can eventually prevent network services are unavoidable (Unal et al. 2018 ). Machine learning has been used in several studies to detect distributed denial of service (DDoS) threats in SDN (Software-Defined Networking) environments (Morioka and Sharbaf 2016 ). ML models are being trained on numerous datasets to build models that can detect cloud attacks with elevated accuracy. Various classifier is implemented in the ML model to identify attacks such as SVM, Decision tree, K-NN (K-Nearest Neighbour), Convolutional Neural Networks (CNNs), Recurrent Neural Networks (RNNs), random forests, and many more. The use of random forest and K-NN classification approaches enables malware detection method proofs to be 99.7% accuracy and 99.9% in several cases (Abed and Anupam 2022 ; Morioka and Sharbaf 2016 ). These classifiers can be used with different feature engineering and feature selection strategies to create machine learning models that effectively handle certain security issues and enhance overall cyber security posture.
Figure 10 represents security threat detection using machine learning algorithms and models. The automatic detection of potential security threats and abnormalities within a file system using machine learning techniques uses a predictive model to identify the threat and classifies it as a malware file or harmless file over the system. Data breaches and other security problems can be prevented because of their ability to assist enterprises in detecting and responding to threats more quickly and effectively. In this process, it involves two important stages. The first stage is the Training stage where the model is being trained using different files. Files are sent as input to train the model. Numerous machine learning algorithms, such as decision trees, random forests, support vector machines (SVM), neural networks, and others, can be used for training the model. After the model is trained, then comes to the security stage where an unknown file is given to the model for analysis the file. For the detection of security threats, supervised learning techniques like classification and regression are frequently used. The machine learning model generates notifications for security professionals to investigate when it spots a potential security danger or abnormality. Automated responses to lessen or control the crisis may also be triggered based on how serious the threat is. Deep learning approach is used to detect pirated software and malware-infected files across the IoT network. Using color picture visualization, the deep CNN is utilized to identify harmful infections in Internet of Things networks. Secure video transmission over the cloud is discussed in the paper (Hossain et al. 2018 ). Researchers have developed Holistic Big Data Integrated Artificial Intelligent Modelling (HBDIAIM) to provide and improve privacy and security in data management (Chen et al. 2021 ). The previously developed model falls short in providing adequate data privacy and security, keeping this shortcoming in mind author (Yazdinejad et al. 2024a ) developed an Auditable Privacy-Preserving Federated Learning (AP2FL) model tailored for electronics in healthcare. AP2FL model provides secure training and aggregation processes on the server side as well as the client side. Thereby protecting and minimizing the risk of data leakage. Researchers primarily focus on Machine learning-based threat detection models to address the challenges within Consumer IoT. Using Federation Learning (FL) techniques data privacy in Consumer IoT is maintained (Namakshenas et al. 2024 ). The author suggests an approach to attack detection that makes use of deep learning (DL) algorithms to identify false data injection (FDI) assaults (Sakhnini et al. 2023 ). In the research paper, the author utilizes federated learning to automatically search for threats in blockchain-based IIoT (Industrial Internet of Things) networks using a threat-hunting framework we call block hunter (Yazdinejad et al. 2022 ).
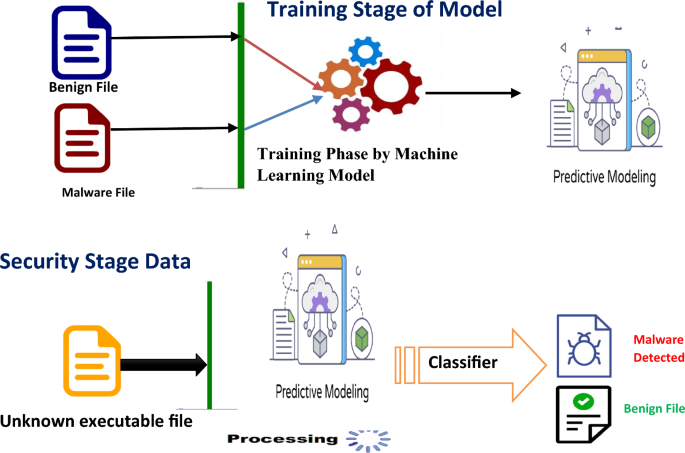
Security threat detection using machine learning technique
Real-life applications of machine learning in malware detection
AT&T Uses machine learning to protect networks and find malware that targets telecom infrastructure.
Mayo Clinic A healthcare organization that implements machine learning techniques to safeguard patient data from malware attacks and unauthorized access.
Bank of America Employs AI and machine learning to improve cyber security safeguards, identifying malware and averting breaches in data.
Cylance A cyber security firm that heavily relies on machine learning to identify and eradicate malware. To identify threats instantly, its algorithm is trained on an extensive dataset of both malicious and benign files.
Amazon Web Services (AWS) AWS uses machine learning techniques to identify threats, examining the logs and network traffic.
Symantec An American consumer-based software company that employs machine learning techniques to identify and categorize malware.
National Security Agency (NSA) To improve national cyber security, the National Security Agency (NSA) uses cutting-edge machine learning algorithms to identify and analyze malware.
9.2 Unlocking the power of blockchain: a cutting-edge safeguard technique for enhanced security in the digital landscape
Blockchain is an emerging decentralized technology that securely stores and authenticates transactions across a network of computers. Its decentralized and open structure makes it a viable option for many companies looking to improve digital security, efficiency, and trust. Although cloud computing is becoming more and more popular for processing and storing data, security, and privacy are still big issues because of the possibility of hostile assaults on wireless and mobile communication networks. Data transfer privacy and system security are improved by using blockchain technology. To put it briefly, a blockchain is auditable, can function as a distributed ledger with digitally signed data, and allows changes to be tracked back to the original data to ensure security. This demonstrates that the security of data may be guaranteed by blockchain technology (Safaei Yaraziz et al. 2023 ). The suggested IAS protocol is developed on top of blockchain technology to guarantee the security and authenticity of data transmission in cloud computing. A potential solution to the security and privacy problems in the Internet of Things is blockchain technology (Williams et al. 2022 ; Waheed et al. 2020 ). For every transaction including proper authentication, data can pass through the blockchain distributed ledger thanks to blockchain technology, which does away with the idea of an IoT central server. Blockchain technology could provide a more effective answer to the issues that IoT systems confront. Transactional privacy, decentralization, the immutability of data, non-repudiation, transparency, pseudonymity, and traceability, as well as integrity, authorization, system transparency, and fault tolerance, are the primary security features of blockchain technology. The Smart contact is verified, put into use, and then shared as a Distributed Ledger Technology (DLT) over a Pier-to-Pier (P2P) network as a function of blockchain (Wylde et al. 2022b ). The authors created and put into use smart, secure fuzzy blockchain architecture. This framework makes use of a unique fuzzy DL model, improved adaptive neuro-fuzzy inference system (ANFIS)-based attack detection, fuzzy matching (FM), and fuzzy control system (FCS) for network attack detection (Yazdinejad et al. 2023 ).
Figure 11 illustrates the specification of blockchain technology concerning cloud environment. Blockchain is a distributed ledger across a peer-to-peer network. Blockchain features can help cloud services reach their full potential and address the many problems that arise. A collection of connected building blocks that are coupled and arranged in an appropriate linear sequence is used to keep a detailed record of all transactions. Decentralization, Security, transparency, availability, traceability, and many more are the essential features of blockchain technology which is highlighted by the figure presented by the author.
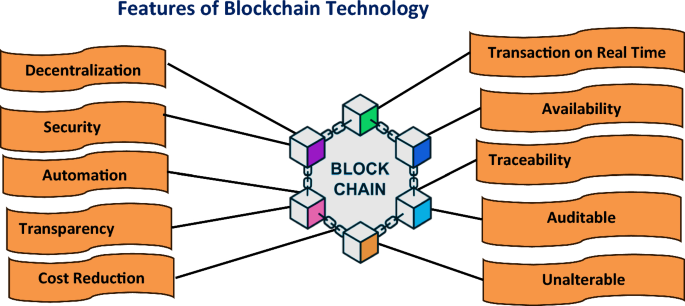
Specifications of blockchain technology
Decentralization Decentralization in blockchain technologies refers to the dividing of control and decision-making across the network users instead of concentrating on the centralized entity. It addresses the limitations of a centralized system in which security is compromised.
Security The network architecture of blockchain technology provides security by minimizing the risk of failure. The allocated characteristics of blockchain strengthen the security. Attacks on any nodes are less likely to put the entire network at risk.
Automation An intelligent system automates the carrying out of the consensus, and removes the requirement of human intervention. Smart contact enhances transaction efficiency. It automatically implements the terms and conditions of the agreement whenever conditions or terms are fulfilled.
Transparency The transactions made on blockchain appeared to every participant over the network. The method not only provides trust and security to the data but also promotes accountability which helps to gain the faith of the user.
Cost Reduction In conventional systems, the settlement of financial transactions might take several days, causing delays and capital lockups. Blockchain eliminates the need for drawn-out clearing and settlement procedures by enabling very immediate transaction settlement.
Transaction in Real Time Transactions in real time can be made over the network. Real-time transaction implements techniques like Proof-of-stake to attain quick acknowledgment of transactions. This technique permits fast agreement among nodes on the validity of transactions.
Availability Transaction availability guarantees a user’s ability to communicate with the network and complete transactions dependably. Availability may still be impacted by network maintenance, upgrades, and sporadic problems.
Traceability Traceability features of blockchain enable to provide of transparent transactions. The transaction can be can be traced by the user. Blockchain is helpful in industries where the origin, transportation, and ownership of assets need to be accurately recorded and validated because of its traceability capabilities.
Auditable Real-time transaction auditing is made possible by the blockchain ledger’s transparency and immutability. At any time, participants can check the transaction history.
Unalterable The ability of blockchain to keep a safe and impenetrable record of transactions is one of its unchangeable features. Once information is posted to the blockchain, it is impossible to change, guaranteeing the information’s integrity and immutability.
Figure 12 portrays the basic components of blockchain. These components work in agreement to form a secure ledger system. Blockchain technology comprises those elements that work in agreement to formulate a secure and decentralized ledger. Supply chain management, decentralized applications, voting systems, healthcare, and property registration are the major applications of technology. Each component plays an important role in blockchain functioning.
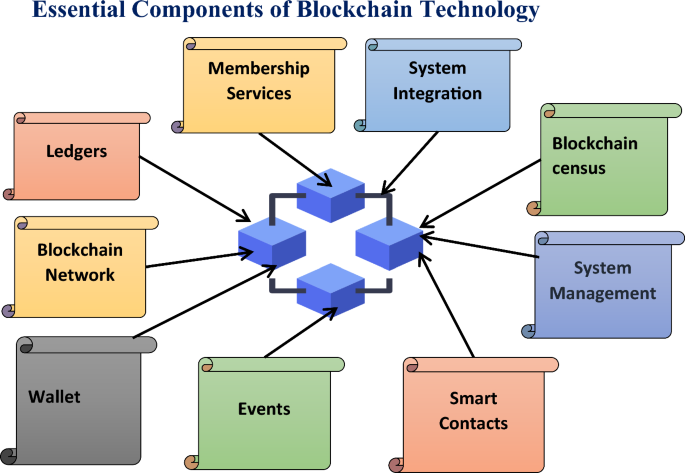
Basic building blocks for blockchain
Ledgers Ledgers in blockchain technology are used to maintain transparency of the record in transactions. Every node contains a replica of the complete ledger, protecting it from being altered or any kind of fraud. The ledger with the help of a chain of blocks carries out transactions; blocks represent every transaction in ledgers.
Blockchain Network In a Blockchain network, the user is referred to as nodes. All the users collectively validate the transaction and record the transaction in a synchronized manner. Blocks are depositors for a cluster of transactions. Blocks contain a timestamp, which is of location of preciously occurred transactions and a cryptographic has for the current blocks.
Wallet Blockchain technology wallets are tools that let users manage and store funds safely. It enables users to access the public and private keys, facilitating the blockchain’s ability to transfer and receive crypto-currency. There are two types of wallets Hot Wallets: Easy for frequent transactions and internet-connected. Cold wallets are offline and thought to be safer for storing money over time.
Events Events are essential for improving the automation, transparency, and usability of blockchain systems. They give decentralized networks a way to communicate and update in real time. The execution of smart contracts or modifications to the ledger’s current state is frequently linked to blockchain events.
Smart Contacts The blockchain records the complete history of smart contract execution, making it transparent and auditable. It has numerous applications such as in supply chain, finance, etc. Based on predetermined criteria, smart contracts carry out actions.
System Management Blockchain technology’s system management characteristics include a variety of operations and procedures meant to guarantee the safety, effectiveness, and appropriate operation of the blockchain network. These characteristics are essential to preserving the dependability and integrity of decentralized systems.
Blockchain Census The blockchain’s consensus techniques, like Proof of Work (PoW) and Proof of Stake (PoS), help make the system resistant to censorship. By requiring a distributed agreement from all network users, these mechanisms make it more difficult for one party to control or restrict transactions.
System Integration Establishing seamless connectivity between different blockchain networks and between blockchain technology and traditional systems is the aim of blockchain system integration. The successful communication and information sharing between diverse systems is greatly dependent upon standards, protocols, and APIs (Application Programming Interfaces).
Membership Services Membership services features of blockchain technology a functions and features for a member or participant management in a blockchain network. It is used to manage access control, rights, and user identity on the network. The elements of the blockchain ecosystem enhance its overall security, governance, and usefulness.
Figure 13 shows the internal workings of the blockchain technology which is used to perform any kind of transaction over the cloud securely. The figure above is a step-by-step explanation of how the transaction takes place over the cloud. In step 1 , first of all, the transaction is generated by any one of the users and request is the directed to the server for processing further. In step 2 , the server after receiving the transaction request creates a block that can appear for the transaction. Next step i.e. step 3 a chain or interconnect block is created using algorithms to authenticate the user and ensure that the request is being made by the authenticated user. Further, in step 4 , this block is distributed to other users or groups of users to grant permission for the transaction to happen. Once the group of users grants permission the transaction or block will be successfully added to the existing blocks that are shown in step 5 in the above figure. If any user disapproves or denies it, then the block will not be added to the existing chain. The modification that has taken place is permanent and cannot be modified further. Therefore, it ensures data security in the cloud environment.
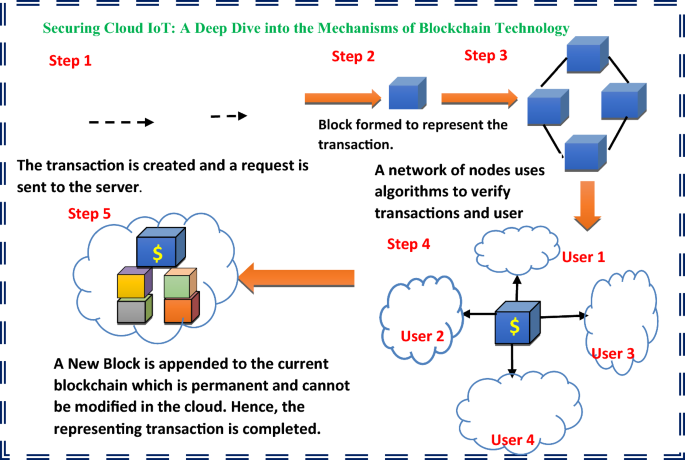
Functioning of blockchain technology in Cloud IoT systems
Real-World Applications of Blockchain Technology in Enhancing Security and Data Protection is as follows:
Walmart Walmart one of the retail companies collaborated with IBM to implement blockchain technology to track the movement of products, maintain food safety, and minimizes the possibility of contamination.
MedRec MedRec is an MIT-developed blockchain-based electronic medical record system that gives individuals more control over their health information while maintaining confidentiality and privacy.Allows for real-time transactions and decentralized energy management by utilizing blockchain to increase the security and efficiency of energy distribution.
Ripple Ripples operates in the financial sector. It uses blockchain techniques to protect the data and enables real-time secure payment.
Follow My Vote Follow My Vote creates a safe, open, and verifiable online voting system using blockchain technology.
uPort uPort is a blockchain-based self-governing identity platform that empowers people to take control of their online personas while improving security and privacy.
10 Unveiling the challenges: addressing current issues in data security and privacy within the Cloud IoT environment
10.1 open ended problems.
The open-ended problems and primary issues about data security and privacy in cloud IoT systems are summarized in Table 3 . Table 3 also provides targeted solutions to address each challenge, thereby ensuring a robust and secure cloud-IoT ecosystem.
10.2 Research gaps
The research gaps of data security and privacy preservation in cloud-IoT technologies are described in Table 4 .
11 Conclusions
The IoT is on the verge of substantial expansion, necessitating secure data transfer and robust cloud storage solutions. As IoT devices become more widespread, the need for enhanced cloud security is critical. Current methods, while helpful, do not fully address modern threats, thus requiring the development of more advanced protective systems. Manufacturers can improve security by creating products grounded in a detailed assessment of IoT security risks and objectives. Effective measures include the implementation of strong authentication methods like One Time Password (OTP) features and robust cryptographic systems. While Machine Learning (ML) is widely used for data protection in various sectors, it faces challenges such as scalability issues with small data sets. Integrating ML with homomorphic encryption shows promise but needs further development. The evolving sophistication of hackers compels reliance on ML and AI for defense strategies. Additionally, blockchain technology, supported by platforms like Ethereum and Hyper-ledger Fabric, offers considerable potential for enhancing security, though more research is necessary to standardize these techniques.
The authors recommend three key solutions:
Develop new security standards and frameworks for cloud-based and IoT devices to tackle modern security challenges.
Create more efficient ML models for real-time attack prediction.
Design robust privacy protection protocols for blockchain technology to safeguard sensitive data.
The authors encountered several limitations during their research, including restricted access to relevant literature, challenges in avoiding plagiarism, difficulties in summarizing a large body of research, integrating information logically, and keeping up with the latest studies.
Data availability
The data and material used in this paper are appropriately referred to and described in this paper.
Code availability
The source code/custom code/software application will be provided when required.
