- Open access
- Published: 09 February 2021

Causal relationships between genetically determined metabolites and human intelligence: a Mendelian randomization study
- Jian Yang ORCID: orcid.org/0000-0003-0868-9781 1 na1 ,
- Binbin Zhao 2 na1 ,
- Li Qian 2 ,
- Fengjie Gao 2 ,
- Yanjuan Fan 2 ,
- Xiaoyan He 2 ,
- Qingyan Ma 2 ,
- Lihong Yang 1 ,
- Bin Yan 1 ,
- Wei Wang 2 &
- Xiancang Ma 2 , 3
Molecular Brain volume 14 , Article number: 29 ( 2021 ) Cite this article
5074 Accesses
2 Citations
3 Altmetric
Metrics details
Intelligence predicts important life and health outcomes, but the biological mechanisms underlying differences in intelligence are not yet understood. The use of genetically determined metabotypes (GDMs) to understand the role of genetic and environmental factors, and their interactions, in human complex traits has been recently proposed. However, this strategy has not been applied to human intelligence. Here we implemented a two-sample Mendelian randomization (MR) analysis using GDMs to assess the causal relationships between genetically determined metabolites and human intelligence. The standard inverse-variance weighted (IVW) method was used for the primary MR analysis and three additional MR methods (MR-Egger, weighted median, and MR-PRESSO) were used for sensitivity analyses. Using 25 genetic variants as instrumental variables (IVs), our study found that 5-oxoproline was associated with better performance in human intelligence tests (P IVW = 9.25 × 10 –5 ). The causal relationship was robust when sensitivity analyses were applied (P MR-Egger = 0.0001, P Weighted median = 6.29 × 10 –6 , P MR-PRESSO = 0.0007), and repeated analysis yielded consistent result (P IVW = 0.0087). Similarly, also dihomo-linoleate (20:2n6) and p-acetamidophenylglucuronide showed robust association with intelligence. Our study provides novel insight by integrating genomics and metabolomics to estimate causal effects of genetically determined metabolites on human intelligence, which help to understanding of the biological mechanisms related to human intelligence.
Introduction
Intelligence affects all aspects of human life [ 1 ]. During the school years, some individuals show higher intelligence, attain better marks in exams, and have better prospects for further education [ 2 , 3 ]. In the workplace, intelligence influences performance, efficiency, the ability to cope with difficulties, and career achievements [ 4 ]. Intelligence is also a predictor of higher quality of life and better health outcomes [ 5 , 6 ]. Revealing the biological bases of individual differences in human intelligence has become a central and enduring aim of psychological and brain sciences. During the past decade, advances in genetic research have greatly promoted our understanding of intelligence [ 7 , 8 , 9 , 10 ]. However, further insight on its biological basis is needed.
Understanding the role of genetic characteristics and their interaction with environmental factors is the key to reveal the biological mechanisms underlying differences in human intelligence [ 11 ]. Currently, omics technologies (such as genomics, metabolomics, etc.) are widely used to provide a comprehensive characterization at the molecular level of the human body as a biological system. These approaches have successfully identified a number of informative biomarkers and greatly advanced our knowledge of the molecular mechanisms responsible for many traits. However, most omics studies focus only on a single layer, and therefore fail to capture information across multiple omics assays [ 12 ]. Recently, researchers have linked metabolomics traits to genomic information through genome-wide association studies (GWAS) on non-targeted metabolic profiling [ 13 , 14 , 15 ]. A large database of genetically determined metabotypes (GDMs) has been thus established to provide comprehensive insights of how genetic variation influences metabolism [ 16 ]. The established GDMs provide important intermediates to reveal the role of the interactions between genetics and metabolic traits in determining differences in human intelligence.
Mendelian randomization (MR) is a novel genetic epidemiology study design using genetic variants as instrumental variables (IVs) to investigate whether a modifiable exposure is causally related to a medically relevant disease risk [ 17 ]. The fundamental assumption utilized in the MR framework is that if genetic variants essentially affect the biological effects of a modifiable exposure, they should be also related to the exposure-related disease risk. Exploiting the fact that inherent genetic variants are not generally susceptible to environmental variables, the MR design can avoid the potential confounding factors that are common in conventional observational studies [ 18 ]. In recent years, the explosion in the number of published GWAS summary data has increased the popularity of MR approaches (and in particular of two-sample MR analysis) as tools to infer the causality of risk factors on complex health outcomes [ 19 , 20 , 21 ]. In this study, using GDMs and the results of GWAS on intelligence, we implement two-sample MR analysis to: (1) assess the causal effects of genetically determined metabolites on human intelligence; (2) investigate the genetic basis that may play a central role in determining the variation of the related metabolites and the differences in human intelligence; (3) identify potential metabolic pathways involved in the biological processes related to intelligence.
GWAS scans with metabolomics traits
Shin et al . reported the most comprehensive exploration of genetic influences on human metabolism so far, by performing a GWAS of non-targeted metabolomics on 7824 healthy adults. [ 16 ]. Metabolic profiling was carried out on fasting serum using high-performance liquid chromatography and gas chromatography separation coupled with tandem mass spectrometry. After quality control, 486 metabolites were retained for genetic analysis, among which 309 were chemically identified and could be further assigned to 8 metabolic groups (amino acids, carbohydrates, cofactors and vitamins, energy, lipids, nucleotides, peptides, and xenobiotics), while the other 177 were classified as ‘unknown’. The final genome-wide association analyses were carried out on approximately 2.1 million single nucleotide polymorphisms (SNPs). Full summary statistics for the 486 metabolites can be found at the Metabolomics GWAS Server ( http://metabolomics.helmholtz-muenchen.de/gwas/ ).
IVs for the 486 metabolites
The foundational principle of MR relies on the existence of valid IVs. A genetic variant is a valid IV if it is (i) significantly associated with the exposure, (ii) independent of confounders, and (iii) associated with the outcome only through the exposure [ 22 ]. To identify valid IVs, we first selected the SNPs with significance P < 1 × 10 −5 , so as to account for a proportion as large as possible of the variance explained for the corresponding metabolite. We next performed a clumping procedure (linkage disequilibrium threshold of r 2 < 0.1 within a 500-kb window) to select the independent SNPs using the PLINK software (v1.9). To avoid the negative impact of weak IVs, we further used the proportion of variation explained by each IV ( R 2 ) and the F statistics to select SNPs strong enough to be valid IVs. Typically, an F statistic > 10 is considered sufficient for MR analysis [ 23 ].
GWAS summary data on intelligence
GWAS summary statistics for intelligence were obtained from the study by Savage et al . [ 10 ]. Briefly, these authors performed a large GWAS meta-analysis of 269,867 individuals from 14 cohorts of European ancestry. Intelligence was assessed using different neurocognitive tests and the general factor of intelligence (Spearman’s g ). Although differences in assessment methods might reduce the power to detect associations in meta-analyses, this approach can at the same time reduce type I errors by removing measurement errors, and therefore identify SNPs with robust associations to the common latent factor underlying intelligence across different methods. Stringent quality control procedures were applied to the summary statistics for each cohort. Association analysis was conducted controlling for covariates of age, sex, genotyping array, socioeconomic status for specific cohort, and twenty European-based ancestry principal components. Finally, a total of 9,295,118 SNPs were included in the meta-analysis.
Statistical analysis
Primary two-sample MR analyses were performed using the standard inverse-variance weighted (IVW) method. The IVW method provides a consistent estimate of causal effects by combining the ratio estimates of each variant in a fixed-effect meta-analysis model [ 23 ]. The P-value was calculated with a standard normal cumulative distribution function on the ratio of the combined causal effect and its standard error. The significance threshold to declare a causal relationship for the IVW-based MR estimate was set, using Bonferroni correction, at P < 1.03 × 10 –4 (= 0.05/486). Associations with P < 0.05, but not reaching the Bonferroni-corrected threshold, were reported as suggestive of association.
The IVW method provides an unbiased estimate under the assumption that all genetic variants are valid IVs. However, this assumption is easily violated, leading to inaccurate estimates, when horizontal pleiotropy occurs (some variants act on the outcome via a different intermediary) [ 24 ]. To avoid the effects of widespread horizontal pleiotropy in MR, we further performed sensitivity analyses using three additional MR methods: the MR-Egger method, which provides a consistent causal effect estimate, even when all genetic variants violate the assumptions defining valid IVs, under a weaker assumption (known as the InSIDE [instrument strength independent of direct effect] assumption) [ 24 ]; the weighted median method, which introduces a weighted median estimator and provides a more precise estimate than MR-Egger regression without the InSIDE assumption [ 25 ]; and the MR-PRESSO method, a newly developed approach which can identify and correct for horizontal pleiotropic outliers in MR [ 26 ]. We further used the MR-PRESSO global test as well as the intercept of the MR-Egger regression to test for pleiotropy, and we also evaluated heterogeneity with the I 2 and the Cochran Q test. Typically, I 2 > 25% or Cochran Q-derived P < 0.05 were used as indicators of possible horizontal pleiotropy. Analyses were carried out using the packages MendelianRandomization and MR-PRESSO in R (version 3.6.1).
Replication
We next used GWAS datasets of four other related outcomes to replicate the findings of our MR estimates. The first dataset was obtained from another GWAS of intelligence with 248,482 samples from the UK Biobank [ 27 ]. Summary statistics of cognitive performance (n = 257,828) and educational attainment (n = 766,345) were obtained from the study of Lee et al. [ 28 ]. Genetic associations with income (n = 286,301) were extracted from the large publicly available Lothian Birth Cohorts of 1921 and 1936 data-sharing resource [ 29 ]. Notably, the Davies et al . reported another GWAS for intelligence with a larger sample size, but the summary data for full dataset is not available due to data permissions [ 30 ].
Metabolic pathway analysis
Metabolic pathway analysis was carried out using the web-based tool suite MetaboAnalyst 4.0 ( https://www.metaboanalyst.ca/ ) [ 31 ]. For this analysis, we extracted all metabolites showing suggestive associations in the IVW estimates (P IVW < 0.05). Two libraries of metabolic pathways or metabolite sets were selected for enrichment analysis, namely the Small Molecule Pathway Database (SMPDB, http://www.smpdb.ca ) [ 32 ] and the Kyoto Encyclopedia of Genes and Genomes (KEGG, https://www.kegg.jp/ ) database [ 33 ]. P-values < 0.05 were considered statistically significant.
Causal effects of the metabolites on intelligence
We selected 3–675 independent genetic variants as IVs for each of the 486 metabolites (Additional file 4 : Table S1). On average, the IVs explained 4.7% (range 0.8–83.5%) of the variance of their respective metabolic traits. The minimum F statistic used to evaluate the strength of these IVs was 20.33. Using these IVs, IVW identified 16 known metabolites and 16 unknown metabolites that might have causal effects on human intelligence (Fig. 1 , Additional file 4 : Table S2). Among the 16 known metabolic traits, 5-oxoproline was significantly associated with intelligence after Bonferroni correction (P IVW = 9.25 × 10 –5 ). Using 25 SNPs as proxy, we observed a 0.24 increase in the score of the Spearman’s g test for an increase of one standard deviation (SD) in the level of 5-oxoproline (β = 2.10; 95% Confidence interval [CI] 0.12 to 0.35). We also found 15 other metabolites to be suggestive for association, including indolelactate (β = − 0.09; 95% CI − 0.81 to − 0.01, P IVW = 0.0313), mannitol (β = − 0.03; 95% CI − 0.06 to − 0.01, P IVW = 0.0223), and 2-oleoylglycerophosphocholine (β = 0.18; 95% CI 0.05 to 0.30, P IVW = 0.0055).
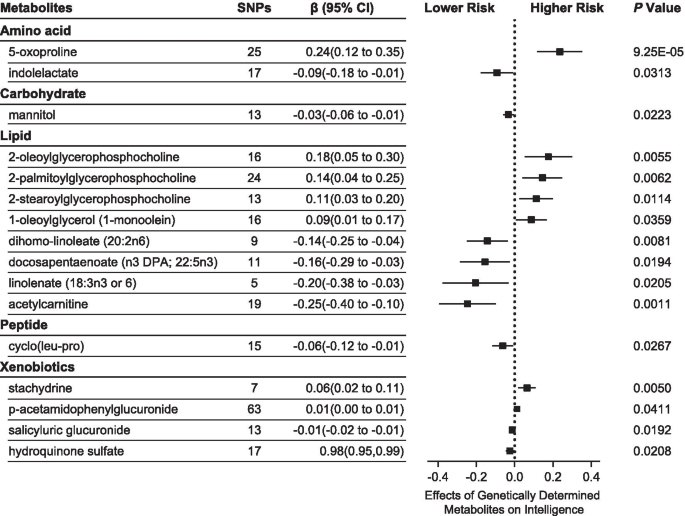
Mendelian randomization associations of genetically determined metabolites on intelligence
Sensitivity analysis
Table 1 shows the results of the sensitivity analyses for the 16 IVW-identified known metabolites. The causal relationship between 5-oxoproline and intelligence was robust when additional MR methods were applied (P MR-Egger = 0.0001, P Weighted median = 6.29 × 10 –6 , P MR-PRESSO = 0.0007), and no horizontal pleiotropy was observed (P Intercept = 0.09, P Global test = 0.06, I 2 = 25%, P Heterogeneity = 0.13). Two other metabolites also showed robust associations with intelligence, namely dihomo-linoleate (20:2n6) (P MR-Egger = 0.0494, P Weighted median = 0.0236, P MR-PRESSO = 0.0293, P Global test = 0.16) and p-acetamidophenylglucuronide (P MR-Egger = 0.0075, P Weighted median = 0.0060, P MR-PRESSO = 0.0454, P Global test = 0.0611), and there were no evidence of horizontal pleiotropy (P Intercept = 0.24, P Global test = 0.17, I 2 = 0%, P Heterogeneity = 0.96 for dihomo-linoleate (20:2n6) and P Intercept =0.06, P Global test = 0.06, I 2 = 17%, P Heterogeneity = 0.13 for p-acetamidophenylglucuronide; Table 1 ). Funnel plots appeared generally symmetrical for all the three metabolites, also suggesting no evidence for horizontal pleiotropy (Additional file 1 : Fig. S1). Dihomo-linoleate (20:2n6) showed a negative association with intelligence (β IVW = − 0.14; 95% CI − 0.25 to − 0.04), while the association between p-acetamidophenylglucuronide and intelligence was positive (β IVW = 0.01; 95% CI 0.00 to 0.01). The causal association between 5-oxoproline and human intelligence is shown on Fig. 2 , while the associations for dihomo-linoleate (20:2n6) and p-acetamidophenylglucuronide with intelligence are represented on Fig. 3 . Notably, the very small effect size for p-acetamidophenylglucuronide on intelligence might limit its potential utility as a biomarker.
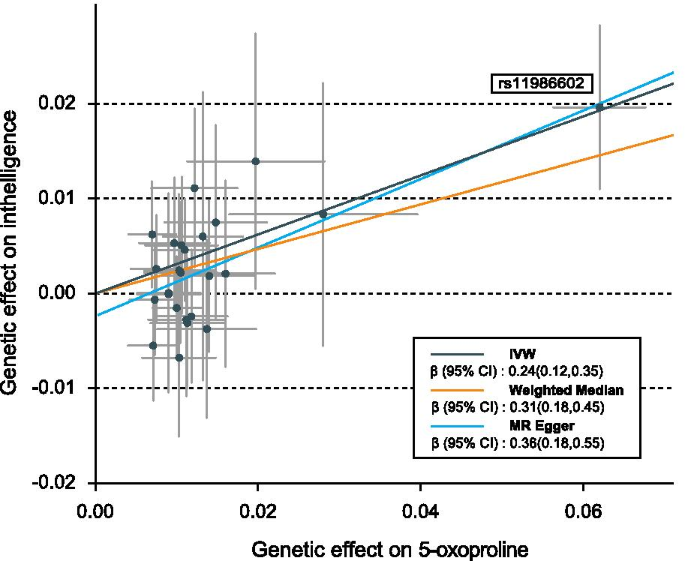
Genetic associations between 5-oxoproline and intelligence
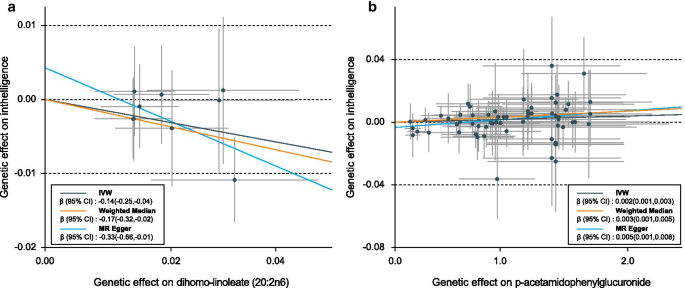
Genetic associations of two suggestive metabolites with intelligence. a Dihomo-linoleate (20:2n6); b p-acetamidophenylglucuronide
Associations with other relevant outcomes
We next repeated the main findings using summary statistics from other data sources. Figure 4 showed the results of causal effects of 5-oxoproline on human intelligence from another data source, cognitive performance, educational attainment, and income. The effect of genetically determined 5-oxoproline on intelligence (Replication) was similar (β = 0.17; 95% CI 0.04 to 0.30, P IVW = 0.0087) to the result of initial MR estimates, and the causal associations were robust when different methods were performed (P Weighted median = 0.0003, P MR-Egger = 0.0035). The results also showed that 5-oxoproline was significantly associated with cognitive performance (P IVW = 0.0001, P Weighted median = 1.44 × 10 –6 , P MR-Egger = 0.0009). However, no evidences for association were found between 5-oxoproline and educational attainment (P IVW = 0.5595, P Weighted median = 0.3417, P MR-Egger = 0.4611), as well as income (P IVW = 0.7854, P Weighted median = 0.4287, P MR-Egger = 0.6178). Besides, the effects of dihomo-linoleate (20:2n6) and p-acetamidophenylglucuronide on intelligence were also significant in the replication stage (Additional file 2 : Fig. S2; Additional file 3 : Fig. S3).
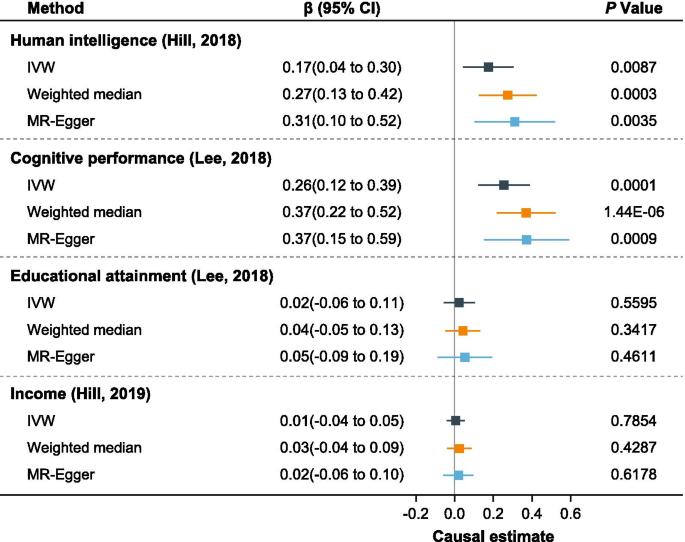
Mendelian randomization associations of 5-oxoproline on other intelligence-related outcomes from other data sources
Genetic basis for the causal associations
We further investigated the genetic variants that affected both metabolite levels and intelligence. Table 2 shows the 25 SNPs used as IV of 5-oxoproline. Among them, rs11986602 showed the most significant association with 5-oxoproline (β = − 0.0620; SE = 0.0029, P = 6.29 × 10 –104 ). Notably, it also showed a strong association signal with intelligence (β = − 0.0196; SE = 0.0044, P = 9.53 × 10 –6 ). Moreover, this SNP had the largest effect sizes on both 5-oxoproline and intelligence, suggesting that the related genetic locus might provide valuable information on the biological mechanisms of intelligence, and that 5-oxoproline might be an important functional intermediate to understand the biological process through which genetics affects intelligence. The IVs for dihomo-linoleate (20:2n6) and p-acetamidophenylglucuronide are shown in Additional file 4 : Tables S3 and S4.
Table 3 shows the results of the metabolic pathway analysis. Based on the 16 known metabolites identified by the IVW method, we detected only one significant metabolic pathway associated with intelligence, namely Alpha linolenic acid and linoleic acid metabolism (P = 0.0062). Two metabolites identified by IVW, docosapentaenoate (n3 DPA; 22:5n3) and linolenate (18:3n3 or 6), are involved in Alpha linolenic acid and linoleic acid metabolism according to the SMPDB database. Importantly, many of the metabolites found by our analysis have not been assigned to any metabolic pathway currently recorded in the SMPDB or KEGG databases. Extensive further research will be needed to explore whether these metabolites are involved in biological processes relevant to differences in human intelligence.
We implemented a two-sample MR analysis to assess the causal relationships between genetically determined metabolites and human intelligence. Using genetic variants as IVs, we found that the genetically determined levels of 5-oxoproline were associated with better performance in human intelligence tests. This causal association was not affected by confounders such as educational attainment and household income, and was well replicated using samples from other data source. Our study also identified other metabolites and metabolic pathways involved in biological processes related to human intelligence, such as dihomo-linoleate (20:2n6) and p-acetamidophenylglucuronide. To the best of our knowledge, this is the first study combining information from genomics and metabolomics to assess the causal effects of metabolome traits on human intelligence.
5-Oxoproline, also known as pyroglutamic acid, is a cyclized derivative of l -glutamic acid that participates substantially in the glutamate and glutathione metabolism [ 34 ]. Disturbances in glutamate and glutathione metabolism can lead to a series of neurologic phenotypes, including developmental delay, ataxia, seizures, and intellectual disability [ 35 ]. Moreover, 5-oxoproline was also developed and sold as an over-the-counter “smart drug” for cognitive and memory improvement [ 36 , 37 ]. However, it was also demonstrated that metabolic acidosis could be caused by excessive 5-oxoproline generation, with multiple adverse effects on many organ systems [ 38 ]. Our study found that elevated levels of 5-oxoproline were associated with a higher score in intelligence tests, supporting the potential usefulness of 5-oxoproline in improving intelligence-related performance. However, more work aimed at understanding the molecular mechanisms involved is needed to further clarify the role of this compound in human intelligence.
Genetic factors played a central role in our study of the causal relationship between metabolic traits and intelligence. The SNP rs11986602 (corresponding to the EXOSC4 gene) was the most significantly associated to both 5-oxoproline levels and human intelligence. Although rarely discussed in the past literature, EXOSC4 is known to be related to the protein kinase R (PKR)-like endoplasmic reticulum kinase (PERK, encoded by the EIF2AK3 gene), which regulates gene expression [ 39 ]. A recent study reported that locally reduced PERK expression or activity could enhance neuronal excitability and improve memory and cognitive function in young mice [ 40 ]. Another study provided evidence that PERK is a key regulator of memory impairments and neurodegeneration in Alzheimer’s disease [ 41 ]. Thus, EXOSC4 might be a causal risk gene participating in physiological processes important for human intelligence.
We further focused on the metabolic pathways that might be involved in the biological processes associated to human intelligence. The only identified metabolic pathway in our study was Alpha linolenic acid and linoleic acid metabolism. Alpha linolenic acid and linoleic acid are long-chain polyunsaturated fatty acids, which are essential nutrients in the development and functioning of the brain [ 42 ]. Many related compounds, such as alpha linolenic acid and docosahexaenoic acid, are involved in the rapid growth and development of the infant brain [ 43 , 44 ]. Our study thus reinforced the importance of alpha linolenic acid and linoleic acid metabolism for human intelligence, providing valuable information for understanding the biological mechanisms related to human intelligence.
The current study has several strengths. First, we implemented a novel MR study design to assess the causal relationships between genetically determined metabolites and human intelligence. By using genetic variants as IVs, the MR approach prevents confounding, reverse causation, and various biases common in observational epidemiological studies. Second, our study provides, indirectly, a comprehensive assessment of the causal effects of metabolites assessed by non-targeted metabolomics on human intelligence. Third, by integrating genomics and metabolomics, our study provides novel insight into the biological mechanisms underlying differences in intelligence.
There are also several limitations that should be noted. First, the GWAS data for intelligence was determined adjusting for socioeconomic status, which was a heritable and correlated secondary trait to intelligence [ 29 , 45 ]. The adjustment for socioeconomic status might cause bias in genetic associations with intelligence for some SNPs [ 46 ]. Second, our study could not avoid the bias of dynastic effect, which induced a correlation between the environment a child is raised in and their genetic inheritance and almost certainly violated the independence assumption of MR [ 47 , 48 ]. Within family GWAS data was useful in avoiding the issue of dynastic effects. However, such data was not available at this stage. Third, our study failed to perform the bi-directional MR analysis which was useful in detecting false positive MR results arising from genetic correlation between traits. The reason was that many of the IVs for intelligence were missing in datasets of metabolites. Finally, the MR estimates from non-experimental date could not provide information towards molecular mechanism, further work should be done to determine the roles of metabolites or genetic variants in development of intelligence.
In summary, our study identified multiple metabolites that might have causal effects on human intelligence, among which 5-oxoproline presented significant association signals after Bonferroni correction. The association was shown to be robust by sensitivity analyses. Our study also highlighted that genetic factors (e.g. the EXOSC4 gene) contributed substantially to the variation of metabolite levels and differences in human intelligence. Moreover, our findings suggest that alpha linolenic acid and linoleic acid metabolism might be involved in the biological processes underlying intelligence. Though further evidence from experimental data is needed, our study provides novel clues that would improve our understanding of the biological mechanisms related to human intelligence.
Availability of data and materials
Full summary statistics for the 486 metabolites are publicly available at the Metabolomics GWAS Server ( http://metabolomics.helmholtz-muenchen.de/gwas/ ). GWAS summary statistics for intelligence are download from http://ctg.cncr.nl/software/summary_statistics .
Abbreviations
Genetically determined metabotype
- Mendelian randomization
Inverse-variance weighted
Instrumental variable
Genome-wide association study
Single nucleotide polymorphism
Instrument strength independent of direct effect
Small Molecule Pathway Database
Kyoto Encyclopedia of Genes and Genomes
Deary IJ. Intelligence. Annu Rev Psychol. 2012;63:453–82.
Article PubMed Google Scholar
Blackwell LS, Trzesniewski KH, Dweck CS. Implicit theories of intelligence predict achievement across an adolescent transition: a longitudinal study and an intervention. Child Dev. 2007;78:246–63.
Deary IJ, Strand S, Smith P, Fernandes C. Intelligence and educational achievement. Intelligence. 2007;35:13–21.
Article Google Scholar
Burks SV, Carpenter JP, Goette L, Rustichini A. Cognitive skills affect economic preferences, strategic behavior, and job attachment. Proc Natl Acad Sci USA. 2009;106:7745–50.
Article CAS PubMed PubMed Central Google Scholar
Gottfredson LS. Why g matters: the complexity of everyday life. Intelligence. 1997;24:79–132.
Deary I. Why do intelligent people live longer? Nature. 2008;456:175–6.
Article CAS PubMed Google Scholar
Plomin R, von Stumm S. The new genetics of intelligence. Nat Rev Genet. 2018;19:148–59.
Panizzon MS, Vuoksimaa E, Spoon KM, Jacobson KC, Lyons MJ, Franz CE, et al. Genetic and environmental influences of general cognitive ability: is g a valid latent construct? Intelligence. 2014;43:65–76.
Article PubMed PubMed Central Google Scholar
Sniekers S, Stringer S, Watanabe K, Jansen PR, Coleman JRI, Krapohl E, et al. Genome-wide association meta-analysis of 78,308 individuals identifies new loci and genes influencing human intelligence. Nat Genet. 2017;49:1107–12.
Savage JE, Jansen PR, Stringer S, Watanabe K, Bryois J, de Leeuw CA, et al. Genome-wide association meta-analysis in 269,867 individuals identifies new genetic and functional links to intelligence. Nat Genet. 2018;50:912–9.
Nisbett RE, Aronson J, Blair C, Dickens W, Flynn J, Halpern DF, et al. Intelligence: new findings and theoretical developments. Am Psychol. 2012;67:130–59.
Dehghan A. Chapter 19 - Linking metabolic phenotyping and genomic information. In: Lindon JC, Nicholson JK, Holmes E, editors. The handbook of metabolic phenotyping. Amsterdam: Elsevier; 2019. p. 561–9.
