Root out friction in every digital experience, super-charge conversion rates, and optimize digital self-service
Uncover insights from any interaction, deliver AI-powered agent coaching, and reduce cost to serve
Increase revenue and loyalty with real-time insights and recommendations delivered to teams on the ground
Know how your people feel and empower managers to improve employee engagement, productivity, and retention
Take action in the moments that matter most along the employee journey and drive bottom line growth
Whatever they’re are saying, wherever they’re saying it, know exactly what’s going on with your people
Get faster, richer insights with qual and quant tools that make powerful market research available to everyone
Run concept tests, pricing studies, prototyping + more with fast, powerful studies designed by UX research experts
Track your brand performance 24/7 and act quickly to respond to opportunities and challenges in your market
Explore the platform powering Experience Management
- Free Account
- Product Demos
- For Digital
- For Customer Care
- For Human Resources
- For Researchers
- Financial Services
- All Industries
Popular Use Cases
- Customer Experience
- Employee Experience
- Net Promoter Score
- Voice of Customer
- Customer Success Hub
- Product Documentation
- Training & Certification
- XM Institute
- Popular Resources
- Customer Stories
- Artificial Intelligence
Market Research
- Partnerships
- Marketplace
The annual gathering of the experience leaders at the world’s iconic brands building breakthrough business results, live in Salt Lake City.
- English/AU & NZ
- Español/Europa
- Español/América Latina
- Português Brasileiro
- REQUEST DEMO
- Experience Management
- Causal Research

Try Qualtrics for free
Causal research: definition, examples and how to use it.
16 min read Causal research enables market researchers to predict hypothetical occurrences & outcomes while improving existing strategies. Discover how this research can decrease employee retention & increase customer success for your business.
What is causal research?
Causal research, also known as explanatory research or causal-comparative research, identifies the extent and nature of cause-and-effect relationships between two or more variables.
It’s often used by companies to determine the impact of changes in products, features, or services process on critical company metrics. Some examples:
- How does rebranding of a product influence intent to purchase?
- How would expansion to a new market segment affect projected sales?
- What would be the impact of a price increase or decrease on customer loyalty?
To maintain the accuracy of causal research, ‘confounding variables’ or influences — e.g. those that could distort the results — are controlled. This is done either by keeping them constant in the creation of data, or by using statistical methods. These variables are identified before the start of the research experiment.
As well as the above, research teams will outline several other variables and principles in causal research:
- Independent variables
The variables that may cause direct changes in another variable. For example, the effect of truancy on a student’s grade point average. The independent variable is therefore class attendance.
- Control variables
These are the components that remain unchanged during the experiment so researchers can better understand what conditions create a cause-and-effect relationship.
This describes the cause-and-effect relationship. When researchers find causation (or the cause), they’ve conducted all the processes necessary to prove it exists.
- Correlation
Any relationship between two variables in the experiment. It’s important to note that correlation doesn’t automatically mean causation. Researchers will typically establish correlation before proving cause-and-effect.
- Experimental design
Researchers use experimental design to define the parameters of the experiment — e.g. categorizing participants into different groups.
- Dependent variables
These are measurable variables that may change or are influenced by the independent variable. For example, in an experiment about whether or not terrain influences running speed, your dependent variable is the terrain.
Why is causal research useful?
It’s useful because it enables market researchers to predict hypothetical occurrences and outcomes while improving existing strategies. This allows businesses to create plans that benefit the company. It’s also a great research method because researchers can immediately see how variables affect each other and under what circumstances.
Also, once the first experiment has been completed, researchers can use the learnings from the analysis to repeat the experiment or apply the findings to other scenarios. Because of this, it’s widely used to help understand the impact of changes in internal or commercial strategy to the business bottom line.
Some examples include:
- Understanding how overall training levels are improved by introducing new courses
- Examining which variations in wording make potential customers more interested in buying a product
- Testing a market’s response to a brand-new line of products and/or services
So, how does causal research compare and differ from other research types?
Well, there are a few research types that are used to find answers to some of the examples above:
1. Exploratory research
As its name suggests, exploratory research involves assessing a situation (or situations) where the problem isn’t clear. Through this approach, researchers can test different avenues and ideas to establish facts and gain a better understanding.
Researchers can also use it to first navigate a topic and identify which variables are important. Because no area is off-limits, the research is flexible and adapts to the investigations as it progresses.
Finally, this approach is unstructured and often involves gathering qualitative data, giving the researcher freedom to progress the research according to their thoughts and assessment. However, this may make results susceptible to researcher bias and may limit the extent to which a topic is explored.
2. Descriptive research
Descriptive research is all about describing the characteristics of the population, phenomenon or scenario studied. It focuses more on the “what” of the research subject than the “why”.
For example, a clothing brand wants to understand the fashion purchasing trends amongst buyers in California — so they conduct a demographic survey of the region, gather population data and then run descriptive research. The study will help them to uncover purchasing patterns amongst fashion buyers in California, but not necessarily why those patterns exist.
As the research happens in a natural setting, variables can cross-contaminate other variables, making it harder to isolate cause and effect relationships. Therefore, further research will be required if more causal information is needed.
Get started on your market research journey with CoreXM
How is causal research different from the other two methods above?
Well, causal research looks at what variables are involved in a problem and ‘why’ they act a certain way. As the experiment takes place in a controlled setting (thanks to controlled variables) it’s easier to identify cause-and-effect amongst variables.
Furthermore, researchers can carry out causal research at any stage in the process, though it’s usually carried out in the later stages once more is known about a particular topic or situation.
Finally, compared to the other two methods, causal research is more structured, and researchers can combine it with exploratory and descriptive research to assist with research goals.
Summary of three research types
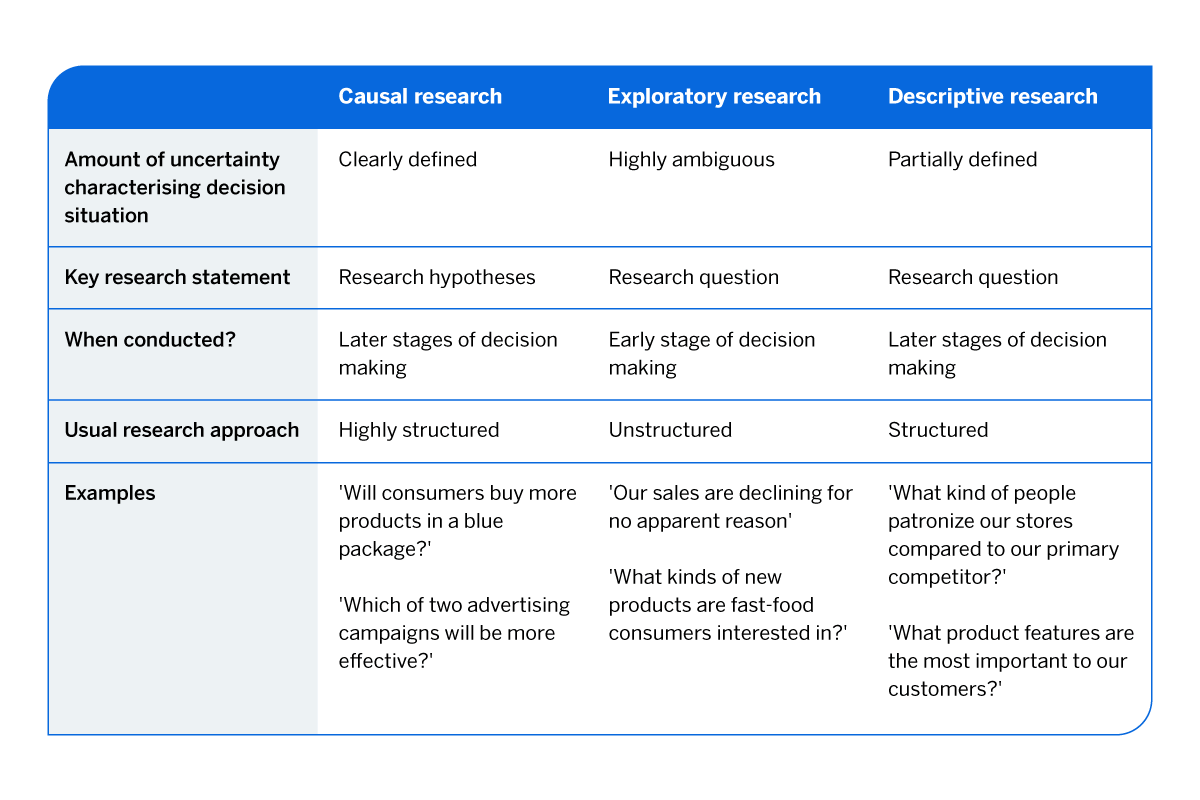
What are the advantages of causal research?
- Improve experiences
By understanding which variables have positive impacts on target variables (like sales revenue or customer loyalty), businesses can improve their processes, return on investment, and the experiences they offer customers and employees.
- Help companies improve internally
By conducting causal research, management can make informed decisions about improving their employee experience and internal operations. For example, understanding which variables led to an increase in staff turnover.
- Repeat experiments to enhance reliability and accuracy of results
When variables are identified, researchers can replicate cause-and-effect with ease, providing them with reliable data and results to draw insights from.
- Test out new theories or ideas
If causal research is able to pinpoint the exact outcome of mixing together different variables, research teams have the ability to test out ideas in the same way to create viable proof of concepts.
- Fix issues quickly
Once an undesirable effect’s cause is identified, researchers and management can take action to reduce the impact of it or remove it entirely, resulting in better outcomes.
What are the disadvantages of causal research?
- Provides information to competitors
If you plan to publish your research, it provides information about your plans to your competitors. For example, they might use your research outcomes to identify what you are up to and enter the market before you.
- Difficult to administer
Causal research is often difficult to administer because it’s not possible to control the effects of extraneous variables.
- Time and money constraints
Budgetary and time constraints can make this type of research expensive to conduct and repeat. Also, if an initial attempt doesn’t provide a cause and effect relationship, the ROI is wasted and could impact the appetite for future repeat experiments.
- Requires additional research to ensure validity
You can’t rely on just the outcomes of causal research as it’s inaccurate. It’s best to conduct other types of research alongside it to confirm its output.
- Trouble establishing cause and effect
Researchers might identify that two variables are connected, but struggle to determine which is the cause and which variable is the effect.
- Risk of contamination
There’s always the risk that people outside your market or area of study could affect the results of your research. For example, if you’re conducting a retail store study, shoppers outside your ‘test parameters’ shop at your store and skew the results.
How can you use causal research effectively?
To better highlight how you can use causal research across functions or markets, here are a few examples:
Market and advertising research
A company might want to know if their new advertising campaign or marketing campaign is having a positive impact. So, their research team can carry out a causal research project to see which variables cause a positive or negative effect on the campaign.
For example, a cold-weather apparel company in a winter ski-resort town may see an increase in sales generated after a targeted campaign to skiers. To see if one caused the other, the research team could set up a duplicate experiment to see if the same campaign would generate sales from non-skiers. If the results reduce or change, then it’s likely that the campaign had a direct effect on skiers to encourage them to purchase products.
Improving customer experiences and loyalty levels
Customers enjoy shopping with brands that align with their own values, and they’re more likely to buy and present the brand positively to other potential shoppers as a result. So, it’s in your best interest to deliver great experiences and retain your customers.
For example, the Harvard Business Review found that an increase in customer retention rates by 5% increased profits by 25% to 95%. But let’s say you want to increase your own, how can you identify which variables contribute to it?Using causal research, you can test hypotheses about which processes, strategies or changes influence customer retention. For example, is it the streamlined checkout? What about the personalized product suggestions? Or maybe it was a new solution that solved their problem? Causal research will help you find out.
Discover how to use analytics to improve customer retention.
Improving problematic employee turnover rates
If your company has a high attrition rate, causal research can help you narrow down the variables or reasons which have the greatest impact on people leaving. This allows you to prioritize your efforts on tackling the issues in the right order, for the best positive outcomes.
For example, through causal research, you might find that employee dissatisfaction due to a lack of communication and transparency from upper management leads to poor morale, which in turn influences employee retention.
To rectify the problem, you could implement a routine feedback loop or session that enables your people to talk to your company’s C-level executives so that they feel heard and understood.
How to conduct causal research first steps to getting started are:
1. Define the purpose of your research
What questions do you have? What do you expect to come out of your research? Think about which variables you need to test out the theory.
2. Pick a random sampling if participants are needed
Using a technology solution to support your sampling, like a database, can help you define who you want your target audience to be, and how random or representative they should be.
3. Set up the controlled experiment
Once you’ve defined which variables you’d like to measure to see if they interact, think about how best to set up the experiment. This could be in-person or in-house via interviews, or it could be done remotely using online surveys.
4. Carry out the experiment
Make sure to keep all irrelevant variables the same, and only change the causal variable (the one that causes the effect) to gather the correct data. Depending on your method, you could be collecting qualitative or quantitative data, so make sure you note your findings across each regularly.
5. Analyze your findings
Either manually or using technology, analyze your data to see if any trends, patterns or correlations emerge. By looking at the data, you’ll be able to see what changes you might need to do next time, or if there are questions that require further research.
6. Verify your findings
Your first attempt gives you the baseline figures to compare the new results to. You can then run another experiment to verify your findings.
7. Do follow-up or supplemental research
You can supplement your original findings by carrying out research that goes deeper into causes or explores the topic in more detail. One of the best ways to do this is to use a survey. See ‘Use surveys to help your experiment’.
Identifying causal relationships between variables
To verify if a causal relationship exists, you have to satisfy the following criteria:
- Nonspurious association
A clear correlation exists between one cause and the effect. In other words, no ‘third’ that relates to both (cause and effect) should exist.
- Temporal sequence
The cause occurs before the effect. For example, increased ad spend on product marketing would contribute to higher product sales.
- Concomitant variation
The variation between the two variables is systematic. For example, if a company doesn’t change its IT policies and technology stack, then changes in employee productivity were not caused by IT policies or technology.
How surveys help your causal research experiments?
There are some surveys that are perfect for assisting researchers with understanding cause and effect. These include:
- Employee Satisfaction Survey – An introductory employee satisfaction survey that provides you with an overview of your current employee experience.
- Manager Feedback Survey – An introductory manager feedback survey geared toward improving your skills as a leader with valuable feedback from your team.
- Net Promoter Score (NPS) Survey – Measure customer loyalty and understand how your customers feel about your product or service using one of the world’s best-recognized metrics.
- Employee Engagement Survey – An entry-level employee engagement survey that provides you with an overview of your current employee experience.
- Customer Satisfaction Survey – Evaluate how satisfied your customers are with your company, including the products and services you provide and how they are treated when they buy from you.
- Employee Exit Interview Survey – Understand why your employees are leaving and how they’ll speak about your company once they’re gone.
- Product Research Survey – Evaluate your consumers’ reaction to a new product or product feature across every stage of the product development journey.
- Brand Awareness Survey – Track the level of brand awareness in your target market, including current and potential future customers.
- Online Purchase Feedback Survey – Find out how well your online shopping experience performs against customer needs and expectations.
That covers the fundamentals of causal research and should give you a foundation for ongoing studies to assess opportunities, problems, and risks across your market, product, customer, and employee segments.
If you want to transform your research, empower your teams and get insights on tap to get ahead of the competition, maybe it’s time to leverage Qualtrics CoreXM.
Qualtrics CoreXM provides a single platform for data collection and analysis across every part of your business — from customer feedback to product concept testing. What’s more, you can integrate it with your existing tools and services thanks to a flexible API.
Qualtrics CoreXM offers you as much or as little power and complexity as you need, so whether you’re running simple surveys or more advanced forms of research, it can deliver every time.
Related resources
Market intelligence 10 min read, marketing insights 11 min read, ethnographic research 11 min read, qualitative vs quantitative research 13 min read, qualitative research questions 11 min read, qualitative research design 12 min read, primary vs secondary research 14 min read, request demo.
Ready to learn more about Qualtrics?
Causal Research: The Complete Guide

Published: February 22, 2023
As we grow up, all humans learn about cause and effect. While it’s not quite as nuanced as causal research, the concept is something our brains begin to comprehend as young as 18 months old. That understanding continues to develop throughout our lives.
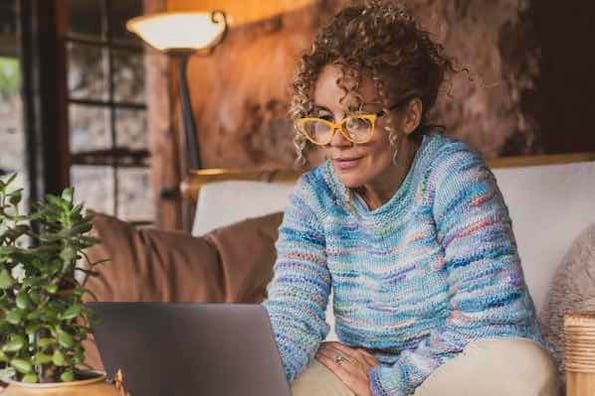
In the marketing world, data collection and market research are invaluable. That’s where causal research, the study of cause and effect, comes in.
First-party data can help you learn more about the impact of your marketing campaigns, improve business metrics like customer loyalty, and conduct research on employee productivity. In this guide, we’ll review what causal research is, how it can improve your marketing efforts, and how to conduct your research.
Table of Contents
What is causal research?
The benefits of causal research, causal research examples, how to conduct causal research.
Causal research is a type of study that evaluates whether two variables (one independent, one dependent) have a cause-and-effect relationship. Experiments are designed to collect statistical evidence that infers there is cause and effect between two situations. Marketers can use causal research to see the effect of product changes, rebranding efforts, and more.
Once your team has conducted causal research, your marketers will develop theories on why the relationship developed. Here, your team can study how the variables interact and determine what strategies to apply to future business needs.
Companies can learn how rebranding a product influences sales, how expansion into new markets will affect revenue, and the impact of pricing changes on customer loyalty. Keep in mind that causality is only probable, rather than proven.
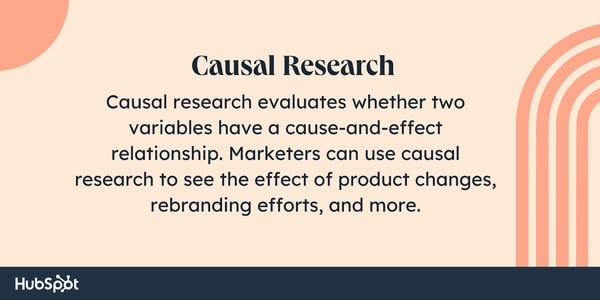
Don't forget to share this post!
Related articles.
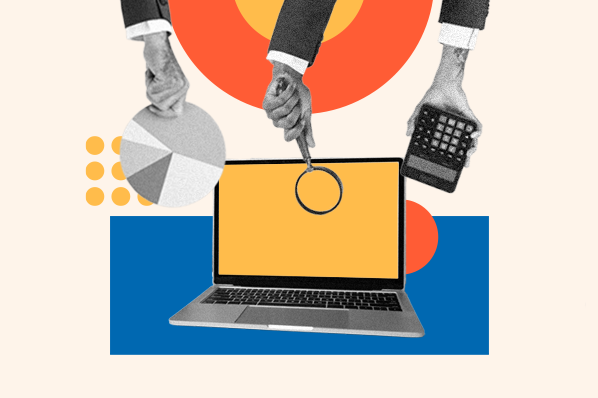
26 Tools & Resources for Conducting Market Research
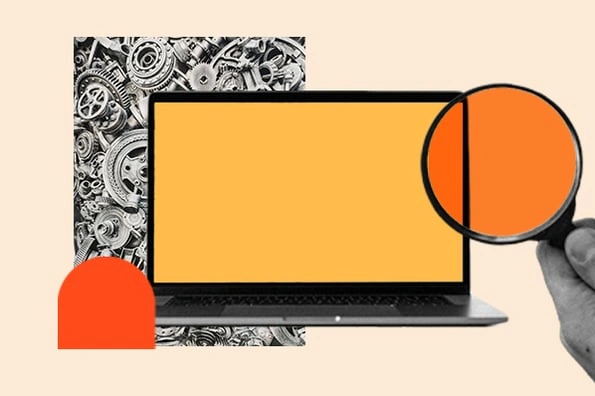
What is a Competitive Analysis — and How Do You Conduct One?
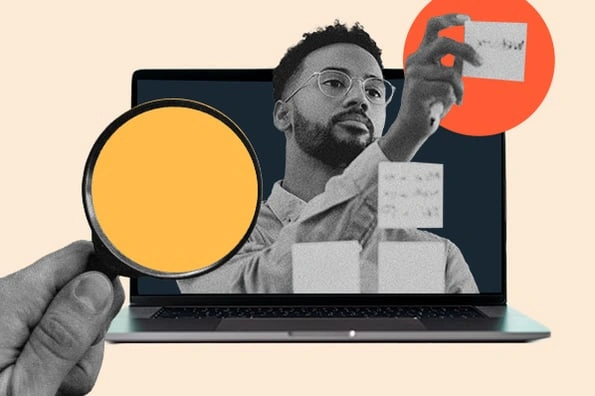
Market Research: A How-To Guide and Template
![causal research in marketing SWOT Analysis: How To Do One [With Template & Examples]](https://blog.hubspot.com/hubfs/marketingplan_20.webp)
SWOT Analysis: How To Do One [With Template & Examples]
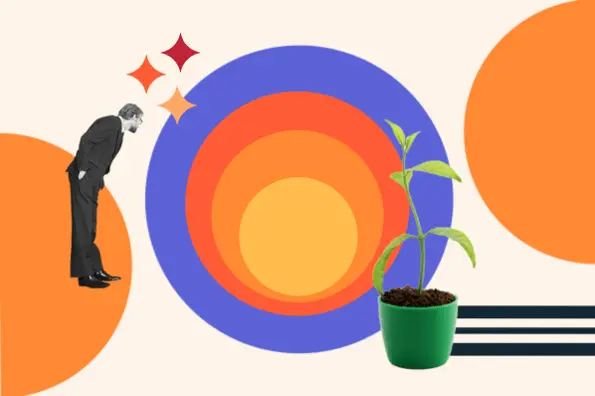
TAM SAM SOM: What Do They Mean & How Do You Calculate Them?
![causal research in marketing How to Run a Competitor Analysis [Free Guide]](https://blog.hubspot.com/hubfs/Google%20Drive%20Integration/how%20to%20do%20a%20competitor%20analysis_122022.jpeg)
How to Run a Competitor Analysis [Free Guide]
![causal research in marketing 5 Challenges Marketers Face in Understanding Audiences [New Data + Market Researcher Tips]](https://blog.hubspot.com/hubfs/challenges%20marketers%20face%20in%20understanding%20the%20customer%20.png)
5 Challenges Marketers Face in Understanding Audiences [New Data + Market Researcher Tips]
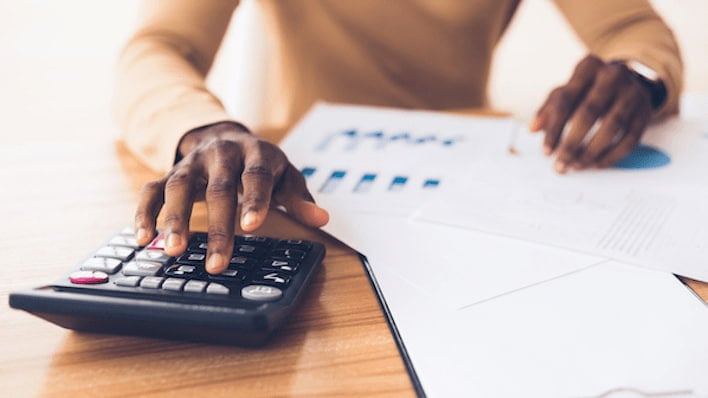
Total Addressable Market (TAM): What It Is & How You Can Calculate It
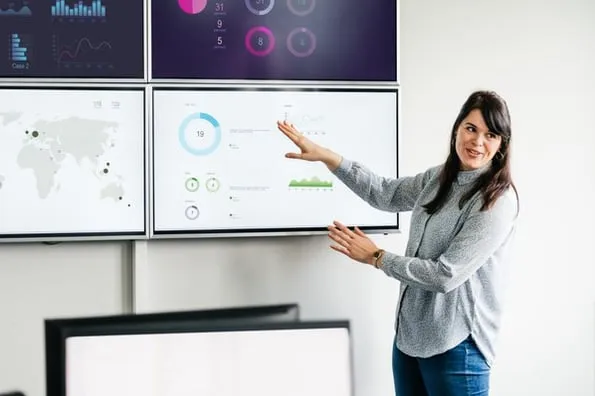
What Is Market Share & How Do You Calculate It?
![causal research in marketing 3 Ways Data Privacy Changes Benefit Marketers [New Data]](https://blog.hubspot.com/hubfs/how-data-privacy-benefits-marketers_1.webp)
3 Ways Data Privacy Changes Benefit Marketers [New Data]
Free Guide & Templates to Help Your Market Research
Marketing software that helps you drive revenue, save time and resources, and measure and optimize your investments — all on one easy-to-use platform
- Skip to main content
- Skip to primary sidebar
- Skip to footer
- QuestionPro

- Solutions Industries Gaming Automotive Sports and events Education Government Travel & Hospitality Financial Services Healthcare Cannabis Technology Use Case NPS+ Communities Audience Contactless surveys Mobile LivePolls Member Experience GDPR Positive People Science 360 Feedback Surveys
- Resources Blog eBooks Survey Templates Case Studies Training Help center