Abdulkader ZA (2022) Cloud data security mechanism using the lightweight cryptography. Optik 271:170084
Article Google Scholar
Abdulsalam YS, Hedabou M (2021) Decentralized data integrity scheme for preserving privacy in cloud computing. In 2021 International conference on security, pattern analysis, and cybernetics (SPAC), Chengdu, China, pp 607–612
Abed AK, Anupam A (2022) Review of security issues in the Internet of Things and artificial intelligence-driven solutions. Internet Technol Lett. https://doi.org/10.1002/spy2.285
Abouelmehdi K, Beni-Hessane A, Khaloufi H (2018) Big healthcare data: preserving security and privacy. J Big Data 5:1
Ahmed W et al (2021) Security in next generation mobile payment systems: a comprehensive survey. IEEE Access 9:115932–115950
Ahmad W, Rasool A, Javed AR, Baker T, Jalil Z (2022) Cyber security in IoT-based cloud computing: a comprehensive survey. Electronics 11:16
Akmal M, Syangtan B, Alchouemi A (2021) Enhancing the security of data in cloud computing environments using Remote Data Auditing. In: 2021 IEEE 6th International conference on innovative technology in intelligent system and industrial applications (CITISIA), Sydney, Australia, pp 1–10
Alabdulatif A, Thilakarathne NN, Kalinaki K (2023) A novel cloud enabled access control model for preserving the security and privacy of medical Big Data. Electronics 12:2646
Albugmi A, Alassafi MO, Walters R, Wills G (2016) Data security in cloud computing. 2016 Fifth International conference on future generation communication technologies (FGCT), London, UK, pp. 55–59
Al-Dhaqm A et al (2021) Digital forensics subdomains: the state of the art and future directions. IEEE Access 9:152476–152502
Al Hamid HA, Rahman SMM, Hossain MS, Almogren A, Alamri A (2017) A security model for preserving the privacy of medical Big Data in a healthcare cloud using a fog computing facility with pairing-based cryptography. IEEE Access 5:22313–22328
Ali J, Roh BH, Lee B, Oh J, Adil M (2020) A machine learning framework for prevention of software-defined networking controller from DDoS attacks and dimensionality reduction of Big Data. In: 2020 International conference on information and communication technology convergence (ICTC), Jeju, Korea (South), pp 515–519
Almutairi W, Moulahi T (2023) Joining federated learning to blockchain for digital forensics in IoT. Computers 12:157
Alnaim AK, Alwakeel AM (2023) Machine-learning-based IoT–edge computing healthcare solutions. Electron MDPI 12:1027
Google Scholar
Alouffi B, Hasnain M, Alharbi A, Alosaimi W, Alyami H, Ayaz M (2021) A systematic literature review on cloud computing security: threats and mitigation strategies. IEEE Access 9:57792–57807
Alrasheed SH, Aiedalhariri M, Adubaykhi SA, El Khediri S (2022) Cloud computing security and challenges: issues, threats, and solutions. In: 2022 5th Conference on cloud and Internet of Things (CIoT), Marrakech, Morocco, pp 166–172
Alzoubi YI, Ahmad AAA, Jaradat A (2021) Fog computing security and privacy issues, open challenges, and blockchain solution: an overview. Int J Electr Comput Eng (IJECE) 11(6):5081–5088
Andrew J, Karthikeyan J (2019) Privacy-preserving Internet of Things: techniques and applications. Int J Eng Adv Technol (IJEAT) 8(6):3229
Arora A, Khanna A, Rastogi A, Agarwal A (2017) Cloud security ecosystem for data security and privacy. In: 2017 7th International conference on cloud computing, data science & engineering - confluence, Noida, India, IEEE, pp 288–292
Atiewi S, Al-Rahayfeh AA, Almiani M, Yussof S, Alfandi O, Abugabah A, Jararweh Y (2020) Scalable and secure Big Data IoT system based on multifactor authentication and lightweight cryptography. IEEE Access 8:113498–113511
Awaysheh FM, Aladwan MN, Alazab M, Alawadi S, Cabaleiro JC, Pena TF (2022) Security by design for Big Data frameworks over cloud computing. IEEE Trans Eng Manage 69(6):3676–3693
Ayofe Azeez NA, Vyver CVD (2019) Security and privacy issues in e-health cloud-based system: a comprehensive content analysis. Egyptian Inform J 20(2):97–108
Basit A, Zafar M, Liu X et al (2021) A comprehensive survey of AI-enabled phishing attacks detection techniques. Telecommun Syst 76:139–154
Bedi RK, Singh J, Gupta SK (2021) An efficient and secure privacy-preserving multi-cloud storage framework for mobile devices. Int J Comput Appl 43:1–11
Bertino E (2016) Big Data security and privacy. In: 2016 IEEE International conference on Big Data (Big Data), Washington, DC, USA, pp 3–3
Binjubeir M, Ahmed AA, Ismail MAB, Sadiq AS, Khurram Khan M (2020) Comprehensive survey on Big Data privacy protection. IEEE Access 8:20067–20079
Butpheng C, Yeh K-H, Xiong H (2020) Security and privacy in IoT-cloud-based e-health systems—a comprehensive review. Symmetry MDPI 12:1191
Campos EM, Saura PF, González-Vidal A, Hernández-Ramos JL, Bernabé JB, Baldini G, Skarmeta A (2022) Evaluating federated learning for intrusion detection in Internet of Things: review and challenges. Comput Netw 203:108661
Cha SC, Hsu TY, Xiang Y, Yeh K-H (2019) Privacy enhancing technologies in the Internet of Things: perspectives and challenges. IEEE Internet Things J 6(2):2159–2187
Chaowei Y, Qunying H, Zhenlong L, Kai L, Fei H (2017) Big Data and cloud computing: innovation opportunities and challenges. Int J Digit Earth. https://doi.org/10.1080/17538947.2016.1239771
Chen J, Ramanathan L, Alazab M (2021) Holistic Big Data integrated artificial intelligent modelling to improve privacy and security in data management of smart cities. Microprocess Microsyst 81:103722
Chen Q, Wu L, Jiang C (2022) ES-PPDA: an efficient and secure privacy-protected data aggregation scheme in the IoT with an edge-based XaaS architecture. J Cloud Comp 11:20
Chenthara S, Ahmed K, Wang H, Whittaker F (2019) Security and privacy-preserving challenges of e-health solutions in cloud computing. IEEE Access 7:74361–74382
Choudhury T, Gupta A, Pradhan S, Kumar P, Rathore YS (2017) Privacy and security of Cloud-Based Internet of Things (IoT). In: 2017 3rd International conference on computational intelligence and networks (CINE), Odisha, India, pp 40–45
Duan H, Zheng Y, Wang C, Yuan X (2019) Treasure collection on foggy islands: building secure network archives for Internet of Things. IEEE Internet Things J 6(2):2637–2650
Dutkiewicz L et al (2022) Privacy-preserving techniques for trustworthy data sharing: opportunities and challenges for future research. In: Curry E, Scerri S, Tuikka T (eds) Data spaces. Springer, Cham
Gai K, Qiu M, Zhao H (2021) Privacy-preserving data encryption strategy for Big Data in mobile cloud computing. IEEE Transact Big Data 7(4):678–688
Ghaffar Z, Ahmed S, Mahmood K, Islam SH, Hassan MM, Fortino G (2020) An improved authentication scheme for remote data access and sharing over cloud storage in cyber-physical-social-systems. IEEE Access 8:47144–47160
Gnana Sophia S, Thanammal KK, Sujatha SS (2023) Secure storage and accessing the data in the cloud using optimized homomorphic encryption. J Control Decision. https://doi.org/10.1080/23307706.2022.2078436
Gupta I, Singh AK, Lee C-N, Buyya R (2022) Cloud computing research center, secure data storage and sharing techniques for data protection in cloud environments: a systematic review, analysis, and future directions. IEEE Access. https://doi.org/10.1109/ACCESS.2022.3188110
Hamzah Amlak GM, Kraidi Al-Saedi KH (2023) Data mining techniques for cloud privacy preservation. Int J Intell Syst Appl Eng 11(6s):246–256
Hassija V, Chamola V, Saxena V, Jain D, Goyal P, Sikdar B (2019) A survey on IoT security: application areas, security threats, and solution architectures. IEEE Access 7:82721–82743
Henze M, Wolters B, Matzutt R, Zimmermann T, Wehrle K (2017) Distributed configuration, authorization and management in the cloud-based Internet of Things. 2017 IEEE Trustcom/BigDataSE/ICESS, Sydney, NSW, Australia, pp 185–192
Himeur Y, Sohail SS, Bensaali F, Amira A, Alazab M (2022) Latest trends of security and privacy in recommender systems: a comprehensive review and future perspectives. Comput Secur 118:102746
Hiremath S, Kunte S (2017) A novel data auditing approach to achieve data privacy and data integrity in cloud computing. In: 2017 International conference on electrical, electronics, communication, computer, and optimization techniques (ICEECCOT), Mysuru, India, pp 306–310
Hong-Yen T, Jiankun H (2019) Privacy-preserving Big Data analytics a comprehensive survey. J Parallel Distribut Comput 134:207–218
Hossain MS, Muhammad G, Abdul W, Song B, Gupta BB (2018) Cloud-assisted secure video transmission and sharing framework for smart cities. Futur Gener Comput Syst 83:596–606
Hou Y, Garg S, Hui L, Jayakody DNK, Jin R, Hossain MS (2020) A data security enhanced access control mechanism in mobile edge computing. IEEE Access 8:136119–136130
Hurrah NN, Parah SA, Sheikh JA, Al-Turjman F, Muhammad K (2019) Secure data transmission framework for confidentiality in IoTs. Ad Hoc Netw 95:101989
Ishaq A, Qadeer B, Shah MA, Bari N (2021) A comparative study on securing electronic health records (EHR) in cloud computing. In: 2021 26th International conference on automation and computing (ICAC), Portsmouth, United Kingdom, pp 1–7
Jahromi AN, Karimipour H, Dehghantanha A, Choo K-KR (2021) Toward detection and attribution of cyber-attacks in iot-enabled cyber-physical systems. IEEE Internet Things J 8(17):13712–13722
Jain SK, Kesswani N (2023) A noise-based privacy-preserving model for the Internet of Things. Complex Intell Syst 9:3655–3679
Jain P, Gyanchandani M, Khare N (2016) Big Data privacy: a technological perspective and review. J Big Data 3:25
Jain P, Gyanchandani M, Khare N (2019) Enhanced secured map reduce layer for Big Data privacy and security. J Big Data 6:30
Javeed D, Gao T, Saeed MS, Khan MT (2023) FOG-Empowered augmented-intelligence-based proactive defensive mechanism for IoT-enabled smart industries. IEEE Internet Things J 10(21):18599–18608. https://doi.org/10.1109/JIOT.2023.3288563
Javeed D, Saeed MS, Ahmad I, Adil M, Kumar P, Najmul Islam AKM (2024a) Quantum-empowered federated learning and 6G wireless networks for IoT security: Concept, challenges and future directions. Futur Gener Comput Syst 160:577–597. https://doi.org/10.1016/j.future.2024.06.023
Javeed D, Saeed MS, Adil M, Kumar P, Jolfaei A (2024b) A federated learning-based zero trust intrusion detection system for Internet of Things. Ad Hoc Netw 162:103540. https://doi.org/10.1016/j.adhoc.2024.103540
Javid T, Faris M, Beenish H, Fahad M (2020) Cybersecurity and data privacy in the cloudlet for preliminary healthcare Big Data analytics. In: 2020 International Conference on Computing and Information Technology (ICCIT-1441), Tabuk, Saudi Arabia, pp 1–4
Jeong J, Joo JWJ, Lee Y, Son Y (2019) Secure cloud storage service using bloom filters for the Internet of Things. IEEE Access 7:60897–60907
Jiang Q, Kumar N, Ma J, Shen J, He D, Chilamkurti N (2016) A privacy-aware two-factor authentication protocol based on elliptic curve cryptography for wireless sensor networks. Int J Network Mgmt. https://doi.org/10.1002/nem.1937
Jusak J, Mahmoud SS, Laurens R, Alsulami M, Fang Q (2022) A new approach for secure cloud-based electronic health record and its experimental testbed. IEEE Access 10:1082–1095
Kaaniche N, Laurent M (2017) Data security and privacy preservation in cloud storage environments based on cryptographic mechanisms. Comput Commun 111:120–141
Kabir AA, Elmedany M, Sharif W, Saeed M (2023) Securing IoT devices against emerging security threats: challenges and mitigation techniques. J Cyber Secur Technol. https://doi.org/10.1080/23742917.2023.2228053
Karie NM, Sahri NM, Yang W, Valli C, Kebande VR (2021) A review of security standards and frameworks for IoT-based smart environments. IEEE Access 9:121975–121995
Kaur K, Syed A, Mohammad A, Halgamuge MN (2017) Review: an evaluation of major threats in cloud computing associated with Big Data. In: 2017 IEEE 2nd International conference on Big Data analysis (ICBDA), Beijing, China, pp 368–372
Khan S, Parkinson S, Qin Y (2017) Fog computing security: a review of current applications and security solutions. J Cloud Comp 6:19
Khan HK, Pradhan R, Chandavarkar BR (2021) Hybrid cryptography for cloud computing. In: 2021 2nd International conference for emerging technology (INCET), Belagavi, India, pp 1–5
Krishnaraj N, Sangeetha S (2022) A study of data privacy in Internet of Things using privacy preserving techniques with its management. Int J Eng Trends Technol 70(3):54–65
Kumar S, Tiwari P, Zymbler M (2019) Internet of Things is a revolutionary approach for future technology enhancement: a review. J Big Data 6:111
Kumar A (2021) Framework for data security using DNA cryptography and HMAC technique in cloud computing. In: 2021 Second International conference on electronics and sustainable communication systems (ICESC), Coimbatore, India, pp 898-903
Kumar V, Alameemi AMA, Kumari A, Ahmad M, Falah MW, Abd El-Latif AA (2022) PSEBVC: provably secure ECC and biometric based authentication framework using smartphone for vehicular cloud environment. IEEE Access 10:84776–84789
Kumar A, Khan SB, Pandey SK et al (2023) Development of a cloud-assisted classification technique for the preservation of secure data storage in smart cities. J Cloud Comp 12:92
Li Y, Zhang F (2022) An efficient certificate-based data integrity auditing protocol for cloud-assisted WBANs. IEEE Internet Things J 9(13):11513–11523
Lin J, Yu W, Zhang N, Yang X, Zhang H, Zhao W (2017) A survey on Internet of Things: architecture, enabling technologies, security and privacy, and applications. IEEE Internet Things J 4(5):1125–1142
Loai AT, Gokay S (2021) Reconsidering Big Data security and privacy in cloud and mobile cloud systems. J King Saud Univ Comput Inform Sci 33(7):810–819
Lone AN, Mustajab S, Alam M (2023) A comprehensive study on cybersecurity challenges and opportunities in the IoT world. Secur Privacy 6:e318
Lu X, Pan Z, Xian H (2020) An efficient and secure data sharing scheme for mobile devices in cloud computing. J Cloud Comp 9:60
Mahfoudhi S, Frehat M, Moulahi T (2019) Enhancing cloud of things performance by avoiding unnecessary data through artificial intelligence tools. In: 2019 15th International wireless communications & mobile computing conference (IWCMC), Tangier, Morocco, pp 1463–1467
Mahrous WA, Farouk M, Darwish SM (2021) An enhanced blockchain-based IoT digital forensics architecture using fuzzy hash. IEEE Access 9:151327–151336
Majeed A, Khan S, Hwang SO (2022) Toward privacy preservation using clustering based anonymization: recent advances and future research outlook. IEEE Access 10:53066–53097
Mei R, Yan HB, He Y, Wang Q, Zhu S, Wen W (2022). Considerations on evaluation of practical cloud data protection. CONCERT 2022. In: Communications in computer and information science, vol 1699. Springer, Singapore
Mihailescu MI, Nita SL, Asalomia BL, Rogobete MG, Racuciu C (2022) Customized authorization process for cloud computing and IoT using attribute-based encryption. In: 2022 14th International conference on electronics, computers and artificial intelligence (ECAI), IEEE Ploiesti, Romania, pp 1–4
Mishra JK, Janarthanan MC (2023) Cloud computing security: machine and deep learning models analysis. Macromol Symp 407:2100521
Mishra K, Bhattacharjee V, Saket S et al (2022) Cloud and Big Data security system’s review principles: a decisive investigation. Wireless Pers Commun 126:1013–1050
Mishra A, Jabar TS, Alzoubi YI, Mishra KN (2023) Enhancing privacy-preserving mechanisms in cloud storage: a novel conceptual framework. Concurr Computat Pract Exper. https://doi.org/10.1002/cpe.7831
Moqurrab SA, Tariq N, Anjum A et al (2022) A Deep learning-based privacy-preserving model for smart healthcare in Internet of medical things using fog computing. Wireless Pers Commun 126:2379–2401
Morioka E, Sharbaf MS (2016) Digital forensics research on cloud computing: An investigation of cloud forensics solutions. In: 2016 IEEE symposium on technologies for homeland security (HST), Waltham, MA, USA, pp 1–6
Moulahi T, El Khediri S, Ullah Khan R, Zidi S (2021) A fog computing data reduction level to enhance the cloud of things performance. Int J Commun Syst 34(9):e4812
Muzammal SM et al (2018) Counter measuring conceivable security threats on smart healthcare devices. IEEE Access 6:20722–20733
Nadian-Ghomsheh A, Farahani B, Kavian M (2021) A hierarchical privacy-preserving IoT architecture for vision-based hand rehabilitation assessment. Multimed Tools Appl 80:31357–31380
Namakshenas D, Yazdinejad A, Dehghantanha A, Srivastava G (2024) Federated quantum-based privacy-preserving threat detection model for consumer Internet of Things. IEEE Trans Consum Electron. https://doi.org/10.1109/TCE.2024.3377550
Nanda P, He X, Yang LT (2020) Security, trust and privacy in cyber (STPCyber): future trends and challenges. Futur Gener Comput Syst 109:446–449
Nasiraee H, Ashouri-Talouki M (2022) Privacy-preserving distributed data access control for CloudIoT. IEEE Trans Dependable Secure Comput 19(4):2476–2487
Navin Prasad S, Rekha C (2023) Blockchain-based IAS protocol to enhance security and privacy in cloud computing. Measur Sens 28:100813
Niu L, Wang F, Li J, Han T, Liu D (2019) Development of agricultural Internet of Things monitoring system combining cloud computing and WeChat technology. In: 2019 IEEE 8th Joint international information technology and artificial intelligence conference (ITAIC), Chongqing, China, pp 1457–1460
Ogunniye G, Kokciyan N (2023) A survey on understanding and representing privacy requirements in the Internet-of-Things. J Art Intell Res 76:163–192
Pandey NK, Kumar K, Saini G et al (2023) Security issues and challenges in a cloud of things-based applications for industrial automation. Ann Oper Res. https://doi.org/10.1007/s10479-023-05285-7
Pathak M, Mishra KN, Singh SP, Mishra A (2023) An automated smart centralised vehicle security system for controlling the vehicle thefts/hacking using IOT and facial recognition. In: 2023 International conference on computational intelligence and knowledge economy (ICCIKE), Dubai, United Arab Emirates, pp 516–521
Pawlicki M, Pawlicka A, Kozik R, Choraś M (2023) The survey and meta-analysis of the attacks, transgressions, countermeasures, and security aspects common to the Cloud Edge and IoT. Neurocomputing 551:126533
Pioli L, Dorneles CF, de Macedo DDJ et al (2022) An overview of data reduction solutions at the edge of IoT systems: a systematic mapping of the literature. Computing 104:1867–1889
Quach S, Thaichon P, Martin KD et al (2022) Digital technologies: tensions in privacy and data. J of the Acad Mark Sci 50:1299–1323
Rachit BS, Ragiri PR (2021) Security trends in Internet of Things: a survey. SN Appl Sci 3:121
Radoglou Grammatikis PI, Sarigiannidis PG, Moscholios ID (2019) Securing the Internet of Things: challenges, threats and solutions. Internet of Things 5:41–70
Rahman SMM, Hossain MA, Hassan MM, Alamri A, Alghamdi A, Pathan M (2016) Secure privacy vault design for distributed multimedia surveillance system. Futur Gener Comput Syst 55:344–352
Ram Mohan P, Murali Krishna S, Siva Kumar AP (2018) Privacy preservation techniques in Big Data analytics: a survey. J Big Data 5:33
Ravi Kumar P, Herbert Raj P, Jelciana P (2018) Exploring data security issues and solutions in cloud computing. Procedia Comput Sci 125:691–697
Ray SM, Dutta S (2020) Big Data security issues from the perspective of IoT and cloud computing: a review. Recent Adv Comput Sci Commun. https://doi.org/10.2174/2666255813666200224092717
Reddy Y (2018) Big Data security in cloud environment. In: 2018 IEEE 4th International Conference on Big Data Security on Cloud (BigDataSecurity), IEEE International conference on high performance and smart computing, (HPSC) and IEEE international conference on intelligent data and security (IDS), Omaha, NE, USA, pp 100–106
Rejin PR, Paul RD, Alavi AH (2019) Verification of data integrity and co-operative loss recovery for secure data storage in cloud computing. Cogent Eng. https://doi.org/10.1080/23311916.2019.1654694
Rodríguez E, Otero B, Canal R (2023) A survey of machine and deep learning methods for privacy protection in the Internet of Things. Sensors 23:1252
Roslin Dayana K, Shobha Rani P (2023) Secure cloud data storage solution with better data accessibility and time efficiency. Automatika J Control Measur Electron Comput Commun 64(4):751–758
Sadhu PK, Yanambaka VP, Abdelgawad A (2022) Internet of Things: security and solutions survey. MDPI Sens 22:7433
Safaei Yaraziz M et al (2023) Recent trends towards privacy-preservation in the Internet of Things, its challenges and future directions. IET Circuits Devices Syst 17(2):53–61
Sakhnini J et al (2023) A generalizable deep neural network method for detecting attacks in industrial cyber-physical systems. IEEE Syst J 17(4):5152–5160
Sarwar K, Yongchareon S, Jian Yu, Rehman SU (2021) A survey on privacy preservation in fog-enabled Internet of Things. ACM Comput Surv 55(1):2
Schiller E, Andy A, Jara F, Jonathan S, Michael Z, Burkhard S (2022a) Landscape of IoT security. Comput Sci Rev 44:100467
Schiller E, Aidoo A, Fuhrer J, Stahl J, Ziörjen M, Stiller B (2022b) Landscape of IoT security. Comput Sci Rev Internet Things 44:100467
Selvarajan S, Srivastava G, Khadidos AO et al (2023) An artificial intelligence lightweight blockchain security model for security and privacy in IIoT systems. J Cloud Comp 12:38
Sharma Y, Gupta H and Khatri S. K. (2019) A Security Model for the Enhancement of Data Privacy in Cloud Computing. 2019 Amity international conference on artificial intelligence (AICAI), Dubai, United Arab Emirates, IEEE, pp 898–902
Shi Y (2018) Data security and privacy protection in public cloud. In 2018 IEEE International Conference on Big Data (Big Data), Seattle, WA, USA, pp 4812–4819
Shin S, Kwon T (2020) A privacy-preserving authentication, authorization, and key agreement scheme for wireless sensor networks in 5G-integrated Internet of Things. IEEE Access 8:67555–67571
Shukla RS (2022) IoT based designing of secure data storage system in distributed cloud system with Big Data using cryptography algorithm. In: 2022 11th International conference on system modeling & advancement in research trends (SMART), Moradabad, India, pp 264-270
Sicari S, Rizzardi A, Coen-Porisini A (2022) Insights into security and privacy towards fog computing evolution. Comput Secur 120:102822
Silva LV, Barbosa P, Marinho R (2018) Security and privacy aware data aggregation on cloud computing. J Internet Serv Appl 9:6
Simsek I (2023) Zero-knowledge and identity-based authentication, authorization, access control, and key exchange for publish/subscribe in Internet of Things. In: 2023 6th conference on cloud and Internet of Things (CIoT), Lisbon, Portugal, pp 47–54
Singh N, Singh AK (2018) Data privacy protection mechanisms in cloud. Data Sci Eng 3:24–39
Sookhak M, Yu FR, Zomaya AY (2018) Auditing Big Data storage in cloud computing using divide and conquer tables. IEEE Trans Parallel Distrib Syst 29(5):999–1012
Stergiou C, Psannis KE, Xifilidis T, Plageras AP, Gupta BB (2018) Security and privacy of Big Data for social networking services in the cloud. IEEE INFOCOM 2018—IEEE conference on computer communications workshops (INFOCOM WKSHPS), Honolulu, HI, USA, pp 438–443
Sumithra R, Parameswari R (2022) Data privacy and data protection security algorithms for Big Data in the cloud. Int J Health Sci 6(S2):7613–7621
Sun PJ (2019) Privacy protection and data security in cloud computing: a survey, challenges, and solutions. IEEE Access 7:147420–147452
Tahirkheli AI, Shiraz M, Hayat B, Idrees M, Sajid A, Ullah R, Ayub N, Kim K-I (2021) A survey on modern cloud computing security over smart city networks: threats, vulnerabilities, consequences, countermeasures, and challenges. Electronics 10:1811
Tang Z (2020) A preliminary study on data security technology in Big Data cloud computing environment. In: 2020 International conference on Big Data & artificial intelligence & software engineering (ICBASE) IEEE, Bangkok, Thailand, pp 27–30
Thabit F, Alhomdy S, Jagtap S (2021) A new data security algorithm for cloud computing based on genetics techniques and logical-mathematical functions. Int J Intell Netw Sci Direct 2:18–33
Tian Y, Kaleemullah MM, Rodhaan MA, Song B, Al-Dhelaan A, Ma T (2019) A privacy-preserving location service for cloud-of-things system. J Parallel Distrib Comput 123:215–222
Ullah F et al (2019) Cyber security threats detection in Internet of Things using deep learning approach. IEEE Access 7:124379–124389
Unal E, Sen-Baidya S, Hewett R (2018) Towards prediction of security attacks on software defined networks: a Big Data analytic approach. In: 2018 IEEE International conference on Big Data (Big Data), Seattle, WA, USA, pp 4582–4588
Waheed N, He X, Ikram M, Usman M, Hashmi SS, Usman M (2020) Security and privacy in IoT using machine learning and blockchain: threats and countermeasures. ACM Comput Surv 53(6):122
Wang H (2021) Research on risk and supervision of financial Big Data application based on cloud computing. In: 2021 IEEE International conference on advances in electrical engineering and computer applications (AEECA), Dalian, China, pp 507–510
Wang F, Wang H, Xue L (2021) Research on data security in Big Data cloud computing environment. In: 2021 IEEE 5th advanced information technology, electronic and automation control conference (IAEAC), Chongqing, China, pp 1446–1450
Wang Y, Ni K, Wang X, Zhu J (2022) Design of automatic weather monitoring and forecasting system based on Internet of Things and Big Data. In: 2022 International conference on sustainable computing and data communication systems (ICSCDS), Erode, India, pp 979–982
Wazid M, Das AK, Hussain R, Succi G, Rodrigues JJPC (2019) Authentication in cloud-driven IoT-based Big Data environment: survey and outlook. J Syst Architect 97:185–196
Williams P, Dutta IK, Daoud H, Bayoumi M (2022) A survey on security in the Internet of Things with a focus on the impact of emerging technologies. Internet Things 19:100564
Wylde V, Rawindaran N, Lawrence J et al (2022a) Cybersecurity, data privacy, and blockchain: a review. SN Comput Sci 3:127
Wylde V, Rawindaran N, Lawrence J, Balasubramanian R, Prakash E, Jayal A, Khan I, Hewage C, Platts J (2022b) Cybersecurity, data privacy, and blockchain: a review. SN Comput Sci 3:127
Xiao Y, Jia Y, Liu C, Cheng X, Yu J, Lv W (2019) Edge computing security: state of the art and challenges. Proc IEEE 107(8):1608–1631
Xie Q, Zhang C, Jia X (2023) Security-aware and efficient data deduplication for edge-assisted cloud storage systems. IEEE Trans Serv Comput 16(03):2191–2202
Yahuza M et al (2020) Systematic review on security and privacy requirements in edge computing: state of the art and future research opportunities. IEEE Access 8:76541–76567
Yan H, Gui W (2021) Efficient identity-based public integrity auditing of shared data in cloud storage with user privacy preserving. IEEE Access 9:45822–45831
Yao H (2022) Data storage security system based on cloud computing. In: 2022 IEEE 2nd International conference on electronic technology, communication and information (ICETCI), Changchun, China, pp 1220–1223
Yazdinejad A, Dehghantanha A, Parizi RM, Hammoudeh M, Karimipour H, Srivastava G (2022) Block hunter: federated learning for cyber threat hunting in blockchain-based IIoT networks. IEEE Trans Industr Inf 18(11):8356–8366
Yazdinejad A, Dehghantanha A, Parizi RM, Srivastava G, Karimipour H (2023) Secure intelligent fuzzy blockchain framework: effective threat detection in IoT networks. Comput Ind 144:2023
Yazdinejad A, Dehghantanha A, Srivastava G (2024a) AP2FL: auditable privacy-preserving federated learning framework for electronics in healthcare. IEEE Trans Consum Electron 70(1):2527–2535
Yazdinejad A, Dehghantanha A, Srivastava G, Karimipour H, Parizi RM (2024b) Hybrid privacy-preserving federated learning against irregular users in next-generation Internet of Things. J Syst Architect 148:103088
Ye C, Cao W, Chen S (2021) Security challenges of blockchain in Internet of Things: systematic literature review. Trans Emerging Tel Tech 32:e4177
Yin H, Chen E, Zhu Y, Zhao C, Feng R, Yau SS (2022) Attribute-based private data sharing with script-driven programmable ciphertext and decentralized key management in blockchain Internet of Things. IEEE Internet Things J 9(13):10625–10639
Yu Y et al (2017) Identity-based remote data integrity checking with perfect data privacy preserving for cloud storage. IEEE Trans Inf Forensics Secur 12(4):767–778
Yu K et al (2022) A blockchain-based shamir’s threshold cryptography scheme for data protection in industrial Internet of Things settings. IEEE Internet Things J 9(11):8154–8167
Zaman S et al (2021) Security threats and artificial intelligence based countermeasures for Internet of Things networks: a comprehensive survey. IEEE Access 9:94668–94690
Zarandi MA, Dara Rozita A, Evan F (2020) A survey of machine learning-based solutions to protect privacy in the Internet of Things. Comput Secur 96:101921
Zhang J, Chen B, Zhao Y, Cheng X, Hu F (2018) Data security and privacy-preserving in edge computing paradigm: survey and open issues. IEEE Access 6:18209–18237
Zhang W, Jin S (2020) Research and application of data privacy protection technology in cloud computing environment based on attribute encryption. In: 2020 IEEE International conference on power, intelligent computing and systems (ICPICS), Shenyang, China, pp 994–996
Zhao X-P, Jiang R (2020) Distributed machine learning oriented data integrity verification scheme in cloud computing environment. IEEE Access 8:26372–26384
Zhou J, Cao Z, Dong X, Vasilakos AV (2017) Security and privacy for cloud-based IoT: challenges. IEEE Commun Mag 55(1):26–33
Zhu H et al (2019) A secure and efficient data integrity verification scheme for Cloud-IoT based on short signature. IEEE Access 7:90036–90044
Download references
Author information
Authors and affiliations.