Chapter Google Scholar
Gieger C, Geistlinger L, Altmaier E, Hrabe de Angelis M, Kronenberg F, Meitinger T, et al. Genetics meets metabolomics: a genome-wide association study of metabolite profiles in human serum. PLoS Genet. 2008;4:1000282.
Article CAS Google Scholar
Suhre K, Shin S-Y, Petersen A-K, Mohney RP, Meredith D, Wägele B, et al. Human metabolic individuality in biomedical and pharmaceutical research. Nature. 2011;477:54–60.
Kettunen J, Tukiainen T, Sarin AP, Ortega-Alonso A, Tikkanen E, Lyytikainen LP, et al. Genome-wide association study identifies multiple loci influencing human serum metabolite levels. Nat Genet. 2012;44:269–76.
Shin SY, Fauman EB, Petersen AK, Krumsiek J, Santos R, Huang J, et al. An atlas of genetic influences on human blood metabolites. Nat Genet. 2014;46:543–50.
Burgess S, Daniel RM, Butterworth AS, Thompson SG, et al. Network Mendelian randomization: using genetic variants as instrumental variables to investigate mediation in causal pathways. Int J Epidemiol. 2015;44:484–95.
Burgess S, Small DS, Thompson SG. A review of instrumental variable estimators for Mendelian randomization. Stat Methods Med Res. 2017;26:2333–55.
Cheung C-L, Tan KCB, Au PCM, Li GHY, Cheung BMY. Evaluation of GDF15 as a therapeutic target of cardiometabolic diseases in human: a Mendelian randomization study. EBioMedicine. 2019;41:85–90.
Sanna S, van Zuydam NR, Mahajan A, Kurilshikov A, Vich Vila A, Vosa U, et al. Causal relationships among the gut microbiome, short-chain fatty acids and metabolic diseases. Nat Genet. 2019;51:600–5.
Haycock PC, Burgess S, Nounu A, Zheng J, Okoli GN, et al. Association between telomere length and risk of cancer and non-neoplastic diseases: a Mendelian randomization study. JAMA Oncol. 2017;3:636–51.
Evans DM, Davey SG. Mendelian randomization: new applications in the coming age of hypothesis-free causality. Annu Rev Genomics Hum Genet. 2015;16:327–50.
Burgess S, Butterworth A, Thompson SG. Mendelian randomization analysis with multiple genetic variants using summarized data. Genet Epidemiol. 2013;37:658–65.
Bowden J, Davey Smith G, Burgess S. Mendelian randomization with invalid instruments: effect estimation and bias detection through Egger regression. Int J Epidemiol. 2015;44:512–25.
Bowden J, Davey Smith G, Haycock PC, Burgess S. Consistent estimation in Mendelian randomization with some invalid instruments using a weighted median estimator. Genet Epidemiol. 2016;40:304–14.
Verbanck M, Chen CY, Neale B, Do R. Detection of widespread horizontal pleiotropy in causal relationships inferred from Mendelian randomization between complex traits and diseases. Nat Genet. 2018;50:693–8.
Hill WD, Marioni RE, Maghzian O, Ritchie SJ, Hagenaars SP, McIntosh AM, et al. A combined analysis of genetically correlated traits identifies 187 loci and a role for neurogenesis and myelination in intelligence. Mol Psychiatry. 2019;24:169–81.
Lee JJ, Wedow R, Okbay A, Kong E, Maghzian O, Zacher M, et al. Gene discovery and polygenic prediction from a genome-wide association study of educational attainment in 11 million individuals. Nat Genet. 2018;50:1112–21.
Hill WD, Davies NM, Ritchie SJ, Skene NG, Bryois J, Bell S, et al. Genome-wide analysis identifies molecular systems and 149 genetic loci associated with income. Nat Commun. 2019;10:1–7.
Davies G, Lam M, Harris SE, Trampush JW, Luciano M, Hill WD, et al. Study of 300,486 individuals identifies 148 independent genetic loci influencing general cognitive function. Nat Commun. 2018;9:2098.
Article PubMed PubMed Central CAS Google Scholar
Chong J, Wishart DS, Xia J. Using metaboanalyst 4.0 for comprehensive and integrative metabolomics data analysis. Curr Protoc Bioinform. 2019;68:86.
Jewison T, Su Y, Disfany FM, Liang Y, Knox C, Maciejewski A, et al. SMPDB 2.0: big improvements to the small molecule pathway database. Nucleic Acids Res. 2014;42:478–84.
Kanehisa M, Goto S, Sato Y, Furumichi M, Tanabe M. KEGG for integration and interpretation of large-scale molecular data sets. Nucleic Acids Res. 2012;40:D109–14.
Ristoff E, Larsson A. Inborn errors in the metabolism of glutathione. Orphanet J Rare Dis. 2007;2:16.
Rumping L, Vringer E, Houwen RHJ, van Hasselt PM, Jans JJM, Verhoeven-Duif NM. Inborn errors of enzymes in glutamate metabolism. J Inherit Metab Dis. 2019;43:200–15.
McDougall GJ Jr, Austin-Wells V, Zimmerman T. Utility of nutraceutical products marketed for cognitive and memory enhancement. J Holist Nurs. 2005;23:415–33.
Grioli S, Lomeo C, Quattropani MC, Spignoli G, Villardita C. Pyroglutamic acid improves the age associated memory impairment. Fundam Clin Pharmacol. 1990;4:169–73.
Hlozek T, Krizek T, Tuma P, Bursova M, Coufal P, Cabala R. Quantification of paracetamol and 5-oxoproline in serum by capillary electrophoresis: implication for clinical toxicology. J Pharm Biomed Anal. 2017;145:616–20.
Raijmakers R, Egberts WV, van Venrooij WJ, Pruijn GJM. Protein–protein interactions between human exosome components support the assembly of RNase PH-type subunits into a six-membered PNPase-like Ring. J Mol Biol. 2002;323:653–63.
Sharma V, Ounallah-Saad H, Chakraborty D, Hleihil M, Sood R, Barrera I, et al. Local inhibition of PERK enhances memory and reverses age-related deterioration of cognitive and neuronal properties. J Neurosci. 2018;38:648–58.
Ohno M. PERK as a hub of multiple pathogenic pathways leading to memory deficits and neurodegeneration in Alzheimer’s disease. Brain Res Bull. 2018;141:72–8.
Janssen CI, Kiliaan AJ. Long-chain polyunsaturated fatty acids (LCPUFA) from genesis to senescence: the influence of LCPUFA on neural development, aging, and neurodegeneration. Prog Lipid Res. 2014;53:1–17.
Innis SM. Dietary omega 3 fatty acids and the developing brain. Brain Res. 2008;1237:35–43.
Simopoulos AP. Evolutionary aspects of diet: the omega-6/omega-3 ratio and the brain. Mol Neurobiol. 2011;44:203–15.
Hill WD, Hagenaars SP, Marioni RE, Harris SE, Liewald DCM, Davies G, et al. Molecular genetic contributions to social deprivation and household income in UK Biobank. Curr Biol. 2016;26:3083–9.
Aschard H, Vilhjálmsson Bjarni J, Joshi Amit D, Price Alkes L, Kraft P. Adjusting for heritable covariates can bias effect estimates in genome-wide association studies. Am J Hum Genet. 2015;96:329–39.
Kong A, Thorleifsson G, Frigge ML, Vilhjalmsson BJ, Young AI, Thorgeirsson TE, et al. The nature of nurture: effects of parental genotypes. Science. 2018;359:424–8.
Koellinger PD, de Vlaming R. Mendelian randomization: the challenge of unobserved environmental confounds. Int J Epidemiol. 2019;48:665–71.
Download references
Acknowledgements
The authors would like to thank the High-Performance Computing Cluster of the First Affiliated Hospital of Xi'an Jiaotong University for data computing support.
The study was funded by the Clinical Research Award of the First Affiliated Hospital of Xi'an Jiaotong University (Grant No. XJTU1AF-CRF-2017-025).
Author information
Jian Yang and Binbin Zhao have contributed equally to this work
Authors and Affiliations
Clinical Research Center, The First Affiliated Hospital of Xi’an Jiaotong University, Xi’an, China
Jian Yang, Lihong Yang & Bin Yan
Department of Psychiatry, the First Affiliated Hospital of Xi’an Jiaotong University, No. 277 Yanta West Road, Xi’an, 700061, China
Binbin Zhao, Li Qian, Fengjie Gao, Yanjuan Fan, Xiaoyan He, Qingyan Ma, Wei Wang & Xiancang Ma
Center for Brain Science, The First Affiliated Hospital of Xi’an Jiaotong University, Xi’an, China
Xiancang Ma
You can also search for this author in PubMed Google Scholar
Contributions
XM and JY were responsible for the study conception and study design. BZ, LQ, FG and YF performed the data collation and statistical analysis. JY and BZ drafted the manuscript. QM and LY were involved in the technical supports. BY and WW contributed to interpretation and editing of the manuscript. All authors read and approved the final manuscript.
Corresponding author
Correspondence to Xiancang Ma .
Ethics declarations
Ethics approval and consent to participate.
Not applicable.
Consent for publication
All authors read and approved the final manuscript.
Competing interests
The authors have no conflict of interest.
Additional information
Publisher's note.
Springer Nature remains neutral with regard to jurisdictional claims in published maps and institutional affiliations.
Supplementary Information
Additional file 1: fig. s1.
. Funnel plots for detecting potential pleiotropy.
Additional file 2: Fig. S2.
Mendelian randomization associations of dihomo-linoleate(20:2n6) on other intelligence-related outcomes from other data sources.
Additional file 3: Fig. S3.
Mendelian randomizationassociations of p-acetamidophenylglucuronide on other intelligence-relatedoutcomes from other data sources.
Additional file 4:
Table S1. Information of the instrumental variables used for Mendelian randomization estimates. Table S2. Unknown metabolites identified by the Mendelian randomization estimates. Table S3. Genetic predictors for dihomo-linoleate (20:2n6) and their association with Intelligence. Table S4. Genetic predictors for p-acetamidophenylglucuronide and their association with Intelligence.
Rights and permissions
Open Access This article is licensed under a Creative Commons Attribution 4.0 International License, which permits use, sharing, adaptation, distribution and reproduction in any medium or format, as long as you give appropriate credit to the original author(s) and the source, provide a link to the Creative Commons licence, and indicate if changes were made. The images or other third party material in this article are included in the article's Creative Commons licence, unless indicated otherwise in a credit line to the material. If material is not included in the article's Creative Commons licence and your intended use is not permitted by statutory regulation or exceeds the permitted use, you will need to obtain permission directly from the copyright holder. To view a copy of this licence, visit http://creativecommons.org/licenses/by/4.0/ . The Creative Commons Public Domain Dedication waiver ( http://creativecommons.org/publicdomain/zero/1.0/ ) applies to the data made available in this article, unless otherwise stated in a credit line to the data.
Reprints and permissions
About this article
Cite this article.
Yang, J., Zhao, B., Qian, L. et al. Causal relationships between genetically determined metabolites and human intelligence: a Mendelian randomization study. Mol Brain 14 , 29 (2021). https://doi.org/10.1186/s13041-021-00743-4
Download citation
Received : 14 May 2020
Accepted : 01 February 2021
Published : 09 February 2021
DOI : https://doi.org/10.1186/s13041-021-00743-4
Share this article
Anyone you share the following link with will be able to read this content:
Sorry, a shareable link is not currently available for this article.
Provided by the Springer Nature SharedIt content-sharing initiative
- Genetically determined metabolite
- Human intelligence
- Metabolic pathway
- 5-Oxoproline
Molecular Brain
ISSN: 1756-6606
- Submission enquiries: Access here and click Contact Us
- General enquiries: [email protected]

Research reveals genetic link to human intelligence
University of Manchester scientists, working with colleagues in Edinburgh and Australia, have provided the first direct biological evidence for a genetic contribution to people's intelligence.
Previous studies on twins and adopted people suggested that there is a substantial genetic contribution to thinking skills, but this new study -- published in the journal Molecular Psychiatry -- is the first to find a genetic contribution by testing people's DNA for genetic variations.
The team studied two types of intelligence in more than 3,500 people from Edinburgh, Aberdeen, Newcastle and Manchester. The paper, by Dr Neil Pendleton and colleagues, found that 40% to 50% of people's differences in these abilities could be traced to genetic differences.
The study examined more than half a million genetic markers on every person in the study. The new findings were made possible using a new type of analysis invented by Professor Peter Visscher and colleagues in Brisbane. As well as the findings in people from Scotland and England, the team checked their results in a separate group of people from Norway.
Dr Pendleton, who led the Manchester team in the Centre for Integrated Genomic Research, said: "This is the first reported research to examine the intelligence of healthy older adults and, using a comprehensive genetic survey, we were able to show a substantial genetic contribution in our ability to think.
"The study confirms the earlier findings of the research in twins. However, that research could not show which genes were or were not contributing to cognitive ability. Our work demonstrates that the number of individual genes involved in intelligence is large, which is similar to other human traits, such as height.
"We can now use the findings to better understand how these genes interact with each other and the environment, which has an equally significant contribution. With our collaborators, we will take this work forward to find the biological mechanisms that could maintain our intellectual abilities and wellbeing in late life. "
The study, in collaboration with Professor Ian Deary at the University of Edinburgh, was funded in Manchester by the Biotechnology and Biological Sciences Research Council.
- Intelligence
- Social Psychology
- Relationships
- Racial Issues
- Genetic code
- Color blindness
- Homosexuality
- Left-handed
- Alzheimer's disease
- Social cognition
- Noam Chomsky
Story Source:
Materials provided by University of Manchester . Note: Content may be edited for style and length.
Journal Reference :
- G Davies, A Tenesa, A Payton, J Yang, S E Harris, D Liewald, X Ke, S Le Hellard, A Christoforou, M Luciano, K McGhee, L Lopez, A J Gow, J Corley, P Redmond, H C Fox, P Haggarty, L J Whalley, G McNeill, M E Goddard, T Espeseth, A J Lundervold, I Reinvang, A Pickles, V M Steen, W Ollier, D J Porteous, M Horan, J M Starr, N Pendleton, P M Visscher, I J Deary. Genome-wide association studies establish that human intelligence is highly heritable and polygenic . Molecular Psychiatry , 2011; DOI: 10.1038/mp.2011.85
Cite This Page :
Explore More
- Vaporizing Old Bags to Make New Plastics
- Ancient Sea Cow Attacked by Croc and Sharks
- Ancient Gene Gives Spiders Their Narrow Waist
- More Fish Species at Risk of Extinction
- Can Fungi Turn Food Waste Into Something Tasty?
- Gene Therapy Gets a Turbo Boost
- Atlantic and Arctic Current Mixing Crucial
- New Model to Enhance Extreme Rainfall Prediction
- Graphene Tech to Revolutionize Batteries
- Drug May Stop Migraines Before Headache Starts
Trending Topics
Strange & offbeat.

An official website of the United States government
The .gov means it’s official. Federal government websites often end in .gov or .mil. Before sharing sensitive information, make sure you’re on a federal government site.
The site is secure. The https:// ensures that you are connecting to the official website and that any information you provide is encrypted and transmitted securely.
- Publications
- Account settings
- My Bibliography
- Collections
- Citation manager
Save citation to file
Email citation, add to collections.
- Create a new collection
- Add to an existing collection
Add to My Bibliography
Your saved search, create a file for external citation management software, your rss feed.
- Search in PubMed
- Search in NLM Catalog
- Add to Search
Genetic influence on human intelligence (Spearman's g): how much?
Affiliation.
- 1 Psychology Department, University of Minnesota, Minneapolis, MN 55455-0344, USA. [email protected]
- PMID: 19634053
- DOI: 10.1080/03014460903103939
The history and conceptual background of the heritability statistic is briefly discussed. The construct of heritability is embedded in the method of structural equation modeling widely used in modern population genetics and in human behavior genetics. The application of structural equation modeling to behavioral phenotypes is shown to be a useful and informative analytic tool, as it implements the research strategy of 'strong inference'. I describe the application of 'strong inference', via the use of structural equation models in the domain of human intelligence, and demonstrate its utility as a means of refuting well formulated scientific hypotheses. The construct of Spearman's g is shown to be a strongly confirmed scientific hypothesis. Genetic and environmental influences are shown to influence g differentially over time, with shared environmental influences predominating early in life, but dissipating to near zero by adulthood. The hypothesis of substantively significant genetic influence on adult g is documented by multiple lines of evidence and numerous replications.
PubMed Disclaimer
Similar articles
- On models and muddles of heritability. Schönemann PH. Schönemann PH. Genetica. 1997;99(2-3):97-108. doi: 10.1007/BF02259513. Genetica. 1997. PMID: 9463078 Review.
- IQ heritability estimation: analyzing genetically-informative data with structural equation models. Gallardo Pujol D, García-Forero C, Kramp U, Maydeu-Olivares A, Andrés-Pueyo A. Gallardo Pujol D, et al. Psicothema. 2007 Feb;19(1):156-62. Psicothema. 2007. PMID: 17295998
- NO CLEAR LINK BETWEEN G LOADINGS AND HERITABILITY: A TWIN STUDY FROM KOREA. Choi YY, Cho SH, Lee KH. Choi YY, et al. Psychol Rep. 2015 Aug;117(1):291-7. doi: 10.2466/19.04.PR0.117c10z1. Epub 2015 Jun 30. Psychol Rep. 2015. PMID: 26126212
- Resolving the genetic and environmental sources of the correlation between height and intelligence: a study of nearly 2600 Norwegian male twin pairs. Sundet JM, Tambs K, Harris JR, Magnus P, Torjussen TM. Sundet JM, et al. Twin Res Hum Genet. 2005 Aug;8(4):307-11. doi: 10.1375/1832427054936745. Twin Res Hum Genet. 2005. PMID: 16176713
- Genetic and environmental influences on adult intelligence and special mental abilities. Bouchard TJ Jr. Bouchard TJ Jr. Hum Biol. 1998 Apr;70(2):257-79. Hum Biol. 1998. PMID: 9549239 Review.
- Effects of adult education on cognitive function and risk of dementia in older adults: a longitudinal analysis. Takeuchi H, Kawashima R. Takeuchi H, et al. Front Aging Neurosci. 2023 Aug 23;15:1212623. doi: 10.3389/fnagi.2023.1212623. eCollection 2023. Front Aging Neurosci. 2023. PMID: 37680542 Free PMC article.
- Schooling substantially improves intelligence, but neither lessens nor widens the impacts of socioeconomics and genetics. Judd N, Sauce B, Klingberg T. Judd N, et al. NPJ Sci Learn. 2022 Dec 15;7(1):33. doi: 10.1038/s41539-022-00148-5. NPJ Sci Learn. 2022. PMID: 36522329 Free PMC article.
- Polygenic scores for schizophrenia and general cognitive ability: associations with six cognitive domains, premorbid intelligence, and cognitive composite score in individuals with a psychotic disorder and in healthy controls. Engen MJ, Lyngstad SH, Ueland T, Simonsen CE, Vaskinn A, Smeland O, Bettella F, Lagerberg TV, Djurovic S, Andreassen OA, Melle I. Engen MJ, et al. Transl Psychiatry. 2020 Nov 30;10(1):416. doi: 10.1038/s41398-020-01094-9. Transl Psychiatry. 2020. PMID: 33257657 Free PMC article.
- Mouse models for studying genetic influences on factors determining smoking cessation success in humans. Hall FS, Markou A, Levin ED, Uhl GR. Hall FS, et al. Ann N Y Acad Sci. 2012 Feb;1248:39-70. doi: 10.1111/j.1749-6632.2011.06415.x. Ann N Y Acad Sci. 2012. PMID: 22304675 Free PMC article. Review.
- Human intelligence and brain networks. Colom R, Karama S, Jung RE, Haier RJ. Colom R, et al. Dialogues Clin Neurosci. 2010;12(4):489-501. doi: 10.31887/DCNS.2010.12.4/rcolom. Dialogues Clin Neurosci. 2010. PMID: 21319494 Free PMC article. Review.
Publication types
- Search in MeSH
Related information
Linkout - more resources, full text sources.
- Taylor & Francis
Other Literature Sources
- The Lens - Patent Citations
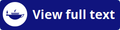
- Citation Manager
NCBI Literature Resources
MeSH PMC Bookshelf Disclaimer
The PubMed wordmark and PubMed logo are registered trademarks of the U.S. Department of Health and Human Services (HHS). Unauthorized use of these marks is strictly prohibited.
Advertisement
Genetic foundations of human intelligence
- Review Article
- Published: 18 March 2009
- Volume 126 , pages 215–232, ( 2009 )
Cite this article
- Ian J. Deary 1 ,
- W. Johnson 1 &
- L. M. Houlihan 1
12k Accesses
236 Citations
56 Altmetric
Explore all metrics
Individual differences in intelligence (cognitive abilities) are a prominent aspect of human psychology, and play a substantial role in influencing important life outcomes. Their phenotypic structure—as described by the science of psychometrics—is well understood and well replicated. Approximately half of the variance in a broad range of cognitive abilities is accounted by a general cognitive factor ( g ), small proportions of cognitive variance are caused by separable broad domains of mental function, and the substantial remainder is caused by variance that is unique to highly specific cognitive skills. The heritability of g is substantial. It increases from a low value in early childhood of about 30%, to well over 50% in adulthood, which continues into old age. Despite this, there is still almost no replicated evidence concerning the individual genes, which have variants that contribute to intelligence differences. Here, we describe the human intelligence phenotype, summarise the evidence for its heritability, provide an overview of and comment on molecular genetic studies, and comment on future progress in the field.
This is a preview of subscription content, log in via an institution to check access.
Access this article
Subscribe and save.
- Get 10 units per month
- Download Article/Chapter or eBook
- 1 Unit = 1 Article or 1 Chapter
- Cancel anytime
Price excludes VAT (USA) Tax calculation will be finalised during checkout.
Instant access to the full article PDF.
