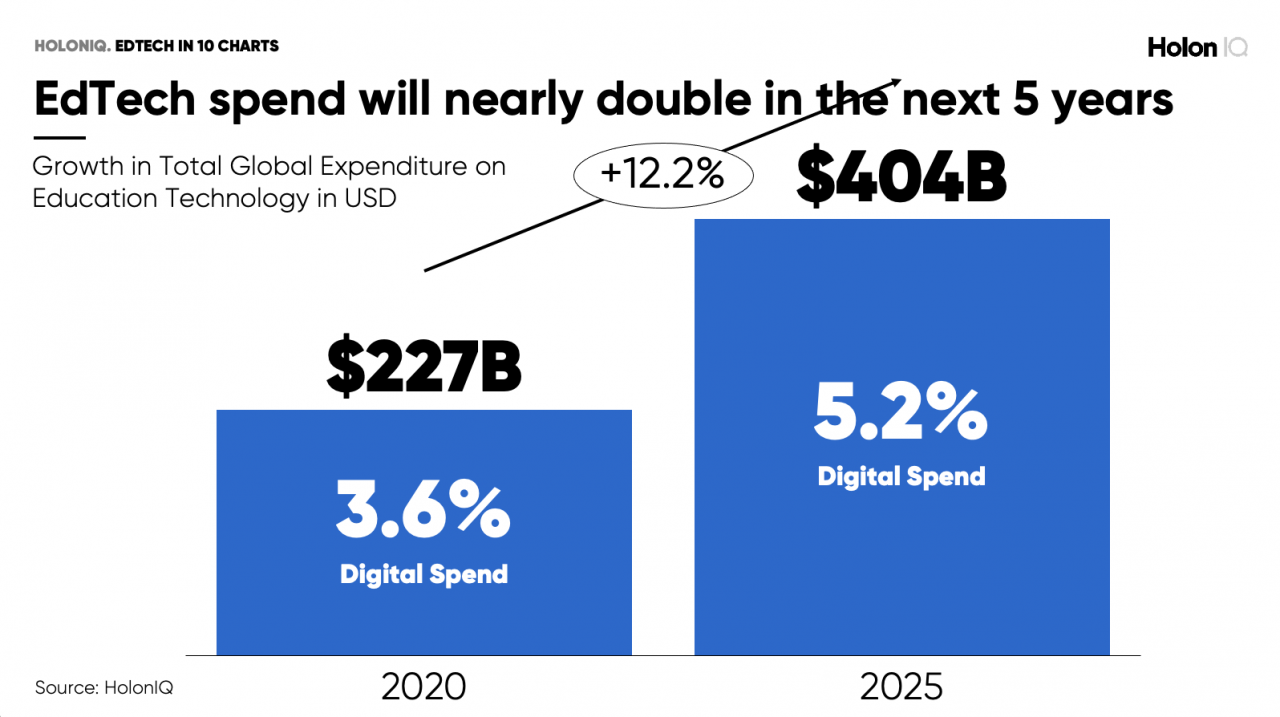

Education Technology in 10 Charts
Everything you need to know about the Global EdTech Market in 10 charts
1. Education will be a $7T+ industry by 2025
Education is one of the world’s single largest industries, making up more than 6% of GDP. We expect total global expenditure from governments, companies and consumers together to reach $7.3T by 2025. Source: HolonIQ

2. UpSkilling an additional 2 billion more learners between now and 2050
By 2050, there will be 2 billion more school, college, university and alternative post-secondary graduates in the world than today, driven primarily by greater participation and population growth. How will current models of education deliver access at the scale, quality and speed required?
3. However, education is starved of capital compared with other sectors.
Innovation requires capital. Governments are struggling to fund education at historic levels, meanwhile schools and institutions are cutting costs and embarking on digital transformation. Education is not drawing on enough private capital to fund the innovation that’s needed. Public-Private Partnerships will be critical to supporting future growth, innovation and access to education.
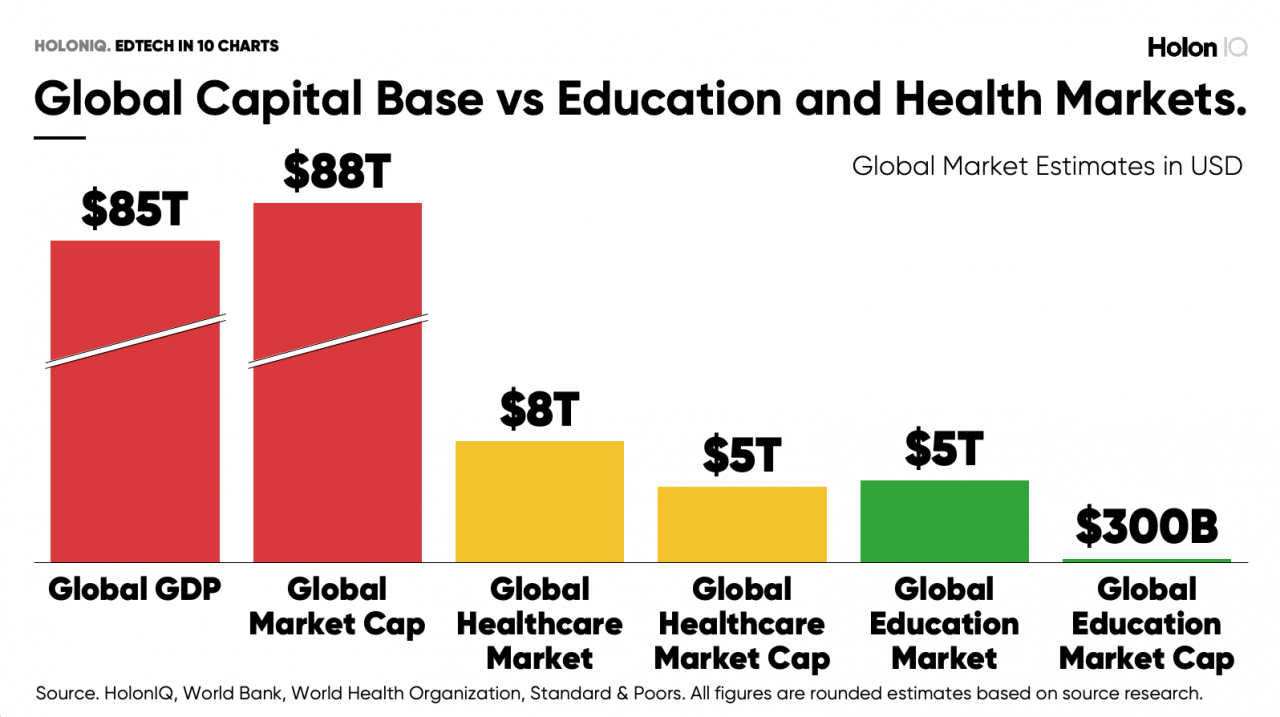
4. Education is grossly under digitized, with less than 4% of global expenditure on tech.
As a sector, education is a digital laggard with less than 4% of overall expenditure allocated to digital, presenting a serious challenge given the scale of what’s to come. The knowledge economy and future skills require massive digital transformation, and, while accelerated through COVID-19, there is still far to go. Source: HolonIQ
5. Digital spend is changing fast, forecast to grow to $404B by 2025.
In 2020, education spent $227B on digital. While we forecast this to grow to $404B by 2025, it is still barely 5% of overall expenditure and considered by many as quite conservative. While the longer term impact of COVID-19 on education models is yet to play out, over the next few years we expect an upswing of spending on digital infrastructure in education and greater spending over the long term in new digital models.