Department of Computer Science & Engineering, Birla Institute of Technology, Jharkhand, India
Mayank Pathak, Kamta Nath Mishra & Satya Prakash Singh
You can also search for this author in PubMed Google Scholar
Contributions
Mayank Pathak, Kamta Nath Mishra and Satya Prakash Singh have equally contributed in writing this paper after having various discussions.
Corresponding author
Correspondence to Kamta Nath Mishra .
Ethics declarations
Conflicts of interest.
Being the corresponding author I declare that there is no conflict of interest with any person or organization for this paper.
Additional information
Publisher's note.
Springer Nature remains neutral with regard to jurisdictional claims in published maps and institutional affiliations.
Rights and permissions
Open Access This article is licensed under a Creative Commons Attribution-NonCommercial-NoDerivatives 4.0 International License, which permits any non-commercial use, sharing, distribution and reproduction in any medium or format, as long as you give appropriate credit to the original author(s) and the source, provide a link to the Creative Commons licence, and indicate if you modified the licensed material. You do not have permission under this licence to share adapted material derived from this article or parts of it. The images or other third party material in this article are included in the article’s Creative Commons licence, unless indicated otherwise in a credit line to the material. If material is not included in the article’s Creative Commons licence and your intended use is not permitted by statutory regulation or exceeds the permitted use, you will need to obtain permission directly from the copyright holder. To view a copy of this licence, visit http://creativecommons.org/licenses/by-nc-nd/4.0/ .
Reprints and permissions
About this article
Pathak, M., Mishra, K.N. & Singh, S.P. Securing data and preserving privacy in cloud IoT-based technologies an analysis of assessing threats and developing effective safeguard. Artif Intell Rev 57 , 269 (2024). https://doi.org/10.1007/s10462-024-10908-x
Download citation
Accepted : 06 August 2024
Published : 27 August 2024
DOI : https://doi.org/10.1007/s10462-024-10908-x
Share this article
Anyone you share the following link with will be able to read this content:
Sorry, a shareable link is not currently available for this article.
Provided by the Springer Nature SharedIt content-sharing initiative
- Blockchain technologies
- Cloud computing
- Data breach
- Data securities
- Digital forensic
- Fog computing
- Internet of Things
- Machine learning
- Privacy protection
- Find a journal
- Publish with us
- Track your research
Academia.edu no longer supports Internet Explorer.
To browse Academia.edu and the wider internet faster and more securely, please take a few seconds to upgrade your browser .
Enter the email address you signed up with and we'll email you a reset link.
- We're Hiring!
- Help Center
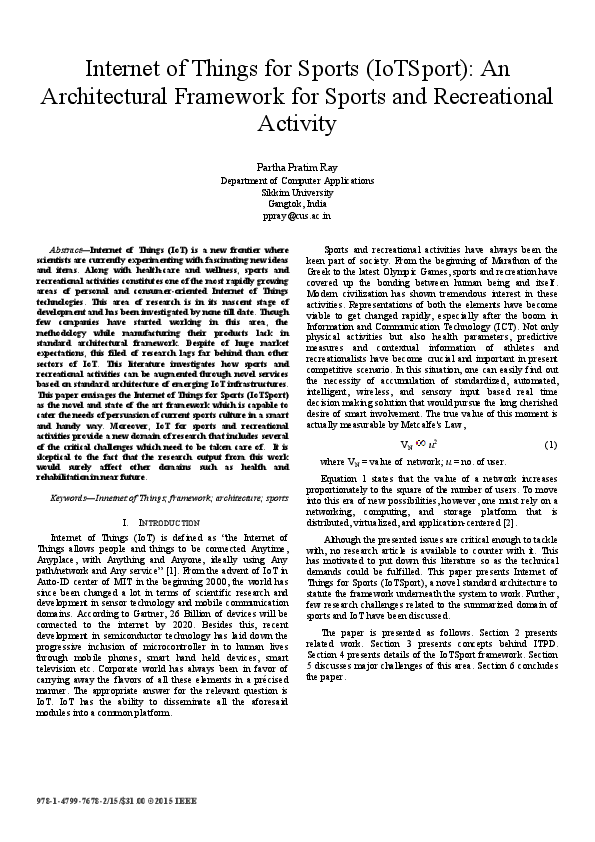
Internet of Things for Sports (IoTSport): An architectural framework for sports and recreational activity

IEEE International Conference on Electrical, Electronics, Signals, Communication and Optimization (EESCO)
Internet of Things (IoT) is a new frontier where scientists are currently experimenting with fascinating new ideas and items. Along with health-care and wellness, sports and recreational activities constitutes one of the most rapidly growing areas of personal and consumer-oriented Internet of Things technologies. This area of research is in its nascent stage of development and has been investigated by none till date. Though few companies have started working in this area, the methodology while manufacturing their products lack in standard architectural framework. Despite of huge market expectations, this filed of research lags far behind than other sectors of IoT. This literature investigates how sports and recreational activities can be augmented through novel services based on standard architecture of emerging IoT infrastructures. This paper envisages the Internet of Things for Sports (IoTSport) as the novel and state of the art framework which is capable to cater the needs of persuasion of current sports culture in a smart and handy way. Moreover, IoT for sports and recreational activities provide a new domain of research that includes several of the critical challenges which need to be taken care of. It is skeptical to the fact that the research output from this work would surely affect other domains such as health and rehabilitation in near future.
Related Papers
Partha Pratim Ray
Sports and recreational activities constitute to be one of the most rapidly growing areas of personal and consumer oriented Internet of Things applications. Although few manufacturers have started developing smart sports products, they seem to lack in standardized architectural framework. No standard architecture has yet been devised by any researchers. This literature investigates how sports and recreational activities can be augmented through novel service based on Internet of Things based standard architecture-Internet 0/ Things Sport. Test results validates that the data convergence time is directly proportional to the number of players. Several research challenges towards sports centric Internet of Things have been presented wh ich need to be tackled in future.
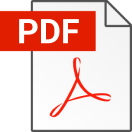
Journal of Communications Software and Systems
Laura Stefanizzi
The introduction of Internet of Things enabling technologies into the sport and recreational activities domain provide an interesting research challenge. Their adoption could significantly improve the sport experience and also the safety level of team sports. Despite this, only few attempts have been done to demonstrate the benefits provided by use of IoT technologies in sport environments. To fill this gap, this paper propose an IoT-aware Sport System based on the jointly use of different innovative technologies and standards. By exploiting the potentialities offered by an ultra-low-power Hybrid Sensing Network (HSN), composed of 6LoWPAN nodes integrating UHF RFID functionalities, the system is able to collect, in real time, both environmental parameters and players’ physiological data. Sensed data are then delivered to a Cloud platform where a monitoring application makes them easily accessible via REST Web Services. A simple proof of concept has demonstrated the appropriateness o...
International Journal of Computing and Digital Systems
THAER MANSI
Khalid Nahar
arXiv (Cornell University)
IOANNIS STAMELOS
IEEE CALCON
Internet of Things (IoT) has gain huge popularity in science community in last few years. Due its prospect in future internet domain many applications area have emerged. Health care is the most vital zone where IoT could play important role. For sake of well being of health, physical activity has become a keen part of modern life. Monitoring physical activity has most of time been under clinical supervision though recent literatures have shown development of few devices in digital domain. They lack in standardized design architecture, hence not applicable to get acquainted with network which has become a mandate to mankind. This paper studies the architectural framework for monitoring physical activity based on IoT in structured way. We propose a novel framework for Internet of Things based Physical Activity Monitoring (PAMIoT) to visualize measure and analyze the physical activities of human in daily life. PAMIoT is envisaged to be promising for physical activity monitoring purposes by complying technology enabled smart living in near future.
Open IoT Ecosystem for Sporting Event Management
sylvain Kubler Kubler , Aziz Bouras , Chantal Bonner Cherifi
By connecting devices, people, vehicles, and infrastructures everywhere in a city, governments and their partners can improve community well-being and other economic and financial aspects (e.g., cost and energy savings). Nonetheless, smart cities are complex ecosystems that comprise many different stakeholders (network operators, managed service providers, logistic centers, and so on), who must work together to provide the best services and unlock the commercial potential of the so-called Internet of Things (IoT). This is one of the major challenges that faces today's smart city movement, and the emerging ''API economy.'' Indeed, while new smart connected objects hit the market every day, they mostly feed ''vertical silos'' (e.g., vertical apps, siloed apps, and so on) that are closed to the rest of the IoT, thus hampering developers to produce new added value across multiple platforms and/or application domains. Within this context, the contribution of this paper is twofold: 1) present the strategic vision and ambition of the EU to overcome this critical vertical silos' issue and 2) introduce the first building blocks underlying an open IoT ecosystem developed as part of an EU (Horizon 2020) Project and a joint project initiative (IoT-EPI). The practicability of this ecosystem, along with a performance analysis, is carried out considering a proof-of-concept for enhanced sporting event management in the context of the forthcoming FIFA World Cup 2022 in Qatar. INDEX TERMS Internet of Things, smart city, interoperability, ecosystem, open innovation, API economy.
Higher Education Studies
Panita Wannapiroon
Sports can build both strength and be fun at the same time. When it comes to a professional athlete's victory, the winner will enhance his reputation and can earn a great deal of money. The problem that athletes frequently encounter is traumas, which can happen in games or during training. Today, the technology is very advanced and modernized. Innovations and perceptual devices can be created to monitor, measure, analyze and evaluate data from the information received from the introduction of an intelligent system of evaluation and prediction in terms of safety and risk prevention with regard to athletes, by collecting heart rate data, blood oxygen measurement, air density around the athletes’ body, body temperature and a temperature in the sports training room. This is the point of view and vision of a sports industry leaders, who perceive the opportunity and advantage associated with the competition and training of both amateur and professional athletes, to develop the athlete...
ISBS - Conference Proceedings Archive
Arnold Baca
Gary Wilkerson
RELATED PAPERS
Irene Ioannidou
Ashutosh Dhekne
International Journal of Advanced Research in Science, Communication and Technology
Kamlesh Kahar
Proceedings of the 25th Australian Computer-Human Interaction Conference on Augmentation, Application, Innovation, Collaboration - OzCHI '13
Tuck Wah Leong
Proceedings
Joel Benesha
Sustainability
Adin Cojocaru
Archives of Sports Medicine
Sergio Stevan Jr.
Bulletin of Electrical Engineering and Informatics
Antar Abdul-Qawy
Asian Journal Of Medical Technology
MOHD RAZALI SAPIEE
International Journal of Engineering Applied Sciences and Technology
Rahul Chawda
International Journal of Distributed Sensor Networks
Gregorio Rubio
Eva Henrietta Dulf
International Journal of Scientific Research in Science and Technology
International Journal of Scientific Research in Science and Technology IJSRST
Personal and Ubiquitous Computing
Peter Thomas
Dorothy Monekosso
WARSE The World Academy of Research in Science and Engineering
International Journal of Information Technology
Dr Naveed Jeelani J Khan
RELATED TOPICS
- We're Hiring!
- Help Center
- Find new research papers in:
- Health Sciences
- Earth Sciences
- Cognitive Science
- Mathematics
- Computer Science
- Academia ©2024
Navigation Menu
Search code, repositories, users, issues, pull requests..., provide feedback.
We read every piece of feedback, and take your input very seriously.
Saved searches
Use saved searches to filter your results more quickly.
To see all available qualifiers, see our documentation .
- Notifications You must be signed in to change notification settings
NPTEL Assignment Answers and Solutions 2024 (July-Dec). Get Answers of Week 1 2 3 4 5 6 7 8 8 10 11 12 for all courses. This guide offers clear and accurate answers for your all assignments across various NPTEL courses
progiez/nptel-assignment-answers
Folders and files.
Name | Name | |||
---|---|---|---|---|
164 Commits | ||||
Repository files navigation
Nptel assignment answers 2024 with solutions (july-dec), how to use this repo to see nptel assignment answers and solutions 2024.
If you're here to find answers for specific NPTEL courses, follow these steps:
Access the Course Folder:
- Navigate to the folder of the course you are interested in. Each course has its own folder named accordingly, such as cloud-computing or computer-architecture .
Locate the Weekly Assignment Files:
- Inside the course folder, you will find files named week-01.md , week-02.md , and so on up to week-12.md . These files contain the assignment answers for each respective week.
Select the Week File:
- Click on the file corresponding to the week you are interested in. For example, if you need answers for Week 3, open the week-03.md file.
Review the Answers:
- Each week-XX.md file provides detailed solutions and explanations for that week’s assignments. Review these files to find the information you need.
By following these steps, you can easily locate and use the assignment answers and solutions for the NPTEL courses provided in this repository. We hope this resource assists you in your studies!
List of Courses
Here's a list of courses currently available in this repository:
- Artificial Intelligence Search Methods for Problem Solving
- Cloud Computing
- Computer Architecture
- Cyber Security and Privacy
- Data Science for Engineers
- Data Structure and Algorithms Using Java
- Database Management System
- Deep Learning for Computer Vision
- Deep Learning IIT Ropar
- Digital Circuits
- Ethical Hacking
- Introduction to Industry 4.0 and Industrial IoT
- Introduction to Internet of Things
- Introduction to Machine Learning IIT KGP
- Introduction to Machine Learning
- Introduction to Operating Systems
- ML and Deep Learning Fundamentals and Applications
- Problem Solving Through Programming in C
- Programming DSA Using Python
- Programming in Java
- Programming in Modern C
- Python for Data Science
- Soft Skill Development
- Soft Skills
- Software Engineering
- Software Testing
- The Joy of Computation Using Python
- Theory of Computation
Note: This repository is intended for educational purposes only. Please use the provided answers as a guide to better understand the course material.
📧 Contact Us
For any queries or support, feel free to reach out to us at [email protected] .
🌐 Connect with Progiez
⭐️ Follow Us
Stay updated with our latest content and updates by following us on our social media platforms!
🚀 About Progiez
Progiez is an online educational platform aimed at providing solutions to various online courses offered by NPTEL, Coursera, LinkedIn Learning, and more. Explore our resources for detailed answers and solutions to enhance your learning experience.
Disclaimer: This repository is intended for educational purposes only. All content is provided for reference and should not be submitted as your own work.
Contributors 3
- Open access
- Published: 15 October 2020
Research on sports fitness management based on blockchain and Internet of Things
- Yu Shan 1 &
- Yuehui Mai 1
EURASIP Journal on Wireless Communications and Networking volume 2020 , Article number: 201 ( 2020 ) Cite this article
4765 Accesses
19 Citations
Metrics details
The amount of exercise and fitness level of sports athletes can be quantitatively evaluated through the measurement of health and sports information, thereby ensuring effective fitness effects. The development of blockchain and Internet of Things technology provides a new perspective and technical means for fitness management technology. In view of the current problems in the field of sports fitness, this paper designs and implements a dynamic management technology for sports fitness based on the concept of Internet of Things and blockchain. First, based on an in-depth analysis of the current status of theoretical research and application of the Internet of health at home and abroad, the theories and methods of sports information and health information collection are studied. A temperature sensor and an acceleration sensor are used to collect human body temperature and exercise steps, respectively, and then estimate human health and exercise conditions. Second, solve the privacy problem in the collection and transmission of the Internet of Things by adding blockchain technology. Finally, the machine-learning method is used to clean and manage the information and data to realize the real-time detection and management of the athlete’s fitness status. The actual case test shows that the functions and technical performance indicators of the dynamic fitness management technology can meet the needs of users in indoor and outdoor fitness management, and promote the development of the sports industry and provide a scientific reference.
1 Introduction
In recent years, with the development of the social economy and the deepening of the popularization of science, people’s awareness and demand for health and safety have also been continuously improved [ 1 , 2 ]. Maintaining a healthy body requires not only a reasonable diet, but more importantly, a scientific exercise habit. A large number of studies have proved that regular aerobic exercise is beneficial to human health and can improve the body’s athletic ability and physical fitness [ 3 ]. In terms of health outcomes and the effectiveness of interventions, accurate quantification of physical exercise and physical health is crucial. If the health information and exercise information of the human body can be collected and analyzed, the athletes can be given effective guidance and intervention in sports and health [ 4 , 5 ].
At present, the collection of health and sports information has gone through several stages of development. In the network-acquisition phase, electronic equipment is used to detect human body-related information, and then, various networks are used to transmit the detected data to realize automatic data collection and upload [ 6 ]. Neil Zhao used acceleration as a relevant parameter to analyze the characteristics of walking or running [ 7 ]. The scholar plotted the relationship between each phase of the walking cycle and the changes in vertical and forward acceleration. The motion can be divided into three directions, namely the X -axis direction, the Y -axis direction, and the Z -axis direction, and the ADXL345 is used to detect the acceleration in the three directions. The armband fitness equipment FIT CORE and FIT LINK launched by Body Media in 2012 include four sensors at the same time. The three-axis acceleration sensor is used to detect human movement. The temperature sensor is used to detect the surface temperature of the human body, and the heat flux sensor is used to detect energy consumption. The technology detects people’s life patterns from three aspects: energy intake, energy consumption, and sleep efficiency. The methods used to transmit data in portable devices include both traditional wired transmission methods and current wireless transmission methods such as Wi-Fi, radio frequency identification, Bluetooth, ZigBee, and other technologies [ 8 , 9 ]. In recent years, researchers at home and abroad have conducted a lot of research and application in the field of sports detection using wireless technology and sensor technology, and promoted the development of sports detection technology [ 10 ]. However, a single collection of motion information parameters has been unable to meet people’s needs, and the method of judging human exercise intensity also needs to be improved. The evaluation and calculation of acceleration combined with human physiological indicators such as temperature and heart rate are the new entry points for research in university laboratories [ 11 , 12 ].
In response to the above problems, based on previous research, this paper proposes a sports fitness management technology based on the Internet of Things and blockchain. The system uses the Internet of Things to collect user movement and physical health information in real time, and interacts with the cloud-computing platform through blockchain encryption technology for data processing and professional diagnosis. The actual case test and analysis illustrate the good effects of the fitness management technology achieved, which can provide a scientific reference model and basis for the establishment of remote real-time dynamic sports fitness management technology using the Internet of Things and blockchain.
2 Related work
2.1 sports fitness management technology.
The progress of the collection of sports fitness information parameters has evolved from the traditional way to the ubiquitous way of collecting today. That is to comprehensively use the existing electronic technology and communication technology to detect various health and sports indexes of the human body at any time and any place, thereby providing a scientific basis for human health assessment and sports calculation [ 13 ]. Through the detection of physical fitness information, health-related data such as body temperature, pulse, blood pressure, and speed and acceleration of human walking (walking or running) are obtained [ 14 ]. People can timely monitor and evaluate their fitness progress based on these parameters. You can also check your own exercise history according to exercise parameters, and adjust the amount of exercise and exercise intensity in time, thus making it possible for people to exercise scientifically. The schematic diagram of the information function of the physical fitness Internet of Things is shown in Fig. 1 .
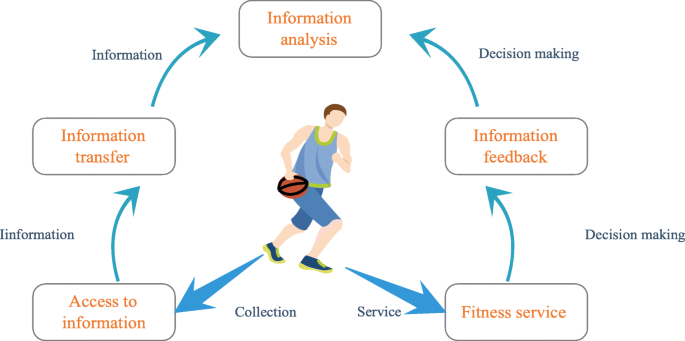
Schematic diagram of the information function of the Internet of Things
To achieve the acceleration signal of human walking motion, we must first analyze the gait characteristics of human motion and use this as a theoretical basis to correctly guide the design and implementation of the acceleration acquisition system [ 15 ]. Throughout the research on this aspect in recent years, it can be found that a large number of research scholars are digging deeply into the model of human walking movement in order to establish a better mathematical model to simulate the characteristics of human movement. Today, the research on fitness theory continues to deepen, and various portable and simple sports data collection devices also follow the trend, which brings great convenience to people’s scientific fitness. Many people now wear a variety of fitness equipment when they start fitness, which is a high-tech way to monitor sports in real time [ 16 ]. However, most of the various fitness equipments currently on the market are expensive and cannot be widely used. In summary, it is necessary to design a physical fitness detection technology with strong real-time performance, easy operation, and reasonable price. The design established in this paper can not only detect the acceleration of human motion, but also detect the body temperature of human body. The acceleration is used to analyze human motion. Moreover, the system can be used in places with high real-time requirements and meets the needs of some users.