Rent this article via DeepDyve
Institutional subscriptions
Similar content being viewed by others
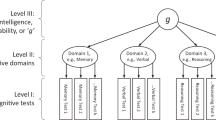
Genetic variation, brain, and intelligence differences
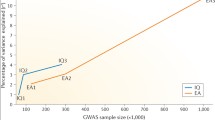
The new genetics of intelligence
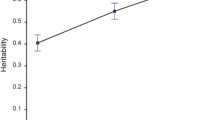
Genetics and intelligence differences: five special findings
Anokhin AP, Muller V, Lindenberger U, Heath AC, Myers E (2006) Genetic influences on dynamic complexity of brain oscillations. Neurosci Lett 397:93–98
PubMed CAS Google Scholar
Barnett JH, Scoriels L, Munafo MR (2008) Meta-analysis of the cognitive effects of the catechol-O-methyltransferase gene Val158/108Met polymorphism. Biol Psychiatry 64:137–144
Batty GD, Deary IJ, Gottfredson LS (2007) Premorbid (early life) IQ and later mortality risk: systematic review. Ann Epidemiol 17:278–288
PubMed Google Scholar
Becker KG, Barnes KC, Bright TJ, Wang SA (2004) The genetic association database. Nat Genet 36:431–432
Bell MA, Fox NA (1992) The relations between frontal brain electrical activity and cognitive development during infancy. Child Dev 63:1142–1163
Bendixen MH, Nexo BA, Bohr VA, Frederiksen H, McGue M, Kolvraa S, Christensen K (2004) A polymorphic marker in the first intron of the Werner gene associates with cognitive function in aged Danish twins. Exp Gerontol 39:1101–1107
Berr C, Richard F, Dufouil C, Amant C, Alperovitch A, Amouyel P (1998) Polymorphism of the prion protein is associated with cognitive impairment in the elderly: the EVA study. Neurology 51:734–737
Bochdanovits Z, Gosso FM, van den Berg L, Rizzu P, Polderman TJ, Pardo LM, Houlihan LM, Luciano M, Starr JM, Harris SE, Deary IJ, de Geus EJ, Boomsma DI, Heutink P, Posthuma D (2009) A functional polymorphism under positive evolutionary selection in ADRB2 is associated with human intelligence with opposite effects in the young and the elderly. Behav Genet 39:15–23
Bouchard TJ Jr (1997) Experience Producing Drive Theory: how genes drive experience and shape personality. Acta Paediatr Suppl 422:60–64
Bouchard TJ (2009 Genetic influence on human intelligence (Spearman’s g): How much? Ann Hum Biol Psychol Sci (in press)
Bouchard TJ Jr, McGue M (1981) Familial studies of intelligence: a review. Science 212:1055–1059
Bouchard TJ Jr, Lykken DT, McGue M, Segal NL, Tellegen A (1990) Sources of human psychological differences: the Minnesota Study of Twins Reared Apart. Science 250:223–228
Burdick KE, Lencz T, Funke B, Finn CT, Szeszko PR, Kane JM, Kucherlapati R, Malhotra AK (2006) Genetic variation in DTNBP1 influences general cognitive ability. Hum Mol Genet 15:1563–1568
Butcher LM, Meaburn E, Dale PS, Sham P, Schalkwyk LC, Craig IW, Plomin R (2005) Association analysis of mild mental impairment using DNA pooling to screen 432 brain-expressed single-nucleotide polymorphisms. Mol Psychiatry 10:384–392
Butcher LM, Davis OS, Craig IW, Plomin R (2008) Genome-wide quantitative trait locus association scan of general cognitive ability using pooled DNA and 500 K single nucleotide polymorphism microarrays. Genes Brain Behav 7:435–446
Buyske S, Bates ME, Gharani N, Matise TC, Tischfield JA, Manowitz P (2006) Cognitive traits link to human chromosomal regions. Behav Genet 36:65–76
Carroll JB (1993) Human cognitive abilities: a survey of factor analytic studies. Cambridge University Press, Cambridge
Google Scholar
Caspi A, Williams B, Kim-Cohen J, Craig IW, Milne BJ, Poulton R, Schalkwyk LC, Taylor A, Werts H, Moffitt TE (2007) Moderation of breastfeeding effects on the IQ by genetic variation in fatty acid metabolism. Proc Natl Acad Sci USA 104:18860–18865
Chelly J, Khelfaoui M, Francis F, Cherif B, Bienvenu T (2006) Genetics and pathophysiology of mental retardation. Eur J Hum Genet 14:701–713
Chiang M-C, Barysheva M, Shattuck DW, Lee AD, Madsen SK, Avedissian C, Klunder AD, Toga AW, McMahon KL, de Zubicaray GI, Wright MJ, Srivastava A, Balov N, Thomson PM (2009) Genetics of brain fiber architecture and intellectual performance. J Neurosci 29:2212–2224
Chorney MJ, Chorney K, Seese N, Owen MJ, Daniels J, McGuffin P, Thompson LA, Detterman DK, Benbow C, Lubinski D, Eley T, Plomin R (1998) A quantitative trait locus associated with cognitive ability in children. Psychol Sci 9:159–166
Comings DE, Wu S, Rostamkhani M, McGue M, Lacono WG, Cheng LS, MacMurray JP (2003) Role of the cholinergic muscarinic 2 receptor (CHRM2) gene in cognition. Mol Psychiatry 8:10–11
Croning MD, Marshall MC, McLaren P, Armstrong JD, Grant SG (2009) G2Cdb: the genes to cognition database. Nucleic Acids Res 37:D846–D851
Davis OS, Kovas Y, Harlaar N, Busfield P, McMillan A, Frances J, Petrill SA, Dale PS, Plomin R (2008) Generalist genes and the Internet generation: etiology of learning abilities by web testing at age 10. Genes Brain Behav 7:455–462
Deary IJ (2000) Looking down on human intelligence. Cambridge University Press, Cambridge
Deary IJ (2008) Why do intelligent people live longer? Nature 456:175–176
Deary IJ, Whalley LJ, Lemmon H, Crawford JR, Starr JM (2000) The stability of individual differences in mental ability from childhood to old age: follow-up of the 1932 Scottish Mental Survey. Intelligence 28:49–55
Deary IJ, Whiteman MC, Pattie A, Starr JM, Hayward C, Wright AF, Carothers A, Whalley LJ (2002) Cognitive change and the APOE epsilon 4 allele. Nature 418:932
Deary IJ, Wright AF, Harris SE, Whalley LJ, Starr JM (2004) Searching for genetic influences on normal cognitive ageing. Trends Cogn Sci 8:178–184
Deary IJ, Taylor MD, Hart CL, Wilson V, Davey Smith G, Blane D, Starr JM (2005a) Intergenerational social mobility and mid-life status attainment: influences of childhood intelligence, childhood social factors, and education. Intelligence 33:455–472
Deary IJ, Harris SE, Fox HC, Hayward C, Wright AF, Starr JM, Whalley LJ (2005b) KLOTHO genotype and cognitive ability in childhood and old age in the same individuals. Neurosci Lett 378:22–27
Deary IJ, Hamilton G, Hayward C, Whalley LJ, Powell J, Starr JM, Lovestone S (2005c) Nicastrin gene polymorphisms, cognitive ability level and cognitive ageing. Neurosci Lett 373:110–114
Deary IJ, Gow AJ, Taylor MD, Corley J, Brett C, Wilson V, Campbell H, Whalley LJ, Visscher PM, Porteous DJ, Starr JM (2007) The Lothian Birth Cohort 1936: a study to examine influences on cognitive ageing from age 11 to age 70 and beyond. BMC Geriatr 7:28
DeLisi LE, Maurizio AM, Svetina C, Ardekani B, Szulc K, Nierenberg J, Leonard J, Harvey PD (2005) Klinefelter’s syndrome (XXY) as a genetic model for psychotic disorders. Am J Med Genet B Neuropsychiatr Genet 135B:15–23
Dempster EL, Toulopoulou T, McDonald C, Bramon E, Walshe M, Wickham H, Sham PC, Murray RM, Collier DA (2006) Episodic memory performance predicted by the 2 bp deletion in exon 6 of the “alpha 7-like” nicotinic receptor subunit gene. Am J Psychiatry 163:1832–1834
Dick DM, Aliev F, Bierut L, Goate A, Rice J, Hinrichs A, Bertelsen S, Wang JC, Dunn G, Kuperman S, Schuckit M, Nurnberger J Jr, Porjesz B, Beglieter H, Kramer J, Hesselbrock V (2006) Linkage analyses of IQ in the collaborative study on the genetics of alcoholism (COGA) sample. Behav Genet 36:77–86
Dick DM, Aliev F, Kramer J, Wang JC, Hinrichs A, Bertelsen S, Kuperman S, Schuckit M, Nurnberger J Jr, Edenberg HJ, Porjesz B, Begleiter H, Hesselbrock V, Goate A, Bierut L (2007) Association of CHRM2 with IQ: converging evidence for a gene influencing intelligence. Behav Genet 37:265–272
Dickens WT, Flynn JR (2001) Heritability estimates versus large environmental effects: the IQ paradox resolved. Psychol Rev 108:346–369
Doyle AE, Ferreira MA, Sklar PB, Lasky-Su J, Petty C, Fusillo SJ, Seidman LJ, Willcutt EG, Smoller JW, Purcell S, Biederman J, Faraone SV (2008) Multivariate genomewide linkage scan of neurocognitive traits and ADHD symptoms: suggestive linkage to 3q13. Am J Med Genet B Neuropsychiatr Genet 147B:1399–1411
Edmonds CJ, Isaacs EB, Visscher PM, Rogers M, Lanigan J, Singhal A et al (2008) Inspection time and cognitive abilities in twins aged 7 to 17 years: age-related changes, heritability, and genetic covariance. Intelligence 36:210–225
Egan MF, Goldberg TE, Kolachana BS, Callicott JH, Mazzanti CM, Straub RE, Goldman D, Weinberger DR (2001) Effect of COMT Val108/158 Met genotype on frontal lobe function and risk for schizophrenia. Proc Natl Acad Sci USA 98:6917–6922
Egan MF, Kojima M, Callicott JH, Goldberg TE, Kolachana BS, Bertolino A, Zaitsev E, Gold B, Goldman D, Dean M, Lu B, Weinberger DR (2003) The BDNF val66met polymorphism affects activity-dependent secretion of BDNF and human memory and hippocampal function. Cell 112:257–269
Eysenck HJ (1939) Primary mental abilities. Br J Educ Psychol 9:270–275
Finkel D, Pedersen NL, McGue M, McClearn GE (1995) Heritability of cognitive abilities in adult twins: comparison of Minnesota and Swedish data. Behav Genet 25:421–431
Fisher SE (2006) Tangled webs: tracing the connections between genes and cognition. Cognition 101:270–297
Flynn JR (1995) IQ gains over time. In: Sternberg RJ (ed) Encyclopedia of human intelligence. Simon and Schuster Macmillan, New York, London, pp 617–623
Flynn JR (2007) What is intelligence? Cambridge University Press, Cambridge
Friedman NP, Miyake A, Young SE, Defries JC, Corley RP, Hewitt JK (2008) Individual differences in executive functions are almost entirely genetic in origin. J Exp Psychol Gen 137:201–225
Froehlich TE, Lanphear BP, Dietrich KN, Cory-Slechta DA, Wang N, Kahn RS (2007) Interactive effects of a DRD4 polymorphism, lead, and sex on executive functions in children. Biol Psychiatry 62:243–249
Galton F (1865) Heredity, talent, and character. Macmillan’s Mag 12:157–166, 318–327
Gardner H (1983) Frames of mind: the theory of multiple intelligences. Basic, New York
Genro JP, Roman T, Zeni CP, Grevet EH, Schmitz M, de Abreu PB, Bau CH, Rohde LA, Hutz MH (2006) No association between dopaminergic polymorphisms and intelligence variability in attention-deficit/hyperactivity disorder. Mol Psychiatry 11:1066–1067
Giedd JN, Schmitt JE, Neale MC (2007) Structural brain magnetic resonance imaging of pediatric twins. Hum Brain Mapp 28:474–481
Goldstein DB, Chikhi L (2002) Human migrations and population structure: what we know and why it matters. Annu Rev Genomics Hum Genet 3:129–152
Gosso MF, de Geus EJ, van Belzen MJ, Polderman TJ, Heutink P, Boomsma DI, Posthuma D (2006a) The SNAP-25 gene is associated with cognitive ability: evidence from a family-based study in two independent Dutch cohorts. Mol Psychiatry 11:878–886
Gosso MF, van Belzen M, de Geus EJ, Polderman JC, Heutink P, Boomsma DI, Posthuma D (2006b) Association between the CHRM2 gene and intelligence in a sample of 304 Dutch families. Genes Brain Behav 5:577–584
Gosso MF, de Geus EJ, Polderman TJ, Boomsma DI, Posthuma D, Heutink P (2007) Exploring the functional role of the CHRM2 gene in human cognition: results from a dense genotyping and brain expression study. BMC Med Genet 8:66
Gosso MF, de Geus EJ, Polderman TJ, Boomsma DI, Heutink P, Posthuma D (2008) Common variants underlying cognitive ability: further evidence for association between the SNAP-25 gene and cognition using a family-based study in two independent Dutch cohorts. Genes Brain Behav 7:355–364
Gottesman II, Gould TD (2003) The endophenotype concept in psychiatry: etymology and strategic intentions. Am J Psychiatry 160:636–645
Gottfredson LS (1997) Why g matters: the complexity of everyday life. Intelligence 24:79–132
Gould SJ (1981) The mismeasure of man, 2nd edn 1996. Norton, New York
Guo G, Stearns E (2002) The social influences on the realization of genetic potential for intellectual development. Soc Forces 80:881–910
Gustafsson JE (1984) A unifying model for the structure of intellectual abilities. Intelligence 8:179–203
Harden KP, Turkheimer E, Loehlin JC (2007) Genotype by environment interaction in adolescents’ cognitive aptitude. Behav Genet 37:273–283
Harris SE, Wright AF, Hayward C, Starr JM, Whalley LJ, Deary IJ (2005) The functional COMT polymorphism, Val 158 Met, is associated with logical memory and the personality trait intellect/imagination in a cohort of healthy 79 year olds. Neurosci Lett 385:1–6
Harris SE, Fox H, Wright AF, Hayward C, Starr JM, Whalley LJ, Deary IJ (2006) The brain-derived neurotrophic factor Val66Met polymorphism is associated with age-related change in reasoning skills. Mol Psychiatry 11:505–513
Harris SE, Fox H, Wright AF, Hayward C, Starr JM, Whalley LJ, Deary IJ (2007) A genetic association analysis of cognitive ability and cognitive ageing using 325 markers for 109 genes associated with oxidative stress or cognition. BMC Genet 8:43–61
Hayes KJ (1962) Genes, drives, and intellect. Psychol Rep 10:299–342
Hegmann JP, Defries JC (1970) Are genetic correlations and environmental correlations correlated? Nature 226:284–286
Herrnstein RJ, Murray CA (1994) The bell curve: intelligence and class structure in American life. Free Press, New York
Higgins JV (1961) An analysis of intelligence of 1, 016 families. University of Minnesota, Minneapolis
Hill L, Chorney MJ, Lubinski D, Thompson LA, Plomin R (2002) A quantitative trait locus not associated with cognitive ability in children: a failure to replicate. Psychol Sci 13:561–562
Hoekstra RA, Bartels M, Boomsma DI (2007) Longitudinal genetic study of verbal and nonverbal IQ from early childhood to young adulthood. Learn Individ Differ 17:97–114
Houlihan LM, Harris SE, Luciano M, Gow AJ, Starr JM, Visscher PM, Deary IJ (2009) Replication study of candidate genes for cognitive abilities: the Lothian Birth Cohort 1936. Genes Brain Behav 8:238–247
Hulshoff Pol HE, Schnack HG, Posthuma D, Mandl RCW, Baare WF, van Oel C, van Haren NE, Collins DL, Evans AC, Amunts K, Buergel U, Zilles K, de Geus E, Boomsma DI, Kahn RS (2006) Genetic contributions to human brain morphology and intelligence. J Neurosci 26:10235–10242
Inlow JK, Restifo LL (2004) Molecular and comparative genetics of mental retardation. Genetics 166:835–881
International Schizophrenia consortium (2008) Rare chromosomal deletions and duplications increase risk of schizophrenia. Nature 455:237–241
Jacobs N, van Os J, Derom C, Thiery E (2007) Heritability of intelligence. Twin Res Hum Genet 10:11–14
Jencks C (1979) Who gets ahead? The determinants of economic success in America. Basic Books, New York
Jensen AR (1998) The g factor: the science of mental ability. Praeger, Westport
Johnson W (2007) Genetic and environmental influences on behavior: capturing all the interplay. Psychol Rev 114:423–440
Johnson W, Bouchard TJ (2005a) Constructive replication of the visual-perceptual-image rotation model in Thurstone’s (1941) battery of 60 tests of mental ability. Intelligence 33:417–430
Johnson W, Bouchard TJ (2005b) The structure of human intelligence: It is verbal, perceptual, and image rotation (VPR), not fluid and crystallized. Intelligence 33:393–416
Johnson W, Bouchard TJ (2007a) Sex differences in mental abilities: g masks the dimensions on which they lie. Intelligence 35:23–39
Johnson W, Bouchard TJ (2007b) Sex differences in mental ability: a proposed means to link them to brain structure and function. Intelligence 35:197–209
Johnson W, Bouchard TJ, Krueger RF, McGue M, Gottesman II (2004) Just one g: consistent results from three test batteries. Intelligence 32:95–107
Johnson W, Bouchard TJ, Mcgue M, Segal NL, Tellegen A, Keyes M, Gottesman II (2007) Genetic and environmental influences on the verbal-perceptual-image rotation (VPR) model of the structure of mental abilities in the Minnesota study of twins reared apart. Intelligence 35:542–562
Johnson W, Jung RE, Colom R, Haier RJ (2008a) Cognitive abilities independent of IQ correlate with regional brain structure. Intelligence 36:18–28
Johnson W, te Nijenhuis J, Bouchard TJ (2008b) Still just 1 g: consistent results from five test batteries. Intelligence 36:81–95
Johnson W, Carothers A, Deary IJ (2009) A role for the X chromosome in sex differences in variability in general intelligence? Perspect Psychol Sci (in press)
Jung RE, Haier RJ (2007) The parieto-frontal integration theory (P-FIT) of intelligence: converging neuroimaging evidence. Behav Brain Sci 30:135–154
Kachiwala SJ, Harris SE, Wright AF, Hayward C, Starr JM, Whalley LJ, Deary IJ (2005) Genetic influences on oxidative stress and their association with normal cognitive ageing. Neurosci Lett 386:116–120
Korenman S, Winship C (2000) A re-analysis of the Bell curve: intelligence, family background, and schooling. In: Arrow K, Bowles S, Durlauf S (eds) Meritocracy and income inequality. Princeton University Press, Princeton, pp 137–178
Kovas Y, Plomin R (2006) Generalist genes: implications for the cognitive sciences. Trends Cogn Sci 10:198–203
Lambert JC, Ferreira S, Gussekloo J, Christiansen L, Brysbaert G, Slagboom E, Cottel D, Petit T, Hauw JJ, DeKosky ST, Richard F, Berr C, Lendon C, Kamboh MI, Mann D, Christensen K, Westendorp R, Amouyel P (2007) Evidence for the association of the S100beta gene with low cognitive performance and dementia in the elderly. Mol Psychiatry 12:870–880
Lander E, Kruglyak L (1995) Genetic dissection of complex traits: guidelines for interpreting and reporting linkage results. Nat Genet 11:241–247
Laumonnier F, Cuthbert PC, Grant SGN (2007) The role of neuronal complexes in human X-linked brain diseases. Am J Hum Genet 80:205–220
Lenroot RK, Schmitt JE, Ordaz SJ, Wallace GL, Neale MC, Lerch JP, Kendler KS, Evans AC, Giedd JN (2009) Differences in genetic and environmental influences on the human cerebral cortex associated with development during childhood and adolescence. Hum Brain Mapp 30:163–174
Lerer E, Levi S, Salomon S, Darvasi A, Yirmiya N, Ebstein RP (2008) Association between the oxytocin receptor (OXTR) gene and autism: relationship to Vineland Adaptive Behavior Scales and cognition. Mol Psychiatry 13:980–988
Luciano M, Wright MJ, Duffy DL, Wainwright MA, Zhu G, Evans DM, Geffen GM, Montgomery GW, Martin NG (2006) Genome-wide scan of IQ finds significant linkage to a quantitative trait locus on 2q. Behav Genet 36:45–55
Luciano M, Lind PA, Deary IJ, Payton A, Posthuma D, Butcher LM, Bochdanovits Z, Whalley LJ, Visscher PM, Harris SE, Polderman TJ, Davis OS, Wright MJ, Starr JM, de Geus EJ, Bates TC, Montgomery GW, Boomsma DI, Martin NG, Plomin R (2008) Testing replication of a 5-SNP set for general cognitive ability in six population samples. Eur J Hum Genet 16:1388–13895
Maher B (2008) Personal genomes: the case of the missing heritability. Nature 456:18–21
McCartney K, Harris MJ, Bernieri F (1990) Growing up and growing apart: a developmental meta-analysis of twin studies. Psychol Bull 107:226–237
McClellan JM, Susser E, King MC (2007) Schizophrenia: a common disease caused by multiple rare alleles. Br J Psychiatry 190:194–199
McDaniel MA (2005) Big-brained people are smarter: a meta-analysis of the relationship between in vivo brain volume and intelligence. Intelligence 33:337–346
McGue M, Bouchard TJ, Iacono WG, Lykken DT (1993) Behavioral genetics of cognitive ability: A life-span perspective. In: R. Plomin, McClearn GE (eds) Nature, Nurture, and Psychology American Psychological Association, Washington, DC, pp 59–76
McQuillan R, Leutenegger AL, Abdel-Rahman R, Franklin CS, Pericic M, Barac-Lauc L, Smolej-Narancic N, Janicijevic B, Polasek O, Tenesa A, Macleod AK, Farrington SM, Rudan P, Hayward C, Vitart V, Rudan I, Wild SH, Dunlop MG, Wright AF, Campbell H, Wilson JF (2008) Runs of homozygosity in European populations. Am J Hum Genet 83:359–372
Mekel-Bobrov N, Posthuma D, Gilbert SL, Lind P, Gosso MF, Luciano M, Harris SE, Bates TC, Polderman TJ, Whalley LJ, Fox H, Starr JM, Evans PD, Montgomery GW, Fernandes C, Heutink P, Martin NG, Boomsma DI, Deary IJ, Wright MJ, de Geus EJ, Lahn BT (2007) The ongoing adaptive evolution of ASPM and microcephalin is not explained by increased intelligence. Hum Mol Genet 16:600–608
Meyer-Lindenberg A, Straub RE, Lipska BK, Verchinski BA, Goldberg T, Callicott JH, Egan MF, Huffaker SS, Mattay VS, Kolachana B, Kleinman JE, Weinberger DR (2007) Genetic evidence implicating DARPP-32 in human frontostriatal structure, function, and cognition. J Clin Invest 117:672–682
Mill J, Caspi A, Williams BS, Craig I, Taylor A, Polo-Tomas M, Berridge CW, Poulton R, Moffitt TE (2006) Prediction of heterogeneity in intelligence and adult prognosis by genetic polymorphisms in the dopamine system among children with attention-deficit/hyperactivity disorder: evidence from 2 birth cohorts. Arch Gen Psychiatry 63:462–469
Mingroni MA (2007) Resolving the IQ paradox: heterosis as a cause of the Flynn effect and other trends. Psychol Rev 114:806–829
Moffitt TE, Caspi A, Rutter M (2006) Measured gene-environment interactions in psychopathology: concepts, research strategies, and implications for research, intervention, and public understanding of genetics. Perspect Psychol Sci 1:5–27
Moises HW, Frieboes RM, Spelzhaus P, Yang L, Kohnke M, Herden-Kirchhoff O, Vetter P, Neppert J, Gottesman II (2001) No association between dopamine D2 receptor gene (DRD2) and human intelligence. Perspect Psychol Sci 108:115–121
CAS Google Scholar
Nichols RC (1978) Twin studies of ability, personality and interests. Homo 29:158–173
Payton A (2006) Investigating cognitive genetics and its implications for the treatment of cognitive deficit. Genes Brain Behav 5:44–53
Payton A, Holland F, Diggle P, Rabbitt P, Horan M, Davidson Y, Gibbons L, Worthington J, Ollier WE, Pendleton N (2003) Cathepsin D exon 2 polymorphism associated with general intelligence in a healthy older population. Mol Psychiatry 8:14–18
Payton A, van den Boogerd E, Davidson Y, Gibbons L, Ollier W, Rabbitt P, Worthington J, Horan M, Pendleton N (2006) Influence and interactions of cathepsin D, HLA-DRB1 and APOE on cognitive abilities in an older non-demented population. Genes Brain Behav 5(Suppl 1):23–31
Pedersen NL, Plomin R, Nesselroade JR, Mcclearn GE (1992) A quantitative genetic analysis of cognitive abilities during the 2nd half of the life-span. Psychol Sci 3:346–353
Penke L, Denissen JJA, Miller GF (2007) The evolutionary genetics of personality. Eur J Pers 21:549–587
Pennington BF, Filipek PA, Lefly D, Chhabildas R, Kennedy DN, Simon JH, Filley CM, Galaburda A, DeFries JC (2000) A twin MRI study of size variations in the human brain. J Cogn Neurosci 12:223–232
Peper JS, Brouwer RM, Boomsma DI, Kahn RS, Poll HEH (2007) Genetic influences on human brain structure: a review of brain imaging studies in twins. Hum Brain Mapp 28:464–473
Plomin R (1986) Development, genes, and psychology. Erlbaum, Hillsdale
Plomin R, Fulker DW, Corley R, DeFries JC (1997) Nature, nurture, and cognitive development from 1 to 16 years: a parent-offspring adoption study. Psychol Sci 8:442–447
Plomin R, Turic DM, Hill L, Turic DE, Stephens M, Williams J, Owen MJ, O’Donovan MC (2004) A functional polymorphism in the succinate-semialdehyde dehydrogenase (aldehyde dehydrogenase 5 family, member A1) gene is associated with cognitive ability. Mol Psychiatry 9:582–586
Plomin R, Kennedy JKJ, Craig IW (2006) The quest for quantitative trait loci associated with intelligence. Intelligence 34:513–526
Plomin R, DeFries JC, McClearn GE, McGuffin P (2008) Behavioral genetics, 5th edn. W. H. Freeman, New York
Porteous DJ, Thomson P, Brandon NJ, Millar JK (2006) The genetics and biology of DISC1: an emerging role in psychosis and cognition. Biol Psychiatry 60:123–131
Posthuma D, de Geus EJC (2006) Progress in the molecular-genetic study of intelligence. Curr Dir Psychol Sci 15:151–155
Posthuma D, de Geus EJ, Boomsma DI (2001) Perceptual speed and IQ are associated through common genetic factors. Behav Genet 31:593–602
Posthuma D, De Geus EJC, Baare WFC, Pol HEH, Kahn RS, Boomsma DI (2002) The association between brain volume and intelligence is of genetic origin. Nat Neurosci 5:83–84
Posthuma D, Baare WFC, Pol HEH, Kahn RS, Boomsma DI, De Geus EJC (2003) Genetic correlations between brain volumes and the WAIS-III dimensions of verbal comprehension, working memory, perceptual organization, and processing speed. Twin Res 6:131–139
Posthuma D, Luciano M, Geus EJ, Wright MJ, Slagboom PE, Montgomery GW, Boomsma DI, Martin NG (2005) A genomewide scan for intelligence identifies quantitative trait loci on 2q and 6p. Am J Hum Genet 77:318–326
Reynolds CA, Gatz M, Berg S, Pedersen NL (2007) Genotype-environment interactions: cognitive aging and social factors. Twin Res Hum Genet 10:241–254
Rigbi A, Kanyas K, Yakir A, Greenbaum L, Pollak Y, Ben-Asher E, Lancet D, Kertzman S, Lerer B (2008) Why do young women smoke? V. Role of direct and interactive effects of nicotinic cholinergic receptor gene variation on neurocognitive function. Genes Brain Behav 7:164–172
Rijsdijk FV, Vernon PA, Boomsma DI (2002) Application of hierarchical genetic models to Raven and WAIS subtests: a Dutch twin study. Behav Genet 32:199–210
Roberts RD, Stankov L (1999) Individual differences in speed of mental processing and human cognitive abilities: Toward a taxonomic model. Learn Individ Differ 11:1–120
Ronnlund M, Nilsson LG (2008) The magnitude, generality, and determinants of Flynn effects on forms of declarative memory and visuospatial ability: Time-sequential analyses of data from a Swedish cohort study. Intelligence 36:192–209
Ropers HH, Hamel BC (2005) X-linked mental retardation. Nat Rev Genet 6:46–57
Ross MT, Grafham DV, Coffey AJ, Scherer S, McLay K et al (2005) The DNA sequence of the human X chromosome. Nature 434:325–337
Rujescu D, Meisenzahl EM, Krejcova S, Giegling I, Zetzsche T, Reiser M, Born CM, Moller HJ, Veske A, Gal A, Finckh U (2007) Plexin B3 is genetically associated with verbal performance and white matter volume in human brain. Mol Psychiatry 12:190–194, 115
Rushton JP, Vernon PA, Bons TA (2007) No evidence that polymorphisms of brain regulator genes Microcephalin and ASPM are associated with general mental ability, head circumference or altruism. Biol Lett 3:157–160
Scarr S, Weinberg RA (1977) Intellectual similarities within families of both adopted and biological children. Intelligence 1:170–191
Shaw P, Greenstein D, Lerch J, Clasen L, Lenroot R, Gogtay N, Evans A, Rapoport J, Giedd J (2006) Intellectual ability and cortical development in children and adolescents. Nature 440:676–679
Shimokata H, Ando F, Niino N, Miyasaka K, Funakoshi A (2005) Cholecystokinin A receptor gene promoter polymorphism and intelligence. Ann Epidemiol 15:196–201
Skodak M (1950) Mental growth of adopted children in the same family. J Genet Psychol 77:3–9
Skodak M, Skeels HM (1949) A final follow-up study of 100 adopted children. J Genet Psychol 75:85–125
Skuse DH (2005) X-linked genes and mental functioning. Hum Mol Genet 14 Spec No 1:R27–R32
Small BJ, Rosnick CB, Fratiglioni L, Backman L (2004) Apolipoprotein E and cognitive performance: a meta-analysis. Psychol Aging 19:592–600
Sowell ER, Thompson PM, Holmes CJ, Jernigan TL, Toga AW (1999) In vivo evidence for post-adolescent brain maturation in frontal and striatal regions. Nat Neurosci 2:859–861
Spearman C (1904) “General intelligence” objectively determined and measured. Am J Psychol 15:201–292
Spearman C (1927) The abilities of man. Macmillan, London
Spinath FM, Ronald A, Harlaar N, Price TS, Plomin R (2003) Phenotypic g early in life: on the etiology of general cognitive ability in a large population sample of twin children aged 2–4 years. Intelligence 31:195–210
Strenze T (2007) Intelligence and socioeconomic success: a meta-analytic review of longitudinal research. Intelligence 35:401–426
Teasdale TW, Owen DR (2008) Secular declines in cognitive test scores: a reversal of the Flynn effect. Intelligence 36:121–126
Thompson PM, Cannon TD, Narr KL, van Erp T, Poutanen VP, Huttunen M, Lonnqvist J, Standertskjold-Nordenstam CG, Kaprio J, Khaledy M, Dail R, Zoumalan CI, Toga AW (2001) Genetic influences on brain structure. Nat Neurosci 4:1253–1258
Thomson PA, Harris SE, Starr JM, Whalley LJ, Porteous DJ, Deary IJ (2005) Association between genotype at an exonic SNP in DISC1 and normal cognitive aging. Neurosci Lett 389:41–45
Thurstone LL (1938) Primary mental abilities. Psychometric Monographs, vol 1
Turkheimer E, Haley A, Waldron M, D’Onofrio B, Gottesman II (2003) Socioeconomic status modifies heritability of IQ in young children. Psychol Sci 14:623–628
Ucok A, Alpsan H, Cakir S, Saruhan-Direskeneli G (2007) Association of a serotonin receptor 2A gene polymorphism with cognitive functions in patients with schizophrenia. Am J Med Genet B Neuropsychiatr Genet 144B:704–707
van den Oord EJCG, Rowe DC (1997) An examination of genotype-environment interactions for academic achievement in an US national longitudinal survey. Intelligence 25:205–228
Article Google Scholar
van der Maas HLJ, Dolan CV, Grasman RPPP, Wicherts JM, Huizenga HA, Raijmakers MEJ (2006) A dynamical model of general intelligence: the positive manifold of intelligence by mutualism. Psychol Rev 113:842–861
van der Sluis S, Willemsen G, de Geus EJ, Boomsma DI, Posthuma D (2008) Gene-environment interaction in adults’ IQ scores: measures of past and present environment. Behav Genet 38:348–360
Visser BA, Ashton MC, Vernon PA (2006) Beyond g: putting multiple intelligences theory to the test. Intelligence 34:487–502
Waller JH (1971) Achievement and social mobility: relationships among IQ score, education, and occupation in two generations. Soc Biol 18:252–259
Watkins MW (2006) Orthogonal higher order structure of the Wechsler Intelligence Scale for Children: fourth edition. Psychol Assess 18:123–125
Wilson RS (1978) Synchronies in mental development: epigenetic perspective. Behav Genet 8:575–576
Wright MJ, Gillespie NA, Luciano M, Zhu G, Martin NG (2008) Genetics of personality and cognition in adolescents. In: Hudziak JJ (ed) Developmental psychopathology and wellness: genetic and environmental influences, 1st edn. American Psychiatric Publishing Inc, Washington, pp 85–108
Download references
Acknowledgments
The work was undertaken by The University of Edinburgh Centre for Cognitive Ageing and Cognitive Epidemiology, part of the cross council Lifelong Health and Wellbeing Initiative. Funding from the BBSRC, EPSRC, ESRC, and MRC is gratefully acknowledged. Wendy Johnson is supported by a Research Councils UK Fellowship. Lorna Houlihan is supported by a grant to Ian Deary from Help the Aged (The Disconnected Mind Project).
Author information
Authors and affiliations.
Department of Psychology, Centre for Cognitive Ageing and Cognitive Epidemiology, University of Edinburgh, 7 George Square, Edinburgh, EH8 9JZ, Scotland, UK
Ian J. Deary, W. Johnson & L. M. Houlihan
You can also search for this author in PubMed Google Scholar
Corresponding author
Correspondence to Ian J. Deary .