Home Market Research Research Tools and Apps
Causal Research: What it is, Tips & Examples
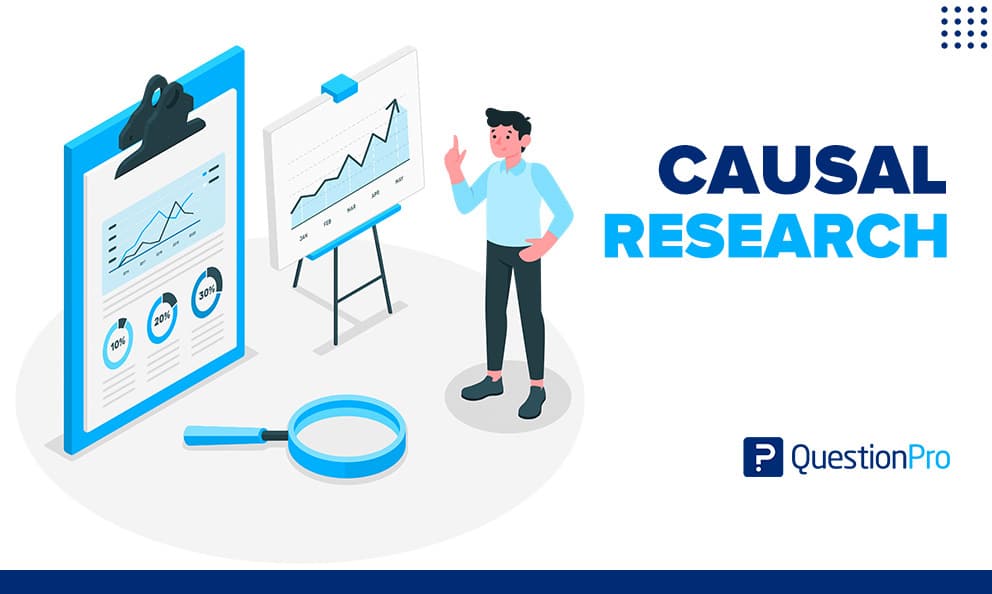
Causal research is classified as conclusive research since it attempts to build a cause-and-effect link between two variables. This research is mainly used to determine the cause of particular behavior. We can use this research to determine what changes occur in an independent variable due to a change in the dependent variable.
It can assist you in evaluating marketing activities, improving internal procedures, and developing more effective business plans. Understanding how one circumstance affects another may help you determine the most effective methods for satisfying your business needs.
LEARN ABOUT: Behavioral Research
This post will explain causal research, define its essential components, describe its benefits and limitations, and provide some important tips.
Content Index
What is causal research?
Temporal sequence, non-spurious association, concomitant variation, the advantages, the disadvantages, causal research examples, causal research tips.
Causal research is also known as explanatory research . It’s a type of research that examines if there’s a cause-and-effect relationship between two separate events. This would occur when there is a change in one of the independent variables, which is causing changes in the dependent variable.
You can use causal research to evaluate the effects of particular changes on existing norms, procedures, and so on. This type of research examines a condition or a research problem to explain the patterns of interactions between variables.
LEARN ABOUT: Research Process Steps
Components of causal research
Only specific causal information can demonstrate the existence of cause-and-effect linkages. The three key components of causal research are as follows:
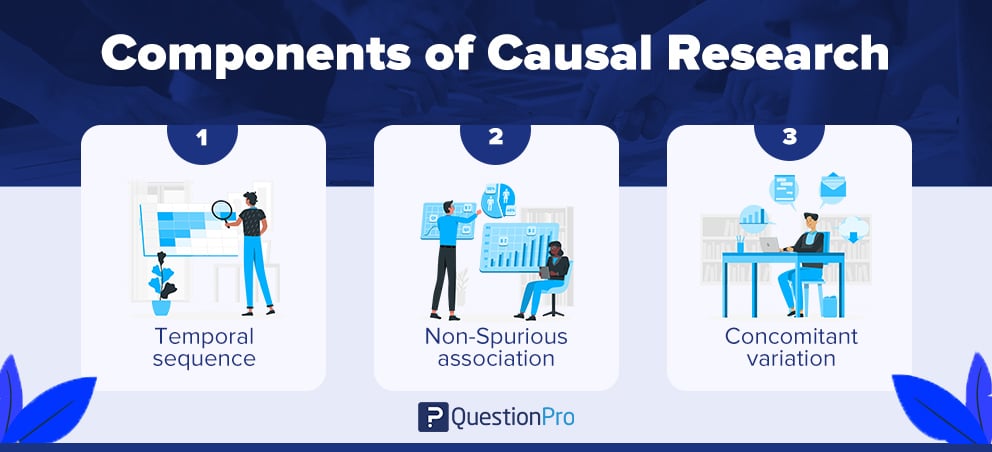
Prior to the effect, the cause must occur. If the cause occurs before the appearance of the effect, the cause and effect can only be linked. For example, if the profit increase occurred before the advertisement aired, it cannot be linked to an increase in advertising spending.
Linked fluctuations between two variables are only allowed if there is no other variable that is related to both cause and effect. For example, a notebook manufacturer has discovered a correlation between notebooks and the autumn season. They see that during this season, more people buy notebooks because students are buying them for the upcoming semester.
During the summer, the company launched an advertisement campaign for notebooks. To test their assumption, they can look up the campaign data to see if the increase in notebook sales was due to the student’s natural rhythm of buying notebooks or the advertisement.
Concomitant variation is defined as a quantitative change in effect that happens solely as a result of a quantitative change in the cause. This means that there must be a steady change between the two variables. You can examine the validity of a cause-and-effect connection by seeing if the independent variable causes a change in the dependent variable.
For example, if any company does not make an attempt to enhance sales by acquiring skilled employees or offering training to them, then the hire of experienced employees cannot be credited for an increase in sales. Other factors may have contributed to the increase in sales.
Causal Research Advantages and Disadvantages
Causal or explanatory research has various advantages for both academics and businesses. As with any other research method, it has a few disadvantages that researchers should be aware of. Let’s look at some of the advantages and disadvantages of this research design .
- Helps in the identification of the causes of system processes. This allows the researcher to take the required steps to resolve issues or improve outcomes.
- It provides replication if it is required.
- Causal research assists in determining the effects of changing procedures and methods.
- Subjects are chosen in a methodical manner. As a result, it is beneficial for improving internal validity .
- The ability to analyze the effects of changes on existing events, processes, phenomena, and so on.
- Finds the sources of variable correlations, bridging the gap in correlational research .
- It is not always possible to monitor the effects of all external factors, so causal research is challenging to do.
- It is time-consuming and might be costly to execute.
- The effect of a large range of factors and variables existing in a particular setting makes it difficult to draw results.
- The most major error in this research is a coincidence. A coincidence between a cause and an effect can sometimes be interpreted as a direction of causality.
- To corroborate the findings of the explanatory research , you must undertake additional types of research. You can’t just make conclusions based on the findings of a causal study.
- It is sometimes simple for a researcher to see that two variables are related, but it can be difficult for a researcher to determine which variable is the cause and which variable is the effect.
Since different industries and fields can carry out causal comparative research , it can serve many different purposes. Let’s discuss 3 examples of causal research:
Advertising Research
Companies can use causal research to enact and study advertising campaigns. For example, six months after a business debuts a new ad in a region. They see a 5% increase in sales revenue.
To assess whether the ad has caused the lift, they run the same ad in randomly selected regions so they can compare sales data across regions over another six months. When sales pick up again in these regions, they can conclude that the ad and sales have a valuable cause-and-effect relationship.
LEARN ABOUT: Ad Testing
Customer Loyalty Research
Businesses can use causal research to determine the best customer retention strategies. They monitor interactions between associates and customers to identify patterns of cause and effect, such as a product demonstration technique leading to increased or decreased sales from the same customers.
For example, a company implements a new individual marketing strategy for a small group of customers and sees a measurable increase in monthly subscriptions. After receiving identical results from several groups, they concluded that the one-to-one marketing strategy has the causal relationship they intended.
Educational Research
Learning specialists, academics, and teachers use causal research to learn more about how politics affects students and identify possible student behavior trends. For example, a university administration notices that more science students drop out of their program in their third year, which is 7% higher than in any other year.
They interview a random group of science students and discover many factors that could lead to these circumstances, including non-university components. Through the in-depth statistical analysis, researchers uncover the top three factors, and management creates a committee to address them in the future.
Causal research is frequently the last type of research done during the research process and is considered definitive. As a result, it is critical to plan the research with specific parameters and goals in mind. Here are some tips for conducting causal research successfully:
1. Understand the parameters of your research
Identify any design strategies that change the way you understand your data. Determine how you acquired data and whether your conclusions are more applicable in practice in some cases than others.
2. Pick a random sampling strategy
Choosing a technique that works best for you when you have participants or subjects is critical. You can use a database to generate a random list, select random selections from sorted categories, or conduct a survey.
3. Determine all possible relations
Examine the different relationships between your independent and dependent variables to build more sophisticated insights and conclusions.
To summarize, causal or explanatory research helps organizations understand how their current activities and behaviors will impact them in the future. This is incredibly useful in a wide range of business scenarios. This research can ensure the outcome of various marketing activities, campaigns, and collaterals. Using the findings of this research program, you will be able to design more successful business strategies that take advantage of every business opportunity.
At QuestionPro, we offer all kinds of necessary tools for researchers to carry out their projects. It can help you get the most out of your data by guiding you through the process.
MORE LIKE THIS
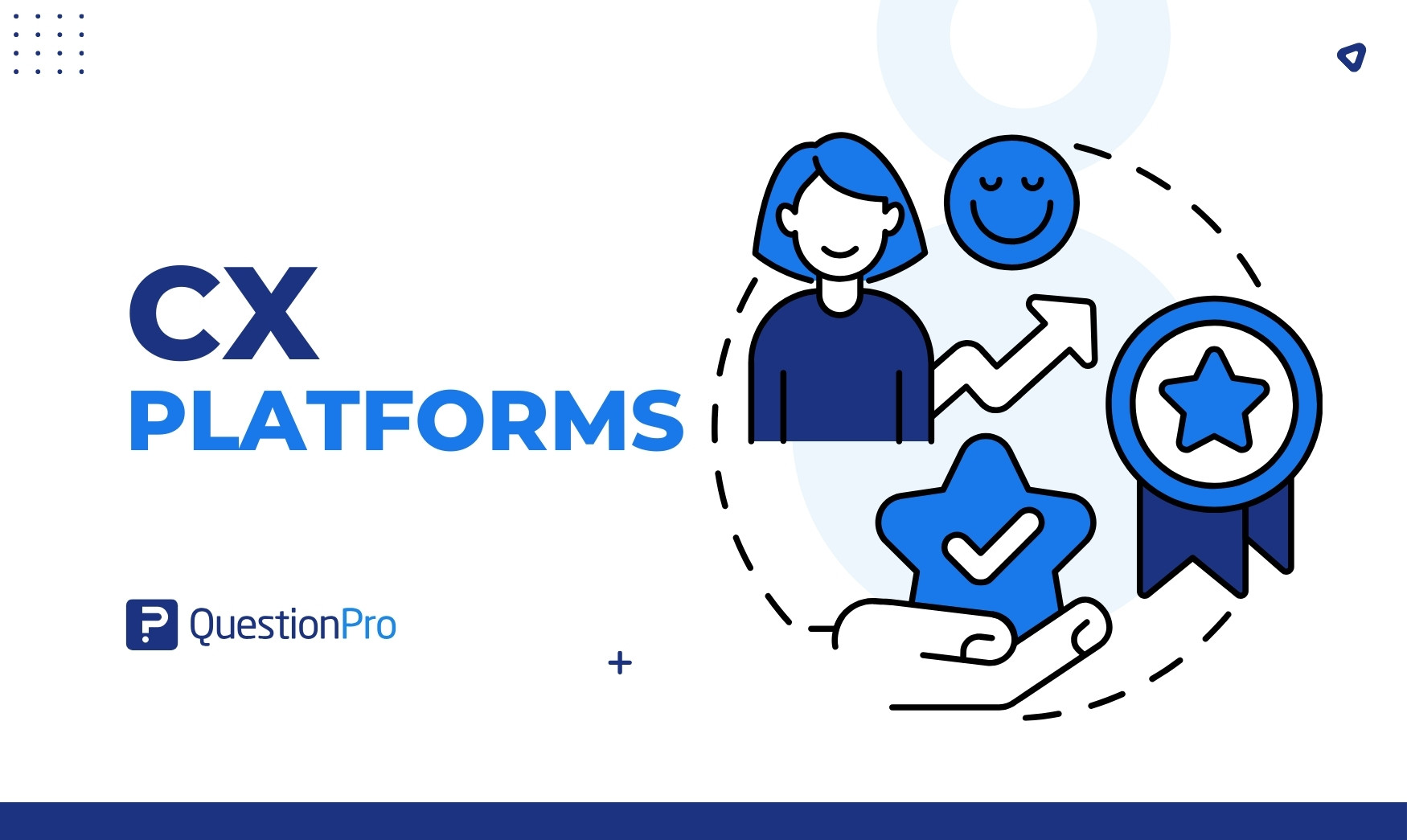
CX Platform: Top 13 CX Platforms to Drive Customer Success
Jun 17, 2024
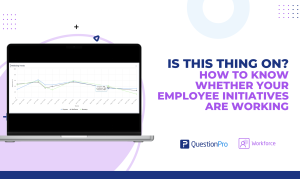
How to Know Whether Your Employee Initiatives are Working
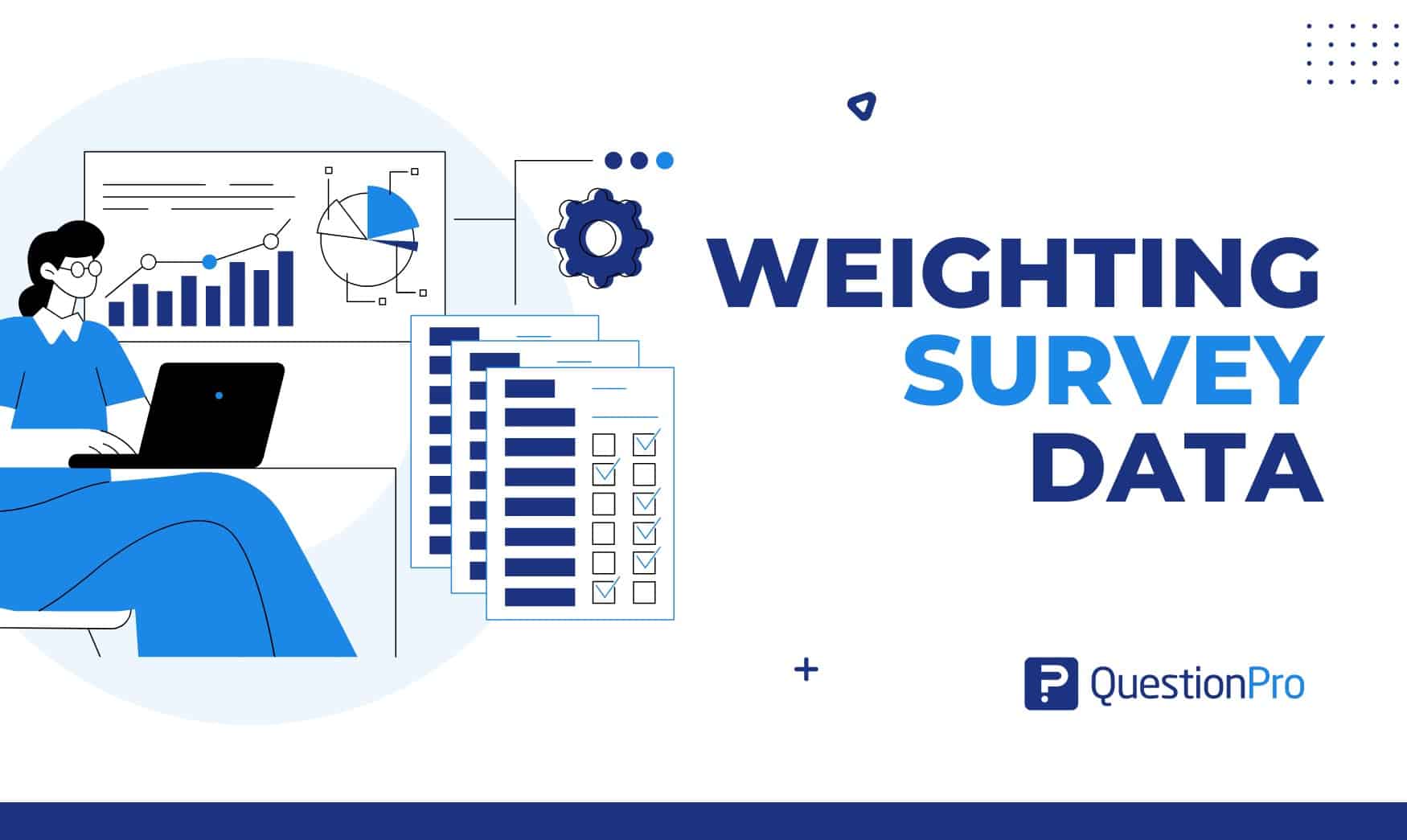
How to Weighting Survey Data to Enhance Your Data Quality?
Jun 12, 2024
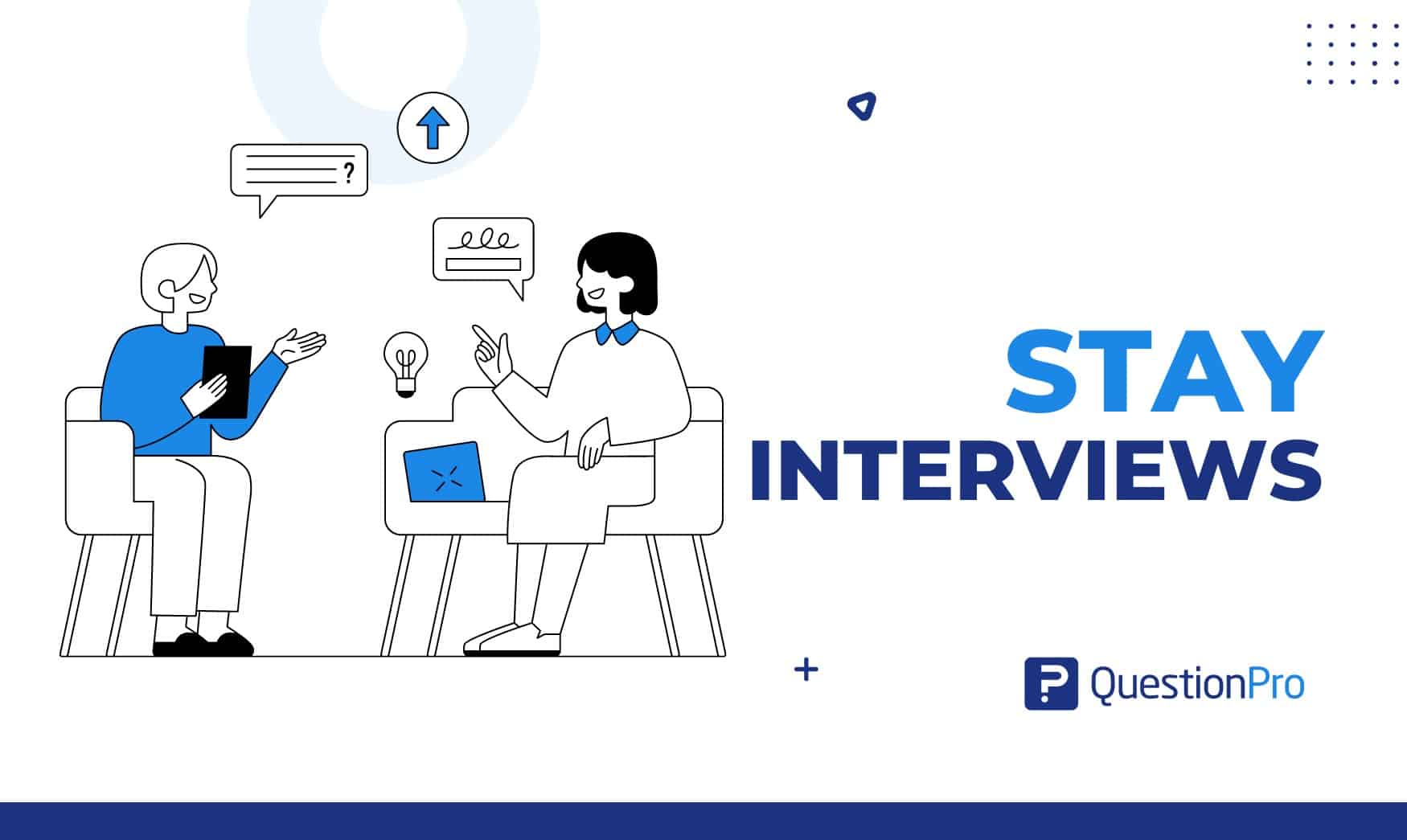
Stay Interviews: What Is It, How to Conduct, 15 Questions
Jun 11, 2024
Other categories
- Academic Research
- Artificial Intelligence
- Assessments
- Brand Awareness
- Case Studies
- Communities
- Consumer Insights
- Customer effort score
- Customer Engagement
- Customer Experience
- Customer Loyalty
- Customer Research
- Customer Satisfaction
- Employee Benefits
- Employee Engagement
- Employee Retention
- Friday Five
- General Data Protection Regulation
- Insights Hub
- Life@QuestionPro
- Market Research
- Mobile diaries
- Mobile Surveys
- New Features
- Online Communities
- Question Types
- Questionnaire
- QuestionPro Products
- Release Notes
- Research Tools and Apps
- Revenue at Risk
- Survey Templates
- Training Tips
- Tuesday CX Thoughts (TCXT)
- Uncategorized
- Video Learning Series
- What’s Coming Up
- Workforce Intelligence
Causal Research: Definition, Design, Tips, Examples
Appinio Research · 21.02.2024 · 34min read
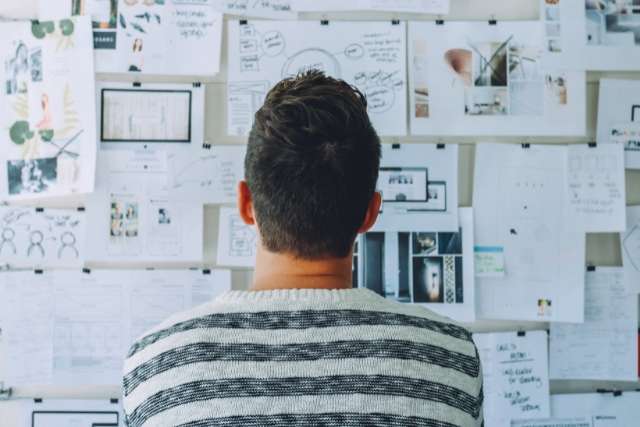
Ever wondered why certain events lead to specific outcomes? Understanding causality—the relationship between cause and effect—is crucial for unraveling the mysteries of the world around us. In this guide on causal research, we delve into the methods, techniques, and principles behind identifying and establishing cause-and-effect relationships between variables. Whether you're a seasoned researcher or new to the field, this guide will equip you with the knowledge and tools to conduct rigorous causal research and draw meaningful conclusions that can inform decision-making and drive positive change.
What is Causal Research?
Causal research is a methodological approach used in scientific inquiry to investigate cause-and-effect relationships between variables. Unlike correlational or descriptive research, which merely examine associations or describe phenomena, causal research aims to determine whether changes in one variable cause changes in another variable.
Importance of Causal Research
Understanding the importance of causal research is crucial for appreciating its role in advancing knowledge and informing decision-making across various fields. Here are key reasons why causal research is significant:
- Establishing Causality: Causal research enables researchers to determine whether changes in one variable directly cause changes in another variable. This helps identify effective interventions, predict outcomes, and inform evidence-based practices.
- Guiding Policy and Practice: By identifying causal relationships, causal research provides empirical evidence to support policy decisions, program interventions, and business strategies. Decision-makers can use causal findings to allocate resources effectively and address societal challenges.
- Informing Predictive Modeling : Causal research contributes to the development of predictive models by elucidating causal mechanisms underlying observed phenomena. Predictive models based on causal relationships can accurately forecast future outcomes and trends.
- Advancing Scientific Knowledge: Causal research contributes to the cumulative body of scientific knowledge by testing hypotheses, refining theories, and uncovering underlying mechanisms of phenomena. It fosters a deeper understanding of complex systems and phenomena.
- Mitigating Confounding Factors: Understanding causal relationships allows researchers to control for confounding variables and reduce bias in their studies. By isolating the effects of specific variables, researchers can draw more valid and reliable conclusions.
Causal Research Distinction from Other Research
Understanding the distinctions between causal research and other types of research methodologies is essential for researchers to choose the most appropriate approach for their study objectives. Let's explore the differences and similarities between causal research and descriptive, exploratory, and correlational research methodologies .
Descriptive vs. Causal Research
Descriptive research focuses on describing characteristics, behaviors, or phenomena without manipulating variables or establishing causal relationships. It provides a snapshot of the current state of affairs but does not attempt to explain why certain phenomena occur.
Causal research , on the other hand, seeks to identify cause-and-effect relationships between variables by systematically manipulating independent variables and observing their effects on dependent variables. Unlike descriptive research, causal research aims to determine whether changes in one variable directly cause changes in another variable.
Similarities:
- Both descriptive and causal research involve empirical observation and data collection.
- Both types of research contribute to the scientific understanding of phenomena, albeit through different approaches.
Differences:
- Descriptive research focuses on describing phenomena, while causal research aims to explain why phenomena occur by identifying causal relationships.
- Descriptive research typically uses observational methods, while causal research often involves experimental designs or causal inference techniques to establish causality.
Exploratory vs. Causal Research
Exploratory research aims to explore new topics, generate hypotheses, or gain initial insights into phenomena. It is often conducted when little is known about a subject and seeks to generate ideas for further investigation.
Causal research , on the other hand, is concerned with testing hypotheses and establishing cause-and-effect relationships between variables. It builds on existing knowledge and seeks to confirm or refute causal hypotheses through systematic investigation.
- Both exploratory and causal research contribute to the generation of knowledge and theory development.
- Both types of research involve systematic inquiry and data analysis to answer research questions.
- Exploratory research focuses on generating hypotheses and exploring new areas of inquiry, while causal research aims to test hypotheses and establish causal relationships.
- Exploratory research is more flexible and open-ended, while causal research follows a more structured and hypothesis-driven approach.
Correlational vs. Causal Research
Correlational research examines the relationship between variables without implying causation. It identifies patterns of association or co-occurrence between variables but does not establish the direction or causality of the relationship.
Causal research , on the other hand, seeks to establish cause-and-effect relationships between variables by systematically manipulating independent variables and observing their effects on dependent variables. It goes beyond mere association to determine whether changes in one variable directly cause changes in another variable.
- Both correlational and causal research involve analyzing relationships between variables.
- Both types of research contribute to understanding the nature of associations between variables.
- Correlational research focuses on identifying patterns of association, while causal research aims to establish causal relationships.
- Correlational research does not manipulate variables, while causal research involves systematically manipulating independent variables to observe their effects on dependent variables.
How to Formulate Causal Research Hypotheses?
Crafting research questions and hypotheses is the foundational step in any research endeavor. Defining your variables clearly and articulating the causal relationship you aim to investigate is essential. Let's explore this process further.
1. Identify Variables
Identifying variables involves recognizing the key factors you will manipulate or measure in your study. These variables can be classified into independent, dependent, and confounding variables.
- Independent Variable (IV): This is the variable you manipulate or control in your study. It is the presumed cause that you want to test.
- Dependent Variable (DV): The dependent variable is the outcome or response you measure. It is affected by changes in the independent variable.
- Confounding Variables: These are extraneous factors that may influence the relationship between the independent and dependent variables, leading to spurious correlations or erroneous causal inferences. Identifying and controlling for confounding variables is crucial for establishing valid causal relationships.
2. Establish Causality
Establishing causality requires meeting specific criteria outlined by scientific methodology. While correlation between variables may suggest a relationship, it does not imply causation. To establish causality, researchers must demonstrate the following:
- Temporal Precedence: The cause must precede the effect in time. In other words, changes in the independent variable must occur before changes in the dependent variable.
- Covariation of Cause and Effect: Changes in the independent variable should be accompanied by corresponding changes in the dependent variable. This demonstrates a consistent pattern of association between the two variables.
- Elimination of Alternative Explanations: Researchers must rule out other possible explanations for the observed relationship between variables. This involves controlling for confounding variables and conducting rigorous experimental designs to isolate the effects of the independent variable.
3. Write Clear and Testable Hypotheses
Hypotheses serve as tentative explanations for the relationship between variables and provide a framework for empirical testing. A well-formulated hypothesis should be:
- Specific: Clearly state the expected relationship between the independent and dependent variables.
- Testable: The hypothesis should be capable of being empirically tested through observation or experimentation.
- Falsifiable: There should be a possibility of proving the hypothesis false through empirical evidence.
For example, a hypothesis in a study examining the effect of exercise on weight loss could be: "Increasing levels of physical activity (IV) will lead to greater weight loss (DV) among participants (compared to those with lower levels of physical activity)."
By formulating clear hypotheses and operationalizing variables, researchers can systematically investigate causal relationships and contribute to the advancement of scientific knowledge.
Causal Research Design
Designing your research study involves making critical decisions about how you will collect and analyze data to investigate causal relationships.
Experimental vs. Observational Designs
One of the first decisions you'll make when designing a study is whether to employ an experimental or observational design. Each approach has its strengths and limitations, and the choice depends on factors such as the research question, feasibility , and ethical considerations.
- Experimental Design: In experimental designs, researchers manipulate the independent variable and observe its effects on the dependent variable while controlling for confounding variables. Random assignment to experimental conditions allows for causal inferences to be drawn. Example: A study testing the effectiveness of a new teaching method on student performance by randomly assigning students to either the experimental group (receiving the new teaching method) or the control group (receiving the traditional method).
- Observational Design: Observational designs involve observing and measuring variables without intervention. Researchers may still examine relationships between variables but cannot establish causality as definitively as in experimental designs. Example: A study observing the association between socioeconomic status and health outcomes by collecting data on income, education level, and health indicators from a sample of participants.
Control and Randomization
Control and randomization are crucial aspects of experimental design that help ensure the validity of causal inferences.
- Control: Controlling for extraneous variables involves holding constant factors that could influence the dependent variable, except for the independent variable under investigation. This helps isolate the effects of the independent variable. Example: In a medication trial, controlling for factors such as age, gender, and pre-existing health conditions ensures that any observed differences in outcomes can be attributed to the medication rather than other variables.
- Randomization: Random assignment of participants to experimental conditions helps distribute potential confounders evenly across groups, reducing the likelihood of systematic biases and allowing for causal conclusions. Example: Randomly assigning patients to treatment and control groups in a clinical trial ensures that both groups are comparable in terms of baseline characteristics, minimizing the influence of extraneous variables on treatment outcomes.
Internal and External Validity
Two key concepts in research design are internal validity and external validity, which relate to the credibility and generalizability of study findings, respectively.
- Internal Validity: Internal validity refers to the extent to which the observed effects can be attributed to the manipulation of the independent variable rather than confounding factors. Experimental designs typically have higher internal validity due to their control over extraneous variables. Example: A study examining the impact of a training program on employee productivity would have high internal validity if it could confidently attribute changes in productivity to the training intervention.
- External Validity: External validity concerns the extent to which study findings can be generalized to other populations, settings, or contexts. While experimental designs prioritize internal validity, they may sacrifice external validity by using highly controlled conditions that do not reflect real-world scenarios. Example: Findings from a laboratory study on memory retention may have limited external validity if the experimental tasks and conditions differ significantly from real-life learning environments.
Types of Experimental Designs
Several types of experimental designs are commonly used in causal research, each with its own strengths and applications.
- Randomized Control Trials (RCTs): RCTs are considered the gold standard for assessing causality in research. Participants are randomly assigned to experimental and control groups, allowing researchers to make causal inferences. Example: A pharmaceutical company testing a new drug's efficacy would use an RCT to compare outcomes between participants receiving the drug and those receiving a placebo.
- Quasi-Experimental Designs: Quasi-experimental designs lack random assignment but still attempt to establish causality by controlling for confounding variables through design or statistical analysis . Example: A study evaluating the effectiveness of a smoking cessation program might compare outcomes between participants who voluntarily enroll in the program and a matched control group of non-enrollees.
By carefully selecting an appropriate research design and addressing considerations such as control, randomization, and validity, researchers can conduct studies that yield credible evidence of causal relationships and contribute valuable insights to their field of inquiry.
Causal Research Data Collection
Collecting data is a critical step in any research study, and the quality of the data directly impacts the validity and reliability of your findings.
Choosing Measurement Instruments
Selecting appropriate measurement instruments is essential for accurately capturing the variables of interest in your study. The choice of measurement instrument depends on factors such as the nature of the variables, the target population , and the research objectives.
- Surveys : Surveys are commonly used to collect self-reported data on attitudes, opinions, behaviors, and demographics . They can be administered through various methods, including paper-and-pencil surveys, online surveys, and telephone interviews.
- Observations: Observational methods involve systematically recording behaviors, events, or phenomena as they occur in natural settings. Observations can be structured (following a predetermined checklist) or unstructured (allowing for flexible data collection).
- Psychological Tests: Psychological tests are standardized instruments designed to measure specific psychological constructs, such as intelligence, personality traits, or emotional functioning. These tests often have established reliability and validity.
- Physiological Measures: Physiological measures, such as heart rate, blood pressure, or brain activity, provide objective data on bodily processes. They are commonly used in health-related research but require specialized equipment and expertise.
- Existing Databases: Researchers may also utilize existing datasets, such as government surveys, public health records, or organizational databases, to answer research questions. Secondary data analysis can be cost-effective and time-saving but may be limited by the availability and quality of data.
Ensuring accurate data collection is the cornerstone of any successful research endeavor. With the right tools in place, you can unlock invaluable insights to drive your causal research forward. From surveys to tests, each instrument offers a unique lens through which to explore your variables of interest.
At Appinio , we understand the importance of robust data collection methods in informing impactful decisions. Let us empower your research journey with our intuitive platform, where you can effortlessly gather real-time consumer insights to fuel your next breakthrough. Ready to take your research to the next level? Book a demo today and see how Appinio can revolutionize your approach to data collection!
Book a Demo
Sampling Techniques
Sampling involves selecting a subset of individuals or units from a larger population to participate in the study. The goal of sampling is to obtain a representative sample that accurately reflects the characteristics of the population of interest.
- Probability Sampling: Probability sampling methods involve randomly selecting participants from the population, ensuring that each member of the population has an equal chance of being included in the sample. Common probability sampling techniques include simple random sampling , stratified sampling, and cluster sampling .
- Non-Probability Sampling: Non-probability sampling methods do not involve random selection and may introduce biases into the sample. Examples of non-probability sampling techniques include convenience sampling, purposive sampling, and snowball sampling.
The choice of sampling technique depends on factors such as the research objectives, population characteristics, resources available, and practical constraints. Researchers should strive to minimize sampling bias and maximize the representativeness of the sample to enhance the generalizability of their findings.
Ethical Considerations
Ethical considerations are paramount in research and involve ensuring the rights, dignity, and well-being of research participants. Researchers must adhere to ethical principles and guidelines established by professional associations and institutional review boards (IRBs).
- Informed Consent: Participants should be fully informed about the nature and purpose of the study, potential risks and benefits, their rights as participants, and any confidentiality measures in place. Informed consent should be obtained voluntarily and without coercion.
- Privacy and Confidentiality: Researchers should take steps to protect the privacy and confidentiality of participants' personal information. This may involve anonymizing data, securing data storage, and limiting access to identifiable information.
- Minimizing Harm: Researchers should mitigate any potential physical, psychological, or social harm to participants. This may involve conducting risk assessments, providing appropriate support services, and debriefing participants after the study.
- Respect for Participants: Researchers should respect participants' autonomy, diversity, and cultural values. They should seek to foster a trusting and respectful relationship with participants throughout the research process.
- Publication and Dissemination: Researchers have a responsibility to accurately report their findings and acknowledge contributions from participants and collaborators. They should adhere to principles of academic integrity and transparency in disseminating research results.
By addressing ethical considerations in research design and conduct, researchers can uphold the integrity of their work, maintain trust with participants and the broader community, and contribute to the responsible advancement of knowledge in their field.
Causal Research Data Analysis
Once data is collected, it must be analyzed to draw meaningful conclusions and assess causal relationships.
Causal Inference Methods
Causal inference methods are statistical techniques used to identify and quantify causal relationships between variables in observational data. While experimental designs provide the most robust evidence for causality, observational studies often require more sophisticated methods to account for confounding factors.
- Difference-in-Differences (DiD): DiD compares changes in outcomes before and after an intervention between a treatment group and a control group, controlling for pre-existing trends. It estimates the average treatment effect by differencing the changes in outcomes between the two groups over time.
- Instrumental Variables (IV): IV analysis relies on instrumental variables—variables that affect the treatment variable but not the outcome—to estimate causal effects in the presence of endogeneity. IVs should be correlated with the treatment but uncorrelated with the error term in the outcome equation.
- Regression Discontinuity (RD): RD designs exploit naturally occurring thresholds or cutoff points to estimate causal effects near the threshold. Participants just above and below the threshold are compared, assuming that they are similar except for their proximity to the threshold.
- Propensity Score Matching (PSM): PSM matches individuals or units based on their propensity scores—the likelihood of receiving the treatment—creating comparable groups with similar observed characteristics. Matching reduces selection bias and allows for causal inference in observational studies.
Assessing Causality Strength
Assessing the strength of causality involves determining the magnitude and direction of causal effects between variables. While statistical significance indicates whether an observed relationship is unlikely to occur by chance, it does not necessarily imply a strong or meaningful effect.
- Effect Size: Effect size measures the magnitude of the relationship between variables, providing information about the practical significance of the results. Standard effect size measures include Cohen's d for mean differences and odds ratios for categorical outcomes.
- Confidence Intervals: Confidence intervals provide a range of values within which the actual effect size is likely to lie with a certain degree of certainty. Narrow confidence intervals indicate greater precision in estimating the true effect size.
- Practical Significance: Practical significance considers whether the observed effect is meaningful or relevant in real-world terms. Researchers should interpret results in the context of their field and the implications for stakeholders.
Handling Confounding Variables
Confounding variables are extraneous factors that may distort the observed relationship between the independent and dependent variables, leading to spurious or biased conclusions. Addressing confounding variables is essential for establishing valid causal inferences.
- Statistical Control: Statistical control involves including confounding variables as covariates in regression models to partially out their effects on the outcome variable. Controlling for confounders reduces bias and strengthens the validity of causal inferences.
- Matching: Matching participants or units based on observed characteristics helps create comparable groups with similar distributions of confounding variables. Matching reduces selection bias and mimics the randomization process in experimental designs.
- Sensitivity Analysis: Sensitivity analysis assesses the robustness of study findings to changes in model specifications or assumptions. By varying analytical choices and examining their impact on results, researchers can identify potential sources of bias and evaluate the stability of causal estimates.
- Subgroup Analysis: Subgroup analysis explores whether the relationship between variables differs across subgroups defined by specific characteristics. Identifying effect modifiers helps understand the conditions under which causal effects may vary.
By employing rigorous causal inference methods, assessing the strength of causality, and addressing confounding variables, researchers can confidently draw valid conclusions about causal relationships in their studies, advancing scientific knowledge and informing evidence-based decision-making.
Causal Research Examples
Examples play a crucial role in understanding the application of causal research methods and their impact across various domains. Let's explore some detailed examples to illustrate how causal research is conducted and its real-world implications:
Example 1: Software as a Service (SaaS) User Retention Analysis
Suppose a SaaS company wants to understand the factors influencing user retention and engagement with their platform. The company conducts a longitudinal observational study, collecting data on user interactions, feature usage, and demographic information over several months.
- Design: The company employs an observational cohort study design, tracking cohorts of users over time to observe changes in retention and engagement metrics. They use analytics tools to collect data on user behavior , such as logins, feature usage, session duration, and customer support interactions.
- Data Collection: Data is collected from the company's platform logs, customer relationship management (CRM) system, and user surveys. Key metrics include user churn rates, active user counts, feature adoption rates, and Net Promoter Scores ( NPS ).
- Analysis: Using statistical techniques like survival analysis and regression modeling, the company identifies factors associated with user retention, such as feature usage patterns, onboarding experiences, customer support interactions, and subscription plan types.
- Findings: The analysis reveals that users who engage with specific features early in their lifecycle have higher retention rates, while those who encounter usability issues or lack personalized onboarding experiences are more likely to churn. The company uses these insights to optimize product features, improve onboarding processes, and enhance customer support strategies to increase user retention and satisfaction.
Example 2: Business Impact of Digital Marketing Campaign
Consider a technology startup launching a digital marketing campaign to promote its new product offering. The company conducts an experimental study to evaluate the effectiveness of different marketing channels in driving website traffic, lead generation, and sales conversions.
- Design: The company implements an A/B testing design, randomly assigning website visitors to different marketing treatment conditions, such as Google Ads, social media ads, email campaigns, or content marketing efforts. They track user interactions and conversion events using web analytics tools and marketing automation platforms.
- Data Collection: Data is collected on website traffic, click-through rates, conversion rates, lead generation, and sales revenue. The company also gathers demographic information and user feedback through surveys and customer interviews to understand the impact of marketing messages and campaign creatives .
- Analysis: Utilizing statistical methods like hypothesis testing and multivariate analysis, the company compares key performance metrics across different marketing channels to assess their effectiveness in driving user engagement and conversion outcomes. They calculate return on investment (ROI) metrics to evaluate the cost-effectiveness of each marketing channel.
- Findings: The analysis reveals that social media ads outperform other marketing channels in generating website traffic and lead conversions, while email campaigns are more effective in nurturing leads and driving sales conversions. Armed with these insights, the company allocates marketing budgets strategically, focusing on channels that yield the highest ROI and adjusting messaging and targeting strategies to optimize campaign performance.
These examples demonstrate the diverse applications of causal research methods in addressing important questions, informing policy decisions, and improving outcomes in various fields. By carefully designing studies, collecting relevant data, employing appropriate analysis techniques, and interpreting findings rigorously, researchers can generate valuable insights into causal relationships and contribute to positive social change.
How to Interpret Causal Research Results?
Interpreting and reporting research findings is a crucial step in the scientific process, ensuring that results are accurately communicated and understood by stakeholders.
Interpreting Statistical Significance
Statistical significance indicates whether the observed results are unlikely to occur by chance alone, but it does not necessarily imply practical or substantive importance. Interpreting statistical significance involves understanding the meaning of p-values and confidence intervals and considering their implications for the research findings.
- P-values: A p-value represents the probability of obtaining the observed results (or more extreme results) if the null hypothesis is true. A p-value below a predetermined threshold (typically 0.05) suggests that the observed results are statistically significant, indicating that the null hypothesis can be rejected in favor of the alternative hypothesis.
- Confidence Intervals: Confidence intervals provide a range of values within which the true population parameter is likely to lie with a certain degree of confidence (e.g., 95%). If the confidence interval does not include the null value, it suggests that the observed effect is statistically significant at the specified confidence level.
Interpreting statistical significance requires considering factors such as sample size, effect size, and the practical relevance of the results rather than relying solely on p-values to draw conclusions.
Discussing Practical Significance
While statistical significance indicates whether an effect exists, practical significance evaluates the magnitude and meaningfulness of the effect in real-world terms. Discussing practical significance involves considering the relevance of the results to stakeholders and assessing their impact on decision-making and practice.
- Effect Size: Effect size measures the magnitude of the observed effect, providing information about its practical importance. Researchers should interpret effect sizes in the context of their field and the scale of measurement (e.g., small, medium, or large effect sizes).
- Contextual Relevance: Consider the implications of the results for stakeholders, policymakers, and practitioners. Are the observed effects meaningful in the context of existing knowledge, theory, or practical applications? How do the findings contribute to addressing real-world problems or informing decision-making?
Discussing practical significance helps contextualize research findings and guide their interpretation and application in practice, beyond statistical significance alone.
Addressing Limitations and Assumptions
No study is without limitations, and researchers should transparently acknowledge and address potential biases, constraints, and uncertainties in their research design and findings.
- Methodological Limitations: Identify any limitations in study design, data collection, or analysis that may affect the validity or generalizability of the results. For example, sampling biases , measurement errors, or confounding variables.
- Assumptions: Discuss any assumptions made in the research process and their implications for the interpretation of results. Assumptions may relate to statistical models, causal inference methods, or theoretical frameworks underlying the study.
- Alternative Explanations: Consider alternative explanations for the observed results and discuss their potential impact on the validity of causal inferences. How robust are the findings to different interpretations or competing hypotheses?
Addressing limitations and assumptions demonstrates transparency and rigor in the research process, allowing readers to critically evaluate the validity and reliability of the findings.
Communicating Findings Clearly
Effectively communicating research findings is essential for disseminating knowledge, informing decision-making, and fostering collaboration and dialogue within the scientific community.
- Clarity and Accessibility: Present findings in a clear, concise, and accessible manner, using plain language and avoiding jargon or technical terminology. Organize information logically and use visual aids (e.g., tables, charts, graphs) to enhance understanding.
- Contextualization: Provide context for the results by summarizing key findings, highlighting their significance, and relating them to existing literature or theoretical frameworks. Discuss the implications of the findings for theory, practice, and future research directions.
- Transparency: Be transparent about the research process, including data collection procedures, analytical methods, and any limitations or uncertainties associated with the findings. Clearly state any conflicts of interest or funding sources that may influence interpretation.
By communicating findings clearly and transparently, researchers can facilitate knowledge exchange, foster trust and credibility, and contribute to evidence-based decision-making.
Causal Research Tips
When conducting causal research, it's essential to approach your study with careful planning, attention to detail, and methodological rigor. Here are some tips to help you navigate the complexities of causal research effectively:
- Define Clear Research Questions: Start by clearly defining your research questions and hypotheses. Articulate the causal relationship you aim to investigate and identify the variables involved.
- Consider Alternative Explanations: Be mindful of potential confounding variables and alternative explanations for the observed relationships. Take steps to control for confounders and address alternative hypotheses in your analysis.
- Prioritize Internal Validity: While external validity is important for generalizability, prioritize internal validity in your study design to ensure that observed effects can be attributed to the manipulation of the independent variable.
- Use Randomization When Possible: If feasible, employ randomization in experimental designs to distribute potential confounders evenly across experimental conditions and enhance the validity of causal inferences.
- Be Transparent About Methods: Provide detailed descriptions of your research methods, including data collection procedures, analytical techniques, and any assumptions or limitations associated with your study.
- Utilize Multiple Methods: Consider using a combination of experimental and observational methods to triangulate findings and strengthen the validity of causal inferences.
- Be Mindful of Sample Size: Ensure that your sample size is adequate to detect meaningful effects and minimize the risk of Type I and Type II errors. Conduct power analyses to determine the sample size needed to achieve sufficient statistical power.
- Validate Measurement Instruments: Validate your measurement instruments to ensure that they are reliable and valid for assessing the variables of interest in your study. Pilot test your instruments if necessary.
- Seek Feedback from Peers: Collaborate with colleagues or seek feedback from peer reviewers to solicit constructive criticism and improve the quality of your research design and analysis.
Conclusion for Causal Research
Mastering causal research empowers researchers to unlock the secrets of cause and effect, shedding light on the intricate relationships between variables in diverse fields. By employing rigorous methods such as experimental designs, causal inference techniques, and careful data analysis, you can uncover causal mechanisms, predict outcomes, and inform evidence-based practices. Through the lens of causal research, complex phenomena become more understandable, and interventions become more effective in addressing societal challenges and driving progress. In a world where understanding the reasons behind events is paramount, causal research serves as a beacon of clarity and insight. Armed with the knowledge and techniques outlined in this guide, you can navigate the complexities of causality with confidence, advancing scientific knowledge, guiding policy decisions, and ultimately making meaningful contributions to our understanding of the world.
How to Conduct Causal Research in Minutes?
Introducing Appinio , your gateway to lightning-fast causal research. As a real-time market research platform, we're revolutionizing how companies gain consumer insights to drive data-driven decisions. With Appinio, conducting your own market research is not only easy but also thrilling. Experience the excitement of market research with Appinio, where fast, intuitive, and impactful insights are just a click away.
Here's why you'll love Appinio:
- Instant Insights: Say goodbye to waiting days for research results. With our platform, you'll go from questions to insights in minutes, empowering you to make decisions at the speed of business.
- User-Friendly Interface: No need for a research degree here! Our intuitive platform is designed for anyone to use, making complex research tasks simple and accessible.
- Global Reach: Reach your target audience wherever they are. With access to over 90 countries and the ability to define precise target groups from 1200+ characteristics, you'll gather comprehensive data to inform your decisions.
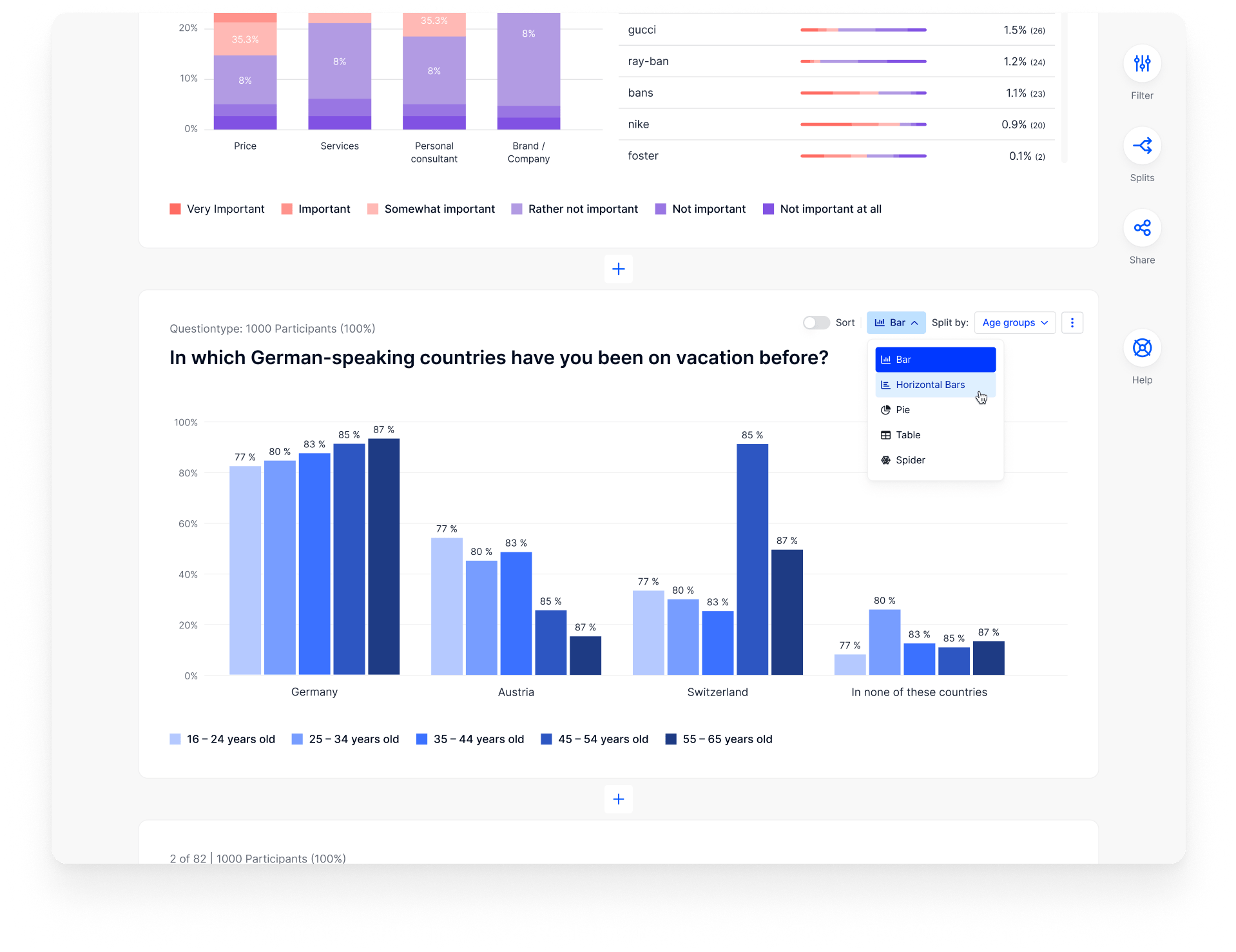
Get free access to the platform!
Join the loop 💌
Be the first to hear about new updates, product news, and data insights. We'll send it all straight to your inbox.
Get the latest market research news straight to your inbox! 💌
Wait, there's more
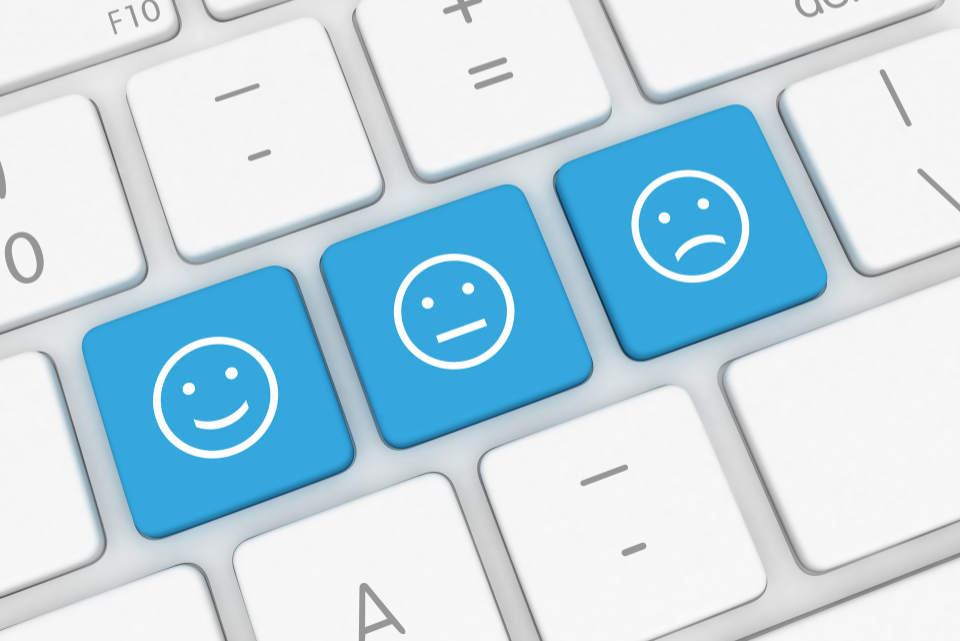
18.06.2024 | 30min read
What is a Pulse Survey? Definition, Types, Questions
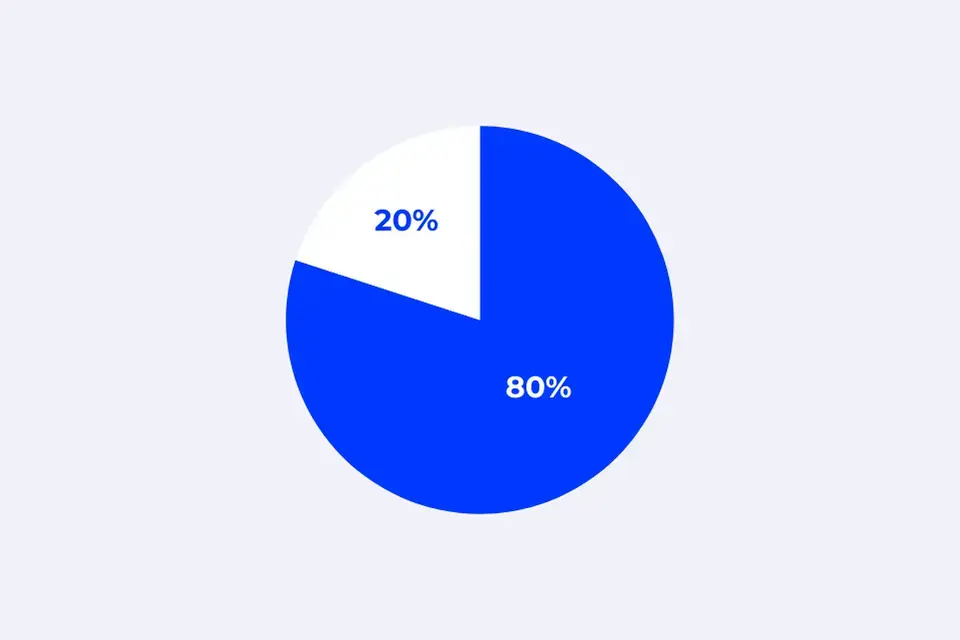
30.05.2024 | 29min read
Pareto Analysis: Definition, Pareto Chart, Examples
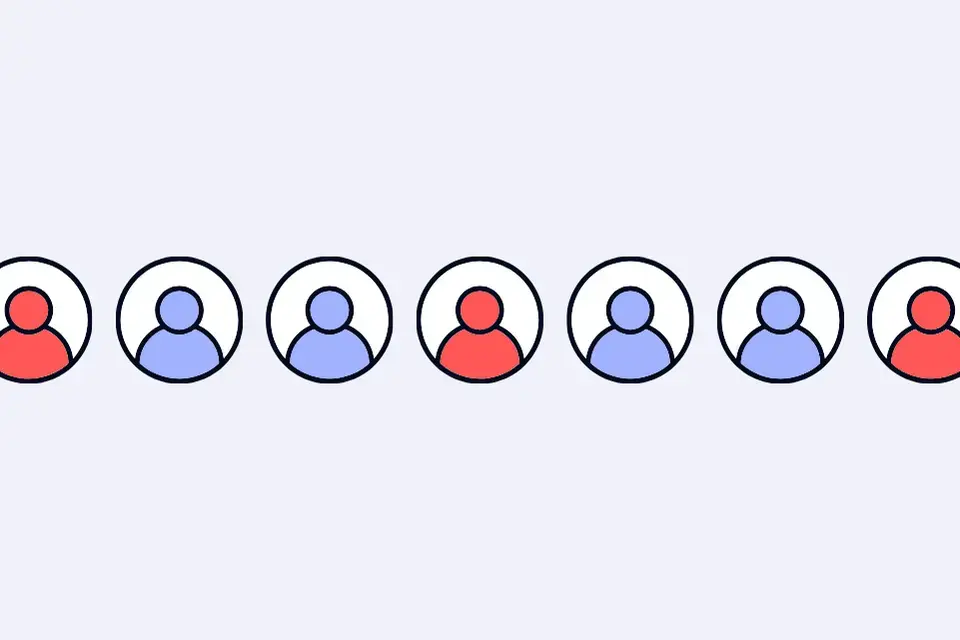
28.05.2024 | 32min read
What is Systematic Sampling? Definition, Types, Examples
What is causal research design?
Last updated
14 May 2023
Reviewed by
Short on time? Get an AI generated summary of this article instead
Examining these relationships gives researchers valuable insights into the mechanisms that drive the phenomena they are investigating.
Organizations primarily use causal research design to identify, determine, and explore the impact of changes within an organization and the market. You can use a causal research design to evaluate the effects of certain changes on existing procedures, norms, and more.
This article explores causal research design, including its elements, advantages, and disadvantages.
Analyze your causal research
Dovetail streamlines causal research analysis to help you uncover and share actionable insights
- Components of causal research
You can demonstrate the existence of cause-and-effect relationships between two factors or variables using specific causal information, allowing you to produce more meaningful results and research implications.
These are the key inputs for causal research:
The timeline of events
Ideally, the cause must occur before the effect. You should review the timeline of two or more separate events to determine the independent variables (cause) from the dependent variables (effect) before developing a hypothesis.
If the cause occurs before the effect, you can link cause and effect and develop a hypothesis .
For instance, an organization may notice a sales increase. Determining the cause would help them reproduce these results.
Upon review, the business realizes that the sales boost occurred right after an advertising campaign. The business can leverage this time-based data to determine whether the advertising campaign is the independent variable that caused a change in sales.
Evaluation of confounding variables
In most cases, you need to pinpoint the variables that comprise a cause-and-effect relationship when using a causal research design. This uncovers a more accurate conclusion.
Co-variations between a cause and effect must be accurate, and a third factor shouldn’t relate to cause and effect.
Observing changes
Variation links between two variables must be clear. A quantitative change in effect must happen solely due to a quantitative change in the cause.
You can test whether the independent variable changes the dependent variable to evaluate the validity of a cause-and-effect relationship. A steady change between the two variables must occur to back up your hypothesis of a genuine causal effect.
- Why is causal research useful?
Causal research allows market researchers to predict hypothetical occurrences and outcomes while enhancing existing strategies. Organizations can use this concept to develop beneficial plans.
Causal research is also useful as market researchers can immediately deduce the effect of the variables on each other under real-world conditions.
Once researchers complete their first experiment, they can use their findings. Applying them to alternative scenarios or repeating the experiment to confirm its validity can produce further insights.
Businesses widely use causal research to identify and comprehend the effect of strategic changes on their profits.
- How does causal research compare and differ from other research types?
Other research types that identify relationships between variables include exploratory and descriptive research .
Here’s how they compare and differ from causal research designs:
Exploratory research
An exploratory research design evaluates situations where a problem or opportunity's boundaries are unclear. You can use this research type to test various hypotheses and assumptions to establish facts and understand a situation more clearly.
You can also use exploratory research design to navigate a topic and discover the relevant variables. This research type allows flexibility and adaptability as the experiment progresses, particularly since no area is off-limits.
It’s worth noting that exploratory research is unstructured and typically involves collecting qualitative data . This provides the freedom to tweak and amend the research approach according to your ongoing thoughts and assessments.
Unfortunately, this exposes the findings to the risk of bias and may limit the extent to which a researcher can explore a topic.
This table compares the key characteristics of causal and exploratory research:
|
|
|
Main research statement | Research hypotheses | Research question |
Amount of uncertainty characterizing decision situation | Clearly defined | Highly ambiguous |
Research approach | Highly structured | Unstructured |
When you conduct it | Later stages of decision-making | Early stages of decision-making |
Descriptive research
This research design involves capturing and describing the traits of a population, situation, or phenomenon. Descriptive research focuses more on the " what " of the research subject and less on the " why ."
Since descriptive research typically happens in a real-world setting, variables can cross-contaminate others. This increases the challenge of isolating cause-and-effect relationships.
You may require further research if you need more causal links.
This table compares the key characteristics of causal and descriptive research.
|
|
|
Main research statement | Research hypotheses | Research question |
Amount of uncertainty characterizing decision situation | Clearly defined | Partially defined |
Research approach | Highly structured | Structured |
When you conduct it | Later stages of decision-making | Later stages of decision-making |
Causal research examines a research question’s variables and how they interact. It’s easier to pinpoint cause and effect since the experiment often happens in a controlled setting.
Researchers can conduct causal research at any stage, but they typically use it once they know more about the topic.
In contrast, causal research tends to be more structured and can be combined with exploratory and descriptive research to help you attain your research goals.
- How can you use causal research effectively?
Here are common ways that market researchers leverage causal research effectively:
Market and advertising research
Do you want to know if your new marketing campaign is affecting your organization positively? You can use causal research to determine the variables causing negative or positive impacts on your campaign.
Improving customer experiences and loyalty levels
Consumers generally enjoy purchasing from brands aligned with their values. They’re more likely to purchase from such brands and positively represent them to others.
You can use causal research to identify the variables contributing to increased or reduced customer acquisition and retention rates.
Could the cause of increased customer retention rates be streamlined checkout?
Perhaps you introduced a new solution geared towards directly solving their immediate problem.
Whatever the reason, causal research can help you identify the cause-and-effect relationship. You can use this to enhance your customer experiences and loyalty levels.
Improving problematic employee turnover rates
Is your organization experiencing skyrocketing attrition rates?
You can leverage the features and benefits of causal research to narrow down the possible explanations or variables with significant effects on employees quitting.
This way, you can prioritize interventions, focusing on the highest priority causal influences, and begin to tackle high employee turnover rates.
- Advantages of causal research
The main benefits of causal research include the following:
Effectively test new ideas
If causal research can pinpoint the precise outcome through combinations of different variables, researchers can test ideas in the same manner to form viable proof of concepts.
Achieve more objective results
Market researchers typically use random sampling techniques to choose experiment participants or subjects in causal research. This reduces the possibility of exterior, sample, or demography-based influences, generating more objective results.
Improved business processes
Causal research helps businesses understand which variables positively impact target variables, such as customer loyalty or sales revenues. This helps them improve their processes, ROI, and customer and employee experiences.
Guarantee reliable and accurate results
Upon identifying the correct variables, researchers can replicate cause and effect effortlessly. This creates reliable data and results to draw insights from.
Internal organization improvements
Businesses that conduct causal research can make informed decisions about improving their internal operations and enhancing employee experiences.
- Disadvantages of causal research
Like any other research method, casual research has its set of drawbacks that include:
Extra research to ensure validity
Researchers can't simply rely on the outcomes of causal research since it isn't always accurate. There may be a need to conduct other research types alongside it to ensure accurate output.
Coincidence
Coincidence tends to be the most significant error in causal research. Researchers often misinterpret a coincidental link between a cause and effect as a direct causal link.
Administration challenges
Causal research can be challenging to administer since it's impossible to control the impact of extraneous variables .
Giving away your competitive advantage
If you intend to publish your research, it exposes your information to the competition.
Competitors may use your research outcomes to identify your plans and strategies to enter the market before you.
- Causal research examples
Multiple fields can use causal research, so it serves different purposes, such as.
Customer loyalty research
Organizations and employees can use causal research to determine the best customer attraction and retention approaches.
They monitor interactions between customers and employees to identify cause-and-effect patterns. That could be a product demonstration technique resulting in higher or lower sales from the same customers.
Example: Business X introduces a new individual marketing strategy for a small customer group and notices a measurable increase in monthly subscriptions.
Upon getting identical results from different groups, the business concludes that the individual marketing strategy resulted in the intended causal relationship.
Advertising research
Businesses can also use causal research to implement and assess advertising campaigns.
Example: Business X notices a 7% increase in sales revenue a few months after a business introduces a new advertisement in a certain region. The business can run the same ad in random regions to compare sales data over the same period.
This will help the company determine whether the ad caused the sales increase. If sales increase in these randomly selected regions, the business could conclude that advertising campaigns and sales share a cause-and-effect relationship.
Educational research
Academics, teachers, and learners can use causal research to explore the impact of politics on learners and pinpoint learner behavior trends.
Example: College X notices that more IT students drop out of their program in their second year, which is 8% higher than any other year.
The college administration can interview a random group of IT students to identify factors leading to this situation, including personal factors and influences.
With the help of in-depth statistical analysis, the institution's researchers can uncover the main factors causing dropout. They can create immediate solutions to address the problem.
Is a causal variable dependent or independent?
When two variables have a cause-and-effect relationship, the cause is often called the independent variable. As such, the effect variable is dependent, i.e., it depends on the independent causal variable. An independent variable is only causal under experimental conditions.
What are the three criteria for causality?
The three conditions for causality are:
Temporality/temporal precedence: The cause must precede the effect.
Rationality: One event predicts the other with an explanation, and the effect must vary in proportion to changes in the cause.
Control for extraneous variables: The covariables must not result from other variables.
Is causal research experimental?
Causal research is mostly explanatory. Causal studies focus on analyzing a situation to explore and explain the patterns of relationships between variables.
Further, experiments are the primary data collection methods in studies with causal research design. However, as a research design, causal research isn't entirely experimental.
What is the difference between experimental and causal research design?
One of the main differences between causal and experimental research is that in causal research, the research subjects are already in groups since the event has already happened.
On the other hand, researchers randomly choose subjects in experimental research before manipulating the variables.
Should you be using a customer insights hub?
Do you want to discover previous research faster?
Do you share your research findings with others?
Do you analyze research data?
Start for free today, add your research, and get to key insights faster
Editor’s picks
Last updated: 18 April 2023
Last updated: 27 February 2023
Last updated: 6 February 2023
Last updated: 15 January 2024
Last updated: 6 October 2023
Last updated: 5 February 2023
Last updated: 16 April 2023
Last updated: 7 March 2023
Last updated: 9 March 2023
Last updated: 12 December 2023
Last updated: 11 March 2024
Last updated: 13 May 2024
Latest articles
Related topics, .css-je19u9{-webkit-align-items:flex-end;-webkit-box-align:flex-end;-ms-flex-align:flex-end;align-items:flex-end;display:-webkit-box;display:-webkit-flex;display:-ms-flexbox;display:flex;-webkit-flex-direction:row;-ms-flex-direction:row;flex-direction:row;-webkit-box-flex-wrap:wrap;-webkit-flex-wrap:wrap;-ms-flex-wrap:wrap;flex-wrap:wrap;-webkit-box-pack:center;-ms-flex-pack:center;-webkit-justify-content:center;justify-content:center;row-gap:0;text-align:center;max-width:671px;}@media (max-width: 1079px){.css-je19u9{max-width:400px;}.css-je19u9>span{white-space:pre;}}@media (max-width: 799px){.css-je19u9{max-width:400px;}.css-je19u9>span{white-space:pre;}} decide what to .css-1kiodld{max-height:56px;display:-webkit-box;display:-webkit-flex;display:-ms-flexbox;display:flex;-webkit-align-items:center;-webkit-box-align:center;-ms-flex-align:center;align-items:center;}@media (max-width: 1079px){.css-1kiodld{display:none;}} build next, decide what to build next.