In order to complete the design of sports fitness management technology based on the Internet of Things, this paper proposes to integrate multiple communication technologies to deeply integrate the Internet of Things and the blockchain, optimize the user experience, and make the connection between healthy Internet of Things applications and people closer. The entire architecture consists of a perception layer, a transmission layer, and an application layer [ 17 ]. Sensors based on the Internet of Things can continuously acquire sports and fitness signals and perform multimodal data transmission and aggregation processing with the service system.
The sports fitness management technology is a data collection system based on the Internet of Things, including health information collection and sports information collection. The system is divided into three layers [ 18 ]. These structures are the lowest perception layer (data collection layer), the network layer (data transmission layer) to transmit data, and the application layer (data processing and display layer) located at the item layer. The architecture of this technology is shown in Fig. 2 . As shown in the figure, the main task of the perception layer of the first layer is the design and implementation of the data collection terminal to complete the acquisition of information. The technologies involved are sensor technology and microprocessor technology [ 19 ]. The second layer of the network transmission layer implements data transmission, that is, wireless communication is used for data transmission, such as ZigBee technology, Bluetooth technology, and Wi-Fi technology. The third layer of application layer is information processing and storage, using PC, smart terminal, and other devices to analyze, display, and apply data.
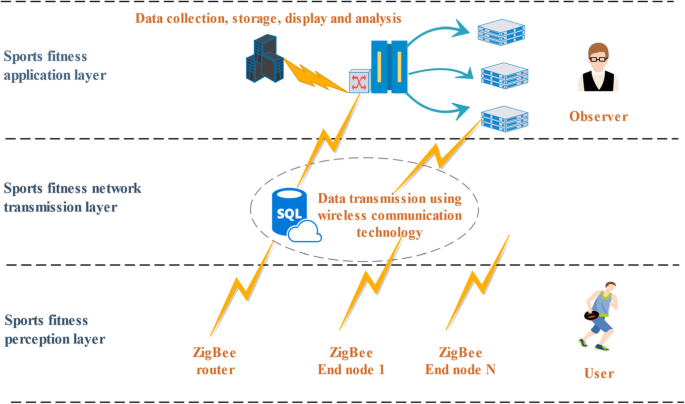
Schematic diagram of the framework of sports fitness management technology system
2.2 IoT privacy protection technology based on blockchain
In recent years, the Internet of Thing (IoT) technology and its innovative applications have rapidly developed in fields such as smart manufacturing, smart homes, and smart cities, which have changed the original production model and brought unprecedented convenience [ 20 ]. However, the ubiquitous object-to-thing interconnection and human-to-thing data exchange also put forward higher security requirements for the collection, transmission, and exchange of sensitive information. The ultra-large-scale concurrent data processing requirements caused by the massive data exchange of the IoT nodes are also a huge challenge to the existing data center’s computing, storage, and stable service capabilities. Blockchain technology with decentralized computing characteristics naturally provides a class of solutions for high concurrency problems.
The Internet of Things usually uses information-aware devices to collect the perception information of the physical world and establish the connection between the information world and the physical world. However, most of these exchanges of perceptual information use the central server as the medium for information storage and forwarding. Once a malicious attack is encountered, the private data stored in the central server will be leaked, causing an inestimable impact. Therefore, the ultra-large-scale concurrent data processing requirements caused by the massive data exchange of the IoT nodes have brought huge challenges to the existing data center computing, storage, and stable service capabilities [ 21 ].
Blockchain technology with decentralized computing characteristics naturally provides a class of solutions for high concurrency problems. Blockchain is not a single technology but a decentralized distributed database formed by combining various technologies such as mathematics, economics, cryptography, and network science in a specific way. The unique characteristics of the blockchain can solve the trust and privacy issues faced in the development of the Internet of Things, and provide technical support for the entire Internet of Things such as transparency, distributed storage, trust, and other aspects [ 22 ].
The hardware of this system is mainly composed of signal nodes, pulse nodes, and sensors as a platform on which nodes are integrated [ 23 ]. On the basis of briefly elaborating the design of ECG, respiration, pulse, temperature, and motion state acquisition module, the paper introduces the signal node as an integration of various modules and main control chip and the overview of the clothing as the acquisition front-end and platform. The schematic diagram of the basic hardware design of the Internet of Things is shown in Fig. 3 .
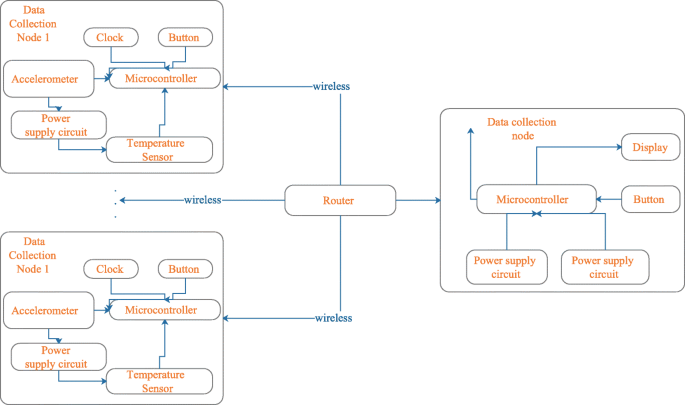
Schematic diagram of the basic hardware design of the Internet of Things
Continuous monitoring of the user’s movement status can achieve effective monitoring when the user falls, violent collisions, and other personal safety conditions. Therefore, the system directly integrates a three-axis acceleration detection module in the signal node to achieve detection of user movement status [ 24 ]. This article selects LIS3DH three-axis MEMS acceleration sensor produced by STMicroelectronics to detect the motion state. The sampling rate is selectable from 1 Hz to 5 kHz, the operating voltage range is 1.713.6 V, the current is 2 μA in low-power sleep mode, and the power consumption is 11 μA in normal operating mode, compatible with I2C and SPI interfaces. As shown in Fig. 3 , the acceleration values of the chip in the X , Y , and Z directions can be measured. LIS3DH outputs the original three-axis acceleration data through the SPVI2C interface and performs further processing according to user needs. In this system, the underlying acceleration data is read directly through the I2C interface.
The amplitude of normal body surface ECG is between 10 μV and 4 mV, the typical value is 1 mV, the signal frequency is 0.05~100 Hz, and 90% of the ECG spectrum energy is concentrated between 0.25 and 35 Hz. The contact ECG acquisition method will inevitably introduce power frequency interference and EMG interference from the body surface. Since this system uses a single-lead two-electrode method to obtain ECG differential signals, a high-precision ADC is required for analog-to-digital conversion [ 25 ]. This chip can perform dual-channel simultaneous acquisition of ECG and respiration signals. At the same time, a 24-bit high-precision A/D conversion function is built-in. Combined with this chip, a signal acquisition and conditioning circuit is built, which can reduce the size of the module and improve the anti-interference ability.
For the real-time collection of the user’s body surface temperature, this system uses the DS18B20 contact digital temperature sensor produced by Maxim to collect the body surface temperature. The chip is packaged in the form of TO-92, and its shape is a straight-in triode. The black part is the temperature-sensing end. In the parasitic power mode of the DS18B20 chip, the sensor only needs to be connected to the GND and DQ signal lines. When the bus is high, the DS 18B20 can obtain energy from the DQ signal line and store the energy in the storage capacitor of the parasitic power supply. When the DQ and the signal level drops, the energy is released for the sensor to work with. Because the system uses a battery with a voltage of 3.7 V to supply power, and the main device power supply is 3.3 V, the normal external power supply method is used.
The system uses a separate wrist node to collect pulse signals. As the heart periodically expands and contracts, the volume of blood vessels in the body also periodically increases and decreases, and the light intensity signal received by the photoelectric receiver exhibits periodic changes with the arterial pulse. The photoelectric volume pulse signal is obtained from the wrist using a reflective photoelectric sensor, and the conditioned signal is led out to the pulse node through the interface, and the analog-to-digital conversion is performed by the main control chip of the signal node.
4 Experiment
Dynamic ECG and other sports fitness collection sensors are used to continuously record 24 h of dynamic ECG and other data, including activity data in different situations such as rest and activities. In addition to monitoring the state of physical fitness, the sensor can also help users discover symptoms such as arrhythmia and myocardial ischemia, providing an important basis for clinical analysis and diagnosis and treatment [ 26 ]. With the development of technology, most sports fitness detection equipment is equipped with various types of filters to eliminate these interferences, but when portable devices are used in sports scenes, the interference of sports may seriously limit the quality of signals such as dynamic electrocardiogram.
In the data processing and transmission model of this paper, the main analysis is the process of data backhaul. The link from the user side to the computing server side uses the millimeter wave frequency band. Millimeter wave communication generally refers to a communication method that uses millimeter waves with a frequency band between 30 and 300 GHz and a wavelength between 1 and 10 mm as a carrier for transmitting data. Due to its beamforming method and high frequency band, the corresponding interferences are few, the transmission is stable and reliable, and it is worth mentioning that it has strong penetrating power to sand and smoke [ 27 ]. Because millimeter wave communication is greatly affected by weather outdoors, this fading problem is not the focus of this study. In communication systems, only when there are no visible obstacles in the transmission link between the signal transmitter and the signal receiver, called the signal transmitter, has a line of sight (LOS) to the signal receiver. When passing obstacles in the middle, there is no line-of-sight (NLOS) between the signal transmitter and the signal receiver. This paper discusses the case of LOS.
Generally, at a given location, the path loss is expressed in dB as follows:
in which P TX represents the total transmit power, P RX represents the total received power, and G TX and G RX represent the transmit and receive antenna gain, respectively. Path loss is also called propagation loss, that is, the loss caused by the propagation of radio waves in space mainly determined by the radiation spread of the transmitted power [ 28 ]. The transmission process is not related to the characteristics of the transmitter and receiver, so the free space path loss described in dB is:
in which f represents the carrier frequency in MHz, γ represents the path loss index, and its value depends on the environment in the transmission path. h represents the transmission distance between nodes in km; A represents the atmosphere and rain attenuation factor. In the simulation, we make the transmission nodes on the backhaul link all LOS.
A layer of Sigmoid function mapping is added to the feature-to-result mapping to limit the predicted value to [0, 1], which can output the probability of different categories. The probability p ( y = 1| x , θ ) represents the probability that y belongs to 1 given the characteristic variable x , and h θ ( x ) = p ( y = 1| x , θ ), then there is a logistic regression model:
in which θ = { θ 0 , θ 1 , ⋯ θ p } represents the coefficient value corresponding to each feature, θ value. It can be obtained by solving the maximum likelihood estimation function [ 29 ]. Assuming that each sample in the data set is independent of each other, the likelihood function:
In practical applications, when classifying feature instances, we choose the one with the largest probability value as the final category, which can be formalized as Formula 5 .
The gradient descent method is often used to obtain the parameter θ , but due to the limited learning ability of the LR model, a lot of artificial feature engineering is usually required to improve the learning ability of the model. How to automatically mine effective features and feature combinations has become an urgent problem to be solved, and using models to explore the combined relationship between features has become an effective way to solve this problem.
In this paper, we mainly analyze a single data stream, assuming that the user’s traffic demand at the data collection end is D . This demand mainly depends on the user and the application they use. In order to reflect, the uncertainty and sensitivity of demand is inversely proportional. Use the following formula to describe the relationship between them [ 30 ]:
Node L provides data services to the terminal. We noticed that in the uplink data transmission link, the energy consumption on node Z is always related to the data traffic and the wireless channel parameters of the next hop node on the edge computing data transmission link. In the downlink, the energy consumption on the downlink node Z also depends on the data traffic and channel parameters. Therefore, the resource allocation method mentioned in this article is applied to the uplink data backhaul link or the downlink in addition to the terminal nodes.
The above describes the design and implementation of sports fitness management technology. In order to verify the feasibility and efficiency of the sports fitness management technology based on the Internet of Things proposed in this article, we have built a sports fitness IoT platform and adopted blockchain technology. We will perform functional tests on the system to analyze and verify the practicability and efficiency of the system. In order to achieve real-time interaction of sensor data, Internet of Things sensors are used to collect sports and fitness data in life scenarios, and a sports and fitness information collection platform based on the Internet of Things is built. In addition, the blockchain technology is used to make the collected information private.
In order to test the reliability of the parameters of the physical fitness management technology in the actual wearing and using process, we organized various types of volunteer experiments. The experiment included observing the state parameters of the subject in a quiet state and the information parameters of the subject in a moving state. Sports fitness management technology can obtain high-quality cardio and other sports fitness data. The human activity information captured by the three-axis acceleration sensor is shown in Fig. 4 .
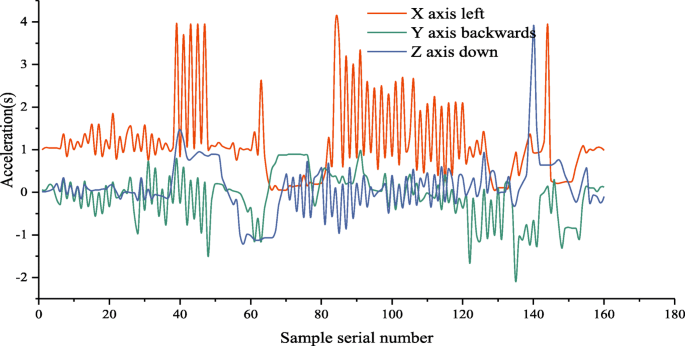
Human body fitness status information based on Internet of Things monitoring
The physical parameters of the subjects collected during the exercise test by the physical fitness management technology are shown in Figs. 5 , 6 , 7 , and 8 . Figures 5 , 6 , 7 , and 8 show the ECG, respiration, and triaxial acceleration sensor information in 4, 6, 8, 10, and the recovery state after exercise. In order to facilitate comparison, each way of physical fitness has been normalized in general, so we can also see the changes in exercise intensity and the relative changes in signal amplitude. It can be seen that, even in the state of high-intensity exercise, the physical fitness management technology can effectively obtain high-quality cardio and other physical fitness.
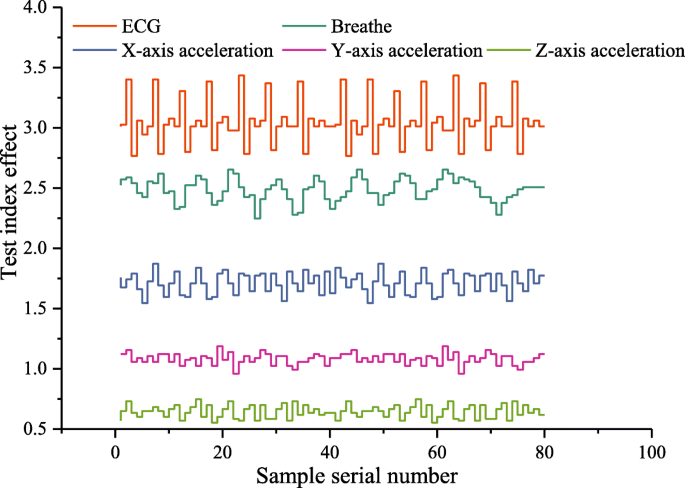
Test values of cardiopulmonary physiological signals—exercise intensity 4 km/h
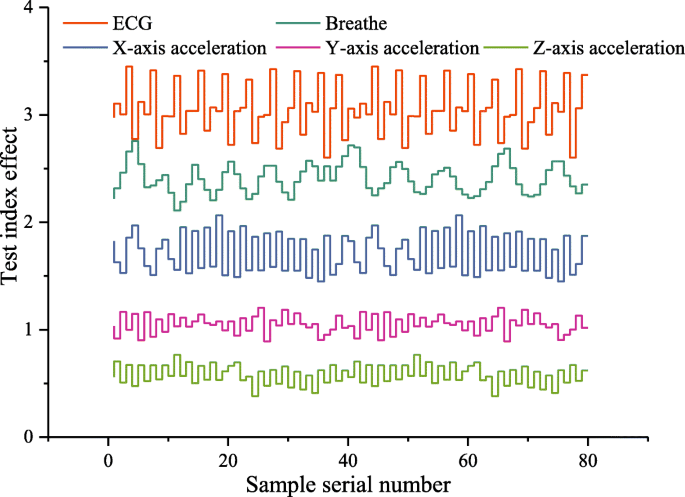
Test values of cardiopulmonary physiological signals—exercise intensity 6 km/h
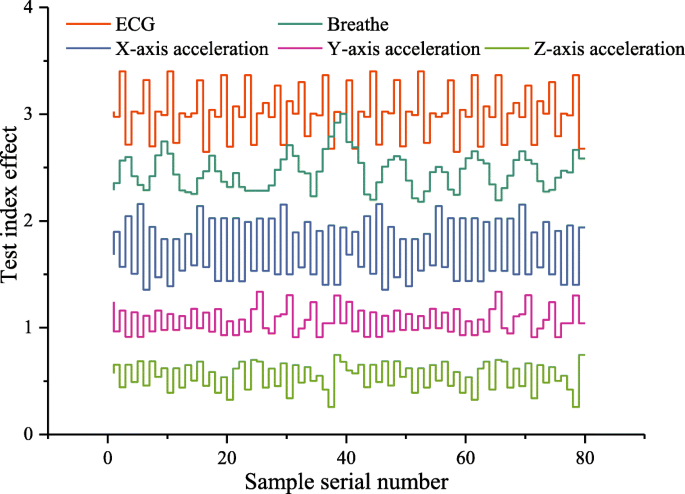
Test values of cardiopulmonary physiological signals—exercise intensity 8 km/h
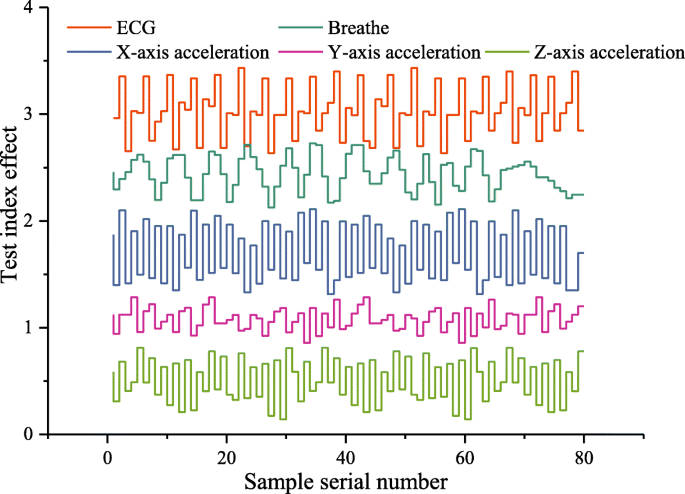
Test values of cardiopulmonary physiological signals—exercise intensity 10 km/h
During the system test, volunteers wore sports fitness management technology, and there was no discomfort during the period. Through the retrospective analysis of the data, it was found that the ECG, breathing, and posture/exercise information of each volunteer can be captured and recorded by the physical fitness management technology, and the overall signal quality is very good. Only in certain situations where the body posture suddenly changes, the ECG baseline drift limit and the amplitude of the respiratory wave change greatly, which may affect the accurate calculation of heart rate and respiratory rate.
Periodic movements, such as walking and running, will introduce movement trajectories on ECG and breathing signals, but generally do not affect the calculation of heart rate and breathing rate. The physical fitness management technology based on the Internet of Things has many advantages compared with the conventional health care system. It upgrades the traditional health care mode of the medical treatment environment to a remote monitoring mode centered on patients or families, while optimizing the allocation of medical resources. It can also bring great convenience and security to the broad masses of the people.
6 Discussion
This paper studies the application prospects of sports fitness management technology based on blockchain and Internet of Things. The system uses a combination of Internet of Things and blockchain technology to collect real-time fitness signals through Internet of Things technology and process and transmit data through blockchain technology. The actual case test and analysis illustrate the good effects of the sports fitness management technology achieved, which can provide a scientific reference model and basis for the establishment of a remote real-time dynamic sports fitness management system using Internet of Things, blockchain, and other technologies. In addition, focusing on the increasing and deepening of people’s needs for fitness in the future, we will continue to tap and improve existing fitness management technologies. We will provide scientific assistance for improving fitness informatization, promoting the development of the sports and health industry, and improving the quality of life of the people.
Availability of data and materials
The datasets used and/or analyzed during the current study are available from the corresponding author on reasonable request.
Abbreviations
Electrocardiograph
G. Wang, S. Zhang, S. Dong, D. Lou, L. Ma, X. Pei, H. Xu, U. Farooq, W. Guo, J. Luo, Stretchable optical sensing patch system integrated heart rate, pulse oxygen saturation, and sweat pH detection. IEEE Trans. Biomed. Eng. 66 (4), 1000–1005 (2019)
Article Google Scholar
D. Hansen, J. Niebauer, V. Cornelissen, O. Barna, D. Neunhuserer, C. Stettler, C. Tonoli, E. Greco, R. Fagard, K. Coninx, Exercise prescription in patients with different combinations of cardiovascular disease risk factors: a consensus statement from the EXPERT working group. Sports Med. 46 (4), 312–326 (2018)
Google Scholar
W. Guangming, Z. Shaomin, D. Shurong, L. Dong, M. Lie, P. Xiachuan, X. Hongsheng, U. Farooq, G. Wei, L. Jikui, Stretchable optical sensing patch system integrated heart rate, pulse oxygen saturation and sweat pH detection. IEEE Trans. Biomed. Eng. 14 (4), 121–136 (2018)
K. Zhang, Y. Zhu, S. Maharjan, Y. Zhang, Edge intelligence and blockchain empowered 5G beyond for the industrial Internet of Things. IEEE Netw. 33 (5), 12–19 (2019)
D. Zhang, G. Lindholm, H. Ratnaweera, Use long short-term memory to enhance Internet of Things for combined sewer overflow monitoring. J. Hydrol. 556 , 409–418 (2018)
S. Yao, Y. Zhao, A. Zhang, S. Hu, H. Shao, C. Zhang, L. Su, T. Abdelzaher, Deep learning for the Internet of Things. Computer 51 (5), 32–41 (2018)
J. Xu, K. Xue, H. Tian, J. Hong, P. Hong, An identity management and authentication scheme based on redactable blockchain for mobile networks. IEEE Trans. Veh. Technol. 16 (4), 666–676 (2020)
T. Wong, B. Paton, Foot posture and its relationship with knee kinematics in barefoot running of the healthy adult. Br. J. Sports Med. 51(4), 411.1-411 (2017).
L.R. West, J. Pham, Unapologetically patriotic – SMA shines the BJSM spotlight on Australian Research. Br. J. Sports Med. 52 (5), 283–283 (2018)
L. Sun, W. Hu, J. Chen, Secure and trustable sharing of electronic radiation oncology treatment record based on blockchain technology. Med. Phys. 25 (6), 55–71 (2018)
H. Subramanian, Decentralized blockchain-based electronic marketplaces. Commun. ACM 61 (1), 78–84 (2018)
Article MathSciNet Google Scholar
Stevenson, Robert, L., Lingel, Jessica, Evolution of the Internet of Things. American Laboratory (2018).
J. M. Roman-Belmonte, C. R. Hortensia, E. C. Rodriguez-Merchan, How blockchain technology can change medicine. Postgraduate Medicine, 00325481.2018.1472996 (2018).