Rights and permissions
Reprints and permissions
About this article
Deary, I.J., Johnson, W. & Houlihan, L.M. Genetic foundations of human intelligence. Hum Genet 126 , 215–232 (2009). https://doi.org/10.1007/s00439-009-0655-4
Download citation
Received : 13 January 2009
Accepted : 08 March 2009
Published : 18 March 2009
Issue Date : July 2009
DOI : https://doi.org/10.1007/s00439-009-0655-4
Share this article
Anyone you share the following link with will be able to read this content:
Sorry, a shareable link is not currently available for this article.
Provided by the Springer Nature SharedIt content-sharing initiative
- Genetic Influence
- General Cognitive Ability
- Nonverbal Ability
- Nonverbal Reasoning
- Intelligence Difference
- Find a journal
- Publish with us
- Track your research
Thank you for visiting nature.com. You are using a browser version with limited support for CSS. To obtain the best experience, we recommend you use a more up to date browser (or turn off compatibility mode in Internet Explorer). In the meantime, to ensure continued support, we are displaying the site without styles and JavaScript.
- View all journals
- Explore content
- About the journal
- Publish with us
- Sign up for alerts
- Perspective
- Published: 02 April 2024
Uniquely human intelligence arose from expanded information capacity
- Jessica F. Cantlon ORCID: orcid.org/0000-0003-0207-5615 1 , 2 &
- Steven T. Piantadosi 3 , 4
Nature Reviews Psychology volume 3 , pages 275–293 ( 2024 ) Cite this article
7510 Accesses
1 Citations
202 Altmetric
Metrics details
- Animal behaviour
- Human behaviour
- Intelligence
Most theories of how human cognition is unique propose specific representational capacities or biases, often thought to arise through evolutionary change. In this Perspective, we argue that the evidence that supports these domain-specific theories is confounded by general information-processing differences. We argue that human uniqueness arises through genetic quantitative increases in the global capacity to process information and share it among systems such as memory, attention and learning. This change explains regularities across numerous subdomains of cognition, behavioural comparisons between species and phenomena in child development. This strict evolutionary continuity theory of human intelligence is consistent with comparative evidence about neural evolution and computational constraints of memory on the ability to represent rules, patterns and abstract generalizations. We show how these differences in the degree of information processing capacity yield differences in kind for human cognition relative to other animals.
This is a preview of subscription content, access via your institution
Access options
Subscribe to this journal
Receive 12 digital issues and online access to articles
$59.00 per year
only $4.92 per issue
Buy this article
- Purchase on SpringerLink
- Instant access to full article PDF
Prices may be subject to local taxes which are calculated during checkout
Similar content being viewed by others
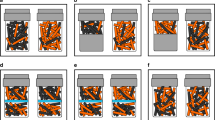
Kea show three signatures of domain-general statistical inference
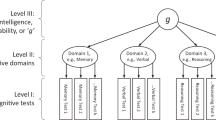
Genetic variation, brain, and intelligence differences
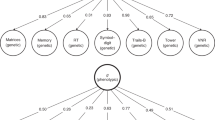
A general dimension of genetic sharing across diverse cognitive traits inferred from molecular data
Burkart, J. M., Schubiger, M. N. & van Schaik, C. P. The evolution of general intelligence. Behav. Brain Sci. 40 , e195 (2017).
Article PubMed Google Scholar
Cosmides, L. & Tooby, J. in The New Cognitive Neurosciences 2nd edn (Gazzaniga, M. S.) 1259–1270 (MIT Press, 2000).
Dehaene, S., Al Roumi, F., Lakretz, Y., Planton, S. & Sablé-Meyer, M. Symbols and mental programs: a hypothesis about human singularity. Trends Cogn. Sci. 26 , 751–766 (2022).
Pinker, S. On language. J. Cogn. Neurosci. 6 , 92–98 (1994).
Pinker, S. in Understanding Moral Sentiments (eds Putnam, H. et al.) 59–80 (Routledge, 2017).
Pinker, S. & Jackendoff, R. The faculty of language: what’s special about it? Cognition 95 , 201–236 (2005).
Povinelli, D. J., Bering, J. M. & Giambrone, S. Toward a science of other minds: escaping the argument by analogy. Cogn. Sci. 24 , 509–541 (2000).
Article Google Scholar
Wellman, H. M. Theory of mind: the state of the art. Eur. J. Dev. Psychol. 15 , 728–755 (2018).
Deacon, T. W. The Symbolic Species: The Co-Evolution of Language and the Brain No. 202 (W. W. Norton, 1998).
Deaner, R. O., Van Schaik, C. P. & Johnson, V. Do some taxa have better domain-general cognition than others? A meta-analysis of nonhuman primate studies. Evol. Psychol . 4 , https://doi.org/10.1177/147470490600400114 (2006).
Greenfield, P. M. Language, tools and brain: the ontogeny and phylogeny of hierarchically organized sequential behavior. Behav. Brain Sci. 14 , 531–551 (1991).
Henrich, J. The Secret of Our Success (Princeton Univ. Press, 2015).
Heyes, C. Cognitive Gadgets: The Cultural Evolution of Thinking (Harvard Univ. Press, 2018).
Passingham, R. E. & Wise, S. P. The Neurobiology of the Prefrontal Cortex: Anatomy, Evolution, and the origin of Insight (Oxford Univ. Press, 2012).
Premack, D. Is language the key to human intelligence? Science 303 , 318–320 (2004).
Premack, D. Why humans are unique: three theories. Perspect. Psychol. Sci. 5 , 22–32 (2010).
Reader, S. M., Hager, Y. & Laland, K. N. The evolution of primate general and cultural intelligence. Philos. Trans. R. Soc. Lond. B Biol. Sci. 366 , 1017–1027 (2011).
Article PubMed PubMed Central Google Scholar
Laland, K. & Seed, A. Understanding human cognitive uniqueness. Annu. Rev. Psychol. 72 , 689–716 (2021).
Tomasello, M. The ultra‐social animal. Eur. J. Soc. Psychol. 44 , 187–194 (2014).
Laland, K. N. Darwin’s Unfinished Symphony: How Culture Made the Human Mind (Princeton Univ. Press, 2017).
Richerson, P. J. & Boyd, R. Not by Genes Alone: How Culture Transformed Human Evolution (Univ. of Chicago Press, 2018).
Sterelny, K. The Evolved Apprentice (MIT Press, 2012).
Turner, C. R. & Walmsley, L. D. Preparedness in cultural learning. Synthese 199 , 81–100 (2021).
Laland, K. N. & Brown, G. R. Sense and Nonsense: Evolutionary Perspectives on Human Behaviour (Oxford Univ. Press, 2011).
Laland, K. N. & Reader, S. M. in Innovation in Cultural Systems: Contributions from Evolutionary Anthropology (eds O’Brien, M. J. & Shennan, S. J.) 37–51 (2010).
Lefebvre, L., Whittle, P., Lascaris, E. & Finkelstein, A. Feeding innovations and forebrain size in birds. Anim. Behav. 53 , 549–560 (1997).
MacLean, E. L. Unraveling the evolution of uniquely human cognition. Proc. Natl Acad. Sci. USA 113 , 6348–6354 (2016).
Moll, H. & Tomasello, M. Cooperation and human cognition: the Vygotskian intelligence hypothesis. Philos Trans R. Soc. Lond. B Biol. Sci. 362 , 639–648 (2007).
Reader, S. M. & Laland, K. N. Social intelligence, innovation, and enhanced brain size in primates. Proc. Natl Acad. Sci. USA 99 , 4436–4441 (2002).
Sol, D., Bacher, S., Reader, S. M. & Lefebvre, L. Brain size predicts the success of mammal species introduced into novel environments. Am. Nat. 172 , S63–S71 (2008).
Tomasello, M. A Natural History of Human Thinking (Harvard Univ. Press, 2018).
Tomasello, M., Kruger, A. C. & Ratner, H. H. Cultural learning. Behav. Brain Sci. 16 , 495–511 (1993).
Van Schaik, C. P. & Burkart, J. M. Social learning and evolution: the cultural intelligence hypothesis. Philos. Trans. R. Soc. Lond. B, Biol. Sci. 366 , 1008–1016 (2011).
Shannon, C. E. A mathematical theory of communication. Bell Syst. Tech. J. 27 , 379–423 (1948).
Stone, J. V. Information Theory: A Tutorial Introduction (Sebtel, 2015).
Wilson, E. O. Sociobiology: The New Synthesis (Harvard Univ. Press, 2000).
Lumsden, C. J. & Wilson, E. O. Précis of genes, mind, and culture. Behav. Brain Sci. 5 , 1–7 (1982).
Barkow, J. H., Cosmides, L. & Tooby, J. (eds) The Adapted Mind: Evolutionary Psychology and the Generation of Culture (Oxford Univ. Press, 1995).
Pinker, S. & Bloom, P. Natural language and natural selection. Behav. Brain Sci. 13 , 707–727 (1990).
Chomsky, N. Persistent topics in linguistic theory. Diogenes 13 , 13–20 (1965).
Garcia, J., Kimeldorf, D. & Koelling, R. Conditioned aversion to saccharin resulting from exposure to gamma radiation. Science 122 , 157–158 (1955).
Ermer, E., Cosmides, L. & Tooby, J. in The Evolution of Mind: Fundamental Questions and Controversies (eds Gangestad, S. W. & Simpson, J. A.) 153–160 (2007).
Sperber, D. in Language, Brain and Cognitive Development: Essays in Honor of Jacques Mehler Vol. 7 (ed. Dupoux, E.) 47–57 (MIT Press, 2001).
Scholl, B. J. & Leslie, A. M. Modularity, development and ‘theory of mind’. Mind Lang. 14 , 131–153 (1999).
Leslie, A. M., Friedman, O. & German, T. P. Core mechanisms in ‘theory of mind’. Trends Cogn. Sci. 8 , 528–533 (2004).
Meltzoff, A. N. & Williamson, R. A. in Imitation: Social, Cognitive, and Theoretical Perspectives (ed. Zelazo, P. D.) 651–682 (Oxford Academic, 2013).
Tomasello, M., Carpenter, M., Call, J., Behne, T. & Moll, H. Understanding and sharing intentions: the origins of cultural cognition. Behav. Brain Sci. 28 , 675–735 (2005).
Rule, J. S., Tenenbaum, J. B. & Piantadosi, S. T. The child as hacker. Trends Cogn. Sci. 24 , 900–915 (2020).
Sablé-Meyer et al. Sensitivity to geometric shape regularity in humans and baboons: a putative signature of human singularity. Proc. Natl Acad. Sci. USA 118 , e2023123118 (2021).
Premack, D. The codes of man and beasts. Behav. Brain Sci. 6 , 125–137 (1983).
Hauser, M. D., Chomsky, N. & Fitch, W. T. The faculty of language: what is it, who has it, and how did it evolve? Science 298 , 1569–1579 (2002).
Penn, D. C., Holyoak, K. J. & Povinelli, D. J. Darwin’s mistake: explaining the discontinuity between human and nonhuman minds. Behav. Brain Sci. 31 , 109–130 (2008).
Chomsky, N. The Minimalist Program (MIT Press, 1995).
Fisher, S. E. & Marcus, G. F. The eloquent ape: genes, brains and the evolution of language. Nat. Rev. Genet. 7 , 9–20 (2006).
Berwick, R. C. & Chomsky, N. Why Only Us: Language and Evolution (MIT Press, 2016).
Herrmann, E., Call, J., Hernández-Lloreda, M. V., Hare, B. & Tomasello, M. Humans have evolved specialized skills of social cognition: the cultural intelligence hypothesis. Science 317 , 1360–1366 (2007).
Hodos, W. in The Neurosciences: Second Study Program (ed. Schmidt, F. O.) 26–39 (Rockefeller Univ. Press, 1970).
Jerison, H. J. Animal intelligence as encephalization. Philos. Trans. R. Soc. B 308 , 21–35 (1985).
Passingham, R. E. Visual discrimination learning after selective prefrontal ablations in monkeys ( Macaca mulatta ). Neuropsychologia 10 , 27-39 (1972).
Passingham, R. Understanding the Prefrontal Cortex: Selective Advantage, Connectivity, and Neural Operations (Oxford Univ. Press, 2021).
Bolhuis, J. J., Brown, G. R., Richardson, R. C. & Laland, K. N. Darwin in mind: new opportunities for evolutionary psychology. PLoS Biol. 9 , e1001109 (2011).
Christiansen, M. H. & Chater, N. Creating Language: Integrating Evolution, Acquisition, and Processing (MIT Press, 2016).
Sol, D. Revisiting the cognitive buffer hypothesis for the evolution of large brains. Biol. Lett. 5 , 130–133 (2009).
Carey, S., Zaitchik, D. & Bascandziev, I. Theories of development: in dialog with Jean Piaget. Dev. Rev. 38 , 36–54 (2015).
Christie, S. & Gentner, D. Language helps children succeed on a classic analogy task. Cogn. Sci. 38 , 383–397 (2014).
Halford, G. S., Wilson, W. H. & Phillips, S. Processing capacity defined by relational complexity: implications for comparative, developmental, and cognitive psychology. Behav. Brain Sci. 21 , 803–831 (1998).
Stone, J. V. Principles of Neural Information Theory (Sebtel, 2018).
Sterling, P. & Laughlin, S. Principles of Neural Design (MIT Press, 2015).
Humphrey, N. K. The Social Function of Intellect (Cambridge Univ. Press, 1976).
Byrne, R. W. & Whiten, A. Machiavellian Intelligence: Social Expertise and the Evolution of Intellect in Monkeys, Apes, and Humans (Oxford Univ. Press, 1988).
Harris, P. L. in Handbook of Child Psychology: Cognition, Perception, and Language (eds D. Kuhn, R. S. Siegler, W. Damon & R. M. Lerner) 811–858 (Wiley, 2006).
Boyd, J. H. Have we found the Holy Grail? Theory of mind as a unifying construct. J. Relig. Health 47 , 366–385 (2008).
Byrne, R. W. & Bates, L. A. Sociality, evolution and cognition. Curr. Biol. 17 , R714–R723 (2007).
Horschler, D. J., MacLean, E. L. & Santos, L. R. Do non-human primates really represent others’ beliefs? Trends Cogn. Sci. 24 , 594–605 (2020).
Lyons, D. E. & Santos, L. R. Ecology, domain specificity, and the origins of theory of mind: is competition the catalyst? Philos. Compass 1 , 481–492 (2006).
Lewis, L. & Krupenye, C. Theory of mind in nonhuman primates. Behav. Brain Rev. 21 , 101–114 (2021).
Google Scholar
Heyes, C. Animal mindreading: what’s the problem? Psychon. Bull. Rev. 22 , 313–327 (2015).
Hare, B., Call, J. & Tomasello, M. Do chimpanzees know what conspecifics know? Anim. Behav. 61 , 139–151 (2001).
Hare, B., Call, J. & Tomasello, M. Chimpanzees deceive a human competitor by hiding. Cognition 101 , 495–514 (2006).
Kano, F. & Call, J. Cross-species variation in gaze following and conspecific preference among great apes, human infants and adults. Anim. Behav. 91 , 137–150 (2014).
MacLean, E. L. & Hare, B. Bonobos and chimpanzees infer the target of another’s attention. Anim. Behav. 83 , 345–353 (2012).
Horner, V., Whiten, A., Flynn, E. & De Waal, F. B. Faithful replication of foraging techniques along cultural transmission chains by chimpanzees and children. Proc. Natl Acad. Sci. USA 103 , 13878–13883 (2006).
van de Waal, E., Claidière, N. & Whiten, A. Wild vervet monkeys copy alternative methods for opening an artificial fruit. Anim. Cogn. 18 , 617–627 (2015).
Tomasello, M., Call, J. & Hare, B. Five primate species follow the visual gaze of conspecifics. Anim. Behav. 55 , 1063–1069 (1998).
Okamoto-Barth, S. & Tomonaga, M. in Cognitive Development in Chimpanzees 155–171 (Springer Tokyo, 2006).
Carpenter, M. & Call, J. in Agency and Joint Attention (ed. Metcalfe, J. & Terrace, H. S.) 49–61 (2013).
Musgrave, S. et al. Teaching varies with task complexity in wild chimpanzees. Proc. Natl Acad. Sci. USA 117 , 969–976 (2020).
Whiten, A. Social learning: peering deeper into ape culture. Curr. Biol. 29 , R845–R847 (2019).
Drayton, L. A. & Santos, L. R. Capuchins’ ( Cebus apella ) sensitivity to others’ goal-directed actions in a helping context. Anim. Cogn. 17 , 689–700 (2014).
Warneken, F., Chen, F. & Tomasello, M. Cooperative activities in young children and chimpanzees. Child Dev. 77 , 640–663 (2006).
Melis, A. P., Hare, B. & Tomasello, M. Engineering cooperation in chimpanzees: tolerance constraints on cooperation. Anim. Behav. 72 , 275–286 (2006).
Bard, K. A., Bakeman, R., Boysen, S. T. & Leavens, D. A. Emotional engagements predict and enhance social cognition in young chimpanzees. Dev. Sci. 17 , 682–696 (2014).
Gergely, G. & Csibra, G. Teleological reasoning in infancy: the naıve theory of rational action. Trends Cogn. Sci. 7 , 287–292 (2003).
Dean, L. G., Kendal, R. L., Schapiro, S. J., Thierry, B. & Laland, K. N. Identification of the social and cognitive processes underlying human cumulative culture. Science 335 , 1114–1118 (2012).
Hamann, K., Warneken, F., Greenberg, J. R. & Tomasello, M. Collaboration encourages equal sharing in children but not in chimpanzees. Nature 476 , 328–331 (2011).
Heyes, C. False belief in infancy: a fresh look. Dev. Sci. 17 , 647–659 (2014).
Phillips, J. et al. Knowledge before belief. Behav. Brain Sci. 44 , e140 (2021).
Rakoczy, H. Foundations of theory of mind and its development in early childhood. Nat. Rev. Psychol. 1 , 223–235 (2022).
Penn, D. C. & Povinelli, D. On the lack of evidence that non-human animals possess anything remotely resembling a ‘theory of mind’. Philos. Trans. R. Soc. Lond. B Biol. Sci. 362 , 731–744 (2007).
Halford, G. S., Wilson, W. H. & Phillips, S. Relational knowledge: the foundation of higher cognition. Trends Cogn. Sci. 14 , 497–505 (2010).
Heyes, C., Chater, N. & Dwyer, D. M. Sinking in: the peripheral Baldwinisation of human cognition. Trends Cogn. Sci. 24 , 884–899 (2020).
Penn, D. C., Holyoak, K. J., Povinelli, D. J. & Vaesen, K. So, are we the massively lucky species? Behav. Brain Sci. 35 , 236 (2012).
Thompson, R. K. & Oden, D. L. Categorical perception and conceptual judgments by nonhuman primates: the paleological monkey and the analogical ape. Cogn. Sci. 24 , 363–396 (2000).
Wasserman, E. A., Castro, L. & Fagot, J. in APA Handbook of Comparative Psychology (eds. Call, J. et al.) 359–384 (American Psychological Association, 2017).
Flemming, T. M., Beran, M. J. & Washburn, D. A. Disconnect in concept learning by rhesus monkeys ( Macaca mulatta ): judgment of relations and relations-between-relations. J. Exp. Psychol. Anim. B 33 , 55 (2007).
Kotovsky, L. & Gentner, D. Comparison and categorization in the development of relational similarity. Child Dev. 67 , 2797–2822 (1996).
Rattermann, M. J. & Gentner, D. More evidence for a relational shift in the development of analogy: children’s performance on a causal-mapping task. Cogn. Dev. 13 , 453–478 (1998).
Gillan, D. J., Premack, D. & Woodruff, G. Reasoning in the chimpanzee: I. Analogical reasoning. J. Exp. Psychol. Anim. B 7 , 1 (1981).
Thompson, R. K., Oden, D. L. & Boysen, S. T. Language-naive chimpanzees ( Pan troglodytes ) judge relations between relations in a conceptual matching-to-sample task. J. Exp. Psychol. Anim. B 23 , 31 (1997).
Oden, D. L., Thompson, R. K. & Premack, D. in The Analogical Mind: Perspectives from Cognitive Science (eds Gentner, D. et al.) 471–491 (2001).
Christie, S., Gentner, D., Call, J. & Haun, D. B. M. Sensitivity to relational similarity and object similarity in apes and children. Curr. Biol. 26 , 531–535 (2016).
Kennedy, E. H. & Fragaszy, D. M. Analogical reasoning in a capuchin monkey ( Cebus apella ). J. Comp. Psychol. 122 , 167 (2008).
Smirnova, A., Zorina, Z., Obozova, T. & Wasserman, E. Crows spontaneously exhibit analogical reasoning. Curr. Biol. 25 , 256–260 (2015).
Visalberghi, E., Sabbatini, G., Taylor, A. H. & Hunt, G. R. in APA Handbook of Comparative Psychology: Perception, Learning, and Cognition (Call, J., Burghardt, G. M., Pepperberg, I. M., Snowdon, C. T. & Zentall, T.) 673–701 (American Psychological Association, 2017).
Fujita, K., Kuroshima, H. & Asai, S. How do tufted capuchin monkeys ( Cebus apella ) understand causality involved in tool use? J. Exp. Psychol. Anim. B 29 , 233 (2003).
Fragaszy, D., Izar, P., Visalberghi, E., Ottoni, E. B. & Oliveira, M. Wild capuchin monkeys use anvils and stone pounding tools. Am. J. Primatol. 64 , 359–366 (2004).
Beck, S. R., Apperly, I. A., Chappell, J., Guthrie, C. & Cutting, N. Making tools isn’t child’s play. Cognition 119 , 301–306 (2011).
Emery, N. J. & Clayton, N. S. Tool use and physical cognition in birds and mammals. Curr. Opin. Neurobiol. 19 , 27–33 (2009).
Kabadayi, C. & Osvath, M. Ravens parallel great apes in flexible planning for tool-use and bartering. Science 357 , 202–204 (2017).
Gallot, Q. & Gruber, T. Spontaneous use and modification of a feather as a tool in a captive common raven. Ethology 125 , 755–758 (2019).
Ruiz, A. M. & Santos, L. R. in Tool Use in Animals (eds Sanz, C. M., Call, J. & Boesch, C.) 119–133 (Cambridge Univ. Press, 2013).
Povinelli, D. J. Folk Physics for Apes (Oxford Univ. Press, 2000).
Sanz, C. M. & Morgan, D. B. Chimpanzee tool technology in the Goualougo Triangle, Republic of Congo. J. Hum. Evol. 52 , 420–433 (2007).
Visalberghi, E. & Limongelli, L. Lack of comprehension of cause–effect relations in tool-using capuchin monkeys ( Cebus apella ). J. Comp. Psychol. 108 , 15–22 (1994).
Seed, A. M., Tebbich, S., Emery, N. J. & Clayton, N. S. Investigating physical cognition in rooks, Corvus frugilegus . Curr. Biol. 16 , 697–701 (2006).
Seed, A., Hanus, D. & Call, J. in Tool Use and Causal Cognition (eds McCormack, T. et al.) 89–110 (Oxford Scholarship Online, 2011).
Want, S. C. & Harris, P. L. Learning from other people’s mistakes: causal understanding in learning to use a tool. Child Dev. 72 , 431–443 (2001).
Corballis, M. C. The Recursive Mind (Princeton Univ. Press, 2011).
Karlsson, F. Constraints on multiple center-embedding of clauses. J. Linguist. 43 , 365–392 (2007).
Gibson, E. & Thomas, J. Memory limitations and structural forgetting: the perception of complex ungrammatical sentences as grammatical. Lang. Cogn. Proc. 14 , 225 (1999).
Everett, D. Cultural constraints on grammar and cognition in Pirahã: another look at the design features of human language. Curr. Anthropol. 46 , 621–646 (2005).
Futrell, R., Stearns, L., Everett, D. L., Piantadosi, S. T. & Gibson, E. A corpus investigation of syntactic embedding in Pirahã. PLoS ONE 11 , e0145289 (2016).
Pullum, G. K. in Current Controversies in Philosophy of Cognitive Science (eds. Lerner, A. J. et al.) 29–45 (Routledge, 2020).
Frank, S. L., Bod, R. & Christiansen, M. H. How hierarchical is language use? Proc. Biol. Sci. 279 , 4522–4531 (2012).
PubMed PubMed Central Google Scholar
Frank, S. L. & Bod, R. Insensitivity of the human sentence-processing system to hierarchical structure. Psychol. Sci. 22 , 829–834 (2011).
Nevins, A., Pesetsky, D. & Rodrigues, C. Evidence and argumentation: a reply to Everett (2009). Language 85 , 671–681 (2009).
Nevins, A., Pesetsky, D. & Rodrigues, C. Pirahã exceptionality: a reassessment. Language 85 , 355–404 (2009).
Everett, D. L. Cultural constraints on grammar in Pirahã: a reply to Nevins, Pesetsky, and Rodrigues. semantics.uchicago.edu , https://semantics.uchicago.edu/kennedy/classes/s07/myths/everett07.pdf (2007).
Everett, D. L. The shrinking Chomskyan corner: a final reply to Nevins, Pesetsky, and Rodrigues. LingBuzz eprints 994 (2013).
Bolhuis, J. J., Tattersall, I., Chomsky, N. & Berwick, R. C. How could language have evolved? PLoS Biol. 12 , e1001934 (2014).
Martins, P. T. & Boeckx, C. Language evolution and complexity considerations: the no half-merge fallacy. PLoS Biol. 17 , e3000389 (2019).
De Boer, B., Thompson, B., Ravignani, A. & Boeckx, C. Evolutionary dynamics do not motivate a single-mutant theory of human language. Sci. Rep. 10 , 1–9 (2020).
Hockett, C. F. The origin of speech. Sci. Am. 203 , 88–97 (1960).
Tomasello, M. Origins of Human Communication (MIT Press, 2010).
Gibson, E. et al. How efficiency shapes human language. Trends Cogn. Sci. 23 , 389–407 (2019).
Piantadosi, S. T., Tily, H. & Gibson, E. The communicative function of ambiguity in language. Cognition 122 , 280–291 (2012).
Kemp, C., Xu, Y. & Regier, T. Semantic typology and efficient communication. Ann. Rev. Linguist. 4 , 109–128 (2018).
Kemp, C. & Regier, T. Kinship categories across languages reflect general communicative principles. Science 336 , 1049–1054 (2012).
Mollica, F. et al. The forms and meanings of grammatical markers support efficient communication. Proc. Natl Acad. Sci. USA 118 , e2025993118 (2021).
Futrell, R. & Hahn, M. Information theory as a bridge between language function and language form. Front. Commun . 7 , https://doi.org/10.3389/fcomm.2022.657725 (2022).
Dąbrowska, E. What exactly is universal grammar, and has anyone seen it? Front Psychol 6 , 852 (2015).
Scholz, B. C. & Pullum, G. K. in Contemporary Debates in Cognitive Science (ed. Stainton, R.) 59–80 (2006).
Pullum, G. K. Theorizing about the syntax of human language: a radical alternative to generative formalisms. Cadernos de Linguística 1 , 1–33 (2020).
Tomasello, M. Language is not an instinct. Cogn. Dev. 10 , 131–156 (1995).
Evans, N. & Levinson, S. C. The myth of language universals: language diversity and its importance for cognitive science. Behav. Brain Sci. 32 , 429–448 (2009).
Fitch, W. T. Toward a computational framework for cognitive biology: unifying approaches from cognitive neuroscience and comparative cognition. Phys. Life Rev. 11 , 329–364 (2014).
Conway, C. M. & Christiansen, M. H. Sequential learning in non-human primates. Trends Cogn. Sci. 5 , 539–546 (2001).
Ferrigno, S., Cheyette, S. J., Piantadosi, S. T. & Cantlon, J. F. Recursive sequence generation in monkeys, children, US adults, and native Amazonians. Sci. Adv. 6 , eaaz1002 (2020).
Liao, D. A., Brecht, K. F., Johnston, M. & Nieder, A. Recursive sequence generation in crows. Sci. Adv. 8 , eabq3356 (2022).
Corballis, M. C. Language evolution: a changing perspective. Trends Cogn. Sci. 21 , 229–236 (2017).
Kaminski, J., Call, J. & Fischer, J. Word learning in a domestic dog: evidence for “fast mapping”. Science 304 , 1682–1683 (2004).
Orlov, T., Yakovlev, V., Hochstein, S. & Zohary, E. Macaque monkeys categorize images by their ordinal number. Nature 404 , 77–80 (2000).