Users report unexpectedly high data usage, especially during streaming sessions.

Users find it hard to navigate from the home page to relevant playlists in the app.

It would be great to have a sleep timer feature, especially for bedtime listening.

I need better filters to find the songs or artists I’m looking for.
Log in or sign up
Get started for free
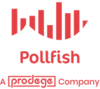
- Pollfish School
- Market Research
- Survey Guides
- Get started
- Understanding Causal Research & Why It’s Important for Your Business
Understanding Causal Research & Why It’s Important for Your Business
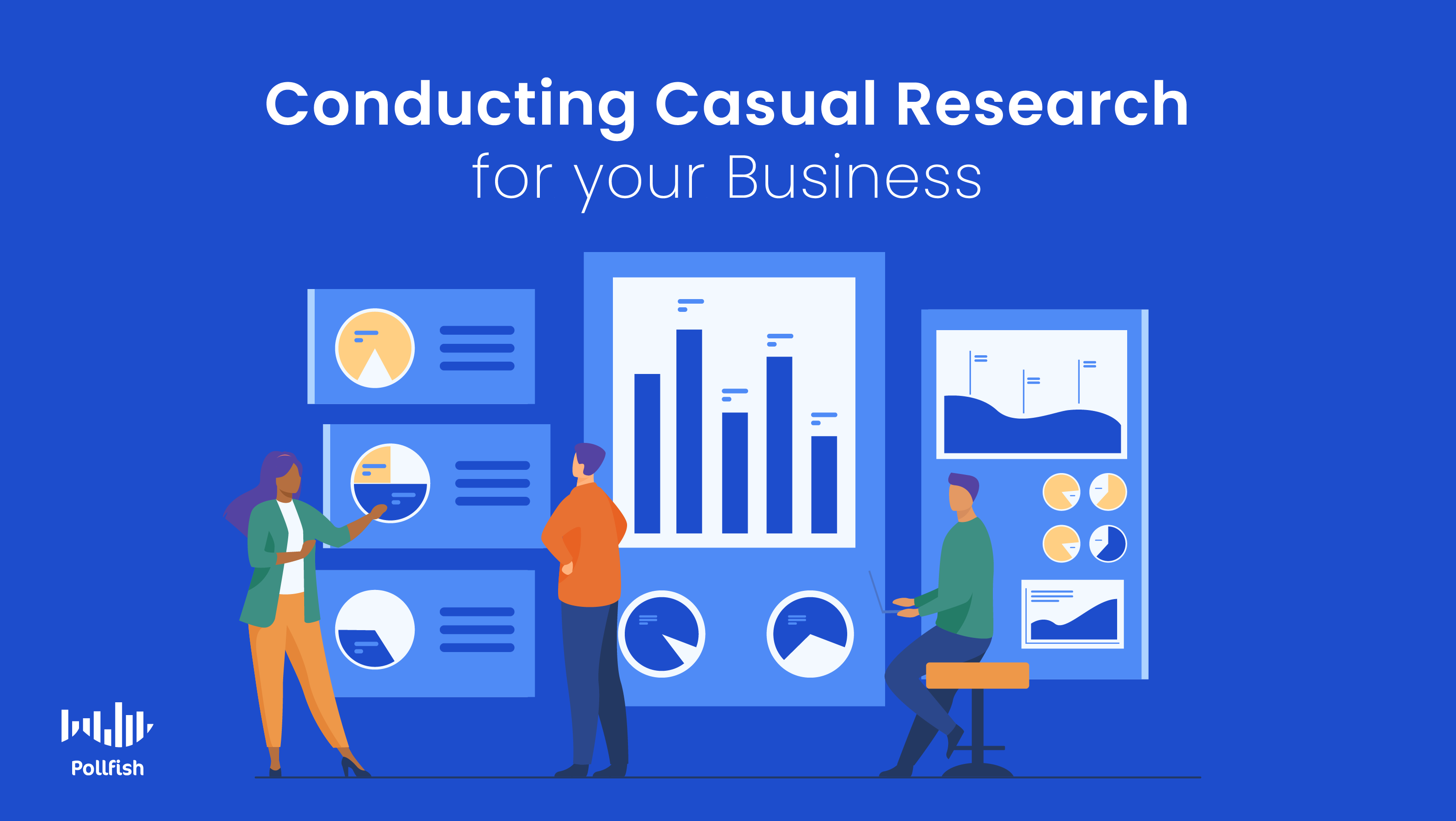
Causal research is one of the foremost kinds of research used with survey research. As such, it is employed across various verticals and can be implemented for any market research project.
Logically following correlational research and by following suit to it, it seeks to understand the relationship between variables — but it takes this pursuit further.
This is because causal research is chiefly involved with finding the cause and effect relationships between variables, as opposed to simply scouting their existence. Once researchers establish that a relationship between two variables exists, they ought to move to causal research to discover if and how they affect one another.
This article explains causal research in full detail, from what it is, to its importance, which surveys are most apt for it and more.
Defining Causal Research
Also called causal-comparative research, causal research seeks to find causal relationships , that is, cause and effect relationships between two or more variables .
Causal research is the latter form of an overall research process, as it follows after correlational research in the sequence of exploratory , descriptive and correlational research. This is because these research methods establish the details and aspects of the research process that lead up to causal research.
Although correlational research finds and explains the relationships between two variables, it does not prove that either of those variables causes the other to behave in some way or vice versa.
Causal research, on the other hand, has the capacity to determine whether one variable affects another variable.
As such, causal research carries two objectives, both of which are concerned with a possible causal relationship:
- Discovering which variables constitute the cause and which the effect.
- Establishing the relationship of the causal variables and their ensuing effect.
The Key Aspects of Causal Research
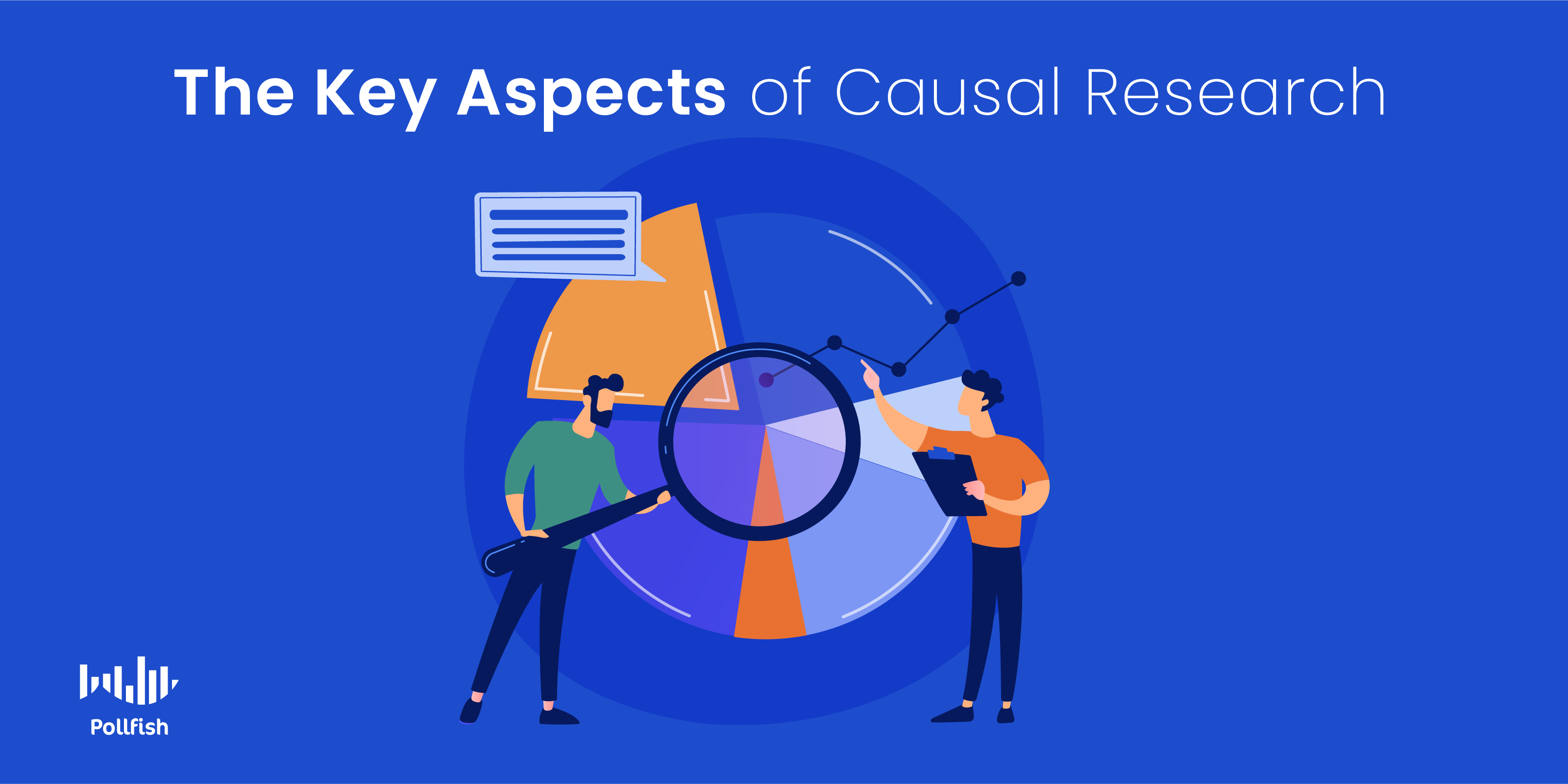
There are various characteristics that form causal research in addition to its two main objectives. Understanding these is key to deciding whether you need to perform this research, along with how to go about it.
The following enumerates the critical aspects of this kind of research:
- It entails discovering the existence of cause and effect relationships between two or more variables, via conducting experiments or testing markets in a controlled setting .
- It’s more scientific than exploratory and descriptive research.
- Market researchers conduct experiments, or test markets, in a controlled setting.
- Involves going beyond simply finding an association or correlation between the variables.
- Studies how a dependent variable is affected by independent variables.
- To convey causality, the variable relationship is framed as: If A happens, then B will take place/ happen.
- It uses a directional hypothesis, in which one (or a set of) independent variables affect another set of variables, known as dependent variables, in a particular manner.
- The studies help researchers understand both the course of the relationship of the variables and its forecasted effects.
- It produces quantitative research and is methodically planned, designed, and formatted,
- It yields statistically conclusive data. The objective of causal research is to test hypotheses about cause-and-effect relationships
Why Your Business Needs Causal Research
With causal research, market researchers can predict hypothetical occurrences and outcome s. This allows a business to create a business plan most beneficial to the company.
Causal research offers an advantage for businesses in that, learning how one variable impacts another helps predict the effects of all kinds of business matters in cause and effect relationships.
This allows companies to inspect and analyze a business strategy before launching it. As such, understanding the causal relationships before enacting this strategy ensures that companies avoid ineffective and costly campaigns.
Additionally, it helps companies discover the variable relationships with favorable outcomes for businesses , empowering them to make educated changes.
Causal research is necessary, as it uses pre-existing data to unearth relationships. This helps find causative variables from a period of time. For example, brands can look into how weather patterns affected in-store versus online shopping from several months ago. They can then compare it to current weather conditions and behaviors.
This particular study helps establish a forecast on how similar weather situations will affect the two forms of shopping.
The causal relationships discovered in this study show businesses how to prepare for certain weather conditions and which form of shopping — digital versus in-store is most auspicious for revenue and which one tends to lag.
Understanding the causal relationships in this scenario allows businesses to better plan for each situation, along with avoiding certain efforts in response.
There are many other independent or predictor variables that can take hold on dependent, aka, response variables in the business realm. In this sector specifically, these are some of the key independent and dependent variables:
Key Independent Variables
- Digital user experience (DX) such as new site features
- Advertisements
- Marketing activity (SEO, SEM, social media announcements, retargeting, etc.)
- Inventory (new products or upgrades)
- Interactions with sales agents
Key Dependent variables (which businesses depend on to be in their favor):
- VoC feedback (whether positive or negative)
- Site traffic
- In-store visits
- Time spent on a website, bounce rates, etc.
An Example of Causal Research for Business
The previous section included an example of how a business can use causal research to their advantage. There are plenty of other instances that require conducting causal research. The following is another example of causal research in business.
For example, to consolidate its fashion market research , an apparel brand considers studying its own business. Specifically, it seeks to determine and measure the impact of changing the color of a signature product.
Let’s say, the brand carries a signature black faux leather jacket and wants to measure the impact of changing its color to beige. The research obtained in this causal study would prove whether or not changing the color on a major product would affect its sales .
Other forms of businesses, including B2B businesses, can follow suit in their causal research, studying any of the independent variables aforementioned and how they affect the dependent variables.
Causal Research Survey Examples
There are various types of surveys you can use in causal research, however, surveys themselves do not prove causality. Causal research is largely dependent on conducting experiments. It is through these experiments that the research can deduce a cause and effect.
Nonetheless, here are some of the survey types to include in your research campaign.
To really connect the dots between cause and effect, we needed to create an experiment. This would include different renditions of the marketing collaterals, different markets, customers at different stages in the purchase cycle, and actions taken by competitors.
- Discovers the aspects of statistical significance within variables.
- Helpful in that causal research is quantitative in essence.
- Delves into past events, occurrences and attitudes in regards to the variables.
- Shows whether the variables changed and how so.
- Can find causative elements between variables over a period of time.
- Useful for studying variables to form predictions and understand outcomes.
- Helps businesses zero in on variables that contribute to or result from certain kinds of customer experiences.
- Allows businesses to test CX in relation to the responses from this survey.
- Measures various matters critical in a business or organization; surveys employees.
- Deployed more frequently, so variables can always be continually tracked.
- Especially useful when bringing new products/services into the market to compare them with previous ones.
- Features 4 subtypes of unique surveys.
How Causal Research Differs from Correlational, Exploratory and Experimental Research
Causal research shares some similarities with some of the other major forms of research, most notably, with correlational and experimental research; however, it is significantly disparate.
Regarding its comparisons to correlational research, while both forms of research study the relationships between variables, causal research goes beyond finding the relationships between variables. It involves uncovering which variable serves as the causal agent (the independent variable) while which is the one being affected (the dependent one).
As such, it involves conducting experiments that manipulate variables in a controlled environment. On the contrary, correlational research does not apply any alterations or conditioning to variables. Instead, it is a purely observational research method.
It detects whether there is a correlation between only 2 variables, whereas causal research can study several at a time. Correlational research can determine that there is no correlation, a positive one or a negative one, yet these relationships do not mean that one variable affects another.
Exploratory research is vastly different from correlational research, as it forms the very foundation of a research problem and establishes a hypothesis for further research. As such, it is conducted as the very first kind of research around a new topic and does not fixate on variables.
Descriptive research, like exploratory research and unlike causal research is conducted early on in the entire research process, following exploratory research. Like exploratory research, it seeks to paint a picture of a problem or phenomenon , as it zeros in an already-established issue and delves further, in pursuit of all the details and conditions surrounding it.
Descriptive research, unlike causal research, only observes; as such, it does not manipulate variables or involve using a control group . It is also not as focused on variables as is causal research; it can be used to inspect and understand various components of research issues.
Experimental research, despite its comparisons with causal research, varies significantly from it. This form of research is propelled by way of a hypothesis, which is used to convey an expected relation between 2 more variables. It implements a scientific research design in which variables are measured, calculated and then compared.
Some research designs employ no manipulation of independent variables and these are used after the effect is uncovered.
While it is primarily concerned with an experiment to confirm a hypothesis, causal research seeks to find whether one variable affects another and how so.
The Advantages and Disadvantages of Causal Research
Causal research offers several benefits for researchers and businesses. However, as with all other research methods, it too carries a few disadvantages that researchers should understand.
The Advantages
- Allows researchers to determine whether the statistical relationship between the two variables found in correlational research has a cause and effect connection
- The ability to delve into more than 2 variables and make predictions.
- Can identify the reasons behind various processes.
- The ability to assess the impacts of changes on existing occurrences, processes, phenomena, etc.
- Can prove or disprove a hypothesis as well as drive the research needed for broadly experimental research.
- Carries the advantages of replication if it is necessary for the studies.
- The systematic selection of subjects gives way to greater levels of internal validation.
- Avoids confusion, as it requires testing all the variables that may be influencing the dependent variables to derive accurate results.
- Finds the causes behind relationships in variables, which attains the gap in correlational research.
- It is considered conclusive research, thus requiring little to no follow-up research or experimentation.
The Disadvantages
- It can fall prey to coincidences, in which similar such relationships and results don’t occur again.
- It takes a while to complete and can be expensive.
- It is difficult to find conclusions due to the impact of a wide scope of factors and variables present in a particular environment.
- Casuality can be inferred, but cannot be proved with 100% certainty.
- It is subject to contamination (influences from variables that are not studied).
How to Conduct Causal Research
Causal research is often the final form of research conducted in the research process and is considered conclusive research. Therefore, it is of utmost importance to plan the research with strict parameters and objectives.
You must clearly lay out what you are trying to prove to avoid bias and dubiousness. Use the findings in exploratory, descriptive and correlational research to enter into the causal research stage.
The objective in this form of research is to unveil the variable that is causing a behavior or phenomenon — the cause, along with the ensuing behavior/phenomenon itself — the effect.
This research reveals which variable is which, along with the direction of the relationship between the variables and their predicted effects.
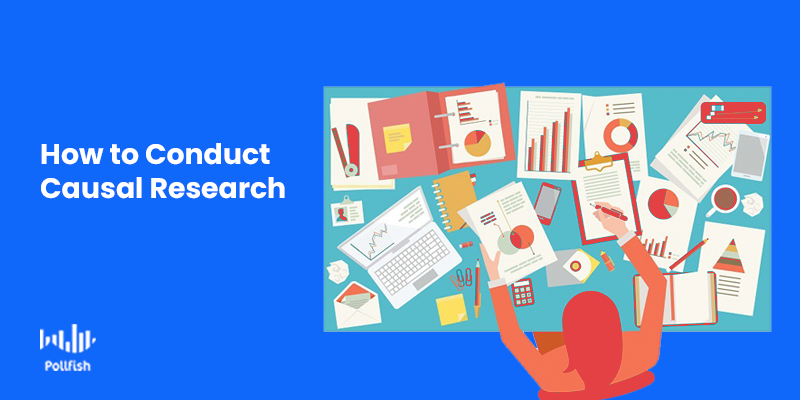
Here are the steps in conducting causal research:
- Find the purpose of the study.
- Ask a question to guide the study.
- Lay down a hypothesis, i.e., the outcome you expect.
- Find the support of the hypothesis.
- Temporal Sequence : The cause must take place before the effect. NOt all correlations will clearly point to this, as the suspected effect may occur before the cause and thereby be unrelated to a cause and effect relationship.
- Concomitant Variation : When the cause changes, there should also be a change in the effect. Ex: If a brand’s ad spend is cut and sales plummet, you can expect the cause behind reduced sales comes from the reduction in ad spending.
- Ending Spurious Correlations : Refers to a common misinterpretation of cause and effect and happens when something thought to be the cause of an effect is actually caused by another variable (unconsidered).
- Conduct the experiment in a controlled setting.
- Manipulate the independent variable(s) to find their effect on the suspected dependent variables.
- Use a control, an independent variable that you don’t manipulate to use as a point of comparison between manipulated and non-manipulated variables.
- Jot down all changes you find within the manipulated and controlled variables, along with the changes in relation to the dependent variables.
- Conduct surveys to add more depth to your research, as they can find certain causes and effects or confirm the findings in your experiment.
Using Causal Research and Going Further
To summarise, causal research allows businesses to understand how current actions and behaviors now will affect it in the future by identifying the cause and effect relationship between variables. This is extremely beneficial for a variety of matters in the business world.
This research can determine the effectiveness of various marketing initiatives, campaigns and collaterals, along with virtually all other changes in a business, such as address change, introducing a new product, offering different promotions and more.
Given the prowess of forming predictions that causal research offers, businesses can use the insights beyond understanding cause and effect. It can form future marketing and advertising strategies, along with testing new ideas that relate or involve the variables from the research.
Although surveys don’t make up the entirety or even the majority of causal research, they can bolster any hypothesis or suspected course of action. As a business, you ought to employ them via a strong online survey platform that allows you to set up numerous surveys and survey campaigns.
Do you want to distribute your survey? Pollfish offers you access to millions of targeted consumers to get survey responses from $0.95 per complete. Launch your survey today.
Privacy Preference Center
Privacy preferences.
- What is Causal Research? Definition + Key Elements

Cause-and-effect relationships happen in all aspects of life, from business to medicine, to marketing, to education, and so much more. They are the invisible threads that connect both our actions and inactions to their outcomes.
Causal research is the type of research that investigates cause-and-effect relationships. It is more comprehensive than descriptive research, which just talks about how things affect each other.
Let’s take a closer look at how you can use informal research to gain insight into your research results and make more informed decisions.

Defining Causal Research
Causal research investigates why one variable (the independent variable) is causing things to change in another ( the dependent variable).
For example, a causal research study about the cause-and-effect relationship between smoking and the prevalence of lung cancer. Smoking prevalence would be the independent variable, while lung cancer prevalence would be the dependent variable.
You would establish that smoking causes lung cancer by modulating the independent variable (smoking) and observing the effects on the dependent variable (lung cancer).
What’s the Difference Between Correlation and Causation
Correlation simply means that two variables are related to each other. But it does not necessarily mean that one variable causes changes in the other.
For example, let’s say there is a correlation between high coffee sales and low ice cream sales. This does not mean that people are not buying ice cream because they prefer coffee.
Both of these variables correlate because they’re influenced by the same factor: cold weather.
The Need for Causal Research

The major reason for investigating causal relationships between variables is better decision-making , which leads to developing effective solutions to complex problems. Here’s a breakdown of how it works:
- Decision-Making
Causal research enables us to figure out how variables relate to each other and how a change in one variable affects another. This helps us make better decisions about resource allocation, problem-solving, and achieving our goals.
In business, for example, customer satisfaction (independent variable) directly impacts sales (dependent variable). If customers are happy with your product or service, they’re more likely to keep returning and recommending it to their friends, which translates into more sales.
- Developing Effective Solutions to Problems
Understanding the causes of a problem, allows you to develop more effective solutions to address it. For example, medical causal research enables you to understand symptoms better, create new prevention strategies, and provide more effective treatment for illnesses.

Examples of Where Causal Relationships Are Critical
Here are a couple of ways you can leverage causal research:
- Policy-making : Causal research informs policy decisions about issues such as education, healthcare, and the environment. Let’s say causal research shows that the availability of junk food in schools directly impacts the prevalence of obesity in teenagers. This would inform the decision to incorporate more healthy food options in schools.
- Marketing strategies : Causal research studies allow you to identify factors that influence customer behavior to develop effective marketing strategies. For example, you can use causal research to reach and attract your target audience with the right content.
- Product development : Causal research enables you to create successful products by understanding users’ pain points and providing products that meet these needs.

Key Elements of Causal Research
Let’s take a deep dive into what it takes to design and conduct a causal study:
- Control and Experimental Groups
In a controlled study, the researchers randomly put people into one of two groups: the control group, who don’t get the treatment, or the experimental group, who do.
Having a control group allows you to compare the effects of the treatment to the effects of no treatment. It enables you to rule out the possibility that any changes in the dependent variable are due to factors other than the treatment.
- Independent variable : The independent variable is the variable that affects the dependent variable. It is the variable that you alter to see the effect on the dependent variable.
- Dependent variable : The dependent variable is the variable that is affected by the independent variable. This is what you measure to see the impact of the independent variable.
An Illustration of How Independent vs Dependent Variable Works in Causal Research
Here’s an illustration to help you understand how to differentiate and use variables in causal research:
Let’s say you want to investigate “ the effect of dieting on weight loss ”, dieting would be the independent variable, and weight loss would be the dependent variable. Next, you would vary the independent variable (dieting) by assigning some participants to a restricted diet and others to a control group.
You will see the cause-and-effect relationship between dieting and weight loss by measuring the dependent variable (weight loss) in both groups.
Skip the setup hassle! Get a head start on your research with our ready-to-use Experimental Research Survey Template
Research Designs for Establishing Causality
There are several ways to investigate the relationship between variables, but here are the most common:
A. Experimental Design
Experimental designs are the gold standard for establishing causality. In an experimental design, the researcher randomly assigns participants to either a control group or an experimental group. The control group does not receive the treatment, while the experimental group does.
Pros of experimental designs :
- Highly rigorous
- Explicitly establishes causality
- Strictly controls for extraneous variables
- Time-consuming and expensive
- Difficult to implement in real-world settings
- Not always ethical
B. Quasi-Experimental Design
A quasi-experimental design attempts to determine the causal relationship without fully randomizing the participant distribution into groups. The primary reason for this is ethical or practical considerations.
Different types of quasi-experimental designs
- Time series design : This design involves collecting data over time on the same group of participants. You see the cause-and-effect relationship by identifying the changes in the dependent variable that coincide with changes in the independent variable.
- Nonequivalent control group design : This design involves comparing an experimental group to a control group that is not randomly assigned. The differences between the two groups explain the cause-and-effect relationship.
- Interrupted time series design : Unlike the time series that measures changes over time, this introduces treatment at a specific point in time. You figure out the relationship between treatment and the dependent variable by looking for any changes that occurred at the time the treatment was introduced.
Pros of quasi-experimental designs
- Cost-effective
- More feasible to implement in real-world settings
- More ethical than experimental designs
- Not as thorough as experimental designs
- May not accurately establish causality
- More susceptible to bias
Establishing Causality without Experiments
Using experiments to determine the cause-and-effect relationship between each dependent variable and the independent variable can be time-consuming and expensive. As a result, the following are cost-effective methods for establishing a causal relationship:
- Longitudinal Studies
Long-term studies are observational studies that follow the same participants or groups over a long period. This way, you can see changes in variables you’re studying over time, and establish a causal relationship between them.
For example, you can use a longitudinal study to determine the effect of a new education program on student performance. You then track students’ academic performance over the years to see if the program improved student performance.
Challenges of Longitudinal Studies
One of the biggest problems of longitudinal studies is confounding variables. These are factors that are related to both the independent variable and the dependent variable.
Confounding variables can make it hard to isolate the cause of an independent variable’s effect. Using the earlier example, if you’re looking at how new educational programs affect student success, you need to make sure you’re controlling for factors such as students’ socio-economic background and their prior academic performance.
- Instrumental Variables (IV) Analysis
Instrumental variable analysis (IV) is a statistical approach that enables you to estimate causal effects in observational studies. An instrumental variable is a variable that is correlated with the independent variable but is not correlated with the dependent variable except through the independent variable.
For example, in academic achievement research, an instrumental variable could be the distance to the nearest college. This variable is correlated with family income but doesn’t correlate with academic achievement except through family income.
Challenges of Instrumental Variables (IV) Analysis
A primary limitation of IV analysis is that it can be challenging to find a good instrumental variable. IV analysis can also be very sensitive to the assumptions of the model.
Challenges and Pitfalls

It is a powerful tool for solving problems, making better decisions, and advancing human knowledge. However, causal research is not without its challenges and pitfalls.
- Confounding Variables
A confounding variable is a variable that correlates with both the independent and dependent variables, and it can make it difficult to isolate the causal effect of the independent variable.
For example, let’s say you are interested in the causal effect of smoking on lung cancer. If you simply compare smokers to nonsmokers, you may find that smokers are more likely to get lung cancer.
However, the relationship between smoking and lung cancer may be confounded by other factors, such as age, socioeconomic status, or exposure to secondhand smoke. These other factors may be responsible for the increased risk of lung cancer in smokers, rather than smoking itself.
Unlock the research secrets that top professionals use: Get the facts you need about Desk Research here
Strategy to Control for Confounding Variables
Confounding variables can lead to misleading results and make it difficult to determine the cause-and-effect between variables. Here are some strategies that allow you to control for confounding variables and improve the reliability of causal research findings:
- Randomized Controlled Trial (RCT)
In an RCT, participants are randomly assigned to either the treatment group or the control group. This ensures that the two groups are comparable on all confounding variables, except for the treatment itself.
- Statistical Methods
Using statistical methods such as multivariate regression analysis allows you to control for multiple confounding variables simultaneously.
Reverse Causation
Reverse Causation is when the relationship between the cause and effect of variables is reversed.
For example, let’s say you want to find a correlation between education and income. You’d expect people with higher levels of education to earn more, right?
Well, what if it’s the other way around? What if people with higher income are only more college-educated because they can afford it and lower-income people can’t?
Strategy to Control for Reverse Causation
Here are some ways to prevent and mitigate the effect of reverse causation:
- Longitudinal study
A longitudinal study follows the same individuals or groups over time. This allows researchers to see how changes in one variable (e.g., education) are associated with changes in another variable (e.g., income) over time.
- Instrumental Variables Analysis
Instrumental variables analysis is a statistical technique that estimates the causal effect of a variable when there is reverse causation.
Real-World Applications
Causal research allows us to identify the root causes of problems and develop solutions that work. Here are some examples of the real-world applications of causal research:
- Healthcare Research:
Causal research enables healthcare professionals to figure out what causes diseases and how to treat them.
For example, medical researchers can use casual research to figure out if a drug or treatment is effective for a specific condition. It also helps determine what causes certain diseases.
Randomized controlled trials (RCTs) are widely regarded as the standard for determining causal relationships in healthcare research. They have been used to determine the effects of multiple medical interventions, such as the effectiveness of new drugs and vaccines, surgery, as well as lifestyle changes on health.
- Public Policy Impact
Causal research can also be used to inform public policy decisions. For example, a causal study showed that early childhood education for disadvantaged children improved their academic performance and reduced their likelihood of dropping out. This has been leveraged to support policies that increase early childhood education access.
You can also use causal research to see if existing policies are working. For example, a causal study proves that giving ex-offenders job training reduces their chances of reoffending. The governments would be motivated to set up, fund, and mandate ex-offenders to take training programs.
Understanding causal effects helps us make informed decisions across different fields such as health, business, lifestyle, public policy, and more. But, this research method has its challenges and limitations.
Using the best practices and strategies in this guide can help you mitigate the limitations of causal research. Start your journey to seamlessly collecting valid data for your research with Formplus .
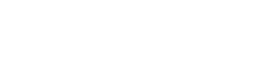
Connect to Formplus, Get Started Now - It's Free!
- casual research
- research design
- Moradeke Owa
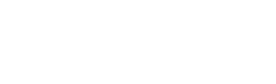
You may also like:
Desk Research: Definition, Types, Application, Pros & Cons
If you are looking for a way to conduct a research study while optimizing your resources, desk research is a great option. Desk research...

43 Market Research Terminologies You Need To Know
Introduction Market research is a process of gathering information to determine the needs, wants, or behaviors of consumers or...
Writing Research Proposals: Tips, Examples & Mistakes
In this article, we’ll discover several tips for writing an effective research proposal and common pitfalls you should look out for.
Projective Techniques In Surveys: Definition, Types & Pros & Cons
Introduction When you’re conducting a survey, you need to find out what people think about things. But how do you get an accurate and...
Formplus - For Seamless Data Collection
Collect data the right way with a versatile data collection tool. try formplus and transform your work productivity today..

Causal Research: The Complete Guide
by Amplitude Marketing | Feb 23, 2023 | Digital Marketing

As we grow up, all humans learn about cause and effect. While it’s not quite as nuanced as causal research, the concept is something our brains begin to comprehend as young as 18 months old. That understanding continues to develop throughout our lives.
![causal research in marketing → Download Now: Market Research Templates [Free Kit]](https://no-cache.hubspot.com/cta/default/53/6ba52ce7-bb69-4b63-965b-4ea21ba905da.png)
In the marketing world, data collection and market research are invaluable. That’s where causal research, the study of cause and effect, comes in.
First-party data can help you learn more about the impact of your marketing campaigns, improve business metrics like customer loyalty, and conduct research on employee productivity. In this guide, we’ll review what causal research is, how it can improve your marketing efforts, and how to conduct your research.
Table of Contents
- What is causal research?
- The Benefits of Causal Research
Causal Research Examples
- How to Conduct Causal Research
Once your team has conducted causal research, your marketers will develop theories on why the relationship developed. Here, your team can study how the variables interact and determine what strategies to apply to future business needs.
Companies can learn how rebranding a product influences sales, how expansion into new markets will affect revenue, and the impact of pricing changes on customer loyalty. Keep in mind that causality is only probable, rather than proven.
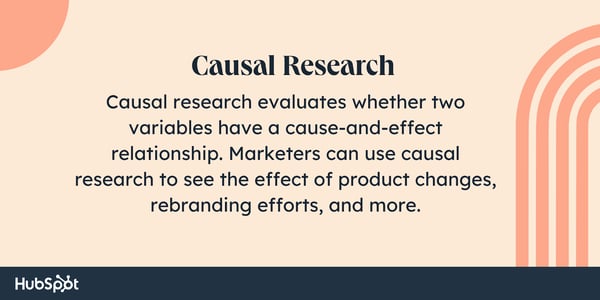

2. Choose your variables.
Once you know what you want to study, you have to choose your variables. You need to know the two variables you’re testing – your dependent and independent variables.
Then, you’ll want to list out other confounding variables that may influence your study. This means identifying variables that might alter your study, including how you collect the data.
Additionally, you’ll need to have control variables set in place so you can compare your results.
3. Pick a random sampling of participants.
Now, it’s time to figure out the sample size of your experiment.
You can use technology to determine who you want your target audience to be and how random the sample should be. You can generate a random list using a database or segment your audience with your marketing software.
4. Set up a controlled experiment.
Ready, set, go. The next step is to actually conduct your experiment.
This could include sending out surveys, conducting interviews, gathering statistics and data, and more. It could also mean setting up an A/B test with your marketing software and changing only one variable in your next marketing campaign, blog post, or webinar.
5. Analyze your findings.
After you’ve conducted your experiment, it’s time to look at the results. Look at the data, and use it to see trends or patterns. Then, you’ll have the answer to your question.
However, it’s important to also analyze different correlations between your two variables to develop a nuanced interpretation. Doing this might help you develop more questions for further research, which is where the next step comes in.
6. Conduct supplemental research and report your findings.
Usually, causal research isn’t a one-and-done process. Once you have your results, you’ll have next steps to go through. You might have more questions that require further research, and if that’s the case you’ll need to conduct supplement research.
Additionally, you might just need to write down what you found. If you have a conclusive result, you can develop in-depth marketing strategies and systems.
I know all of this sounds great, but you might also be wondering how to apply this to your business and marketing team. That’s why we’ll review a few examples of causal research below.
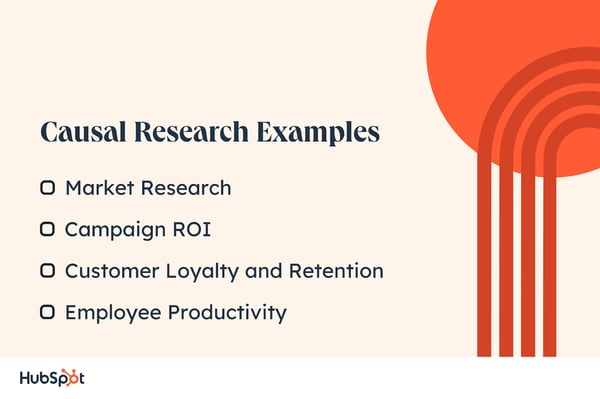
Recent Posts
- How Instagram Reels Helped Me Hit 6 Figures in My First Year in Business
- These AI Tools Upped My Productivity, Here’s How
- The 13 Best Free Graphic Design Software for Marketers and Beginners
- How Eastside Golf Redefines Golf With Contemporary Design and Inclusivity
- Become a Shopify Web Design Pro: How To DIY Your Shopify Store
- February 2024
- January 2024
- December 2023
- November 2023
- October 2023
- September 2023
- August 2023
- February 2023
- January 2023
- December 2022
- November 2022
- October 2022
- September 2022
- August 2022
- February 2022
- January 2022
- December 2021
- August 2021
- Digital Marketing
- Social Media
- Entries feed
- Comments feed
- WordPress.org
Share this post with your friends!
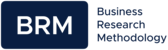
Causal Research (Explanatory research)
Causal research, also known as explanatory research is conducted in order to identify the extent and nature of cause-and-effect relationships. Causal research can be conducted in order to assess impacts of specific changes on existing norms, various processes etc.
Causal studies focus on an analysis of a situation or a specific problem to explain the patterns of relationships between variables. Experiments are the most popular primary data collection methods in studies with causal research design.
The presence of cause cause-and-effect relationships can be confirmed only if specific causal evidence exists. Causal evidence has three important components:
1. Temporal sequence . The cause must occur before the effect. For example, it would not be appropriate to credit the increase in sales to rebranding efforts if the increase had started before the rebranding.
2. Concomitant variation . The variation must be systematic between the two variables. For example, if a company doesn’t change its employee training and development practices, then changes in customer satisfaction cannot be caused by employee training and development.
3. Nonspurious association . Any covarioaton between a cause and an effect must be true and not simply due to other variable. In other words, there should be no a ‘third’ factor that relates to both, cause, as well as, effect.
The table below compares the main characteristics of causal research to exploratory and descriptive research designs: [1]
Amount of uncertainty characterising decision situation | Clearly defined | Highly ambiguous | Partially defined |
Key research statement | Research hypotheses | Research question | Research question |
When conducted? | Later stages of decision making | Early stage of decision making | Later stages of decision making |
Usual research approach | Highly structured | Unstructured | Structured |
Examples | ‘Will consumers buy more products in a blue package?’ ‘Which of two advertising campaigns will be more effective?’ | ‘Our sales are declining for no apparent reason’ ‘What kinds of new products are fast-food consumers interested in?’ | ‘What kind of people patronize our stores compared to our primary competitor?’ ‘What product features are the most important to our customers?’ |
Main characteristics of research designs
Examples of Causal Research (Explanatory Research)
The following are examples of research objectives for causal research design:
- To assess the impacts of foreign direct investment on the levels of economic growth in Taiwan
- To analyse the effects of re-branding initiatives on the levels of customer loyalty
- To identify the nature of impact of work process re-engineering on the levels of employee motivation
Advantages of Causal Research (Explanatory Research)
- Causal studies may play an instrumental role in terms of identifying reasons behind a wide range of processes, as well as, assessing the impacts of changes on existing norms, processes etc.
- Causal studies usually offer the advantages of replication if necessity arises
- This type of studies are associated with greater levels of internal validity due to systematic selection of subjects
Disadvantages of Causal Research (Explanatory Research)
- Coincidences in events may be perceived as cause-and-effect relationships. For example, Punxatawney Phil was able to forecast the duration of winter for five consecutive years, nevertheless, it is just a rodent without intellect and forecasting powers, i.e. it was a coincidence.
- It can be difficult to reach appropriate conclusions on the basis of causal research findings. This is due to the impact of a wide range of factors and variables in social environment. In other words, while casualty can be inferred, it cannot be proved with a high level of certainty.
- It certain cases, while correlation between two variables can be effectively established; identifying which variable is a cause and which one is the impact can be a difficult task to accomplish.
My e-book, The Ultimate Guide to Writing a Dissertation in Business Studies: a step by step assistance contains discussions of theory and application of research designs. The e-book also explains all stages of the research process starting from the selection of the research area to writing personal reflection. Important elements of dissertations such as research philosophy , research approach , methods of data collection , data analysis and sampling are explained in this e-book in simple words.
John Dudovskiy
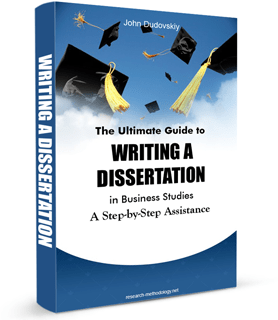
[1] Source: Zikmund, W.G., Babin, J., Carr, J. & Griffin, M. (2012) “Business Research Methods: with Qualtrics Printed Access Card” Cengage Learning