6. Advanced technologies will embed into education delivery and learning processes.
Applications of advanced technology in education and learning will hit their strides by 2025 with AR/VR and Artificial Intelligence becoming increasingly integrated into core education delivery and learning processes. While virtual and simulated training is already becoming mainstream in adult training and upskilling, we are also seeing greater usage of these technologies in formal education settings.
7. Venture Capital investment in EdTech is up 2x on 2018 at $16B in 2020.
Venture Capital investors can see the favourable dynamics of the global education and training market, investing $16B in 2020, up from $8.2B in 2018. This will continue to grow, but is not evenly spread across the globe and weighted heavily towards late-stage mega-rounds.
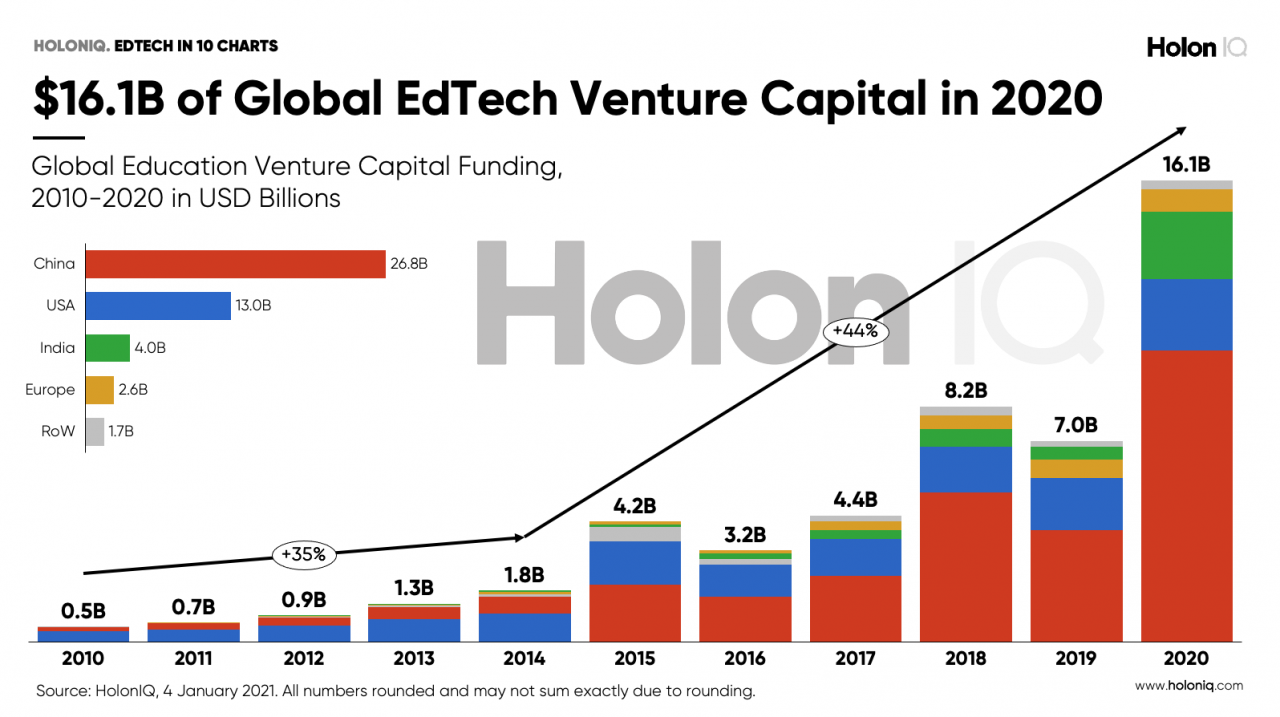
8. China is a key driver of growth in VC investment, making up 60%+ of all Global VC in 2020.
China, with the largest education market in the world, has led education VC investment growth over the past five years. China now makes up over 60% of all Global VC investment in education, the USA 15%, India, 14% and Europe 5% in 2020. While not as large, VC investment in Indian EdTech is worthy of note, growing almost 4x since 2018.Source: HolonIQ
9. VC investment drives IPOs. We expect 100+ education companies with Market Cap’s > $1B by 2025.
Venture Capital investment ultimately drives more exits in the form of public listings. In 2015 there were 10 listed education companies with Market Cap’s > $1B, growing to 30 in 2018 and we expect 100+ publicly listed education companies valued at over $1B by 2025.
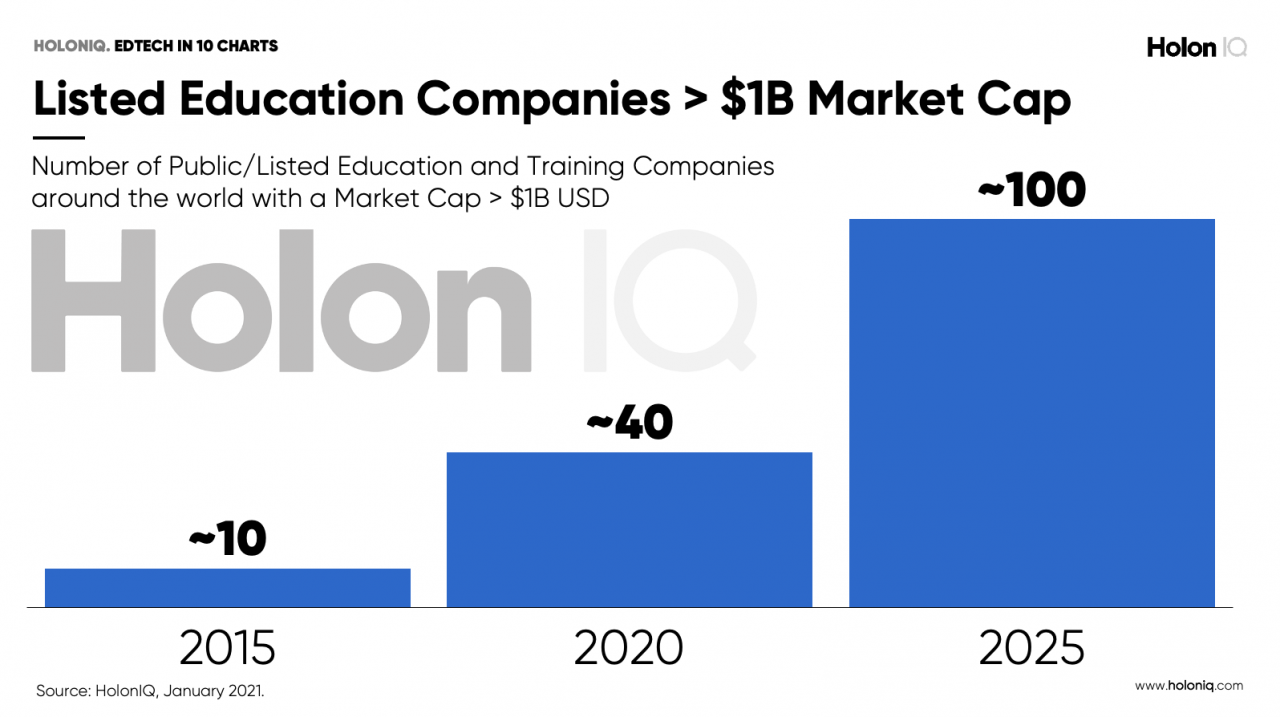
10. However, the devil is in the detail when it comes to education.
Education is a complex system. When it comes to understanding market dynamics and which technologies, business models and parts of the sector are likely to grow or shrink, it pays to understand the nuance. The Global Learning Landscape taxonomy, consisting of 50 clusters of innovation helps to unpack this complexity. HolonIQ maps and monitors each of these 50 market segments through our Global Market Intelligence Platform .
Ready to get started?
Get a personalized walkthrough of HolonIQ's Global Intelligence Platform.
Latest Insights
Global Insights from HolonIQ’s Intelligence Unit.Powered by our Global Impact Intelligence Platform.
August 12, 2024
2024 Australia & New Zealand EdTech 50