Savage-Rumbaugh, E. S., Shanker, S. & Taylor, T. J. Apes, Language, and the Human Mind (Oxford Univ. Press, 1998).
Savage-Rumbaugh, E. S. et al. Language comprehension in ape and child. Monogr. Soc. Res. Child . 58 , 1-222i-252 (1993).
Terrace, H. S., Son, L. K. & Brannon, E. M. Serial expertise of rhesus macaques. Psychol. Sci. 14 , 66–73 (2003).
Chemla, E., Dautriche, I., Buccola, B. & Fagot, J. Constraints on the lexicons of human languages have cognitive roots present in baboons ( Papio papio ). Proc. Natl Acad. Sci. USA 116 , 14926–14930 (2019).
Dautriche, I., Buccola, B., Berthet, M., Fagot, J. & Chemla, E. Evidence for compositionality in baboons ( Papio papio ) through the test case of negation. Sci. Rep. 12 , 1–12 (2022).
Newport, E. L. Maturational constraints on language learning. Cogn. Sci. 14 , 11–28 (1990).
Terrace, H. S. Why Chimpanzees Can’t Learn Language and only Humans Can (Columbia Univ. Press, 2019).
Hurford, J. R. Human uniqueness, learned symbols and recursive thought. Eur. Rev. 12 , 551–565 (2004).
Culicover, P. W. & Jackendoff, R. Simpler Syntax (Oxford Univ. Press, 2005).
Goldberg, A. E. Construction Grammar: A Construction Grammar Approach to Argument Structure (Univ. of Chicago Press, 1995).
Mollica, F. & Piantadosi, S. T. Humans store about 1.5 megabytes of information during language acquisition. R. Soc. Open Sci. 6 , 181393 (2019).
Spelke, E. S. in Language in Mind: Advances in the Study of Language and Thought (eds. Gentner, D. & Goldin-Meadow, S.) 277–311 (MIT Press, 2003).
Premack, D. Human and animal cognition: continuity and discontinuity. Proc. Natl Acad. Sci. USA 104 , 13861–13867 (2007).
Collins, A. M. & Quillian, M. R. Retrieval time from semantic memory. J. Verb. Learn Verb. Behav. 8 , 240–247 (1969).
Isbilen, E. S. & Christiansen, M. H. Chunk‐based memory constraints on the cultural evolution of language. Top Cogn. Sci. 12 , 713–726 (2020).
Levinson, S. C. Space in Language and Cognition: Explorations in Cognitive Diversity Vol. 5 (Cambridge Univ. Press, 2003).
Bitterman, M. E. The comparative analysis of learning: are the laws of learning the same in all animals? Science 188 , 699–709 (1975).
Bryer, M. A. et al. The evolution of quantitative sensitivity. Philos. Trans. R. Soc. Lond B Biol. Sci. 377 , 20200529 (2022).
Harlow, H. F. The formation of learning sets. Psychol. Rev. 56 , 51–65 (1949).
Rumbaugh, D. M. & Pate, J. L. in Behavioral Evolution and Integrative Levels: The T. C. Schneirla Conferences Series (eds Greenboch, G. & Tobach, E.) 221–241 (Psychology Press, 2014).
Byrne, R. W. Primate cognition: comparing problems and skills. Am. J. Primatol. 37 , 127–141 (1995).
MacLean, E. L. et al. The evolution of self-control. Proc. Natl Acad. Sci. USA , 111 , E2140–E2148 (2014).
Shettleworth, S. J. Modularity, comparative cognition and human uniqueness. Philos. Trans. R. Soc. Lond. B, Biol. Sci. 367 , 2794–2802 (2012).
Warren, J. M. Reversal learning and the formation of learning sets by cats and rhesus monkeys. J. Comp. Physiol. Pscyh. 61 , 421 (1966).
Strong, P. N. & Hedges, M. Comparative studies in simple oddity learning: I. Cats, raccoons, monkeys, and chimpanzees. Psychon. Sci. 5 , 13–14 (1966).
Strong, P. N. Comparative studies in oddity learning: III. Apparatus transfer in chimpanzees and children. Psychon. Sci. 7 , 43–43 (1967).
Overman, W., Bachevalier, J., Miller, M. & Moore, K. Children’s performance on “animal tests” of oddity: implications for cognitive processes required for tests of oddity and delayed nonmatch to sample. J. Exp. Child Psychol. 62 , 223–242 (1996).
Shultz, S. & Dunbar, R. I. M. Species differences in executive function correlate with hippocampus volume and neocortex ratio across nonhuman primates. J Comp. Psychol. 124 , 252 (2010).
Miles, R. C. Discrimination-learning sets. Behav. Nonhuman Primates 1 , 51–95 (1965).
Fobes, J. L. & King, J. E. in Primate Behavior (eds. Fobes, J. L. & King, J. E) 327–360 (Academic Press, 1982).
Pope, S. M. et al. Optional-switch cognitive flexibility in primates: chimpanzees’ ( Pan troglodytes ) intermediate susceptibility to cognitive set. J. Comp. Psychol. 134 , 98 (2020).
Roberts, A. C., Robbins, T. W. & Everitt, B. J. The effects of intradimensional and extradimensional shifts on visual discrimination learning in humans and non-human primates. Q. J. Exp. Psychol. B 40 , 321–341 (1988).
PubMed Google Scholar
Gossette, R. L. & Slonim, P. Spatial SDR performances across three species of New World monkeys. Psychon. Sci. 14 , 222–223 (1969).
Rumbaugh, D. M. in Primate Behavior: Developments in Field and Laboratory Research Vol. 1 (ed. Rosenblum, L. A.) 1–70 (Academic Press, 1970).
Landauer, T. K. How much do people remember? Some estimates of the quantity of learned information in long‐term memory. Cogn. Sci. 10 , 477–493 (1986).
De Beni, R. & Cornoldi, C. Effects of the mnemotechnique of loci in the memorization of concrete words. Acta Psychologica 60 , 11–24 (1985).
Legge, E. L., Madan, C. R., Ng, E. T. & Caplan, J. B. Building a memory palace in minutes: equivalent memory performance using virtual versus conventional environments with the method of loci. Acta Psychol. 141 , 380–390 (2012).
Brady, T. F., Konkle, T., Alvarez, G. A. & Oliva, A. Visual long-term memory has a massive storage capacity for object details. Proc. Natl Acad. Sci. USA 105 , 14325–14329 (2008).
Fagot, J. & Cook, R. G. Evidence for large long-term memory capacities in baboons and pigeons and its implications for learning and the evolution of cognition. Proc. Natl Acad. Sci. USA 103 , 17564–17567 (2006).
Gibson, B., Wasserman, E. & Luck, S. J. Qualitative similarities in the visual short-term memory of pigeons and people. Psychon. Bull. Rev. 18 , 979–984 (2011).
Diamond, A. Developmental time course in human infants and infant monkeys, and the neural bases of higher cognitive functions. Ann. N. Y. Acad. Sci. 608 , 637–676 (1990).
Hauser, M. D. Perseveration, inhibition and the prefrontal cortex: a new look. Curr. Opin. Neurobiol. 9 , 214–222 (1999).
Washburn, D. A. Stroop-like effects for monkeys and humans: processing speed or strength of association? Psychol. Sci. 5 , 375–379 (1994).
Richland, L. E. & Burchinal, M. R. Early executive function predicts reasoning development. Psychol. Sci. 24 , 87–92 (2013).
Brady, R. J. & Hampton, R. R. Nonverbal working memory for novel images in rhesus monkeys. Curr. Biol. 28 , 3903–3910 (2018).
Catchpole, C. K. & Slater, P. J. Bird Song: Biological Themes and Variations (Cambridge Univ. Press, 2003).
Terrace, H. S. The phylogeny and ontogeny of serial memory: list learning by pigeons and monkeys. Psychol. Sci. 4 , 162–169 (1993).
Ghirlanda, S., Lind, J. & Enquist, M. Memory for stimulus sequences: a divide between humans and other animals? R. Soc. Open Sci. 4 , 161011 (2017).
Izumi, A. Effect of temporal separation on tone-sequence discrimination in monkeys. Hear. Res. 175 , 75–81 (2003).
Fagot, J. & De Lillo, C. A comparative study of working memory: immediate serial spatial recall in baboons ( Papio papio ) and humans. Neuropsychologia 49 , 3870–3880 (2011).
Malassis, R., Dehaene, S. & Fagot, J. Baboons ( Papio papio ) process a context-free but not a context-sensitive grammar. Sci. Rep. 10 , 1–12 (2020).
Devkar, D. T., Wright, A. A. & Ma, W. J. The same type of visual working memory limitations in humans and monkeys. J. Vis. 15 , 13–13 (2015).
Lind, J., Enquist, M. & Ghirlanda, S. Animal memory: a review of delayed matching-to-sample data. Behav. Process. 117 , 52–58 (2015).
Wright, A. A., Santiago, H. C., Sands, S. R., Kendrick, D. R. & Cook, R. G. Memory processing of serial lists by pigeons, monkeys, and people. Science 229 , 287–289 (1985).
Inoue, S. & Matsuzawa, T. Working memory of numerals in chimpanzees. Curr. Biol. 17 , R1004–R1005 (2007).
Washburn, D. A., Gulledge, J. P., James, F. & Rumbaugh, D. M. A species difference in visuospatial working memory: does language link “what” with “where”? Int. J. Comp. Psychol . 20 https://doi.org/10.46867/ijcp.2007.20.01.02 (2007).
Greenfield, P. M. Language, tools, and brain revisited. Behav. Brain Sci. 21 , 159–163 (1998).
Spelke, E. S. & Kinzler, K. D. Core knowledge. Dev. Sci. 10 , 89–96 (2007).
Aslin, R. N., Saffran, J. R. & Newport, E. L. in The Emergence of Language (ed. MacWhinney, B.) 359–380 (Psychology Press, 1999).
Aslin, R. N., Saffran, J. R. & Newport, E. L. Computation of conditional probability statistics by 8-month-old infants. Psychol. Sci. 9 , 321–324 (1998).
Saffran, J. R., Aslin, R. N. & Newport, E. L. Statistical learning by 8-month-old infants. Science 274 , 1926–1928 (1996).
Terrace, H. S. The simultaneous chain: a new approach to serial learning. Trends Cogn. Sci. 9 , 202–210 (2005).
Cantlon, J. F. & Brannon, E. M. Shared system for ordering small and large numbers in monkeys and humans. Psychol. Sci. 17 , 401–406 (2006).
Vallortigara, G. Core knowledge of object, number, and geometry: a comparative and neural approach. Cogn. Neuropsychol. 29 , 213–236 (2012).
Ferrigno, S., Hughes, K. D. & Cantlon, J. F. Precocious quantitative cognition in monkeys. Psychon. Bull. Rev. 23 , 141–147 (2016).
Smith, J. D. et al. Implicit and explicit categorization: a tale of four species. Neurosci. Biobehav. Rev. 36 , 2355–2369 (2012).
Tomasello, M., Hare, B. & Fogleman, T. The ontogeny of gaze following in chimpanzees, Pan troglodytes , and rhesus macaques, Macaca mulatta . Anim. Behav. 61 , 335–343 (2001).
Kuhl, P. K. & Miller, J. D. Speech perception by the chinchilla: identification functions for synthetic VOT stimuli. J. Acoust. Soc. Am. 63 , 905–917 (1978).
Piaget, J. & Inhelder, B. The Psychology of the Child (Basic Books, 1969).
Xu, F. & Kushnir, T. Infants are rational constructivist learners. Curr. Direc. Psychol. Sci. 22 , 28–32 (2013).
Kail, R. Speed of information processing: developmental change and links to intelligence. J. School Psychol. 38 , 51–61 (2000).
Zelazo, P. D. et al. The development of executive function in early childhood. Monogr. Soc. Res. Child Dev . 63 , i-151 (2003).
Frye, D., Zelazo, P. D., Brooks, P. J. & Samuels, M. C. Inference and action in early causal reasoning. Dev. Psychol. 32 , 120 (1996).
Corballis, M. C. Recursion, language, and starlings. Cogn. Sci. 31 , 697–704 (2007).
Rey, A., Perruchet, P. & Fagot, J. Centre-embedded structures are a by-product of associative learning and working memory constraints: evidence from baboons ( Papio Papio ). Cognition 123 , 180–184 (2012).
Le Corre, M. & Carey, S. One, two, three, four, nothing more: how numerals are mapped onto core knowledge of number in the acquisition of the counting principles. Cognition 105 , 395–438 (2007).
Terrace, H. S., Petitto, L. A., Sanders, R. J. & Bever, T. G. Can an ape create a sentence? Science 206 , 891–902 (1979).
Pepperberg, I. M. Animal language studies: what happened? Psychon. Bull. Rev. 24 , 181–185 (2017).
Rice, M. L. et al. Mean length of utterance levels in 6-month intervals for children 3 to 9 years with and without language impairments. J. Speech Lang. Hear. Res. 53 , 333–349 (2010).
Lillard, A. Developing a cultural theory of mind: the CIAO approach. Curr. Dir. Psychol. Sci. 8 , 57–61 (1999).
Sabbagh, M. A., Xu, F., Carlson, S. M., Moses, L. J. & Lee, K. The development of executive functioning and theory of mind: a comparison of Chinese and US preschoolers. Psychol. Sci. 17 , 74–81 (2006).
Devine, R. T. & Hughes, C. Relations between false belief understanding and executive function in early childhood: a meta-analysis. Child Dev. 85 , 1777–1794 (2014).
Andrews, G., Halford, G. S., Bunch, K. M., Bowden, D. & Jones, T. Theory of mind and relational complexity. Child Dev. 74 , 1476–1499 (2003).
Stearns, R. E., Hartmanis, J. & Lewis, P. M. in 6th Annu. Symp. Switching Circuit Theory and Logical Design 179–190 (IEEE, 1965).
Hopcroft, J. E. & Ullman, J. D. Some results on tape-bounded Turing machines. JACM 16 , 168–177 (1969).
Chomsky, N. Syntactic Structures (Mouton, 1957).
Gentner, T. Q., Fenn, K. M., Margoliash, D. & Nusbaum, H. C. Recursive syntactic pattern learning by songbirds. Nature 440 , 1204–1207 (2006).
Fitch, W. T. & Hauser, M. D. Computational constraints on syntactic processing in a nonhuman primate. Science 303 , 377–380 (2004).
Perruchet, P. & Rey, A. Does the mastery of center-embedded linguistic structures distinguish humans from nonhuman primates? Psychon. Bull. Rev. 12 , 307–313 (2005).
Dedhe, A. M., Piantadosi, S. T. & Cantlon, J. F. Cognitive mechanisms underlying recursive pattern processing in human adults. Cogn. Sci. 47 , e13273 (2023).
Reich, P. A. The finiteness of natural language. Language 45 , 831–843 (1969).
Pullum, G. K. & Gazdar, G. Natural languages and context-free languages. Linguist. Philos. 4 , 471–504 (1982).
Jäger, G. & Rogers, J. Formal language theory: refining the Chomsky hierarchy. Philos. Trans. R. Soc. Lond. B, Biol. Sci. 367 , 1956–1970 (2012).
Higginbotham, J. in The Formal Complexity of Natural Language (ed. Savitch, W. J.) 335–348 (Springer Netherlands, 1984).
Shieber, S. M. in The Formal Complexity of Natural Language (ed. Savitch, W. J.) 320–334 (Springer Netherlands, 1985).
Savitch, W. J., Bach, E., Marsh, W. E. & Safran-Naveh, G. (eds) The Formal Complexity of Natural Language Vol. 33 (Springer Science & Business Media, 2012).
Papadimitriou, C. H. Computational Complexity (Pearson, 1993).
Bommasani, R. et al. On the opportunities and risks of foundation models. Preprint at arXiv https://doi.org/10.48550/arXiv.2108.07258 (2021).
Srivastava, A. et al. Beyond the imitation game: quantifying and extrapolating the capabilities of language models. Preprint at arXiv https://doi.org/10.48550/arXiv.2206.04615 (2022).
Ganguli, D. et al. Predictability and surprise in large generative models. In ACM Conf. on Fairness, Accountability, and Transparency 1747–1764 (ACM, 2022).
Kaplan, J. et al. Scaling laws for neural language models. Preprint at arXiv https://doi.org/10.48550/arXiv.2001.08361 (2020).
Zhang, C., Bengio, S., Hardt, M., Recht, B. & Vinyals, O. Understanding deep learning requires rethinking generalization. Preprint at arXiv https://doi.org/10.48550/arXiv.1611.03530 (2017).
Zhang, C., Bengio, S., Hardt, M., Recht, B. & Vinyals, O. Understanding deep learning (still) requires rethinking generalization. Commun. ACM 64 , 107–115 (2021).
Bubeck, S. et al. Sparks of artificial general intelligence: early experiments with gpt-4. Preprint at arXiv https://doi.org/10.48550/arXiv.2303.12712 (2023).
Mahowald, K. et al. Dissociating language and thought in large language models: a cognitive perspective. Preprint at arXiv https://doi.org/10.48550/arXiv.2301.06627 (2023).
Sutton, H. The bitter lesson. Incomplete Ideas http://www.incompleteideas.net/IncIdeas/BitterLesson.html (2019).
Laland, K. N., Kendal, J. R. & Brown, G. R. The niche construction perspective: implications for evolution and human behaviour. J. Evol. Psychol. 5 , 51–66 (2007).
Clarke, E. & Heyes, C. The swashbuckling anthropologist: Henrich on the secret of our success. Biol. Philos. 32 , 289–305 (2017).
Lefebvre, L., Reader, S. M. & Sol, D. Brains, innovations and evolution in birds and primates. Brain Behav. Evol. 63 , 233 (2004).
Ong, W. J. & Hartley, J. Orality and Literacy (Routledge, 2013).
Tinbergen, N. On aims and methods of ethology. Z. Tierpsychol. 20 , 410–433 (1963).
Krubitzer, L. In search of a unifying theory of complex brain evolution. Ann. N. Y. Acad. Sci. 1156 , 44–67 (2009).
Preuss, T. M. & Wise, S. P. Evolution of prefrontal cortex. Neuropsychopharmacology 47 , 3–19 (2022).
Smaers, J. B., Gómez-Robles, A., Parks, A. N. & Sherwood, C. C. Exceptional evolutionary expansion of prefrontal cortex in great apes and humans. Curr. Biol. 27 , 714–720 (2017).
Herculano-Houzel, S. The Human Advantage: A New Understanding of How Our Brain Became Remarkable (MIT Press, 2016).
Van Essen, D. C. et al. Cerebral cortical folding, parcellation, and connectivity in humans, nonhuman primates, and mice. Proc. Natl Acad. Sci. USA 116 , 26173–26180 (2019).
Herculano-Houzel, S. Numbers of neurons as biological correlates of cognitive capability. Curr. Opin. Behav. Sci. 16 , 1–7 (2017).
Rakic, P. Pre-and post-developmental neurogenesis in primates. Clin. Neurosci. Res. 2 , 29–39 (2002).
Rakic, P. A small step for the cell, a giant leap for mankind: a hypothesis of neocortical expansion during evolution. Trends Neurosci. 18 , 383–388 (1995).
Charvet, C. J. & Finlay, B. L. Evo-devo and the primate isocortex: the central organizing role of intrinsic gradients of neurogenesis. Brain Behav. Evol. 84 , 81–92 (2014).
Clancy, B., Darlington, R. B. & Finlay, B. L. Translating developmental time across mammalian species. NeuroScience 105 , 7–17 (2001).
Takahashi, T., Nowakowski, R. S. & Caviness, V. S. Jr The mathematics of neocortical neuronogenesis. Dev. Neurosci. 19 , 17–22 (1997).
Zembrzycki, A. et al. Genetic mechanisms control the linear scaling between related cortical primary and higher order sensory areas. eLife 4 , e11416 (2015).
Marchetto, M. C. et al. Species specific maturation profiles of human, chimpanzee and bonobo neural cells. eLife 8 , e37527 (2019).
Cahalane, D. J., Charvet, C. J. & Finlay, B. L. Modeling local and cross-species neuron number variations in the cerebral cortex as arising from a common mechanism. Proc. Natl Acad. Sci. USA 111 , 17642–17647 (2014).
Nieder, A. Inside the corvid brain—probing the physiology of cognition in crows. Curr. Opin. Behav. Sci. 16 , 8–14 (2017).
Güntürkün, O., Ströckens, F., Scarf, D. & Colombo, M. Apes, feathered apes, and pigeons: differences and similarities. Curr. Opin. Behav. Sci. 16 , 35–40 (2017).
Güntürkün, O., von Eugen, K., Packheiser, J. & Pusch, R. Avian pallial circuits and cognition: a comparison to mammals. Curr. Opin. Neurobiol. 71 , 29–36 (2021).
Cowan, N. The magical mystery four: how is working memory capacity limited, and why? Curr. Dir. Psychol. Sci. 19 , 51–57 (2010).
Waltz, J. A., Lau, A., Grewal, S. K. & Holyoak, K. J. The role of working memory in analogical mapping. Mem. Cogn. 28 , 1205–1212 (2000).
Krems, J. A. & Wilkes, J. Why are conversations limited to about four people? A theoretical exploration of the conversation size constraint. Evol. Hum. Behav. 40 , 140–147 (2019).
Chater, N. & Oaksford, M. Ten years of the rational analysis of cognition. Trends Cogn. Sci. 3 , 57–65 (1999).
Geisler, W. S. Ideal observer analysis. Vis. Neurosci. 10 , 12 (2003).
Sims, C. R. Rate–distortion theory and human perception. Cognition 152 , 181–198 (2016).
Gershman, S. J. Origin of perseveration in the trade-off between reward and complexity. Cognition 204 , 104394 (2020).
Bhui, R., Lai, L. & Gershman, S. J. Resource-rational decision making. Curr. Opin. Behav. Sci. 41 , 15–21 (2021).
Polanía, R., Woodford, M. & Ruff, C. C. Efficient coding of subjective value. Nat. Neurosci. 22 , 134–142 (2019).
Sims, C. R. Efficient coding explains the universal law of generalization in human perception. Science 360 , 652–656 (2018).
Hahn, M., Futrell, R., Levy, R. & Gibson, E. A resource-rational model of human processing of recursive linguistic structure. Proc. Natl Acad. Sci. USA 119 , e2122602119 (2022).
Cheyette, S. J. & Piantadosi, S. T. A unified account of numerosity perception. Nat. Hum. Behav. 4 , 1265–1272 (2020).
Yang, Y. & Piantadosi, S. T. One model for the learning of language. Proc. Natl Acad. Sci. USA 119 , e2021865119 (2022).
Planton, S. et al. A theory of memory for binary sequences: evidence for a mental compression algorithm in humans. PLoS Comput. Biol. 17 , e1008598 (2021).
Grünwald, P. D. The Minimum Description Length Principle (MIT Press, 2007).
Hutter, M. Universal Artificial Intelligence: Sequential Decisions Based on Algorithmic Probability (Springer Science & Business Media, 2004).
Solomonoff, R. J. A formal theory of inductive inference. Part I. Inform. Control. 7 , 1–22 (1964).
Piantadosi, S. T., Tenenbaum, J. B., & Goodman, N. D. Bootstrapping in a language of thought: a formal model of numerical concept learning. Cognition 123 , 199–217 (2012).
Hick, W. E. On the rate of gain of information. Q. J. Exp. Psychol. 4 , 11–26 (1952).
Miller, G. Human memory and the storage of information. IRE Trans. Inform. Theor 2 , 129–137 (1956).
Posner, M. I. & Rossman, E. Effect of size and location of informational transforms upon short-term retention. J. Exp. Psychol. 70 , 496 (1965).
Shannon, C. E. Prediction and entropy of printed English. Bell Syst. Tech. J. 30 , 50–64 (1951).
Reber, A. S. Transfer of syntactic structure in synthetic languages. J. Exp. Psychol. 81 , 115 (1969).
Macmillan, N. A. & Creelman, C. D. Detection Theory: A User’s Guide (Psychology Press, 2004).
Chittka, L., Skorupski, P. & Raine, N. E. Speed–accuracy tradeoffs in animal decision making. Trends Ecol. Evol. 24 , 400–407 (2009).
Baddeley, A. The magical number seven: still magic after all these years? Psychol. Rev. 101 , 353–356 (1994).
Download references
Acknowledgements
The authors thank H. Clatterbuck for substantive comments and conceptual feedback on this manuscript, and C. Kaicher for help with scholarship, editing and formatting the article. This work was supported by the National Institute of Health (NIH) (grant number R01HD107840) and National Science Foundation (NSF) (grant numbers DRL2201843 and DRL2026416).
Author information
Authors and affiliations.
Department of Psychology, Carnegie Mellon University, Pittsburgh, PA, USA
Jessica F. Cantlon
Neuroscience Institute, Carnegie Mellon University, Pittsburgh, PA, USA
Department of Psychology, University of California, Berkeley, CA, USA
- Steven T. Piantadosi
Helen Wills Neuroscience Institute, University of California, Berkeley, CA, USA
You can also search for this author in PubMed Google Scholar
Contributions
The authors contributed equally to all aspects of the article.
Corresponding authors
Correspondence to Jessica F. Cantlon or Steven T. Piantadosi .
Ethics declarations
Competing interests.
The authors declare no competing interests.
Peer review
Peer review information.
Nature Reviews Psychology thanks Stella Christie and the other, anonymous, reviewer(s) for their contribution to the peer review of this work.
Additional information
Publisher’s note Springer Nature remains neutral with regard to jurisdictional claims in published maps and institutional affiliations.
Rights and permissions
Springer Nature or its licensor (e.g. a society or other partner) holds exclusive rights to this article under a publishing agreement with the author(s) or other rightsholder(s); author self-archiving of the accepted manuscript version of this article is solely governed by the terms of such publishing agreement and applicable law.
Reprints and permissions
About this article
Cite this article.
Cantlon, J.F., Piantadosi, S.T. Uniquely human intelligence arose from expanded information capacity. Nat Rev Psychol 3 , 275–293 (2024). https://doi.org/10.1038/s44159-024-00283-3
Download citation
Accepted : 23 January 2024
Published : 02 April 2024
Issue Date : April 2024
DOI : https://doi.org/10.1038/s44159-024-00283-3
Share this article
Anyone you share the following link with will be able to read this content:
Sorry, a shareable link is not currently available for this article.
Provided by the Springer Nature SharedIt content-sharing initiative
This article is cited by
Language is primarily a tool for communication rather than thought.
- Evelina Fedorenko
- Edward A. F. Gibson
Nature (2024)
Quick links
- Explore articles by subject
- Guide to authors
- Editorial policies
Sign up for the Nature Briefing newsletter — what matters in science, free to your inbox daily.


An official website of the United States government
The .gov means it’s official. Federal government websites often end in .gov or .mil. Before sharing sensitive information, make sure you’re on a federal government site.
The site is secure. The https:// ensures that you are connecting to the official website and that any information you provide is encrypted and transmitted securely.
- Publications
- Account settings
Preview improvements coming to the PMC website in October 2024. Learn More or Try it out now .
- Advanced Search
- Journal List
- Europe PMC Author Manuscripts
Genome-wide association studies establish that human intelligence is highly heritable and polygenic
Gail davies.
1 Department of Psychology, The University of Edinburgh, 7 George Square, Edinburgh EH8 9JZ, UK
Albert Tenesa
2 Colon Cancer Genetics Group, MRC Human Genetics Unit, Western General Hospital, The University of Edinburgh, Edinburgh, UK
3 The Roslin Institute, Royal (Dick) School of Veterinary Studies, The University of Edinburgh, Roslin, UK
Antony Payton
4 Centre for Integrated Genomic Medical Research, University of Manchester, Stopford Building, Oxford Road, Manchester M13 9PT
5 Queensland Institute of Medical Research, 300 Herston Road, Brisbane, Queensland 4006, Australia
Sarah E. Harris
6 Medical Genetics Section, The University of Edinburgh Molecular Medicine Centre, Institute of Genetics and Molecular Medicine, Western General Hospital Edinburgh, UK
7 Centre for Cognitive Ageing and Cognitive Epidemiology, The University of Edinburgh, 7 George Square, Edinburgh EH8 9JZ, UK
David Liewald
8 Institute of Child Health, University College London, 30 Guilford Street, London WC1N 1EH
Stephanie Le Hellard
9 Dr Einar Martens Research Group for Biological Psychiatry, Department of Clinical Medicine, University of Bergen, Bergen, Norway
Andrea Christoforou
Michelle luciano, kevin mcghee, lorna lopez, alan j. gow, janie corley, paul redmond, helen c. fox.