In order to continue enjoying our site, we ask that you confirm your identity as a human. Thank you very much for your cooperation.
Marketing91
Causal Research – Meaning, Explanation, Examples, Components
June 12, 2023 | By Hitesh Bhasin | Filed Under: Marketing
Causal research can be defined as a research method that is used to determine the cause and effect relationship between two variables. This research is used mainly to identify the cause of the given behavior. Using causal research, we decide what variations take place in an independent variable with the change in the dependent variable.
Table of Contents
Meaning and explanation of causal researches
The meaning of causal research is to determine the relationship between a cause and effect. It is also known as explanatory research. A variation in an independent variable is observed, which is assumed to be causing changes in the dependent variable. The changes in the independent variable are measured due to the variation taking place in the dependent variable.
To get the accurate output, other confounding variables that might influence the results are kept constant while creating the data or are controlled using statistical methods. The nature of causal research is very complicated as a researcher can never be sure that no other hidden variables are influencing the causal relationship between two variables. For example, when a company wants to study the behavior of their consumers towards the changing price of their goods, they use causal research.
They might test the behavior of customers depending on different variables. Still, they can never be sure as there can be some hidden variables that might affect the decisions of customers. For instance, no matter how much caution you to take to get the accurate results but there can always be a few psychological considerations that a consumer might be influencing the concerns of the customer even when he is not aware.
The cause and effect relationship between two variables can only be confirmed if causal evidence exists that support the relationship.
The following are the three components for causal evidence
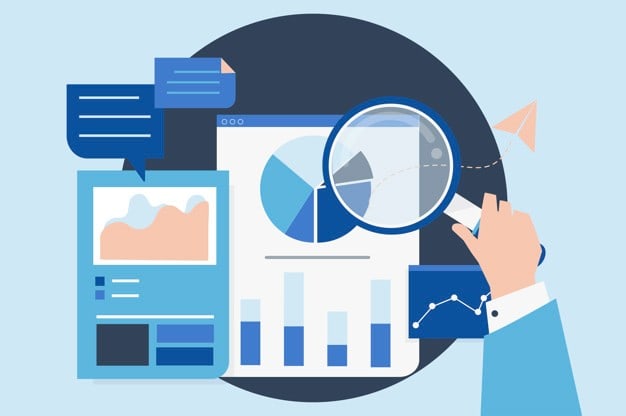
1. Non-Spurious association
The correlated variation between two variables can only be valid if there is no other variable related to both cause and effect.
2. Temporal sequence
A cause and effect can exclusively be connected if the cause has taken place before the occurrence of the effect. For example, it is not right to assume the cause of a dip in sales was the new entrants in the market when sales were already decreasing before the entrance of new entrants.
3. Concomitant variation
Concomitant variation is referred to as the quantitative change occurred in effect is only because of the quantitative change happened in the cause. That means the variation taking place between two variables must be systematic.
For example, if a company does not put effort into increasing sales by hiring skilled employees or by providing training to the employees, then the credit of an increase in sales can’t be given to the recruitment of experienced employees. There will be other causes which caused an increase in sales.
Advantages of causal researches
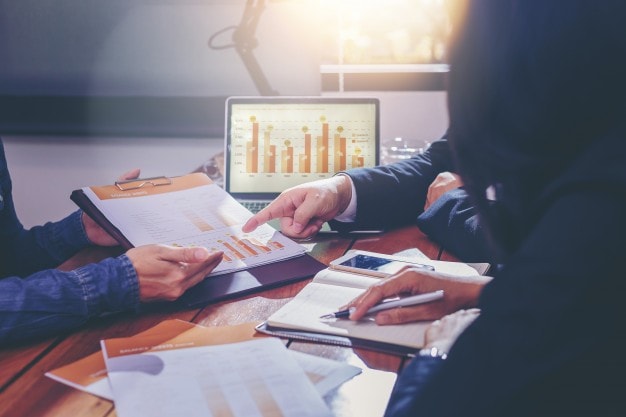
- Causal research helps identify the causes behind processes taking place in the system. Having this knowledge helps the researcher to take necessary actions to fix the problems or to optimize the outcomes.
- Causal research provides the benefits of replication if there is a need for it.
- Causal research helps identify the impacts of changing the processes and existing methods.
- In causal research, the subjects are selected systematically. Because of this, causal research is helpful for higher levels of internal validity.
Disadvantages of causal research
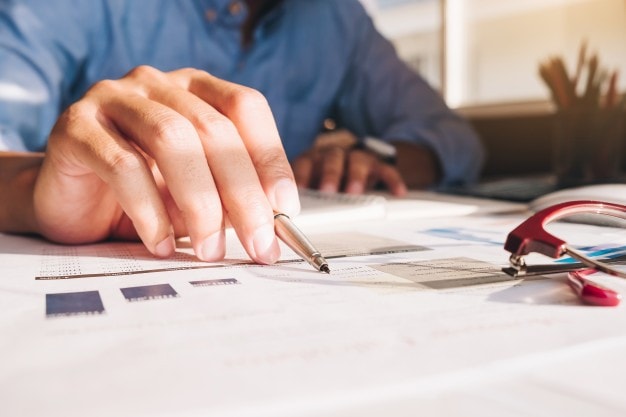
- The causal research is difficult to administer because sometimes it is not possible to control the effects of all extraneous variables.
- Causal research is one of the most expensive research to conduct. The management requires a great deal of money and time to conduct research. Sometimes it costs more than 1 or 2 million dollars to test real-life two advertising campaigns.
- One disadvantage of causal research is that it provides information about your plans to your competitors. For example, they might use the outcomes of your research to identify what you are up to and enter the market before you.
- The findings of causal research are always inaccurate because there will always be a few previous causes or hidden causes that will be affecting the outcome of your research. For example, if you are planning to study the performance of a new advertising campaign in an already established market. Then it is difficult for you to do this as you don’t know the advertising campaign solely influences the performance of your business understudy or it is affected by the previous advertising campaigns .
- The results of your research can be contaminated as there will always be a few people outside your market that might affect the results of your study.
- Another disadvantage of using causal research is that it takes a long time to conduct this research. The accuracy of the causal research is directly proportional to the time you spend on the research as you are required to spend more time to study the long-term effects of a marketing program.
- Coincidence in causal research is the biggest flaw of the research. Sometimes, the coincidence between a cause and an effect can be assumed as a cause and effect relationship.
- You can’t conclude merely depending on the outcomes of the causal research. You are required to conduct other types of research alongside the causal research to confirm its output.
- Sometimes, it is easy for a researcher to identify that two variables are connected, but to determine which variable is the cause and which variable is the effect is challenging for a researcher.
Examples of Causal Research
- To test the market for a new product by collecting data about its sales potential.
- To check the performance or effectiveness of a new advertising campaign to decide whether to continue it or not.
- To measure the improvement in the performance of employees after providing them training on a new skill.
- To examine the effects of re- branding initiatives based on the level of loyalty of customers.
Liked this post? Check out the complete series on Market research
Related posts:
- Research brief: Meaning, Components, Importance & Ways to Prepare
- Free Rider Problem – Explanation, Solutions and Examples
- Mass marketing definition and explanation with examples
- What are the Functions of Wholesalers? Explanation & Examples
- What is Outbound Marketing? Concept, Explanation and Examples
- Qualitative Research: Meaning, and Features of Qualitative Research
- Mass Market – Definition of Mass market and explanation
- What is KISS Principle (Keep it Simple, Stupid)? Explanation & How to Use It
- Advocacy Groups: Definition, Explanation and History
- Sales Agreement – Meaning, Components and Samples
About Hitesh Bhasin
Hitesh Bhasin is the CEO of Marketing91 and has over a decade of experience in the marketing field. He is an accomplished author of thousands of insightful articles, including in-depth analyses of brands and companies. Holding an MBA in Marketing, Hitesh manages several offline ventures, where he applies all the concepts of Marketing that he writes about.
All Knowledge Banks (Hub Pages)
- Marketing Hub
- Management Hub
- Marketing Strategy
- Advertising Hub
- Branding Hub
- Market Research
- Small Business Marketing
- Sales and Selling
- Marketing Careers
- Internet Marketing
- Business Model of Brands
- Marketing Mix of Brands
- Brand Competitors
- Strategy of Brands
- SWOT of Brands
- Customer Management
- Top 10 Lists
Leave a Reply Cancel reply
Your email address will not be published. Required fields are marked *
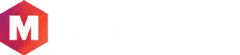
- About Marketing91
- Marketing91 Team
- Privacy Policy
- Cookie Policy
- Terms of Use
- Editorial Policy
WE WRITE ON
- Digital Marketing
- Human Resources
- Operations Management
- Marketing News
- Marketing mix's
- Competitors
Click through the PLOS taxonomy to find articles in your field.
For more information about PLOS Subject Areas, click here .
Loading metrics
Open Access
Peer-reviewed
Research Article
How causal machine learning can leverage marketing strategies: Assessing and improving the performance of a coupon campaign
Roles Data curation, Formal analysis, Writing – original draft
* E-mail: [email protected]
Affiliation University of Helsinki, Faculty of Social Sciences, Economics, Helsinki, Finland