E. Rodby-Bousquet, A. Agústsson, G. Jónsdóttir, T. Czuba, G. Hgglund, Interrater reliability and construct validity of the posture and postural ability scale in adults with cerebral palsy in supine, prone, sitting and standing positions. Clin. Rehabil. 28 (1), 82–90 (2014)
D.G. Roche, M.K. Taylor, S.A. Binning, J.L. Johansen, P. Domenici, J.F. Steffensen, Unsteady flow affects swimming energetics in a labriform fish (Cymatogaster aggregata). J. Exp. Biol. 217 (41), 43–56 (2014)
G. Querzola, C. Lovati, C. Mariani, L. Pantoni, A semi-quantitative sport-specific assessment of recurrent traumatic brain injury: the TraQ questionnaire and its application in American football. Neurol. Sci. 56 (77), 23–36 (2019)
H.J. Pula, C.E. Trenzado, S. García-Mesa, C. Fallola, A. Sanz, Effects of different culture systems on growth, immune status, and other physiological parameters of tench (Tinca tinca). Aquaculture 485 , 101–110 (2018)
H. Ozercan, I. Ibrahim, M. Atalay, E. Ayday, C. Alkan, Realizing the potential of blockchain technologies in genomics. Genome Res. 60 (4), 33–43 (2018)
R. O. Nielsen, I. Shrier, M. Casals, A. Nettel-Aguirre, E. Verhagen, Statement on methods in sport injury research from the 1st METHODS MATTER Meeting, Copenhagen, 2019. British Journal of Sports Medicine, bjsports-2019-101323 (2020).
A. Nangsuay, R. Meijerhof, I. Vandenanker, M.J.W. Heetkamp, V.D.S. Morita, B. Kemp, H. Vandenbrand, Effects of breeder age, broiler strain, and eggshell temperature on development and physiological status of embryos and hatchlings. Poultry Ence 13 (2), 1–36 (2016)
R. Matsugaki, M. Sakata, H. Ito, Y. Matsushima, S. Saeki, Effects of a physical therapist led workplace personal-fitness management program for manufacturing industry workers: a randomized controlled trial. Journal of Occupational and Environmental Medicine publish ahead of print 53 (4), 632–643 (2019)
E. Mark, D.K. Halstead, K. Walter, C.O.S. Moffatt, Medicine, fitness, sport-related concussion in children and adolescents. Pediatrics 23 (3), 432–446 (2018)
H. Li, K. Ota, M. Dong, Learning IoT in edge: deep learning for the Internet of Things with edge computing. IEEE Netw. 32 (1), 96–101 (2018)
G. Katharine, Experimenting with blockchain: can one technology boost both data integrity and patients’ pocketbooks? Nat. Med. 23 (4), 111–126 (2018)
B.O. Kahraman, Y.S. Sengul, K. Turhan, O.K. Orhan, S.S. Buse, Developing a reliable core stability assessment battery for patients with nonspecific low back pain. Spine 56 (5), 332–346 (2016)
O.H. Ibrahim, I.A. Mert, A. Erman, A. Can, Realizing the potential of blockchain technologies in genomics. Genome Res. 207 (44), 116–123 (2018)
G. Gregory, Management science in sports (Studies in the Management Sciences, Vol. 4). J. Oper. Res. Soc. 23 (4), 333–343 (1978)
S. Eugene, T. Thanassis, H. Wendy, Analytics for the Internet of Things. ACM Comput. Surv. 51 (4), 1–36 (2018)
S. Breitbach, S. Tug, P. Simon, Conventional and genetic talent identification in sports: will recent developments trace talent? Sports Med. 44 (11), 1489–1503 (2014)
L. Li, J. Liu, L. Cheng, S. Qiu, Z. Zhang, CreditCoin: a privacy-preserving blockchain-based incentive announcement network for communications of smart vehicles. IEEE Trans. Intell. Transp. Syst. 19 (99), 2204–2220 (2018)
Download references
Acknowledgements
Author information, authors and affiliations.
Department of Physical Education, South China Agriculture University, Guangzhou, 510640, China
Yu Shan & Yuehui Mai
You can also search for this author in PubMed Google Scholar
Contributions
Yu Shan is responsible for the collection of experimental data, and Yuehui Mai is responsible for the preparation of the paper. The author(s) read and approved the final manuscript.

Corresponding author
Correspondence to Yu Shan .
Ethics declarations
Ethics approval and consent to participate.
This article does not contain any studies with human participants or animals performed by any of the authors.
Consent for publication
All authors agree to submit this version and claim that no part of this manuscript has been published or submitted elsewhere.
Competing interests
The authors declare that they have no conflict of interest.
Additional information
Publisher’s note.
Springer Nature remains neutral with regard to jurisdictional claims in published maps and institutional affiliations.
Rights and permissions
Open Access This article is licensed under a Creative Commons Attribution 4.0 International License, which permits use, sharing, adaptation, distribution and reproduction in any medium or format, as long as you give appropriate credit to the original author(s) and the source, provide a link to the Creative Commons licence, and indicate if changes were made. The images or other third party material in this article are included in the article's Creative Commons licence, unless indicated otherwise in a credit line to the material. If material is not included in the article's Creative Commons licence and your intended use is not permitted by statutory regulation or exceeds the permitted use, you will need to obtain permission directly from the copyright holder. To view a copy of this licence, visit http://creativecommons.org/licenses/by/4.0/ .
Reprints and permissions
About this article
Cite this article.
Shan, Y., Mai, Y. Research on sports fitness management based on blockchain and Internet of Things. J Wireless Com Network 2020 , 201 (2020). https://doi.org/10.1186/s13638-020-01821-2
Download citation
Received : 24 June 2020
Accepted : 29 September 2020
Published : 15 October 2020
DOI : https://doi.org/10.1186/s13638-020-01821-2
Share this article
Anyone you share the following link with will be able to read this content:
Sorry, a shareable link is not currently available for this article.
Provided by the Springer Nature SharedIt content-sharing initiative
- Sports fitness management
- Internet of Things
- Wireless network
- Zone link technology
IoT in Sports: Taking Sports to the Next Level
Updated on Feb 15th, 2024
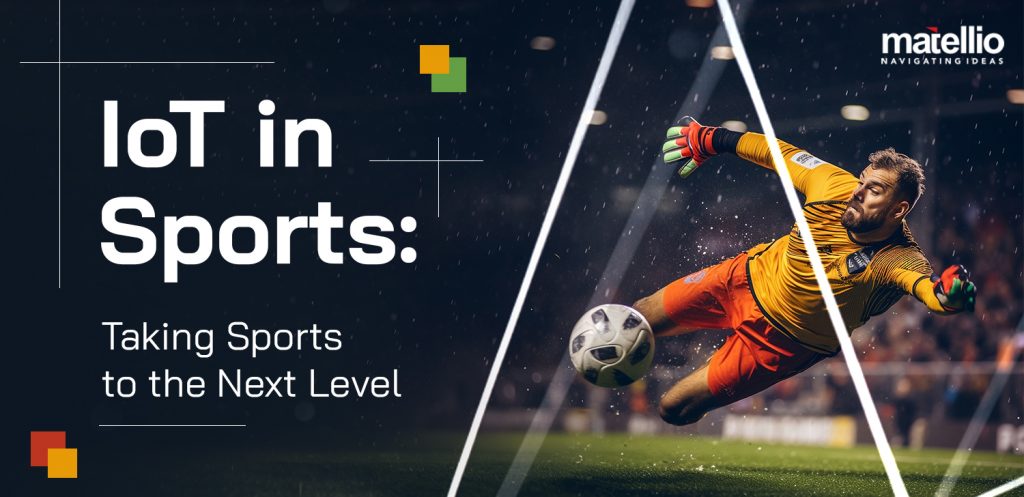
Have you heard about Fitbits and the Apple Watch? The very common fitness trackers used these days especially by athletes and fitness freaks to check the vitals and their performance metrics like heart rate and metabolism in real-time. Not just these, today many big sport companies like Adidas are using different apps to track their user’s performance and health.
In the ever-evolving world of sports, a new player has emerged on the field, quite literally — the Internet of Things (IoT), changing the game in ways we could never have imagined.
As athletes are pushing the boundaries of human performance, technology in sports is racing ahead to keep up with it. From enhancing athlete performance to revolutionizing fan experiences, IoT is leaving its mark on the sporting arena like never before.
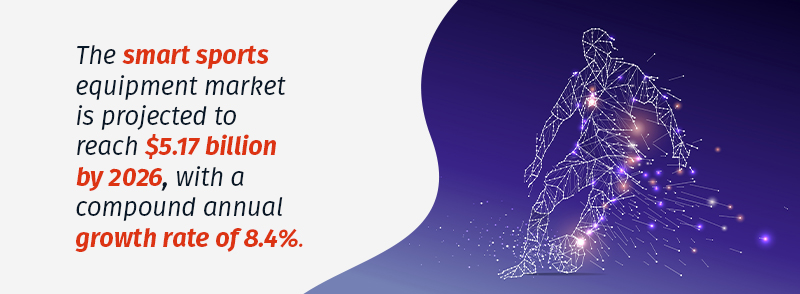
The IoT applications in sports industry will undeniably be a game-changer . So, if you are pla nning to kick off your business in the same , then lace up your sneakers, grab your favorite jersey, and go for digital transformation services .
- IoT is reshaping the sports industry by providing actionable data, enhancing safety, and delivering immersive fan experiences. Embracing IoT technology will let you stay competitive, efficient, and forward-thinking in an increasingly digital world.
- The IoT landscape in sports is ever evolving. Staying updated with the latest technologies and trends is essential to harness its full potential.
- IoT opens up opportunities for organizations to generate revenue through data monetization, innovative products, and services. Subscription-based IoT solutions and data insights are valuable assets.
Table of Contents
IoT in Sports: The Race that Never Stops
As the name suggests, internet of things gives items the power to connect and exchange data via internet. And if we talk in terms of sports, it’s the use of smart devices and sensors connected to the internet to collect and share data during sporting events. These devices can track athletes’ performance, monitor equipment, and provide real-time information to coaches, players, as well as their fans. It’s like giving sports a digital upgrade, offering insights that help improve training, enhance safety, and make the game more exciting for everyone involved.
The sensors can be embedded in equipment, clothing, playing fields and more to gather data on various aspects of the game, like player movements, ball speed, environmental conditions, and more. This data can be analyzed to optimize training routines, prevent injuries, and strategize during matches.
For fans, IoT provides an immersive experience by offering real-time statistics, interactive apps, and even augmented reality experiences, making sports more enjoyable and engaging.
Also Read- IoT Consulting Services: Everything You Need to Know
IoT in sports is transforming the way we play, watch, and enjoy sports with the power of data and connectivity.
The very latest example of the well-known company – Microsoft. They have lately launched an innovative IoT-powered cricket bat which features a card-sized sensor for gathering data on the bat’s angle, swing, and speed.
If you want to stay competitive in the sports world, providing IoT connected devices and gadgets is a must. So, contact us now for IoT development services and make your business stand for the long run.
IoT Applications in Sports
IoT (Internet of Things) applications in sports are transforming the way athletes get trained, compete, and fans experience their favorite games. These technological advancements are enhancing performance, safety, and the overall excitement of sports events. Let’s see some of the compelling IoT applications in the world of sports:
Smart Wearables for Athletes
You can use IoT in wearable devices like smartwatches and fitness trackers for athletes, so that they can easily track their performance and various other metrics like heart rate, steps taken, distance covered, and even sleep patterns via sensors. This can help them in getting valuable insights to better up training process and overall health.
Player and Ball Tracking
For sports like tennis and soccer, you can use IoT technology to check on the player’s movement and even the movement of the ball. This data is not only valuable for teams and coaches but also will level up the viewing experience for fans by providing real-time statistics and visualizations.
Injury Prevention
Implementing IoT sensors in sports equipment, like helmets and shin guards can let players monitor impact forces and detect potentially dangerous situations. This will help them to prevent injuries and ensure safety while playing sports.
Environmental Monitoring
IoT sensors can also be used to check the environmental conditions like temperature, humidity, and air quality for outdoor sports. This information will enable the athletes to play in a safe and comfortable environment.
Fan Engagement
You can also provide IoT-powered mobile apps to fans for getting interactive experiences during matches. Your users will be able to access live statistics replays and even participate in virtual games related to the sport they’re watching, making the fan experience more immersive.
Venue Management
With IoT, your users can manage large sports venues efficiently. Sensors monitor crowd flow, parking availability, and even restroom conditions, giving attendees a seamless experience.
Game Strategy
IoT generated data can be used by coaches and analysts to develop game strategies. For instance, in American football, coaches use data on player movements to design effective offensive and defensive plays.
Athlete Recovery
This can be used as the most effective feature. After intense games or training sessions, you can help athletes recover by monitoring factors like hydration levels, muscle fatigue, and sleep quality through IoT devices.
Broadcasting Enhancements
The very commonly used these days- Drones. With IoT technology you can let the broadcasters capture unique angles and perspectives of the game. Drones equipped with IoT sensors can provide breathtaking aerial views, enhancing the overall viewing experience for fans.
IoT helps athletes perform at their best, keeping them safe, engaging fans in new ways, and providing valuable insights to teams and coaches. Such enterprise solutions are win-win for everyone involved in the world of sports.
Real-Life Use Cases of IoT in Sports
After learning about where in sports you can use IoT, let’s now explore some real-life examples of how IoT, or the Internet of Things, is making a significant impact on the world of sports:
Player Performance Monitoring
NBA’s Smart Basketball: The NBA introduced a smart basketball that has sensors in it that track the ball’s movement wherever it goes and at what speed. This data helps players improve their shooting accuracy and dribbling skills.
Athlete Health and Safety
Zebra Technologies in NFL: NFL players wear shoulder pads with RFID to track their movements and collisions. This data helps them play safely and prevent any injuries.
Smart Wearables
Wearable Fitness Trackers: Athletes use wearable devices like Fitbit and Garmin to track their heart rate, steps, and sleep patterns. These wearables help athletes track their health and fitness levels.
IBM’s IoT for Wimbledon: Wimbledon uses IoT sensors to monitor weather and air quality, ensuring players and spectators play in their comfort zone and safely.
Smart Stadiums: Stadiums like Levi Stadium, home of the San Francisco 49ers, are using IoT to provide enhanced experiences for fans. Features include mobile ticketing, interactive apps, and seated food delivery.
Smart Helmets in Football: Football helmets have IoT sensors that can detect the force and location of impacts, helping to identify possible concussions.
Training Enhancement
Zepp Sports Sensors: Zepp’s IoT sensors attach to sports equipment (e.g., golf clubs, tennis rackets), which further provide detailed performance analytics to improve an athlete’s game.
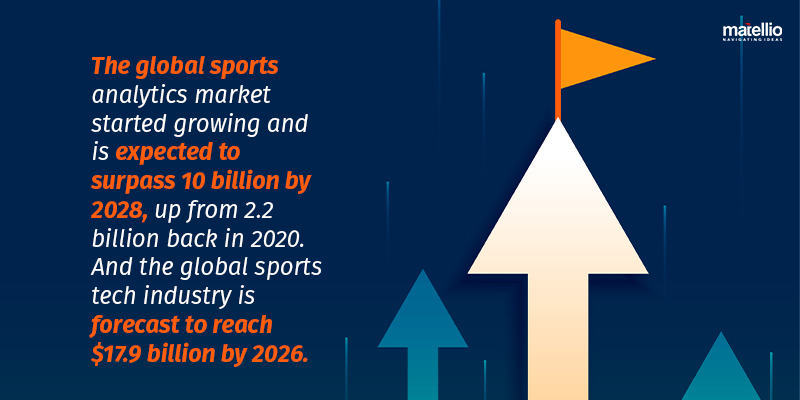
Today, IoT is being used by various industries to make sports healthier and more enjoyable. With so many IoT applications in sports, it must be clear now that this field is certainly going to shoot up. So, if you are thinking of taking your business to a competitive edge, this is the right time. Contact us for such technology consulting services and or follow the development process below for better clarity.
IoT in Sports: Step-by-step Development Process
While developing IoT solutions for sports, you should follow a systematic process of designing, building, testing, and deploying connected devices and applications that could enhance athlete performance, fan engagement, and overall sports experiences.
Here’s a step-by-step development process for you:
Frame a Plan
The first thing you should do before starting with any development is to plan a strategy. You can take the help of us for this, we will work closely with you, our valued client, and all stakeholders involved, including athletes, coaches, fans, and sports administrators. Together, we’ll define your unique needs and goals for implementing IoT technology in sports.
Market Research
We don’t just develop; we craft solutions tailored to your goals. Our team will conduct thorough market research to analyze existing IoT solutions in the sports industry. We’ll identify their strengths and weaknesses, aligning our strategy with your objectives. We’ll stay updated on the latest IoT technologies and platforms suitable for your specific requirements, whether it’s enhancing performance, engaging fans, ensuring player safety, or optimizing venue operations.
Hardware Selection and Integration
We understand that hardware forms the backbone of your IoT ecosystem. Our experts will meticulously select high-quality IoT components, such as sensors, actuators, wearables, and communication modules, customized to meet your needs. Seamless integration of these components is our commitment to you.
Software Development
Crafting a reliable and efficient IoT app is at the core of our services. We’ll design and develop an IoT software solution tailored to your unique requirements. Trust us to partner with you to ensure that your software effectively collects and analyzes data from sensors, securely transfers it to the cloud, and presents it through an intuitive interface.
Also Read- IoT Software Development: A Complete Guide
Data Analytics and Insights
Translating data into actionable insights is our forte. Our data analytics algorithms, incorporating machine learning and AI techniques, will process and analyze the collected data. This ensures that you receive valuable information to make informed decisions.
Make sure to hire us especially for AI development services , as it requires experience and knowledge to build it right.
User Interface and Experience
Your users’ experience is paramount. We will design and develop user interfaces that cater to athletes, coaches, fans, and administrators, delivering intuitive and engaging experiences. Our experienced designers will ensure that these interfaces align with the unique requirements of each user group.
Integration and Testing
After developing both hardware and software components, we will seamlessly integrate them, creating a functional IoT system. Rigorous testing, including functionality, usability, and performance testing, will be conducted to guarantee that our solution fulfills the defined objectives.
Pilot Testing and Feedback
Prior to the official launch, we’ll initiate thorough pilot testing within controlled environments, like sports facilities or training grounds. Client input is invaluable during this stage, as it helps us identify areas that require improvement.
Refinement and Deployment
Based on feedback, we’ll make the necessary enhancements and proceed to deploy the solution within real-world sports environments. Continuous monitoring ensures that performance, data accuracy, and user satisfaction are maintained. We commit to regular updates to incorporate new features, improvements, and security updates, aligned with your evolving needs and the latest technologies.
With this comprehensive approach, you can count on us to effectively craft IoT solutions that drive enhanced experiences for athletes, coaches, fans, and sports industry stakeholders.
We look forward to partnering with you to bring your vision to life.
Also Read- How to Hire an IoT Development Company?
IoT has completely revolutionized the world of sports, shaping it to be more enjoyable and engaging. With the ability to connect and communicate with countless devices and systems, IoT technology has opened many possibilities, making it a must-have for every business. And, as technology continues to evolve, we can expect to see even more innovative IoT applications. The future of IoT is bright, and it will undoubtedly play a significant role in shaping the world of tomorrow.
So, introduce IoT in your business today and make it ready for the next-gen future.
Contact us for IoT development services to design, test, implement, and maintain IoT systems. We have a proven track record of expertise in IoT technologies, with a team of experienced engineers and developers who understand the intricacies of IoT systems and their applications in the sports domain.
Our tailored solutions, commitment to innovation, and dedication to delivering results will drive success in the dynamic and competitive sports industry. Partner with us to get the best of the solution on the spot.
Related articles
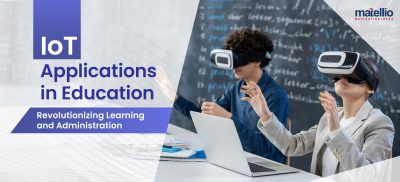
IoT Applications in Education: Revolutionizing Learning and Administration
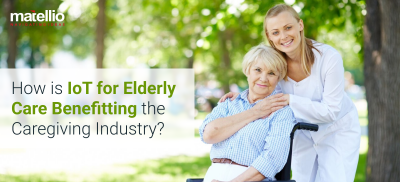
How is IoT for Elderly Care Benefitting the Caregiving Industry?
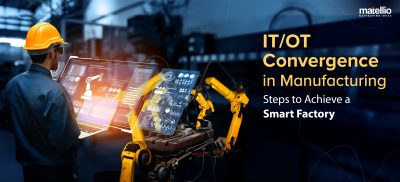
IT/OT Convergence in Manufacturing: Steps to Achieve a Smart Factory
Enquire now.
Facing Development Hurdles? Let’s Conquer Them Together. Our expert team is ready to tackle your challenges, from streamlining processes to scaling your tech.
- Advanced search
- Peer review
- Record : found
- Abstract : found
- Article : found
The Design of Sports Games under the Internet of Things Fitness by Deep Reinforcement Learning

Read this article at
- Download PDF
- Review article
- Invite someone to review
This study explores the application of deep reinforcement learning (DRL) in the Internet of Things (IoT) sports game design. The fundamentals of DRL are deeply understood by investigating the current state of IoT fitness applications and the most popular sports game design architectures. The research object is the ball return decision problem of the popular game of table tennis robot return. Deep deterministic policy gradients are proposed by applying DRL to the ball return decision of a table tennis robot. It mainly uses the probability distribution function to represent the optimal decision solution in the Markov Model decision process to optimize the ball return accuracy and network running time. The results show that in the central area of the table, the accuracy of returning the ball is higher, reaching 67.2654%. Different tolerance radii have different convergence curves. When r = 5 cm, the curve converges earlier. After 500,000 iterations, the curve converges, and the accuracy rate is close to 100%. When r = 2 cm and the number of iterations is 800,000, the curve begins to converge, and the accuracy rate reaches 96.9587%. When r = 1 cm, it starts to converge after 800,000 iterations, and the accuracy is close to 56.6953%. The proposed table tennis robot returns the ball in line with the requirements of the actual environment. It has practical application and reference value for developing IoT fitness and sports.
Related collections
Computer Vision, Deep Learning, Deep Reinforcement Learning, IoT
Most cited references 25
How to train your robot with deep reinforcement learning: lessons we have learned.

- Abstract : not found
- Article : not found
Intelligent decision-making of online shopping behavior based on internet of things
Voronoi-based multi-robot autonomous exploration in unknown environments via deep reinforcement learning, author and article information , contributors, affiliations, author notes.
Academic Editor: Vijay Kumar
Author information
This is an open access article distributed under the Creative Commons Attribution License, which permits unrestricted use, distribution, and reproduction in any medium, provided the original work is properly cited.
Comment on this article
Similar content 78 .