10 Institute of Applied Health Sciences, University of Aberdeen, Foresterhill, Aberdeen AB25 2ZD
Paul Haggarty
11 Nutrition and Epigenetics Group, Rowett Institute of Nutrition and Health, University of Aberdeen, Greenburn Road, Bucksburn, Aberdeen AB21 9SB
Lawrence J. Whalley
Geraldine mcneill, michael e. goddard.
12 Department of Food and Agricultural Systems, University of Melbourne, Parkville 3011, Australia and Victorian Department of Primary Industries, Bundoora, Australia
Thomas Espeseth
13 Center for the Study of Human Cognition, Department of Psychology, University of Oslo, Box 1094, Blindern, N-0317 Oslo, Norway
Astri J. Lundervold
14 Department of Biological and Medical Psychology, University of Bergen, Bergen, Norway
Ivar Reinvang
Andrew pickles.
15 School of Epidemiology and Health Science, Department of Medicine, University of Manchester, Manchester M13 9PT, UK

Vidar M. Steen
16 Center for Medical Genetics and Molecular Medicine, Haukeland University Hospital, Bergen, Norway.
William Ollier
David j. porteous, michael horan.
17 School of Community-Based Medicine, Neurodegeneration Research Group, University of Manchester, Clinical sciences Building, Salford Royal NHS Foundation Trust, Salford M6 8HD
John M. Starr
18 Geriatric Medicine Unit, The University of Edinburgh, Royal Victoria Hospital, Edinburgh EH4 2DN
Neil Pendleton
Peter m. visscher, ian j. deary, associated data.
General intelligence is an important human quantitative trait that accounts for much of the variation in diverse cognitive abilities. Individual differences in intelligence are strongly associated with many important life outcomes, including educational and occupational attainments, income, health and lifespan 1 , 2 . Data from twin and family studies are consistent with a high heritability of intelligence 3 , but this inference has been controversial. We conducted a genome-wide analysis of 3511 unrelated adults with data on 549 692 SNPs and detailed phenotypes on cognitive traits. We estimate that 40% of the variation in crystallized-type intelligence and 51% of the variation in fluid-type intelligence between individuals is accounted for by linkage disequilibrium between genotyped common SNP markers and unknown causal variants. These estimates provide lower bounds for the narrow-sense heritability of the traits. We partitioned genetic variation on individual chromosomes and found that, on average, longer chromosomes explain more variation. Finally, using just SNP data we predicted approximately 1% of the variance of crystallized and fluid cognitive phenotypes in an independent sample ( P = 0.009 and 0.028, respectively). Our results unequivocally confirm that a substantial proportion of individual differences in human intelligence is due to genetic variation, and are consistent with many genes of small effects underlying the additive genetic influences on intelligence.
Introduction
People differ in their cognitive abilities, and the origins and impacts of these differences are sought after and much debated. The quantitative trait of general intelligence reflects the fact that diverse cognitive abilities show universally positive covariation; that is, no matter the cognitive task being undertaken, much of the human variation in any cognitive task will be caused by people’s differences in general intelligence 4 . The latent trait of general intelligence ranks people very similarly, irrespective of the group of cognitive tests used to extract it 5 . Human intelligence is highly stable through the lifecourse and the resemblance between relatives suggests it is highly heritable throughout adulthood 3 . Intelligence is strongly associated with many important outcomes in life. People with higher intelligence tend to have more education, more professional occupations, higher incomes, and longer lives 1 , 2 . The foundations of intelligence differences in brain structure and function are becoming increasingly clear 6 - 8 . Especially in later adulthood, an important distinction is made between general fluid and crystallized intelligences, with the former showing earlier and more rapid age-related decline 9 , 10 .
Intelligence is highly familial, yet the extent and nature of the genetic contribution to intelligence differences has been controversial 11 . Twin and adoption studies suggest that additive genetic effects contribute over half of the population variance in intelligence in adulthood 3 , 6 . However, no single genes or gene variants have been identified that are robustly associated with intelligence-related phenotypes 3 . Moreover, it has been suggested that the apparent high heritability for intelligence is the result of a correlation (confounding) between genetic and environmental factors and that breaking up this correlation would result in the trait being much less heritable 12 , 13 .
We present here the results of a genome-wide association study (GWAS) which examines cognitive ability phenotype-genotype associations in the five cohorts which constitute the CAGES (Cognitive Ageing Genetics in England and Scotland) project: the Lothian Birth Cohorts of 1921 and 1936 (LBC1921, LBC1936), the Aberdeen Birth Cohort 1936 (ABC1936), and the Manchester and Newcastle Longitudinal Studies of Cognitive Aging ( Supplementary Table 1 ). All five cohorts comprise non-clinical samples of relatively healthy people from middle to older adulthood with detailed, though not identical, cognitive phenotypes.
Materials and Methods
Participants: discovery cohorts.
The five cohorts within the CAGES project are the Lothian Birth Cohorts of 1921 14 , 15 and 1936 16 (LBC1921, LBC1936), the Aberdeen Birth Cohort 1936 (ABC1936) 14 , 15 , and the Manchester and Newcastle Longitudinal Studies of Cognitive Aging cohorts 17 ( Supplementary Table 1 ). Together these cohorts comprise 3511 healthy individuals with detailed cognitive abilities measured in middle to older adulthood.
Lothian Birth Cohort 1921
The LBC1921 is a longitudinal study of cognitive ageing. All participants were born in 1921 and completed the Moray House Test (MHT) No. 12 assessment of general intelligence in the Scottish Mental Survey 1932 at a mean age of 11 years 14 , 18 . Their recruitment and re-testing in old age has been described previously 14 , 15 . Relatively healthy surviving participants of the Scottish Mental Survey 1932 were identified within Edinburgh and its surrounding area, the Lothians. A total of 550 individuals (234 men and 316 women) were recruited and tested at a mean age of 79.1 years (SD = 0.6) 15 . The LBC1921 participants were tested individually and completed a battery of cognitive tests: The Moray House Test No. 12 (MHT) 18 , Raven’s Standard Progressive Matrices 19 , Verbal Fluency 20 , and Logical Memory 21 . Participants also completed the National Adult Reading Test (NART) 22 . Following informed consent, venesected whole blood was collected for DNA extraction. Ethical approval for all projects was obtained from the Lothian Research Ethics Committee.
Lothian Birth Cohort 1936
The Lothian Birth Cohort 1936 (LBC1936) is a longitudinal study of cognitive ageing. All participants were born in 1936 and had completed the MHT in the Scottish Mental Survey 1947 at a mean age of 11 years 14 , 23 . Their recruitment and re-testing in old age has been described previously 16 . Relatively healthy surviving participants of the Scottish Mental Survey 1947 were identified within Edinburgh and its surrounding area, the Lothians. A total of 1091 participants (548 men and 543 women) were recruited and tested individually at a mean age of 69.5 years (SD = 0.8). The LBC1936 participants completed a large battery of cognitive tests 16 . For the purposes of the present study, only those tests relevant to the phenotypes analysed here are described. These are: the MHT, and a battery of cognitive tests consisting of 6 tests from the Wechsler Adult Intelligence Scale-III UK (WAIS-III UK [Ref. 24 ]): Digit Symbol Coding, Block Design, Matrix Reasoning, Digit Span Backwards, Symbol Search, and Letter-number Sequencing. Participants also completed the NART 22 . Following informed consent, venesected whole blood was collected for DNA extraction. Ethical approval for all projects was obtained from Scotland’s Multicentre Research Ethics Committee and the Lothian Research Ethics Committee.
Aberdeen Birth Cohort 1936
The Aberdeen Birth Cohort 1936 (ABC1936) is a longitudinal study of cognitive ageing. All participants were born in 1936 and had completed a version of the MHT test of general intelligence in the Scottish Mental Survey 1947 at mean age 11 years 14 , 23 . Their recruitment and re-testing in old age has been described previously 14 , 15 . A total of 498 relatively healthy participants (243 men, 255 women) was traced and tested at mean age 64.6 years (SD = 0.9). The cognitive tests carried out were: the NART 22 , Raven’s Standard Progressive Matrices 19 , the Rey Auditory Verbal Learning Test 20 , Digit Symbol and Block Design sub-tests of the Wechsler Adult Intelligence Scale-Revised 25 , and the Uses of Common Objects Test 22 . Following informed consent, venesected whole blood was collected for DNA extraction. Ethical approval for all projects was obtained from the Grampian Research Ethics Committee.
Manchester and Newcastle Longitudinal Studies of Cognitive Ageing Cohorts
The University of Manchester Age and Cognitive Performance Research Centre (ACPRC) programme began in 1983 and this study has documented longitudinal trajectories in cognitive function in a large sample of older adults in the North of England, UK 17 . Recruitment took place in Newcastle and Greater Manchester between 1983 and 1992. At the outset of the study, 6063 volunteers were available, (1825 men, 4238 women), with a median age of 65 years (range 44 to 93 years). Over the period 1983 to 2003, two alternating batteries of cognitive tasks applied biennially were designed to measure fluid and crystallized aspects of intelligence. These included: the Alice Heim 4 (AH4) parts 1 and 2 tests of general intelligence; Mill Hill Vocabulary A and B vocabulary tests; the Cattell and Cattell Culture Fair intelligence tests; and the Wechsler Adult Intelligence Scale Vocabulary test. Detailed task descriptions were provided previously 17 . Following informed consent, venesected whole blood was collected for DNA extraction. Ethical approval for all projects was obtained from the University of Manchester.
Participants: replication cohort
The Norwegian Cognitive NeuroGenetics cohort (NCNG) consists of 670 participants, ranging from 18 to 79 years of age (mean = 47.6; SD = 18.3) ( Supplementary Table 1 ). The participants completed a battery of psychometric tests, assessing general cognition, memory, attention, and speed of processing faculties. Informed consent was approved by the relevant regional ethical committee for medical research (Project ID: S-03116). Permission to obtain and store blood samples for genotyping together with cognitive and MRI data in a bio-bank and to establish a registry with relevant information for a time period of 10 years was granted by the Norwegian Department of Health.
Cognitive phenotypes
For each of the cohorts we constructed cognitive phenotypes of fluid-type and crystallized-type intelligence. Crystallized-type intelligence is typically assessed using tests of acquired knowledge, and most often through tests of vocabulary. Fluid-type intelligence tends to involve unfamiliar, sometimes abstract, materials, to involve on-the-spot thinking, to be completed under time pressure, and to rely relatively little on prior knowledge. Here, to represent crystallized intelligence ( g c ), we used: the National Adult Reading Test in the Lothian Birth Cohorts of 1921 and 1936, and the Aberdeen Birth Cohort 1936; the Mill Hill Vocabulary Test in the Manchester and Newcastle samples; and the Wechsler Adult Intelligence Scale Vocabulary subtest in the Norwegian Cognitive NeuroGenetics sample. For fluid-type intelligence, principal components analyses (PCA) were used in the following cohorts to derive a general intelligence factor ( g f ): the Lothian Birth Cohorts 1921 and 1936, the Aberdeen Birth Cohort 1936, and the Norwegian Cognitive NeuroGenetics sample. In each case, the scores on a number of fluid-type cognitive tests were subjected to PCA. The tests used to form the g f factor in the LBC1921 were the Moray House Test, Raven’s Matrices, Logical Memory, and Verbal Fluency. For the LBC1936 the six tests from the WAIS-III UK were used. The ABC1936 g f factor included Raven’s Progressive Matrices, Digit Symbol, Uses of Common Objects, and AVLT. For the NCNG sample, a hierarchy of PCA analyses was used (see Supplementary Methods for further details). In all cases a single component was indicated and was extracted. Thus, individuals’ scores on the first unrotated principal component were used as the indicator of general fluid-type intelligence ( g f ). For g f in the Manchester and Newcastle samples empirical Bayes’s estimates for each individual were obtained from a random effects model fitted by maximum likelihood (ML) to the standardized age-regressed residuals obtained for each sex from the Alice Heim 4 test and the Cattell Culture Fair test scores. All of the phenotypes were corrected for age and sex (with the exception of Manchester and Newcastle g f which was derived separately for males and females) and the standardized residuals were used for all subsequent analyses.
Genotyping and Quality Control
Discovery cohort.
A total of 3511 CAGES participants had DNA extracted and were genotyped for 599 011 common single nucleotide polymorphisms (SNPs) using the Illumina610-Quadv1 chip ( Supplementary Table 1 ). We applied stringent quality control analyses of the genotype data and retained 549 692 of the 599 011 SNPs on the Illumina 610 chip in 3511 individuals (2115 females) ( Supplementary Figure 1 ). Individuals were excluded from this study based on unresolved gender discrepancy, relatedness, call rate (≤ 0.95), and evidence of non-Caucasian descent. SNPs were included in the analyses if they met the following conditions: call rate ≥ 0.98, minor allele frequency ≥ 0.01, and Hardy-Weinberg equilibrium test with P ≥ 0.001. Differences in allele frequencies between the discovery and replication samples were investigated, and no sizeable deviations were observed ( Supplementary Figure 1 ). We tested for population stratification within each cohort and excluded any outliers. The first four components from a multidimensional scaling (MDS) analysis of the SNP data, based on the remaining individuals, were included as covariates in subsequent analyses ( Supplementary Figure 2 ). A total of 3400 and 3482 samples with both phenotypic and SNP data were available for g f and g c , respectively.
Replication cohort
NCNG DNA samples were newly extracted from blood using the Qiagen Gentra Autopure LS system. They were genotyped on the Illumina Human610-Quad Beadchip. A strict quality control protocol was applied which resulted in a final dataset of 554 225 SNPs genotyped in a homogenous Norwegian sample of 670 individuals (See Supplementary Methods for further details).
Statistical Analysis
Genotype-phenotype analyses were performed within each cohort 26 . Meta-analysis was implemented using an inverse variance weighted model ( www.sph.umich.edu/csg/abecasis/Metal ). Gene-based tests for association were carried out using results from the meta-analyses 27 .
Statistical analyses to estimate the proportion of variation explained by all SNPs were performed as in Yang et al . 28 . This method captures the variance in the trait that is due to linkage disequilibrium between genotyped SNPs and unknown causal variants 28 , 30 . The interpretation of the estimate of variance accounted for is different from that estimated from traditional family or twin studies, because these latter designs capture the variation due to all causal variants in the genome and, possibly, variation due to environmental factors, for example when the effect of shared environment is larger in monozygotic twin pairs than in dizygotic pairs 30 . We firstly estimated pairwise genetic relationships between 3511 individuals from CAGES from 549 692 autosomal SNPs. We excluded one individual from any pair that had an estimated coefficient of relatedness of > 0.025 and retained 3291 individuals for analysis: 1800 from Scotland and 1491 from Northern England. The reason for excluding close relatives is that: (i) their phenotypic covariance will have a large impact on the estimate of variance explained; (ii) the phenotypic covariance of close relatives captures the effects of all causal variants in the genome and not just those in close LD with the genotyped SNPs; and (iii) phenotypic covariance of close relatives can include effects due to shared environment 30 . We fitted a linear mixed model y = μ + g + e , where y is phenotype, μ is the mean term, g is the aggregate additive genetic effect of all the SNPs, and e is the residual effect. We have previously demonstrated that this model is mathematically equivalent to the model of fitting all the SNPs 28 ; i.e., y = μ + ∑ x i b i + e , where x i is the number of copies of the reference allele for a SNP i with its additive effect of b i . The covariance structure fitted in the data was the relationship estimated from all SNPs; i.e., cov( y j , y k )= A jk σ g 2 + σ e 2 , where A jk is SNP-derived genetic relationship between individuals j and k, σ g 2 is the additive genetic variance and σ e 2 is the residual variance. We used restricted maximum likelihood (REML) to estimate additive genetic variance. To maintain consistency with the single SNP analyses, four principal components were fitted as covariates in the model. Genome-partitioning of genetic variation was done similarly, by fitting chromosomal relationships estimated from all SNPs on a particular chromosome. The cross-validation prediction analyses were done by including the genotypes of all individuals but setting the phenotype of individuals in the validation cohort to unknown, and then performing a regression of the phenotype on the best linear unbiased predictor 28 . For the Norwegian sample, SNP effects were estimated from the entire CAGES sample by exploiting the mathematical equivalence of a model based upon genome-wide genetic effects and individual SNP effects 29 .
Analyses of individual SNPs and genes did not result in any replicable genome-wide significant association ( Fig. 1a,c , Supplementary Figures 3-6 , and Supplementary Tables 3,4 ). A gene-based test for association 27 showed one genome-wide significant association ( P = 9.2 × 10 −7 ), with formin-binding protein 1-like ( FNBP1L ) on g f ( Supplementary Figure 7 ). This single genome-wide association result for FNBP1L did not replicate in the independent NCNG sample ( P = 0.211, gene-based test).

Manhattan plot showing meta-analysis results for g f . The −log 10 P values (y axis) of 549,692 SNPs in 3,400 individuals are presented based on their choromosomal position (x axis). The red line is the genome-wide significance threshold 5× 10 −8 ( a ). Manhattan plot showing meta-analysis results for g c . The −log 10 P values (y axis) of 549,692 SNPs in 3,482 individuals are presented based on their chromosomal position (x axis). The red line is the genome-wide significance threshold 5× 10 −8 ( b ). Quantile-quantile plots of the meta-analysis P-values for g f . The black circles represent the observed data, the red line is the expectation under the null hypothesis of no association, and the black curves are the boundaries of the 95% confidence interval. A clear deviation from the expected values is evident ( c ). Quantile-quantile plots of the meta-analysis P values for g c . The black circles represent the observed data, the red line is the expectation under the null hypothesis of no association, and the black curves are the boundaries of the 95% confidence interval. A clear deviation from the expected values is evident ( d ).
We observed that the test statistic for association from the meta-analysis, but not the individual cohort analyses, was inflated for both g f and g c ( Figures 1 b,d and Supplementary Figures 4,6 ). Inflated test statistics are indicative of either population stratification or polygenic variation. There was no strong evidence of population stratification within each of the five discovery cohorts ( Supplementary Figure 2 ). Moreover, four multi-dimensional scaling (MDS) components were fitted in each individual cohort analysis to account for the effects of possible subtle population stratification. Therefore, we reasoned that the inflation of the test statistic across the genome was indicative of polygenic variation.
We quantified the proportion of phenotypic variation accounted for by all genotyped SNPs, using an analysis method we recently developed 28 (See Supplementary Method for further details and Supplementary Figure 8 ). This model is mathematically equivalent to fitting all SNPs in the model, provided that the SNP effects are treated as random 28 . Therefore, our estimate of additive genetic variance is that explained from considering all SNPs simultaneously. Because there are many more ungenotyped genetic variants in the genome than there are genotyped SNPs, this is likely to be due to LD between genotyped SNPs and unknown causal variants. Further details on and explanation of this method can be found in a recent detailed commentary on the method provided by Visscher and collegaues 30 . We estimated that a proportion of 0.40 (SE = 0.11, P = 5.7 × 10 −5 , likelihood-ratio test) and 0.51 (SE = 0.11, P = 1.2 × 10 −7 , likelihood-ratio test) of the phenotypic variance can be explained by all SNPs for g c and g f , respectively ( Table 1 ). Analysing the English and Scottish samples separately or fitting 20 principal components as covariates in the model of analysis did not change the results markedly, nor did the inclusion of pairs of individuals whose estimated relatedness was > 0.025 ( Supplementary Table 2 ). We subsequently partitioned additive genetic variation to individual chromosomes using the software package GCTA 31 , fitting all chromosomes simultaneously, and found that, on average, longer chromosomes explain more variation ( Figure 2 ).

The numbers in the circles and squares are the chromosome numbers.
N | 3254 | 3181 |
(se) | 0.40 (0.11) | 0.51 (0.11) |
value | 5.7 × 10 | 1.2 × 10 |
Estimates of the proportion of phenotypic variance explained by all SNPs for the traits g f and g c from the combined CAGES samples. h 2 = proportion of phenotypic variance accounted for by fitting all SNPs
To further corroborate evidence of polygenic variation, we tested whether phenotypes for intelligence could be predicted solely from SNP data 32 , 33 . We performed cross-validation analyses in which four of the five CAGES cohorts were used to estimate SNP effects while the remaining cohort was used to estimate the correlation between the phenotype and the predictor created from all autosomal SNPs ( Table 2 and see Supplementary Method for further detail). For g f , four of the five prediction analyses showed significant ( P < 0.05) results. The correlations for the five analyses fell consistently in a narrow band of values between 0.067 and 0.148 (mean R = 0.11). For g c , three of the five prediction analyses showed significant results, and the correlations for the five analyses ranged between 0.049 and 0.133 (mean R = 0.081). Non-significance of some of the associations in Table 2 should not be taken to mean that there are different results in different cohorts. The standard errors of the estimates of correlation in Table 2 vary from ~0.03 (LBC1936) to ~0.05 (LBC1921), and none is significantly different from the other, either by trait or by validation cohort.
Validation cohort | ||||
---|---|---|---|---|
Lothian Birth Cohort 1921 | 0.098 | 0.014 | 0.133 | 1.3 × 10 |
Lothian Birth Cohort 1936 | 0.094 | 1.5 × 10 | 0.082 | 4.9 × 10 |
Aberdeen Birth Cohort 1936 | 0.067 | 0.11 | 0.049 | 0.16 |
Newcastle | 0.137 | 7.5 × 10 | 0.057 | 0.06 |
Manchester | 0.148 | 1.3 × 10 | 0.086 | 7.5 × 10 |
NCNG | 0.076 | 0.028 | 0.092 | 0.009 |
For the CAGES samples each cohort, in turn, was used as the validation cohort and the predictor was generated from a joint analysis of the four remaining cohorts. A joint analysis of the five UK cohorts was used to create the predictor for the NCNG cohort. R is the correlation coefficient between the observed phenotype and the predicted value for each individual based on genetic information. P indicates the statistical significance (one-sided t-test, since the alternative hypothesis is that the predictor is positively correlated with outcome) of the correlation coefficient R .
We next used the entire set of five CAGES samples to estimate SNP effects and predicted cognitive phenotypes in the independent NCNG (Norwegian NeuroGognitive Genetics) sample. For g f and g c , the correlations between phenotype and predictor were, respectively, 0.076 ( P = 0.028, one-sided t-test) and 0.092 ( P = 0.009, one-sided t-test). Individuals with a higher predicted score had, on average, a higher phenotype. Thus, SNP effects estimated in the discovery cohort are significantly predictive of cognitive phenotype outcomes in a fully independent cohort.
Here we report results from a GWAS of intelligence in middle to older adulthood. Despite the fact that no specific genetic variants have been robustly associated with human intelligence, apart perhaps for APOE at older ages 34 , 35 , our results show for the first time that a substantial proportion (approximately 40 to 50%) of variation in human intelligence is associated with common SNPs (Minor allele frequency (MAF) > 0.01) that are in LD with causal variants. These results are consistent with a highly polygenic model because we detect variation across the entire genome. If the narrow-sense heritability for intelligence is approximately 0.6 in the age groups studied in the CAGES samples 3 , 36 , then not all additive variation is accounted for by our analyses. One reason for this difference could be that causal variants for intelligence have, on average, a lower MAF than the SNPs on the chip used. Such a frequency difference causes imperfect LD between the genotyped SNPs and unobserved causal variants. Traditional pedigree analysis is not affected by such imperfect LD because it is based on the correct expected identity-by-descent coefficients at loci (including loci with causal variants) of relatives. It is also possible that causal variants are present in regions of the genome not well covered by the commercial SNP arrays. Nevertheless, our results suggest that common SNPs that are in LD with unknown causal variants account for more than half of all additive genetic variation for human intelligence. The method we have used here does not attempt to test the effects of single SNPs; rather, it tests their accumulated effects. It estimates the joint effect of genotyped SNPs and that effect reflects their linkage disequilibrium (LD) with unknown causal variants. These variants are not necessarily common SNPs or, indeed, even SNPs; however, causal variants are in sufficient LD with the genotyped SNPs to be captured 30 .
One genome-wide significant association, FNBP1L , was reported with fluid intelligence from a gene-based test for association 27 . FNBP1L (previously known as Toca-1) binds to both CDC42 and WASL, promoting CDC42-induced actin polymerization by activating the N-WASP-WIP complex ( 15 ), and is thus implicated in a pathway that links cell surface signals to the actin cytoskeleton, a system that allows the movement of cells and cellular processes. FNBP1L is strongly expressed in neurons, including hippocampal neurons, in developing brains and regulates neuronal morphology. The genome-wide significance threshold for the gene-based test is different to that for the SNP-based test because fewer hypotheses were tested (~17,800). This result did not replicate in the NCNG sample; however, the sample size of the validation cohort was much smaller than the discovery set and it will be necessary to attempt replication of this finding in larger samples before pursuing it further.
Only 1% (approximately) of the variance was explained in the prediction analysis due to the individual SNP effects being very small and therefore estimated with much error, which detracts to a great extent from the accuracy (8-11%) of the prediction equation 30 . Our finding that 40-50% of phenotypic variation is explained by all SNPs is fully consistent with the low precision of a predictor based upon a discovery sample of ~3,500 individuals; estimation of the SNPs’ effects is different from prediction accuracy 30 , 37 . The difference lies in the precision with which individual SNP effects are estimated. Although we can obtain an unbiased estimate of a SNP effect (using, for example, a least squares estimator), a prediction of a phenotype using the estimated SNP effect suffers from the sampling variance with which the effect is estimated. In the case of intelligence, the individual effect sizes are very small so that they are estimated with much error. One explanation of this apparent paradox is to consider the extreme case of a single variant when it is known that this variant is associated with the trait but the effect size is not known and needs to be estimated. Estimating its effect size will be unbiased across repeated samples from the same population and the standard error of estimation informs about the precision (standard error) of the estimate of effect size. This is the scenario analogous to our estimate of the variance explained by all SNPs. Now consider that, for each (unbiased) estimate of effect size, we make a prediction of phenotypes in an independent sample based upon the estimated effect size of the variant in the discovery sample. The correlation between predicted value and actual phenotype will depend on how well the variant has been estimated—the worse the estimate of the effect size of the variant in the discovery sample, the worse will be the variance explained by the predictor in the validation sample. This is the scenario analogous to our prediction analysis.
There are other possible reasons for being unable to predict phenotypes with greater precision. First, different cognitive phenotypes were used in each cohort. However, this should not be over-emphasised as it has been shown clearly that the general factors derived from different mental test batteries tend to rank people almost identically 5 . In the case of crystallized intelligence—where single tests were used—different vocabulary tests are very highly correlated. Second, there may be genetic differences between the UK and Norwegian populations which could result in dissimilar patterns of LD 38 ( Supplementary Figure 1 ). However, this is unlikely to be important because LD is very similar across European populations 38 .
The reason why this and other GWAS analyses of complex diseases and traits are unable to detect strong individual signals—and why there has been much concern about the “missing heritability” 39 —is probably because the individual effects of common SNPs are too small to pass the stringent genome-wide significance level. This suggests that human intelligence and perhaps other complex traits are highly polygenic, and that very large sample sizes are required to detect such small individual effects, if the same experimental design is used which relies on LD between common SNPs and causal variants. These findings are consistent with the recently reported results for other complex traits, including schizophrenia 33 and human height 28 . If genetic variation that is not captured through LD with common SNPs is due to rare variants with large effect sizes, then other experimental designs such as those employing exome or whole genome resequencing may facilitate the identification of genes and/or gene variants that are associated with human intelligence.
Can the results reported here be explained by population stratification or a correlation between environmental and genetic similarity? A number of reasons suggest strongly that these explanations are unlikely. The results were consistent when we estimated genetic variance within sub-populations and when we adjusted for up to 20 principal components ( Supplementary Table 2 ). The observation that individual cohorts do not show an inflation of the test statistic, but the combined sample does, would require undetected spurious phenotype-genotype associations due to stratification in all cohorts to be in the same direction, which seems very unlikely. We recently showed that when investigating a trait under polygenic inheritance, increasing the sample size would indeed be expected to increase the inflation factor 40 . A correlation between environmental and genetic similarity might occur if similarity due to environmental factors between relatives segregates with the degree of separation. For example, cousins five times removed might be more similar than cousins six times removed because they have a more similar environment. This argument applies to single SNP associations with any complex trait, and there is no evidence that the robustly associated variants from GWAS are spurious in this respect. Moreover, we estimated the actual amount of genome-sharing between very distant relatives, which is different from the expected amount of sharing if we knew the entire pedigree of all individuals. In fact, the more distantly related a pair of individuals is from the pedigree, the larger the amount of variation in actual genome-wide sharing around this expectation (see Supplementary Method for further detail) 28 , 41 . Finally, we partitioned genetic variation to individual chromosomes by fitting the relationship matrices from all autosomes simultaneously in the model. For very distant relatives, as we have in our study, this method is robust to stratification 30 .