Roles Conceptualization, Writing – review & editing
Affiliation University of Fribourg, Department of Economics, Fribourg, Switzerland
- Henrika Langen,
- Martin Huber
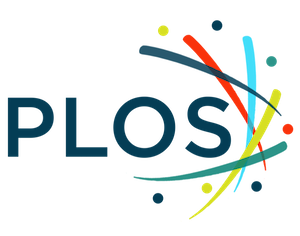
- Published: January 11, 2023
- https://doi.org/10.1371/journal.pone.0278937
- Reader Comments
We apply causal machine learning algorithms to assess the causal effect of a marketing intervention, namely a coupon campaign, on the sales of a retailer. Besides assessing the average impacts of different types of coupons, we also investigate the heterogeneity of causal effects across different subgroups of customers, e.g., between clients with relatively high vs. low prior purchases. Finally, we use optimal policy learning to determine (in a data-driven way) which customer groups should be targeted by the coupon campaign in order to maximize the marketing intervention’s effectiveness in terms of sales. We find that only two out of the five coupon categories examined, namely coupons applicable to the product categories of drugstore items and other food, have a statistically significant positive effect on retailer sales. The assessment of group average treatment effects reveals substantial differences in the impact of coupon provision across customer groups, particularly across customer groups as defined by prior purchases at the store, with drugstore coupons being particularly effective among customers with high prior purchases and other food coupons among customers with low prior purchases. Our study provides a use case for the application of causal machine learning in business analytics to evaluate the causal impact of specific firm policies (like marketing campaigns) for decision support.
Citation: Langen H, Huber M (2023) How causal machine learning can leverage marketing strategies: Assessing and improving the performance of a coupon campaign. PLoS ONE 18(1): e0278937. https://doi.org/10.1371/journal.pone.0278937
Editor: Baogui Xin, Shandong University of Science and Technology, CHINA
Received: August 26, 2022; Accepted: November 23, 2022; Published: January 11, 2023
Copyright: © 2023 Langen, Huber. This is an open access article distributed under the terms of the Creative Commons Attribution License , which permits unrestricted use, distribution, and reproduction in any medium, provided the original author and source are credited.
Data Availability: All data are available from the kaggle database ( https://www.kaggle.com/datasets/vasudeva009/predicting-coupon-redemption ).
Funding: The authors received no specific funding for this work. Open Access funded by Helsinki University Library.
Competing interests: The authors have declared that no competing interests exist.
1 Introduction
Over the last two decades, the amount of customer data available to marketers has increased dramatically with new data types such as social media, clickstream, search query and supermarket scanner data on the rise. The increasing availability of customer Big Data has spawned a new stream of literature on machine learning (ML) methods and tools in the field of business and marketing. The ML literature on designing marketing campaigns ranges from research on modelling customer behavior (e.g., [ 1 , 2 ]), price sensitivity (e.g., [ 3 ]) and purchase decisions (e.g., [ 4 ]) to studies on the development of personalized product recommendation systems (e.g., [ 5 , 6 ]), customer churn management (e.g., [ 7 ]) and acquisition of new customers (e.g., [ 8 ]).
A common feature of these studies is that they are based on predictive ML, i.e., on identifying patterns of variables in the data in order to use them for predicting an outcome of interest (e.g., sales). Predictive ML algorithms use one part of the data to train models that allow predicting the outcome based on patterns found in the data. Then, the other part of the data is used to identify the model with the best performance, where performance is measured by how close the predicted outcomes are to the observed ones. To minimize the prediction error and thereby maximize the predictive power of a model, a predictive ML algorithm trades off bias, i.e., the systematic deviation of the chosen model specification from the true predictive model, and variance, i.e., the sensitivity of the predictions to which data is used for training the model.
Predictive ML models generally do not provide insights about the causal effects of specific variables or interventions (such as a marketing campaign) on the outcome of interest. When multiple variables capture the same relevant predictive feature, i.e., are correlated with that feature, ML algorithms may identify some of these variables as relevant predictors while attaching little importance to others, regardless of the variables’ causal effect on the outcome. For instance, variables that do not directly or only modestly affect the outcome may enter the predictive model as relevant predictors, simply because they are correlated with other variables that actually affect the outcome. For this reason, it may happen that these other variables play little or no role in the predictive model, even though they have a causal impact on the outcome, because they provide little additional information for the prediction. Therefore, predictive ML is generally not suitable for the causal analysis of ‘what if’ questions, such as how a change in a coupon campaign strategy will affect customer behavior, which would be relevant for decision support, e.g., for optimally designing a marketing campaign.
To improve on the shortcomings of predictive ML in evaluating the impact of implementing vs. not implementing a specific intervention, a fast growing literature in econometrics and statistics has been developing so-called causal ML algorithms. In this paper, we demonstrate the application of such methods in the context of business analytics for decision support, namely for evaluating a marketing intervention. More precisely, we make use of the so-called causal forest by Athey et al [ 9 ] to assess the causal effect of coupon campaigns, in which customers were provided coupons for different product types, on customers’ purchasing behavior, i.e., the difference in their expected behavior with and without being targeted by a coupon campaign. While predictive ML algorithms are not able to isolate the causal effects of coupons on customers’ purchasing behavior from the influence of background characteristics (e.g., socio-economic characteristics and price sensitivity) which jointly influence coupon reception and purchasing behavior, the causal forest approach can do so under certain assumptions.
One crucial condition is that all variables that jointly affect coupon reception and purchasing behavior are observed in the data and can thus be controlled for. This condition is known as selection-on-observables or unconfoundedness assumption. Under further conditions on the quality of the ML models used in the causal forest for predicting purchasing behavior and coupon reception as a function of the observed variables, the causal forest approach permits evaluating the average impact of the coupons on all customers, as well as across specific subgroups or customer segments (e.g., different age groups). Our results suggest, for instance, a positive overall effect of coupons for drugstore items. For coupons applicable to ready-to-eat food as well as meat and seafood, on the other hand, we do not find a statistically significant overall effect. An analysis of the effect of drugstore coupons across different customer subgroups reveals that these coupons affect customers with high pre-campaign spending as well as low- to middle-income customers particularly strongly.
Furthermore, we apply optimal policy learning based on ML as proposed by Athey and Wager [ 10 ], in order to learn from the data which customer segments should be optimally targeted by coupon campaigns such that the overall effect is maximized. In contrast to predictive ML, optimal policy learning (under certain conditions) allows determining the coupon strategy which is most effective in terms of its impact on sales. This is obtained by a data-driven customer segmentation in which only those segments with sufficiently high effects are targeted by a coupon campaign. The estimated optimal policy for coupons applicable to meat and seafood, for instance, suggests that such coupons should be issued to the following three target groups: low-income customers whose pre-campaign spending did not exceed a certain level, middle-to-high-income customers aged 46 years or older who purchased something from the store in the period prior to the campaign, and middle-to-high-income households with at least five members who did not purchase anything from the store in the pre-campaign period.
The paper proceeds as follows. Section 2 outlines the current state of quantitative research in the marketing literature. Section 3 motivates the application of causal ML methods in the field of marketing and discusses the contribution of our study to marketing research. Section 4 introduces and describes the retailer sales data to be analyzed. Section 5 defines the causal effects of interest based on so-called counterfactual reasoning and discusses the conditions required for applying causal ML (such as the selection-on-observables assumption). Section 6 describes the algorithms for causal analysis and optimal policy learning. Section 7 provides the results of the evaluation of the coupon campaigns as well as the optimal coupon allocation. Section 8 concludes.
2 Related literature
The impact evaluation of discounts plays a significant role in the earlier marketing literature from the ‘pre-Big-Data era’, see e.g., [ 11 – 14 ] for studies on causal effects of coupon provision. However, the last two decades have seen a surge of predictive ML applications in business analytics, which appear to increasingly dominate causal analysis, also in marketing. In a keyword-search-based literature review, Mariani et al [ 15 ] find that the number of publications on predictive ML and Artificial Intelligence (AI) in marketing, consumer research and psychology has grown exponentially in the past decade (2010–21). The systematic literature reviews by Mustak et al [ 16 ] as well as Ma and Sun [ 17 ] paint a similar picture, with the latter stating that the rise of ML in marketing began with applications of support vector machines, a specific type of ML algorithms. This was then followed by studies introducing text analysis, topic modelling and reinforcement learning into marketing research, as well as by marketing applications of deep learning and network embedding. Questions about the impact of marketing campaigns, the influence of certain external factors on the success of a campaign and the heterogeneity of campaign effects across customer segments appeared to become comparatively less important (see e.g., [ 17 , 18 ]), even though most recently, the marketing literature saw first applications of causal ML alogithms (such as causal trees).
The following sections summarize the current state of research on discount campaigns using (traditional) causal inference (Section 2.1), predictive ML (Section 2.2) and causal ML 2.3.
2.1 Causal inference in marketing
A large number of studies assess the causal effects of marketing campaigns on consumer response. These studies typically rely on (field) experiments or traditional methods for causal inference based on observational data.
Studies on the effectiveness of coupons based on field experiments can be found in the early marketing literature but also more recently. Bawa and Shoemaker [ 19 ] assessed the impact of coupons on sales in a field experiment in which coupons for a particular brand were given to both, retail store customers that had previously purchased the advertised brand and to households that had not. Fong et al [ 20 ] conducted a field experiment in which they randomly distributed mobile tickets with different discount depths among individuals who were in different designated areas at a given time. They evaluated the effects of discount depth and proximity to the advertised movie theater on the use of these coupons. Other examples of field studies include Taylor and Long Tolbert [ 21 ], Heilman et al [ 22 ], Venkatesan and Farris [ 23 ] as well as Gose et al [ 24 ] to name only a few examples.
The field of observational studies on coupons has recently experienced methodological developments towards improved inference methods and a larger awareness of endogeneity issues. Early observational studies on the effects of coupons were frequently based on estimating theoretically derived models of consumer utility considerations, attitudes, and/or behavior using regressions. Sethuraman and Mittelstaedt [ 25 ] use aggregate scanner panel data to estimate the impact of coupons for national brands and private labels on the sales share of private label products, leveraging differences in coupon provision across product categories. Srinivasan et al [ 26 ] evaluate the effect of exposure to coupons on beverage sales among customers who do not redeem coupons by fitting a regression model to aggregate brand sale and coupon redemption data from three retail stores. They find that exposure to coupons may also increase sales among coupon nonusers. Papatla and Krishnamurti [ 27 ] estimate the longer-term effects of coupon promotions on the customers’ loyalty to brands and price sensitivity in the weeks following the coupon campaign. Their study reveals that coupon redemption reduces brand loyalty and increase price sensitivity in the long run. Other regression-based studies on the effect of coupon redemption and/or reception on sales and brand choice include papers by Neslin [ 28 ], Raju et al [ 12 ], Chiang [ 29 ], Dhar and Raju [ 30 ] and Sun [ 31 ].
Rubin and Waterman [ 32 ] apply propensity score matching to evaluate the effect of marketing interventions aimed at physicians in order to promote the prescription of a ‘lifestyle’ drug. They also rank the physicians according to their estimated expected individual-level effects, which in turn can be used to derive a tailored marketing strategy. Li et al [ 33 ] propose an estimator that combines dimensionality reduction and nearest-neighbor matching to estimate the effect of high discount promotions in promotional emails on customers’ response to those emails. Among others, Berning and Zheng [ 34 ], Venkatesan and Farris [ 23 ] as well as Danaher et al [ 35 ] apply instrumental variable approaches to investigate exposure and/or redemption effects of coupon campaigns by isolating exogenous variation in coupon provision or redemption.
Dafny et al [ 36 ] assess the effect of co-payment coupons, i.e., coupons issued by pharmaceutical companies that reduce insured patients’ out-of-pocket costs for promoted drugs, on the patients’ decision between brand-name drugs and bioequivalent generics by means of difference-in-differences as well as triple-difference models. They find that co-payment coupons significantly increase sales of branded products while reducing the sales of bioequivalent generics. Reimers and Xie [ 37 ] assess the effect of e-coupon provision on alcohol sales by means of a difference-in-differences approach, exploiting the fact that the restaurants in their sample issued e-coupons at different points in time. They find that the provision of e-coupons increases demand for alcoholic beverages both during and after the promotion period. Guan et al [ 38 ] use a difference-in-differences approach to evaluate whether providing coupons to retail stores leads to changes in food purchases from before to after the campaign, and find that providing coupons significantly increases food purchases, especially purchases of ready-to-eat foods. Other studies analyze the effect of a farmers’ market coupon initiative on fruit and vegetable purchases in low-income neighbourhoods [ 39 ], that of coupons and other social media marketing tools on product sales [ 40 ], and that of coupons distributed by a hair salon on customer spending per visit, frequency of visits, and attrition [ 41 ].
Another domain of the marketing literature investigates the heterogeneity of marketing effects across customer characteristics and the circumstances under which customers are targeted by coupon and other promotional campaigns. Among them, Gopalakrishnan and Park [ 42 ] analyse whether high- and low-consumption customers, as defined by their purchasing behavior during the 12 months prior to the experiment, differ in their responsiveness to coupon campaigns. Andrews et al [ 43 ] study whether the level of occupancy (or crowdedness) of a subway affects passengers’ response to mobile advertising campaigns and find a statistically significant positive association. Based on a field experiment, Spiekerman et al [ 44 ] conclude that proximity to the location for which coupons are distributed influences coupon redemption, and that this association is much more pronounced in the city center than in suburban areas.
Furthermore, several studies evaluate how certain configurations of coupons, such as face value, distribution method and expiry date, affect consumer behavior. The experimental studies by Zheng et al [ 45 ] as well as Biswase et al [ 46 ] assess how the size of discounts affects consumers’ perceptions of product quality and purchase intentions. Leone and Srinivasan [ 13 ] use supermarket scanner data to analyze the effect of coupon face value on sales and profits, while Anderson and Simester [ 47 ] study the long-term effects of discount size on the purchasing behavior of new and established customers in an experimental setting. Other contributions as e.g., [ 11 , 14 , 42 , 48 , 49 ] analyze how further aspects of coupon and discount campaign design affect consumer behavior.
2.2 Predictive ML in marketing
In recent years, many studies have focused on ML-based prediction of coupon redemption and associated sales. They use ML algorithms to model customer behavior as a function of customers’ previous transactions, their response to past coupon/discount campaigns and their socio-economic characteristics in order to predict the likelihood of customers to redeem coupons or take up discounts and make purchases.
Pusztová and Babič [ 50 ] as well as He and Jiang [ 51 ] compare the performance of different ML-based classification algorithms in predicting coupon redemption in digital marketing campaigns. The former study concludes that so-called Support Vector Machines provide the most accurate predictions, while the latter study finds that the gradient boosting framework ‘XGBoost’ performs best. Greenstein et al [ 52 ] introduce an algorithm that combines co-clustering and random forest classification to predict redemption of mobile restaurant coupons based on demographic and contextual variables such as the consumer’s distance to the restaurant relative to the size of the coupon discount. Ren et al [ 53 ] develop a two-stage model for estimating the probability of coupon redemption. In the first stage, customers are clustered based on their past purchase and redemption behavior, while the second stage consists of fitting prediction models for the different customer clusters. Furthermore, several studies such as [ 45 , 54 , 55 ] predict customer behavior in the context of coupon or other discount campaigns by means of several ML methods.
2.3 Causal ML in marketing
The rise of predictive ML has prompted e.g., Anderson [ 56 ], Lycett [ 57 ] and Erevellese et al [ 58 ] to argue that theory-based causal inference has lost some of its relevance for business decisions in the light of the large data sets and sophisticated predictive ML methods available to marketers today. However, these views were soon challenged in several studies that emphasize the importance of causal reasoning and risks of basing decisions solely on correlations, see e.g., [ 59 , 60 ]. More recently, a growing number of contributions have stressed the importance of integrating ML and causal inference, see e.g., [ 18 ]. Among them is Hunermund et al [ 61 ], who investigate the use of causal methods in business analytics by combining qualitative interviews and quantitative surveys among data scientists and managers in a mixed-methods research design. They document an ongoing shift in corporate decision making away from an exclusive focus on predictive ML and towards the use of causal methods, based on both observational and experimental data.
Recently, the use of causal ML in marketing research appears to be increasing. To the best of our knowledge, however, there are virtually no studies that evaluate the causal effect of coupon campaigns on customer behavior using causal ML, as we do in this paper. Smith et al [ 62 ] use predictive ML for deriving optimal coupon targeting strategies and estimate the profits that would accrue under those strategies out of sample, i.e., in parts of the data not used for deriving the strategies. The estimations make reference to the so-called potential outcomes framework for defining causal effects when applying causal inference or causal ML. However, the study by Smith et al [ 62 ] is conceptually different from ours in that it uses the potential outcomes framework to compare coupon targeting strategies inferred from different predictive ML algorithms, while we apply a causal ML algorithm, namely the optimal policy learning approach of Athey and Wager [ 10 ], to derive the optimal coupon targeting strategy.
One study in the field of marketing which does consider causal ML is [ 63 ]. They assess the performance of so-called Double ML, see [ 64 ], and propensity score matching, see [ 65 ], for estimating the causal effect of conversion ads on Facebook. Such ads aim to increase online activity like page visits, sales and views on an external website. For their analysis, the authors take advantage of the fact that Facebook offers businesses the opportunity to assess their ad campaigns by means of randomized experiments. Gordon et al [ 63 ] compare the effect estimates based on Double ML and propensity score matching with those from the experiments, finding that Double ML outperforms propensity score matching, but that both approaches overestimate the effect substantially. This highlights the importance of observing and appropriately controlling for all factors jointly affecting the intervention and customer behavior when causally assessing marketing interventions. Also Huber et al [ 66 ] consider Double ML when analyzing observational data to investigate whether discounted tickets induce Swiss railway customers to reschedule their journeys, e.g., to shift demand away from peak hours.
Narang et al [ 67 ] apply causal forests, the causal ML framework developed by Wager and Athey [ 68 ] as well as Athey et al [ 9 ] also used in this study (see Section 6), to assess the heterogeneity across shoppers in how mobile app failures affect the frequency, volume, and monetary value of their purchases. Guo et al [ 69 ] assess the effect of a law requiring pharmaceutical firms to disclose their marketing payments to physicians on the firms’ payments to physicians using a Difference-in-Differences approach and assess expected individual-level effect heterogeneity by means of causal forests. Zhang and Luo [ 70 ] incorporate causal forests in their study on modelling restaurant survival as a function of photos posted on social networks. They find that the total volume of user-generated content and the extent to which user photos are rated as helpful have a significant positive effect on the likelihood of restaurant survival. Another study from the domain of marketing that uses causal ML is Cagala et al [ 71 ]. The authors estimate the optimal strategy for distributing gifts among potential donors in a fundraising campaign that maximizes expected net donations. They find that the identified optimal targeting rule outperforms different non-targeted gift distribution rules, even when the optimal targeting rule is estimated based only on publicly available geographic information or on data from a previous fundraising campaign conducted in a similar sample.
In addition to the causal ML applications outlined here, there is another strand of literature that aims to explain AI-generated decisions, namely eXplainable Artificial Intelligence (XAI). The field of XAI encompasses artificial intelligence (AI) methods that generate explanations for decisions made by AI in order to make them understandable to humans. The standard approach is to generate business decisions for the problem at hand using AI and to create a second, but interpretable model that approximates the original “black-box” model which produced the decision (see, for example, [ 72 ]). XAI applications in the field of marketing include studies by Haag et al [ 73 ] and Marín Díaz [ 74 ].
3 Context and contribution
In the following, we will use coupon promotions as a running example to highlight the merits of causal ML in business analytics and marketing research. In the context of coupon campaigning strategies, marketers are arguably interested not only in predicting customer behavior, but also in measuring the causal effects of alternative campaigns on customer behavior. Such effects correspond to the difference in the customers’ (average) behavior when being vs. not being addressed by a particular campaign. Intuitively, this requires comparing a customer’s observed behavior under the actual assigned coupon with the potential (and not directly observed) behavior that would have occurred had coupon provision been different from that actually observed, an approach commonly referred to as counterfactual reasoning. Such a causal assessment is necessary for determining whether and to which extent a campaign is effective in altering customer behavior and for understanding how customer behavior would change if coupons were distributed differently.
Predictive ML models, however, do not inform about causal effects of campaigns. In a predictive ML model, the predictive power of coupon provision on customer behavior generally does not correspond to such a causal effect, because it is affected by so-called regularization bias. The latter arises because ML algorithms generally shrink the importance of (some or all) predictors in order to reduce the variance of prediction, ideally to improve predictive performance. Regularization bias may occur, for instance, when coupon provision is strongly correlated with other (good) predictors (such as previous purchases) and/or when its effect on consumer behavior is comparably small, so that coupon provision has little predictive value. A further issue is selection bias, meaning that coupons may pick up the effects of other variables whose importance has been shrunk by the ML algorithm. The implementation of coupon campaigns should be based on estimations of causal effects that avoid regularization and selection bias, as is the case with causal ML algorithms such as DML and causal forests, if certain conditions hold.
The importance of estimating the causal effect of coupon campaigns in the context of decision support, rather than merely predicting customer behavior, can be illustrated by means of a simple example. Suppose a retailer estimates a model to predict sales based on observational data from a previous coupon campaign in which (in an attempt to re-activate dormant customers) coupons were distributed primarily among customers who had not been in the store for a while, rather than among frequent shoppers. The estimated predictive model might indicate a negative association between coupon provision and sales, since the coupon campaign is likely to re-activate only some inactive customers, so that the (formerly) inactive customers on average purchase less than the frequent shoppers. The true effect of receiving a coupon, however, might actually be positive. A positive effect implies that when comparing two groups of (formerly) inactive customers with comparable background characteristics (like willingness to buy), where the first receives coupons while the second does not, the average purchases of the first group are higher. The predictive model therefore confuses (or confounds) the causal effect of the coupon campaign with that of being a dormant vs. a frequent shopper, thus incorrectly pointing to a negative effect.
In a second scenario, the retailer decides to issue coupons in the store. This way, frequent shoppers are regularly provided with coupons, while dormant customers rarely if ever receive any. A predictive model now detects a positive relationship between the provision of coupons and sales, although the actual effect of providing coupons could be negative, namely if frequent customers use the coupons for products they would have bought anyway. If the campaigns were evaluated using predictive methods and the results were misinterpreted as causal, marketers would come to the conclusion that the first campaign was ineffective while the second was effective. Causal methods, on the other hand, enable marketers under certain conditions to control for such biases (e.g., due to differences in purchasing behavior between frequent and dormant customers) and to consistently estimate the effect of coupon campaigns. Further, these methods can also be applied to assess effect heterogeneity and identify an optimal coupon distribution scheme (or policy) that targets those customers whose average purchases are sufficiently responsive to receiving a coupon.
In causal studies on discounts, the impact of providing coupons is typically assessed either based on random experiments or observational data from previous campaigns when controlling for observed characteristics (or covariates) that are likely to be associated with both coupon provision and consumer behavior. Traditional, non-ML-based causal inference methods require the researcher or analyst to manually select covariates based on theoretical considerations, domain knowledge, intuition and/or previous empirical findings. Examples for such covariates in the context of campaign evaluations include past purchasing behavior, exposure to previous campaigns, and socio-economic characteristics such as age, gender, or income. Manual selection of covariates entails the risk of omitting important control variables and may even be practically infeasible in Big Data contexts with a very large set of potential covariates (e.g., collected from online platforms), including unstructured data containing, e.g., text or clickstreams. Furthermore, conventional causal inference methods require the researcher to specify how, i.e., through which functional form (like, e.g., a linear model), the selected covariates are associated with coupon provision and purchasing behavior.
Causal ML methods, in contrast, permit taking advantage of the full amount of information in the data to detect relevant covariates (which have an important influence on coupon provision and consumer behavior) in a data-driven way and to control for them, as well as to flexibly estimate the functional form of statistical associations. Still, the observational data have to meet certain conditions, as described in Section 5.2.
We assess the causal effect of receiving coupons on customer spending by means of the causal forest, a causal ML method developed by Wager and Athey [ 68 ] that draws on the ML technique of random forests. Just as the random forest algorithm, the causal forest approach draws random subsamples from the data and then creates decision trees in each subsample using a random set of observable variables. To grow these trees, the observations are divided into two subgroups at different values of the variables under consideration, and the algorithm selects the split that fulfils a given splitting criterion. The same is repeated in each of the resulting subsamples, which are commonly referred to as nodes, until a pre-defined stopping rule is met. Finally, the algorithm averages over all trees.
Causal and random forests differ with regard to the splitting criteria they employ to split a sample based on the observed variables, while both methods rely on averaging the respective splitting approach over many samples, which are repeatedly drawn from the original data. The random forest algorithm aims to learn a model that can predict an outcome based on covariates. It therefore splits the covariate space such that the residuals between the observed outcomes and the mean outcomes within subgroups resulting from that split is minimized. Thus, the mean outcome in a subgroup serves as prediction for the outcome of all observations in that subgroup (or node). The causal forest approach, on the other hand, aims at determining splitting rules that maximize the heterogeneity of estimated treatment effects between nodes. Therefore, the algorithm focusses on the conditional average treatment effect (CATE) rather than on the conditional average outcome as splitting criterion and chooses the splitting rule such that the estimated effects differ most between the resulting nodes. The CATE estimates ultimately provided by the causal forest (by averaging over many samples) are also known as individualized effect estimates, as they are a function of an individual’s covariates. This permits assessing the effect heterogeneity of coupon provision across customer characteristics and campaign periods.
Like other causal ML methods, the causal forest approach combines effect estimation based on so-called Neyman [ 75 ]-orthogonal scores with sample splitting for the estimation of the CATEs in nodes defined upon the covariates. Put simply, methods based on orthogonal scores first predict treatment and outcome models as functions of the covariates and then use these models to ‘purge’ the influence of the covariates from the treatment and the outcome before estimating treatment effects. In this process, the treatment and outcome models, which are often referred to as plug-in parameters for the effect estimation to follow, can be learnt by ML algorithms. The purpose of purging any influence of the covariates from both the treatment and the outcome (a process called orthogonalization) is to make effect estimation more robust to approximation errors in the prediction of either plug-in parameter. Such approximation errors may arise from ML-related regularization biases, i.e., from neglecting less predictive covariates of the treatment and/or outcome in the estimation of the respective plug-in parameters.
Sample splitting, on the other hand, aims to avoid overfitting in the estimation of CATEs, i.e., fitting the effect heterogeneity model too strongly to the particularities of the estimation sample, such that the causal forest picks up not only the actual differences of causal effects across covariates, but also random noise. In order to prevent such overfitting bias, the random sample used for learning a causal tree is itself randomly split into two subsamples, one for building the tree by following the procedure mentioned above, while the other one is used for estimating the treatment effect in every node of the learnt causal tree. That is, by following the splitting rules learnt in the first subsample, the algorithm populates the nodes of the estimated tree with the observations from the second subsample and calculates the CATE in each node based on the observations that fall into the respective node. Trees that are estimated based on this sample splitting procedure are commonly referred to as ‘honest’ trees (because they avoid overfitting) (see Section 6.1 for a more technical discussion of the causal forest algorithm as implemented in our application).
The argument for counterfactual reasoning further above also applies to the objective of optimizing the distribution of coupons across customer segments, i.e., optimal policy learning, as discussed in [ 76 – 79 ]. Basing optimization on predictive ML models, as advocated in several studies on predicting coupon redemption (e.g., [ 52 – 54 ]), ignores the fact that predictive models do generally not provide information on causal effects and their heterogeneity across different customer segments. Optimal policy learning as suggested by Athey and Wager [ 10 ], on the other hand, is a causal ML approach for determining treatment rules which ensures that those customers for whom the largest effects can be expected are targeted by the campaign. In Section 6.4, we demonstrate the use optimal policy learning for detecting customer segments that should or should not be targeted by coupon campaigns in order to maximize the effectiveness of these campaigns.
In our empirical application, we analyze sales data on coupon campaigns of a retailer provided as part of the AmExpert 2019—Machine Learning Hackathon [ 80 ]. The data set contains information on socio-economic characteristics of retail store customers, coupons received during the campaigns as well as coupon redemption and purchasing behavior. The retail store ran several campaigns issuing coupons with discounts for certain products, with some coupons being applicable to individual products only and others to a range of products. In each of the 18 partially time-overlapping campaigns falling into the time span covered by the data set, the store distributed 1 to 208 different coupon types each applicable to up to 12,000 products, most of which belong to the same product category. The coupons were distributed in such a way that each customer received 0 to 37 different coupons per campaign with the composition of this set of coupons varying between the recipients. Apart from the information on provided coupons, the data set contains details on all purchases made by each registered customer between January 2012 and July 2013, including the date of the transaction, the redeemed coupons, the product type of each purchased product and the price paid.
For our analysis, we group the coupons into five broad categories mirroring the products they can be used for. More concisely, we distinguish between coupons applicable for ready-to-eat food items, meat and seafood, other food, drugstore items and other non-food products. One could arguably also be interested in more fine-grained coupon categories or in a paricular coupon or discount type rather than in our broader coupon categories, which would, however, require a larger data set to obtain satisfactory statistical power. Due to the temporal overlap of campaign periods, we need to redefine them such that each of the resulting artificially generated campaign periods coincides with the validity period of a given set of coupons. That is, all coupons which are valid in some artificial campaign period are valid during the entire period. By doing so, we can fully attribute changes in purchasing behavior from one artificial campaign period to another to the coupons valid in the respective periods. From now on, the 33 newly defined artificial campaign periods will simply be referred to as campaign periods. To account for differences in the duration of campaign periods, we consider the average per-day expenditures per customer and campaign period as our outcome of interest. For estimating the causal effect of coupon provision on the buying behavior, we pool the customer-specific purchases across campaign periods, yielding 33 observations per customer.
Table 1 provides some descriptive statistics for our data, namely on observed customer characteristics, the share of coupons redeemed and daily in-store spending (descriptive statistics on the composition of daily expenditures by product type are provided in S1 Table ). The table reports the mean of these variables in the total sample of 50,624 observations, as well as among observations that received a coupon and among those that did not. Further, it contains the mean difference in these variables between coupon receivers and non-receivers, as well as the p-value of a two-sample t-test. In some 30% of the observations, customers received at least one coupon. Furthermore, customers who received a coupon had on average higher expenditures in the retail store than customers who did not, suggesting that the retailer did not target its previous campaigns to re-activate dormant customers.
- PPT PowerPoint slide
- PNG larger image
- TIFF original image
https://doi.org/10.1371/journal.pone.0278937.t001
We also see that the retailer does not have information on the socio-economic characteristics of all customers in the registry, but only for about half of them, as the corresponding variable values are unknown for many observations (see the coding ‘unknown’). Such a high rate of non-response in variables can entail selection bias when estimating the effects of interest. For this reason, we will conduct several robustness checks in the empirical analysis to follow further below (see Section 7.5). The descriptive statistics also reveal that some socio-economic characteristics as well as their observability are correlated with the reception of coupons. For example, customers aged 70 years or older are less likely to be targeted by a coupon campaign. The main difference in the likelihood of receiving a coupon seems to be between customers whose socioeconomic characteristics are not available and those whose characteristics are known, with the former less likely to receive a coupon.
As is noted in several studies (e.g., [ 35 , 44 ]) coupon redemption rates are typically low, not exceeding 1 to 3% on average. This is also the case in our data, as only in 3% of the observations of coupon recipients did they actually redeem a coupon. However, as mentioned further above, coupons may not only influence customer behavior when redeemed, but may also serve as an advertising tool which attracts customers to the store even without them redeeming the coupon.
5 Identification
5.1 definition of causal effects.
We are interested in estimating the causal effect of a specific intervention, commonly referred to as ‘treatment’ in causal analysis and henceforth denoted by D , on an outcome of interest, denoted by Y (throughout this paper, capital letters denote random variables and small letters specific values of random variables). In our context, D reflects the reception or non-reception of coupons and Y the purchasing behavior, measured as the average per-day expenditures during the coupon validity period. In the simplest treatment definition, D is binary and takes the value 1 when the respective customer is provided with a coupon and 0 if this is not the case. Mathematically speaking, the value d which treatment D can take satisfies d ∈ {0, 1}. The set of observations with d = 1 is commonly referred to as the treatment group, those for which d = 0 are called control group. Our subsequent discussion of causal effects and the statistical assumptions required for their measurement will focus on this binary treatment case for the sake of simplicity. However, our empirical analysis will also separately consider the effects of receiving coupons for five product categories, by running separate estimations for the comparison of each category to not receiving any coupons. This implies that the assumptions introduced in Section 5.2 need to hold for each of these categories. For discussions of multi-valued treatments, see e.g., [ 81 , 82 ].
For defining the causal effect of coupon provision, we rely on the potential outcome framework pioneered by Neyman [ 83 ] and Rubin [ 84 ]. Let Y ( d ) denote the potential (rather than observed) outcome under a specific treatment value d ∈ {0, 1}. That is, Y (1) corresponds to a customer’s potential purchasing behavior if she received a coupon, while Y (0) is the behavior without a coupon. The causal effect of the coupon thus corresponds to the difference in the purchasing behavior with and without coupon, Y (1) − Y (0), but can unfortunately not be directly assessed for any customer. This is due to the impossibility of observing customers at the same point in time under two mutually exclusive coupon assignments (1 vs. 0), which is known as the ‘fundamental problem of causal inference’, see [ 85 ]. This follows from the fact that the outcome Y which is observed in the data corresponds to the potential purchasing behavior under the coupon assignment actually received, namely Y = Y (1) for those receiving a coupon ( d = 1), and Y (0) = Y for those who do not ( d = 0). For coupon recipients, however, Y (0) cannot be observed in the data, while for customers without a coupon Y (1) remains unknown.
5.2 Discussion of identifying assumptions
In order to identify the ATE defined in the previous section, we need to impose several identifying assumptions, which are outlined in this section. We note that in the subsequent discussion, ‘⊥’ stands for statistical independence. Further, X denotes the set of covariates that should not be affected by treatment D and therefore be observed before or at, but not after, treatment.
Assumption 1 (conditional independence of the treatment) :
Y ( d )⊥ D | X for all d ∈ {0, 1}.
Assumption 1 states that the treatment is conditionally independent of the outcome when controlling for the covariates, and is known as ‘selection on observables’, ‘unconfoundedness’ or ‘ignorable treatment assignment’, see e.g., [ 65 ]. The assumption implies that there are no unobservables jointly affecting the treatment assignment and the outcomes conditional on the covariates. This condition is satisfied if the coupons are quasi-randomly distributed among observations with the same values in X . The retailer may therefore base the distributing of coupons on customer or market characteristics observed in the data, however, not on unobserved characteristics that affect purchasing behavior even after controlling for the observed ones.
We control for the variables in Table 1 , period fixed effects, the customers’ average daily pre-campaign spending by product category, as well as for the coupons she received and redeemed in the period prior to the campaign. When evaluating the effect of specific coupon categories, we also include dummies that indicate whether a customer received coupons from another category at the moment of treatment assignment. This is because the availability of other coupons influences purchase behavior and is likely to be correlated with the probability of receiving coupons of the category under study. The reason for including period fixed effects is that there is no information available on holidays or weekdays on which the store is closed or has shortened opening hours, that is, circumstances that may affect purchasing behavior. Also, the retailer is likely to distribute coupons differently across campaign periods. Including pre-campaign expenditures allows controlling for general differences in purchasing behavior between customers that might be correlated with the likelihood of receiving coupons, since the retailer presumably bases decisions about whom to allocate which coupon(s) on past purchasing behavior.
The covariates considered in our estimation are similar to those included in studies on the effect of coupon campaigns that rely on traditional causal inference approaches, see, e.g., [ 86 , 87 ]. In both studies, the covariates contain demographic characteristics as well as a proxy for the customers’ economic situation and their purchasing behavior prior to the coupon campaign. Unlike the methods used in these studies, however, the causal ML approach applied here permits controlling for covariates in a flexible, possibly non-linear way, and does not require pre-selecting variables. Studies on predicting coupon redemption by ML frequently rely exclusively on customer behavior and coupon characteristics as predictors of coupon redemption, while not including socio-demographic characteristics of customers, see e.g., [ 52 ] use and [ 51 ].
In their study on the performance of causal ML in evaluating Facebook ads, Gordon et al [ 63 ] include users’ gender, age and household size but—unlike our data—their data lack information on users’ economic situation, such as their income, employment status, or wealth. They also use several Facebook-specific covariates measuring users’ activity on Facebook (likes, posts, type of device used and interests explicitely expressed on Facebook). Furthermore, they take into account users’ response to earlier ads from other companies, which is comparable to the covariates on pre-campaign purchasing behavior, coupon reception and coupon redemption considered in our analysis.
Despite the large differences in the amount of information available in the Facebook study and our analysis, we cannot automatically conclude that the set of covariates in our analysis is insufficient for satisfying Assumption 1. In fact, the algorithms used by Facebook to determine the target audience for ad placement might be more complex and information-hungry than a retailer’s coupon strategy. In order to properly assess the causal effect of Facebook ads, any of this information incorporated in Facebook’s ad placement algorithms that also drives the outcome of interest, namely customer response, would need to be controlled for. Likewise, our evaluation needs to control for outcome-relevant information based on which the retailer determined the coupon assignment. If the retailer based the distribution of coupons systematically on covariates observed in its customer database (but not on other factors), then Assumption 1 holds in our context.
Assumption 2 (common support) :
0 < Pr ( D = 1| X )<1.
Assumption 2 states that the conditional probability of being treated given X , in the following referred to as the treatment propensity score, is larger than zero and smaller than one. This so-called common support condition implies that for all values the covariates might take, customers have a non-zero chance of being treated and a non-zero chance of not being treated. While this assumption is imposed w.r.t. to the total of a (large) population, meaning that both treated and non-treated customers exist conditional on X , we can and should also verify it in the data. In our sample, common support appears to be satisfied, as there exist no combinations of covariate values for which either only customers with coupons (of a certain category) or no coupons exist. S1 – S3 Figs show the distribution of the estimated propensity scores for receiving coupons (of a particular type) among recipients and non-recipients of that particular coupon(s). The distributions overlap (although the overlap is partially thin), i.e., for each observation in one group, observations can be found in the other group that are comparable with respect to the propensity score.
Another condition that needs to be satisfied is the so-called Stable Unit Treatment Value Assumption (SUTVA), see e.g., [ 88 ]. In our context, SUTVA rules out that the coupons provided to one individual affect the potential outcome of another individual. The assumption that there are no inter-personal spillover effects of coupon campaigns may be problematic in our setting. Customers receiving coupons may induce their peers to make purchases by, for instance, telling peers about the products they bought when redeeming the coupon or by visiting the store together with peers. On the other hand, customers with coupons may also redeem their coupons to buy the coupon-discounted products not only for themselves but also for their peers, thereby reducing the purchases made by their peers. Such scenarios appear particularly likely when there are several members of the same household in the customer base. There is ongoing research on how to deal with such SUTVA violations under certain assumptions like the observability of groups affected by spillovers, see e.g., [ 89 – 95 ]. However, in our data set, the relationships between customers are not observable, meaning the data does not allow accounting for possible spillovers of providing coupons to one customer on the outcomes of other customers. If such spillovers existed in our case, they could entail an under- or overestimation of the effect of coupons on purchasing behavior, depending on whether the spillovers occur primarily through treated customers inducing non-treated peers to make purchases or through treated customers redeeming coupons to purchase products for their peers, with the former entailing an overestimation of the outcome under non-treatment and the latter leading to an underestimation.
SUTVA also requires that for every individual in the population, there is a single potential outcome value associated with each treatment state, meaning that there are no different versions of the coupons leading to different potential outcomes. In many empirical applications, it appears likely that at least some aspects of SUTVA are violated, and for this reason, there exist several relaxations of this assumption. In our case, the requirement that there be no different treatment versions is particularly problematic given that we group different coupons into broader categories. The treatment of being provided with coupon(s) from one category comprises the receipt of different coupons, each applicable to a distinct set of products from the respective product category. If a customer is not equally interested in all products belonging to that product category, the customer may only redeem a coupon and/or change her purchasing behavior if the coupon is applicable to certain products. For this reason, we are in a setting where there are different treatment versions, each possibly associated with a different potential outcome.
VanderWeele and Hernan [ 96 ] relax the original SUTVA by allowing for the existence of different unobservable versions of the treatment as long as there are no different versions of non-treatment and the treatment versions are assigned randomly conditional on the covariates X . This permits assessing the average effects of certain bundles of coupons (rather than specific coupons as under the original SUTVA) vs. not receiving any coupons. Indeed, the assumption that there is only one version of non-treatment is satisfied in our analysis of the effect of receiving some vs. no coupons, under the assumption that the marketer has not run any undocumented discount campaigns during the study period. Furthermore, when assessing the effects of coupons applicable to specific product categories, we control for all other coupons that each customer received at treatment assignment, which in turn creates non-treatment states that are necessarily equal after controlling for other coupons. Table 1 and S2 Table show that the coupons were distributed under consideration of the covariates in the customer registry. We must now assume that the propensity of receiving a coupon (version) differs only depending on observed characteristics, but not on characteristics that are not available to us. This issue can be easily circumvent in practice as long as the information on customers available to marketing campaign planners is also available to those evaluating the campaign.
5.3 Inter-temporal spillover effects
While our main analysis focusses on the (short-term) effect of coupon provision on purchasing behavior during the validity period of the coupon (rather than longer-term coupon-induced behavioral shifts), our framework does not rule out the existence of inter-temporal spillover effects on customers’ purchasing behavior. By including pre-campaign coupon reception and redemption as control variables, we aim at controlling for the fact that previous coupons may influence customer behavior in the current outcome period. As a further inter-temporal effect, however, customers may advance their purchases planned for later periods towards a more recent campaign period in which they receive coupons applicable to the products they are interested in. This needs to be kept in mind when interpreting the effects.
In order to get an impression of the extent to which coupons induce inter-temporal spillover effects and, on the other hand, longer-term increases in customer retention, we also assess the effect of coupon provision in campaign period t on daily expenditures in subsequent periods, namely in t + 1 and t + 2. It should, however, be noted that the estimated effect is the total effect of coupon reception on purchasing behavior in these subsequent periods. That is, it does not only capture the longer-term coupon-induced change in purchases at the store (net of spillovers from advancing purchases in periods in which the customer has applicable coupons). Rather, it also captures how coupon provision in t affects purchasing behavior in t + 1 and t + 2 through changing the likelihood of coupon reception in these later periods (e.g., because the customer redeems coupons in t or the coupons incentivize her to increase her purchases in t ). Disentangling the direct effect of coupon provision on purchasing behavior in subsequent periods from the indirect effect mediated via increasing the likelihood of coupon provision in these later periods would require estimating dynamic treatment effects of treatment sequences, such as the sequence of coupon reception in t and non-reception in t + 1 (see [ 97 ] for an approach to estimating dynamic treatment effects by means of DML).
Furthermore, it is worth noting that some coupons valid in t may still be valid in t + 1 and even t + 2. The estimated effect of coupon provision in t on purchasing behavior in later periods therefore also partially captures the treatment effect of coupons during their validity period. A look at the data shows that the likelihood of having a valid coupon in t + 1 or t + 2 is highly correlated with that of having a coupon in t (conditional on X ), be it due to the effect of coupons on re-provision or because the validity period of coupons exceeds that of the artificially created campaign periods. Part of the estimated longer-term effect is therefore likely attributable to the indirect effect of coupon provision in t on daily expenditures in t + 1 and t + 2, via increasing the probability that the customer has valid coupons in these subsequent periods.
6 Empirical strategy
In the following, let i ∈ {1, …., n } be an index for the n = 1, 582 customers in the data set and t ∈ {1, …, T } with T = 32 an index for the campaign period. Then, { Y i , t , D i , t , X i , t } denote the outcome, the treatment and the covariates, respectively, for individual i in campaign period t . Treatment D i , t is a binary indicator measuring exposure to a coupon campaign (of a specific type) and Y i , t denotes the outcome, defined as average per-day expenditures of customer i in period t . The covariates X i , t , all measured prior to or at the time of treatment assignment, include socio-economic variables (see Table 1 ), the average daily spending by product type in the period prior to the campaign t − 1, and variables that measure both whether the customer received coupons in t − 1 and whether he/she redeemed any. For estimating the effect of a particular coupon type, X i , t also contains variables on what other coupon types were provided to the customer in t ; in addition, it includes information not only about whether the customer received coupons in t − 1, but also about what type of coupons.
As the functional form of E [ Y | D , X ] is unknown, we use the causal forest approach by Wager and Athey [ 68 ] to estimate θ in a data-driven way rather than regressing Y on D and X based on a parametric model. The causal forest algorithm learns models for predicting the average of Y as a function of X , denoted by μ ( X ) = E [ Y | X ], and for predicting the probability of being treated conditional on X , which is commonly referred to as the propensity score, p ( X ) = Pr ( D = 1| X ). The estimates of these so-called plug-in parameters μ ( X ) and p ( X ) are incorporated into the estimation of treatment effects.
Section 6.1 describes the estimation of individualized treatment effect estimates for every observation in the sample as a function of its covariates X (CATEs) based on the causal forest. The estimated parameters obtained from the latter then permit estimating the ATE, as described in Section 6.2. Section 6.3 demonstrates the use of the causal forest estimates for evaluating treatment effects in different customer segments defined by selected covariates. Finally, Section 6.4 shows how to determine which customers should optimally be targeted by the different coupon campaigns to maximize effectiveness in terms of purchases.
6.1 Treatment effect heterogeneity
In order to generate the causal forest, the algorithm draws 2,000 random samples that contain 50% of the observations in the data set. In each random sample, it estimates a causal tree as follows: first, a randomly selected subset of covariates is chosen, the number of which is determined by means of cross-validation. The algorithm then uses these covariates for splitting the sample into two subsamples such that the CATEs in the two resulting subsamples are as heterogeneous as possible. More precisely, the algorithm determines both the covariate and the value at which the sample should be split (e.g., age < 25 vs. age ≥ 25) to maximize effect heterogeneity. Intuitively, the algorithm considers all possible splits on values of the considered covariates to find the optimal split in terms of effect heterogeneity. These nodes are split into a larger number of nodes by repeating the splitting procedure until no further splits can be performed without yielding nodes containing less than a certain number of treated and control observations, where the minimum number of observations is determined by cross-validation. The causal forest is finally obtained by averaging over the splitting structure of all 2,000 causal trees.
For the sake of comprehensibility, we have presented a somewhat simplified version of the causal forest algorithm, as provided in the grf package. For one, the causal_forest function does not determine the splitting rules by estimating the CATEs in all possible subsamples. The algorithm, rather, approximates the between-node effect heterogeneity generated through every potential split by means of a gradient for each observation in order to improve computational efficiency. Additionally, the algorithm involves several conditions for formulating splitting rules that aim at avoiding imbalance in the size of the nodes. Explaining these rules in detail would go beyond the scope of this discussion. The manual to the grf package, however, provides all the details (see [ 101 ]). Finally, the cross-validation procedure to determine the number of covariates used for building causal trees, the minimum node size as well as different other hyperparameters (see [ 101 ] for more details) provided in the grf package relies on a measure of the estimation error suggested by Nie and Wager [ 102 ]. This error measure is tailored to the evaluation of treatment effects (rather than predicting outcomes as in predictive ML), but is only one of different possible measures. The algorithm splits the data into two subsamples, then grows causal forests using 100 distinct randomly selected sets of hyperparameters and finally selects the set of hyperparameters with the smallest estimated error. We note that a complication in defining an error measure to be minimized by cross-validation in the context of causal ML is that the true CATEs are not observed. Therefore, the causal forest algorithm cannot compare observed and predicted CATEs for cross-validation purposes, while predictive algorithms can compare predicted and observed outcomes.
6.2 Average treatment effect
In our application, we estimate the ATE using the average_treatment_effect function provided in the grf package for R by Tibshirani et al [ 100 ], with standard errors clustered at the customer level.
6.3 Group average treatment effects
6.4 Optimal policy learning
The optimal policy learning approach by Athey and Wager [ 10 ] goes one step further, in the sense that it does not only estimate the effect of coupon provision in predefined customer groups. Rather, it exploits the heterogeneity in coupon effects to determine the coupon distribution rule that maximizes the overall effect of the coupon campaign. Based on observed covariates, the coupon distribution rule distinguishes customer segments that are likely to increase their purchasing behavior upon receiving a coupon from those customer groups not anticipated to respond positively to the campaign. More formally, the algorithm considers specific decision (or policy) rules for whether a coupon should be offered to a customer as a function of the covariate values in X , e.g., the customer’s age. Let us denote by π ( X ) such a decision rule, which could, for instance, impose that only elderly, but not younger, customers obtain a coupon.
Mathematically speaking, the rule maps a customer’s observed characteristics to the binary treatment decision of whether or not to target the customer through the coupon campaign: π : X → 0, 1. Optimal policy learning consists of learning the optimal rule among an assumably limited set of implementable candidate policies, where we use Π to denote this set. For instance, another possible rule of how to distribute coupons (in addition to the age-based rule) could be to offer them only to customers with a high volume of previous purchases. Then, both the age- and purchase-dependent rule would enter the set of feasible coupon policies provided in Π.
The optimal policy learning approach does not require defining the policies to be considered a priori, but only the number of customer segments between which coupon allocation can differ and the set of covariates that can be considered for determining these customer segments. Thus, the approach identifies the optimal coupon policy in a data-driven way. To determine the optimal coupon distribution strategy, i.e., the one that maximizes the objective function in 5 , the algorithm applies a tree-based approach that considers all possible covariate-defined sample splits for generating the customer segmentation (according to the pre-defined number of segments) and all possible coupon assignment strategies within these segments. The resulting coupon distribution rule can be represented as a decision (or policy) tree, i.e., a tree-shaped graph indicating at which values of which covariate the sample is split and which of the resulting customer segments shall receive coupons.
We estimate decision trees of depth 3, implying that we distinguish 8 customer segments for defining the optimal distribution of coupons by means of the policytree package for R by Sverdrup et al [ 107 ]. For determining the customer segments, we use all the customer characteristics available in the data set, i.e., age and income group, family size, marital status, and dwelling type. We redefine these variables by setting all missing values to -1, which allows us to omit the variables that indicate missing observations. Then, we also include the customers’ pre-campaign purchasing behavior. Since the algorithm performs a sample split at every possible value of each covariate, i.e., at each observed value, continuous variables can cause performance issues by driving up the number of sample splits. We, therefore, round the pre-campaign average daily expenditures to round values, namely to the nearest 100 for values between 0 and 1,000 and to the nearest 200 for values between 1,000 and 2,000. Further, we group all 157 observations with average daily expenditures of 2,000 or more into one category and include dummies that indicate whether a customer purchased items from the different product categories in the period prior to the campaign. This way, we still capture pre-campaign differences in purchasing behavior well, while substantially reducing the number of sample splits that need to be performed.
6.5 Robustness checks and goodness of fit assessment
As described in Section 4, our data set contains a large number of observations with missing socio-economic information. To investigate the robustness of our results with respect to these missing values, we perform the entire analysis on a reduced data set containing only observations of customers whose socio-economic background is known, i.e., on a data set with 13,792 observations of the purchasing behavior of n = 431 individuals.
In a second robustness check, we rerun our estimation of the ATE of receiving coupons (of a certain type) in the full sample, but using an alternative causal ML approach, namely Double ML [ 64 ]. Just as the causal forest approach, Double ML relies on combining effect estimation based on Neyman [ 75 ]-orthogonal scores with sample splitting. For estimating the plug-in parameters, we use Lasso regression with 10-fold cross-validation after augmenting the set of covariates with interaction and second order terms. The estimation is performed in the statistical software R [ 99 ] by means of the causalDML function provided in the causalDML package by Knaus [ 108 ] (see the manual to the causalDML package for more details).
Finally, we also assess how well the causal forest approach captures the total effect of coupon reception as well as effect heterogeneity. The fact that the true CATEs and ATE are unobservable prevents us from relying on performance measures that are commonly used to evaluate the performance of ML algorithms for predictive purposes. We therefore investigate the goodness of fit of the causal forest by means of the test_calibration function included in the grf package. Put simply, this function regresses the estimated CATEs on the average of all CATEs as estimated by the causal forest as well as on the difference between the CATE estimate for the respective observation and the average CATE estimate. A coefficient close to 1 on the average of all forest predictions suggests that the mean forest estimate for the CATEs is correct. A coefficient close to 1 for the difference between the individual CATE estimate and the average over all estimates indicates that the heterogeneity estimates from the forest are well calibrated. Further, this coefficient informs about the presence of heterogeneity. If it is significantly larger than 0, we can reject the null hypothesis of no heterogeneity.
7 Empirical results
7.1 treatment effect heterogeneity.
Fig 1 shows the distribution of the individualized treatment effects (CATEs) as estimated by means of the causal forest algorithm outlined in Section 6.1. The treatment effect of being provided with any coupon is positive for the vast majority of observations and, except for some outliers, ranges between -100 and 200 monetary units. Similarly, the provision of drugstore coupons and coupons applicable to other food have a positive effect for the majority of observations. The distributions of the effects of coupons applicable to ready-to-eat food as well as meat and seafood, however, seem to be rather centered around zero, such that the estimates are positive and negative, respectively, for about half of the observations. For coupons applicable to other non-food products, we even find a negative effect on daily expenditures for the majority of observations. The plots suggest greater heterogeneity in the treatment effects of the individual coupon categories than when pooling all coupons. It appears that the effects of the different coupon categories cancel each other out to some extent when combined in one analysis, implying that the different coupon categories should best be analyzed separately.
Distribution of CATE estimates for receiving any coupon, as well as coupons applicable to ready-to-eat food, meat and seafood, other food, drugstore products and other non-food products, denoted in monetary units.
https://doi.org/10.1371/journal.pone.0278937.g001
The heterogeneities in CATEs as revealed by the causal forest approach suggest not just assessing the ATE, as in Section 7.2, but also investigating how the effect of coupons (of certain categories) differs between customer groups defined by covariates X , see Section 7.3. Finally, heterogeneous effects motivate our aim to learn the optimal coupon distribution scheme across customers that maximizes the expected ATE of coupon provision, see Section 7.4.
7.2 The causal effect of receiving coupons
Table 2 shows the estimated ATE of receiving any coupon on daily expenditures in the campaign period, as well as that of receiving coupons from each of the five coupon categories, based on the AIPW approach outlined in Section 6.2. The results suggest that receiving any coupon has a positive and statistically significant effect on daily expenditures during the campaign period. Providing a customer with a coupon increases her expected daily expenditures by some 60 monetary units. The effect estimates for the different coupon categories provide a more nuanced picture. Provision of coupons for drugstore items and other food has a statistically significant positive effect on daily spending during the campaign period. Receiving coupons that belong to these categories increases expected average daily expenditures during the validity period by some 80 and 78 monetary units, respectively. Handing out coupons applicable to other non-food products, on the other hand, is estimated to decrease a customer’s expected average daily expenditures by some 24 monetary units, with this result also being statistically significant. The estimated ATE of providing coupons from the other two categories has no statistically significant effect on the customers’ expected daily spending during the campaign period. A possible explanation for the insignificant or significantly negative effect of these latter three coupon types is that the receipt of such coupons may not incentivize people to buy, but that such coupons are mainly used for products that the coupon recipient would have purchased anyway.
https://doi.org/10.1371/journal.pone.0278937.t002
As discussed in Section 5.2, coupon provision may, on the one hand, have longer-term positive effects on purchasing behavior by increasing customer loyalty, and on the other hand, bring about inter-temporal spillovers by inducing customers to advance their purchases to periods when they have coupons applicable to them. We therefore also take a look at the overall effect of coupon reception in t on daily expenditures in the following campaign period ( t + 1) and the period thereafter ( t + 2) (see Table 3 ). The results suggest that the effect of coupon provision on daily expenditures is sustainable, i.e., coupon provision in t not only has a short-term effect on purchases in t , but also has a statistically significant, albeit smaller, effect on purchases in subsequent periods. This may be due to a coupon-induced increase in customer retention (but also to indirect effects, see the discussion in Section 5.2).
https://doi.org/10.1371/journal.pone.0278937.t003
The longer-term effect of drugstore and other food coupons is also positive and statistically significant, with drugstore coupons showing an even larger effect on purchasing behavior in both post-treatment periods than in the short term. Coupons applicable to other non-food products, that in the short run have a statistically significant negative effect, show a statistically significant positive effect on daily spending in the subsequent periods. One possible explanation for this finding is that, while in the short run these coupons were only redeemed for the purchase of products that would have also been purchased without the coupons, in the longer term they may have increased customer loyalty.
The estimated effect of meat and seafood coupons on expenditures in t + 1 and t + 2 is not statistically significant, while that of ready-to-eat food coupons is even significantly negative for the outcome in t + 1, which may indicate spillover effects that are not offset by positive expenditure-increasing effects. For ready-to-eat food and meat/seafood coupons, we can therefore conclude that they do not seem to be an effective marketing tool for increasing customer spending, neither in the short nor in the longer run.
In the following, we will again focus on the short-term effect of coupon provision in period t on daily expenditures in period t . The next section examines effect heterogeneity with regard to selected customer characteristics. This is because the provision of coupons could significantly increase spending of certain customer groups, despite not having a statistically significant effect on the overall customer base. Similarly, providing coupons applicable to drugstore or other food could have a significant impact on purchasing behavior only among certain subgroups of customers.
7.3 Group average treatment effects
In this section, we assess how the provision of coupons affects different customer groups, by estimating Group Average Treatment Effects (GATEs) as discussed in Section 6.3. We investigate how the effect of providing coupons differs depending on customers’ age, income, family size and pre-campaign expenditures. Furthermore, we also examine the GATEs of those coupon categories with a highly statistically significant ATE, i.e., drugstore coupons and coupons applicable to other food.
Fig 2 shows the GATEs of receiving any coupon by age, income, family size and pre-campaign expenditures, respectively. The graphs suggests that providing coupons has a positive effect on purchasing behavior in every customer group and most effect estimates are statistically significant. The effect appears to be particularly large among customers from smaller households and among those who made either no or large purchases in the period prior to the campaign.
GATE estimates of receiving any coupon by age, income, family size and pre-campaign expenditures with 95% confidence interval, denoted in monetary units.
https://doi.org/10.1371/journal.pone.0278937.g002
The GATE charts in Fig 3 show that in almost all subgroups considered, the provision of coupons for other food has a positive effect on daily spending, which is in many cases statistically significant. The most pronounced differences in GATEs can be found among customer subgroups defined by average daily spending prior to the campaign period. The effect of food coupons tends to be high and statistically significant for previously inactive customers, while it is much smaller, though still statistically significant, for customers with high pre-period spending. This may suggest that coupons for other food have the potential to reactivate dormant customers. This hypothesis is also supported by the fact that the provision of coupons applicable to other food has a relatively large statistically significant effect among customers for whom information on socio-economic characteristics is not available. Customers for whom no information is available may be more likely to have low loyalty to the store and to be rather inactive, but they may be reactivated by providing them with other food coupons. These results suggest that coupons applicable to other food are efficient for inactive and non-frequent customers, while they have less impact on the purchasing behavior of frequent shoppers.
GATE estimates of coupons applicable to other food items with 95% confidence interval, denoted in monetary units.
https://doi.org/10.1371/journal.pone.0278937.g003
Fig 4 shows that providing drugstore coupons has a positive effect on daily spending for almost all subgroups considered and that the effect is statistically significant in most cases. Again, the largest difference can be found in the GATE estimates by pre-campaign spending. The effect of drugstore coupons on average per-day expenditures is larger the higher the customer’s pre-campaign spending, which is the reverse pattern of what we find for coupons applicable to other food. This suggests that other food coupons are more efficient at reactivating dormant customers and drugstore coupons at retaining frequent shoppers. S4 Fig shows the GATE plots for ready-to-eat food coupons, S5 Fig those for meat/seafood coupons, and S6 Fig those for coupons applicable to other non food products.
GATE estimates of drugstore coupons with 95% confidence interval, denoted in monetary units.
https://doi.org/10.1371/journal.pone.0278937.g004
7.4 The optimal distribution of coupons
While the ML-based estimation of ATEs and GATEs are very useful tools for evaluating the average effects of coupon campaigns (across subgroups), it is not necessarily most appropriate for optimizing strategies for later coupon campaigns. For the latter task, we apply the optimal policy learning framework by Athey and Wager [ 10 ] to determine which customer groups should be provided with coupons in order to maximize their average impact.
Fig 5 shows the optimal distribution rules (or policies) for each coupon category as suggested by the optimal policy tree outlined in Section 6.4. The optimal rule for ready-to-eat food coupons (decision tree (a)) suggests providing ready-to-eat food coupons to customers with no drugstore purchases in the pre-campaign period if their marital status is unknown (a value of -1 for the variables family size, marital status, age group and income group implies missingness, see Section 6.4) and their age is unknown or they are not older than 26, or if their marital status is known and they live in a household with at most three members. The retailer should further provide ready-to-eat food coupons to customers who purchased drugstore products in the pre-period if their income is in one of the lowest four income groups or unknown, or if their age is unknown and their average daily purchases in the pre-period were less than 50 monetary units (as daily expenditures are rounded for creating policy trees, all customers with daily expenditures below 50 monetary units fulfil the condition ‘Daily Expenditure Preperiod < = 0’).
Depth-3 trees for optimal provision of coupons applicable to (a) ready-to-eat food, (b) meat and seafood, (c) other food, (d) drugstore products as well as (e) other non-food products.
https://doi.org/10.1371/journal.pone.0278937.g005
The optimal distribution rule for drugstore coupons (decision tree (d)) proposes providing drugstore coupons to those customers with unknown, low, or middle incomes if their daily pre-campaign expenditures did not exceed 900 monetary units and/or their family does not have more than 2 members. Customers belonging to the higher-middle income group should receive drugstore coupons if their average in-store spending did not exceed 100 monetary units per day in the period before the campaign. Customers belonging to the high-income group, finally, should only be provided with drugstore coupons if they purchased other food products at the store in the pre-campaign period.
The distribution rules paint a similar picture as the GATE estimates in Section 7.1 about which customer groups are likely to be positively impacted by the provision of certain coupon types. In contrast to the effect heterogeneity analysis across pre-specified subgroups in Section 7.1, the optimal policy tree determines the covariate values at which the sample should optimally be split as well as the groups of coupon recipients and non-recipients in a data-driven way, in order to maximize the average effect of the campaign.
The other decision trees can be interpreted accordingly. A look at the covariates used for sample splitting in those other decision trees shows that each observed customer characteristic is used for defining the distribution rules of at least one coupon type.
7.5 Robustness checks and goodness of fit assessment
The ATEs based on the reduced data set containing only observations of customers whose socio-economic background is known are presented in Table 4 . They are close to the ATE estimates in the full data set, although the standard errors are considerably larger, due to the much smaller number of observations.
https://doi.org/10.1371/journal.pone.0278937.t004
The GATE and policy tree plots are provided in the Supporting information Section (see S7 – S12 Figs for the GATE estimates of receiving any coupon, ready-to-eat food coupons, meat/seafood coupons, other food coupons, drugstore coupons and other non-food coupons, respectively. The policy tree plots are provided in S13 Fig ). The GATE plots show similar patterns in how different customer groups are affected by each coupon type, although they are not exactly identical with the GATEs estimated in the full sample. In the policy tree plots, the splitting rules are based on a similar set of variables with similar cutting points as those estimated in the full data set. These results suggest that the large number of observations with missing socio-economic information does not introduce systematic bias into the estimation of the treatment effects and the optimal coupon distribution scheme. The longer-term ATE estimates are provided in Table 5 .
https://doi.org/10.1371/journal.pone.0278937.t005
The (short-term) ATE estimates obtained from Double ML with Lasso regression are provided in Table 6 and the longer-term Double ML-based ATE estimates in Table 7 . They are similar to the causal forest estimates, indicating that the results are robust to the ML algorithm used for predicting the plug-in parameters.
https://doi.org/10.1371/journal.pone.0278937.t006
https://doi.org/10.1371/journal.pone.0278937.t007
Finally, Table 8 provides the results for the goodness-of-fit tests. The latter do not point to a misspecification of the CATE models of receiving any coupons, coupons for other food items, drugstore coupons and coupons for other non-food items. Furthermore, they indicate that the causal forests for receiving any coupon and drugstore coupons appropriately capture effect heterogeneity. For other food coupons, the other coupon category that showed a statistically significant positive effect on sales, however, we cannot reject the null hypothesis that there is no effect heterogeneity. For the categories of ready-to-eat food and meat/seafood coupons, however, the tests do not suggest that the mean forest estimates are correct or that the causal forests appropriately capture effect heterogeneity. We recall that for these coupons, we did not find any significant effects on customer spending.
https://doi.org/10.1371/journal.pone.0278937.t008
8 Conclusion
In this paper, we presented different causal ML methods and applied them to evaluate a marketing intervention, namely a coupon campaign in a retail store. We assessed the average effects of coupons for different product categories, as well as the extent to which the effects differed across predefined subgroups of customers, for instance, between clients with relatively high vs. low prior purchases. Finally, we determined in a data-driven way which customer segments should optimally be provided with a coupon to maximize effectiveness in terms of sales, a method known as optimal policy learning.
We found that coupons for only two out of five product categories, namely ‘drugstore articles’ and ‘other food’, had a positive and statistically significant overall effect on purchases, while receiving coupons for other non-food products actually significantly reduced customers’ daily spending. Additionally, we detected several customer segments whose purchasing behavior was influenced particularly strongly by certain types of coupons and should therefore be targeted by coupon campaigns to maximize the effect on sales. Such information appears very useful for retailers to optimally design marketing campaigns with regard to their impact.
Causal ML methods can be applied to evaluate and optimize business strategies also in other domains than the one considered in this paper, if sufficiently rich observational or experimental data on treatments (like business decisions or firm policies), outcomes (like key performance indicators or sales), and covariates (like customer characteristics) are available. Further potential applications for causal ML are the evaluation and optimization of online marketing interventions (like online ads), loyalty programs and other campaigns for tackling customer attrition and churn, but also the assessment of different employee benefit plans and compensation schemes, designs of job postings, or in-house training programs, among many other potential use cases.
Supporting information
S1 table. daily expenditures by product type..
Mean of daily expenditures by brand and product type in the total sample (’Overall‘), among coupon receivers and non-receivers as well as the mean difference across treatment states and the p-value of a two-sample t-test.
https://doi.org/10.1371/journal.pone.0278937.s001
S2 Table. Descriptive statistics, variable means among (non-)recipients of different coupon types.
Mean of the variables among the treated who received a coupon of a certain category and of those who did not. The coupons of each category are applicable to the following product categories defined by the retailer: (a) ready-to-eat food coupons: ‘Bakery’, ‘Restaurant’, ‘Prepared Food’, ‘Dairy, Juices & Snacks’, (b) meat and seafood coupons: ‘Meat’, ‘Packaged Meat’, ‘Seafood’, (c) coupons applicable to other food: ‘Grocery’, ‘Salads’, ‘Vegetables (cut)’, ‘Natural Products’, (d) drugstore coupons: ‘Pharmaceutical’, ‘Skin & Hair Care’, and (e) coupons applicable to other non-food products: ‘Flowers & Plants’, ‘Garden’, ‘Travel’, ‘Miscellaneous’.
https://doi.org/10.1371/journal.pone.0278937.s002
S1 Fig. Propensity scores of receiving any coupon & ready-to-eat food coupons.
Distribution of propensity scores of receiving any coupon among observations that received (a) no coupon and (b) any coupon, as well as that of the propensity scores of receiving ready-to-eat food coupons among observations that (c) did not and (d) did receive ready-to-eat food coupons. The plots are produced with the logspline command in R with the lower and upper bounds of the support of the propensity scores are set to 0 and 1.
https://doi.org/10.1371/journal.pone.0278937.s003
S2 Fig. Propensity scores of receiving meat/seafood coupon & other food coupons.
Distribution of propensity scores of receiving meat/seafood coupons among observations that (a) did not and (b) did receive meat/seafood coupons, as well as that of the propensity scores of receiving other food coupons among observations that (c) did not and (d) did receive other food coupons. The plots are produced with the logspline command in R with the lower and upper bounds of the support of the propensity scores are set to 0 and 1.
https://doi.org/10.1371/journal.pone.0278937.s004
S3 Fig. Propensity scores of receiving drugstore coupons & other non-food coupons.
Distribution of propensity scores of receiving drugstore coupons among observations that (a) did not and (b) did receive drugstore coupons, as well as that of the propensity scores of receiving other non-food coupons among observations that (c) did not and (d) did receive other non-food coupons. The plots are produced with the logspline command in R with the lower and upper bounds of the support of the propensity scores are set to 0 and 1.
https://doi.org/10.1371/journal.pone.0278937.s005
S4 Fig. GATEs of ready-to-eat food coupons.
GATE estimates of ready-to-eat food coupons with 95% confidence interval, denoted in monetary units.
https://doi.org/10.1371/journal.pone.0278937.s006
S5 Fig. GATEs of meat/seafood coupons.
GATE estimates of meat/seafood coupons with 95% confidence interval, denoted in monetary units.
https://doi.org/10.1371/journal.pone.0278937.s007
S6 Fig. GATEs of non-food coupons.
GATE estimates of coupons applicable to other non-food products with 95% confidence interval, denoted in monetary units.
https://doi.org/10.1371/journal.pone.0278937.s008
S7 Fig. GATEs of receiving any coupon, estimated in reduced data set.
GATE estimates of receiving any coupon with 95% confidence interval, estimated in reduced data set and denoted in monetary units.
https://doi.org/10.1371/journal.pone.0278937.s009
S8 Fig. GATEs of ready-to-eat food coupons, estimated in reduced data set.
GATE estimates of ready-to-eat food coupons with 95% confidence interval, estimated in reduced data set and denoted in monetary units.
https://doi.org/10.1371/journal.pone.0278937.s010
S9 Fig. GATEs of meat/seafood coupons, estimated in reduced data set.
GATEs of meat and seafood coupons with 95% confidence interval, estimated in reduced data set and denoted in monetary units.
https://doi.org/10.1371/journal.pone.0278937.s011
S10 Fig. GATEs of other food coupons, estimated in reduced data set.
GATE estimates of coupons applicable to other food items with 95% confidence interval, estimated in reduced data set and denoted in monetary units.
https://doi.org/10.1371/journal.pone.0278937.s012
S11 Fig. GATEs of drugstore coupons, estimated in reduced data set.
GATE estimates of drugstore coupons with 95% confidence interval, estimated in reduced data set and denoted in monetary units.
https://doi.org/10.1371/journal.pone.0278937.s013
S12 Fig. GATEs of other non-food coupons, estimated in reduced data set.
GATE estimates of coupons applicable to other non-food items with 95% confidence interval, estimated in reduced data set and denoted in monetary units.
https://doi.org/10.1371/journal.pone.0278937.s014
S13 Fig. Depth-3 trees for optimal coupon provision, estimated in reduced data set.
Depth-3 trees for optimally distributing coupons applicable to (a) ready-to-eat food, (b) meat and seafood, (c) other food, (d) drugstore products and (e) other non-food products, estimated in reduced data set.
https://doi.org/10.1371/journal.pone.0278937.s015
- View Article
- Google Scholar
- 8. Luk C, Choy K, Lam H. Design of an intelligent customer identification model in e-Commerce logistics industry. In: MATEC Web of Conferences. vol. 255. EDP Sciences; 2019. p. 04003.
- 33. Li S, Vlassis N, Kawale J, Fu Y. Matching via Dimensionality Reduction for Estimation of Treatment Effects in Digital Marketing Campaigns. In: IJCAI; 2016. p. 3768–3774.
- 34. Berning JP, Zheng H. The Effect of Retail Grocery Coupons for Breakfast Cereals on Household Purchasing Behavior. In: 2011 Annual Meeting, July 24-26, 2011, Pittsburgh, Pennsylvania. 103661. Agricultural and Applied Economics Association; 2011.
- PubMed/NCBI
- 51. He J, Jiang W. Understanding Users’ Coupon Usage Behaviors in E-Commerce Environments. In: 2017 IEEE International Symposium on Parallel and Distributed Processing with Applications and 2017 IEEE International Conference on Ubiquitous Computing and Communications (ISPA/IUCC). IEEE; 2017. p. 1047–1053.
- 55. Xiao F, Li L, Xu W, Zhao J, Yang X, Lang J, et al. DMBGN: Deep Multi-Behavior Graph Networks for Voucher Redemption Rate Prediction. In: Proceedings of the 27th ACM SIGKDD Conference on Knowledge Discovery & Data Mining; 2021. p. 3786–3794.
- 57. Lycett M. ‘Datafication’: making sense of (big) data in a complex world; 2013.
- 61. Hünermund P, Kaminski J, Schmitt C. Causal Machine Learning and Business Decision Making. Available at SSRN 3867326. 2021;.
- 62. Smith AN, Seiler S, Aggarwal I. Optimal Price Targeting. Available at SSRN 3975957. 2021;.
- 63. Gordon BR, Moakler R, Zettelmeyer F. Close Enough? A Large-Scale Exploration of Non-Experimental Approaches to Advertising Measurement. arXiv preprint arXiv:220107055. 2022;.
- 66. Huber M, Meier J, Wallimann H. Business analytics meets artificial intelligence: Assessing the demand effects of discounts on Swiss train tickets. arXiv preprint arXiv:210501426. 2021;.
- 67. Narang U, Shankar V, Narayanan S. The Impact of Mobile App Failures on Purchases in Online and Offline Channels. Working Paper; 2019.
- 71. Cagala T, Glogowsky U, Rincke J, Strittmatter A. Optimal Targeting in Fundraising: A Causal Machine-Learning Approach. arXiv preprint arXiv:210310251. 2021;.
- 73. Haag F, Hopf K, Menelau Vasconcelos P, Staake T. Augmented Cross-Selling Through Explainable AI—A Case From Energy Retailing. 2022;.
- 80. Hackathon AML. Predicting Coupon Redemption. 2019. url: https://www.kaggle.com/vasudeva009/predicting-coupon-redemption .
- 82. Lechner M. Identification and estimation of causal effects of multiple treatments under the conditional independence assumption. In: Lechner M, Pfeiffer F, editors. Econometric Evaluations of Active Labor Market Policies in Europe. Heidelberg: Physica; 2001.
- 95. Qu Z, Xiong R, Liu J, Imbens G. Efficient Treatment Effect Estimation in Observational Studies under Heterogeneous Partial Interference. arXiv preprint arXiv:210712420. 2021;.
- 97. Bodory H, Huber M, Lafférs L. Evaluating (weighted) dynamic treatment effects by double machine learning. arXiv preprint arXiv:201200370. 2020.
- 99. R Core Team. R: A Language and Environment for Statistical Computing; 2022. Available from: https://www.R-project.org/ .
- 100. Tibshirani J, Athey S, Sverdrup E, Wager S. grf: Generalized Random Forests. R package version 2.2.0. 2022. url: https://CRAN.R-project.org/package=grf .
- 101. Athey S, Friedberg R, Hadad V, Hirshberg D, Miner L, Sverdrup E, et al. generalized random forests (grf 2.1.0). 2022. url: https://grf-labs.github.io/grf/REFERENCE.html .
- 108. Knaus MC. causalDML: Causal Double Machine Learning; 2020.
Cause-related marketing: a systematic review of the literature
- Original Article
- Open access
- Published: 08 January 2022
- Volume 20 , pages 25–64, ( 2023 )
Cite this article
You have full access to this open access article
- Hina Yaqub Bhatti ORCID: orcid.org/0000-0003-2157-7418 1 , 2 ,
- M. Mercedes Galan-Ladero 1 &
- Clementina Galera-Casquet 1
15k Accesses
22 Citations
1 Altmetric
Explore all metrics
Cause-Related Marketing (CRM) is one of the most versatile activities among the Corporate Social Responsibility (CSR) initiatives. Though CRM is extensively researched, however, only a few authors have performed systematic literature reviews on CRM. Therefore, more systematic reviews of CRM are still needed to complete and bring together the more contributions, advances, and different existing research lines. Thus, this paper provides a comprehensive overview of the existing literature in CRM from the two keywords: “Cause-Related Marketing” and “Cause Marketing”, and the time period ranges from 1988 to 2020. In this study, rigorous protocol is used in synthesizing 344 English articles drawing upon e-journal database searches. These articles were categorized by time-wise development, country-wise development, methodological development, cross-cultural analysis, the role of journals. This study also carried out the Bibliometric Analyses. The review highlights that the concept of CRM has evolved from being considered a marketing mix tool (a promotion tool), to being considered as a CSR initiative, with a more strategic character. Our findings revealed that only a few journals published articles on CRM. Geographically, the CRM study was initiated in North America, followed by Europe and Oceania, and Asian and Sub-Saharan African countries. From the third decade, there was more collaboration in cross-cultural studies and the use of mixed-method (qualitative and quantitative studies) approach. Lastly, this study shows the most manifest research gaps in CRM that opens avenue for future research.
Similar content being viewed by others
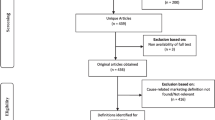
Rethinking the boundaries of cause-related marketing: Addressing the need for a new definition
Theoretical Background: Introduction to Cause-Related Marketing
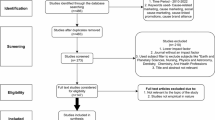
Factors affecting attitude and purchase intention towards cause-related marketing: a systematic literature review using TCCM approach
Avoid common mistakes on your manuscript.
1 Introduction
Cause-Related Marketing (CRM) is a versatile and growing activity in the marketing field. It provides opportunities to profit and non-profit organizations, and consumers, to participate in a social cause (Varadarajan & Menon, 1988 ). Since 1988, CRM initiatives have gradually increased for more than three decades. CRM allows to achieve the societal and financial corporate establishment’s goals, as well as provide the opportunity to consumers to participate in an altruistic act.
Formally, the first CRM campaign named in this way was carried out by American Express (AMEX) in 1983, in the United States. The purpose of this program was to increase the usage of the AMEX credit card, but also collect money to be donated for the renovation of the Statue of Liberty. This project was developed from September to December, and the donation was $1.7 million (Varadarajan & Menon, 1988 ). Since then, and according to the IEG Sponsorship Report, cause sponsorship spending in North America has grown continuously Footnote 1 from $120 million in 1990 until $2.23 billion in 2019, as shown in Fig. 1 . In 2020, due to the COVID-19 pandemic, U.S. sponsorship value was $10 Billion (annually), which approximately increased 38% (IEG, 2020 ).
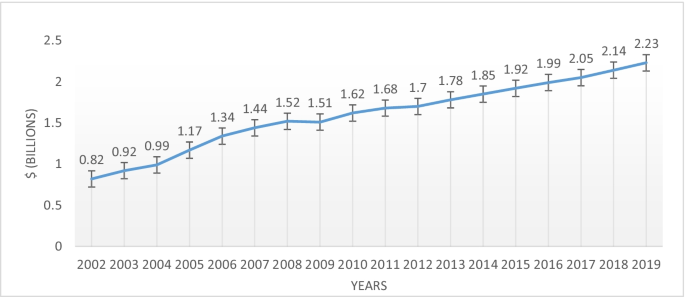
IEG Sponsorship Report from 2002 to 2019. Note: We only include data since 2002, because previous data are not available on the IEG Sponsorship Report. Source: IEG (2020)
Consequently, the practice of CRM has also increased for the last three decades because more profit organizations have engaged in CRM activities (Adomaviciute et al., 2016 ), non-profit organizations have maintained environmental protection, health, and other worthy causes (Grolleau et al., 2016 ), and when consumers purchase the CRM products for support the cause, they have a prosocial behavior (Chang & Chu, 2020 ) and feel happy (Jeong & Kim, 2020 ; Vrontis et al., 2020 ).
During this time, CRM has become a topic of considerable debate in both managerial and academic circles worldwide. Although some systematic literature has been presented on this topic (see, for example, Guerreiro et al., 2016 ; Lafferty et al., 2016 ; Natarajan et al., 2016 ; or Thomas et al., 2020 ), an updated systematic literature review is required. Thus, we present a new systematic literature review: (1) To complete the review of the academic research articles in the area of CRM, from 1988 to 2020, with the perspective of profit organizations, non-profit organizations, and consumers over the last three decades; (2) To include cross- cultural studies; (3) To include studies carried out in developed and developing countries; (4) To include studies executed in different societies (e.g. Muslim societies, Western societies with Christian traditions, etc.); and (5) To conduct a bibliometric analysis using VOSViewer Software.
Thus, the main objective of this paper is to provide a systematic literature review of the existing research in the field of Cause-Related Marketing. More specifically, our aim is to find influential papers that have shaped this field and provide the overview of historical development in the field of research, focusing first on previously analyzed criteria: Time-Wise Development of CRM Literature, Country-Wise Development of CRM Literature, Methodological development in CRM Literature, and Role of Journal in Development of CRM literature. But also, this study carries out a systematic review with a bibliometric analysis. On the one hand, the systematic review helps the researchers to improve the rigor of prior reviewing literature. On the other hand, bibliometric analysis helps to analyze divergent views and examine the development of the CRM field.
Hence, this paper has followed two steps in the systematic literature review on CRM: (1) to select the inclusion and exclusion criteria, and (2) to analyze the evolution of CRM in seven different categories.
First step : Inclusion and Exclusion Criteria.
This research only included published papers in journals, from 1988 to 2020 (data sources such as working papers, reports, newspapers, textbooks, conference papers, or theses / dissertations, were not included).
Two keywords, “Cause-Related Marketing” and “Cause Marketing”, were used to search the databases (SAGE Publications, JSTOR, Emerald Full Text, Springer, John Wiley Publications, Elsevier, Taylor and Francis, and Google Scholar).
This research also used conceptual review and empirical studies of different countries.
This research only included papers written in English (i.e., non-English language research articles were excluded).
This study considered the date of publication of the journal as the date of the research articles.
Second step : Academic researchers have used qualitative and quantitative methods for literature review to organize and provide the above underlying findings on CRM. And according to Liu et al., ( 2015 ), Bibliometric Analysis is a tool to examine literature review. Thus, this study has also provided a static and systematic picture of the research (Aria & Cuccurullo, 2017 ). This study relies on bibliometric techniques such as author-citation analysis, or co-words or co-occurrence analysis, and co-citation analysis of authors through VOSviewer software (version 1.6.5). Following Thomas et al. ( 2020 ), we selected Time-Wise Development of CRM Literature, Country-Wise Development of CRM Literature, and Methodological Development in CRM Literature. And we added other analysis such as Role of Journal in Development of CRM literature, author-citation analysis, and Co-words or Co-occurrence analysis, proposed by Poje & Groff ( 2021 ). We also considered adding a new category that had not been considered in previous studies: cross-cultural analysis.
Therefore, the structure of this paper is organized as follow: firstly, we show the CRM theoretical background (with a previous introduction to CSR, to link it with CRM, because CRM is generally considered under the umbrella of CSR – e.g., Kotler and Lee, 2005 ; Galan-Ladero, 2012 ); secondly, we offer the results of our literature analysis in the CRM field; later, we discuss these results; and finally, we offer the main conclusions, also considering the main limitations of this study and further research.
2 Background
Since the inauguration of the third millennium, Corporate Social Responsibility (CSR) has become a globally hot issue by the rapid change of the environment. A large number of organizations, from developed and developing countries, have focused on CSR.
CSR, defined as “a concept whereby companies integrate social and environmental concerns in their business operations and in their interaction with their stakeholders on a voluntary basis ” (European Commission, 2001), has a wide range of history: it started in Western countries, but later, it spread all over the world. Antecedents of CSR can be found at 18th and 19th Centuries, with the creation of welfare schemes adopted with a paternalistic approach, to protect companies and retain employees with improved life quality (Carroll 2008 ). But it is in the twentieth Century, and specifically after World War II, when scholars and practitioners discussed about the social responsibilities, and successful businesses also adopted such responsibilities (Heald, 1970 ).
Thus, CSR started to be established and, in the last seven decades, it has played different roles:
The 1950s was the first era that established the current CSR. Successful business leaders and board of directors moved towards the ethics of society. Bowen, the first who coined the term, introduced the concept, and provided the initial definition of CSR, described as “ the obligations of businessmen to pursue those policies, to make those decisions, or to follow those lines of action which are desirable the objectives and values of our society ” (Bowen, 1953 , p. 6). In this area, Heald ( 1957 ), discussed that businesses do not only serve on economic performance work, but they also serve on humane and constructive social policies.
The 1960s : Many of the definitions of CSR are formalized. Walton ( 1967 ) was a prime thinker who addresses the different aspects of CSR: “ In short, the new concept of social responsibility recognizes the intimacy of the relationships between the corporation and society and realizes that such relationships must be kept in mind by top managers as the corporation and the related groups pursue their respective goals ( Walton, 1967 , p. 18)”.
The 1970s : Friedman described that the social responsibility of business is to enhance profits and maximize shareholder value. Therefore, Carroll ( 1979 ) came in this decade with the new concept of CSR, defined as “ the social responsibility of business encompasses the economic, legal, ethical, and discretionary expectations that society has of organizations at a given point in time ”.
The 1980s : the notions of stakeholder management and business ethics had become the main integral part of the business (Carroll, 2008 ). In 1980, Jones proposed that CSR is a process, not the outcome, and CSR, when engaged in as a process of decision making, should constitute CSR behavior by the corporation (Jones, 1980 ). Also, Aupperle et al., ( 1983 ) suggested that four aspects include CSR: economic, legal, ethical, and voluntary or philanthropic responsibilities.
The 1990s : Carroll ( 1991 ) revised the concept of CSR and introduced the “Pyramid of Corporate Social Responsibility”. He described four main responsibilities of the company: economic responsibility (“be profitable”), legal responsibility (“obey the laws and regulations”), ethical responsibility (“do what is just and fair”), and philanthropic responsibility (“be a corporate citizen”). During this decade, Elkington ( 2001 ) introduced another concept of CSR, the “Triple Bottom Line”, which focuses on three issues: social responsibility (“people”), environmental responsibility (“planet”), and economic responsibility (“profit”).
In the first decade of the twenty-first century (The 2000s), CSR extends to emerging markets. After the collapse of Enron, Footnote 2 many organizations and corporations focused on establishing CSR departments, hiring CSR consultants and CSR managers. On the other hand, in 2002, ISO Committee on Consumer Policy play an important role in ISO 26000, an international standard that present a guideline on Corporate Social Responsibility. Footnote 3
In the second decade of the twenty-first century (The 2010s), Kramer and Porter ( 2011 ) introduced the concept of “creating shared value”, which becomes the core objective of business strategies. 2015 is an important year because the “2030 Agenda for Sustainable Development”, with the “Sustainable Development Goals” (SDGs), was launched. SDGs covered a wide range of global areas, such as fighting against climate change, removing poverty and hunger, as well as promoting sustainable consumption, among others.
Therefore, different theories have been created and adapted during all this time. The most important theories are Carroll’s CSR Pyramid Theory, Footnote 4 Triple Bottom Line Theory, Footnote 5 Stakeholder Theory, Footnote 6 and Corporate Citizenship Theory. Footnote 7
On the other hand, CSR initiatives, formed as a part of the core business activities, provide long-term financial security and growth for stakeholders but also increase the market position (Bhattacharyya et al., 2008 ). Under the big umbrella of CSR, different initiatives have appeared, and they have become growing popular among profit organizations worldwide. Kotler et al., ( 2012 ) explained six different types of CSR initiatives (see Table 1 ), which included cause promotion, cause-related marketing, corporate social marketing, corporate philanthropy, community volunteering, and socially responsible business practices.
According to Thomas et al., ( 2011 ), CSR has received significant attention in both academic and business societies. CRM, as one of these initiatives, has progressed in social responsibility and allows firms to link their philanthropic activities and strategic marketing goals. On the other hand, CRM activities also have been an increasing part of the corporate marketing plans (Gupta & Pirsch, 2006a ). Therefore, this study especially focuses on this CSR initiative: Cause-Related Marketing (CRM).
The first definition of Cause-Related Marketing (CRM) was introduced by Varadarajan and Menon ( 1988 , p. 60), as “ the process of formulating and implementing marketing activities that are characterized by an offer from the firm to contribute a specified amount to a designated cause when consumers engage in revenue-providing exchanges that satisfy organizational and individual objectives”. This definition provides two main streams: to support the charitable cause and to satisfy organizational and individual objectives.
On the other hand, the most essential and significant benefit of the CRM is shown as a win-win-win situation (for the profit organizations, non-profit organizations, and consumers - Adkins, 1999 ). CRM campaigns increase the number of sales for the organization, as well as enhance the number of donations to the non-profit organizations (Deb & Amawate, 2019 ). CRM campaigns also give the best chance to profit organizations to attract the customers towards organization and enhance customer loyalty (Galan-Ladero et al., 2013b ), as well as they create or enhance emotional engagement with target customers, build a strong relationship with them (Cone et al., 2003 ; Docherty & Hibbert, 2003 ), and also maintain the company’s goodwill (Chang & Chu, 2020 ). Consequently:
The for-profit organizations use CRM as a strategic tool to build a strong brand image in the customer’s mind (Ahluwalia & Bedi, 2015 ). And the internal benefit of the for-profit organization is to help increase the employee’s self-esteem, commitment, and loyalty (Cone et al., 2003 ; Polonsky & Wood, 2001 ).
The non-profit organizations try to increase awareness about the cause, educate the customers, and support the charitable cause (Nowak and Clarke, 2003 ). On the other hand, CRM in non-profit organizations increases the number of donors (Docherty & Hibbert, 2003 ; Polonsky & Wood, 2001 ).
For consumers , charitable causes, linked to their purchases, boost their feeling of happiness and inner satisfaction (Chaabane & Parguel, 2016 ), and they also feel good when helping others (Imas, 2014
3 Analysis and Main results
Due to an increasing number of CRM research papers that identify the most essential and main contributions in the field, and to objectify the outcomes, then bibliometric analysis is introduced. Zupic and Čater ( 2015 ) explained the five main bibliographic methods, which consists of citation analysis, co-citation analysis, bibliographic coupling, co-author analysis and co-word analysis. In this study, we apply Co-words or Co-occurrence analysis, Co-citation analysis, and cited journals analysis. These analyses were run on VOS-software.
3.1 Analysis of the different definitions of CRM
A wide variety of definitions of CRM have been contributed since 1988 (see Appendix 1, Table 7 ). In Table 2 , we summarize the main CRM definitions, from 1988 to 2020, according to the main keywords included in them: CRM as an activity (a marketing activity and/or a CSR activity), as a strategy, as a marketing mix tool, and as an alliance (between profit and nonprofit organizations). Thus, we can observe that there is not a general, unanimous agreement about its definition yet. However, the concept of CRM has evolved from being considered a short-term marketing mix tool (a promotion tool), to being considered a CSR initiative, with a more strategic character.
3.2 Time-wise development of CRM literature
However, in this study, we start from the time-wise development of Cause-Related Marketing. First, we identify the number of research articles into three time periods (decades): 1988–2000, 2001–2010, and 2011–2020 (previous systematic literature did not classify them into decades). With the growing body of Cause-Related Marketing, it is better to organize it in three decades because differences are appreciated, depending on the time.
Varadarajan and Menon introduced the CRM term in academia in 1988, and the following three decades witnessed gradual growth in CRM literature. Table 3 shows the annual evolution of this figure from 1988 to 2020.
Thus, we can classify the three decades based on CRM literature progression:
Introductory decade (1988–2000). The field of CRM was introduced in this period with a limited number of published articles (13). However, these articles were very innovative and aroused interest in this new solidarity initiative.
Emerging decade (2001–2010). The CRM field grabbed the attention of researchers in this second decade, with a notable increase in the published literature, especially in the last two years of this decade. The number of published articles reached 74. Consequently, CRM became an interesting and novel research topic, broadening its scope.
Most thriving decade (2011–2020). CRM literature witnessed a boom in the third decade, especially in the last two years of this decade. Thus, 257 articles related to the field of CRM were published in different journals only in this third decade.
In summary, we can indicate that CRM publications have grown significantly over the three decades analyzed, because more and more research papers have been published on this topic.
3.3 Author-based citation analysis
Author-based studies have long been one of the most important aspects of bibliometric analysis. This analysis includes the ranking of authors by the number of researches carried out, the citations of their research articles, their evolutions, or the analysis of co-authors’ collaborations. Table 4 shows the five most cited authors (and their specific works) from first decade (1988–2000), second decade (2001–2010), and third decade (2011–2020).
In this analysis, the most cited authors (and their corresponding works) for each decade have been the following:
From the first decade, the most cited authors are: Varadarajan ( 1988 ), with 734 citations; followed by Webb ( 1998 ), with 498; Smith ( 1991 ), with 121; File ( 1998 ), with 110; and Ross (1992), with 15 citations.
In the second decade, Barone (2007) is the most quoted, with 243 citations; followed by Gupta ( 2006a ), with 189; Lafferty ( 2005 ), with 187; Cui ( 2003 ), with 152; and Berglind ( 2005 ), with 103 citations.
During the third decade, Christofi (2020a) has been cited 176 times; Robinson (2012) has 150; Bae (2020), 149; Priporas (2020), 135; and Koschate-Fischer (2012), 129 citations.
In summary, we can highlight that Varadarajan ( 1988 ) is the most cited author of all time, with the first academic paper published on CRM, and serves as a reference for researchers around the world. And by far the next most cited authors are Webb ( 1998 ) and Barone (2007).
3.4 Co-words or co-occurrence analysis
A co-word analysis may be described as “ a content analysis technique that uses patterns of co-occurrence of pairs of items… in a corpus of texts to identify the relationships between ideas within the subject areas ” (He, 1999 , p. 134). Thus, co-words or co-occurrence analysis is a content analysis that connects words in the title of the research paper or abstract. The main idea of the co-word analysis is to connect any identified patterns into a map of contextual space. We also applied this analysis to each decade.
3.4.1 First phase (period 1988–2000)
For the 13 articles published from 1988 to 2000, the co-word analysis identifies four clusters consisting of the following words (with the minimum number of occurrences of keywords defined as 1; out of 26 keywords in this period, 26 met the threshold).
The first cluster includes consumer attitude, market segmentation, marketing strategy, profitability, and social responsibility (as shown in Fig. 2 , red color).
The second cluster deals with cause-related marketing, consumer perceptions, and philanthropy (as shown in Fig. 2 , green color).
The third cluster consists of charitable organizations and crm (as shown in Fig. 2 , blue color).
The last cluster relates to corporate philanthropy (as shown in Fig. 2 , yellow color).
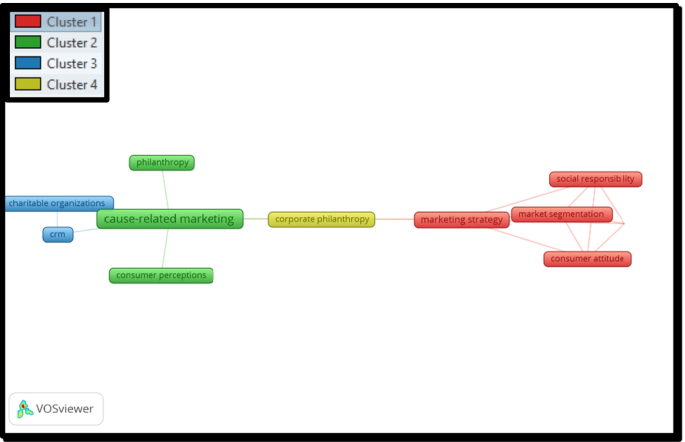
Co-Word analysis for the period 1988 to 2000
In summary, the co-word analysis shows that, for the period from 1988 to 2000, the focused keyword is Cause-Related Marketing . Other important keywords are philanthropy and consumer perception .
3.4.2 Second phase (period 2001–2010)
Based on the selection of 74 articles for the period 2001–2010, the co-word analysis shows a more precise picture than it does in the introductory decade (to narrow down the result, the minimum number of the occurrence of keywords was defined as 2; out of 160 keywords, and 27 meet the reduction criteria).
A notable cluster derived by the co-word analysis (Fig. 3 , red color) consists of the words brand alliances, cause-related marketing, corporate philanthropy, corporate social responsibility, donations, reputation, social responsibility, sponsorship, and work .
A second cluster (Fig. 3 , green color) comprises brand, company, consumer, framework, impact, information, price, responses, and skepticism.
The third cluster (Fig. 3 , blue color) is related to advertising, brand, cause marketing, consumer behavior, marketing, and purchase intention .
The fourth cluster (Fig. 3 , yellow color) consists of choice, corporate images, and purchase intention.
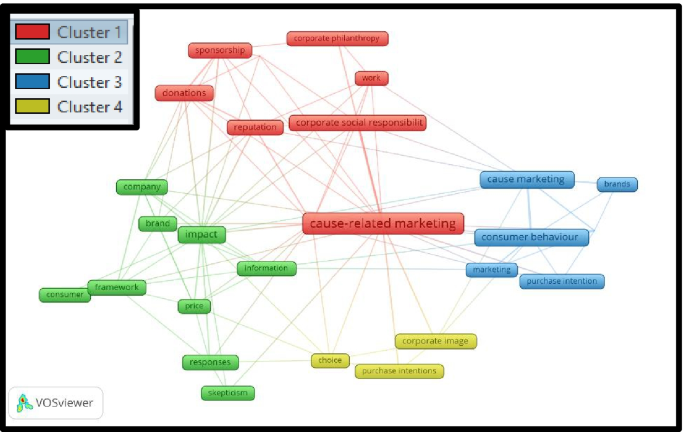
Co-word analysis for the period 2001 to 2010
In summary, the co-word analysis shows that, from 2001 to 2010, the Cause-Related Marketing keyword related to the other striking keywords, such as corporate social responsibility, reputation, corporate image , and purchase intention .
3.4.3 Third phase (period 2011–2020)
Based on the selection of 257 articles for the period 2011–2020, the co-word analysis shows a more precise picture than it does in the previous two decades. To narrow down the result, the minimum number of the occurrence of keywords was defined as 5 (out of 825 keywords, and 40 meet the reduction criteria). The most notable clusters derived from the co-word analysis are:
First cluster (Fig. 4 , red color): it consists of the words attitude, attitudes, brand, choice, consumer responses, credibility, fit, impact, knowledge, motivation, responses, social-responsibility, sponsorship, and sustainability.
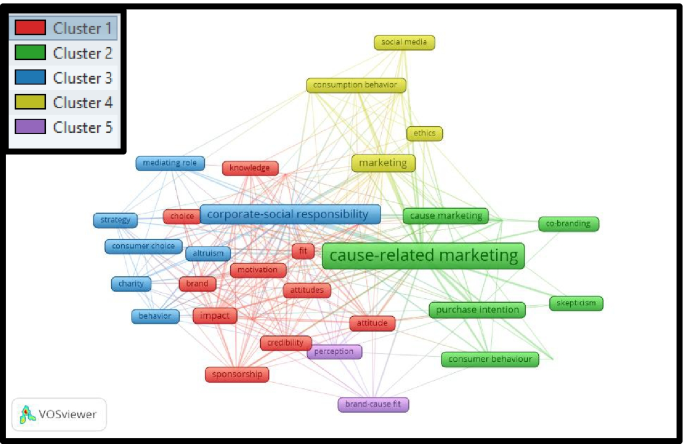
Co-word analysis for the period 2011 to 2020
The second cluster (Fig. 4 , green color) comprises altruism, cause marketing, cause-related marketing, co-branding, consumer behavior, purchase intention, and skepticism.
The third cluster (Fig. 4 , blue color) comprises altruism, behavior, charity, consumer choice, corporate social responsibility, mediating role, and s trategy .
The fourth cluster (Fig. 4 , yellow color) comprises consumption behavior, corporate strategy, ethics, marketing, millennials, and social media.
The fifth cluster (Fig. 4 , purple color) comprises brand-cause fit, corporate social responsibility, and perceptio n.
In summary, the co-word analysis shows that, also for the period from 2011 to 2020, the most focused keyword is Cause-Related Marketing . Other significant keywords are ethics , purchase intention , consumer behavior , and attitudes .
Consequently, the whole co-word analysis shows that all around the world, the researchers are focused on the one keyword that is “ Cause-Related Marketing ”, and the other most emphasis keywords are philanthropy and consumer perception , in the first decade; to evolve toward CSR, reputation, corporate image , and purchase intention , in the second decade; and finally focused on ethics, purchase intention, consumer behavior , and attitudes , in the third decade.
3.5 Co-citation analysis
A co-citation analysis is described as “ the scholarly contributions of authors who are frequently co-cited are likely to embody similar or related concepts ” (Nerur et al., 2008 , p. 321). Co-citation analysis can explain how referential frameworks of the Cause-Related Marketing field at different stages of its development affected evolutions in its general construction.
3.5.1 First phase for the period 1988–2000
Based on the co-citation analysis, for the period 1988-2000 (Fig. 5 ), it has been seen that there is predominately one cluster with a total 58 authors distributed in one cluster namely cluster – 1 with red color (minimum of the documents for an author should 1 and minimum citation of an author should be 1).
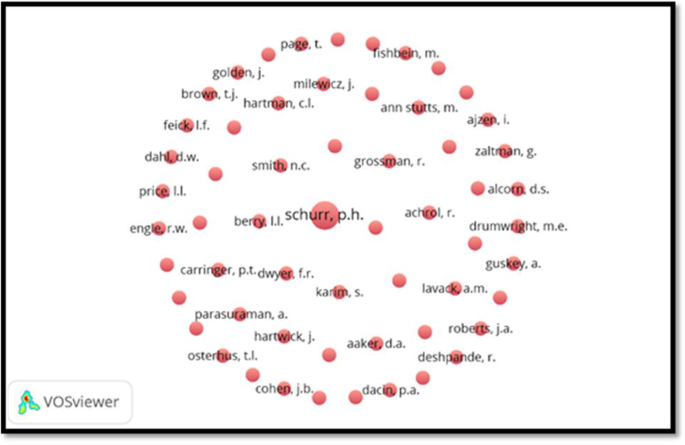
Co-citation analysis of authors for the period 1988–2000
There is a wide variety of authors cited in the papers on CRM in the first decade (but only once). Schurr is the only author who receives 2 citations in this decade.
3.5.2 Second phase for the period 2001–2010
According to co-citation analysis for the period 2001-2010 (Fig. 6 ), it has been noted that there are predominately two clusters with a total 1502 authors (minimum citation of an author should be 10 and the maximum citation of the author should be 24).
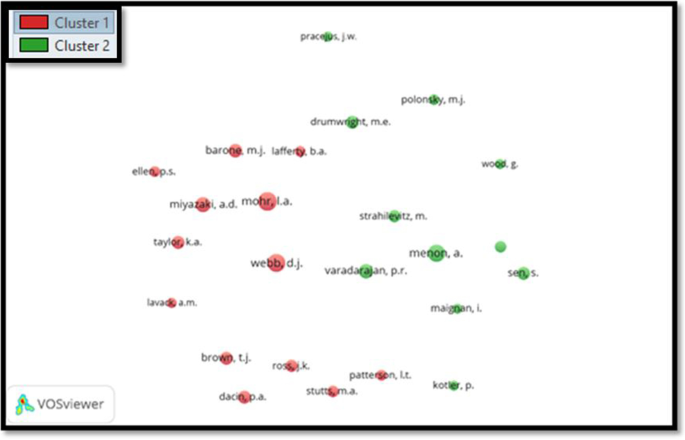
Co-citation analysis of authors for the period 2001–2010
In this analysis, we selected five top co-authors who have a high citation, such as Mohr (34 citations), Webb (32 citations), Menon (30 citations), Miyazaki (24 citations), and Varadarajan (21 citations).
3.5.3 Third phase for the period 2011–2020
According to co-citation analysis for the period 2011-2020 (Fig. 7 ), it has been noted that there are predominately four clusters, with a total of 10,108 authors and 193 thresholds (minimum of the documents for an author should be 20 and minimum citation of an author should be 5; the maximum citation of the author should be 200).
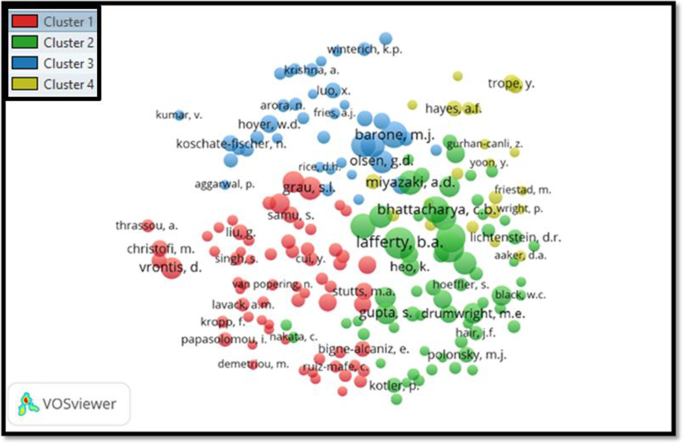
Co-citation analysis of authors for the period 2011–2020
In this analysis, we selected the five top co-authors who had the highest citation, such as Lafferty (200 citations), Mohr (176 citations), Webb (167 citations), Barone (136 citations), and Bhattacharya (136 citations).
In summary, the co-citation analysis shows that Lafferty is the most co-cited author in all time. And the next most co-cited authors are Mohr and Webb.
3.6 Cross-cultural analysis
Cultural norms and beliefs have a significant impact on shaping people’s perceptions, attitudes, and behavior (Steenkamp, 2001 ). Lavack and Kropp ( 2003 ) identified the research gap of cross-cultural studies in the field of CRM. Hence, they conducted the first cross-cultural research in the field of CRM by including four countries from different regions such as Australia (Oceania), Canada (North America), Korea (East Asia) and Norway (Europe), and investigated the consumers’ role values towards the CRM. Since the third decade, more researchers have been participating and collaborating in cross-cultural studies. Table 5 details transversal research that has studied CRM comparing different countries.
According to the cross-sectional analysis, the nation has a different background of consumer and corporate cultures that varies from country to country. Sekaran ( 1983 , p. 68) defined it as “ Culturally normed behavior and patterns of socialization could often stem from a mix of religious beliefs, economic and political exigencies and so on. Sorting these out in a clear-cut fashion would be extremely difficult, if not totally impossible ”. Therefore, the scholars are taking more consideration in cross-cultural CRM study from the second decade. In this study, Table 5 shows that researchers from USA (i.e., North America) and South Korea (i.e., East Asia) studied together two times on culture analysis, one times with India (i.e., South Asia), and one time with Poland (i.e., Europe), one times with Philippines (i.e., East Asia) as well as China (i.e., East Asia). In addition, Italian researchers (i.e., Europe) studied one time on culture analysis with Japan (i.e., East Asia) and one time with Brazil (i.e., South America). Furthermore, India (i.e., South Asia) collaborated with Philippines (i.e., East Asia). Cross-cultural analysis in Table 5 shows that the researchers worked on four different cultures analysis rather than two cultures (i.e., Lavack & Kropp, 2003 ; and Schyvinck & Willem, 2019 ).
3.7 Country-wise development of CRM literature
Figure 8 reports regional (i.e., country-wise) participation of different researchers in the development of CRM literature. As this concept was introduced in the USA (Varadarajan and Menon, 1988 ), the studies from the first and second decades usually belonged to this geographical region. Thus, most of the research in CRM literature was published by researchers from US Universities: 92% of the contributions in the first decade (i.e., 12 research articles), and 40% (i.e., 30 research articles) in the second decade. However, some British researchers also contributed to CRM literature in the second decade, with 11% (i.e., 8 research articles) share of total CRM publications. Nevertheless, in the third decade, most of the CRM literature was published by Asian researchers. Hence, Indian researchers, with 9% (i.e., 24 research articles), and Taiwanese scholars, with 5% (i.e., 12 research articles), jointly published almost 14% of the articles in that decade. Although the American contributions fell to 33% (but only in relative terms, since in absolute terms they reached 85 research articles), their overall contribution remains the highest of all countries. And participation of British scholars was 6% (i.e., 15 research articles) in the third decade. In this Fig. 8 , we observed that the USA research publications from every decade are very extensive, in comparison to other countries.
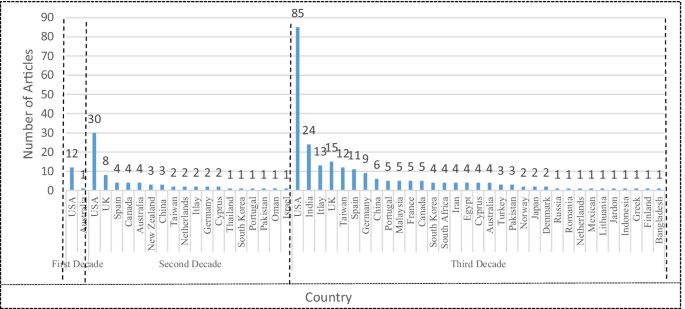
List of cited Country-Wise Development of CRM Literature. Source: Own Elaboration
3.8 Methodological development in CRM literature
Methodological development in CRM literature is graphically shown in Fig. 9 . It is observed that most research work is employed by experimental design. In the first decade (1988–2000), researchers focused on qualitative or quantitative research in the field of CRM; whereas a mixed-method approach has been used in the second (2001–2010) and third decade (2011–2020). In this analysis, we observe that, in general, quantitative studies significantly outnumber qualitative studies, especially in the third decade.
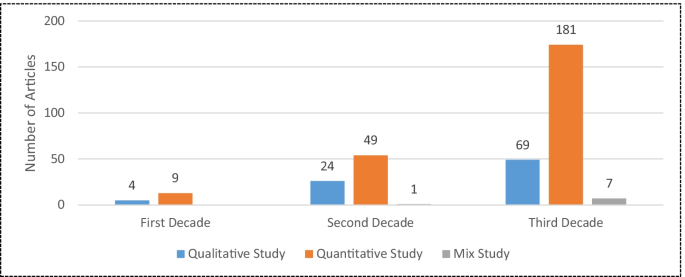
Methodological development in CRM Literature. Source: Own Elaboration
3.9 Role of journal in development of CRM literature
Although a total of 141 journals have published articles explaining the concept of CRM from different perspectives (see Appendix 2, Table 8 ), only six journals published more than ten CRM papers. These journals are International Marketing Review (26), International Review on Public and Nonprofit Marketing (20), Journal of Nonprofit & Public Sector Marketing (17), International Journal of Nonprofit and Voluntary Sector Marketing (15), Journal of Business Ethics (13), and Journal of Business Research (10). In the field of CRM, almost 100 journals have been published only a single article since its conceptualization in 1988 (they are also shown in Appendix 2, Table 8 ).
There are two indicators to measure the scientific influence of scholarly journals, such as Journal Citation Reports and Scimago Journal & Country Rank. This study only considers the Scimago Journal & Country Rank because there are more research articles in this rank, which divides the set of journals into four quartiles (i.e., Q1, Q2, Q3, and Q4). According to Scimago Journal & Country Rank (SJR), we observed that 51 journals publishing about CRM are Q1, 36 are Q2, 16 are Q3, and 5 are Q4. On the other hand, 8 journals are not included yet in any Quartil. and 25 journals are not in this index.
According to Persson et al. ( 2009 ), for the bibliographical data, we used BibExcel, which presents co-occurrence of references in the bibliographic of research articles. Therefore, in this study we find that five most cited journals by each decade, from the first decade (1988–2000), are: Journal of Marketing (1 document; cited in 734 articles), Journal of Public Policy & Marketing (1 document; cited in 498 articles), Journal of Consumer Marketing (1 document; cited in 121 articles), Journal of Business Ethics (1 document; cited in 110 articles), and Journal of the Academy of Marketing Science (2 document; cited in 51 articles). From the second decade (2001–2010): Journal of Consumer Marketing (3 documents; cited in 369 articles), Journal of Business Research (3 documents; cited in 280 articles), Journal of Retailing (1 document; cited in 243 articles), Journal of Nonprofit and Public Sector Marketing (11 documents; cited in 215 articles), and Journal of Advertising (5 documents; cited in 172 articles). And from the third decade (2011–2020): Journal of Marketing Review (25 documents; cited in 842 articles), Journal of Business Ethics (11 documents; cited in 458 articles), International Journal of Nonprofit and Voluntary Sector Marketing (9 documents; cited in 407 articles), International Journal of Advertising (8 documents; cited in 347 articles), and Journal of Marketing (2 documents; cited in 244 articles).
For this analysis, we observed that the percentage of the most cited paper, published in the Journal of Marketing , dropped due to the introduction of different journals, such as Journal of Marketing Review , International Journal of Advertising , or International Journal of Nonprofit and Voluntary Sector Marketing . But, on the other hand, the Journal of Business Ethics has increased the citations.
4 Discussion
This research provides an inclusive review of the systematic literature with respect to three decades: the introductory decade (1988–2000), the emerging decade (2001–2010), and the thriving decade (2011–2020).
In this study, we observed that North American researchers are more involved in Cause-Related Marketing. This may be due to the importance that CRM has had in the USA since its inception, and the acceptance that CRM has had among American companies and consumers. Such as Cone ( 2010 ) showed, 88% of the American consumers supported the cause, 85% of the consumers had a good image of the company or product supporting a noble cause and cared about it, and 90% of the consumers demanded companies to find the right cause to support. More recently, another research also studied that 87% of American consumers would purchase a CRM product if the company supported the charitable cause (business2community, 2020 ).
The graphical presentation of the Time-Wise Development (see Fig. 1 ) shows 13 articles published until 2000 (first decade), 74 articles from 2001 to 2010 (second decade), and 257 articles from 2011 to 2020 (third decade). Natarajan et al. ( 2016 , p. 248) and Thomas et al. ( 2020 , p. 5) verified almost similar findings of the time-wise development from 1988 to 2016. But after that, the research on Cause-Related Marketing has abruptly increased in the last two years (2019–2020). We noticed that, in 2020, the researchers are more actively involved in the CRM field than the previous years to publish the research articles.
As observed in Fig. 8 , the academicians and researchers from 37 different countries have significantly contributed to CRM studies. A large portion of CRM studies are conducted in two regions (i.e., North America and Europe). Thomas et al. ( 2020 ) shows similar results. Asian (i.e., Indian and Taiwanese) researchers have taken more interest in CRM and they have been publishing more and more articles since the third decade. On the other hand, we also noticed that the CRM topic was first introduced in Western societies (with Christian tradition). But after the first decade and during the second decade, CRM studies were also introduced in Muslim countries, such as Pakistan (1 research article) and Oman (1 research article). From the third decade, the researchers also explored other Muslim countries, such as Malaysia (5 research articles), Iran (4 research articles), Egypt (4 research articles), Turkey (3 research articles), Pakistan (3 research articles), Jordan (1 research article), Indonesia (1 research article), and Bangladesh (1 research article). So, in summary, we can highlight that researchers have been exploring the Muslim world in the field of CRM after the second decade.
And about the methodological development in CRM literature (see Fig. 9 ), the researchers have used more quantitative studies, compared to qualitative studies. Thomas et al. ( 2020 , p. 7) also found a similar result. Thus, the trend seems to be for quantitative studies to continue to predominate over qualitative ones in the coming years, although mixed methods are experiencing slight growth. However, the combination of both types of studies, qualitative and quantitative, could offer more complete studies on CRM.
Lastly, Table 6 presents the journals involvement to publish CRM research articles. In our study, the key publications journals are International Marketing Review, and International Review on Public and Nonprofit Marketing. Our results have been slightly different from Thomas et al. ( 2020 )‘s and Natarajan et al. ( 2016 )‘s. These researchers found that the Journal of Nonprofit & Public Sector Marketing was the one that had published more research articles on this topic. But probably this difference is because they only considered up to 2016.
Our research also discovered different results from previous studies with respect to databases, partly due to the number of databases considered and the greater number of years analyzed in our study.
5 Conclusion
Cause-Related Marketing (CRM) is considered as an initiative that involves a donation to a specific cause, at a specific period of time, usually with a limited donation amount, and which depends on product sales and consumer behavior.
Therefore, the main objective of this study was to provide a comprehensive systematic review of the literature on CRM, categorizing each article by time-wise development, country-wise development, methodological development, and role of journals. Cross-cultural analysis and bibliometric analysis were also included, as a new contribution of this research, in comparison to previous studies.
The main studies have been classified in three decades, which present significant differences. In the introductory decade (1990–2000), the field of CRM was introduced with limited published articles with the role of CRM in two different regions, such as North America, and Oceania.
First three Journals such as International Marketing Review, International Review on Public and Nonprofit Marketing, or Journal of Nonprofit and Public Sector Marketing, play a starring role to publish CRM research papers. In the emerging decade (2001–2010), researchers explored more regions, such as East Asia, South Asia, and the Middle East. In this time frame, mix approach studies and cross-cultural studies were introduced for the first time in the field of CRM. And in the most thriving decade (2011–2020), scholars analyzed CRM in two more regions, such as North Africa and Sub-Saharan Africa. In this era, more scholars were interested in collaborating with other geographical regions such as North America and Europe. The number of published papers on CRM grew significantly.
However, this current study has some limitations. First, this research considered only two keywords: “Cause-Related Marketing” and “Cause Marketing”. Thus, other terms might be also considered, such as “Social Cause” or “Cause–brand alliance”. Secondly, the selection of the studies was limited only to the peer-reviewed journal research articles published in English. Maybe research articles in other languages could be also interesting. Thirdly, this current study just focuses on the full-text journal papers. Abstracts, theses, working papers, and conference proceedings were ignored. Fourthly, this study has used a limited number of databases to find the research articles: SAGE Journals, JSTOR, Emerald Insight, Springer, Wiley Online Library, Elsevier, Taylor & Francis Online, and Google Scholar. Other databases, such as EBSCO and ABI/INFORM, could have been also considered.
Anyway, we also found different gaps in CRM research, so further research could be developed in these aspects. First, most academic scholars have largely focused on the developed countries, such as the USA and the UK, and less in developing countries (especially in the first and second decades). Although studies about CRM in developing countries increased in the third decade, the gap still exists. More studies are needed about developing countries because the researchers may find different results. In addition, more studies are also required to compare developed and developing countries, because researchers could find different interesting outcomes about CRM campaigns.
Secondly, the growing popularity of the internet and social media could be more considered by the companies, which could focus on digital marketing. Therefore, consumers could be more involved in a digital CRM campaign (Handa & Gupta, 2020 ). Only few studies have been conducted in this area, so the gap still exists, both in developing and developed countries.
Thirdly, various studies are conducted on the cross-culture context. But more research is needed to investigate the cross-cultural context, comparing developing and developed countries, and also Western and Eastern countries. Causes and consumer preferences or attitudes could be different from one country to another country.
Fourthly, few studies have been conducted in the mix approach (including qualitative and quantitative studies). More research is required for a better understanding of the mixed methodological approach in CRM. The most common and well-known approaches to mixing methods are Triangulation Design, Embedded Design, the Explanatory Design, and the Exploratory Design. These methodologies could be discussed in CRM programs.
Fifthly, profit and non-profit organizations depend on each other in CRM campaigns. When both organizations develop CRM strategies, they can acquire risk. Few studies have been conducted on profit and non-profit organizations with CRM programs; therefore, this also needs to be discussed.
Finally, and according to Chéron et al. ( 2012 ), consumers positively view those CRM campaigns that take place for extended periods of time, and they might be disappointed with short duration campaigns. Thus, time frame of the CRM campaign can have a significant impact on the consumers’ perception. Consequently, the campaign’s time duration is another factor that is needed to be more discussed by researchers.
Except in 2009, the only year with a slight decrease compared to the previous year (− 0.3%) but recovered in the following year.
In 2000, Enron was the seventh-largest corporation by growth revenue in the gas and utility market in the United States territory. At the end of 2001, Enron started to face financial strategy manipulation. Consequently, on 2nd December 2001, Enron declared bankruptcy because of the fraudulent activity of corporate governance.
The ISO standard also consists of the ISO 14001 (Environmental Management System) and ISO 9001 (Quality Management System).
Carroll ( 1979 ) presented the “Carroll’s CSR Pyramid Theory”, which consisted of the economic, legal, ethical, and philanthropic lines.
Elkington (1994) presented the “Triple Bottom Line Theory”, which explained the economic, social, and environmental lines.
Freeman (1984) introduced the “Stakeholder Theory”, which described the role and participation of stakeholders to enchance CSR.
Matten and Crane (2005) described “the role of the corporation in administering citizenship rights for individuals”.
Adkins, S. (1999). Cause-related marketing: Who cares wins . Boston, MA: Butterworth-Heinemann.
Google Scholar
Adkins, S. (2007). Cause related marketing : Routledge.
Adomaviciute, K., Bzikadze, G., Cherian, J., & Urbonavicius, S. (2016). Cause-related marketing as a commercially and socially oriented activity: What factors influence and moderate the purchasing? Engineering Economics, 27 (5), 578–585.
Article Google Scholar
Ahluwalia, A., & Bedi, M. (2015). Cause related marketing: A win-win approach (a conceptual framework). Asia Pacific Journal of Research, 1 (XV), 177–185.
Aria, M., & Cuccurullo, C. (2017). Bibliometrix: An R-tool for comprehensive science mapping analysis. Journal of Informetrics, 11 (4), 959–975.
Aupperle, K., Hatfield, J. D., & Carroll, A. B. (1983). Instrument development and application in corporate social responsibility. Paper presented at the academy of management proceedings.
Bae, M. (2017). Matching cause-related marketing campaign to culture. Asian Journal of Communication, 27 (4), 415–432.
Barnes, N. G. (1998). Partners in profits: Small businesses move slowly into cause-related marketing. Journal of Small Business Strategy, 9 (1), 47–55.
Basil, D. Z., & Herr, P. M. (2003). Dangerous donations? The effects of cause-related marketing on charity attitude. Journal of Nonprofit & Public Sector Marketing, 11 (1), 59–76.
Bautista Jr., R., Jeong, L. S., & Pandey, S. (2020). Will cause-related marketing affect the American and Filipino college Students' purchase intention? Asia-Pacific Social Science Review, 20 (4).
Beise-Zee, R. (2013). Cause-related marketing. Encyclopedia of Corporate Social Responsibility, 321–326.
Bennett, R. (2002). Corporate perspectives on cause related marketing. Journal of Nonprofit & Public Sector Marketing, 10 (1), 41–59.
Bergkvist, L., & Taylor, C. R. (2016). Leveraged marketing communications: A framework for explaining the effects of secondary brand associations. AMS review, 6 (3), 157–175.
Berglind, M., & Nakata, C. (2005). Cause-related marketing: More buck than bang? Business Horizons, 48 (5), 443–453.
Bhattacharyya, S. S., Sahay, A., Arora, A. P., & Chaturvedi, A. (2008). A toolkit for designing firm level strategic corporate social responsibility (CSR) initiatives. Social Responsibility Journal.
Bloom, P. N., Hoeffler, S., Keller, K. L., & Meza, C. E. B. (2006). How social-cause marketing affects consumer perceptions. MIT Sloan Management Review, 47 (2), 49.
Boenigk, S., & Schuchardt, V. (2013). Cause-related marketing campaigns with luxury firms: An experimental study of campaign characteristics, attitudes, and donations. International Journal of Nonprofit and Voluntary Sector Marketing, 18 (2), 101–121.
Bowen, H. (1953). Bowen, HR,(1953), Social Responsibilities of the Businessman: New York: Harper & Row.
Business2community (2020), “The Rise of Cause Marketing”, available at: https://www.business2community.com/social-business/the-rise-of-cause-marketing-02286159 (accessed January 4, 2021).
Carringer, P. T. (1994). Not just a worthy cause: Cause-related marketing delivers the goods and the good. American Advertising, 10 (1), 16–19.
Carroll, A. B. (1979). A three-dimensional conceptual model of corporate performance. Academy of Management Review, 4 (4), 497–505.
Carroll, A. B. (1991). The pyramid of corporate social responsibility: Toward the moral management of organizational stakeholders. Business Horizons, 34 (4), 39–48.
Carroll, A. B. (2008). A history of corporate social responsibility: Concepts and practices. The Oxford handbook of corporate social responsibility, 1.
Chaabane, A. M., & Parguel, B. (2016). The double-edge effect of retailers’ cause-related marketing: When scepticism cools the warm-glow effect. International Journal Retail & Distribution Management .
Chang, C.-T. (2012). Missing ingredients in cause-related advertising: The right formula of execution style and cause framing. International Journal of Advertising, 31 (2), 231–256.
Chang, C.-T., & Chu, X.-Y. M. (2020). The give and take of cause-related marketing: Purchasing cause-related products licenses consumer indulgence. Journal of the Academy of Marketing Science, 48 (2), 203–221.
Chéron, E., Kohlbacher, F., & Kusuma, K. (2012). The effects of brand-cause fit and campaign duration on consumer perception of cause-related marketing in Japan. Journal of consumer marketing .
Choi, S., Lee, S., & Friske, W. (2018). The effects of featured advertising and package labeling on sustainability of cause-related marketing (CRM) products. Sustainability, 10 (9), 3011.
Christofi, M., Kaufmann, H. R., Vrontis, D., & Leonidou, E. (2013). Cause-related marketing and strategic agility: An integrated framework for gaining the competitive advantage. World Review of Entrepreneurship, Management and Sustainable Development, 9 (4), 518–542.
Christofi, M., Leonidou, E., & Vrontis, D. (2015). Cause–related marketing, product innovation and extraordinary sustainable leadership: The root towards sustainability. Global Business and Economics Review, 17 (1), 93–111.
Cone, C. L., Feldman, M. A., & DaSilva, A. T. (2003). Causes and effects. Harvard Business Review, 81 (7), 95–101.
Cone (2010). “2010 Cause Evolution Study”, available at https://www.conecomm.com/research-blog/2010-cause-evolution-study (accessed January 4, 2021).
Cosgrave, D., & O'Dwyer, M. (2020). Ethical standards and perceptions of CRM among millennial consumers. International Marketing Review .
Cui, Y., Trent, E. S., Sullivan, P. M., & Matiru, G. N. (2003). Cause-related marketing: How generation Y responds. International Journal of Retail & Distribution Management .
Deb, M., & Amawate, V. (2019). Extending the knowledge on cause-related marketing (CrM) campaign with focus on skepticism. VINE Journal of Information and Knowledge Management Systems.
Docherty, S., & Hibbert, S. (2003). Examining company experiences of a UK cause-related marketing campaign. International Journal of Nonprofit and Voluntary Sector Marketing, 8 (4), 378–389.
Du, L., Hou, J., & Huang, Y. (2008). Mechanisms of power and action for cause-related marketing. Baltic Journal of Management .
Elkington, J. (2001). The triple bottom line for 21st century business. The Earthscan reader in business and sustainable development, 20–43.
Endacott, R. W. J. (2004). Consumers and CRM: A national and global perspective. Journal of consumer marketing .
Engage for food (2021): Official website. Retrieved from https://engageforgood.com/iegs-growth-cause-marketing/ (25 th July 2021)
Ferraris, A., Del Giudice, M., Grandhi, B., & Cillo, V. (2019). Refining the relation between cause-related marketing and consumers purchase intentions: A cross-country analysis. International Marketing Review.
File, K. M., & Prince, R. A. (1998). Cause related marketing and corporate philanthropy in the privately held enterprise. Journal of Business Ethics, 17 (14), 1529–1539.
Fromherz, K. (2006). Cause-related marketing. American Nurseryman, 203 (6), 46.
Galan-Ladero, M. M. (2012). Variables that influence attitude toward cause-related marketing and determinants of satisfaction and loyalty in the ‘solidarity purchase’. International Review on Public and Nonprofit Marketing, 9 (2), 199–200.
Galan-Ladero, M. M., Galera-Casquet, C., Valero-Amaro, V., & Barroso-Mendez, M. J. (2013a). Sustainable, socially responsible business: The cause-related marketing case. A review of the conceptual framework. Journal of Security and Sustainability Issues, 2 (4), 35–46.
Galan-Ladero, M. M., Galera-Casquet, C., & Wymer, W. (2013b). Attitudes towards cause-related marketing: Determinants of satisfaction and loyalty. International Review on Public and Nonprofit Marketing, 10 (3), 253–269.
Gregory, G., Ngo, L., & Miller, R. (2019). Branding for non-profits: Explaining new donor decision-making in the charity sector. Journal of Product & Brand Management .
Grolleau, G., Ibanez, L., & Lavoie, N. (2016). Cause-related marketing of products with a negative externality. Journal of Business Research, 69 (10), 4321–4330.
Guerreiro, J., Rita, P., & Trigueiros, D. (2016). A text mining-based review of cause-related marketing literature. Journal of Business Ethics, 139 (1), 111–128.
Gupta, S., & Pirsch, J. (2006a). The company-cause-customer fit decision in cause-related marketing. Journal of Consumer Marketing.
Gupta, S., & Pirsch, J. (2006b). A taxonomy of cause-related marketing research: Current findings and future research directions. Journal of Nonprofit & Public Sector Marketing, 15 (1–2), 25–43.
Hajjat, M. M. (2003). Effect of cause-related marketing on attitudes and purchase intentions: The moderating role of cause involvement and donation size. Journal of Nonprofit & Public Sector Marketing, 11 (1), 93–109.
Handa, M., & Gupta, S. (2020). Digital cause-related marketing campaigns: Relationship between brand-cause fit and behavioural intentions. Journal of Indian Business Research, 12 (1), 63–78.
Hawkens, G., & Stead, J. (1996). Who chooses? The family and their buying decisions. The influence of partnership between companies and charities on family purchasing decisions . NCH Action for Children.
Hawkins, R. (2015). Shifting conceptualizations of ethical consumption: Cause-related marketing in India and the USA. Geoforum, 67 , 172–182.
He. (1999). Knowledge discovery through co-word analysis.
He, H., Chao, M. M., & Zhu, W. (2019). Cause-related marketing and employee engagement: The roles of admiration, implicit morality beliefs, and moral identity. Journal of Business Research, 95 , 83–92.
Heald, M. (1957). Management's responsibility to society: The growth of an idea. The Business History Review, 375–384.
Heald, M. (1970). The social responsibilities of business: Company and community 1900–1960 . Transaction Publishers.
Heidarian, E. (2019). The impact of trust propensity on consumers’ cause-related marketing purchase intentions and the moderating role of culture and gender. Journal of International Consumer Marketing, 31 (4), 345–362.
IEG (2018). “Cause Sponsorship Spending To Total $2.14 Billion In 2018”, available at: https://www.sponsorship.com/Latest-Thinking/Sponsorship-Infographics/Cause-Sponsorship-Spending-To-Total-$2-14-Billion.aspx (accessed July 26, 2021).
IEG (2019). “Sponsorship spending of causes to grow 4.6% in 2019”, available at: https://www.sponsorship.com/Latest-Thinking/Sponsorship-Infographics/Sponsorship-Spending-of-Causes-to-Grow-4-6%2D%2Din-201.aspx (accessed January 4, 2021).
IEG (2020). “ Executive Summary” , available at: https://www.sponsorship.com/Latest-Thinking/Sponsorship-Infographics/Executive-Summary-page.aspx (accessed July 26, 2021).
Imas, A. (2014). Working for the “warm glow”: On the benefits and limits of prosocial incentives. Journal of Public Economics, 114 , 14–18.
Jahdi, K. (2014). Cause-related marketing (CaRM) and corporate social responsibility (CSR). Social Responsibility Journal.
Jeong, H. J., & Kim, J. (2020). Benefits of cause-related marketing for companies and nonprofits: Focusing on the roles of self-corporate congruency and issue involvement. International Review on Public and Nonprofit Marketing, 17 , 317–330.
Jones, T. M. (1980). Corporate social responsibility revisited, redefined. California Management Review, 22 (3), 59–67.
Jung, M. J., Naughton, J. P., Tahoun, A., & Wang, C. (2018). Do firms strategically disseminate? Evidence from corporate use of social media. The Accounting Review, 93 (4), 225–252.
Kim, Y. J., & Lee, W. N. (2009). Overcoming consumer skepticism in cause-related marketing: The effects of corporate social responsibility and donation size claim objectivity. Journal of Promotion Management, 15 (4), 465–483.
Kim, J.-E., & Johnson, K. K. (2013). The impact of moral emotions on cause-related marketing campaigns: A cross-cultural examination. Journal of Business Ethics, 112 (1), 79–90.
Kotler, P., Hessekiel, D., & Lee, N. (2012). Good works!: Marketing and corporate initiatives that build a better world... And the bottom line . John Wiley & Sons.
Book Google Scholar
Kotler, P., & Lee, N. (2005). Corporate social responsibility - doing the most good for your company “, John Wiley & Sons. INC, New Jersey.
Kramer, M. R., & Porter, M. (2011). Creating shared value (Vol. 17).
Kumar, P., Singh, S. K., Pereira, V., & Leonidou, E. (2020). Cause-related marketing and service innovation in emerging country healthcare. International Marketing Review.
La Ferle, C., Kuber, G., & Edwards, S. M. (2013). Factors impacting responses to cause-related marketing in India and the United States: Novelty, altruistic motives, and company origin. Journal of Business Research, 66 (3), 364–373.
Lafferty, B. A., & Goldsmith, R. E. (2005). Cause–brand alliances: Does the cause help the brand or does the brand help the cause? Journal of Business Research, 58 (4), 423–429.
Lafferty, B. A., Lueth, A. K., & McCafferty, R. (2016). An evolutionary process model of cause-related marketing and systematic review of the empirical literature. Psychology & Marketing, 33 (11), 951–970.
Lantos, G. P. (2001). The boundaries of strategic corporate social responsibility. Journal of consumer marketing.
Larson, B. V., Flaherty, K. E., Zablah, A. R., Brown, T. J., & Wiener, J. L. (2008). Linking cause-related marketing to sales force responses and performance in a direct selling context. Journal of the Academy of Marketing Science, 36 (2), 271–277.
Lavack, A. M., & Kropp, F. (2003). A cross-cultural comparison of consumer attitudes toward cause-related marketing. Social Marketing Quarterly, 9 (2), 3–16.
Lee Thomas, M., Mullen, L. G., & Fraedrich, J. (2011). Increased word-of-mouth via strategic cause-related marketing. International Journal of Nonprofit and Voluntary Sector Marketing, 16 (1), 36–49.
Liston-Heyes, C., & Liu, G. (2010). Cause-related marketing in the retail and finance sectors: An exploratory study of the determinants of cause selection and nonprofit alliances. Nonprofit and Voluntary Sector Quarterly, 39 (1), 77–101.
Liston-Heyes, C., & Liu, G. (2013). A study of non-profit organisations in cause-related marketing. European Journal of Marketing.
Liu, G. (2013). Impacts of instrumental versus relational centered logic on cause-related marketing decision making. Journal of Business Ethics, 113 (2), 243–263.
Liu, G., & Ko, W.-W. (2011). An analysis of cause-related marketing implementation strategies through social alliance: Partnership conditions and strategic objectives. Journal of Business Ethics, 100 (2), 253–281.
Liu, G., & Ko, W. W. (2014). An integrated model of cause-related marketing strategy development. AMS review, 4 (3–4), 78–95.
Liu, Z., Yin, Y., Liu, W., & Dunford, M. (2015). Visualizing the intellectual structure and evolution of innovation systems research: A bibliometric analysis. Scientometrics, 103 (1), 135–158.
Manojkumar, S. S., & Sharma, D. M. S. (2018). An empirical study to measure the effect of brand loyalty and skepticism on purchase intention with reference to cause related Marketing in Ahmedabad City. Journal of Management , 5(2).
Mullen, J. (1997). Performance-based corporate philanthropy: How" giving smart" can further corporate goals. Public Relations Quarterly, 42 (2), 42.
Natarajan, T., Balasubramaniam, S. A., & Jublee, D. I. (2016). A journey of cause related marketing from 1988 to 2016. International Journal of Business and Management, 11 (11), 247–263.
Nerur, S. P., Rasheed, A. A., & Natarajan, V. (2008). The intellectual structure of the strategic management field: An author co-citation analysis. Strategic Management Journal, 29 (3), 319–336.
Nowak, L. I., & Clarke, T. K. (2003). Cause-related marketing: Keys to successful relationships with corporate sponsors and their customers. Journal of Nonprofit & Public Sector Marketing, 11 (1), 137–149.
Persson, O., Danell, R., & Schneider, J. W. (2009). How to use Bibexcel for various types of bibliometric analysis. Celebrating scholarly communication studies: A Festschrift for Olle Persson at his 60th birthday, 5 , 9-24.
Poje, T., & Groff, M. Z. (2021). Mapping ethics education in accounting research: A bibliometric analysis. Journal of Business Ethics, 1–22.
Polonsky, M. J., & Macdonald, E. K. (2000). Exploring the link between cause-related marketing and brand building. International Journal of Nonprofit and Voluntary Sector Marketing, 5 (1), 46–57.
Polonsky, M. J., & Speed, R. (2001). Linking sponsorship and cause related marketing. European Journal of Marketing.
Polonsky, M. J., & Wood, G. (2001). Can the overcommercialization of cause-related marketing harm society? Journal of Macromarketing, 21 (1), 8–22.
Pracejus, J. W., Olsen, G. D., & Brown, N. R. (2003). On the prevalence and impact of vague quantifiers in the advertising of cause-related marketing (CRM). Journal of Advertising, 32 (4), 19–28.
Pringle, H., & Thompson, M. (1999). Brand spirit: how cause-related marketing builds brands . Chichester: John Wiley and Sons.
Pringle, H., & Thompson, M. (2001). How cause-related marketing builds brands. Chichester, New York: Weinheim, Brisbane, Singapore and Toronto: John Wiley & Sons.
Ptacek, J. J., & Salazar, G. (1997). Enlightened self-interest: Selling business on the benefits of cause-related marketing. Nonprofit World, 15 , 9–9.
Royd-Taylor, L. (2007). Cause-related marketing: A new perspective on achieving campaign objectives amongst fast moving consumer goods. Strategic Change, 16 (1–2), 79–86.
Runté, M., Basil, D. Z., & Deshpande, S. (2009). Cause-related marketing from the nonprofit's perspective: Classifying goals and experienced outcomes. Journal of Nonprofit & Public Sector Marketing, 21 (3), 255–270.
Sabri, O. (2018). The detrimental effect of cause-related marketing parodies. Journal of Business Ethics, 151 (2), 517–537.
Samu, S., & Wymer Jr., W. W. (2001). Nonprofit-business alliance model: Formation and outcomes. Journal of Nonprofit & Public Sector Marketing, 9 (1–2), 45–61.
Santoro, G., Bresciani, S., Bertoldi, B., & Liu, Y. (2019). Cause-related marketing, brand loyalty and corporate social responsibility: A cross-country analysis of Italian and Japanese consumers. International Marketing Review.
Schyvinck, C., & Willem, A. (2019). From cause-related marketing strategy to implementation in professional basketball organizations: A matter of alignment. European Sport Management Quarterly, 19 (1), 58–79.
Sekaran, U. (1983). Methodological and theoretical issues and advancements in cross-cultural research. Journal of International Business Studies, 14 (2), 61–73.
Smith, S. M., & Alcorn, D. S. (1991). Cause marketing: A new direction in the marketing of corporate responsibility. Journal of consumer marketing.
Srivastava, R. (2020). For brand alliance social or cause related marketing which one is better? A study in emerging markets. International Journal of Nonprofit and Voluntary Sector Marketing, 25 (2), e1656.
Steenkamp, J. B. E. (2001). The role of national culture in international marketing research. International Marketing Review.
Stumpf, M., & Teufl, I. (2014). Empirische Untersuchung des Einsatzes von cause related marketing cause related marketing (pp. 97–115). Springer.
Svensson, G., & Wood, G. (2007). Cause related marketing? Commercialism or altruism: Finding the balance? International Journal of Electronic Customer Relationship Management, 1 (3), 231–241.
Svensson, G., & Wood, G. (2011). A model of cause-related marketing for “profit-driven” and “non-profit” organizations. European Business Review.
Tangari, A. H., Folse, J. A. G., Burton, S., & Kees, J. (2010). The moderating influence of consumers' temporal orientation on the framing of societal needs and corporate responses in cause-related marketing campaigns. Journal of Advertising, 39 (2), 35–50.
Thamaraiselvan, N., Arasu, B. S., & Inbaraj, J. D. (2017). Role of celebrity in cause related marketing. International Review on Public and Nonprofit Marketing, 14 (3), 341–357.
Thomas, M. L., Fraedrich, J. P., & Mullen, L. G. (2011). Successful cause-related marketing partnering as a means to aligning corporate and philanthropic goals: An empirical study. Academy of Marketing Studies Journal, 15 (2), 113.
Thomas, S., Kureshi, S., & Vatavwala, S. (2020). Cause-related marketing research (1988–2016): An academic review and classification. Journal of Nonprofit & Public Sector Marketing, 32 (5), 488–516.
Till, B. D., & Nowak, L. I. (2000). Toward effective use of cause-related marketing alliances. Journal of Product & Brand Management.
Vaidyanathan, R., Aggarwal, P., & Kozłowski, W. (2013). Interdependent self-construal in collectivist cultures: Effects on compliance in a cause-related marketing context. Journal of Marketing Communications, 19 (1), 44–57.
Van den Brink, D., Odekerken-Schröder, G., & Pauwels, P. (2006). The effect of strategic and tactical cause-related marketing on consumers' brand loyalty. Journal of consumer marketing.
Varadarajan, P. R., & Menon, A. (1988). Cause-related marketing: A coalignment of marketing strategy and corporate philanthropy. Journal of Marketing, 52 (3), 58–74.
Vrontis, D., Thrassou, A., Christofi, M., Shams, R., & Czinkota, M. R. (2020). Cause-related marketing in international business: What works and what does not? International Marketing Review.
Walton, C. C. (1967). Corporate social responsibilities . Wadsworth Publishing Company.
Wang, Y. (2014). Individualism/collectivism, charitable giving, and cause-related marketing: A comparison of Chinese and Americans. International Journal of Nonprofit and Voluntary Sector Marketing, 19 (1), 40–51.
Webb, D. J., & Mohr, L. A. (1998). A typology of consumer responses to cause-related marketing: From skeptics to socially concerned. Journal of Public Policy & Marketing, 17 (2), 226–238.
Woo, H., Kim, S., & Childs, M. L. (2019). Is this for our sake or their sake? Cross-cultural effects of message focus in cause-related marketing. International Marketing Review.
Woodroof, P. J., Deitz, G. D., Howie, K. M., & Evans, R. D. (2019). The effect of cause-related marketing on firm value: A look at Fortune’s most admired all-stars. Journal of the Academy of Marketing Science, 47 (5), 899–918.
Yun, J. T., Duff, B. R., Vargas, P., Himelboim, I., & Sundaram, H. (2019). Can we find the right balance in cause-related marketing? Analyzing the boundaries of balance theory in evaluating brand-cause partnerships. Psychology & Marketing, 36 (11), 989–1002.
Zupic, I., & Čater, T. (2015). Bibliometric methods in management and organization. Organizational Research Methods, 18 (3), 429–472.
Download references
Open Access funding provided thanks to the CRUE-CSIC agreement with Springer Nature.
Author information
Authors and affiliations.
University of Extremadura, Badajoz, Spain
Hina Yaqub Bhatti, M. Mercedes Galan-Ladero & Clementina Galera-Casquet
Riphah International University, Lahore, Pakistan
Hina Yaqub Bhatti
You can also search for this author in PubMed Google Scholar
Corresponding author
Correspondence to M. Mercedes Galan-Ladero .
Additional information
Publisher’s note.
Springer Nature remains neutral with regard to jurisdictional claims in published maps and institutional affiliations.
Rights and permissions
Open Access This article is licensed under a Creative Commons Attribution 4.0 International License, which permits use, sharing, adaptation, distribution and reproduction in any medium or format, as long as you give appropriate credit to the original author(s) and the source, provide a link to the Creative Commons licence, and indicate if changes were made. The images or other third party material in this article are included in the article's Creative Commons licence, unless indicated otherwise in a credit line to the material. If material is not included in the article's Creative Commons licence and your intended use is not permitted by statutory regulation or exceeds the permitted use, you will need to obtain permission directly from the copyright holder. To view a copy of this licence, visit http://creativecommons.org/licenses/by/4.0/ .
Reprints and permissions
About this article
Bhatti, H.Y., Galan-Ladero, M.M. & Galera-Casquet, C. Cause-related marketing: a systematic review of the literature. Int Rev Public Nonprofit Mark 20 , 25–64 (2023). https://doi.org/10.1007/s12208-021-00326-y
Download citation
Received : 30 March 2021
Accepted : 21 November 2021
Published : 08 January 2022
Issue Date : March 2023
DOI : https://doi.org/10.1007/s12208-021-00326-y
Share this article
Anyone you share the following link with will be able to read this content:
Sorry, a shareable link is not currently available for this article.
Provided by the Springer Nature SharedIt content-sharing initiative
- Cause-Related Marketing (CRM)
- Corporate Social Responsibility (CSR)
- Systematic Literature Review (SLR)
- Bibliometric Analysis
- Find a journal
- Publish with us
- Track your research
How To Conduct Marketing Research That Improves Business Outcomes