5 Strategic Shifts in Higher Education
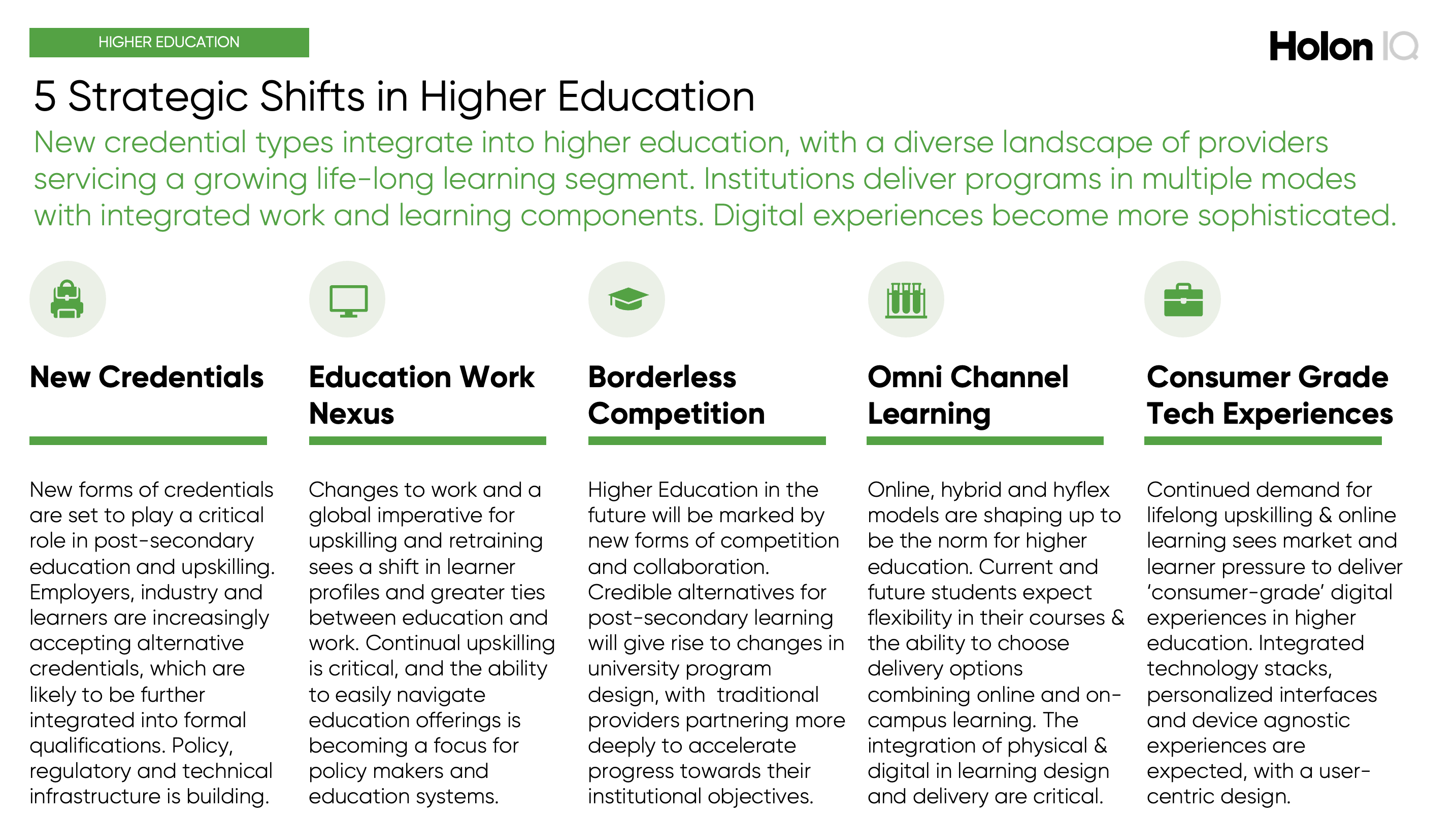
June 17, 2024
2024 Europe EdTech 200
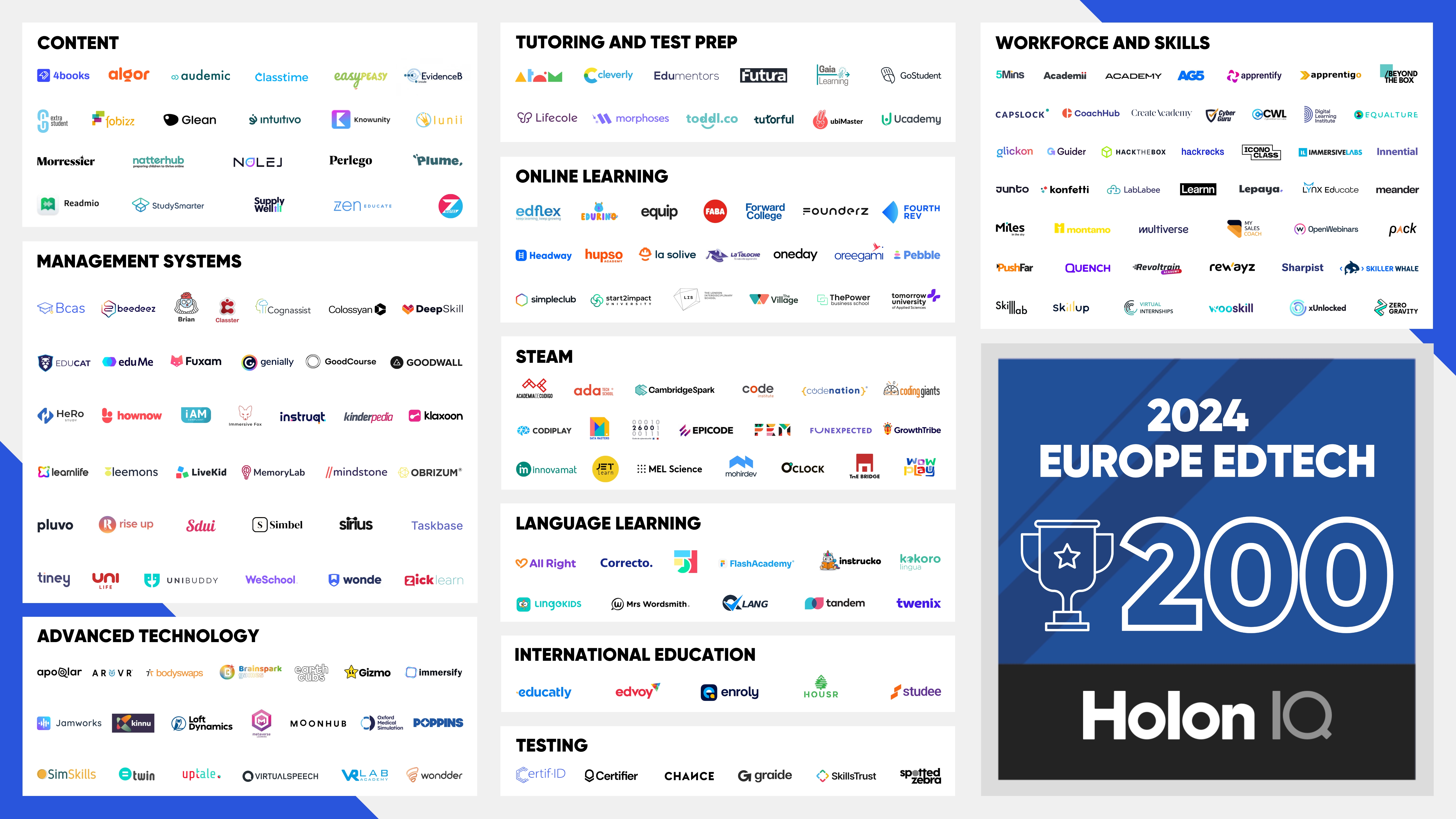
June 5, 2024
Announcing the 2024 Indo-Pacific Climate Tech 100
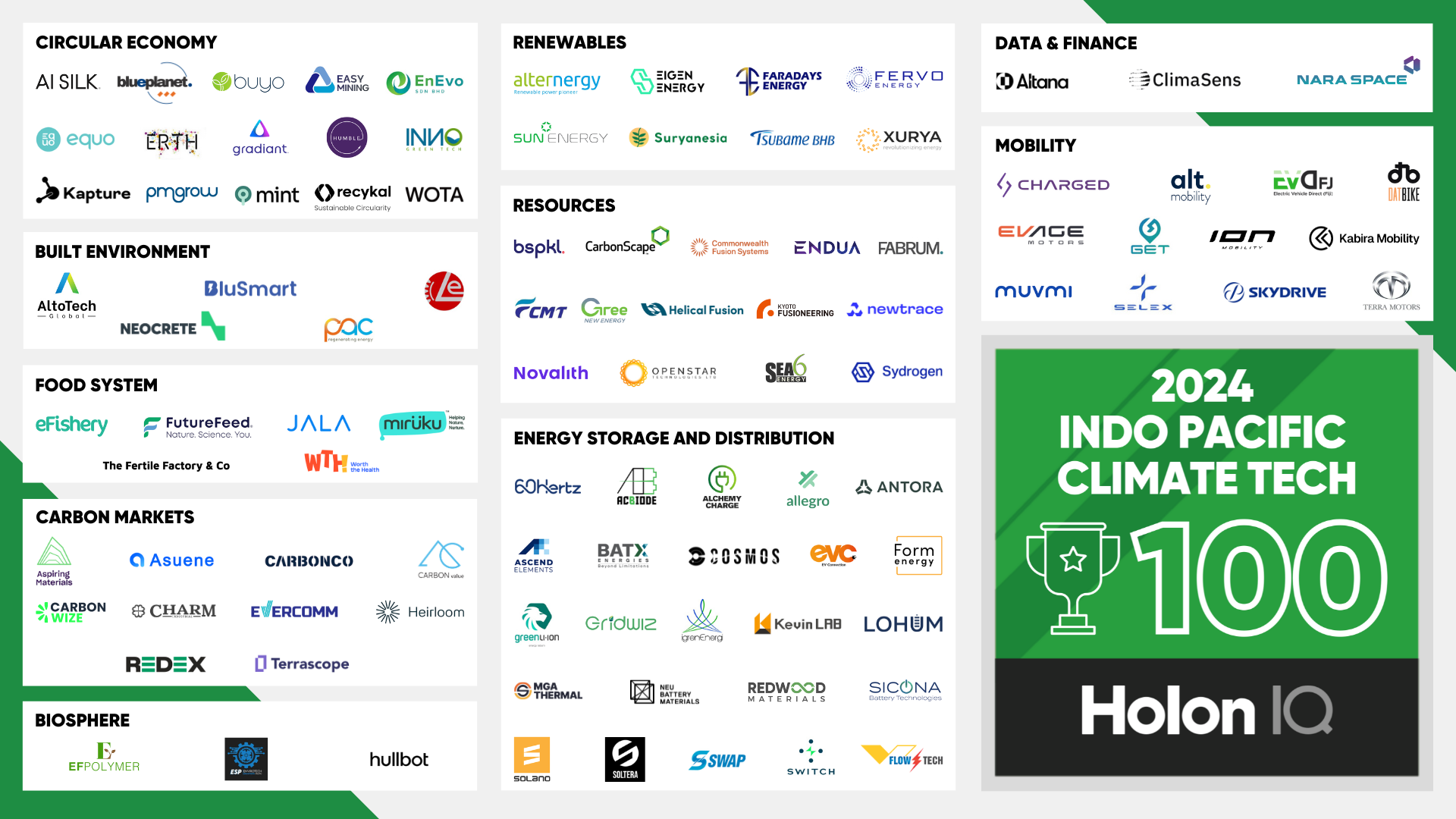
May 23, 2024
2024 Global Student Flows - US Outlook and Forecast Update
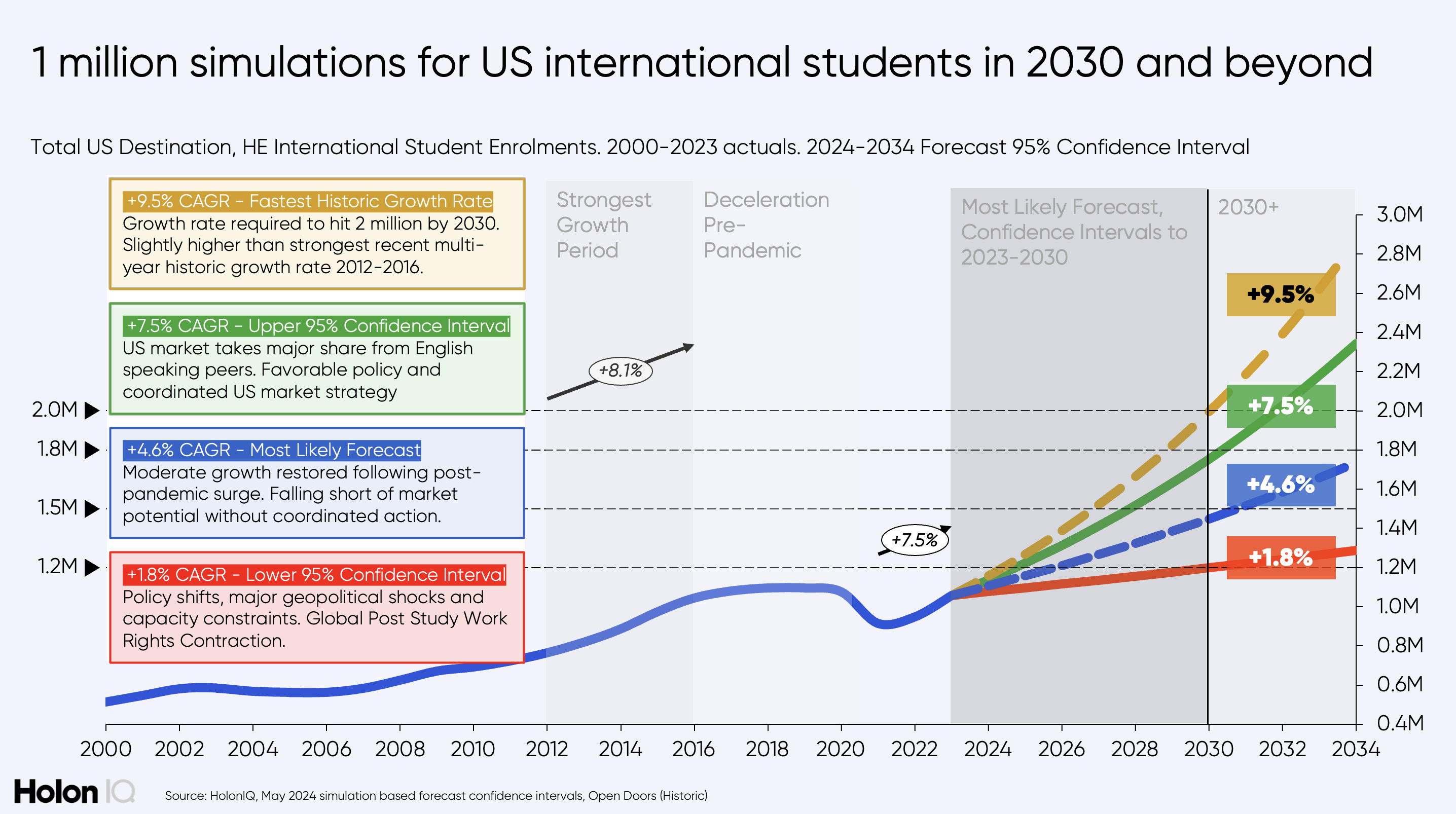
May 17, 2024
2024 Puerto Rico Impact 50
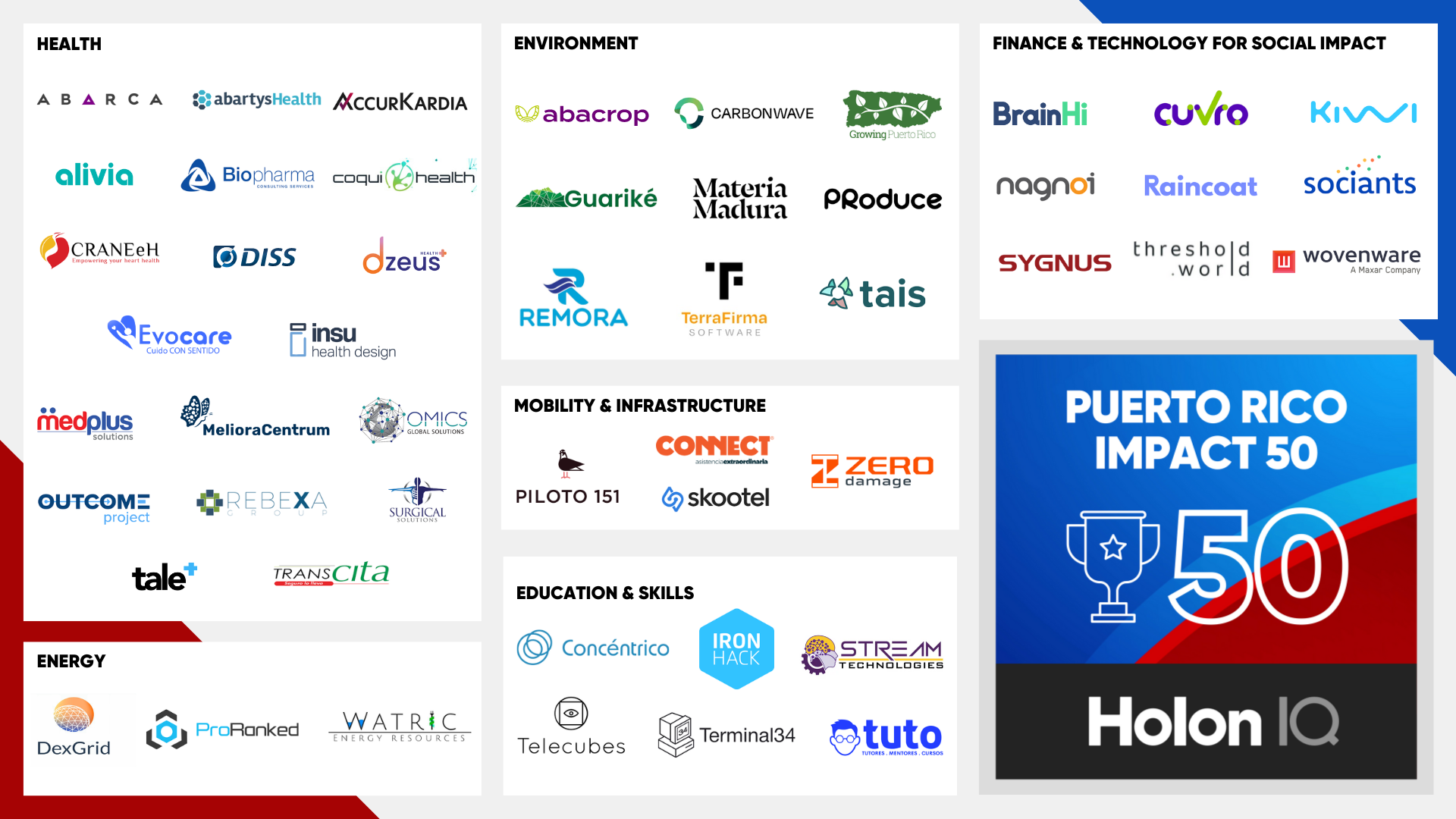
Sign up for our free weekly newsletters
Join 80,000+ free weekly newsletter subscribers.
How Educators Feel About the Impact of Technology, in Charts