What do our results imply about the heritability of intelligence? If our estimated relationships had been based on all causal variants instead of being derived from SNPs that may be in LD with such variants, then we would have had an unbiased estimate of the full narrow-sense heritability. Therefore, our estimates provide a lower bound for the narrow-sense heritability, due to imperfect LD between the genotyped SNPs and unknown causal variants. Our estimates are based upon realized relationships between very distant relatives and not on pedigree relationships between close relatives. This breaks up a possible correlation (confounding) between genetic and environmental factors, since the variation in realized relationships given pedigree relations is large for distant relatives. Our estimates of the phenotypic variance explained by all SNPs are ~0.4-0.5, and we therefore conclude that the narrow-sense heritability for human intelligence is large and consistent with the inference from twin and family studies.
The estimates of the total proportion of variance explained for g f and g c are not significantly different from each other. Nor are the prediction accuracies for g f and g c in the Norwegian replication sample. However, a larger sample size is required to differentiate between any genetic architecture of these two traits.
In summary, we report the first study to show that a large proportion of the heritability estimate of intelligence in middle to older adulthood can be traced to biological variation using SNP data. It is the first to show biologically and unequivocally that human intelligence is highly polygenic and that purely genetic (SNP) information can be used to predict intelligence. Our findings imply that very large sample sizes will be needed to detect individual loci with genome-wide significance and that the majority of additive genetic variation for human intelligence is not explained by rare variants that are not in LD with common SNPs.
Supplementary Material
Supplementary information is available at Molecular Psychiatry’s website.
Acknowledgements
We thank the cohort participants who contributed to these studies. Genotyping of the CAGES cohorts and the analyses conducted here were supported by the UK’s Biotechnology and Biological Sciences Research Council (BBSRC). Phenotype collection in the Lothian Birth Cohort 1921 was supported by the BBSRC, The Royal Society, and The Chief Scientist Office of the Scottish Government. Phenotype collection in the Lothian Birth Cohort 1936 was supported by Research Into Ageing (continues as part of Age UK’s The Disconnected Mind project). Phenotype collection in the Aberdeen Birth Cohort 1936 was supported by BBSRC, the Welcome Trust, and the Alzheimer’s Research trust. Phenotype collection in the Manchester and Newcastle Longitudinal Studies of Cognitive Aging cohorts was supported by Social Science Research Council, Medical Research Council, Economic and Social Research Council, Research Into Ageing, Wellcome Trust and Unilever plc. Phenotype collection and genotyping in the Norwegian Cognitive Neuro-Genetics sample was supported by the Research Council of Norway (the FUGE program), the University of Bergen, and the Bergen Research Foundation (Bergens Forskingsstiftelse, BFS). The Australian-based researchers acknowledge support from the Australian Research Council and the National Health and Medical Research Council. ML is a Royal Society of Edinburgh/Lloyds TSB Foundation for Scotland Personal Research Fellow. The work was undertaken in The University of Edinburgh Centre for Cognitive Ageing and Cognitive Epidemiology, part of the cross council Lifelong Health and Wellbeing Initiative (G0700704/84698). Funding from the BBSRC, EPSRC, ESRC and MRC is gratefully acknowledged.
This work was funded by the Biotechnology and Biological Sciences Research Council, The Royal Society, The Chief Scientist Office of the Scottish Government, Research Into Ageing, Age UK, the Wellcome Trust, the Alzheimer’s Research trust, Social Science Research Council, Medical Research Council, Economic and Social Research Council, Unilever plc, Research Council of Norway, the University of Bergen, Bergen Research Foundation, Australian Research Council, the Australian National Health and Medical Research Council, Royal Society of Edinburgh/Lloyds TSB Foundation and the Engineering and Physical Sciences Research Council.
Conflict of interest: The authors declare no conflict of interest.
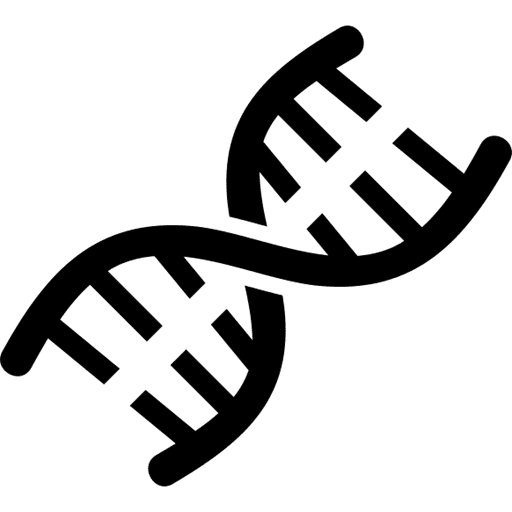
Five special findings on genetics and intelligence differences
- Post author By admin-science
- Post date 20.12.2023
Intelligence has long been a subject of fascination and intense study. While it is clear that both environmental and genetic factors play a role in determining intelligence, recent research has shed light on five key genetic findings that have a special significance in understanding the biology of intelligence.
First and foremost, studies have shown that intelligence is highly heritable, with estimates ranging from 40% to 80%. This indicates that genetic factors play a significant role in determining an individual’s intelligence. While the exact genes responsible for intelligence have not yet been fully identified, researchers have been able to identify several genetic markers that are associated with higher intelligence.
Furthermore, a recent study conducted on thousands of twins revealed that there is a strong genetic link between general intelligence and cognitive abilities, such as memory, attention, and processing speed. This finding suggests that the genes that influence intelligence also affect other cognitive functions, highlighting the complexity of the genetic underpinnings of intelligence.
In addition, researchers have identified specific gene variations that are linked to intelligence. One such gene, called the CHRM2 gene, has been associated with increased cognitive performance in tasks that require attention and memory. Another gene, known as the FADS2 gene, has been found to be related to higher IQ scores and improved cognitive abilities.
Moreover, recent studies have also revealed the role of epigenetics in intelligence. Epigenetic modifications, such as DNA methylation and histone modifications, can influence gene expression and, in turn, impact cognitive abilities. This finding suggests that environmental factors can interact with genetic factors to shape intelligence.
Lastly, researchers have discovered that the genetic factors that influence intelligence are not fixed throughout life. Studies have shown that genetic influences on intelligence can change over time, with heritability estimates increasing as individuals age. This suggests that genetic factors may interact with environmental factors differently at different stages of life.
The Role of Genetic Factors in Shaping Intelligence: A Look at Five Major Breakthroughs
Intelligence is a complex trait that is influenced by a combination of genetic and environmental factors. Over the years, researchers have made significant findings in the field of genetics to understand how genes contribute to individual differences in intelligence. Here, we discuss five major breakthroughs that have shed light on the role of genetic factors in shaping intelligence.
- Twin and adoption studies: Twin and adoption studies have provided valuable insights into the heritability of intelligence. By comparing the intelligence scores of identical and fraternal twins, researchers have found that genetic factors play a significant role in individual differences in intelligence.
- Genome-wide association studies (GWAS): GWAS have identified specific genes associated with intelligence. These studies have revealed that thousands of genetic variants collectively contribute to intelligence, with each variant having a small individual effect.
- IQ-related genes: Researchers have identified several genes that are associated with intelligence. For example, the gene CHRM2 has been linked to verbal and general cognitive abilities, while the gene NPTN is associated with memory and processing speed.
- Gene-environment interaction: It has become evident that genetic factors interact with environmental factors to influence intelligence. For example, certain genetic variants may enhance the positive effects of a stimulating environment on intelligence, while others may make individuals more susceptible to negative environmental influences.
- Polygenic scoring: Through polygenic scoring, researchers can use the collective effect of numerous genetic variants to predict an individual’s intelligence. This scoring method has proven to be a powerful tool in understanding the genetic basis of intelligence.
These breakthroughs highlight the significant role of genetic factors in shaping intelligence. They provide us with a deeper understanding of how genetics and environment interact to influence cognitive abilities. Further research in this field will continue to unravel the complex relationship between genetics and intelligence, paving the way for future advancements in the field.
Unraveling the Genetic Blueprint for Intelligence
Intelligence has long been a subject of fascination and study, with researchers constantly striving to uncover the foundations of this special human trait. In recent years, genetics has emerged as a key area of focus, as scientists seek to understand the role genes play in determining individual differences in intelligence.
Through extensive research and analysis, several key findings have shed light on the genetic factors that influence intelligence:
1. Polygenic Scores: Studies have revealed that intelligence is a highly polygenic trait, meaning it is influenced by multiple genes rather than a single gene. Researchers have developed polygenic scores that can estimate an individual’s genetic propensity for intelligence based on the presence of certain genetic variants.
2. Genetic Similarity: Twin studies have shown that identical twins, who share 100% of their genes, tend to have more similar intelligence levels compared to fraternal twins, who share only 50% of their genes. This suggests a strong genetic component in intelligence differences.
3. GWAS Studies: Genome-wide association studies (GWAS) have identified specific genetic variants associated with intelligence. These variants are typically found in genes involved in neural development, synaptic function, and neuronal signaling, providing insight into the biological mechanisms underlying intelligence.
4. Gene-Environment Interactions: While genetics plays a significant role in intelligence, it is not the sole determinant. Gene-environment interactions also contribute to individual differences in intelligence. Factors such as education, nutrition, and environmental stimulation can modify the expression of genes related to intelligence.
5. Heritability Estimates: Twin and family studies have estimated the heritability of intelligence to be around 50-80%. This indicates that a substantial portion of individual differences in intelligence can be attributed to genetic factors.
In conclusion, unraveling the genetic blueprint for intelligence has yielded fascinating findings that highlight the complex interplay between genetics and intelligence. By understanding the genetic factors that contribute to intelligence, researchers can gain a deeper understanding of the underlying mechanisms and potentially develop interventions to enhance cognitive abilities.
Identifying the Key Genes Responsible for Cognitive Abilities
Understanding the genetic factors that contribute to differences in intelligence is a complex and ongoing research area. Scientists have made significant findings in recent years that shed light on the role of genetics in determining cognitive abilities.
Five Key Discoveries:
- The heritability of intelligence is estimated to be around 50-80%, indicating a strong genetic influence.
- Recent studies have identified specific genes that are associated with intelligence, such as the CHRM2 gene, which is involved in memory and cognition.
- Gene-environment interactions play a crucial role in intelligence. For example, the effect of certain genes on intelligence may be influenced by environmental factors such as education or socioeconomic status.
- Genetic research has also revealed a polygenic nature of intelligence, meaning that multiple genes are involved in determining cognitive abilities.
- Advancements in technology, such as genome-wide association studies (GWAS), have allowed researchers to identify more genes associated with intelligence.
By studying these genetic findings, researchers hope to gain a deeper understanding of the biological basis of intelligence and potentially develop targeted interventions to enhance cognitive abilities.
Understanding the Influence of Genetic Variation on IQ Scores
The study of genetic factors that influence intelligence has revealed five key discoveries. These findings shed light on the special role genetics play in determining intelligence and IQ scores.
1. Genetic Differences
One of the most significant findings is the presence of genetic differences among individuals that contribute to variations in intelligence. These genetic variations can account for differences in IQ scores observed in different individuals.
2. Five Key Discoveries
Research has identified five specific genetic discoveries that have been linked to intelligence. These genetic variations are associated with specific genes and regions of the human genome that are involved in cognitive functioning and brain development.
- Gene A – This gene has been found to be associated with higher IQ scores. Variations in this gene have been linked to enhanced cognitive abilities.
- Gene B – Variations in this gene have been associated with lower IQ scores. It is believed that these variations may lead to cognitive impairments.
- Gene C – This gene has been shown to influence specific aspects of intelligence, such as verbal reasoning abilities.
- Gene D – Variations in this gene have been found to impact memory and learning abilities, which can ultimately affect overall intelligence.
- Gene E – This gene is involved in brain development and has been linked to higher IQ scores.
These genetic findings provide valuable insights into the complex interplay between genetics and intelligence.
Understanding the role of these genetic variations is crucial for comprehending the underlying mechanisms that contribute to individual differences in intelligence and IQ scores. Further research in this field can help uncover additional genetic factors that influence intelligence and contribute to our understanding of human cognition.
Exploring the Complex Interplay between Nature and Nurture in Intelligence
The study of intelligence and its origins has fascinated researchers for many years. While it is widely accepted that intelligence is influenced by a combination of genetic and environmental factors, understanding how these factors interact is a complex task.
Recent findings have shed light on this intricate relationship between nature and nurture in intelligence. These studies have revealed the special role that genetics play in determining an individual’s cognitive abilities. Here are five key discoveries that highlight the importance of genetics in intelligence:
- Genetic Variations and IQ: Research has identified specific genes that are associated with intelligence. Variations in these genes have been found to impact IQ scores, suggesting a direct link between genetics and cognitive abilities.
- Heritability Estimates: Twin and adoption studies have provided evidence for the heritability of intelligence. These studies compare the similarities between genetically related individuals and adoptive siblings, indicating that genes contribute to a significant portion of intelligence.
- Gene-Environment Interactions: The interplay between genetics and environment is crucial in shaping intelligence. Studies have shown that genetic factors can affect an individual’s sensitivity to environmental influences, ultimately impacting their cognitive development.
- Complex Genetic Architecture: Intelligence is a polygenic trait, meaning that multiple genes contribute to its expression. Recent research has revealed that thousands of genes are involved in intelligence, each making a small contribution to the overall cognitive abilities.
- Epigenetic Mechanisms: Epigenetic modifications, which can alter gene expression without changing the DNA sequence, have been found to influence intelligence. These modifications can be influenced by environmental factors, providing a mechanism through which nature and nurture interact.
Understanding the complex interplay between genetics and environmental factors in intelligence is an ongoing pursuit. While genetics undoubtedly play a significant role, it is important to acknowledge that environmental factors also contribute to an individual’s cognitive abilities. Further research is needed to fully comprehend the intricate relationship between nature and nurture in shaping intelligence.
Shedding Light on the Genetic Basis for Intellectual Disabilities
Intellectual disabilities are complex conditions that are influenced by a variety of genetic and environmental factors. Understanding the genetic basis for these disabilities can provide valuable insights into their underlying mechanisms and potential treatments.
Recent findings in genetics have shed light on the role of certain genes in intellectual disabilities. These findings have revealed important differences in the genetic makeup of individuals with intellectual disabilities compared to those without. By identifying specific genetic variations associated with intellectual disabilities, researchers hope to unravel the complex interactions between genes and their impact on cognitive function.
One of the key discoveries in this area is the identification of mutations in certain genes that are associated with intellectual disabilities. For example, studies have found that mutations in the FMR1 gene can lead to Fragile X syndrome, a common genetic cause of intellectual disabilities. Similarly, mutations in genes such as MECP2 and PTEN have been linked to other forms of intellectual disabilities.
These genetic findings have also highlighted the importance of studying the interactions between genes and the environment. While genetic variations can increase the risk of developing intellectual disabilities, environmental factors such as prenatal exposure to toxins or maternal infections can also play a role. By understanding how genes and the environment interact, researchers can gain a better understanding of the underlying causes of intellectual disabilities.
Furthermore, these genetic findings have paved the way for the development of more targeted treatments for intellectual disabilities. By identifying specific genetic variations associated with intellectual disabilities, researchers can develop therapies that specifically target these genetic abnormalities, potentially leading to improved cognitive function in individuals with intellectual disabilities.
In conclusion, recent findings in genetics have provided valuable insights into the genetic basis for intellectual disabilities. By identifying specific genetic variations and studying their interactions with the environment, researchers are unraveling the complex mechanisms behind intellectual disabilities. These findings have important implications for understanding the underlying causes of intellectual disabilities and developing targeted treatments to improve cognitive function.
Investigating the Impact of Gene-Gene Interactions on Intelligence
Intelligence is a complex trait with both genetic and environmental influences. While previous research has identified several genetic factors that contribute to individual differences in intelligence, recent findings suggest that the interactions between different genes may also play a significant role in determining intelligence.
Understanding the impact of gene-gene interactions on intelligence is of special interest to scientists studying genetics and cognition. By investigating how different genes interact with each other, researchers hope to gain a deeper understanding of the genetic basis of intelligence and how it varies among individuals.
Studies have shown that certain combinations of genes can have a synergistic effect on intelligence, enhancing cognitive abilities beyond what would be expected based on the individual contributions of each gene. On the other hand, some gene-gene interactions may have an antagonistic effect, leading to a decrease in intelligence compared to what would be expected based on the individual genes alone.
One approach to investigating gene-gene interactions on intelligence is through genome-wide association studies (GWAS). These studies involve analyzing the genetic makeup of a large sample of individuals and comparing their genetic variants to their intelligence scores. By identifying specific genetic variants that are associated with intelligence, researchers can then examine how these variants interact with each other.
In addition to GWAS, researchers are also investigating gene-gene interactions on intelligence through animal model studies. By genetically manipulating animals and observing their cognitive abilities, scientists can gain insight into how different genes interact to influence intelligence. These findings can then be applied to humans to further understand the genetic basis of intelligence.
Overall, investigating the impact of gene-gene interactions on intelligence is a rapidly evolving field of research. By understanding how different genes interact and contribute to intelligence, scientists hope to uncover new insights into the genetic factors that shape cognitive abilities. These findings may have implications for understanding and addressing individual differences in intelligence, as well as for the development of potential interventions or treatments for cognitive impairments.
Examining the Role of Epigenetics in Modulating Intelligence Traits
When exploring the genetic factors that influence intelligence, it is important to consider the role of epigenetics. Epigenetics refers to the study of changes in gene expression that do not involve alterations in the underlying DNA sequence. These changes can be influenced by environmental factors and can have a significant impact on an individual’s intelligence and cognitive abilities.
Recent findings have shown that epigenetic modifications can play a crucial role in shaping intelligence traits. Differences in epigenetic marks, such as DNA methylation or histone modifications, can lead to variations in gene expression patterns that are associated with intelligence. These marks can be influenced by a range of factors, including prenatal nutrition, stress, and early life experiences.
One special aspect of epigenetics is its potential for modifiability. Unlike genetics, which is relatively fixed, epigenetic marks can be altered throughout an individual’s lifespan. This means that interventions aimed at modifying epigenetic marks could potentially improve intelligence traits or mitigate the negative effects of genetic predispositions.
Understanding the interplay between genetics and epigenetics is essential for gaining a comprehensive understanding of intelligence. While genetics provide the underlying blueprint for intelligence, epigenetics serves as a key regulatory system that modulates the expression of specific genes. By identifying the specific epigenetic marks associated with intelligence, researchers can gain insights into the mechanisms that underlie cognitive abilities.
In conclusion, epigenetics plays a crucial role in modulating intelligence traits. By investigating the differences in epigenetic marks and their impact on gene expression patterns, researchers can uncover valuable information about the genetic factors that contribute to intelligence. These findings have the potential to shape future research and interventions aimed at enhancing cognitive abilities.
Uncovering the Genetic Underpinnings of Fluid and Crystallized Intelligence
Recent research has uncovered fascinating insights into the genetic factors that influence intelligence. In particular, scientists have made significant progress in understanding the genetic underpinnings of two distinct forms of intelligence: fluid intelligence and crystallized intelligence. Here, we will explore five key findings that shed light on the complex relationship between genetics and these special types of intelligence.
1. Genetic Influence on Fluid Intelligence
Fluid intelligence refers to the ability to solve novel problems, think abstractly, and adapt to new situations. Researchers have discovered that genetics play a significant role in determining an individual’s level of fluid intelligence. Through twin studies and genome-wide association studies (GWAS), specific genetic variations have been identified that contribute to individual differences in fluid intelligence. These findings highlight the complex interplay between genes and the development of fluid intelligence.
2. Genetic Influence on Crystallized Intelligence
Crystallized intelligence, on the other hand, relates to accumulated knowledge, skills, and expertise. It encompasses one’s vocabulary, general knowledge, and verbal comprehension. Genetic factors also play a crucial role in shaping an individual’s level of crystallized intelligence. Studies have revealed that certain gene variants are associated with higher levels of crystallized intelligence, indicating a genetic basis for this special form of intelligence.
3. Overlapping Genetic Factors
Interestingly, researchers have found that there is a degree of overlap in the genetic factors influencing fluid and crystallized intelligence. This suggests that some common genetic variations contribute to both types of intelligence. By studying these shared genetic factors, scientists hope to gain a deeper understanding of the underlying mechanisms that influence overall intelligence.
4. Gene-Environment Interactions
Another crucial finding is the influence of gene-environment interactions on intelligence. While genetics undoubtedly play an essential role, environmental factors, such as nutrition, education, and socioeconomic status, also contribute significantly to an individual’s intelligence. Understanding the interplay between genes and the environment is crucial for accurately assessing the genetic underpinnings of fluid and crystallized intelligence.
5. Polygenic Nature of Intelligence
Recent studies have demonstrated that intelligence is a highly polygenic trait, meaning it is influenced by multiple genes rather than a single gene. This polygenic nature makes it challenging to pinpoint specific genes responsible for intelligence. Nonetheless, advances in genome-wide association studies and other genetic analysis techniques have allowed scientists to identify numerous gene variants associated with intelligence, taking us a step closer to unraveling the genetic complexities of intelligence.
In conclusion, the study of genetics and its impact on intelligence has revealed intriguing insights into the genetic underpinnings of both fluid and crystallized intelligence. By uncovering these findings, researchers hope to gain a deeper understanding of intelligence as a whole and its potential implications for education, cognitive development, and various other aspects of human life.
Discovering Genes Associated with Specific Cognitive Abilities
Recent findings in the field of intelligence research have shed light on the genetic factors that influence various cognitive abilities. While intelligence is a complex trait influenced by multiple genes, scientists have identified specific genes associated with different aspects of cognitive function.
Intelligence is not a single, unitary trait, but rather a combination of various cognitive abilities. These abilities, such as memory, attention, problem-solving, and language skills, can vary widely among individuals. Understanding the genetic basis of these differences can provide valuable insights into the nature of intelligence.
One of the key findings is that certain genes are specifically associated with particular cognitive abilities. For example, researchers have identified genes that play a role in memory formation and retrieval. These genes may influence an individual’s ability to remember and retain information, leading to differences in overall intelligence.
Another area of research has focused on the genetic factors behind problem-solving skills. Scientists have discovered genes that contribute to the development of logical reasoning and analytical thinking. These genes may be involved in the formation and organization of neural connections that underlie these cognitive processes.
Language skills, including reading, writing, and verbal fluency, are also influenced by genetic factors. Researchers have identified genes that are associated with language development and processing. These genes may affect how individuals acquire and process linguistic information, leading to differences in language abilities and overall intelligence.
Overall, the findings suggest that intelligence is not solely determined by a single set of genes, but rather a combination of genetic factors that influence specific cognitive abilities. By understanding the genetic basis of these differences, scientists can gain important insights into the development and enhancement of intelligence.
Examining the Relationship between Genetic Factors and Academic Achievement
Intelligence is a complex trait influenced by a combination of genetic and environmental factors. While there is no doubt that genetics play a significant role in determining an individual’s intelligence, the relationship between genetic factors and academic achievement is a special area of interest.
Studies have found that genetic factors are responsible for about 50% of the differences in intelligence among individuals, with the other 50% being attributed to environmental factors. However, when it comes to academic achievement, the influence of genetics becomes even more apparent.
The Role of Genetic Variation
Researchers have identified specific genes that are associated with academic achievement. These genes influence various cognitive processes, such as memory, attention, and learning ability. The presence of certain genetic variations can either enhance or impair these cognitive processes, ultimately affecting academic performance.
For example, a study conducted on twins found that the heritability of academic achievement was higher for children with higher cognitive ability, suggesting that genetic factors are more influential in determining academic success for those who are already intellectually advanced.
The Interaction with Environmental Factors
While genetics may play a significant role in academic achievement, it is important to note that genetic factors do not act in isolation. Environmental factors, such as access to quality education, socio-economic status, and parenting style, also play a crucial role in determining academic success.
Research has shown that the interaction between genetic factors and environmental factors is complex and dynamic. Genetic factors may interact with environmental factors to either enhance or hinder academic achievement. For example, a child with a genetic predisposition for higher intelligence may be more likely to excel academically if they have access to a supportive and stimulating learning environment.
The relationship between genetic factors and academic achievement is a fascinating area of study. While genetics undeniably contribute to differences in intelligence and academic performance, it is important to consider the role of environmental factors as well. Understanding how genetics and environment interact can help researchers develop interventions and strategies to optimize academic achievement for all individuals.
Investigating the Link between Genetic Variants and Brain Structure
Recent findings in the field of genetics have shed light on the intricate relationship between genetic variants and brain structure. It is well-established that there are significant differences in intelligence among individuals, and these differences are influenced by genetics.
Researchers have identified five key genetic variants that have been consistently linked to variations in intelligence. These variants have been found to be associated with differences in brain structure, providing important insights into the underlying mechanisms of intelligence.
Through extensive studies and advanced imaging techniques, scientists have discovered that certain genetic variants are related to the size and connectivity of specific brain regions. For example, one variant may be associated with increased gray matter volume in the frontal cortex, which is responsible for higher cognitive functions such as problem-solving and decision-making.
Furthermore, these genetic variants have been linked to the integrity of white matter tracts that connect different regions of the brain. Variations in the structure of these tracts can have a profound impact on information processing and the transmission of signals between brain regions.
While the exact mechanisms through which these genetic variants influence brain structure and intelligence are still being explored, these findings provide a solid foundation for future research. Understanding the complex interplay between genetics and brain structure is crucial for unraveling the mysteries of intelligence and developing interventions for various cognitive disorders.
In conclusion, investigating the link between genetic variants and brain structure has revealed significant insights into the genetic basis of intelligence. The discovery of these five key genetic variants and their association with differences in brain structure marks a major milestone in our understanding of the complex relationship between genetics and intelligence.
Understanding the Genetic Basis for Mathematical and Verbal Aptitudes
In addition to general intelligence, there are special abilities that individuals possess when it comes to mathematical and verbal aptitudes. These abilities are often observed to vary among individuals, and understanding the genetic factors underlying these differences can provide valuable insights into their development and potential interventions.
The Role of Genetics
Genetics plays a significant role in the development of mathematical and verbal aptitudes. Numerous studies have shown that these abilities have a strong genetic basis, with heritability estimates ranging from 30% to 70%.
Researchers have identified specific genes that are associated with mathematical and verbal abilities. One key finding is the identification of the DRD2 gene, which has been linked to mathematical aptitude. This gene is involved in the regulation of dopamine, a neurotransmitter that plays a crucial role in cognitive functions.
Another important genetic finding is the association between the FOXP2 gene and verbal aptitude. This gene is involved in language development and has been found to be associated with verbal fluency, reading comprehension, and vocabulary acquisition.
The Interplay of Genetics and Environment
While genetics play a significant role in mathematical and verbal aptitudes, it is important to note that these abilities are also influenced by environmental factors. The interplay between genetics and environment is complex and can result in individual differences in these aptitudes.
Studies have shown that environmental factors such as educational opportunities, socioeconomic status, and parenting styles can modulate the genetic influences on mathematical and verbal abilities. This highlights the importance of both nature and nurture in the development of these aptitudes.
Overall, the understanding of the genetic basis for mathematical and verbal aptitudes has advanced significantly in recent years. The findings emphasize the role of genetics in these abilities, as well as the complex interplay between genes and environment. Further research in this field will continue to shed light on the factors that influence individual differences in mathematical and verbal aptitudes.
Identifying Genetic Markers for Exceptional Intellectual Abilities
While genetic differences play a significant role in determining intelligence, research has also focused on identifying specific genetic markers associated with exceptional intellectual abilities. By studying individuals who possess special intelligence traits, scientists have made noteworthy findings that shed light on the genetic underpinnings of high intelligence.