Would you send marketing emails about retirement home options to people who have just graduated college? Or run beer commercials during Saturday morning cartoons? Probably not, because the people who would see those emails and ads are not the audience for those products.
Marketing research helps you target the best audiences for your product and identify the types of campaigns that will appeal to them. Observing demographic trends and competitor strategies will provide you with valuable insights that can innovate and expand your product offerings.
Depending on their budgets and requirements, every organization will conduct their market research uniquely. However, they generally include the following steps (though they may differ in name or approach):
- Identify your target audience
- Analyze your competitors’ marketing activities
- Find opportunities for stronger markets
- Evaluate engagement
- Identify problems and create solutions
- Run tests based on your findings
- Restructure your marketing efforts based on your results
1. Identify Your Target Audience
Say you have a coffee brand and you want to maximize your marketing dollars. Targeting the entirety of the coffee-drinking population is too wide-reaching to be an effective strategy, and you’ll end up wasting money trying to engage people who are unlikely to want your product. Fans of frozen, heavily sweetened coffee drinks aren’t likely to resonate with an advertisement for a simple, unsweetened shot of espresso, after all.
Start identifying your target audience by using primary data (also known as direct data) and secondary data. Focus groups, surveys, interviews, and questionnaires are forms of primary data that give you control over who you talk to and the questions you ask.
There are plenty of sources you can use for secondary research , such as census data, articles and reports in industry publications, and online databases. You can also search and monitor social media platforms to see what people are saying about products and services similar to yours.
After analyzing and segmenting this data, you can create buyer personas —fictional representations of your target markets that incorporate their demographic information, pain points, and preferred methods of communication. These will be useful guides to help you develop your strategies and messaging.
2. Analyze Your Competitors’ Marketing Activities
See what other businesses in your industry are doing by conducting a competitive analysis to get an overview of the landscape and identify trends and tactics you may be able to leverage in your own efforts. Study what their ads are like and where they appear. Who do they seem to be targeting? What social media platforms do they use? What kinds of content do they create, and how often? Do they get a lot of mentions and pieces in the press? What is their overall brand profile and market share?
3. Find Opportunities for Stronger Markets
Consider functionality, features, pricing, performance, customer service, and brand reputation. Brainstorm strategies to position your product or service in a way that will appeal to an underserved or overlooked target market. One way you might do this is by offering a low-cost option targeted toward people facing socioeconomic hardship. There may even be an opportunity to provide a complementary product or service, which is not only a kind and generous thing to do, but also a great opportunity for brand awareness and perception.
4. Evaluate Engagement
Different audiences prefer to engage with brands on different platforms. Some consumers spend a lot of time on X or Instagram, while others prefer reading newsletters via email. The social management platform Sprout Social has some useful information on the demographics of various social media platforms .
Content formats play a part in engagement as well. Some audiences like reading copy, while others prefer short-form video. If your target audience isn’t very active in certain channels, pull back and invest in the ones they do use.
5. Identify Problems and Create Solutions
If you find areas where your efforts are falling short and you’re not seeing the response or conversion rate you’d hoped for, check whether the problem might be your messaging, the format, timing, or other factors. For example, you might be using an ineffective channel.
If you’re struggling with consumer engagement or creating products that aren’t optimized for your market, you may be facing a value proposition issue. The Pace University MBA offers Value Creation as an elective or Marketing Management course that will teach you how to find innovative opportunities for consumer engagement through the use of technology.
6. Run Tests Based on Your Findings
Rather than spend a lot of marketing dollars on a tweak that might not work, test it first. A/B testing is everywhere in digital marketing—these tests are easy and cheap to run, and they can deliver valuable results quickly.
For example, try A/B tests by sending emails with two different subject headings or creating two slightly different landing pages to see which one gets more engagement.
7. Restructure Your Marketing Efforts to Better Connect to Your Target Audience
You may only need to make small changes, such as emphasizing a certain feature of your product in messaging or focusing more on social channels with a wider audience of younger users, such as TikTok. Or you may need to completely rethink your marketing strategy or even adapt your product or service to meet customer needs and preferences. In our hypothetical coffee example, you may find that your target audience prizes convenience. That may lead you to offer your coffee in pods and adapt your messaging to highlight the ease and speed of making your coffee in a pod machine.
The Value of Marketing Research
Marketing research is a critical part of strategic planning. It helps business leaders make informed decisions that minimize risks, maximize returns, and ensure that resources are allocated efficiently.
Marketing isn’t static, especially in a crowded and competitive business landscape. Businesses must engage in market research activities consistently to A) add or refine products and services and B) monitor how their target market might be changing. Customer tastes and needs evolve, and external factors such as new technologies , economic developments, and changes in cultural norms can completely shift the market. Well-executed marketing research can help ensure you always know who your target audience is, what they want, and what you need to do to attract them.
How an MBA Prepares You To Make an Impact
An MBA can provide you with an advanced understanding of business operations, including marketing, to prepare you for leadership and management roles. Pace University’s MBA program offers a Marketing Management concentration that enables you to dive deeper into marketing research, including learning frameworks for gathering and analyzing information. All of the marketing-focused courses in the program will integrate research in some way, and the concentration overall is dedicated to teaching you how to create and improve the performance of your marketing campaigns. Armed with this expertise, you can make an ongoing impact on organizational objectives as well as the trajectory of your career over a lifetime.
About the Pace University Online MBA and Lubin School of Business
The Pace University online Master of Business Administration (MBA) gives professionals a hands-on learning experience to master the ins and outs of business theory through real world application. This 100% online program has been handcrafted by expert faculty to ensure that students are career-ready upon completion. Choose a Corporate Finance, Marketing Management, or general business concentration.
For over a century, Pace University’s Lubin School of Business has been preparing professionals for fulfilling careers in business. From responsive professor communication to hands-on, project-based learning, we do everything we can to help students succeed.
Pace’s Lubin School of Business has dual accreditation by the Association to Advance Collegiate Schools of Business (AACSB) International, an elite distinction shared by fewer than 2% of business schools in the world. With graduates thriving in companies like AIG, Nickelodeon, L’Oreal, Tesla, and many others, Pace equips students with vital business knowledge, while also creating innovative thinkers that companies want.
Connect with us
- [email protected]
- (800) 874-PACE --> view directory
Related Categories
- Marketing Strategy
- Five Forces
- Business Lists
- Competitors
- Marketing and Strategy ›
Causal Marketing - Meaning, Importance & Example
What is causal marketing.
Causal marketing is when an organization is involved with a charitable cause whether directly or indirectly. We tend to see that the primary customers and stakeholders who identify with such causes start identifying with the organisation as well, and this known as causal marketing. It is more like establishing a cause and effect relationship. Causal marketing helps consumers relate to the organization they are attached to in ways which are more like cause and effect relationships.
Causal as a term refers to something indicating the cause of or constituting the cause of. If a phenomenon is causal it may be involving causation or be marked by cause and effect. Sometimes causal phenomenon generates cause and effect relationships too, and they also showcase the same at times. The cause and effect relationship are very much highlighted. The term causal can be better understood by looking at the term called causal marketing.
Importance of Causal Marketing
In today’s age, consumers want to associate with something good. They want all the attributes in relation to them to be associated with good causes too. Therefore, it is an imperative for an organization which wants to relate on a very deep level with its consumers, to practice causal marketing. Causal marketing can be a large range on advertising, activities or campaigns that allow organizations to showcase support for a good cause more specifically a charitable cause.
This is the way causal marketing is a win-win situation for the organization. It ensures uplifts brand image, a greater brand recall and an effective impression. Causal marketing or social marketing leads to greater business and also leads to the very pivotal and positive change in society. There is no particular financial gain in all cases of causal marketing but there is a certain positive image and impression left in the minds of the consumers associated with that organization. The gain is of a residual type, doing good and therefore having a better and positive brand image. Causal marketing also fulfils the engagement criterion. We see that nowadays engagement is of paramount importance and leads to greater number of consumers. It not only leads to increased awareness about the organization but a genuine potential for increased sales. It also creates a certain competitive advantage over other competitors, and acts as a differentiator for existing and new customers. An improved brand image and greater brand awareness are complimentary benefits, very important for a new entrant and/or an organization wanting to make a comeback or trying to revive.
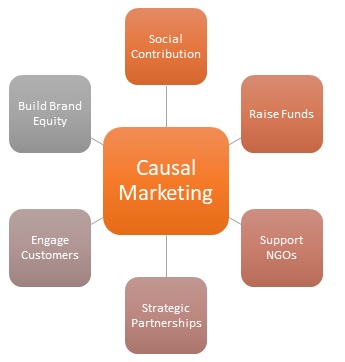
Causal Marketing helps a company to:
1. Social Contribution as a part of CSR
2. Raise Funds
3. Support NGOs & have strategic partnerships
4. Engage Customers
5. Build Brand Equity
6. Drive business & sales
- Causal Research
- E-Marketing
- 5 Cs of Marketing
- 4 Ps of Marketing
- Marketing Function
- Marketing Mix
Advantages of Causal Marketing
Some advantages of causal marketing are:
1. Strengthening of relationships with customers by widespread awareness due to causal marketing campaigns.
2. Increased sales with time, due to increased consumer engagement.
3. Improved brand image
4. Greater brand awareness and customer retention
5. Merger of commercial interests and core competency of the organization
6. Improved customer and employee loyalty
Disadvantages of Causal Marketing
Certain drawbacks of causal marketing are:
1. Efforts unaligned with natural cause: A company may seem to associate with any good cause but something that doesn’t align with their natural cause, i.e., to have better performance leads to an opposite effect on the consumers’ mind. The organizations effort can look unaligned with the cause hence becoming an uneasy situation for the organization.
2. Causes which aren’t very much known are mostly to have no effect on consumers. It becomes very difficult for them to relate to something they are not aware about. For example a NGO which is not registered in the parent country of the organization is something the consumers of hat organization will not be able to relate or associate with.
3. The association is not merely writing a cheque for the cause, the company needs to be involved using its core competencies, lining the cause with its natural manner. The firm or organization might look cheap and may likely have a damaged image in the minds of the consumers which is the exact opposite outcome of what they are trying to make happen.
Example of Causal Marketing
We will take an example of the Red Kettle campaign for causal marketing. In this campaign, we see, the Salvation Army Southern territory partnered with DipJar in order to improve the red kettle marketing campaign. The Red kettle campaign earlier was placing red color buckets outside stores or coffee shops to donate funds. But due to the association with Dipjar, Red kettle campaign was taken to a different level where now one could donate with credit cards. The mechanism would to dip the credit cards in a dipjar and donate $1 towards the cause. The Salvation Army saw a gap in their process and filled it with the association with Dipjar. Red kettle Dip jars now placed at the café billing counters and right at the doors of supermarkets and their billing counters. The swiping makes donation effortless and easy. This shows us how an essential gap was filled up with a causal marketing approach.
Hence, this concludes the definition of Causal Marketing along with its overview.
This article has been researched & authored by the Business Concepts Team . It has been reviewed & published by the MBA Skool Team. The content on MBA Skool has been created for educational & academic purpose only.
Browse the definition and meaning of more similar terms. The Management Dictionary covers over 1800 business concepts from 5 categories.
Continue Reading:
- Sales Management
- Market Segmentation
- Brand Equity
- Positioning
- Selling Concept
- Marketing & Strategy Terms
- Human Resources (HR) Terms
- Operations & SCM Terms
- IT & Systems Terms
- Statistics Terms