- RPLAD3: anomaly detection of blackhole, grayhole, and selective forwarding attacks in wireless sensor network-based Internet of Things Authors: Zainab Alansari , Nor Badrul Anuar , Amirrudin Kamsin …
- zk-IoT: Securing the Internet of Things with Zero-Knowledge Proofs on Blockchain Platforms Authors: Gholamreza Ramezan , Ehsan Meamari
- UAV IoT Framework Views and Challenges: Towards Protecting Drones as “Things” Authors: Thomas Lagkas , Vasileios Argyriou , Stamatia Bibi …
Cited by 1
- Retracted: The Design of Sports Games under the Internet of Things Fitness by Deep Reinforcement Learning Authors: Computational Intelligence and Neuroscience
IEEE Account
- Change Username/Password
- Update Address
Purchase Details
- Payment Options
- Order History
- View Purchased Documents
Profile Information
- Communications Preferences
- Profession and Education
- Technical Interests
- US & Canada: +1 800 678 4333
- Worldwide: +1 732 981 0060
- Contact & Support
- About IEEE Xplore
- Accessibility
- Terms of Use
- Nondiscrimination Policy
- Privacy & Opting Out of Cookies
A not-for-profit organization, IEEE is the world's largest technical professional organization dedicated to advancing technology for the benefit of humanity. © Copyright 2024 IEEE - All rights reserved. Use of this web site signifies your agreement to the terms and conditions.
Information
- Author Services
Initiatives
You are accessing a machine-readable page. In order to be human-readable, please install an RSS reader.
All articles published by MDPI are made immediately available worldwide under an open access license. No special permission is required to reuse all or part of the article published by MDPI, including figures and tables. For articles published under an open access Creative Common CC BY license, any part of the article may be reused without permission provided that the original article is clearly cited. For more information, please refer to https://www.mdpi.com/openaccess .
Feature papers represent the most advanced research with significant potential for high impact in the field. A Feature Paper should be a substantial original Article that involves several techniques or approaches, provides an outlook for future research directions and describes possible research applications.
Feature papers are submitted upon individual invitation or recommendation by the scientific editors and must receive positive feedback from the reviewers.
Editor’s Choice articles are based on recommendations by the scientific editors of MDPI journals from around the world. Editors select a small number of articles recently published in the journal that they believe will be particularly interesting to readers, or important in the respective research area. The aim is to provide a snapshot of some of the most exciting work published in the various research areas of the journal.
Original Submission Date Received: .
- Active Journals
- Find a Journal
- Proceedings Series
- For Authors
- For Reviewers
- For Editors
- For Librarians
- For Publishers
- For Societies
- For Conference Organizers
- Open Access Policy
- Institutional Open Access Program
- Special Issues Guidelines
- Editorial Process
- Research and Publication Ethics
- Article Processing Charges
- Testimonials
- Preprints.org
- SciProfiles
- Encyclopedia
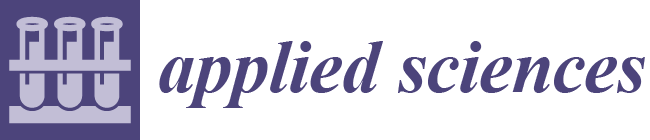
Article Menu

- Subscribe SciFeed
- Recommended Articles
- Google Scholar
- on Google Scholar
- Table of Contents
Find support for a specific problem in the support section of our website.
Please let us know what you think of our products and services.
Visit our dedicated information section to learn more about MDPI.
JSmol Viewer
Technological breakthroughs in sport: current practice and future potential of artificial intelligence, virtual reality, augmented reality, and modern data visualization in performance analysis.
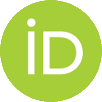
1. Introduction
2. overview, 3. performance analysis in sports.
- Observational Analysis: This refers to the methodical process of gathering, documenting, and assessing data by directly observing athletes or sporting events. It involves trained observers who meticulously record various facets of athletic performance. This analytical approach serves as a foundation for comprehensive assessments of athletes’ strengths, weaknesses, and areas for improvement. It can be performed using just the naked eye and recorded or supplemented with external data from different sources [ 4 , 59 ].
- Video Analysis: A technique that utilizes recorded game or training footage to study and review an athlete’s performance or a team’s tactical approach. It allows for the analysis of various aspects such as skills, techniques, player movement, and team formations. The use of various video speeds, from slow motion to frame-by-frame, enables intricate scrutiny of every action, providing detailed feedback to athletes and coaches [ 11 , 47 , 51 , 52 , 53 , 55 , 60 , 61 ].
- Notational Analysis: This systematic process involves the recording and analysis of discrete events that occur during a match or training session. Each event is notated or coded to provide a quantitative means of recording the performance. The data gathered provide an objective record of performance and can offer insights into the effectiveness of tactics, strategies, and individual player actions [ 10 , 56 , 62 , 63 ].
- Time-Motion Analysis: This is a method used to quantify the physical demands of a sport, by recording and categorizing all movements of an athlete during a match or training session. It provides insight into the duration and intensity of various activities, the frequency of specific movements, and the rest periods. The information gathered can guide training and recovery programs and help evaluate players’ physical performance during the competition [ 57 , 58 , 60 , 61 , 62 , 64 , 65 , 66 ].
- Data Analysis from Wearable Devices: Wearable technology has gained significant traction in sports, with devices like Global Positioning System (GPS) trackers, heart rate monitors, and accelerometers now commonly used. These devices capture a range of physiological and biomechanical data, including heart rate, speed, distance covered, body temperature, and sleep quality. Analysts use this data to monitor player health, access games and training sessions’ external loads, and optimize performance [ 47 , 48 , 49 , 50 , 51 , 57 , 67 , 68 , 69 ].
Computers Evaluation and Performance Analysis in Sports
4. artificial intelligence (ai) in sports performance analysis, 4.1. definition and history, 4.2. how ai can contribute to sports performance analysis.
- Data Collection: AI transforms traditional data collection methods into precise, large-scale processes, reducing manual bias.
- Advanced Data Analysis: AI automates data analysis, identifying hidden patterns and trends within large datasets for performance enhancement and strategy planning.
- Enhanced Video Analysis: AI-driven video analysis is faster, more precise, and more comprehensive than manual reviews, offering insights into player movements, team formations, and tactics.
- Notational Analysis: AI streamlines notational analysis by automating the recognition and annotation of specific actions, improving both efficiency and accuracy.
- Time-Motion Analysis: AI revolutionizes time-motion analysis by providing real-time insights into athlete energy expenditure and fatigue levels, leading to better player substitution strategies.
- Wearable Device Data Analysis: AI analyzes real-time data from wearable devices, predicting potential health risks and suggesting personalized training programs.
- Injury Prevention: AI in computer vision analyzes player biomechanics, identifies harmful patterns, and suggests technique modifications, enhancing player safety.
5. Virtual Reality (VR) and Augmented Reality (AR) in Sports Performance Analysis
5.1. definition and history, 5.2. how vr and ar can contribute to sports performance analysis.
- Creating Immersive Coaching and Training Environments: VR and AR technologies immerse athletes in realistic training scenarios, providing real-time coaching feedback.
- Skill and Strategy Development: These technologies offer interactive, repeatable scenarios that challenge and refine athletes’ skills. Also, to visualize game scenarios, positioning, and tactics in a comprehensive and interactive manner.
- Delivering Tactical Insights: AR overlays digital information onto the physical environment, assisting athletes in making strategic decisions during gameplay.
- Providing Real-time Athlete Feedback: Athletes receive immediate feedback and performance data in their field of vision, allowing adjustments during training.
- Facilitating Data-Driven Decision-Making: Coaches and athletes can make informed decisions by accessing comprehensive performance metrics and tactical insights.
- Supporting Rehabilitation: VR and AR can be used for aiding in injury prevention and rehabilitation.
- Bridging the Gap Between Physical and Mental Preparedness : VR and AR develop cognitive awareness and psychological readiness, complementing physical training for high-pressure situations.
- Visualizing Performance Data: VR and AR help athletes better understand performance data, enhancing their decision-making and mental acuity.
- Enriching Spectator Experiences: AR enhances the viewing experience for spectators by overlaying live broadcasts with dynamic graphics and statistics, providing deeper insights into the game.
6. Data Visualization (DV) in Sports Performance Analysis
6.1. definition and history.
“A picture is worth a thousand words”.
6.2. How DV Can Contribute to the Sports Performance Analysis Traditional Methodology
- Simplified Data Interpretation: DV simplifies complex performance data, making it more understandable.
- Valuable Insights: It helps extract valuable insights from extensive datasets, enabling data-driven decision-making in sports.
- Tactical Analysis: Heatmaps, motion paths, and other visualizations enable coaches to analyze player movements, tactics, and positioning strategies effectively.
- Narrative Power: DV goes beyond numbers, crafting visual narratives that empower coaches, athletes, and analysts to uncover deeper insights.
- Real-Time Decision-Making: Dynamic dashboards with real-time statistics assist coaches and analysts in making better decisions during games, benefiting data-driven broadcasting.
- Historical Data Trends: DV helps in identifying historical trends, strengths, and weaknesses, aiding teams in strategic planning and player selection.
- Predictive Analytics: DV can enable predictions about overall performance, individual player contributions, and team selections based on historical and current data.
7. Discussion
8. conclusions, 9. future directions, author contributions, institutional review board statement, informed consent statement, data availability statement, conflicts of interest.
- Mackenzie, R.; Cushion, C. Performance Analysis in Football: A Critical Review and Implications for Future Research. J. Sport. Sci. 2013 , 31 , 639–676. [ Google Scholar ] [ CrossRef ]
- Araujo, D.; Coucerio, M.; Seifert, L.; Sarmento, H.; Davids, K. Artificial Intelligence for Pattern Recognition in Sports: Classifying Actions and Performance Signatures in Artificial Intelligence in Sport Performance Analysis , 1st ed.; Routledge: London, UK, 2021. [ Google Scholar ]
- Hausdorff, J.M. Gait Dynamics, Fractals and Falls: Finding Meaning in the Stride-to-Stride Fluctuations of Human Walking. Hum. Mov. Sci. 2007 , 26 , 555–589. [ Google Scholar ] [ CrossRef ] [ PubMed ]
- Liebermann, D.G.; Katz, L.; Hughes, M.D.; Bartlett, R.M.; McClements, J.; Franks, I.M. Advances in the Application of Information Technology to Sport Performance. J. Sport. Sci. 2002 , 20 , 755–769. [ Google Scholar ] [ CrossRef ] [ PubMed ]
- Watanabe, N.M.; Shapiro, S.; Drayer, J. Big Data and Analytics in Sport Management. J. Sport Manag. 2021 , 35 , 197–202. [ Google Scholar ] [ CrossRef ]
- Goes, F.R.; Meerhoff, L.A.; Bueno, M.J.O.; Rodrigues, D.M.; Moura, F.A.; Brink, M.S.; Elferink-Gemser, M.T.; Knobbe, A.J.; Cunha, S.A.; Torres, R.S.; et al. Unlocking the Potential of Big Data to Support Tactical Performance Analysis in Professional Soccer: A Systematic Review. Eur. J. Sport Sci. 2021 , 21 , 481–496. [ Google Scholar ] [ CrossRef ] [ PubMed ]
- Rein, R.; Memmert, D. Big Data and Tactical Analysis in Elite Soccer: Future Challenges and Opportunities for Sports Science. SpringerPlus 2016 , 5 , 1410. [ Google Scholar ] [ CrossRef ]
- Hughes, M.D.; Bartlett, R.M. The Use of Performance Indicators in Performance Analysis. J. Sport. Sci. 2002 , 20 , 739–754. [ Google Scholar ] [ CrossRef ] [ PubMed ]
- Hughes, M.; Franks, I. Notational Analysis—A Review of Literature. In Notational Analysis of Sport ; Hughes, M., Franks, I., Eds.; Routledge: London, UK, 2004. [ Google Scholar ]
- O’Donoghue, P. Research Methods for Sports Performance Analysis ; Routledge: London, UK, 2010. [ Google Scholar ]
- Clephas, C.; Foster, M.; Stergiou, P.; Katz, L. Performance Analysis of the Flip Turn in Swimming: The Relationship between Pressures and Performance Times. J. Hum. Sport Exerc. 2022 , 17 , 74–82. [ Google Scholar ] [ CrossRef ]
- Muñoz-Llerena, A.; Caballero-Blanco, P.; Hernández-Hernández, E. Fostering Youth Female Athletes’ Decision-Making Skills through Competitive Volleyball: A Mixed Methods Design. Int. J. Environ. Res. Public Health 2022 , 19 , 13261. [ Google Scholar ] [ CrossRef ]
- Krizkova, S.; Tomaskova, H.; Tirkolaee, E.B. Sport Performance Analysis with a Focus on Racket Sports: A Review. Appl. Sci. 2021 , 11 , 9212. [ Google Scholar ] [ CrossRef ]
- Cust, E.E.; Sweeting, A.J.; Ball, K.; Robertson, S. Machine and Deep Learning for Sport-Specific Movement Recognition: A Systematic Review of Model Development and Performance. J. Sport. Sci. 2019 , 37 , 568–600. [ Google Scholar ] [ CrossRef ] [ PubMed ]
- Sands, W.A.; Kavanaugh, A.A.; Murray, S.R.; McNeal, J.R.; Jemni, M. Modern Techniques and Technologies Applied to Training and Performance Monitoring. Int. J. Sports Physiol. Perform. 2017 , 12 (Suppl. S2), 63–72. [ Google Scholar ] [ CrossRef ] [ PubMed ]
- Lames, M.; McGarry, T. On the Search for Reliable Performance Indicators in Game Sports. Int. J. Perform. Anal. Sport 2007 , 7 , 62–79. [ Google Scholar ] [ CrossRef ]
- Gréhaigne, J.F.; Godbout, P. Dynamic Systems Theory and Team Sport Coaching. Quest 2014 , 66 , 96–116. [ Google Scholar ] [ CrossRef ]
- Libet, J.M.; Lewinsohn, P.M. Concept of Social Skill with Special Reference to the Behavior of Depressed Persons. J. Consult. Clin. Psychol. 1973 , 40 , 304–312. [ Google Scholar ] [ CrossRef ] [ PubMed ]
- Rein, R.; Raabe, D.; Memmert, D. “Which Pass Is Better?” Novel Approaches to Assess Passing Effectiveness in Elite Soccer. Hum. Mov. Sci. 2017 , 55 , 172–181. [ Google Scholar ] [ CrossRef ] [ PubMed ]
- Muthukrishnan, N.; Maleki, F.; Ovens, K.; Reinhold, C.; Forghani, B.; Forghani, R. Brief History of Artificial Intelligence. Neuroimaging Clin. N. Am. 2020 , 30 , 393–399. [ Google Scholar ] [ CrossRef ]
- Jiang, F.; Jiang, Y.; Zhi, H.; Dong, Y.; Li, H.; Ma, S.; Wang, Y.; Dong, Q.; Shen, H.; Wang, Y. Artificial Intelligence in Healthcare: Past, Present and Future. Stroke Vasc. Neurol. 2017 , 2 , 230–243. [ Google Scholar ] [ CrossRef ]
- Chen, Y.; Perez, Y. Business Model Design: Lessons Learned from Tesla Motors. In Towards a Sustainable Economy ; Springer International Publishing AG: Cham, Switzerland, 2018; pp. 53–69. [ Google Scholar ] [ CrossRef ]
- Novatchkov, H.; Baca, A. Artificial Intelligence in Sports on the Example of Weight Training. J. Sport. Sci. Med. 2013 , 12 , 27. [ Google Scholar ]
- Li, L.; Li, L. Summary of the Research Status of Artificial Intelligence in Sports Performance Analysis of Athletes. Open Access Libr. J. 2023 , 10 , e10539. [ Google Scholar ] [ CrossRef ]
- Xu, J. Prediction and Planning of Sports Competition Based on Deep Neural Network. Comput. Intell. Neurosci. 2022 , 2022 , 1906580. [ Google Scholar ] [ CrossRef ] [ PubMed ]
- Sorrentino, R.M.; Levy, R.; Katz, L.; Peng, X. Virtual Visualization: Preparation for the Olympic Games Long-Track Speed Skating. Int. J. Comput. Sci. Sport 2005 , 4 , 39–44. [ Google Scholar ]
- Sorrentino, R.M.; Katz, L. Virtual Reality and Elite Athletes. Med. Sci. Sport. Exerc. 2002 , 34 , 38. [ Google Scholar ] [ CrossRef ]
- Katz, L.; Parker, H.; Levy, R. Virtual Reality. In Computer in Sport ; Dabnichki, P., Baca, A., Eds.; WIT Press: Southampton, SO, USA, 2008; pp. 3–41. [ Google Scholar ]
- Azuma, R.T. A Survey of Augmented Reality. Presence Teleoperators Virtual Environ. 1997 , 6 , 355–385. [ Google Scholar ] [ CrossRef ]
- Michalski, S.C.; Szpak, A.; Loetscher, T. Using Virtual Environments to Improve Real-World Motor Skills in Sports: A Systematic Review. Front. Psychol. 2019 , 10 , 466681. [ Google Scholar ] [ CrossRef ] [ PubMed ]
- Rauschnabel, P.A. Augmented Reality Is Eating the Real-World! The Substitution of Physical Products by Holograms. Int. J. Inf. Manag. 2021 , 57 , 102279. [ Google Scholar ] [ CrossRef ]
- Greenhough, B.; Barrett, S.; Towlson, C.; Abt, G. Perceptions of Professional Soccer Coaches, Support Staff and Players toward Virtual Reality and the Factors That Modify Their Intention to Use It. PLoS ONE 2021 , 16 , e0261378. [ Google Scholar ] [ CrossRef ]
- Le Noury, P.; Polman, R.; Maloney, M.; Gorman, A. A Narrative Review of the Current State of Extended Reality Technology and How It Can Be Utilised in Sport. Sports Med. 2022 , 52 , 1473–1489. [ Google Scholar ] [ CrossRef ]
- Neumann, D.L.; Moffitt, R.L.; Thomas, P.R.; Loveday, K.; Watling, D.P.; Lombard, C.L.; Antonova, S.; Tremeer, M.A. A Systematic Review of the Application of Interactive Virtual Reality to Sport. Virtual Real. 2018 , 22 , 183–198. [ Google Scholar ] [ CrossRef ]
- Bideau, B.; Kulpa, R.; Vignais, N.; Brault, S.; Multon, F.; Craig, C. Using Virtual Reality to Analyze Sports Performance. IEEE Comput. Graph Appl. 2010 , 30 , 14–21. [ Google Scholar ] [ CrossRef ]
- Faure, C.; Limballe, A.; Bideau, B.; Kulpa, R. Virtual Reality to Assess and Train Team Ball Sports Performance: A Scoping Review. J. Sport. Sci. 2020 , 38 , 192–205. [ Google Scholar ] [ CrossRef ] [ PubMed ]
- Pastel, S.; Marlok, J.; Bandow, N.; Witte, K. Application of Eye-Tracking Systems Integrated into Immersive Virtual Reality and Possible Transfer to the Sports Sector—A Systematic Review. Multimed. Tools Appl. 2023 , 82 , 4181–4208. [ Google Scholar ] [ CrossRef ]
- Liu, P.; Lu, L.; Zhang, J.; Huo, T.; Liu, S.; Ye, Z. Application of Artificial Intelligence in Medicine: An Overview. Curr. Med. Sci. 2021 , 41 , 1105–1115. [ Google Scholar ] [ CrossRef ] [ PubMed ]
- Provost, F.; Fawcett, T. Data Science for Business: What You Need to Know about Data Mining and Data-Analytic Thinking , 1st ed.; O’Reilly Media: Sebastopol, CA, USA, 2013. [ Google Scholar ]
- Knaflic, C.N. Storytelling with Data: A Data Visualization Guide for Business Professionals , 1st ed.; Wiley: Hoboken, NJ, USA, 2015. [ Google Scholar ]
- Fry, M.J.; Ohlmann, J.W. Introduction to the Special Issue on Analytics in Sports, Part II: Sports Scheduling Applications. Interfaces 2012 , 42 , 229–231. [ Google Scholar ] [ CrossRef ]
- Fry, M.J.; Ohlmann, J.W. Introduction to the Special Issue on Analytics in Sports, Part I: General Sports Applications. Interfaces 2012 , 42 , 105–108. [ Google Scholar ] [ CrossRef ]
- Yang, H.; Luo, C. Accuracy Analysis of Sports Performance Prediction Based on BP Neural Network Intelligent Algorithm. Secur. Commun. Netw. 2022 , 2022 , 4198920. [ Google Scholar ] [ CrossRef ]
- Sarlis, V.; Chatziilias, V.; Tjortjis, C.; Mandalidis, D. A Data Science Approach Analysing the Impact of Injuries on Basketball Player and Team Performance. Inf. Syst. 2021 , 99 , 101750. [ Google Scholar ] [ CrossRef ]
- Pino-Ortega, J.; Rojas-Valverde, D.; Gómez-Carmona, C.D.; Rico-González, M. Training Design, Performance Analysis, and Talent Identification-A Systematic Review about the Most Relevant Variables through the Principal Component Analysis in Soccer, Basketball, and Rugby. Int. J. Environ. Res. Public Health 2021 , 18 , 2642. [ Google Scholar ] [ CrossRef ]
- Lutz, J.; Memmert, D.; Raabe, D.; Dornberger, R.; Donath, L. Wearables for Integrative Performance and Tactic Analyses: Opportunities, Challenges, and Future Directions. Int. J. Environ. Res. Public Health 2019 , 17 , 59. [ Google Scholar ] [ CrossRef ]
- Freitas, R.; Volossovitch, A.; Almeida, C.H.; Vleck, V. Elite-Level Defensive Performance in Football: A Systematic Review. Ger. J. Exerc. Sport Res. 2023 , 53 , 458–470. [ Google Scholar ] [ CrossRef ]
- Strauss, A.; Sparks, M.; Pienaar, C. The Use of GPS Analysis to Quantify the Internal and External Match Demands of Semi-Elite Level Female Soccer Players during a Tournament. J. Sport. Sci. Med. 2019 , 18 , 73. [ Google Scholar ] [ PubMed ]