- Share article
Most teachers, principals, and district leaders believe that their schools’ recent investments in technology are—at least to some extent—paying off in terms of student academic gains, according to an EdWeek Research Center survey conducted in February.
And well over half of the teachers surveyed say that 1-to-1 computing has allowed them to some extent to focus more on students’ individual academic needs.
But despite those promising data points, the proportion of educators rating the benefits of new education technology as game changing is much smaller. And growing pains remain as education technology hits an inflection point three years after the pandemic kicked off unprecedented investments in new digital learning tools.
Chief among those challenges: Many ed-tech tools are not aligned with federal education requirements, technology’s potential to truly accelerate student learning remains underutilized, and, as the data below show, tech fatigue remains an ongoing concern .
While most teachers, principals, and district leaders say that teachers in their schools or districts are invigorated by technology—it’s a slim majority of 51 percent. Nearly half feel just the opposite—they are worn out by all the tech use in schools.
Below is a more detailed look at some findings about technology use in education from the EdWeek Research Center survey:

Data analysis for this article was provided by the EdWeek Research Center. Learn more about the center’s work.
A version of this article appeared in the April 05, 2023 edition of Education Week as How Educators Feel About the Impact Of Technology, in Charts

Sign Up for EdWeek Tech Leader
Edweek top school jobs.

Sign Up & Sign In

Become an Insider
Sign up today to receive premium content.
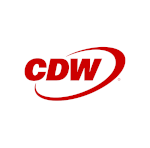
21st-Century Classroom Technology Use is on the Rise (Infographic)

Meghan is an associate editor with EdTech . She enjoys coffee, cats and science fiction TV.
About 63 percent of K–12 teachers use technology in the classroom daily . A new survey from the University of Phoenix College of Education found that daily classroom tech use is up from 55 percent in 2016.
While a whopping 86 percent of educators indicate they use laptops in classrooms the most, the use of other tools is on the rise. About 58 percent of teachers use educational apps, 41 percent use social media and 21 percent use 3D printers .
About 47 percent of teachers say they are embracing social media to collaborate with other teachers .
Even with this increase in tech use — some of it innovative — 25 percent of teachers indicate they are intimidated by students’ knowledge and use of tech.
“This data suggests that many teachers are introducing ed tech cautiously,” says Pamela Roggeman, academic dean for the College of Education, in a press release . “In some cases, they are unfamiliar with certain resources, but more often, they worry that personal devices will become an unwelcome distraction.”

For more on the 21st-century classroom, look at the University of Phoenix infographic below.
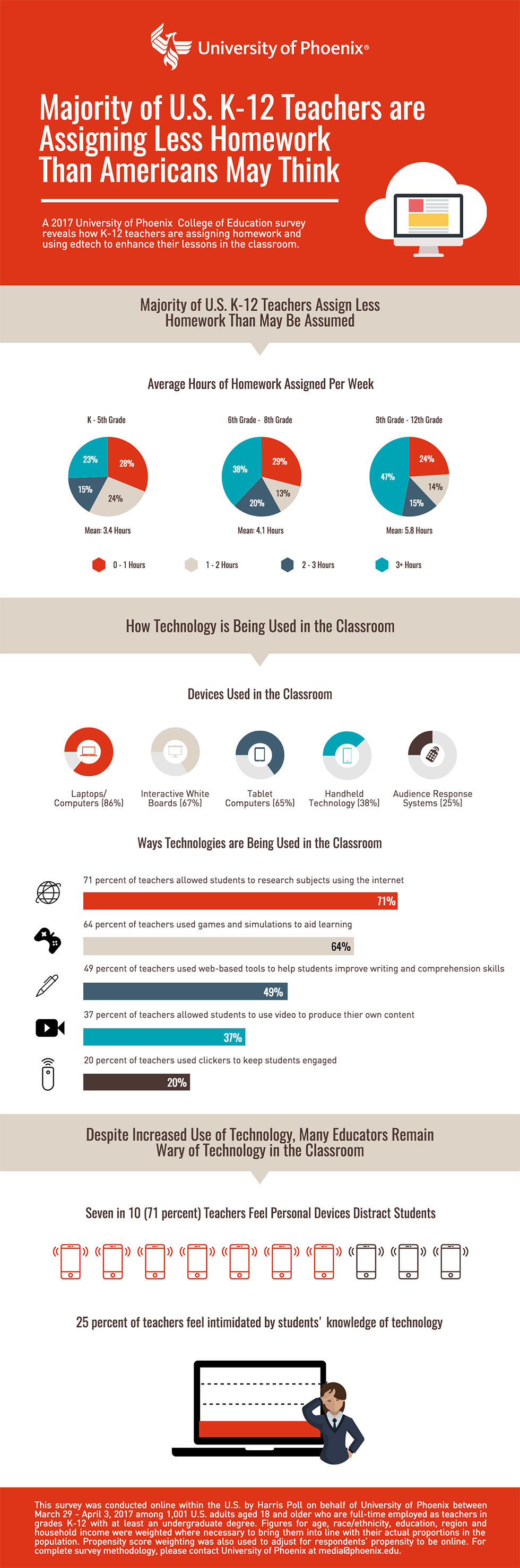
- 3D printing
- Social Media
Related Articles
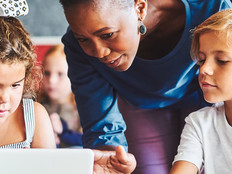
CDW Education Events
Find out what's happening in your area.
Copyright © 2024 CDW LLC 200 N. Milwaukee Avenue , Vernon Hills, IL 60061 Do Not Sell My Personal Information
Suggestions or feedback?
MIT News | Massachusetts Institute of Technology
- Machine learning
- Sustainability
- Black holes
- Classes and programs
Departments
- Aeronautics and Astronautics
- Brain and Cognitive Sciences
- Architecture
- Political Science
- Mechanical Engineering
Centers, Labs, & Programs
- Abdul Latif Jameel Poverty Action Lab (J-PAL)
- Picower Institute for Learning and Memory
- Lincoln Laboratory
- School of Architecture + Planning
- School of Engineering
- School of Humanities, Arts, and Social Sciences
- Sloan School of Management
- School of Science
- MIT Schwarzman College of Computing
What 126 studies say about education technology
Press contact :.
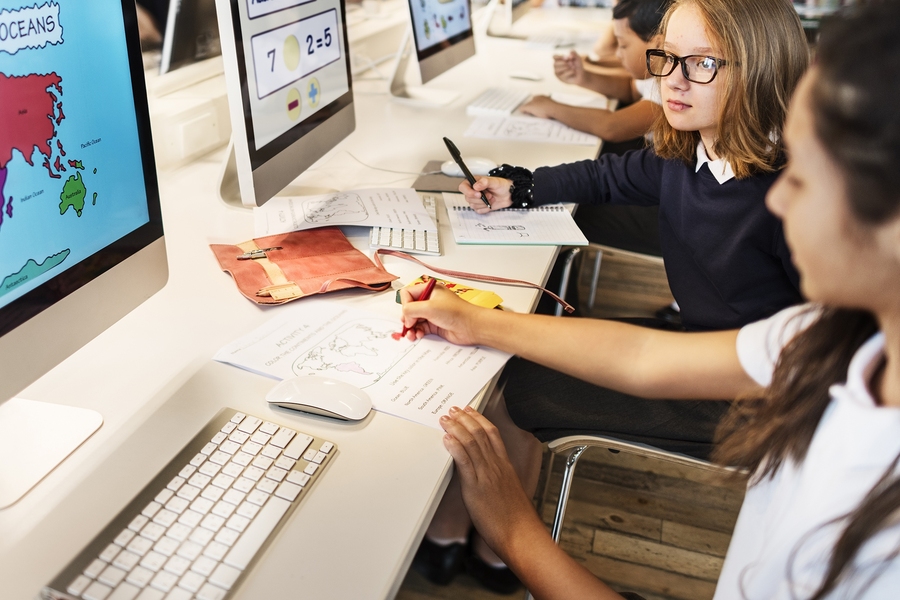
Previous image Next image
In recent years, there has been widespread excitement around the transformative potential of technology in education. In the United States alone, spending on education technology has now exceeded $13 billion . Programs and policies to promote the use of education technology may expand access to quality education, support students’ learning in innovative ways, and help families navigate complex school systems.
However, the rapid development of education technology in the United States is occurring in a context of deep and persistent inequality . Depending on how programs are designed, how they are used, and who can access them, education technologies could alleviate or aggravate existing disparities. To harness education technology’s full potential, education decision-makers, product developers, and funders need to understand the ways in which technology can help — or in some cases hurt — student learning.
To address this need, J-PAL North America recently released a new publication summarizing 126 rigorous evaluations of different uses of education technology. Drawing primarily from research in developed countries, the publication looks at randomized evaluations and regression discontinuity designs across four broad categories: (1) access to technology, (2) computer-assisted learning or educational software, (3) technology-enabled nudges in education, and (4) online learning.
This growing body of evidence suggests some areas of promise and points to four key lessons on education technology.
First, supplying computers and internet alone generally do not improve students’ academic outcomes from kindergarten to 12th grade, but do increase computer usage and improve computer proficiency. Disparities in access to information and communication technologies can exacerbate existing educational inequalities. Students without access at school or at home may struggle to complete web-based assignments and may have a hard time developing digital literacy skills.
Broadly, programs to expand access to technology have been effective at increasing use of computers and improving computer skills. However, computer distribution and internet subsidy programs generally did not improve grades and test scores and in some cases led to adverse impacts on academic achievement. The limited rigorous evidence suggests that distributing computers may have a more direct impact on learning outcomes at the postsecondary level.
Second, educational software (often called “computer-assisted learning”) programs designed to help students develop particular skills have shown enormous promise in improving learning outcomes, particularly in math. Targeting instruction to meet students’ learning levels has been found to be effective in improving student learning, but large class sizes with a wide range of learning levels can make it hard for teachers to personalize instruction. Software has the potential to overcome traditional classroom constraints by customizing activities for each student. Educational software programs range from light-touch homework support tools to more intensive interventions that re-orient the classroom around the use of software.
Most educational software that have been rigorously evaluated help students practice particular skills through personalized tutoring approaches. Computer-assisted learning programs have shown enormous promise in improving academic achievement, especially in math. Of all 30 studies of computer-assisted learning programs, 20 reported statistically significant positive effects, 15 of which were focused on improving math outcomes.
Third, technology-based nudges — such as text message reminders — can have meaningful, if modest, impacts on a variety of education-related outcomes, often at extremely low costs. Low-cost interventions like text message reminders can successfully support students and families at each stage of schooling. Text messages with reminders, tips, goal-setting tools, and encouragement can increase parental engagement in learning activities, such as reading with their elementary-aged children.
Middle and high schools, meanwhile, can help parents support their children by providing families with information about how well their children are doing in school. Colleges can increase application and enrollment rates by leveraging technology to suggest specific action items, streamline financial aid procedures, and/or provide personalized support to high school students.
Online courses are developing a growing presence in education, but the limited experimental evidence suggests that online-only courses lower student academic achievement compared to in-person courses. In four of six studies that directly compared the impact of taking a course online versus in-person only, student performance was lower in the online courses. However, students performed similarly in courses with both in-person and online components compared to traditional face-to-face classes.
The new publication is meant to be a resource for decision-makers interested in learning which uses of education technology go beyond the hype to truly help students learn. At the same time, the publication outlines key open questions about the impacts of education technology, including questions relating to the long-term impacts of education technology and the impacts of education technology on different types of learners.
To help answer these questions, J-PAL North America’s Education, Technology, and Opportunity Initiative is working to build the evidence base on promising uses of education technology by partnering directly with education leaders.
Education leaders are invited to submit letters of interest to partner with J-PAL North America through its Innovation Competition . Anyone interested in learning more about how to apply is encouraged to contact initiative manager Vincent Quan .
Share this news article on:
Related links.
- J-PAL Education, Technology, and Opportunity Initiative
- Education, Technology, and Opportunity Innovation Competition
- Article: "Will Technology Transform Education for the Better?"
- Abdul Latif Jameel Poverty Action Lab
- Department of Economics
Related Topics
- School of Humanities Arts and Social Sciences
- Education, teaching, academics
- Technology and society
- Computer science and technology
Related Articles
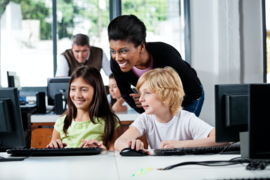
J-PAL North America calls for proposals from education leaders
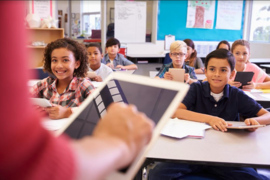
J-PAL North America’s Education, Technology, and Opportunity Innovation Competition announces inaugural partners
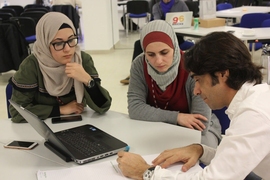
New learning opportunities for displaced persons
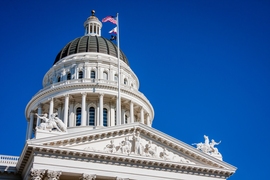
J-PAL North America announces new partnerships with three state and local governments
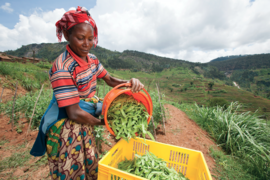
A new way to measure women’s and girls’ empowerment in impact evaluations
Previous item Next item
More MIT News