What is MBA Skool? About Us
MBA Skool is a Knowledge Resource for Management Students, Aspirants & Professionals.
Business Courses
- Operations & SCM
- Human Resources
Quizzes & Skills
- Management Quizzes
- Skills Tests
Quizzes test your expertise in business and Skill tests evaluate your management traits
Related Content
- Inventory Costs
- Sales Quota
- Quality Control
- Training and Development
- Capacity Management
- Work Life Balance
- More Definitions
All Business Sections
- Business Concepts
- SWOT Analysis
- Marketing Strategy & Mix
- PESTLE Analysis
- Five Forces Analysis
- Top Brand Lists
Write for Us
- Submit Content
- Privacy Policy
- Contribute Content
- Web Stories

Thank you for visiting nature.com. You are using a browser version with limited support for CSS. To obtain the best experience, we recommend you use a more up to date browser (or turn off compatibility mode in Internet Explorer). In the meantime, to ensure continued support, we are displaying the site without styles and JavaScript.
- View all journals
- Explore content
- About the journal
- Publish with us
- Sign up for alerts
- Open access
- Published: 12 June 2024
Hybrid working from home improves retention without damaging performance
- Nicholas Bloom ORCID: orcid.org/0000-0002-1600-7819 1 na1 ,
- Ruobing Han ORCID: orcid.org/0000-0001-9126-5503 2 na1 &
- James Liang 3 , 4
Nature ( 2024 ) Cite this article
29k Accesses
482 Altmetric
Metrics details
- Research management
Working from home has become standard for employees with a university degree. The most common scheme, which has been adopted by around 100 million employees in Europe and North America, is a hybrid schedule, in which individuals spend a mix of days at home and at work each week 1 , 2 . However, the effects of hybrid working on employees and firms have been debated, and some executives argue that it damages productivity, innovation and career development 3 , 4 , 5 . Here we ran a six-month randomized control trial investigating the effects of hybrid working from home on 1,612 employees in a Chinese technology company in 2021–2022. We found that hybrid working improved job satisfaction and reduced quit rates by one-third. The reduction in quit rates was significant for non-managers, female employees and those with long commutes. Null equivalence tests showed that hybrid working did not affect performance grades over the next two years of reviews. We found no evidence for a difference in promotions over the next two years overall, or for any major employee subgroup. Finally, null equivalence tests showed that hybrid working had no effect on the lines of code written by computer-engineer employees. We also found that the 395 managers in the experiment revised their surveyed views about the effect of hybrid working on productivity, from a perceived negative effect (−2.6% on average) before the experiment to a perceived positive one (+1.0%) after the experiment. These results indicate that a hybrid schedule with two days a week working from home does not damage performance.
Similar content being viewed by others
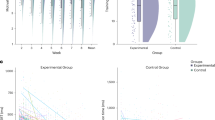
Cognitive control training with domain-general response inhibition does not change children’s brains or behavior
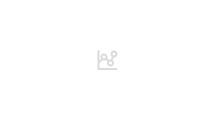
The impact of artificial intelligence on employment: the role of virtual agglomeration
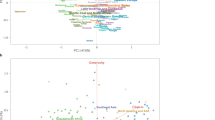
Principal component analysis
Working from home (WFH) surged after the COVID-19 pandemic, with university-graduate employees typically WFH for one to two days a week during 2023 (refs. 2 , 6 ). Previous causal research on WFH has focused on employees who are fully remote, usually working on independent tasks in call-centre, data-entry and helpdesk roles. This literature has found that the effects of fully remote working on productivity are often negative, which has resulted in calls to curtail WFH 5 , 6 , 7 , 8 , 9 , 10 , 11 , 12 . However, there are two challenges when it comes to interpreting this literature. First, more than 70% of employees WFH globally are on a hybrid schedule. This group comprises more than 100 million individuals, with the most common working pattern being three days a week in the office and two days a week at home 2 , 8 , 9 . Second, most employees who are regularly WFH are university graduates in creative team jobs that are important in science, law, finance, information technology (IT) and other industries, rather than performing repetitive data-entry or call processing tasks 10 , 11 .
This paper addresses the gap in previous studies in two key ways. First, it uses a randomized control trial to examine the causal effect of a hybrid schedule in which employees are allowed to WFH two days per week. Second, it focuses on university-graduate employees in software engineering, marketing, accounting and finance, whose activities are mainly creative team tasks.
Our study describes a randomized control trial from August 2021 to January 2022, which involved 1,612 graduate employees in the Airfare and IT divisions of a large Chinese travel technology multinational called Trip.com. Employees were randomized by even or odd birthdays into the option to WFH on Wednesday and Friday and come into the office on the other three days, or to come into the office on all five days.
We found that in the hybrid WFH (‘treatment’) group, attrition rates dropped by one-third (mean control = 7.20, mean treat = 4.80, t (1610) = 2.02, P = 0.043) and work satisfaction scores improved (mean control = 7.84, mean treat = 8.19, t (1343) = 4.17, P < 0.001). Employees reported that WFH saved on commuting time and costs and afforded them the flexibility to attend to occasional personal tasks during the day (and catch up in the evenings or weekends). These effects on reduced attrition were significant for non-managerial employees (mean control = 8.59, mean treat = 5.33, t (1215) = 2.23, P = 0.026), female employees (mean control = 9.19, mean treat = 4.18, t (568) = 2.40, P = 0.017) and those with long (above-median) commutes (mean control = 6.00, mean treat = 2.89, t (609) = 1.87, P = 0.062).
At the same time, we found no evidence of a significant effect on employees’ performance reviews, on the basis of null equivalence tests, and no evidence of a difference in promotion rates over periods of up to two years (‘Null results’ section of the Methods ). We did find significant differences in pre-experiment beliefs about the effects of WFH on productivity between non-managers and managers. Before the experiment, managers tended to have more negative views, reporting that hybrid WFH would be likely to affect productivity by −2.6%, whereas non-managers had more positive views (+0.7%) ( t (1313) = −4.56, P < 0.001). After the experiment, the views of managers increased to +1.0%, converging towards non-managers’ views (mean non-manager = 1.62, mean manager = 1.05, t (1343) = −0.945, P = 0.345). This highlights how the experience of hybrid working leads to a more positive assessment of its effect on productivity—consistent with the overall experience in Asia, the Americas and Europe throughout the pandemic, where perceptions of WFH improved considerably 13 .
The experiment
The experiment took place at Trip.com, the third-largest global travel agent by sales in 2019. Trip.com was established in 1999, was quoted on NASDAQ in 2003 and was worth about US$20 billion at the time of the experiment. It is headquartered in Shanghai, with offices across China and internationally, and has roughly 35,000 employees.
In the summer of 2021, Trip.com decided to evaluate the effects of hybrid WFH on the 1,612 engineering, marketing and finance employees in the Airfare and IT divisions, spanning 395 managers and 1,217 non-managers. All experimental participants were surveyed at baseline, with questions on expectations, background and their interest in volunteering for early participation in the experiment. The firm randomized employees with an odd-number birthday (born on the first, third, fifth and so on day of the month) into the treatment group.
Figure 1 shows two pictures of employees working in the office to highlight three points. First, in the second half of 2021, COVID incidence rates in Shanghai were so low that employees were neither masked nor socially distanced at the office. Although the COVID pandemic had led to lockdowns in early 2020 and during 2022, during the second half of 2021, Shanghai employees were free to come to work, and typically were unmasked in the office. Second, employees worked in modern open-plan offices in desk groupings of four or six colleagues from the same team, reflecting the importance of collaboration. Third, the office is a large modern building, similar to many large Asian, European and North American offices.
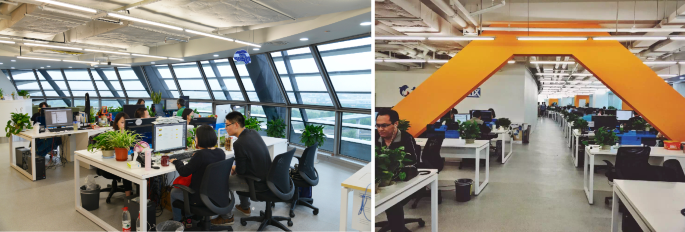
Pictures of Trip.com employees in the office during the experiment. The people in the experimental sample are typically in their mid-30s, and 65% are male. All of them have a university undergraduate degree and 32% have a postgraduate degree, usually in computer science, accounting or finance, at the master’s or PhD level. They have 6.4 years tenure on average and 48% of employees have children (Extended Data Table 1 ).
Effects on employee retention
One key motivation for Trip.com in running the experiment was to evaluate how hybrid WFH affected employee attrition and job satisfaction. The net effect was to reduce attrition over the experiment by 2.4%, which against the control-group base of 7.2% was a one-third (33%) reduction in attrition (mean control = 7.20, mean treat = 4.80, t (1610) = 2.02, P = 0.043). Consistent with this reduction in quit rates, employees in the treatment group also registered more positive responses to job-satisfaction surveys (mean control = 7.84, mean treat = 8.19, t (1343) = 4.17, P < 0.001). Employees were anonymously surveyed on 21 January 2022, and employees in the treatment group showed significantly higher scores on a scale from 0 (lowest) to 10 (highest) in ‘work–life balance’, ‘work satisfaction’, ‘life satisfaction’ and ‘recommendation to friends’, and significantly lower scores in ‘intention to quit’ (Extended Data Table 2 ).
One possible explanation for the lower quit rates in the treatment group is that quit rates in the control group increased because the individuals in this group were annoyed about being randomized out of the experiment. However, quit rates in the same Airfare and IT divisions were 9.8% in the six months before the experiment—higher than the rate for the control group during the experimental period. Quit rates over the experimental period in the two other Trip.com divisions for which we have data (Business Trips and Marketing) were 10.5% and 9.8%—again higher than that for the control group during the experimental period. This suggests that, if anything, the control-group quit rates were reduced rather than increased by the experiment, possibly because some of them guessed (correctly) that the policy would be rolled out to all employees once the experiment ended.
Figure 2 shows the change in attrition rates by three splits of the data. First, we examined the effect on attrition for the 1,217 non-managers and 395 managers separately. We saw a significant drop in attrition of 3.3 percentage points for the non-managers, which against a control-group base of 8.6% is a 40% reduction (mean control = 8.59, mean treat = 5.33, t (1215) = 2.23, P = 0.026). By contrast, there was an insignificant increase in attrition for managers (mean control = 2.96, mean treat = 3.13, t (393) = −0.098, P = 0.922). We also found that non-managers were more enthusiastic before the experiment, with a volunteering rate of 35% (versus 22% for managers), matching the media sentiment that although non-managerial employees are enthusiastic about WFH, many managers are not ( t (1610) = 4.86, P < 0.001).

Data on 1,612 employees’ attrition until 23 January 2022. Top left, all employees. Only 1,259 employees filled out the baseline survey question on commuting length, so the commute-length (two ways) sample is for 1,259 employees. Sample sizes are 820 and 792 for control and treatment; 1,217 and 395 for non-managers and managers; 570 and 1,042 for women and men; and 648 and 611 for short and long commuters, respectively. Two-tailed t -tests for the attrition difference within each group between the control and treatment groups are (difference = 2.40, s.e. = 1.18, confidence interval (CI) = [0.0748, 4.72], P = 0.043) for all employees; (difference = 3.26, s.e. = 1.46, CI = [0.392, 6.12], P = 0.026) for non-managers; (difference = −0.169, s.e. = 1.73, CI = [−3.57, 3.23], P = 0.922) for managers; (difference = 5.01, s.e. = 2.08, CI = [0.915, 9.10], P = 0.017) for women; (difference = 0.997, s.e. = 1.43, CI = [−1.82, 3.81], P = 0.487) for men; (difference = 2.61, s.e. = 1.93, CI = [−1.19, 6.41], P = 0.178) for employees with median (90 min, two-way) or shorter commutes; and (difference = 3.11, s.e. = 1.66, CI = [−0.156, 6.37], P = 0.062) for above-median (90 min, two-way) commuters.
Second, we examined the effect on attrition by total commute length, splitting the sample into people with shorter and longer total commutes on the basis of the median commute duration (two-way commutes of 1.5 h or less versus those exceeding 1.5 h, with 648 and 611 employees, respectively). We found that there was a larger reduction in quit rates (52%) for those with a long commute (mean control = 6.00, mean treat = 2.89, t (609) = 1.87, P = 0.062). The reduction in quit rates was similarly large for employees with a long commute if we instead defined a long commute as a two-way commute time exceeding 2 h (mean control = 7.33, mean treat = 1.89, t (307) = 2.31, P = 0.021). Employees who volunteered to take part in the experiment had longer one-way commute durations (Extended Data Table 3 ; mean non-volunteer = 0.80, mean volunteer = 0.89, t (1257) = −3.68, P < 0.001). This is not surprising given that the most frequently cited benefit of WFH is no commute 1 .
Third, we examined the effect on attrition by gender, examining the 570 female and 1,042 male employees separately. We found that there was a 54% reduction in quit rates for female employees (mean control = 9.2, mean treat = 4.2, t (568) = 2.40, P = 0.017). For male employees, there was an insignificant 16% reduction in quit rates (mean control = 6.15, mean treat = 5.15, t (1040) = 0.70, P = 0.487). This greater reduction in quit rates among female individuals echoes the findings of previous studies 6 , 14 , 15 , 16 , which suggest that women place greater value on remote work than men do. Notably, although the treatment effect of WFH was significantly larger for female employees, volunteers were less likely to be female (mean non-volunteer = 0.37, mean volunteer = 0.32, t (1610) = −2.02, P = 0.043); this might suggest that women have greater concerns about negative career signalling by volunteering to WFH.
Employee performance and promotions
Another key question for Trip.com was the effect of hybrid WFH on employee performance. To assess that, we examined four measures of performance: six-monthly performance reviews and promotion outcomes for up to two years after the start of the experiment, detailed performance evaluations, and the lines of code written by the computer engineers. We also collected self-assessed productivity effects of hybrid working from experimental participants before and after the experiment to evaluate employee perceptions.
Performance reviews are important within Trip.com as they determine employees’ pay and career progression, so are carefully conducted. The review process for each employee is built on formal assessments provided by their managers, co-workers, direct reports and, if appropriate, customers. They are reviewed by employees, collated by managers and by the human resources team, and then discussed between the manager and the employee. This lengthy process takes several weeks, providing a well-grounded measure of employee performance. Although these reviews are not perfect, given their tight link to pay and career development, both managers and employees put a large amount of effort into making these informative measures of performance.
Figure 3 reports the distribution of performance grades for treatment and control employees for the four half-year periods: July to December 2021, January to June 2022, July to December 2022 and January to June 2023. These four performance reviews span a two-year period from the start of the experimental period. Across all review periods, we found no difference in reviews between the treatment and control groups (Extended Data Table 4 and ‘Null results’ section of the Methods ).

Results from performance reviews of 1,507 employees in July–December 2021, 1,355 employees in January–June 2022, 1,301 employees in July–December 2022 and 1,254 employees in January–June 2023. Samples are lower over time owing to employee attrition from the original experimental sample. Two-tailed t -tests for the performance difference within each period between the control and treatment groups, after assigning each letter grade a numeric value from 1 (D) to 5 (A), are (difference = 0.056, s.e. = 0.043, CI = [−0.029, 0.14], P = 0.198) for July–December 2021; (difference = 0.034, s.e. = 0.044, CI = [−0.0529, 0.122], P = 0.440) for January–June 2022; (difference = −0.019, s.e. = 0.046, CI = [−0.11, 0.072], P = 0.677) for July to December 2022; and (difference = 0.046, s.e. = 0.051, CI = [−0.054, 0.146], P = 0.369) for January–June 2023. The null equivalence tests are included in the ‘Null results’ section of the Methods .
Figure 4 reports the distribution of promotion outcomes for the treatment and control employees for the same periods. We see no evidence of a difference in promotion rates across treatment and control employees. This is an important result given the evidence that fully remote working can damage employee development and promotions 14 , 17 , 18 .