- Pons, E.; García-Calvo, T.; Resta, R.; Blanco, H.; del Campo, R.L.; García, J.D.; Pulido, J.J. A Comparison of a GPS Device and a Multi-Camera Video Technology during Official Soccer Matches: Agreement between Systems. PLoS ONE 2019 , 14 , e0220729. [ Google Scholar ] [ CrossRef ] [ PubMed ]
- Grainger, M.; Weisberg, A.; Stergiou, P.; Katz, L. Comparison of Two Methods in the Estimation of Vertical Jump Height. J. Hum. Sport Exerc. 2020 , 15 , 623–632. [ Google Scholar ] [ CrossRef ]
- Rana, M.; Mittal, V. Wearable Sensors for Real-Time Kinematics Analysis in Sports: A Review. IEEE Sens. J. 2021 , 21 , 1187–1207. [ Google Scholar ] [ CrossRef ]
- Hendricks, S.; Till, K.; Den Hollander, S.; Savage, T.N.; Roberts, S.P.; Tierney, G.; Burger, N.; Kerr, H.; Kemp, S.; Cross, M.; et al. Consensus on a Video Analysis Framework of Descriptors and Definitions by the Rugby Union Video Analysis Consensus Group. Br. J. Sports Med. 2020 , 54 , 566–572. [ Google Scholar ] [ CrossRef ] [ PubMed ]
- Linke, D.; Link, D.; Lames, M. Football-Specific Validity of TRACAB’s Optical Video Tracking Systems. PLoS ONE 2020 , 15 , e0230179. [ Google Scholar ] [ CrossRef ] [ PubMed ]
- Clephas, C.; Stergiou, P.; Tyreman, H.; Katz, L. Predicting Olympic Success by Regression Modeling in Sport—An Analysis of the Beginning of the 21st Century. In Proceedings of the 13th World Congress of Performance Analysis of Sport and 13th International Symposium on Computer Science in Sport, Vienna, Austria, 10–13 September 2023; pp. 60–63. [ Google Scholar ] [ CrossRef ]
- Macaulay, C.A.J.; Katz, L.; Stergiou, P.; Stefanyshyn, D.; Tomaghelli, L. Kinematic and Kinetic Analysis of Overhand, Sidearm and Underhand Lacrosse Shot Techniques. J. Sport. Sci. 2017 , 35 , 2350–2356. [ Google Scholar ] [ CrossRef ] [ PubMed ]
- Andreassen, K.; Johansen, D.; Johansen, H.; Baptista, I.; Pettersen, S.A.; Riegler, M.; Halvorsen, P. Real-Time Analysis of Physical Performance Parameters in Elite Soccer. In Proceedings of the International Conference on Content-Based Multimedia Indexing (CBMI), Dublin, Ireland, 4–6 September 2019. [ Google Scholar ] [ CrossRef ]
- Palucci Vieira, L.H.; Carling, C.; Barbieri, F.A.; Aquino, R.; Santiago, P.R.P. Match Running Performance in Young Soccer Players: A Systematic Review. Sport. Med. 2019 , 49 , 289–318. [ Google Scholar ] [ CrossRef ]
- Murtagh, C.F.; Naughton, R.J.; McRobert, A.P.; O’Boyle, A.; Morgans, R.; Drust, B.; Erskine, R.M. A Coding System to Quantify Powerful Actions in Soccer Match Play: A Pilot Study. Res. Q. Exerc. Sport 2019 , 90 , 234–243. [ Google Scholar ] [ CrossRef ]
- Hopkins, W.G. Quantification of Training in Competitive Sports. Methods and Applications. Sports Med. 1991 , 12 , 161–183. [ Google Scholar ] [ CrossRef ]
- Pappalardo, L.; Cintia, P.; Rossi, A.; Massucco, E.; Ferragina, P.; Pedreschi, D.; Giannotti, F. A Public Data Set of Spatio-Temporal Match Events in Soccer Competitions. Sci. Data 2019 , 6 , 236. [ Google Scholar ] [ CrossRef ] [ PubMed ]
- Di Salvo, V.; Gregson, W.; Atkinson, G.; Tordoff, P.; Drust, B. Analysis of High Intensity Activity in Premier League Soccer. Int. J. Sports Med. 2009 , 30 , 205–212. [ Google Scholar ] [ CrossRef ] [ PubMed ]
- Carling, C. Analysis of Physical Activity Profiles When Running with the Ball in a Professional Soccer Team. J. Sport. Sci. 2010 , 28 , 319–326. [ Google Scholar ] [ CrossRef ] [ PubMed ]
- Wright, C.; Atkins, S.; Polman, R.; Jones, B.; Sargeson, L. Factors Associated with Goals and Goal Scoring Opportunities in Professional Soccer. Int. J. Perform. Anal. Sport 2011 , 11 , 439–449. [ Google Scholar ] [ CrossRef ]
- Mohr, M.; Krustrup, P.; Bangsbo, J. Match Performance of High-Standard Soccer Players with Special Reference to Development of Fatigue. J. Sport. Sci. 2003 , 21 , 519–528. [ Google Scholar ] [ CrossRef ] [ PubMed ]
- Di Salvo, V.; Baron, R.; Tschan, H.; Calderon Montero, F.J.; Bachl, N.; Pigozzi, F. Performance Characteristics According to Playing Position in Elite Soccer. Int. J. Sports Med. 2007 , 28 , 222–227. [ Google Scholar ] [ CrossRef ] [ PubMed ]
- Rampinini, E.; Impellizzeri, F.M.; Castagna, C.; Coutts, A.J.; Wisløff, U. Technical Performance during Soccer Matches of the Italian Serie A League: Effect of Fatigue and Competitive Level. J. Sci. Med. Sport 2009 , 12 , 227–233. [ Google Scholar ] [ CrossRef ] [ PubMed ]
- de Magalhaes, F.A.; Vannozzi, G.; Gatta, G.; Fantozzi, S. Wearable Inertial Sensors in Swimming Motion Analysis: A Systematic Review. J. Sport. Sci. 2015 , 33 , 732–745. [ Google Scholar ] [ CrossRef ]
- Horton, J.F.; Stergiou, P.; Fung, T.S.; Katz, L. Comparison of Polar M600 Optical Heart Rate and ECG Heart Rate during Exercise. Med. Sci. Sports Exerc. 2017 , 49 , 2600–2607. [ Google Scholar ] [ CrossRef ]
- Benson, L.C.; Clermont, C.A.; Bošnjak, E.; Ferber, R. The Use of Wearable Devices for Walking and Running Gait Analysis Outside of the Lab: A Systematic Review. Gait Posture 2018 , 63 , 124–138. [ Google Scholar ] [ CrossRef ]
- Casals, M.; Finch, C.F. Sports Biostatistician: A Critical Member of All Sports Science and Medicine Teams for Injury Prevention. Br. J. Sports Med. 2018 , 52 , 1457–1461. [ Google Scholar ] [ CrossRef ]
- Wright, C.; Atkins, S.; Jones, B.; Todd, J. The Role of Performance Analysts within the Coaching Process: Performance Analysts Survey “The Role of Performance Analysts in Elite Football Club Settings”. Int. J. Perform. Anal. Sport 2013 , 13 , 240–261. [ Google Scholar ] [ CrossRef ]
- Nicholls, S.B.; James, N.; Wells, J.; Parmar, N. Performance Analysis Practice within Olympic and Paralympic Sports: A Comparison of Coach and Analyst Experiences. Int. J. Perform. Anal. Sport 2022 , 22 , 343–351. [ Google Scholar ] [ CrossRef ]
- Wright, C.; Atkins, S.; Jones, B. An Analysis of Elite Coaches’ Engagement with Performance Analysis Services (Match, Notational Analysis and Technique Analysis). Int. J. Perform. Anal. Sport 2012 , 12 , 436–451. [ Google Scholar ] [ CrossRef ]
- Edwards, A.D.N. The Rise of the Graphical User Interface. Libr. Hi Tech 1996 , 14 , 46–50. [ Google Scholar ] [ CrossRef ]
- Oulasvirta, A.; Dayama, N.R.; Shiripour, M.; John, M.; Karrenbauer, A. Combinatorial Optimization of Graphical User Interface Designs. Proc. IEEE 2020 , 108 , 434–464. [ Google Scholar ] [ CrossRef ]
- Glowniak, J. The Internet as an Information Source for Geriatricians. Drugs Aging 1997 , 10 , 169–173. [ Google Scholar ] [ CrossRef ] [ PubMed ]
- Berndt, E.R.; Rappaport, N.J. Price and Quality of Desktop and Mobile Personal Computers: A Quarter-Century Historical Overview. Am. Econ. Rev. 2001 , 91 , 268–273. [ Google Scholar ] [ CrossRef ]
- Ross, S.J.; Hill, J.L.; Chen, M.Y.; Joseph, A.D.; Culler, D.E.; Brewer, E.A. A Composable Framework for Secure Multi-Modal Access to Internet Services from Post-PC Devices. Mob. Netw. Appl. 2002 , 7 , 389–406. [ Google Scholar ] [ CrossRef ]
- Makki, S.A.M.; Pissinou, N.; Daroux, P. Mobile and Wireless Internet Access. Comput. Commun. 2003 , 26 , 734–746. [ Google Scholar ] [ CrossRef ]
- Ko, A.J.; Abraham, R.; Beckwith, L.; Blackwell, A.; Burnett, M.; Erwig, M.; Scaffidi, C.; Lawrance, J.; Lieberman, H.; Myers, B.; et al. The State of the Art in End-User Software Engineering. ACM Comput. Surv. 2011 , 43 , 1–44. [ Google Scholar ] [ CrossRef ]
- Herbig, P.A.; Kramer, H. The Effect of Information Overload on the Innovation Choice Process. J. Consum. Mark. 1994 , 11 , 45–54. [ Google Scholar ] [ CrossRef ]
- Park, H.J.; Zhang, Y. Technology Readiness and Technology Paradox of Unmanned Convenience Store Users. J. Retail. Consum. Serv. 2022 , 65 , 102523. [ Google Scholar ] [ CrossRef ]
- Sagiroglu, S.; Sinanc, D. Big Data: A Review. In Proceedings of the International Conference on Collaboration Technologies and Systems (CTS), San Diego, CA, USA, 20–24 May 2013. [ Google Scholar ] [ CrossRef ]
- Passfield, L.; Hopker, J.G. A Mine of Information: Can Sports Analytics Provide Wisdom from Your Data? Int. J. Sports Physiol. Perform. 2017 , 12 , 851–855. [ Google Scholar ] [ CrossRef ] [ PubMed ]
- Gomez-Ruano, M.A.; Ibáñez, S.J.; Leicht, A.S. Editorial: Performance Analysis in Sport. Front. Psychol. 2020 , 11 , 611634. [ Google Scholar ] [ CrossRef ] [ PubMed ]
- Morgulev, E.; Azar, O.H.; Lidor, R. Sports Analytics and the Big-Data Era. Int. J. Data Sci. Anal. 2018 , 5 , 213–222. [ Google Scholar ] [ CrossRef ]
- Isichei, B.C.; Leung, C.K.; Nguyen, L.T.; Morrow, L.B.; Ngo, A.T.; Pham, T.D.; Cuzzocrea, A. Sports Data Management, Mining, and Visualization ; Lecture Notes in Networks and Systems; Springer: Cham, Switzerland, 2022; Volume 450 LNNS, pp. 141–153. [ Google Scholar ] [ CrossRef ]
- McCulloch, W.S.; Pitts, W. A Logical Calculus of the Ideas Immanent in Nervous Activity. Bull. Math. Biophys. 1943 , 5 , 115–133. [ Google Scholar ] [ CrossRef ]
- Turing, A.M.I. Computing machinery and intelligence. Mind 1950 , LIX , 433–460. [ Google Scholar ] [ CrossRef ]
- McCarthy, J.; Minsky, M.L.; Rochester, N.; Shannon, C.E. A Proposal for the Dartmouth Summer Research Project on Artificial Intelligence, August 31, 1955. AI Mag. 2006 , 27 , 12. [ Google Scholar ] [ CrossRef ]
- Rosenblatt, F. The Perceptron: A Probabilistic Model for Information Storage and Organization in the Brain. Psychol. Rev. 1958 , 65 , 386–408. [ Google Scholar ] [ CrossRef ]
- Hendler, J. Avoiding Another AI Winter. IEEE Intell. Syst. 2008 , 23 , 2–4. [ Google Scholar ] [ CrossRef ]
- Russell, S.; Norvig, P. Artificial Intelligence: A Modern Approach , 3rd ed.; Pearson: London, UK, 2009. [ Google Scholar ]
- Janiesch, C.; Zschech, P.; Heinrich, K. Machine Learning and Deep Learning. Electron. Mark. 2021 , 31 , 685–695. [ Google Scholar ] [ CrossRef ]
- Radford, A.; Wu, J.; Child, R.; Luan, D.; Amodei, D.; Sutskever, I. Language Models Are Unsupervised Multitask Learners. OpenAi Blog 2019 , 1 , 9. [ Google Scholar ]
- Openai, A.R.; Openai, K.N.; Openai, T.S.; Openai, I.S. Improving Language Understanding by Generative Pre-Training. OpenAi Blog 2018 . [ Google Scholar ]
- Brown, T.B.; Mann, B.; Ryder, N.; Subbiah, M.; Kaplan, J.; Dhariwal, P.; Neelakantan, A.; Shyam, P.; Sastry, G.; Askell, A.; et al. Language Models Are Few-Shot Learners. In Proceedings of the Advances in Neural Information Processing Systems 33 (NeurIPS 2020), Virtual, 6–12 December 2020. [ Google Scholar ]
- Zemková, E.; Plakias, S.; Moustakidis, S.; Kokkotis, C.; Papalexi, M.; Tsatalas, T.; Giakas, G.; Tsaopoulos, D. Identifying Soccer Players’ Playing Styles: A Systematic Review. J. Funct. Morphol. Kinesiol. 2023 , 8 , 104. [ Google Scholar ] [ CrossRef ]
- Goodfellow, Y.; Bengio, Y.; Courville, A. Deep Learning ; The MIT Press: Cambridge, MA, USA, 2018. [ Google Scholar ]
- Lecun, Y.; Bengio, Y.; Hinton, G. Deep Learning. Nature 2015 , 521 , 436–444. [ Google Scholar ] [ CrossRef ]
- Claudino, J.G.; Capanema, D.d.O.; de Souza, T.V.; Serrão, J.C.; Machado Pereira, A.C.; Nassis, G.P. Current Approaches to the Use of Artificial Intelligence for Injury Risk Assessment and Performance Prediction in Team Sports: A Systematic Review. Sport. Med. Open 2019 , 5 , 28. [ Google Scholar ] [ CrossRef ]
- López-Valenciano, A.; Ayala, F.; Puerta, J.M.; De Ste Croix, M.B.A.; Vera-Garcia, F.J.; Hernández-Sánchez, S.; Ruiz-Pérez, I.; Myer, G.D. A Preventive Model for Muscle Injuries: A Novel Approach Based on Learning Algorithms. Med. Sci. Sports Exerc. 2018 , 50 , 915–927. [ Google Scholar ] [ CrossRef ]
- Host, K.; Ivašić-Kos, M. An Overview of Human Action Recognition in Sports Based on Computer Vision. Heliyon 2022 , 8 , e09633. [ Google Scholar ] [ CrossRef ]
- Naik, B.T.; Hashmi, M.F.; Bokde, N.D. A Comprehensive Review of Computer Vision in Sports: Open Issues, Future Trends and Research Directions. Appl. Sci. 2022 , 12 , 4429. [ Google Scholar ] [ CrossRef ]
- Przednowek, K.; Krzeszowski, T.; Przednowek, K.H.; Lenik, P. A System for Analysing the Basketball Free Throw Trajectory Based on Particle Swarm Optimization. Appl. Sci. 2018 , 8 , 2090. [ Google Scholar ] [ CrossRef ]
- Wang, T.; Li, T. Deep Learning-Based Football Player Detection in Videos. Comput. Intell. Neurosci. 2022 , 2022 , 3540642. [ Google Scholar ] [ CrossRef ] [ PubMed ]
- Pai, P.F.; ChangLiao, L.H.; Lin, K.P. Analyzing Basketball Games by a Support Vector Machines with Decision Tree Model. Neural Comput. Appl. 2017 , 28 , 4159–4167. [ Google Scholar ] [ CrossRef ]
- Seshadri, D.R.; Li, R.T.; Voos, J.E.; Rowbottom, J.R.; Alfes, C.M.; Zorman, C.A.; Drummond, C.K. Wearable Sensors for Monitoring the Internal and External Workload of the Athlete. NPJ Digit. Med. 2019 , 2 , 71. [ Google Scholar ] [ CrossRef ] [ PubMed ]
- Kempe, M.; Grunz, A.; Memmert, D. Detecting Tactical Patterns in Basketball: Comparison of Merge Self-Organising Maps and Dynamic Controlled Neural Networks. Eur. J. Sport Sci. 2015 , 15 , 249–255. [ Google Scholar ] [ CrossRef ] [ PubMed ]
- Rauschnabel, P.A.; Felix, R.; Hinsch, C.; Shahab, H.; Alt, F. What Is XR? Towards a Framework for Augmented and Virtual Reality. Comput. Hum. Behav. 2022 , 133 , 107289. [ Google Scholar ] [ CrossRef ]
- Dargan, S.; Bansal, S.; Kumar, M.; Mittal, A.; Kumar, K. Augmented Reality: A Comprehensive Review. Arch. Comput. Methods Eng. 2023 , 30 , 1057–1080. [ Google Scholar ] [ CrossRef ]
- Milgram, P.; Takemura, H.; Utsumi, A.; Kishino, F. Augmented Reality: A Class of Displays on the Reality-Virtuality Continuum. In Telemanipulator and Telepresence Technologies ; SPIE: Bellingham, WA, USA, 1995; Volume 2351, pp. 282–292. [ Google Scholar ] [ CrossRef ]
- Gutierrez, N. The Ballad of Morton Heilig: On VR’s Mythic Past. JCMS J. Cine Media Stud. 2023 , 62 , 86–106. [ Google Scholar ] [ CrossRef ]
- Sutherland, I. A Head-Mounted Three Dimensional Display. In Proceedings of the AFIPS ’68, San Francisco, CA, USA, 9–11 December 1968; Volume 33, pp. 757–764. [ Google Scholar ] [ CrossRef ]
- Lanier, J. Virtual Reality The Promise of the Future. Interact. Learn. Int. 1992 , 8 , 275–279. [ Google Scholar ]
- Cruz-Neira, C.; Sandin, D.J.; DeFanti, T.A.; Kenyon, R.V.; Hart, J.C. The CAVE: Audio Visual Experience Automatic Virtual Environment. Commun. ACM 1992 , 35 , 64–72. [ Google Scholar ] [ CrossRef ]
- Berryman, D.R. Augmented Reality: A Review. Med. Ref. Serv. Q. 2012 , 31 , 212–218. [ Google Scholar ] [ CrossRef ] [ PubMed ]
- Berton, A.; Longo, U.G.; Candela, V.; Fioravanti, S.; Giannone, L.; Arcangeli, V.; Alciati, V.; Berton, C.; Facchinetti, G.; Marchetti, A.; et al. Virtual Reality, Augmented Reality, Gamification, and Telerehabilitation: Psychological Impact on Orthopedic Patients’ Rehabilitation. J. Clin. Med. 2020 , 9 , 2567. [ Google Scholar ] [ CrossRef ] [ PubMed ]
- Brepohl, P.C.A.; Leite, H. Virtual Reality Applied to Physiotherapy: A Review of Current Knowledge. Virtual Real. 2022 , 27 , 71–95. [ Google Scholar ] [ CrossRef ]
- Lin, Y.T.; Lee, W.C.; Hsieh, R.L. Active Video Games for Knee Osteoarthritis Improve Mobility but Not WOMAC Score: A Randomized Controlled Trial. Ann. Phys. Rehabil. Med. 2020 , 63 , 458–465. [ Google Scholar ] [ CrossRef ]
- Carnevale, A.; Mannocchi, I.; Sassi, M.S.H.; Carli, M.; De Luca, G.; Longo, U.G.; Denaro, V.; Schena, E. Virtual Reality for Shoulder Rehabilitation: Accuracy Evaluation of Oculus Quest 2. Sensors 2022 , 22 , 5511. [ Google Scholar ] [ CrossRef ]
- Tanaka, K.; Parker, J.R.; Baradoy, G.; Sheehan, D.; Holash, J.R.; Katz, L. A Comparison of Exergaming Interfaces for Use in Rehabilitation Programs and Research. Loading 2012 , 6 , 69–81. [ Google Scholar ]
- Shen, Y.; Ong, S.K.; Nee, A.Y.C. Augmented Reality for Collaborative Product Design and Development. Des. Stud. 2010 , 31 , 118–145. [ Google Scholar ] [ CrossRef ]
- Savela, N.; Oksanen, A.; Kaakinen, M.; Noreikis, M.; Xiao, Y. Does Augmented Reality Affect Sociability, Entertainment, and Learning? A Field Experiment. Appl. Sci. 2020 , 10 , 1392. [ Google Scholar ] [ CrossRef ]
- Campelo, A.M.; Katz, L. Older Adults’ Perceptions of the Usefulness of Technologies for Engaging in Physical Activity: Using Focus Groups to Explore Physical Literacy. Int. J. Environ. Res. Public Health 2020 , 17 , 1144. [ Google Scholar ] [ CrossRef ]
- Campelo, A.M.; Weisberg, A.; Sheehan, D.P.; Schneider, K.; Cossich, V.R.A.; Katz, L. Physical and Affective Physical Literacy Domains Improved after a Six-Week Exergame Exercise Program in Older Adults: A Randomized Controlled Clinical Trial. Games Health J. 2023 , 12 , 366–376. [ Google Scholar ] [ CrossRef ]
- Quqandi, E.; Joy, M.; Drumm, I.; Rushton, M. Augmented Reality in Supporting Healthcare and Nursing Independent Learning: Narrative Review. Comput. Inform. Nurs. 2023 , 41 , 281–291. [ Google Scholar ] [ CrossRef ] [ PubMed ]
- Vidal-Balea, A.; Blanco-Novoa, O.; Picallo-Guembe, I.; Celaya-Echarri, M.; Fraga-Lamas, P.; Lopez-Iturri, P.; Azpilicueta, L.; Falcone, F.; Fernández-Caramés, T.M. Analysis, Design and Practical Validation of an Augmented Reality Teaching System Based on Microsoft HoloLens 2 and Edge Computing. Eng. Proc. 2020 , 2 , 52. [ Google Scholar ] [ CrossRef ]
- Wyss, C.; Bührer, W.; Furrer, F.; Degonda, A.; Hiss, J.A. Innovative Teacher Education with the Augmented Reality Device Microsoft Hololens—Results of an Exploratory Study and Pedagogical Considerations. Multimodal Technol. Interact. 2021 , 5 , 45. [ Google Scholar ] [ CrossRef ]
- du Système Nerveux, C. Anaerobic upper and lower body power measurements and perception of fatigue during a kick boxing match. J. Sports Med. Phys. Fitness 2013 , 53 , 455–460. [ Google Scholar ]
- Putranto, J.S.; Heriyanto, J.; Kenny; Achmad, S.; Kurniawan, A. Implementation of Virtual Reality Technology for Sports Education and Training: Systematic Literature Review. Procedia Comput. Sci. 2023 , 216 , 293–300. [ Google Scholar ] [ CrossRef ]
- Pagé, C.; Bernier, P.M.; Trempe, M. Using Video Simulations and Virtual Reality to Improve Decision-Making Skills in Basketball. J. Sport. Sci. 2019 , 37 , 2403–2410. [ Google Scholar ] [ CrossRef ]
- Tanaka, K. 3D Action Reconstruction Using Virtual Player to Assist Karate Training. In Proceedings of the 2017 IEEE Virtual Reality (VR), Los Angeles, CA, USA, 18–22 March 2017; pp. 395–396. [ Google Scholar ] [ CrossRef ]
- Thatcher, B.; Ivanov, G.; Szerovay, M.; Mills, G. Virtual Reality Technology in Football Coaching: Barriers and Opportunities. Int. Sport Coach. J. 2020 , 8 , 234–243. [ Google Scholar ] [ CrossRef ]
- Panchuk, D.; Klusemann, M.J.; Hadlow, S.M. Exploring the Effectiveness of Immersive Video for Training Decision-Making Capability in Elite, Youth Basketball Players. Front. Psychol. 2018 , 9 , 356501. [ Google Scholar ] [ CrossRef ]
- Soltani, P.; Morice, A.H.P. Augmented Reality Tools for Sports Education and Training. Comput. Educ. 2020 , 155 , 103923. [ Google Scholar ] [ CrossRef ]
- Bozyer, Z. Augmented Reality in Sports: Today and Tomorrow. Int. J. Sci. Cult. Sport 2015 , 3 , 314. [ Google Scholar ] [ CrossRef ]
- Sawan, N.; Eltweri, A.; De Lucia, C.; Cavaliere, L.P.L.; Faccia, A.; Moşteanu, N.R. Mixed and Augmented Reality Applications in the Sport Industry. In Proceedings of the EBEE ‘20: Proceedings of the 2020 2nd International Conference on E-Business and E-commerce Engineering, Bangkok, Thailand, 29–31 December 2020; pp. 55–59. [ Google Scholar ] [ CrossRef ]
- Goebert, C.; Greenhalgh, G.P. A New Reality: Fan Perceptions of Augmented Reality Readiness in Sport Marketing. Comput. Human Behav. 2020 , 106 , 106231. [ Google Scholar ] [ CrossRef ]
- Goebert, C. Augmented Reality in Sport Marketing: Uses and Directions. Sport. Innov. J. 2020 , 1 , 134–151. [ Google Scholar ] [ CrossRef ] [ PubMed ]
- Kittel, A.; Larkin, P.; Cunningham, I.; Spittle, M. 360° Virtual Reality: A SWOT Analysis in Comparison to Virtual Reality. Front. Psychol. 2020 , 11 , 563474. [ Google Scholar ] [ CrossRef ] [ PubMed ]
- Fortes, L.S.; Almeida, S.S.; Praça, G.M.; Nascimento-Júnior, J.R.A.; Lima-Junior, D.; Barbosa, B.T.; Ferreira, M.E.C. Virtual Reality Promotes Greater Improvements than Video-Stimulation Screen on Perceptual-Cognitive Skills in Young Soccer Athletes. Hum. Mov. Sci. 2021 , 79 , 102856. [ Google Scholar ] [ CrossRef ]
- Wood, G.; Wright, D.J.; Harris, D.; Pal, A.; Franklin, Z.C.; Vine, S.J. Testing the Construct Validity of a Soccer-Specific Virtual Reality Simulator Using Novice, Academy, and Professional Soccer Players. Virtual Real. 2021 , 25 , 43–51. [ Google Scholar ] [ CrossRef ]
- Düking, P.; Holmberg, H.C.; Sperlich, B. The Potential Usefulness of Virtual Reality Systems for Athletes: A Short SWOT Analysis. Front. Physiol. 2018 , 9 , 322635. [ Google Scholar ] [ CrossRef ] [ PubMed ]
- Mascret, N.; Montagne, G.; Devrièse-Sence, A.; Vu, A.; Kulpa, R. Acceptance by Athletes of a Virtual Reality Head-Mounted Display Intended to Enhance Sport Performance. Psychol. Sport Exerc. 2022 , 61 , 102201. [ Google Scholar ] [ CrossRef ]
- Anderson, F.; Grossman, T.; Matejka, J.; Fitzmaurice, G. YouMove: Enhancing Movement Training with an Augmented Reality Mirror. In Proceedings of the 26th Annual ACM Symposium on User Interface Software and Technology, St. Andrews, UK, 8–11 October 2013; pp. 311–320. [ Google Scholar ] [ CrossRef ]