3 Questions: From the bench to the battlefield
Read full story →
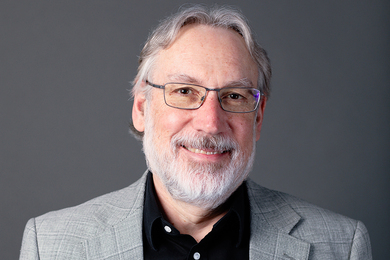
Duane Boning named vice provost for international activities

Q&A: Undergraduate admissions in the wake of the 2023 Supreme Court ruling
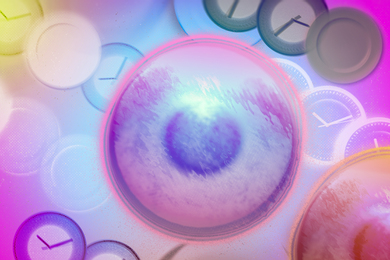
Study reveals the benefits and downside of fasting
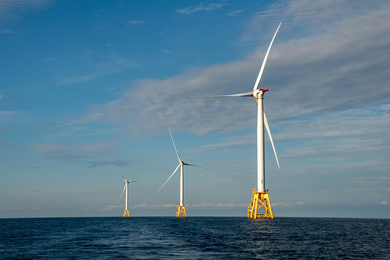
MIT engineers’ new theory could improve the design and operation of wind farms

Engineering and matters of the heart
- More news on MIT News homepage →
Massachusetts Institute of Technology 77 Massachusetts Avenue, Cambridge, MA, USA
- Map (opens in new window)
- Events (opens in new window)
- People (opens in new window)
- Careers (opens in new window)
- Accessibility
- Social Media Hub
- MIT on Facebook
- MIT on YouTube
- MIT on Instagram
Global Education Monitoring Report
Technology in education
As recognised in the Incheon Declaration, the achievement of SDG 4 is dependent on opportunities and challenges posed by technology, a relationship that was strengthened by the onset of the COVID-19 pandemic. Technology appears in six out of the ten targets in the fourth Sustainable Development goal on education. These references recognize that technology affects education through five distinct channels, as input, means of delivery, skill, tool for planning, and providing a social and cultural context.
There are often bitter divisions in how the role of technology is viewed, however. These divisions are widening as the technology is evolving at breakneck speed. The 2023 GEM Report on technology and education explores these debates, examining education challenges to which appropriate use of technology can offer solutions (access, equity and inclusion; quality; technology advancement; system management), while recognizing that many solutions proposed may also be detrimental.
The report also explores three system-wide conditions (access to technology, governance regulation, and teacher preparation) that need to be met for any technology in education to reach its full potential. It provides the mid-term assessment of progress towards SDG 4 , which was summarized in a brochure and promoted at the 2023 SDG Summit.
The 2023 GEM Report and 200 PEER country profiles on technology and education were launched on 26 July. A recording of the global launch event can be watched here and a south-south dialogue between Ministers of education in Latin America and Africa here .
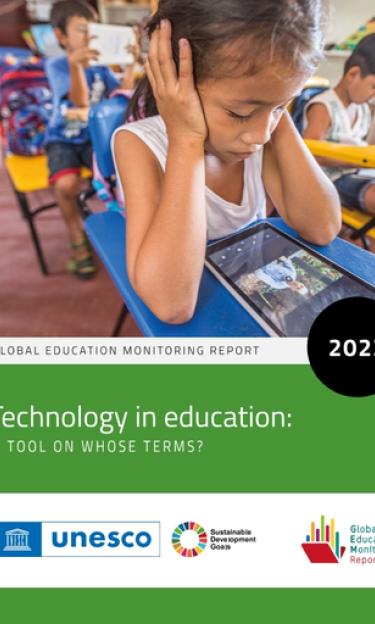
Background material

Watch the launch event
Consultations

The GEM Report is partnering with Restless Development to mobilize youth globally to inform the development of the 2023 Youth Report, exploring how technology can address various education challenges.

The GEM Report ran a consultation process to collect feedback and evidence on the proposed lines of research of the 2023 concept note.

Related resources
on technology and education
in quality and school infrastructure

Related content
Monitoring SDG 4: Quality
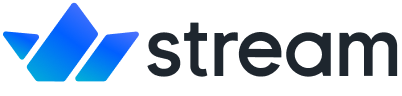
36 EdTech Statistics & Facts: The State of Technology in Education

To understand the future of technology in education, we need to know what’s happening now. We’ve seen recent changes in the industry that seem to be paving the way for a new wave of online learning for entrepreneurs, edtech developers , instructors, and learners alike. A deep dive into these changes can show what we might expect in the coming years.
Here, we’ll look at facts: What are the current benchmarks and what has changed in technology and education?
Editor’s Choice: Top Technology in Education Statistics
Edtech industry trends, how technology impacts educational outcomes, types of technology used in the classroom, the state of education technology jobs, edtech market size statistics, final takeaway: what can we make of all this.