Promotion outcomes for 1,522 employees in July–December 2021, 1,378 employees in January–June 2022, 1,314 employees in July–December 2022 and 1,283 employees in January–June 2023. Samples are lower over time owing to employee attrition from the original experimental sample. Two-tailed t -tests for the promotion difference within each period between the control and treatment groups are (difference = −0.86, s.e. = 1.34, CI = [−3.51, 1.74], P = 0.509) for July–December 2021 promotions; (difference = 0.12, s.e. = 0.85, CI = [−1.54, 1.78], P = 0.892) for January–June 2022 promotions; (difference = −0.51, s.e. = 1.12, CI = [−2.72, 1.70], P = 0.651) for July–December 2022 promotions; and (difference = −0.99, s.e. = 1.02, CI = [−2.99, 1.00], P = 0.328) for January–June 2023 promotions. The null equivalence tests are included in the ‘Null results’ section of the Methods .
We also analysed the effects of treatment on performance grades and promotions for a variety of subgroups, including managers, employees with a manager in the treatment group, longer-tenured employees, longer-commuting employees, women, employees with children, computer engineers and those living further away, as well as looking at whether internet speed had any effect. We found no evidence of a difference in response to treatment across these groups (Extended Data Table 5 ).
The experiment also analysed two other measures of employee performance. First, the performance reviews at Trip.com have subcomponents for individual activities such as ‘innovation’, ‘leadership’, ‘development’ and ‘execution’ (nine categories in all) when these are important for an individual employee’s role. We collected these data and analysed these scores for the four six-month performance review periods. We found no evidence of a difference across these nine major categories over the four performance review periods (Extended Data Table 6 ). This indicates that for categories that involve softer skills or more team-focused activities—such as development and innovation—there is no evidence for a material effect of being randomized into the hybrid WFH treatment. Second, for the 653 computer engineers, we obtained data on the lines of code uploaded by each engineer each day. For this ‘lines of code submitted’ measure, we found no difference between employees in the control and treatment groups (Extended Data Fig. 1 and ‘Null results’ section of the Methods ).
Self-assessed productivity
All experiment participants were polled before the experiment in a baseline survey on 29 and 30 July 2021, which included a two-part question on their beliefs about the effects of hybrid WFH on productivity. Employees were asked ‘What is your expectation for the impact of hybrid WFH on your productivity?’, with three options of ‘positive’, ‘about the same’ or ‘negative’. Individuals who chose the answer ‘positive’ were then offered a set of options asking how positive they felt, ranging from [5% to 15%] up to [35% or more], and similarly so for negative choices. For aggregate impacts we took the mid-points of each bin, and 42.5% for >35% and –42.5% for <−35%. Employees were resurveyed with the same question after the end of the experiment on 21 January 2022.
The left panel of Fig. 5 shows that employees’ pre-experimental beliefs about WFH and productivity were extremely varied. The baseline mean was –0.1%, but with widespread variation (standard deviation of 11%). This spread should be unsurprising to anyone who has been following the active debate about the effects of remote work on productivity. At the end-line survey conducted on 21 January 2022, the mean of these beliefs had significantly increased to 1.5%, revealing that the experience of hybrid working led to a small improvement in average employee beliefs about the productivity impact of hybrid working (mean baseline = −0.06%, mean endline = 1.48%, t (2658) = −3.84, P < 0.001). This could be because hybrid WFH saves employees commuting time and is less physically tiring, and, with intermittent breaks between group time and quiet individual time, can improve performance 19 , 20 , 21 , 22 .
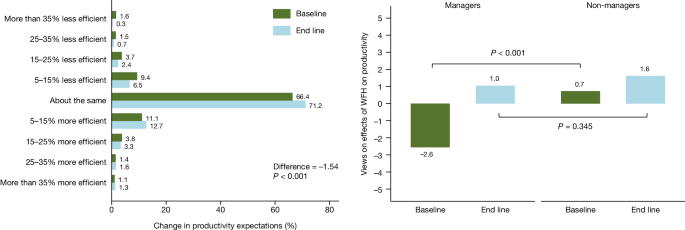
Sample from 1,315 employees (314 managers, 1,001 non-managers) at the baseline and 1,345 employees (324 managers, 1,021 non-managers) at the end line. Two-tailed t -tests for the difference in productivity expectations between baseline and end line, after assigning a numeric value corresponding to the midpoint of the bucket, are (baseline mean = −0.058, end-line mean = 1.48, difference = −1.54, s.e. = 0.40, CI = [−2.33, −0.753], P < 0.001). Two-tailed t -tests for the baseline difference between the productivity expectations of managers and non-managers are (difference = −3.28, s.e. = 0.72, CI = [−4.69, −1.86], P < 0.001), and the t -tests for the end-line difference are (difference = −0.571, s.e. = 0.604, CI = [−1.76, 0.615], P = 0.345).
The right panel of Fig. 5 shows that in the baseline survey, managers were negative about the perceived effect of hybrid work on their productivity, with a mean effect of −2.6%. Non-managers, by contrast, were significantly more positive, at +0.7% in the baseline survey (mean non-manager = 0.7%, mean manager = −2.6%, t (1313) = −4.56, P < 0.001). At the end of the experiment, the views of managers improved to 1.0%, with no evidence of a difference from the non-managers’ mean value of 1.6% (mean non-manager = 1.62%, mean manager = 1.05%, t (1343) = −0.95, P = 0.345). Hence, the experiment led managers to positively update their views about how hybrid WFH affects productivity, and to more closely align with non-managers.
Of note, we saw that employees in the treatment and control groups had similar increases in self-assessed productivity (difference 0.58%, s.d. = 0.59%). Employees from four other divisions in Trip.com were also polled about the productivity impact of hybrid WFH after the end of the experiment in March 2022, with a mean estimate of +2.8% on a sample of 3,461 responses—similar to the 1.5% end line for the experimental sample. This suggests that even close exposure to hybrid WFH is sufficient for employees to change their views, consistent with previous evidence of a positive society-wide shift in perceptions about WFH productivity after the 2020 pandemic 8 .
Once the experiment ended, the Trip.com executive committee examined the data and voted to extend the hybrid WFH policy to all employees in all divisions of the company with immediate effect. Their logic was that each quit cost the company approximately US$20,000 in recruitment and training, so a one-third reduction in attrition for the firm would generate millions of dollars in savings. This was publicly announced on 14 February 2022, with wide coverage in the Chinese media. Since then, other Chinese tech firms have adopted similar hybrid policies 23 .
This highlights how, contrary to the previous causal research focused on fully remote work, which found mostly negative effects on productivity 5 , 6 , 7 , hybrid remote work can leave performance unchanged. This suggests that hybrid working can be profitably adopted by organizations, given its effect on reducing attrition, which is estimated to cost about 50% of an individual’s annual salary for graduate employees 24 . Hybrid working also offers large gains for society by providing a valuable amenity (perk) to employees, reducing commuting and easing child-care 6 , 25 , 26 .
The experiment was conducted in a Chinese technology firm based in Shanghai. Although it might not be possible to replicate these results perfectly in other situations, Trip.com is a large multinational firm with global suppliers, customers and investors. Its offices are modern buildings that look similar to those in many American, Asian and European cities. Trip employees worked 8.6 h per day on average, close to the 8 h per day that is usual for US graduate employees 27 . The business had a large drop in revenue in 2020 (see Extended Data Fig. 4 ), followed by roughly flat revenues through the 2021 experiment period into 2022, so this was not a period of exceptionally fast or slow growth. As such, we believe that these results— that is, the finding that allowing employees to WFH two days per week reduces quit rates and has a limited effect on performance—would probably extend to other organizations. Also, this experiment analysed the effects of working three days per week in the office and two days per week from home. So, our findings might not replicate to all other hybrid work arrangements, but we believe that they could extend to other hybrid settings with a similar number of days in the office, such as two or four days a week. We are not sure whether the results would extend to more remote settings such as one day a week (or less) in the office, owing to potential challenges around training, innovating and culture in fully remote settings.
Finally, we should point out two implications of the experimental design. First, full enrolment into hybrid schemes is important because of concerns that volunteering might be seen as a negative signal about career ambitions. The low volunteer rate among female employees, despite their high implied value (from the large reductions in quit rates observed), is particularly notable in this regard. Second, there is value in experimentation. Before the experiment, managers were net-negative in their views on the productivity impact of hybrid working, but after the experiment, their views became net-positive. This highlights the benefits of experimentation for firms to evaluate new working practices and technologies.
Location and set-up
Our experiment took place at Trip.com in Shanghai, China. In July 2021, Trip.com decided to evaluate hybrid WFH after seeing its popularity amongst US tech firms. The first step took place on 27 July 2021, when the firm surveyed 1,612 eligible engineers, marketing and finance employees in the Airfare and IT divisions about the option of hybrid WFH. They excluded interns and rookies who were in probation periods because on-site learning and mentoring are particularly important for those individuals. Trip.com chose these two divisions as representative of the firm, with a mix of employee types to assess any potentially heterogeneous impacts. About half of the employees in these divisions are technical employees, writing software code for the website, and front-end or back-end operating systems. The remainder work in business development, with tasks such as talking to airlines, travel agents or vendors to develop new services and products; in market planning and executing advertising and marketing campaigns; and in business services, dealing with a range of financial, regulatory and strategy issues. Across these groups, 395 individuals were managers and 1,217 non-managers, providing a large enough sample of both groups to evaluate their response to hybrid WFH.
Randomization
The employees were sent an email outlining how the six-month experiment offered them the option (but not the obligation) to WFH on Wednesday and Friday. After the initial email and two follow-up reminders, a group of 518 employees volunteered. The firm randomized employees with odd birthdays—those born on the first, third, fifth and so on of the month—into eligibility for the hybrid WFH scheme starting on the week of 9 August. Those with even birthdays—born on the second, fourth, sixth and so on of the month—were not eligible, so formed the control group.
The top management at the firm was surprised at the low volunteer rate for the optional hybrid WFH scheme. They suspected that many employees were hesitating because of concerns that volunteering would be seen as a negative signal of ambition and productivity. This is not unreasonable. For example, a previous study 28 found in the US firm they evaluated that WFH employees were negatively selected on productivity. So, on 6 September, all of the remaining 1,094 non-volunteer employees were told that they were also included in the program. The odd-birthday employees were again randomized into the hybrid WFH treatment and began the experiment on the week of 13 September. In this paper we analyse the two groups together, but examining the volunteer and non-volunteer groups individually yields similar findings of reduced quit rates and no impact on performance.
Employee characteristics and balancing tests
Figure 1 shows some pictures of employees working in the office (left side). Employees all worked in modern open-plan offices in desk groupings of four or six colleagues from the same team. By contrast, when WFH, they usually worked alone in their apartments, typically in the living room or kitchen (see Extended Data Fig. 2 ).
The individuals in the experimental sample are typically in their mid-30s. About two-thirds are male, all of them have a university undergraduate degree and almost one-third have a graduate degree (typically a master’s degree). In addition, nearly half of the employees have children (details in Extended Data Table 1 ).
In Extended Data Table 7 we confirm that this sample is also balanced across the treatment and control groups, by conducting a two-sample t -test. The exceptions are from random variation given that the sampling was by even or odd day-of-month birthday—the control sample is 0.5 years older ( P = 0.06), and this is presumably linked to why those in this group have 0.06% more children ( P = 0.02) and 0.4 years more tenure ( P = 0.09).
In Extended Data Table 3 , we examine the decision to volunteer for the WFH experiment. We see that volunteers were significantly less likely to be managers (mean non-volunteer = 0.28, mean volunteer = 0.17, t (1610) = −4.85, P < 0.001) and had longer commute times (hours) (mean non-volunteer = 0.80, mean volunteer = 0.89, t (1257) = 3.68, P < 0.001). Notably, we don’t find evidence of a relationship between volunteering and previous performance scores (mean non-volunteer = 3.81, mean volunteer = 3.81, t (1580) = −0.02, P = 0.985), highlighting, at least in this case, the lack of evidence for any negative (or positive) selection effects around WFH.
Extended Data Fig. 3 plots the take-up rates of WFH on Wednesday and Friday by volunteer and non-volunteer groups. We see a few notable facts. First, take-up overall was about 55% for volunteers and 40% for non-volunteers, indicating that both groups tended to WFH only one day, typically Friday, each week. At Trip.com, large meetings and product launches often happen mid-week, so Fridays are seen as a better day to WFH. Second, the take-up rate even for non-volunteers was 40%, indicating that Trip.com’s suspicion that many employees did not volunteer out of fear of negative signalling was well-founded, and highlighting that amenities like WFH, holiday, maternity or paternity leave might need to be mandatory to ensure reasonable take-up rates. Third, take-up surged on Fridays before major holidays. Many employees returned to their home towns, using their WFH day to travel home on the quieter Thursday evening or Friday morning. Finally, take-up rates jumped for both treatment-group and control-group employees in late January 2022 after a case of COVID in the Shanghai headquarters. Trip.com allowed all employees at that point to WFH, so the experiment effectively ended early on Friday 21 January. The measure of an employee’s daily WFH take-up excludes leave, sick leave or occasions when they cannot come to the office owing to extreme bad weather (typhoon) or to the COVID outbreak in the company.
Null results
To interpret the main null results, we conduct null equivalence tests using the two one-sided tests (TOST) procedure in R (refs. 29 , 30 ). This test required us to specify the smallest effect size of interest (SESOI). For the results pertaining to performance review measures, we use 0.5 as the SESOI. This corresponds to half of a consecutive letter grade increase or decrease, because we had assigned numeric values to performance letter grades in increments of 1, with the lowest letter grade D being 1, and the highest letter grade A being 5. We performed equivalence tests for a two-sample Welch’s t -test using equivalence bounds of ±0.5. The TOST procedure yielded significant results using the default alpha of 0.05 for the tests against both the upper and the lower equivalence bounds for the performance measures for July–December 2021 ( t (1504) = −10.20, P < 0.001)), January–June 2022 ( t (1353) = −10.57, P < 0.001)), July–December 2022 ( t (1299) = 10.34, P < 0.001)) and January–June 2023 ( t (1248) = −8.80, P < 0.001)). The equivalence test is therefore significant, which means we can reject the hypothesis that the true effect of the treatment on performance is larger than 0.5 or smaller than −0.5. So, we interpret the performance effects of the treatment to be actually null on the basis of the SESOI we used, as opposed to no evidence of a difference in performance.
We conducted null equivalence results for the effect of the treatment on promotions using 2 as the SESOI, corresponding to ±2 percentage points (pp) difference in promotion rates. Although we can reject the null hypothesis that the true effect of treatment on promotion is larger than 2 pp or smaller than −2 pp in January–June 2022 ( t (1376) = −2.22, P = 0.013) and July–December 2022 ( t (1306) = 1.33, P = 0.092), we fail to reject the null equivalence hypothesis in July–December 2021 ( t (1513) = 0.83, P = 0.203) and January–June 2023 ( t (1250) = 0.98, P = 0.163). Thus, we interpret the results on promotion as no evidence of a difference between promotion rates across treatment and control employees.
We also conducted the equivalence test for lines of code using 29 lines of code per day as the SESOI, which corresponds to 10% of the mean number of lines of code for the control group. We arrive at this SESOI on the basis of rounding down the productivity effects of previous findings 8 , 10 . We can reject the equivalence null hypothesis for lines of code ( t (92362) = −2.74, P = 0.003)) so we interpret the effect of the treatment as a null effect.
Volunteer versus non-volunteer groups
In the main paper we pool the volunteer and non-volunteer groups. In Extended Data Table 5 we examine the impacts on performance and promotions and we see no evidence of a difference in performance and promotion treatment effects for volunteer versus non-volunteer groups (column 9).
Performance subcategories
The company has a rigorous performance-reviewing process every six months that determines employees’ pay and promotion, so is carefully conducted. The review process for each employee is built on formal reviews provided by their managers, project leaders and sometimes co-workers (peer review). Managers are more like an employee’s direct managers for organizational purposes, but for a particular project, the project leader could be another higher-level employee. In such a case, the manager of the employee would ask that project leader for an opinion on the employee’s contribution to the project. An individual’s overall score is a weighted sum of scores from various subcategories that managers have broad flexibility over defining, because tasks differ across employees, and managers would give a score for each task. For example, an employee running a team themselves will have subcategories around developing their direct reports (leadership and communication), whereas an employee running a server network will have subcategories around efficiency and execution. The performance subcategory data come from the text of the performance review. We first used the most popular Chinese word segmentation package in Python, named Jieba, to identify the most frequent Chinese words from task titles across four performance reviews. We also removed meaningless words and incorporated common expressions such as key performance indicators (‘KPI’), objectives and key results (‘OKR’), ‘rate’ and ‘%’. This process resulted in a total of 236 unique words and expressions. We then manually categorized those most frequent keywords into nine major subcategories (see below) by meanings and relevance. Finally, on the basis of the presence of keywords in the task title, tasks were grouped into the following subcategories:
Communication tasks are those that involve communication, collaboration, cooperation, coordination, participation, suggestion, assistance, organization, sharing and relationships.
Development tasks are those that involve coding or codes, data or datasets, systems, techniques and skills.
Efficiency tasks are those that involve cost reduction, ratios, return on investment (ROI), rate, %, improvement, growth, lifting, adding, optimizing, profit, receiving, gross merchandise value (GMV), OKR, KPI, work and goal.
Execution tasks are those that involve execution, conducting, maintenance, delivery, output, quality, contribution and workload.
Innovation tasks are those that involve development, R&D and innovation.
Leadership tasks are those that involve leadership, managing or management, approval, internal, strategy, coordination and planning.
Learning tasks are those that involve learning, growing, maturing, talent, ability, value competitiveness and personal improvement.
Project tasks are those that involve project, supply, product, business line, cooperation and clients.
Risk tasks are those that involve risk, compliance, supervision, recording and monitoring, safety, rules and privacy.
Data sources
Data were provided by a combination of Trip.com sources, including human resources records, performance reviews and two surveys. All data were anonymized and coded using a scrambled individual ID code, so no personally identifiable information was shared with the Stanford team. The data were drawn directly from the Trip.com administrative data systems on a monthly basis. Gender is collected by Trip.com from employees when they join the company.
The full sample has 1,612 experiment participants, but we have 1,507, 1,355, 1,301 and 1,254 employees, respectively, in the subsamples for the four performance reviews from July–December 2021, January–June 2022, July–December 2022 and January–June 2023. These smaller samples are due to attrition. In addition, for the first performance review in July–December 2021, 105 employees did not have sufficient pre-experiment tenure to support a performance review (they had joined the firm less than three months before the experimental draw). The review text data covers 1,507,1,339,1,290 and 1,246 people, as some employees do have an overall score and review text but do not have additional and task-specific scores. The reason is that these employees do not have the full range of all tasks, so their managers did not write the full review script. For the two surveys, Trip.com used Starbucks vouchers to incentivize response and collected responses from 1,315 employees (314 managers, 1,001 non-managers) at the baseline on the left, and that of 1,345 employees (324 managers, 1,021 non-managers) at the end line.
All tests used two-sided Student t -tests unless otherwise stated. Analysis was run on Stata v17 and v18, R version 4.2.2. Unless stated otherwise, no additional covariates are included in the tests. The null hypothesis for all of the tests excluding null equivalence tests is a coefficient of zero (for example, zero difference between treatment and control).
Inclusion and ethics statement
The design and execution of the experiment was run by Trip.com. No participants were forced to WFH owing to the experiment (the entire firm was, however, forced to WFH during the pandemic lockdown). The treatment sample had the option but not the obligation to WFH on Wednesday or Friday. The experiment was designed, initiated and run by Trip.com. N.B. and R.H. were invited to analyse the data from the experiment, with consent for data collection coming from Trip.com internally. The experiment was exempt under institutional review board (IRB) approval guidelines because it was designed and initiated by Trip.com, before N.B. and R.H. were invited to analyse the data. Only anonymous data were shared with the Stanford team. Trip.com based the experimental design and execution on their previous experience with WFH randomized control trials 17 .
Reporting summary
Further information on research design is available in the Nature Portfolio Reporting Summary linked to this article.
Data availability
The data necessary to reproduce the primary results of this study can be found at https://doi.org/10.7910/DVN/6X4ZZL . These data have been anonymized and split into individual files to ensure that no individual is identifiable. All figures and tables can be replicated using this data.
Code availability
The code necessary to reproduce the primary results of this study can be found at https://doi.org/10.7910/DVN/6X4ZZL .
Working From Home Research: Survey of Workplace Attitudes and Arrangements; https://wfhresearch.com/ (2023).
Aksoy, C. G. et al. Working from Home Around the Globe: 2023 Report. EconPol Policy Brief No. 53 (EconPol, 2023).
McGlauflin, P. JPMorgan CEO Jamie Dimon chides managers who work from home: ‘I don’t know how you can be a leader and not be completely accessible to your people’. Fortune (11 July 2023).
Kelly, J. Goldman Sachs tells employees to return to the office by July 14, as Wall Street pushes back on the work-from-home trend. Forbes (5 May 2021).
Goswami, R. Elon Musk: Working from home is ‘morally wrong’ when service workers still have to show up. CNBC (16 May 2023).
Barrero, J. M., Bloom, N. & Davis, S. J. Why Working from Home Will Stick . National Bureau of Economic Research (NBER) Working Paper No. 28731 (NBER, 2021).
The Economist. The working from home illusion fades. Economist (28 Jun 2023).
Barrero, J. M., Bloom, N. & Davis, S. J. The Evolution of Working from Home . Stanford Institute for Economic Policy Research (SIEPR) Working Paper No. 23-19 (SIEPR, 2023).
Flex Index. The Flex Report Q1 2023 (Scoop Technologies, 2023).
Wuchty, S., Jones, B. F. & Uzzi, B. The increasing dominance of teams in production of knowledge. Science 316 , 1036–1039 (2007).
Article ADS CAS PubMed Google Scholar
Pabilonia, S. W. & Vernon, V. Telework, wages, and time use in the United States. Rev. Econ. Household 20 , 687–734 (2022).
Article Google Scholar
Brucks, M. S. & Levav, J. Virtual communication curbs creative idea generation. Nature 605 , 108–112 (2022).
Aksoy, C. G. et al. Working from home around the world. EconPol Forum 23 , 38–41 (2022).
MathSciNet Google Scholar
Emanuel, N., Harrington, E. & Pallais, A. The Power of Proximity to Coworkers: Training for Tomorrow or Productivity Today? National Bureau of Economic Research (NBER) Working Paper No. 31880 (NBER, 2023).
Goldin, C. Understanding the Economic Impact Of COVID-19 on Women . National Bureau of Economic Research (NBER) Working Paper No. 29974 (NBER, 2022).
Angelici, M. & Profeta, P. Smart-Working: Work Flexibility Without Constraints . CESifo Working Paper No. 8165 (CESifo, 2020).
Bloom, N., Liang, J., Roberts, J. & Ying, Z. J. Does working from home work? Evidence from a Chinese experiment. Q. J. Econ. 130 , 165–218 (2015).
Yang, L. et al. The effects of remote work on collaboration among information workers. Nat. Hum. Behav. 6 , 43–54 (2022).
Article PubMed Google Scholar
Girotra, K., Terwiesch, C. & Ulrich, K. T. Idea generation and the quality of the best idea. Manag. Sci. 56 , 591–605 (2010).
Stroebe, W. & Diehl, M. Why groups are less effective than their members: on productivity losses in idea-generating groups. Eur. Rev. Soc. Psychol. 5 , 271–303 (2011).
Bernstein, E., Shore, J. & Lazear, D. How intermittent breaks in interaction improve collective intelligence. Proc. Natl Acad. Sci. USA 115 , 8734–8739 (2018).
Article ADS CAS PubMed PubMed Central Google Scholar
Choudhury, P., Khanna, T., Makridis, C. A. & Schirmann, K. Is Hybrid Work the Best of Both Worlds? Evidence from a Field Experiment . Harvard Business School Working Paper No. 22-063 (Harvard Business School, 2022).
Dreamteam Payroll. Alibaba’s new welfare plus extra days off work without reducing salary; https://www.dreamteampayrolloutsource.co.th/en/alibaba-welfare-benefit/ (2022).
Allen, D. G. Retaining Talent: A Guide to Analyzing and Managing Employee Turnover (Society for Human Resources Management, 2008).
Mas, A. & Pallais, A. Valuing alternative work arrangements. Am. Econ. Rev. 107 , 3722–3759 (2017).
Wood, S. et al. Satisfaction with one’s job and working at home in the COVID-19 pandemic: a two-wave study. Appl. Psychol. 72 , 1409–1429 (2023).
US Bureau of Labor Statistics. American Time Use Survey; https://www.bls.gov/charts/american-time-use/emp-by-ftpt-job-edu-h.htm .
Emanuel, N. & Harrington, E. Working remotely? Selection, treatment, and the market for remote work. Am. Econ. J. Appl. Econ. (in the press).
Points of significance. Nat. Hum. Behav. 7 , 293–294 (2023).
Lakens, D., Scheel, A. M. & Isager, P. M. Equivalence testing for psychological research: a tutorial. Adv. Meth. Pract. Psychol. Sci. 1 , 259–269 (2018).
Download references
Acknowledgements
We thank the Smith Richardson Foundation for funding; J. Cao, T. Zhang, S. Ye, F. Chen, X. Zhang, Y. He, J. Li, B. Ye and M. Akan for data, advice and logistical support; D. Yilin for research assistance; S. Ayan, S. Buckman, S. Gurung, M. Jackson and P. Lambert for draft feedback; and J. Sun for project leadership.
Author information
These authors contributed equally: Nicholas Bloom, Ruobing Han
Authors and Affiliations
Department of Economics, Stanford University, Stanford, CA, USA
Nicholas Bloom
Shenzhen Finance lnstitute, School of Management and Economics, The Chinese University of Hong Kong, Shenzhen, China
Ruobing Han
National School of Development, Peking University, Beijing, China
James Liang
Trip.com, Shanghai, China
You can also search for this author in PubMed Google Scholar
Contributions
N.B. oversaw the analysis, presented the results and wrote the main drafts of the paper. He was the principal investigator on the research grant supporting the research. R.H. supervised data collection and analysed the data, presented the results and helped to draft the paper. J.L. initiated and designed the study, discussed the results and analysis and facilitated the Trip.com engagement. N.B. and R.H. are co-first authors.
Corresponding authors
Correspondence to Nicholas Bloom , Ruobing Han or James Liang .
Ethics declarations
Competing interests.
No funding was received from Trip.com. J.L. is the co-founder, former CEO and current chairman of Trip.com, with equity holdings in Trip.com. No other co-author has any financial relationship with Trip.com. Neither the results nor the paper was pre-screened by anyone. The experiment was registered with the American Economic Association on 16 August 2021 after the experiment had begun but before N.B. and R.H. had received any data. Only anonymous data were shared with the Stanford team.
Peer review
Peer review information.
Nature thanks Sooyeol Kim and the other, anonymous, reviewer(s) for their contribution to the peer review of this work. Peer reviewer reports are available.
Additional information
Publisher’s note Springer Nature remains neutral with regard to jurisdictional claims in published maps and institutional affiliations.
Extended data figures and tables
Extended data fig. 1 wfh had no effect on lines of code written..
The data coves the experimental period starting on 9 August 2021 for the first wave and 13 September for the second wave, running to 23 January 2022, for both waves. Lines of code submitted per day is available for 653 employees whose primary role was writing code, spanning a total of 95,494 days. Lines are those uploaded to trip.com on a daily basis. Data plotted on a log-2 scale for readability. Reported P value is calculated using a two-sided t -test on the number of code lines and the difference is for control minus treatment. When using log 2 (code lines) the difference has a P value of 0.750 (noting the sample is 27,605 days because of dropping 0 values). When using log 2 (1 + code lines) the difference has a P value of 0.0103, with treatment having the higher average values. The null equivalence tests are included in the ‘Null results’ section of the Methods .
Extended Data Fig. 2 Home (October 2021).
Employees set up basic working environments in their living rooms, studies, or kitchens, and bring back company laptops if necessary.
Extended Data Fig. 3 Take-up rate for WFH treatment and control by volunteer status.
Data for 1,612 employees from 9 August 2021 (volunteers) and 13 September (non-volunteers) to 23 January 2022. Public holidays, personal holidays and excused absence (for example, sick leave) are excluded. Take-up rate is percentage of Wednesday and Friday each week they WFH.
Extended Data Fig. 4 Trip.com revenues.
Trip.com revenues from 2000 to 2023.
Supplementary information
Reporting summary, peer review file, rights and permissions.
Open Access This article is licensed under a Creative Commons Attribution 4.0 International License, which permits use, sharing, adaptation, distribution and reproduction in any medium or format, as long as you give appropriate credit to the original author(s) and the source, provide a link to the Creative Commons licence, and indicate if changes were made. The images or other third party material in this article are included in the article’s Creative Commons licence, unless indicated otherwise in a credit line to the material. If material is not included in the article’s Creative Commons licence and your intended use is not permitted by statutory regulation or exceeds the permitted use, you will need to obtain permission directly from the copyright holder. To view a copy of this licence, visit http://creativecommons.org/licenses/by/4.0/ .
Reprints and permissions
About this article
Cite this article.
Bloom, N., Han, R. & Liang, J. Hybrid working from home improves retention without damaging performance. Nature (2024). https://doi.org/10.1038/s41586-024-07500-2
Download citation
Received : 15 August 2023
Accepted : 30 April 2024
Published : 12 June 2024
DOI : https://doi.org/10.1038/s41586-024-07500-2
Share this article
Anyone you share the following link with will be able to read this content:
Sorry, a shareable link is not currently available for this article.
Provided by the Springer Nature SharedIt content-sharing initiative
By submitting a comment you agree to abide by our Terms and Community Guidelines . If you find something abusive or that does not comply with our terms or guidelines please flag it as inappropriate.
Quick links
- Explore articles by subject
- Guide to authors
- Editorial policies
Sign up for the Nature Briefing newsletter — what matters in science, free to your inbox daily.

We serve the public by pursuing a growing economy and stable financial system that work for all of us.
- Center for Indian Country Development
- Opportunity & Inclusive Growth Institute
Monetary Policy
- Banking Supervision
- Financial Services
- Community Development & Outreach
- Board of Directors
- Advisory Councils
Work With Us
- Internships
- Job Profiles
- Speakers Bureau
- Doing Business with the Minneapolis Fed
Overview & Mission
The ninth district, our history, diversity & inclusion, region & community.
We examine economic issues that deeply affect our communities.
- Request a Speaker
- Publications Archive
- Agriculture & Farming
- Community & Economic Development
- Early Childhood Development
- Employment & Labor Markets
- Indian Country
- K-12 Education
- Manufacturing
- Small Business
- Regional Economic Indicators
Community Development & Engagement
The bakken oil patch.
We conduct world-class research to inform and inspire policymakers and the public.
Research Groups
Economic research.
- Immigration
- Macroeconomics
- Minimum Wage
- Technology & Innovation
- Too Big To Fail
- Trade & Globalization
- Wages, Income, Wealth
Data & Reporting
- Income Distributions and Dynamics in America
- Minnesota Public Education Dashboard
- Inflation Calculator
- Recessions in Perspective
- Market-based Probabilities
We provide the banking community with timely information and useful guidance.
- Become a Member Bank
- Discount Window & Payments System Risk
- Appeals Procedures
- Mergers & Acquisitions (Regulatory Applications)
- Business Continuity
- Paycheck Protection Program Liquidity Facility
- Financial Studies & Community Banking
- Market-Based Probabilities
- Statistical & Structure Reports
Banking Topics
- Credit & Financial Markets
- Borrowing & Lending
- Too Big to Fail
For Consumers
Large bank stress test tool, banking in the ninth archive.
We explore policy topics that are important for advancing prosperity across our region.
Policy Topics
- Labor Market Policies
- Public Policy
Racism & the Economy
Domestic outsourcing is on the rise. what happens when the practice is banned.
June 17, 2024
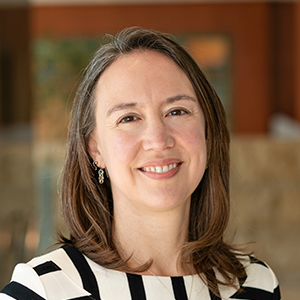
Article Highlights
- Research suggests outsourced workers earn less than non-outsourced workers doing same jobs
- Ban on domestic outsourcing in Mexico increased wages without decreasing employment or output
- Domestic outsourcing may play a role in balance of bargaining power between firms and workers
“What’s disgusting? Union busting! What’s outrageous? Harvard’s wages!” Students at Harvard University chanted these lines in 2001 in an effort to pressure the university to pay all employees who worked on campus a minimum of $10.25 per hour. While almost all workers employed directly by Harvard earned that amount, hundreds of subcontract employees, particularly custodians and dining hall staff, did not.
The rise of domestic outsourcing—that is, when a company obtains employees through a subcontracting firm rather than hiring the workers directly—is a defining feature of many economies over the past 20 years, including the United States. No single authoritative source tracks outsourced workers, but one careful study found that the share of outsourced workers in cleaning, logistics, and security in the U.S. increased from less than 10 percent in 1950 to more than 20 percent by 2015.
Outsourcing is prevalent in high- and low-paying jobs, for support functions as well as companies’ core activities. For instance, Google garnered bad press in 2021 for its “two-tier work force of generously compensated full-time employees and less expensive temps and contractors,” while health care workers employed by Kaiser Permanente went on strike in 2023 in part due to the health care giant’s outsourcing of critical health care duties.
“Understanding labor arrangements like gig work and domestic outsourcing can yield important cues about the future of work and how regulations might affect workers’ compensation and rights,” said Institute Senior Economist Illenin Kondo, who studies outsourcing policy in a new Institute working paper .
Same job, less pay
If you’re doing the same job, even working at the same place, why does the company name on your paycheck matter? Most of us probably think that how much we make is a function of the type of work we do: Teacher or doctor, bus driver or airline pilot. And that certainly matters. But economic research shows that the firm you work for matters too. A number of studies in several different countries—the United States , France , Germany —all concluded that outsourced workers earn less than workers who are doing the same jobs but who have not been outsourced.
One reason companies use domestic outsourcing, then, is to minimize labor costs and maximize profits. This motivation seems particularly strong in Mexico. Kondo and his co-authors focus specifically on Mexico’s manufacturing sector, which made up almost one-quarter of the country’s GDP in 2023. In manufacturing, domestic outsourcing ballooned from 5 to 20 percent between 2000 and 2020.
As in the United States, Mexican firms owe payroll taxes on their employees, including a social security tax, which are set as a percent of the employee’s wages. Unlike in the United States, firms are also required by law to share 10 percent of their profits with their employees. Add in the right to unionize and the three months’ severance, and the bottom line is: In Mexico, employees are expensive.
In April 2021, the Mexican government passed a law requiring companies to directly hire all employees engaged in a company’s core business operations. The law also established effective monitoring of outsourcing and imposed heavy penalties for violations.
The new law quickly had its intended effect. From its high of over 20 percent in 2019, the share of the manufacturing workforce that was outsourced dropped to around 2.1 percent in 2023 (see figure).
Labor moves in, wages go up
Moving from staffing agency to manufacturing firm would not be much of a victory for workers if wages stagnated or many workers lost their jobs. Happily for workers, the economists find that wages went up after the new law—by a lot. The average wage for manufacturing workers increased by about 35 percent relative to average wages before the new law. Firms’ social security payments, benefits payments, and profit-sharing payments all went up, too. The reform appears to have brought more people into a higher-paying part of the economy.
In addition, the economists find that neither employment levels nor other inputs to the production process, such as purchases of raw materials or energy, declined after workers were “insourced.” With these inputs holding steady, so too did manufacturing firms’ output.
The economists interpret these findings as evidence that prior to the reform, Mexican manufacturing firms had a great deal of power in the labor market. They had been able to pay workers less than what they were “worth”—that is, less than what they were contributing to the productivity of the firm.
“One way to think about this type of firm power is that firms are not employing as many workers as they would in a competitive market, out of a desire to reduce their wage bill,” Kondo said. “The more workers the firm demands, the more wages go up, and so firms think maybe they should pull back a little bit because then they make more profit.”
The ban did lead to a few negative outcomes for firms. After the reform, the probability that a firm would close shop increased by 1 percentage point. In addition, firms’ capital expenditures declined by 2.8 percent. These effects appear fairly small relative to the impact on wages. However, the reform is still new, and it will be worth studying how the economic outcomes evolve over time.
Domestic outsourcing and the changing American workplace
While the economic environments of Mexico and the United States differ, there are indications that labor is on the back foot in both countries. “The decline in worker power [is] one of the major structural trends in the U.S. economy,” economists Anna Stansbury and Lawrence Summers, former Secretary of the Treasury, wrote in 2020.
One indication of this decline is that the share of economic output that goes to workers in the form of wages has been falling since the 1980s while the share that goes to owners of capital has increased. According to one estimate , in 2022, labor’s share of income in the U.S. hit its lowest level since the Great Depression.
Another workplace trend that economists have observed is that workers are being “sorted” by wages: More and more, higher-wage and lower-wage workers are employed at different companies. This trend affects what workers are paid because some firms are simply more prestigious and productive than others, and these profits are shared with employees. “When janitors work at Goldman Sachs as Goldman Sachs employees, they tend to share in the firm’s huge productivity benefits,” former Institute advisor Lawrence Katz said in a 2017 interview . “But if they work for Joe’s Janitorial Services, they no longer share in those rents.”
Workers employed directly at Goldman Sachs also enjoy the company’s generous benefits packages. Both for legal reasons and to meet employees’ perceptions of fairness, firms cannot have 170 different benefits packages for 1,700 employees. Productive, prestigious firms tend to offer generous packages to attract and retain their high-paid employees—but then these benefits must be offered to other employees as well. Contracting out some types of jobs allows the firm to decrease what it pays in benefits.
Union-negotiated wages and benefits packages may also help explain why some workers are paid more for the same job. This was part of the story at Harvard, where Katz has been a professor of economics since 1986. “The driving force was that Harvard had been contracting out a lot of janitorial work, security guards, food services. Part of that was to undercut the union wage and to get out of paying the same benefits that were really expensive,” Katz said in the 2017 interview.
All these factors affect the balance of power between workers and their employers. “To me, all this points ultimately to which workers are included and which workers are not included,” Kondo said about the role of outsourcing. “Does this boundary between staff and non-staff, between insourced and outsourced, does it potentially change the bargaining position of the worker vis-a-vis the firm?”
When outsourcing works
While outsourcing appears to have some harmful effects for workers, it is not wholly black and white. According to Katz, many workers in alternative work arrangements (including temporary help agency workers and contract company workers) appreciate the flexibility to choose their work and their schedule. Some may even see their wages increase . On the firm side, outsourcing may allow firms to respond more quickly and flexibly to changes in demand for their products or services, which could help the economy recover following recessions, a benefit for workers too.
And sometimes, outsourcing may be the least-worst option for firms facing labor shortages, the situation that some hospitals in the Twin Cities find themselves in. With difficulty filling open positions for sonographers, MRI technicians, nurse anesthetists, and other jobs, a St. Paul hospital has had to supplement in-house recruitment by temporarily filling these vital health care roles via staffing agencies, according to a senior recruiter at the hospital. It is an expensive solution, but the other options are reducing care or overburdening existing staff. And while the hospital hopes to reduce its use of outsourcing, there are some advantages, such as the ability to temporarily bring in more experienced nurses than the hospital could afford to hire outright. These nurses can provide training to others as well as critical care for complex procedures.
Understanding the various factors that lead to outsourcing as well as the diverse effects on employees will help inform policies that promote a strong, inclusive labor market for firms and workers.
Lisa Camner McKay is a senior writer with the Opportunity & Inclusive Growth Institute at the Minneapolis Fed. In this role, she creates content for diverse audiences in support of the Institute’s policy and research work.
Related Content
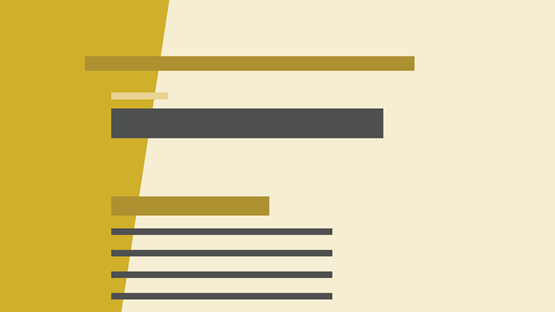
Outsourcing Policy and Worker Outcomes: Causal Evidence from a Mexican Ban
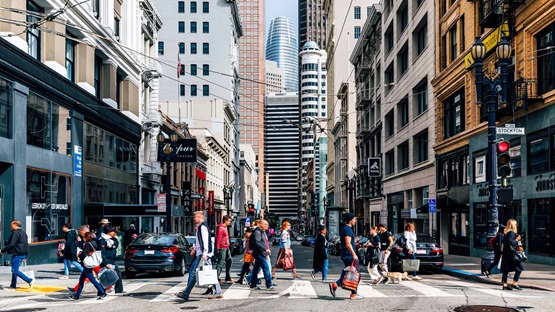
Big city, higher pay
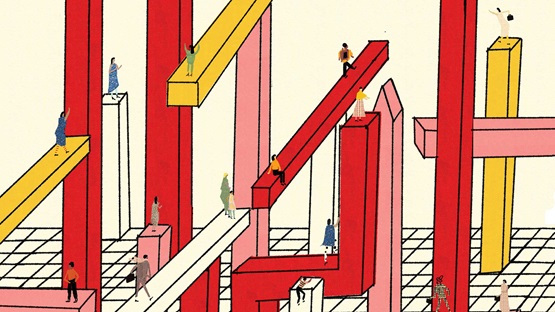
The many dimensions of income inequality
Sign up for news and events.

IMAGES
VIDEO
COMMENTS
Help companies improve internally. By conducting causal research, management can make informed decisions about improving their employee experience and internal operations. For example, understanding which variables led to an increase in staff turnover. Repeat experiments to enhance reliability and accuracy of results.
That's where causal research, the study of cause and effect, comes in. First-party data can help you learn more about the impact of your marketing campaigns, improve business metrics like customer loyalty, and conduct research on employee productivity. In this guide, we'll review what causal research is, how it can improve your marketing ...
Causal research is also known as explanatory research. It's a type of research that examines if there's a cause-and-effect relationship between two separate events. ... Market Research Survey Software Real-time, automated and advanced market research survey software & tool to create surveys, collect data and analyze results for actionable ...
Differences: Exploratory research focuses on generating hypotheses and exploring new areas of inquiry, while causal research aims to test hypotheses and establish causal relationships. Exploratory research is more flexible and open-ended, while causal research follows a more structured and hypothesis-driven approach.
Causal research allows market researchers to predict hypothetical occurrences and outcomes while enhancing existing strategies. Organizations can use this concept to develop beneficial plans. Causal research is also useful as market researchers can immediately deduce the effect of the variables on each other under real-world conditions.
Causal research can help you assess marketing initiatives, improve internal processes and create more effective business plans. Learning how one situation affects another can help you determine the best strategies for addressing your needs. ... Causal research, sometimes referred to as explanatory research, is a type of study that evaluates ...
It yields statistically conclusive data. The objective of causal research is to test hypotheses about cause-and-effect relationships; Why Your Business Needs Causal Research. With causal research, market researchers can predict hypothetical occurrences and outcome s. This allows a business to create a business plan most beneficial to the company.
Causal research is the type of research that investigates cause-and-effect relationships. It is more comprehensive than descriptive research, which just talks about how things affect each other. ... Marketing strategies: Causal research studies allow you to identify factors that influence customer behavior to develop effective marketing ...
Overview of Causal Research. Causal Research is the most sophisticated research market researchers conduct. Its goal is to establish causal relationships—cause and effect—between two or more variables [i]. With causal research, market researchers conduct experiments, or test markets, in a controlled setting.
In the marketing world, data collection and market research are invaluable. That's where causal research, the study of cause and effect, comes in. First-party data can help you learn more about the impact of your marketing campaigns, improve business metrics like customer loyalty, and conduct research on employee productivity.
The causal research could be used for two things. First measuring the significance of the effect, like quantifying the percentage increase in accidents that can be contributed by road rage. Second, observing how the relationship between the variables works (i.e., enraged drivers are prone to accelerating dangerously or taking more risks ...
In the seventh video of the "Marketing Research" series, Francisco Tigre Moura discusses causal research designs, methods and applications in marketing. Mark...
Causal research is a type of market research that aims to establish cause-and-effect relationships between variables. It can help you understand how changing one factor, such as price, packaging ...
Causal research, also known as explanatory research is conducted in order to identify the extent and nature of cause-and-effect relationships. Causal research can be conducted in order to assess impacts of specific changes on existing norms, various processes etc. Causal studies focus on an analysis of a situation or a specific problem to ...
In marketing research, one-on-one surveys can also be a form of causal research. For example, one-on-one interviews, which are a personal interview combined with the use of a product, reveal ...
Causal Research is the most sophisticated research market researchers conduct. Its goal is to establish causal relationships—cause and effect—between two or more variables [i]. With causal research, market researchers conduct experiments, or test markets, in a controlled setting. Researchers study how a dependent or response variable ...
The accuracy of the causal research is directly proportional to the time you spend on the research as you are required to spend more time to study the long-term effects of a marketing program. Coincidence in causal research is the biggest flaw of the research. Sometimes, the coincidence between a cause and an effect can be assumed as a cause ...
Causal research. Causal research, is the investigation of ( research into) cause -relationships. [1] [2] [3] To determine causality, variation in the variable presumed to influence the difference in another variable (s) must be detected, and then the variations from the other variable (s) must be calculated (s).
The paper proceeds as follows. Section 2 outlines the current state of quantitative research in the marketing literature. Section 3 motivates the application of causal ML methods in the field of marketing and discusses the contribution of our study to marketing research. Section 4 introduces and describes the retailer sales data to be analyzed.
Causal Research Types. This type of research can take two forms: Experimental. The research performs structured experiments to vary one variable and find the effect on the behavior/end result. In marketing it can be test marketing or focus group studies to determine the cause and end result. Simulation based
Cause-Related Marketing (CRM) is one of the most versatile activities among the Corporate Social Responsibility (CSR) initiatives. Though CRM is extensively researched, however, only a few authors have performed systematic literature reviews on CRM. Therefore, more systematic reviews of CRM are still needed to complete and bring together the more contributions, advances, and different existing ...
Abstract. Causal knowledge is one of the most useful types of knowledge. Causal research aims to investigate causal relationships and therefore always involves one or more independent variables (or hypothesized causes) and their relationships with one or multiple dependent variables. Causal relationships can be tested using statistical and ...
The Value of Marketing Research. Marketing research is a critical part of strategic planning. It helps business leaders make informed decisions that minimize risks, maximize returns, and ensure that resources are allocated efficiently. Marketing isn't static, especially in a crowded and competitive business landscape.
Causal marketing can be a large range on advertising, activities or campaigns that allow organizations to showcase support for a good cause more specifically a charitable cause. This is the way causal marketing is a win-win situation for the organization. It ensures uplifts brand image, a greater brand recall and an effective impression.
Post navigation "Beyond the black box: Toward a new paradigm of statistics in science" (talks this Thursday in London by Jessica Hullman, Hadley Wickham, and me) Subscribe to this free newsletter and get a heads-up on our scheduled posts a week early! This well-known paradox of R-squared is still buggin me.
Working from home (WFH) surged after the COVID-19 pandemic, with university-graduate employees typically WFH for one to two days a week during 2023 (refs. 2,6). Previous causal research on WFH has ...
Outsourcing is prevalent in high- and low-paying jobs, for support functions as well as companies' core activities. For instance, Google garnered bad press in 2021 for its "two-tier work force of generously compensated full-time employees and less expensive temps and contractors," while health care workers employed by Kaiser Permanente went on strike in 2023 in part due to the health ...