- Kiani, S.; Rezaei, I.; Abasi, S.; Zakerabasali, S.; Yazdani, A. Technical Aspects of Virtual Augmented Reality-Based Rehabilitation Systems for Musculoskeletal Disorders of the Lower Limbs: A Systematic Review. BMC Musculoskelet. Disord. 2023 , 24 , 4. [ Google Scholar ] [ CrossRef ]
- Lal, H.; Mohanta, S.; Kumar, J.; Patralekh, M.K.; Lall, L.; Katariya, H.; Arya, R.K. Telemedicine-Rehabilitation and Virtual Reality in Orthopaedics and Sports Medicine. Indian J. Orthop. 2022 , 57 , 7–19. [ Google Scholar ] [ CrossRef ]
- Chen, Z.; Ye, S.; Chu, X.; Xia, H.; Zhang, H.; Qu, H.; Wu, Y. Augmenting Sports Videos with VisCommentator. IEEE Trans. Vis. Comput. Graph. 2023 , 28 , 824–834. [ Google Scholar ] [ CrossRef ]
- Harris, D.J.; Buckingham, G.; Wilson, M.R.; Brookes, J.; Mushtaq, F.; Mon-Williams, M.; Vine, S.J. Exploring Sensorimotor Performance and User Experience within a Virtual Reality Golf Putting Simulator. Virtual Real. 2021 , 25 , 647–654. [ Google Scholar ] [ CrossRef ]
- Le Noury, P.; Buszard, T.; Reid, M.; Farrow, D. Examining the Representativeness of a Virtual Reality Environment for Simulation of Tennis Performance. J. Sport. Sci. 2021 , 39 , 412–420. [ Google Scholar ] [ CrossRef ] [ PubMed ]
- Adams, K.; Kiefer, A.; Panchuk, D.; Hunter, A.; MacPherson, R.; Spratford, W. From the Field of Play to the Laboratory: Recreating the Demands of Competition with Augmented Reality Simulated Sport. J. Sport. Sci. 2020 , 38 , 486–493. [ Google Scholar ] [ CrossRef ] [ PubMed ]
- Petri, K.; Bandow, N.; Masik, S.; Witte, K. Improvement of Early Recognition of Attacks in Karate Kumite Due to Training in Virtual Reality. J. Sport Area 2019 , 4 , 294–308. [ Google Scholar ] [ CrossRef ] [ PubMed ]
- Nimphius, S.; Jordan, M.J. Show Me the Data, Jerry! Data Visualization and Transparency. Int. J. Sports Physiol. Perform. 2020 , 15 , 1353–1355. [ Google Scholar ] [ CrossRef ]
- Bertamini, M.; Makin, A.D.J. Brain Activity in Response to Visual Symmetry. Symmetry 2014 , 6 , 975–996. [ Google Scholar ] [ CrossRef ]
- Perin, C.; Vuillemot, R.; Stolper, C.D.; Stasko, J.T.; Wood, J.; Carpendale, S. State of the Art of Sports Data Visualization. Comput. Graph Forum 2018 , 37 , 663–686. [ Google Scholar ] [ CrossRef ]
- Wainer, H. Graphical Visions from William Playfair to John Tukey. Stat. Sci. 1990 , 5 , 340–346. [ Google Scholar ] [ CrossRef ]
- Spence, I. William Playfair and the Psychology of Graphs. In Proceedings of the 2006 JSM Proceedings: Papers presented at the Joint Statistical Meetings, Seattle, WA, USA, 6–10 August 2006; pp. 2426–2436. [ Google Scholar ]
- Bostock, M.; Ogievetsky, V.; Heer, J. D 3 : Data-Driven Documents. IEEE Trans. Vis. Comput. Graph. 2010 , 17 , 2301–2309. [ Google Scholar ] [ CrossRef ]
- Heer, J.; Shneiderman, B. Interactive Dynamics for Visual Analysis. Commun. ACM 2012 , 55 , 45–54. [ Google Scholar ] [ CrossRef ]
- Zaremski, J.L.; Pazik, M.; Duncan, J.; Horodyski, M. Infographic. Workload in Throwing Dominant Sports: An Infographic Summary. Br. J. Sports Med. 2023 , 57 , 181–182. [ Google Scholar ] [ CrossRef ] [ PubMed ]
- Vuillemot, R.; Perin, C. Sports Tournament Predictions Using Direct Manipulation. IEEE Comput. Graph Appl. 2016 , 36 , 62–71. [ Google Scholar ] [ CrossRef ] [ PubMed ]
- Lage, M.; Ono, J.P.; Cervone, D.; Chiang, J.; Dietrich, C.; Silva, C.T. StatCast Dashboard: Exploration of Spatiotemporal Baseball Data. IEEE Comput. Graph Appl. 2016 , 36 , 28–37. [ Google Scholar ] [ CrossRef ] [ PubMed ]
- Vinué, G. A Web Application for Interactive Visualization of European Basketball Data. Big Data 2020 , 8 , 70–86. [ Google Scholar ] [ CrossRef ] [ PubMed ]
- Wu, Y.; Lan, J.; Shu, X.; Ji, C.; Zhao, K.; Wang, J.; Zhang, H. ITTVis: Interactive Visualization of Table Tennis Data. IEEE Trans. Vis. Comput. Graph. 2018 , 24 , 709–718. [ Google Scholar ] [ CrossRef ] [ PubMed ]
- Du, M.; Yuan, X. A Survey of Competitive Sports Data Visualization and Visual Analysis. J. Vis. 2021 , 24 , 47–67. [ Google Scholar ] [ CrossRef ]
- Basole, R.C.; Saupe, D. Sports Data Visualization [Guest Editors’ Introduction]. IEEE Comput. Graph Appl. 2016 , 36 , 24–26. [ Google Scholar ] [ CrossRef ]
- Thornton, H.R.; Delaney, J.A.; Duthie, G.M.; Dascombe, B.J. Developing Athlete Monitoring Systems in Team Sports: Data Analysis and Visualization. Int. J. Sports Physiol. Perform. 2019 , 14 , 698–705. [ Google Scholar ] [ CrossRef ]
- Pokharel, S.; Zhu, Y. Analysis and Visualization of Sports Performance Anxiety in Tennis Matches. In Proceedings of the 13th International Symposium—ISVC 2018, Las Vegas, NV, USA, 19–21 November 2018; Volume 11241 LNCS, pp. 407–419. [ Google Scholar ] [ CrossRef ]
- Pingali, G.; Opalach, A.; Jean, Y.; Carlbom, I. Visualization of Sports Using Motion Trajectories: Providing Insights into Performance, Style, and Strategy. In Proceedings of the Visualization, 2001. VIS ’01, San Diego, CA, USA, 21–26 October 2001; pp. 75–82. [ Google Scholar ] [ CrossRef ]
- Tani, T.; Huang, H.-H.; Kawagoe, K. Sports Play Visualization System Using Trajectory Mining Method. Procedia Technol. 2014 , 18 , 100–103. [ Google Scholar ] [ CrossRef ]
- Zhi, Q.; Lin, S.; Sukumar, P.T.; Metoyer, R. GameViews: Understanding and Supporting Data-Driven Sports Storytelling. In Proceedings of the CHI Conference on Human Factors in Computing Systems, Glasgow, UK, 4–9 May 2019; pp. 4–9. [ Google Scholar ]
- Chen, Z.; Yang, Q.; Xie, X.; Beyer, J.; Xia, H.; Wu, Y.; Pfister, H. Sporthesia: Augmenting Sports Videos Using Natural Language. IEEE Trans. Vis. Comput. Graph. 2023 , 29 , 918–928. [ Google Scholar ] [ CrossRef ]
- Liu, A.; Mahapatra, R.P.; Mayuri, A.V.R. Hybrid Design for Sports Data Visualization Using AI and Big Data Analytics. Complex Intell. Syst. 2023 , 9 , 2969–2980. [ Google Scholar ] [ CrossRef ]
- Orlando, A. AI for Sport in the EU Legal Framework. In Proceedings of the IEEE International Workshop on Sport, Technology and Research (STAR), Trento, Italy, 6–8 July 2022; pp. 100–105. [ Google Scholar ] [ CrossRef ]
- Casonato, C.; Marchetti, B. Prime Osservazioni Sulla Proposta Di Regolamento Dell ‘Unione Europea in Materia Di Intelligenza Artificiale. BioLaw J. 2021 , 3 , 415–437. [ Google Scholar ]
Click here to enlarge figure
Method | Pros | Cons |
---|---|---|
Observational Analysis | ||
Video Analysis | ||
Notational Analysis | ||
Time-Motion Analysis | ||
Data Analysis from Wearables |
Augmented Reality (AR) | Virtual Reality (VR) | ||
---|---|---|---|
Google Glass (2013) | Google Cardboard (2014) | ||
Microsoft HoloLens (2016) | Zeiss VR One (2014) | ||
Magic Leap One (2018) | Samsung Gear VR (2015) | ||
Microsoft HoloLens 2 (2019) | Oculus Rift (2016) | ||
Apple Vison PRO (2023) | HTC Vive (2016) | ||
PlayStation VR (2016) | |||
Oculus Quest (2019) | |||
Valve Index (2019) | |||
Meta Quest 2 (2020) | |||
Apple Vison PRO (2023) |
Type | Definition | Usage | Example |
---|---|---|---|
Bar Chart | A simple chart where rectangular bars represent data points, often used to compare different categories or values. | To compare the velocity of different players or competitors. | |
Line Chart | A chart that displays data points as markers connected by lines, useful for showing trends over time or continuous data. | To depict a tennis player’s accuracy over multiple matches. | |
Pie Chart | A circular chart divided into slices, each representing a portion of the whole, suitable for displaying proportions. | To demonstrate the percentage of player’s shot attempts during a basketball match from different positions on the court. | |
Scatter Plot | A graph with points that represent individual data points on two axes, helpful for showing the relationship between two variables. | To represent the relationship between a soccer player’s distance covered and their heart rate during a match. | |
Histogram | A chart that displays the distribution of a continuous dataset into bins or intervals, used to understand the data’s frequency distribution. | To illustrate the frequency of lap times in a swimmer’s training session. | |
Area Chart | Similar to a line chart but with the area beneath the line filled, suitable for showing cumulative data or stacked values. | To illustrate the internal workload of an athlete during period of training (e.g., a month) | |
Box Plot (Box-and-Whisker Plot) | A graphical representation of the data’s summary statistics, including the median, quartiles, and outliers, providing insights into the data’s spread. | To summarize the volleyball player’s serve accuracy. Boxplot makes it easier to find outliers. | |
Heatmap | A matrix-like representation of data using colors to indicate values, helpful for visualizing relationships in a table. | To highlight areas where a player spent the most time, indicating their preferred positions on the field. | |
Radar Chart (Spider Chart) | A chart with multiple axes radiating from a central point, useful for comparing multiple variables on different scales. | To compare the agility, speed, endurance, and lower limb strength of different track and field athletes. The chart can showcase each athlete’s strengths and weaknesses in these areas. | |
Bubble Chart | Like a scatter plot, but with additional size dimensions represented by the size of the data points. | Plot the age, height, and weight of basketball players in a bubble chart to visualize the distribution of these attributes within the team. The size of each bubble can represent a player’s minutes played in recent games. | |
Gantt Chart | A type of bar chart used in project management to show the timing and duration of tasks or events. | To outline the training schedule of a triathlete preparing for a competition. The chart can depict the phases of swimming, cycling, and running training leading up to the event. | |
Pareto Chart | A combination of a bar chart and a line chart, used to prioritize the most significant factors within a dataset. | Use a Pareto chart to prioritize the types of injuries occurring in a football team. The chart can help identify the most common injuries that need to be addressed to improve player safety. | |
Sankey Diagram | A visual representation of flow between different variables, often used for illustrating energy or material flows. | Illustrate the flow of ball possession between players in a basketball game using a Sankey diagram. The diagram can show the paths through which the ball moves during different plays. | |
Tree Map | A hierarchical chart that represents data as nested rectangles, useful for displaying hierarchical data structures. | To represent the proportion of players in each position category, such as forwards, backs, and scrum-halves. | |
Word Cloud | A visual representation of text data, where words are sized according to their frequency in the dataset. | The cloud can highlight the most frequently mentioned terms, reflecting players’ sentiments and thoughts about the match. |
The statements, opinions and data contained in all publications are solely those of the individual author(s) and contributor(s) and not of MDPI and/or the editor(s). MDPI and/or the editor(s) disclaim responsibility for any injury to people or property resulting from any ideas, methods, instructions or products referred to in the content. |
Share and Cite
Cossich, V.R.A.; Carlgren, D.; Holash, R.J.; Katz, L. Technological Breakthroughs in Sport: Current Practice and Future Potential of Artificial Intelligence, Virtual Reality, Augmented Reality, and Modern Data Visualization in Performance Analysis. Appl. Sci. 2023 , 13 , 12965. https://doi.org/10.3390/app132312965
Cossich VRA, Carlgren D, Holash RJ, Katz L. Technological Breakthroughs in Sport: Current Practice and Future Potential of Artificial Intelligence, Virtual Reality, Augmented Reality, and Modern Data Visualization in Performance Analysis. Applied Sciences . 2023; 13(23):12965. https://doi.org/10.3390/app132312965
Cossich, Victor R. A., Dave Carlgren, Robert John Holash, and Larry Katz. 2023. "Technological Breakthroughs in Sport: Current Practice and Future Potential of Artificial Intelligence, Virtual Reality, Augmented Reality, and Modern Data Visualization in Performance Analysis" Applied Sciences 13, no. 23: 12965. https://doi.org/10.3390/app132312965
Article Metrics
Article access statistics, further information, mdpi initiatives, follow mdpi.
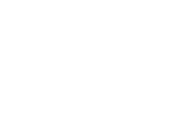
Subscribe to receive issue release notifications and newsletters from MDPI journals

IMAGES
VIDEO
COMMENTS
Hence there are enormous challenges and criticism about the historical neglect of environmental impact by the sports industry, particularly about over-consumption (Gammelsæter and Loland, 2023) driven by the expansion of international sports (McCullough et al., 2020), sports tourism, elite sports' commercial connections (Fourie and Santana ...
In the realm of power systems, the Internet of Things (IoT) emerges as a transformative force, steering a shift towards sustainable and distributed energy solutions for global economic growth. This comprehensive investigation navigates through various applications of IoT, unfolding its benefits and multifaceted impacts on society, the environment, and the economy. Real-world applications ...
A multitude of IoT-enabled devices are continually being explored and introduced annually, fostering robust competition among researchers and businesses seeking to leverage the IoT landscape, given the substantial market potential of these devices. The selection of IoT architectures, communication protocols, and components is contingent upon the task's nature and the data sensitivity the ...
¯ Rural residents reported an average of 3.8 days/week that their life activities were restricted by their physical health compared to 2.5 days in non-rural areas, and for mental health an ...
Another research indicated that IoT has led to the emergence of the Internet of Nano Things (IoNT) paradigm. Subsequent research endeavors have been focused on defining network topologies at the nanoscale that are safe, scalable, and dependable. ... This paper used a confusion matrix to compute AUC-ROC curve values, precision, accuracy, and ...
The main study of this research is the Internet of Things (IoT) and the vital application in the Hospitality Industry. The methodology that has been used in this paper is based on literature review, which tries to find the gap in the international literature and understand the concepts which are under examination.
The Internet of Things (IoT) is a powerful technology adopted in various industries. Applications of the IoT aim to enhance automation, productivity, and user comfort in a cloud and distributive computing environment. Cloud computing automatically stores and analyzes the large amounts of data generated by IoT-based applications. Cloud computing has become a crucial component of the information ...
A UNIT OF I2OR 2583 | P a g e. IoT for Augm enting Performance of Profes sional Sports. Training. Ankur Gupta 1, Gaurav Gupta 2, Latika Kharb 3. 12 Student Scholar (MCA), 3 Professor, 123 Jagan ...
The Internet of Things (IoT)-centric concepts like augmented reality, high-resolution video streaming, self-driven cars, smart environment, e-health care, etc. have a ubiquitous presence now. These applications require higher data-rates, large bandwidth, increased capacity, low latency and high throughput. In light of these emerging concepts, IoT has revolutionized the world by providing ...
Several research challenges towards sports centric Internet of Things have been presented wh ich need to be tackled in future. Download Free PDF View PDF. ... To fill this gap, this paper propose an IoT-aware Sport System based on the jointly use of different innovative technologies and standards. By exploiting the potentialities offered by an ...
This paper proposes a sport-service-oriented architecture framework and describes an example of design and implementation of an IoT application in sports based on sport-skills researches. Especially, the focus is on wearable applications with inertial sensors because sports applications require simple and durable motion-sensing systems. Skateboarding is introduced as a target sport to ...
This research investigates sports and fitness IoT systems and data analytics. Predictive models and machine learning algorithms can analyze enormous volumes of data, find patterns, and provide personalized advice to enhance performance and training. It also explores how VR and AR might be utilized with IoT to create realistic, interactive ...
NPTEL Assignment Answers and Solutions 2024 (July-Dec). Get Answers of Week 1 2 3 4 5 6 7 8 8 10 11 12 for all courses. This guide offers clear and accurate answers ...
This paper contributes to the existing body of research by exploring the synergistic potential of AI and IoT in sports. By combining these technologies, it proposes a proactive approach to managing risks and enhancing performance, emphasizing the importance of athlete safety without compromising on achieving peak results.
transformative role of IoT in sports nutrition, offering a comprehensive review of the subject. 1.1 Introduction to IoT and its relevance in sports nutrition IoT, as defined by Atzori, Iera, and Morabito (2010), encompasses a network of interconnected devices and sensors capable of collecting, transmitting, and analyzing data. Its
2.2 IoT privacy protection technology based on blockchain. In recent years, the Internet of Thing (IoT) technology and its innovative applications have rapidly developed in fields such as smart manufacturing, smart homes, and smart cities, which have changed the original production model and brought unprecedented convenience [].However, the ubiquitous object-to-thing interconnection and human ...
In this paper, we comprehensively survey the most recent and important research works conducted in the area of wearable Internet of Things (IoT) and classify the wearables into four major clusters ...
Abstract: This research paper embarks on an in-depth exploration of the critical role played by the Internet of Things (IoT) in advancing waste management systems in the context of smart city development. Drawing from a comprehensive review of twenty scholarly articles, our study begins by highlighting the fundamental significance of IoT as the driving force behind the evolution of smart cities.
Research in this field shows that with AIoT, IoT devices are enabled to learn from data and make swift decisions when an abnormal behavior is detected in the network in real-time. 95 Chakrabarty and Engels 68 proposed a framework for the IoT enabled smart city using AI to mitigate a range of present and future cyber threats. The massive ...
IoT is reshaping the sports industry by providing actionable data, enhancing safety, and delivering immersive fan experiences. Embracing IoT technology will let you stay competitive, efficient, and forward-thinking in an increasingly digital world. The IoT landscape in sports is ever evolving.
Overall 38 papers were initially selected and invited for potential inclusion in the VSI SpliTech 2019. After conducted peer-review process, based on the JCLP procedures, 29 of them were selected for inclusion in the VSI SpliTech 2019. ... Based on the recent analysed research works on the use of IoT technologies in the e-health and for the ...
The fundamentals of DRL are deeply understood by investigating the current state of IoT fitness applications and the most popular sports game design architectures. The research object is the ball return decision problem of the popular game of table tennis robot return.
Internet of Things (IoT) technology has attracted much attention in recent years for its potential to alleviate the strain on healthcare systems caused by an aging population and a rise in chronic illness. Standardization is a key issue limiting progress in this area, and thus this paper proposes a standard model for application in future IoT healthcare systems. This survey paper then presents ...
We are currently witnessing an unprecedented era of digital transformation in sports, driven by the revolutions in Artificial Intelligence (AI), Virtual Reality (VR), Augmented Reality (AR), and Data Visualization (DV). These technologies hold the promise of redefining sports performance analysis, automating data collection, creating immersive training environments, and enhancing decision ...
IoT can transform the sports industry as it enables breakthrough results by bridging the physical and digital world in innovative ways and drives value by integrating data sources and connecting with fans through many technologies. Integrating the data gathered by different IoT sources and improving the connectivity of systems can increase ...
This paper aims to present the role of IoT in cr ating smart envi onment n different areas. In particular, th concept of smart school is considered in detail. The research shows the application of IoT devices for secure and energy-sav classroo settings, thus maintaining appropriate learni environ ent for a h eving an effic ent educati nal process.