In the United States, students with more than 60 minutes per week of device use achieved higher academic results, a metric that varies country to country ( McKinsey ).
81% of US educators stated that their ability to use EdTech improved during school building closures in response to COVID-19 in 2020 ( EdWeek ).
In 2015, 49% of students worldwide reported that they had taken an online course in the past 12 months. A year later, 81% of college students believed that digital learning technology, including virtual classroom chat , helped them boost their grades ( Statista ).
As of April 2020, 73% of US households always had access to the internet for online learning ( USAFacts ).
As of Summer 2020, 93% of US teachers reported conducting at least some of their teaching online from home ( EdWeek ).
65% of faculty support the use of open educational resources in teaching ( Statista ).
In 2015, 56% of students worldwide reported the desire for instructors to enable them to use their devices in the classroom ( Statista ).
As of 2015, 79% of potential learners in the United States were unaware of massive open online courses ( Statista ).
In 2018, 74% of US teachers reported that their students did not have district-provided computers and estimated that as many as 75% or more students had computer access at home. Yet, only 21% of these teachers believed that they were very knowledgeable about the topic ( NCES ).
19% of learners who earned a competency badge from a massive online open course professed that they would use the badge on their resume ( Statista ).
19% and 28% of US teachers respectively report that they assign technology-based homework often or at least sometimes ( NCES ).
As of April 2020, over 9 million children still lacked internet access from home ( USAFacts ).
Families in rural areas, as of April 2020, were 18% less likely than those in other geographic locations to have internet access ( USAFacts ).
58% of education professionals now have a more positive opinion of EdTech in response to 2020’s COVID-19 school closures ( EdWeek ).
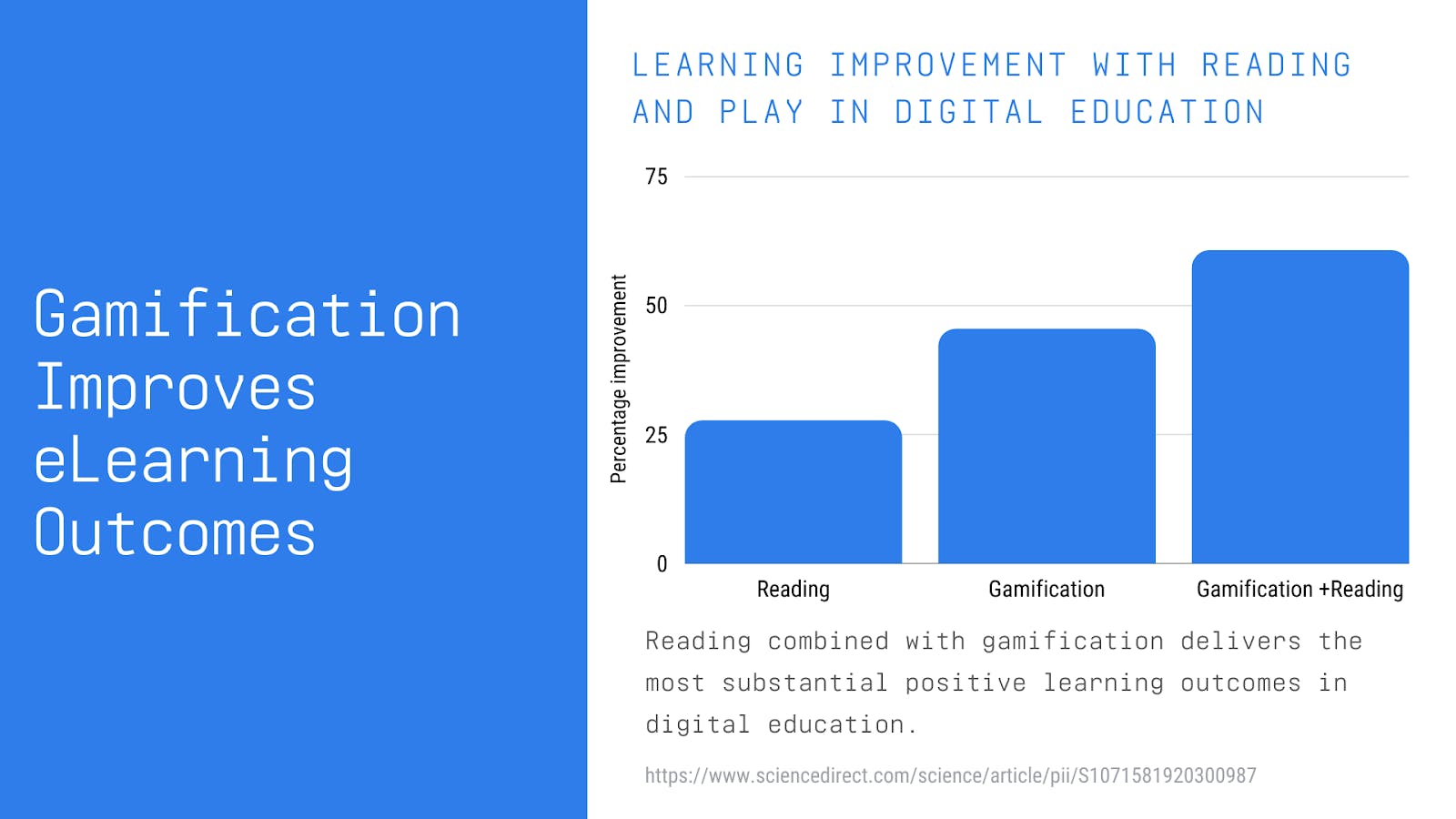
In North America, data projectors have a more positive impact on in-person educational outcomes than internet-connected computers, laptops, or tablets ( McKinsey ).
12% and 32% of US teachers respectively believe that smartphones are very useful or somewhat useful for school assignments ( NCES ).
When teachers alone use devices in the classroom, the educational outcomes are higher than when students alone use them ( McKinsey ).
Gamification alone can improve outcomes by 45.45%, and 60.67% when combined with reading ( IJCS ).
Challenge-based gamification in e-learning can improve educational outcomes by as much as 89.45% ( IJCS ).
53% of teachers believe that students’ increased access to individual computers makes teaching easier, especially with school closures ( EdWeek ).
71% of US students claim to use laptops in the classroom versus the global average of 37% ( McKinsey ).
In 2015, 56% of US students reported using a laptop at least once a week in the classroom ( Statista ).
In 2016, the most common tech used in PreK-12 classrooms were online educational videos, educational apps or software, and websites for research followed by games that focused on specific content or skills, eBooks, simulations, and e-magazines ( Statista ).
As of 2018, 26% of US teachers reported that their students used school-provided computers in the classroom, 40% reported that some students are not able to take them home, and only 8% reported that the school district provided hot spots for students to take home ( NCES ).
Students use mobile technology to seek help and engage in computer-supported collaborative learning with a positive educational impact in literacy, mathematics, science, art, and history ( CEP ).
2.5% of US K-12 students, as of April 2020, used district-provided internet to access online learning from home ( USAFacts ).
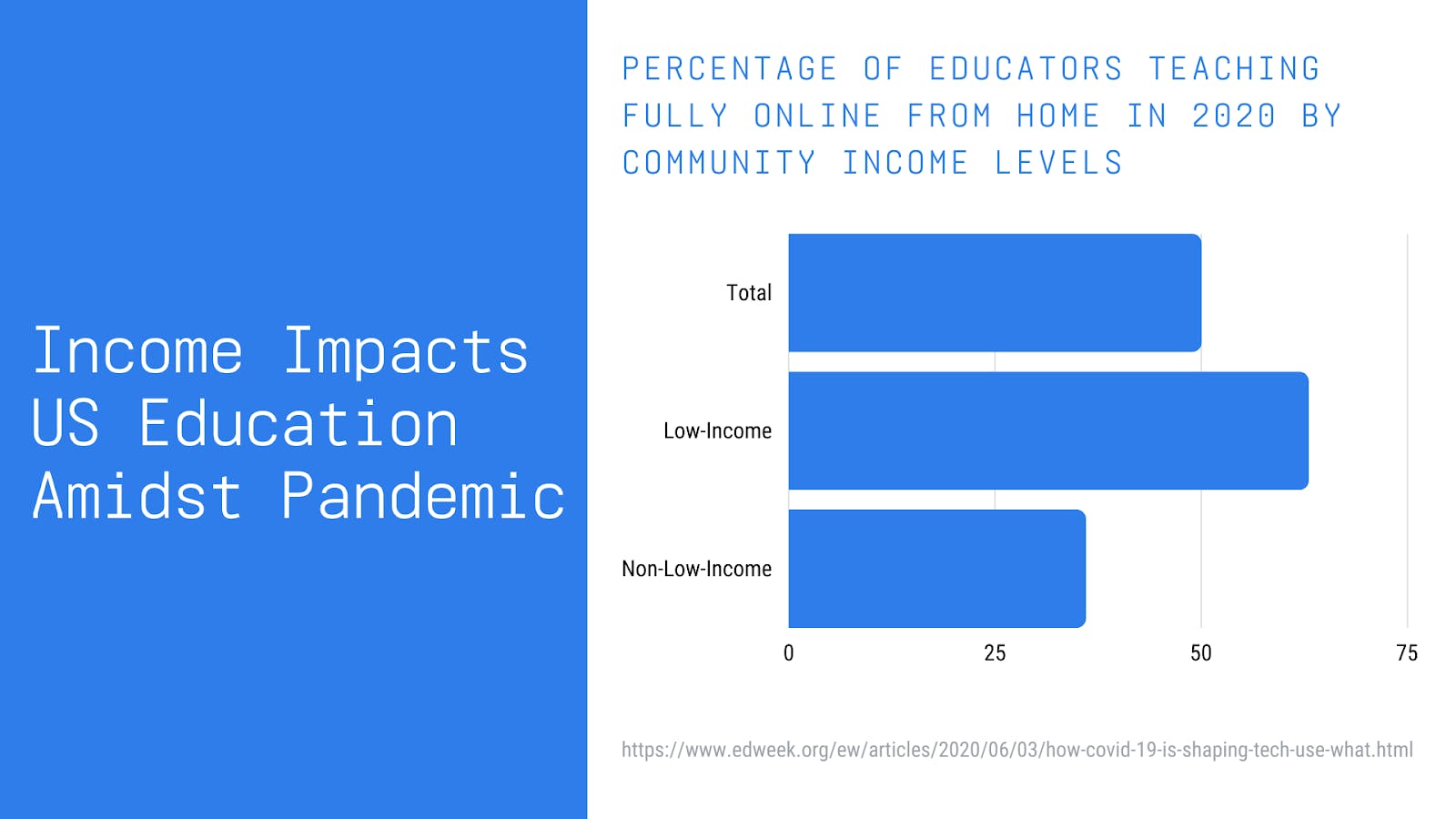
In 2020, traditional medical educators reduced teaching hours as they were redeployed into clinical practice in response to COVID-19, leading to increased awareness and adoption of the available education technologies ( MedEdPublish ).
As of Summer 2020, 50% of US educators reported teaching all of their classes online from home, 63% at schools with fewer low-income students, and 36% at schools with more low-income students ( EdWeek ).
Job growth for instructional coordinators is expected to grow 6% more rapidly than average between 2019 and 2029 ( BLS ).
9 out of 10 teachers reported that they spent more time troubleshooting technology in response to the COVID-19 school closures in 2020 ( EdWeek ).
Some of the best entry-level jobs for EdTech majors are education technician, computer teacher, and technology coordinator because these career paths can eventually lead to jobs as chief learning officer, technical education teacher, and senior support analyst ( Zippa ).
In 2016, self-paced elearning industry made up $46.67 billion of the global market share and, by 2022, that number is projected to exceed $243 billion ( Statista ).
As of August 2020, the global education and training market is expected to reach $7.3 trillion by 2025 ( Holon IQ ).
In 2019, the global education technology market was estimated at 76.4 billion ( Grandview ).
The global education technology market could reach $404 billion by 2025, as of August 2020 ( Holon IQ ).
EdTech is expected to have a compound annual growth rate (CAGR) of 18.1% year-over-year through 2027 ( Grandview ).
EdTech has seen many changes in the past several years, more so in the last 12 months. The greatest challenge, it seems, may be to find ways to increase home internet access for a minority of K-12 learners. Still, whether you’re a developer, entrepreneur, or you work the education system, you can expect the industry to continue to grow in the direction it is currently headed... with an even stronger focus on technology.
If you want a way to improve your e-learning app, learning management system or individual digital classroom communication with a customized messaging experience, learn more about how you can leverage our chat APIs and SDKs .
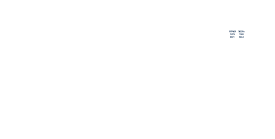
Global Education
By: Hannah Ritchie , Veronika Samborska , Natasha Ahuja , Esteban Ortiz-Ospina and Max Roser
A good education offers individuals the opportunity to lead richer, more interesting lives. At a societal level, it creates opportunities for humanity to solve its pressing problems.
The world has gone through a dramatic transition over the last few centuries, from one where very few had any basic education to one where most people do. This is not only reflected in the inputs to education – enrollment and attendance – but also in outcomes, where literacy rates have greatly improved.
Getting children into school is also not enough. What they learn matters. There are large differences in educational outcomes : in low-income countries, most children cannot read by the end of primary school. These inequalities in education exacerbate poverty and existing inequalities in global incomes .
On this page, you can find all of our writing and data on global education.
Key insights on Global Education
The world has made substantial progress in increasing basic levels of education.
Access to education is now seen as a fundamental right – in many cases, it’s the government’s duty to provide it.
But formal education is a very recent phenomenon. In the chart, we see the share of the adult population – those older than 15 – that has received some basic education and those who haven’t.
In the early 1800s, fewer than 1 in 5 adults had some basic education. Education was a luxury; in all places, it was only available to a small elite.
But you can see that this share has grown dramatically, such that this ratio is now reversed. Less than 1 in 5 adults has not received any formal education.
This is reflected in literacy data , too: 200 years ago, very few could read and write. Now most adults have basic literacy skills.
What you should know about this data
- Basic education is defined as receiving some kind of formal primary, secondary, or tertiary (post-secondary) education.
- This indicator does not tell us how long a person received formal education. They could have received a full program of schooling, or may only have been in attendance for a short period. To account for such differences, researchers measure the mean years of schooling or the expected years of schooling .
Despite being in school, many children learn very little
International statistics often focus on attendance as the marker of educational progress.
However, being in school does not guarantee that a child receives high-quality education. In fact, in many countries, the data shows that children learn very little.
Just half – 48% – of the world’s children can read with comprehension by the end of primary school. It’s based on data collected over a 9-year period, with 2016 as the average year of collection.
This is shown in the chart, where we plot averages across countries with different income levels. 1
The situation in low-income countries is incredibly worrying, with 90% of children unable to read by that age.
This can be improved – even among high-income countries. The best-performing countries have rates as low as 2%. That’s more than four times lower than the average across high-income countries.
Making sure that every child gets to go to school is essential. But the world also needs to focus on what children learn once they’re in the classroom.
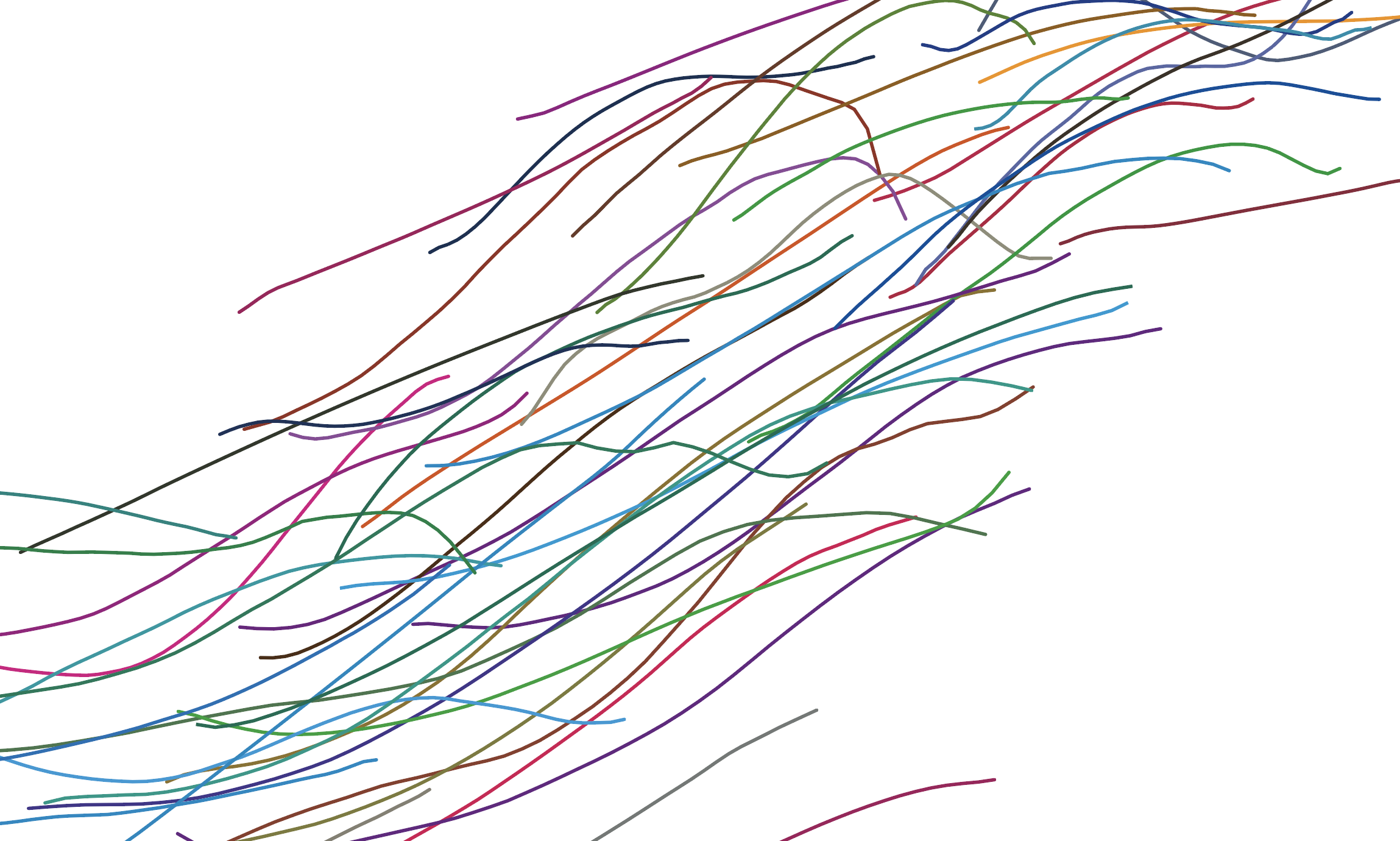
Millions of children learn only very little. How can the world provide a better education to the next generation?
Research suggests that many children – especially in the world’s poorest countries – learn only very little in school. What can we do to improve this?
- This data does not capture total literacy over someone’s lifetime. Many children will learn to read eventually, even if they cannot read by the end of primary school. However, this means they are in a constant state of “catching up” and will leave formal education far behind where they could be.
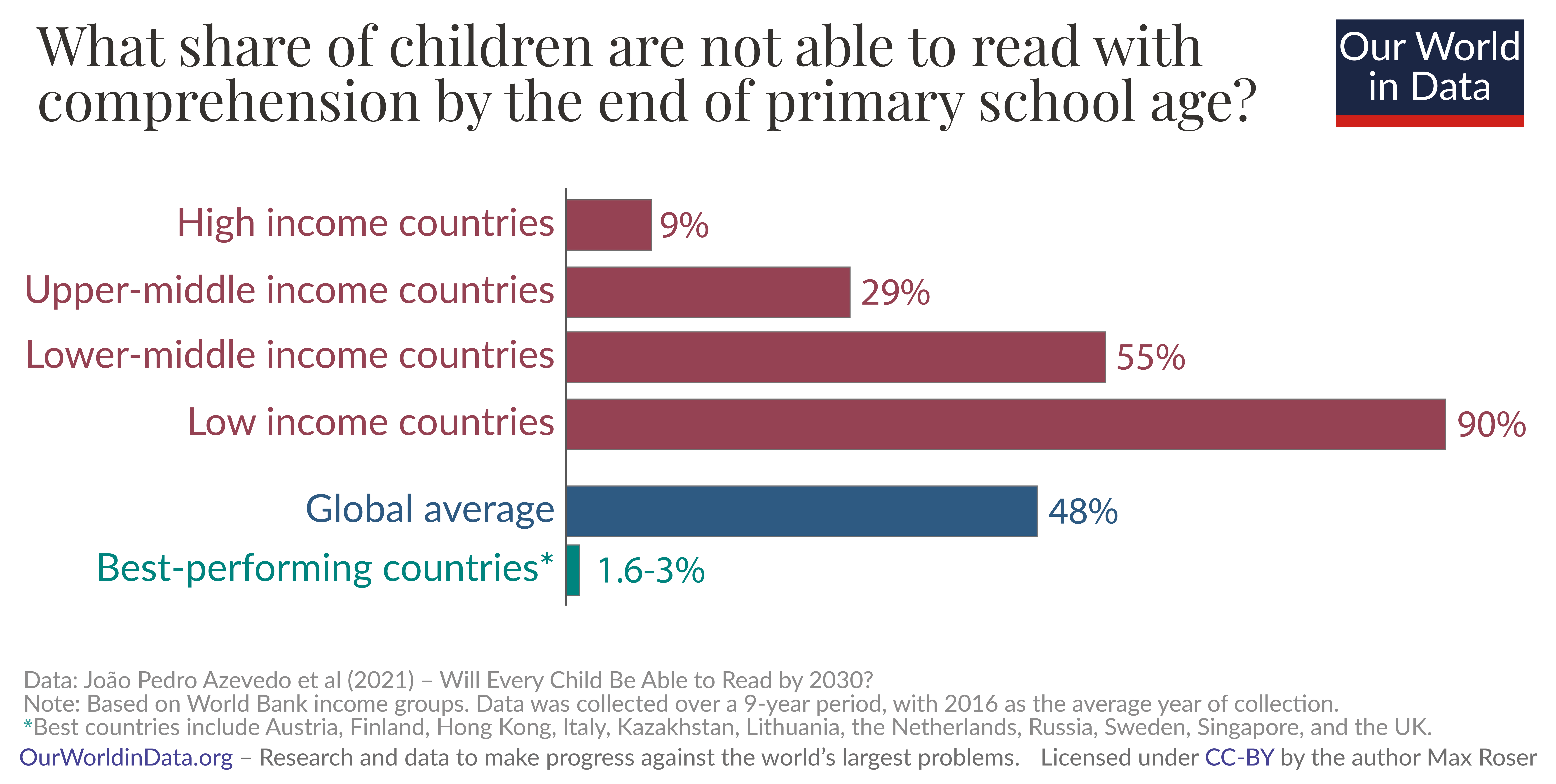
Children across the world receive very different amounts of quality learning
There are still significant inequalities in the amount of education children get across the world.
This can be measured as the total number of years that children spend in school. However, researchers can also adjust for the quality of education to estimate how many years of quality learning they receive. This is done using an indicator called “learning-adjusted years of schooling”.
On the map, you see vast differences across the world.
In many of the world’s poorest countries, children receive less than three years of learning-adjusted schooling. In most rich countries, this is more than 10 years.
Across most countries in South Asia and Sub-Saharan Africa – where the largest share of children live – the average years of quality schooling are less than 7.
- Learning-adjusted years of schooling merge the quantity and quality of education into one metric, accounting for the fact that similar durations of schooling can yield different learning outcomes.
- Learning-adjusted years is computed by adjusting the expected years of school based on the quality of learning, as measured by the harmonized test scores from various international student achievement testing programs. The adjustment involves multiplying the expected years of school by the ratio of the most recent harmonized test score to 625. Here, 625 signifies advanced attainment on the TIMSS (Trends in International Mathematics and Science Study) test, with 300 representing minimal attainment. These scores are measured in TIMSS-equivalent units.
Hundreds of millions of children worldwide do not go to school
While most children worldwide get the opportunity to go to school, hundreds of millions still don’t.
In the chart, we see the number of children who aren’t in school across primary and secondary education.
This number was around 244 million in 2023.
Many children who attend primary school drop out and do not attend secondary school. That means many more children or adolescents are missing from secondary school than primary education.
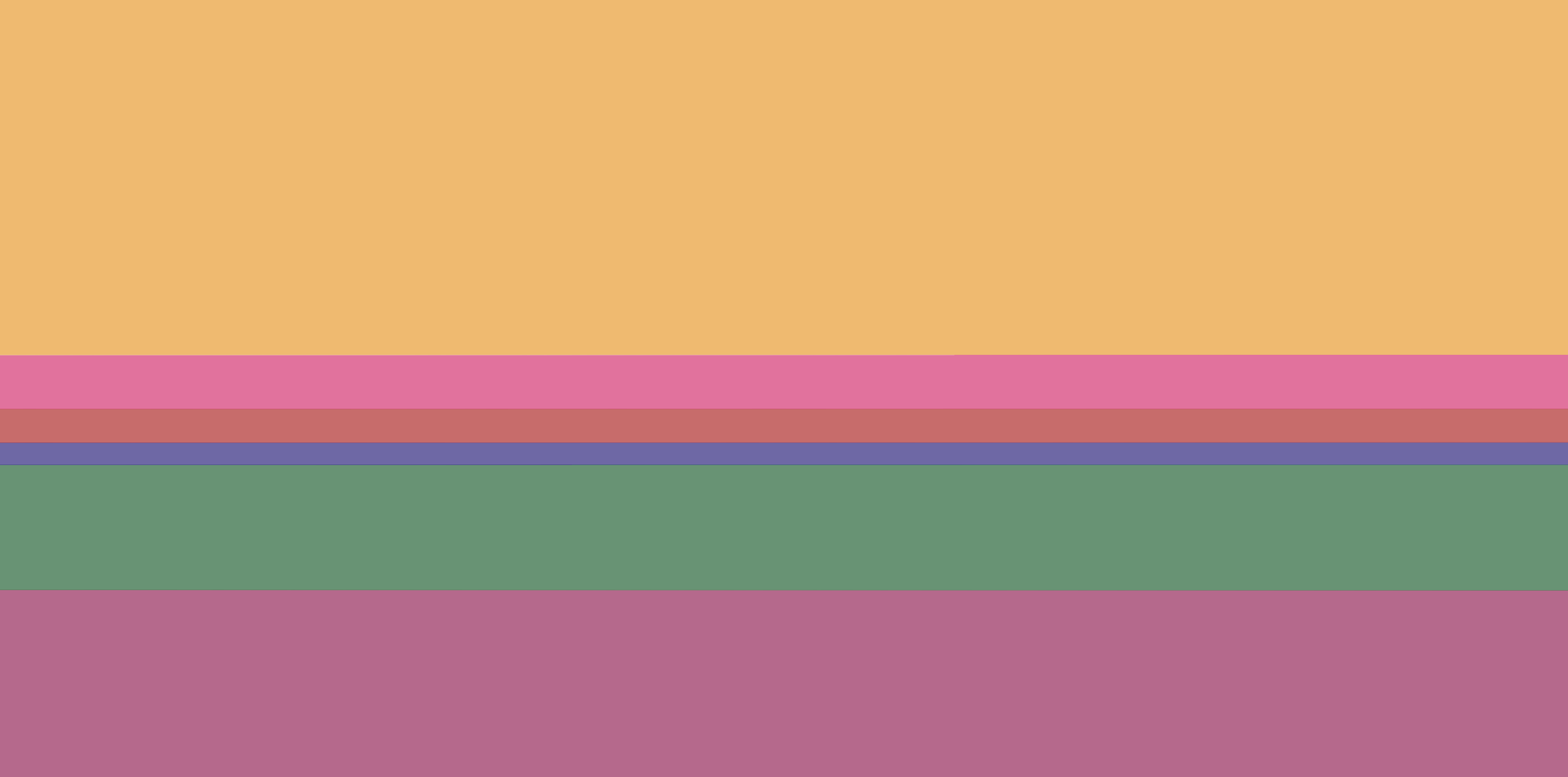
Access to basic education: almost 60 million children of primary school age are not in school
The world has made a lot of progress in recent generations, but millions of children are still not in school.