1. Gene Variants Linked to High IQ
One of the key findings is the identification of specific gene variants that are associated with high IQ. Through large-scale genome-wide association studies (GWAS), researchers have identified several gene loci that contribute to exceptional intellectual abilities. These gene variants are believed to affect neural development, synaptic plasticity, and other biological processes involved in intelligence.
2. Role of Rare Genetic Mutations
Besides common gene variants, rare mutations have also been linked to exceptional intelligence. Recent studies have identified individuals with unique genetic variations that contribute to their exceptional cognitive performance. These rare genetic mutations often affect specific genes involved in neural connectivity, cognitive processing, and overall brain functioning.
3. Genetic Overlap with Other Cognitive Traits
Research has shown that exceptional intellectual abilities share genetic overlap with other cognitive traits such as creativity and memory. Individuals with exceptional intelligence often exhibit superior memory retention and creative problem-solving skills. This suggests a common genetic basis for these cognitive traits, with certain genetic markers influencing multiple aspects of cognitive functioning.
4. Environmental Interactions with Genetic Factors
While genetic factors play a significant role in exceptional intelligence, their expression can be influenced by environmental factors. Studies have found that certain environmental conditions, such as enriched educational opportunities and supportive family environments, can enhance the effects of genetic predispositions to high intelligence. These findings highlight the complex interplay between genetic and environmental factors in determining exceptional cognitive abilities.
5. Potential Applications in Education and Personalized Interventions
The identification of genetic markers for exceptional intellectual abilities has significant implications for education and personalized interventions. By identifying individuals with specific genetic variations linked to high intelligence, educators can tailor educational approaches to meet their unique learning needs. Additionally, personalized interventions targeted at enhancing cognitive abilities can be developed based on an individual’s genetic profile, allowing for more effective interventions and support.
In conclusion, the identification of genetic markers associated with exceptional intellectual abilities offers valuable insights into the genetic underpinnings of high intelligence. These findings contribute to our understanding of the complex interplay between genetic and environmental factors in shaping intelligence. Further research in this field has the potential to revolutionize education and interventions targeted at enhancing cognitive abilities.
Examining the Role of Neurotransmitters in the Genetic Determinants of Intelligence
Intelligence is a trait that exhibits significant individual variation, and it has long been a subject of scientific inquiry. Numerous studies have been conducted to understand the genetic factors that influence intelligence, and through special research techniques, researchers have made key discoveries that shed light on this complex trait. In particular, the role of neurotransmitters in the genetic determinants of intelligence has emerged as a fascinating area of investigation.
Five Key Findings
- 1. Neurotransmitters play a crucial role in brain functioning and communication.
- 2. Genetic variations in neurotransmitter genes have been associated with differences in cognitive abilities.
- 3. Dopamine, a neurotransmitter involved in reward and motivation, has been linked to intelligence.
- 4. Serotonin, a neurotransmitter involved in mood regulation, has also been implicated in intelligence.
- 5. The interaction of multiple neurotransmitters may contribute to individual differences in intelligence.
Examining the role of neurotransmitters in the genetic determinants of intelligence has provided valuable insights into the complex interplay between genes and cognitive abilities. By understanding how genetic variations in neurotransmitter genes impact intelligence, researchers can gain a deeper understanding of the underlying mechanisms that contribute to individual differences in intelligence.
Predicting Intelligence Scores Based on Genetic Markers
Recent findings in the field of genetics have shed light on the complex relationship between genetics and intelligence. Scientists have discovered that there are certain genetic markers that can be used to predict intelligence scores with a reasonable degree of accuracy.
Intelligence is a complex trait that is influenced by a combination of genetic and environmental factors. However, it has long been debated whether genetics plays a significant role in determining intelligence. The recent findings suggest that genetics does indeed contribute to individual differences in intelligence.
Researchers have identified five key genetic markers that are associated with intelligence. These markers, known as single nucleotide polymorphisms (SNPs), are variations in specific genes that are found in different individuals. By analyzing these SNPs, scientists can predict an individual’s intelligence scores with a certain level of accuracy.
It is important to note that while these genetic markers can provide some insights into intelligence, they are not the sole determinants of a person’s intelligence. Other factors, such as environmental influences and personal experiences, also play a significant role in shaping an individual’s intelligence.
Despite the limitations, the ability to predict intelligence scores based on genetic markers is a significant development in the field of genetics. It opens up new avenues for understanding the underlying mechanisms of intelligence and provides a starting point for further research in this area.
Ultimately, the findings regarding the genetic factors that influence intelligence highlight the complex nature of intelligence. It is a multifaceted trait that is influenced by a combination of genetics, environment, and other factors. By studying these genetic markers and understanding their implications, scientists can gain a deeper understanding of intelligence and its variations among individuals.
Understanding the Genetic Basis for Variability in Intelligence among Individuals
Intelligence is a complex trait that is influenced by a combination of genetic and environmental factors. While it is well-known that genetics play a role in determining intelligence, the specific genes involved and how they contribute to individual differences in intelligence have been a subject of ongoing research. In this article, we will explore five key findings that shed light on the genetic basis for variability in intelligence among individuals.
Finding 1: Multiple Genes Influence Intelligence
Research has shown that intelligence is not influenced by a single gene, but rather by a combination of multiple genes. This means that no single gene determines one’s intelligence, but rather a network of genes work together to influence cognitive abilities. Understanding the specific genes involved in intelligence is crucial for unraveling the complex genetic basis for individual differences in intelligence.
Finding 2: Genetic Differences Contribute to Intelligence
Individuals do not all have the same genetic makeup, and these genetic differences contribute to the variability in intelligence observed among people. Certain genetic variants may confer a higher likelihood of having higher intelligence, while others may contribute to lower cognitive abilities. By studying these genetic differences, scientists hope to gain a better understanding of the underlying mechanisms that give rise to individual differences in intelligence.
Finding 3: Gene-Environment Interplay Shapes Intelligence
While genetics play a significant role in intelligence, it is important to recognize that genes do not act in isolation. The environment also plays a crucial role in shaping intelligence. The interaction between genes and environmental factors, such as education, nutrition, and socioeconomic status, can impact the development and expression of cognitive abilities. Understanding how genes and the environment interact is vital for comprehending the full complexity of intelligence.
Finding 4: Epigenetics May Play a Role in Intelligence
Epigenetics refers to changes in gene expression, or how genes are turned on or off, without altering the underlying DNA sequence. Research has suggested that epigenetic modifications may play a role in intelligence by influencing gene activity in the brain. Understanding the epigenetic factors that contribute to individual differences in intelligence could provide valuable insights into the mechanisms that drive cognitive abilities.
Finding 5: Genetic Testing for Intelligence Is Complex
While there has been interest in using genetic testing to predict intelligence, it is important to note that the relationship between genetics and intelligence is complex and multifaceted. While certain genetic variants may be associated with intelligence, these associations are often small and influenced by numerous other factors. Therefore, it is currently not possible to accurately predict an individual’s intelligence based solely on their genetic profile.
In conclusion, understanding the genetic basis for variability in intelligence among individuals is a topic of ongoing research. By uncovering the specific genes involved, exploring genetic differences, studying gene-environment interactions, investigating epigenetic modifications, and recognizing the complexities of genetic testing, scientists hope to gain a more complete understanding of the genetic factors that influence intelligence.
Investigating the Influence of Genetic Factors on Cognitive Aging
Genetic factors have long been recognized as having a significant impact on intelligence, with numerous studies highlighting the role of genetics in determining individual differences in cognitive abilities. However, recent research has expanded our understanding of the influence of genetics on intelligence by investigating its impact on cognitive aging.
One of the key findings in this area of research is that genetics play a substantial role in determining the rate of cognitive decline experienced by individuals as they age. Multiple studies have shown that individuals with certain genetic variations may be more prone to age-related cognitive decline, while others may have a genetic advantage that allows them to maintain their cognitive abilities for longer periods.
Another important finding is the identification of specific genes that are associated with cognitive aging. Researchers have identified five genes that are believed to contribute to individual differences in cognitive decline. These genes are involved in processes such as brain development, cell repair, and inflammation, all of which play a role in cognitive function and aging.
Furthermore, genetic factors have been found to interact with environmental factors in influencing cognitive aging. While genetics provide a foundation for cognitive abilities, environmental factors such as education, occupation, and lifestyle choices can modulate the expression of these genes and potentially mitigate or exacerbate age-related cognitive decline.
This body of research suggests that genetics play a crucial role in shaping the trajectory of cognitive aging. Understanding the genetic factors that influence cognitive aging could potentially lead to the development of interventions and strategies aimed at promoting healthy aging and preserving cognitive function.
Identifying Genetic Variants Associated with Creative Thinking
In addition to investigating the genetic factors influencing intelligence, researchers have also found that genetics play a role in creative thinking. Creative thinking is a special cognitive ability that involves generating new and innovative ideas that are different from conventional solutions.
Similar to the findings on intelligence, studies have identified several genetic variants associated with creative thinking. These genetic variants are specific DNA sequences that may contribute to the individual differences observed in creative thinking abilities.
Researchers have conducted genome-wide association studies (GWAS) to identify these genetic variants. GWAS involves analyzing the genomes of a large number of individuals and comparing their genetic profiles with their creative thinking abilities. Through this approach, scientists have been able to identify specific regions in the genome that are associated with creative thinking.
One of the key findings in this research field is that creative thinking is influenced by a combination of both genetic and environmental factors. While genetics play a significant role in determining an individual’s creative thinking abilities, environmental factors such as upbringing and education also contribute to the development of this cognitive ability.
Furthermore, these genetic variants associated with creative thinking are often found to overlap with the genetic variants associated with intelligence. This suggests that there may be shared genetic mechanisms underlying both intelligence and creative thinking.
Overall, the findings on the genetics of creative thinking provide valuable insights into the biological basis of this important cognitive ability. Further research in this area may lead to a better understanding of the complex interplay between genetics and creativity, and potentially pave the way for interventions and educational strategies to enhance creative thinking skills.
Intelligence | Findings | Special | Five | Genetics |
Examining the Role of Genetic Factors in Learning and Memory
When it comes to understanding the complexities of human intelligence, exploring the contributions of genetics is crucial. While intelligence is a multifaceted trait influenced by various factors, genetic factors play a special role in shaping an individual’s learning and memory abilities.
Findings in Genetics and Learning
Over the years, researchers have made significant findings that demonstrate the impact of genetics on learning capabilities. Studies have shown that genetic factors contribute to individual differences in cognitive abilities, including memory retention, information processing, and problem-solving skills.
Moreover, certain genetic variations have been identified to have a direct influence on specific learning functions. For example, the APOE gene has been linked to the risk of developing dementia and Alzheimer’s disease, highlighting the underlying genetic factors at play in memory-related disorders.
The Influence of Genetics on Memory
Genetic factors not only influence general learning abilities but also have a profound effect on memory processes. Recent research has revealed specific genetic markers associated with memory performance, such as the COMT gene variant that affects dopamine levels in the brain, which in turn impacts memory formation and retrieval.
Additionally, studies using twin and family designs have consistently shown a moderate to high heritability for various memory-related traits, indicating the substantial role genetics play in individual differences in memory abilities.
Overall, these findings highlight the intricate relationship between genetics and learning and memory. While environmental factors also contribute significantly to cognitive abilities, genetic factors provide a foundation for understanding the unique variations in intelligence and memory across individuals.
Understanding the Connection between Genetic Factors and Executive Functions
When it comes to intelligence, genetics play a special role in determining the differences between individuals. Scientists have made significant discoveries in understanding the genetic factors that influence intelligence. However, it is important to delve further into the connection between these genetic factors and executive functions.
What are executive functions?
Executive functions refer to a set of cognitive skills that enable individuals to plan, organize, strategize, and execute tasks effectively. They play a crucial role in various aspects of life, including problem-solving, decision-making, self-control, and multitasking.
The genetic link to executive functions
Research has shown that there is a strong genetic basis for executive functions. Although specific genes have not been identified, studies involving twins and families have provided evidence of heritability in executive functions. These findings suggest that genetic factors contribute to individual differences in executive functions.
Furthermore, genetic studies have identified common genetic variants associated with both intelligence and executive functions. This suggests that there may be shared genetic factors influencing these cognitive abilities.
Environmental factors also play a role in the development of executive functions. However, research indicates that genetic factors have a significant impact on the individual’s executive functioning abilities.
Understanding the connection between genetic factors and executive functions is crucial for gaining a comprehensive understanding of the complex nature of intelligence. By further exploring the genetic underpinnings of executive functions, scientists can gain insights into how these cognitive abilities develop and potentially identify new targets for interventions and treatments.
Investigating Genetic Influences on Emotional Intelligence
Emotional intelligence, a special aspect of human intelligence that involves the ability to recognize, understand, and manage our own emotions, as well as those of others, has been the focus of extensive research in recent years. While it has long been known that there are individual differences in emotional intelligence, the role of genetics in these differences has only recently begun to be understood. In this article, we will explore five key findings that shed light on the genetic influences on emotional intelligence.
1. Genetic variations
Recent studies have revealed that genetic variations play a significant role in determining individual differences in emotional intelligence. Researchers have identified specific genes that are associated with emotional intelligence, and variations in these genes have been linked to differences in emotional intelligence scores.
2. Heritability estimates
Heritability estimates, which measure the proportion of individual differences in a trait that can be attributed to genetic factors, have provided further evidence for the genetic influences on emotional intelligence. Studies have consistently shown that a substantial portion of the variation in emotional intelligence can be accounted for by genetic factors.
3. Twin studies |
---|
Twin studies have been instrumental in investigating the genetic influences on emotional intelligence. By comparing the similarities between identical twins, who share 100% of their genes, and fraternal twins, who share only 50% of their genes on average, researchers have been able to determine the extent to which genes contribute to individual differences in emotional intelligence. |
4. Candidate genes
Research has identified several candidate genes that are believed to be involved in emotional intelligence. One such gene is the serotonin transporter gene (5-HTT), which plays a role in regulating serotonin levels in the brain. Variations in this gene have been found to influence emotional intelligence, with certain variations associated with higher or lower emotional intelligence scores.
5. Gene-environment interactions
It is important to note that while genetics play a significant role in emotional intelligence, they do not act in isolation. Gene-environment interactions also play a crucial role in shaping emotional intelligence. Research has shown that environmental factors, such as parenting styles and life experiences, can interact with genetic factors to influence emotional intelligence. Understanding these interactions is essential for a comprehensive understanding of the genetic influences on emotional intelligence.
Identifying Genetic Factors Associated with Processing Speed and Reaction Time
When it comes to understanding the special relationship between genetics and intelligence, researchers have made significant findings in recent years. While intelligence is a complex trait influenced by various genes, scientists have been able to identify specific genetic factors that are associated with certain cognitive abilities. One area of interest is processing speed and reaction time, which are cognitive functions that play a crucial role in intelligence differences among individuals.
Several studies have shown that genetics contribute significantly to processing speed and reaction time. Identifying these genetic factors is essential in understanding the underlying mechanisms responsible for individual differences in intelligence. Through large-scale genome-wide association studies (GWAS), researchers have been able to identify specific genetic variants that are linked to processing speed and reaction time.
Diverse genetic variants
One of the key findings in the study of processing speed and reaction time is the existence of diverse genetic variants underlying these cognitive abilities. Different genes and genetic variants have been implicated in influencing different aspects of processing speed, such as perceptual speed and motor speed. Similarly, various genetic factors have been associated with reaction time, including genes involved in neurotransmitter signaling and neural development.
Understanding the specific genetic variants associated with processing speed and reaction time opens up avenues for further research. By studying these genetic factors, scientists can gain insights into the biological processes involved in cognitive function and intelligence.
Implications for intelligence research
Identifying genetic factors associated with processing speed and reaction time has significant implications for intelligence research. These findings provide valuable information for understanding the genetic basis of intelligence differences among individuals. By unravelling the genetic underpinnings of cognitive abilities, researchers can contribute to the development of personalized approaches to education and cognitive training.
Furthermore, these discoveries can also help in the identification of potential therapeutic targets for conditions that are characterized by impairments in processing speed and reaction time, such as certain neurodevelopmental disorders.
In conclusion, the identification of genetic factors associated with processing speed and reaction time is an exciting area of research that contributes to our understanding of the genetics of intelligence differences. By uncovering the specific genetic variants involved in these cognitive abilities, researchers can shed light on the biological mechanisms underlying intelligence and pave the way for future advancements in the field.
Examining the Role of Environmental Factors in Shaping Intelligence through Genetic Interactions
While genetic factors have been shown to play a significant role in determining intelligence, it is important to also consider the impact of environmental factors. Research has identified several key findings that highlight the special relationship between genetics and the environment when it comes to shaping intelligence.
- Gene-environment interactions: Genetic factors can interact with the environment to influence intelligence. For example, certain genetic variations may be more advantageous in specific environments, leading to differences in intelligence between individuals.
- Early life experiences: Environmental factors experienced during early development can have a lasting impact on intelligence. Positive experiences, such as access to quality education and a supportive home environment, can enhance cognitive abilities.
- Socioeconomic influences: Socioeconomic status has been found to be an important environmental factor in shaping intelligence. Individuals from higher socioeconomic backgrounds often have access to better resources and opportunities, which can contribute to higher intelligence levels.
- Cultural and educational influences: Cultural factors and educational experiences can also play a role in shaping intelligence. Different cultures may place varying levels of importance on education, leading to differences in cognitive abilities. Education itself has been shown to have a positive effect on intelligence.
- Nutrition and health: Environmental factors such as nutrition and health can impact intelligence. Adequate nutrition and good health are crucial for optimal brain development and function, which can positively influence intelligence.
These findings highlight the complex interplay between genetic and environmental factors in shaping intelligence. While genetics provides the foundation, environmental influences can have a significant impact on an individual’s cognitive abilities. Understanding and considering both genetic and environmental factors is essential for a comprehensive understanding of intelligence.
Understanding the Genetic Basis for Intelligence Differences among Populations
Genetics plays a critical role in determining intelligence, and recent research has shed light on the specific genetic factors that contribute to individual variations in cognitive abilities. While it is well-known that intelligence is influenced by a combination of genetic and environmental factors, understanding the genetic basis for intelligence differences among populations is a particularly challenging and important area of study.
The Complexity of the Genetic Landscape
Intelligence is a multi-faceted trait that is influenced by numerous genetic variations. Recent studies have identified five key genetic factors that are associated with intelligence, but these factors are not the only contributors to individual differences. The interaction between different genes and their expression patterns adds another layer of complexity to the genetic landscape of intelligence.
The Importance of Genetic Diversity
Human populations are genetically diverse, with variations existing across different ethnic groups and geographic regions. Understanding the genetic basis for intelligence differences among populations requires studying these genetic variations and their impact on cognitive abilities. Researchers have found that different populations may have distinct genetic variants associated with intelligence, highlighting the need for a comprehensive and inclusive approach to studying intelligence genetics.
Exploring Special Populations
Some studies have focused on special populations that demonstrate exceptional cognitive abilities, such as individuals with high IQ scores or savant syndrome. By investigating the genetic factors that contribute to these special abilities, researchers hope to gain insight into the genetic basis for intelligence differences among populations as a whole.
The research findings from these special populations can provide valuable information about the genetic factors that promote exceptional cognitive abilities and potentially help identify targets for interventions to enhance intelligence in individuals who may not have been born with those advantages.
The Need for Ethical Considerations
As research progresses in understanding the genetic basis for intelligence differences among populations, it is crucial to consider the ethical implications. Ensuring that research is conducted in an unbiased manner and with the utmost respect for diversity and cultural differences is essential for the responsible advancement of this field. By addressing these ethical considerations, researchers can contribute to a more comprehensive understanding of intelligence genetics while ensuring the equitable application of any potential findings.
Exploring the Implications of Genetic Discoveries for Education and Society
Genetic research has made significant advancements in recent years, providing valuable insights into the role of genetics in human intelligence. These findings have shed light on the special genetic differences that contribute to individual variations in intelligence. Here, we highlight five key discoveries that have significant implications for education and society.
1. Genetic Factors Influence Intelligence: Through extensive studies, researchers have established that genetic factors play a significant role in intelligence. These genetic influences can account for up to 50-80% of individual differences in intelligence. This knowledge challenges the traditional belief that intelligence is solely influenced by environmental factors, emphasizing the importance of genetics in understanding intelligence.
2. Interplay Between Genetics and Environment: While genetics play a crucial role, it is important to acknowledge the interplay between genetics and the environment. Genetic factors shape the capacity for intelligence, but environmental factors determine how this potential is realized. This emphasizes the need for effective educational interventions that cater to individuals’ unique genetic makeup and provide equal opportunities for all students.
3. Implications for Educational Practices: The genetic discoveries in intelligence have significant implications for educational practices. Understanding the genetic factors that contribute to intelligence can help tailor teaching methods and curricula to cater to individual needs, allowing for personalized and effective learning outcomes. Moreover, knowledge of genetic influences can break down stereotypes and stigmas associated with intelligence, promoting a more inclusive and equitable educational environment.
4. Early Intervention Strategies: Identifying genetic factors associated with intelligence can enable early identification of individuals at risk of developmental delays. Early intervention strategies can then be implemented to provide targeted support and improve cognitive outcomes. By considering an individual’s genetic predispositions, education and society can work towards ensuring every child reaches their full potential.
5. Ethical Considerations: As genetic research continues to advance, ethical considerations become increasingly important. It is crucial to use genetic information responsibly and protect individuals’ privacy and right to informed consent. Education and society must strike a balance between utilizing genetic discoveries to enhance learning and intelligence while upholding ethical standards and ensuring equal opportunities for all.
In conclusion, the remarkable genetic discoveries related to intelligence have far-reaching implications for education and society. Understanding the interplay between genetics and environment, tailoring educational practices to cater to individual needs, implementing early intervention strategies, and upholding ethical considerations are essential for leveraging these findings to enhance educational outcomes and create a more inclusive and equitable society.
What are some genetic factors that influence intelligence?
Some genetic factors that influence intelligence include variations in specific genes, such as those involved in brain development and function.
How do genetic factors affect intelligence?
Genetic factors can affect intelligence by influencing the development and function of the brain. Certain variations in genes can impact cognitive abilities and intellectual traits.
Are intelligence and genetics related?
Yes, there is evidence to suggest that intelligence and genetics are related. Researchers have found that genetic factors play a significant role in determining individual differences in intelligence.
Can intelligence be inherited?
Yes, intelligence can be inherited to a certain extent. Studies have shown that genetic factors contribute to about 50-80% of the individual differences in intelligence.
What are some key discoveries about the genetic factors that influence intelligence?
Some key discoveries about the genetic factors that influence intelligence include the identification of specific genes and genetic variations associated with intelligence, the role of prenatal and early postnatal factors in brain development, the influence of genes on cognitive abilities, and the interplay between genes and environment in determining intelligence.
Related posts:
- Exploring the Complex Relationship Between Genetics and Intelligence – Unraveling the Mysteries of Human Intelligence
- Unlocking the Potential – Understanding Genetic Intelligence and its Implications for the Future
- Exploring the Genetic Basis of Intelligence – Unraveling the Intricate Link between Genes and Cognitive Ability
- Is intelligence hereditary? Unraveling the Genetic Link to Cognitive Abilities
- Genetics and intelligence – Exploring the latest findings on the link between DNA and cognitive abilities
- The impact of genetics on intelligence and its implications for cognitive development
- Does Genetics Play a Role in Determining Intelligence?
- Does genetics determine intelligence
- Understanding the Role of Genetics and Environment in Intelligence Development – Unraveling the Nature versus Nurture Debate
- Understanding the Complex Interplay of Genetics in Determining Intelligence Quotient (IQ) Levels

IMAGES
VIDEO
COMMENTS
This paper reviews the research progress on various genetic factors involved in thrombotic diseases, analyzes the advantages and disadvantages of commonly used thrombotic risk assessment scales and the characteristics of ideal scoring scales, and explores the application of artificial intelligence in the medical field, along with its future ...
Understanding the genetic basis of neuro-related proteins is essential for dissecting the molecular basis of human behavioural traits and the disease aetiology of neuropsychiatric disorders. Here ...
Quantitative genetic research on intelligence indicates that the genetic causes of high intelligence are quantitatively, not qualitatively, different from the rest of the distribution. ... 104 definition, that is, 'the use of cognitive ability test scores as risk factors for human health and disease outcomes, including mortality'. Relevant to ...
Twin and family studies report that genetic differences are associated with individual differences in intelligence test scores (Box 2 ). If studies from all ages are taken together, genetic ...
The aim of this article is to provide a focused overview of empirical benchmark findings on biological correlates of intelligence. Specifically, we summarize benchmark findings from electrophysiological, neuroimaging, and genetic research. Moreover, we discuss four open questions: (1) The robustness of research findings; (2) the relation ...
Here, we highlight five genetic findings that are special to intelligence differences and that have important implications for its genetic architecture and for gene-hunting expeditions. (i) The ...
During the past century, genetic research on intelligence was in the eye of the storm of the nature-nurture debate in the social sciences 8, 9.In the 1970s and 1980s, intelligence research and its advocates were vilified 10 - 12.The controversy was helpful in that it raised the quality and quantity threshold for the acceptance of genetic research on intelligence.
Historically, the central working hypothesis in the neuroscience of human intelligence differences has been that size matters 52,53. Empirical research in this tradition began in the nineteenth ...
Intelligence predicts important life and health outcomes, but the biological mechanisms underlying differences in intelligence are not yet understood. The use of genetically determined metabotypes (GDMs) to understand the role of genetic and environmental factors, and their interactions, in human complex traits has been recently proposed. However, this strategy has not been applied to human ...
University of Manchester. (2011, August 12). Research reveals genetic link to human intelligence. ScienceDaily. Retrieved August 25, 2024 from www.sciencedaily.com / releases / 2011 / 08 ...
The construct of Spearman's g is shown to be a strongly confirmed scientific hypothesis. Genetic and environmental influences are shown to influence g differentially over time, with shared environmental influences predominating early in life, but dissipating to near zero by adulthood. The hypothesis of substantively significant genetic ...
Abstract. This article provides an overview of the biometric and molecular genetic studies of human psychometric intelligence. In the biometric research, special attention is given to the ...
The predictive power of GPSs derived from genome-wide association studies (GWAS) of intelligence has increased in the past 2 years from 1% to 4%. The latest EA GPS, EA3, predicts more than 10% of ...
Note that heritability estimates apply to a sample at a given time; the estimate might be different in other groups, and in the same group at other times. Often, in human studies, only additive genetic factors are considered. Different methods are used to estimate heritability of intelligence (and other phenotypes). They give different estimates.
Genetic factors in human intelligence. Plomin (gene mapping) Compared children who have high IQ and average IQ to see if they have different genes which make them more intelligence. They found a variant of IGF-2 which was more common in children with high IQs it was responsible for a difference of 4 ID points.
Intelligence Is a Polygenic Trait. The latest and largest genetic association study of intelligence to date identified 206 genomic loci and implicated 1,041 genes, adding 191 novel loci and 963 novel genes to previously associated with cognitive ability (Savage et al., 2018 ).
Researchers have previously shown that a person's IQ is highly influenced by genetic factors, and have even identified certain genes that play a role. They've also shown that performance in school has genetic factors. But it's been unclear whether the same genes that influence IQ also influence grades and test scores.
General intelligence is an important human quantitative trait that accounts for much of the variation in diverse cognitive abilities. Individual differences in intelligence are strongly associated ...
Individual differences in intelligence (cognitive abilities) are a prominent aspect of human psychology, and play a substantial role in influencing important life outcomes. Their phenotypic structure—as described by the science of psychometrics—is well understood and well replicated. Approximately half of the variance in a broad range of cognitive abilities is accounted by a general ...
Evolutionary theories of human intelligence that take the chimpanzee mind as a starting point and factor the effects of scaling-up on cognition are grounded in genetic physiology.
Heritability estimates for cognitive ability. The first three estimates are based on correlations among. monozygotic and dizygotic twins ( = 0.75 and = 0.38, respectively) from the report by ...
General intelligence is an important human quantitative trait that accounts for much of the variation in diverse cognitive abilities. Individual differences in intelligence are strongly associated with many important life outcomes, including educational and occupational attainments, income, health and lifespan 1,2.Data from twin and family studies are consistent with a high heritability of ...
Intelligence has long been a subject of fascination and intense study. While it is clear that both environmental and genetic factors play a role in determining intelligence, recent research has shed light on five key genetic findings that have a special significance in understanding the biology of intelligence.