The gender gap in school attendance has closed across most of the world
Globally, until recently, boys were more likely to attend school than girls. The world has focused on closing this gap to ensure every child gets the opportunity to go to school.
Today, these gender gaps have largely disappeared. In the chart, we see the difference in the global enrollment rates for primary, secondary, and tertiary (post-secondary) education. The share of children who complete primary school is also shown.
We see these lines converging over time, and recently they met: rates between boys and girls are the same.
For tertiary education, young women are now more likely than young men to be enrolled.
While the differences are small globally, there are some countries where the differences are still large: girls in Afghanistan, for example, are much less likely to go to school than boys.
Research & Writing
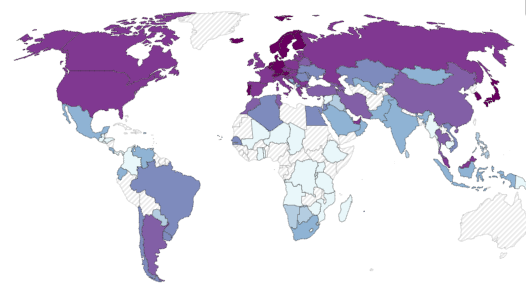
Talent is everywhere, opportunity is not. We are all losing out because of this.
Access to basic education: almost 60 million children of primary school age are not in school, interactive charts on global education.
This data comes from a paper by João Pedro Azevedo et al.
João Pedro Azevedo, Diana Goldemberg, Silvia Montoya, Reema Nayar, Halsey Rogers, Jaime Saavedra, Brian William Stacy (2021) – “ Will Every Child Be Able to Read by 2030? Why Eliminating Learning Poverty Will Be Harder Than You Think, and What to Do About It .” World Bank Policy Research Working Paper 9588, March 2021.
Cite this work
Our articles and data visualizations rely on work from many different people and organizations. When citing this topic page, please also cite the underlying data sources. This topic page can be cited as:
BibTeX citation
Reuse this work freely
All visualizations, data, and code produced by Our World in Data are completely open access under the Creative Commons BY license . You have the permission to use, distribute, and reproduce these in any medium, provided the source and authors are credited.
The data produced by third parties and made available by Our World in Data is subject to the license terms from the original third-party authors. We will always indicate the original source of the data in our documentation, so you should always check the license of any such third-party data before use and redistribution.
All of our charts can be embedded in any site.
Our World in Data is free and accessible for everyone.
Help us do this work by making a donation.
EdTech usage during the pandemic: A story in four graphs

- Ravi Gurumurthy
About Nesta
Nesta is an innovation foundation. For us, innovation means turning bold ideas into reality and changing lives for the better. We use our expertise, skills and funding in areas where there are big challenges facing society.
As schools have been disrupted by the COVID-19 pandemic over the last year, reliance on education technology (EdTech) has increased. For many, it’s been a lifeline - helping with remote teaching and assessment, or connecting children with friends and support - but not all children’s experience of the pandemic and remote learning have been equal.
As part of a wider partnership with the Department for Education to support more effective use of technology, Nesta and SchoolDash have collaborated to analyse usage data in England from three EdTech industry partners, spanning from January 2020 (before the first national school closures) to March 2021. We set out to understand more about how children’s experience of the pandemic and EdTech have varied, particularly for more disadvantaged children. The analysis tells a story about EdTech during the pandemic in four graphs:
- EdTech use has increased, but we can see signs of ‘remote learning fatigue’ too.
- Schools with more children eligible for free school meals (FSM) were slower to adopt these tools, although they had caught up by the second nationwide school closures in Spring 2021 .
- Trends in device-type data suggest the ‘device disadvantage gap’ (the availability of laptops or desktop PCs to access EdTech platforms) has narrowed.
- However, the ‘engagement gap’ between schools with more children eligible for FSMs and other categories of schools looks more persistent.
A note on the analysis
Data from three different products has been explored. In order to respect their confidentiality, they are not named in this report - but we are hugely grateful to them for sharing this data with us to further our understanding of EdTech during the pandemic. This analysis only includes data from England, and so any references to schools closing/reopening refers to lockdown measures in England specifically. All three tools are used mainly in primary schools, but have the following broad characteristics:
- Tool 1: A maths learning platform
- Tool 2: A platform accessed by parents and children to improve writing
- Tool 3: A platform to support parental engagement.
Data for Tool 3 is not included within the visualisations below, as we only had access to data from a smaller sample of schools, covering a shorter period. Where analysis of this data does/does not support findings of wider analysis this is indicated in the text.
1. As we might expect, EdTech use has increased – but we can see signs of ‘remote learning fatigue’ too
Use of technology increased significantly as COVID-19 caused partial school closures. Figure 1 shows a pattern where each new national school closure is marked by a spike in activity (teacher sign-ups were over 10 and four times higher at the start of the March 2020 closures than the pre-closures average for tools 1 and 2 respectively). This supports previous analysis of four EdTech platforms which saw increases in usage of between 2 and 7 times pre-closures average .
Figure 1: Relative weekly usage of Tool 1 and 2
Image Description
Each spike in activity is followed by a slow decline - both in the rate of new users, but also measures of activity such as ‘challenges set’, ‘challenges done’ and ‘lessons saved’. Focus groups carried out with users during the Spring Term 2021 referenced ‘remote learning fatigue’ among pupils and parents, which might account for some of this decline in use. Despite this, usage remains higher than pre-COVID averages for Tool 1 across the year, and higher for Tool 2 apart from during the Autumn Term 2020. This trend is reflected in the data available from Tool 3, where the total number of parent and teacher logins declined during January and February 2021.
2. A closer look at who is using platforms shows that at the beginning of the pandemic relative adoption of these tools was lower in schools with more children eligible for free school meals (FSM). However, by the second nationwide school closures in Spring 2021, adoption had caught up with other categories of schools.
We can gain an overview of relative adoption in different categories of schools by measuring teacher-facing activity, such as ‘challenges set’ and ‘lessons saved’. Comparing teacher-facing activity across schools with different proportions of children eligible for free school meals (FSM) reveals changes over time in who is likely to be using Tools 1 and 2 [Figure 2].
Figure 2. : Relative adoption in schools by deprivation level
If teacher activity was evenly distributed across all children, we would expect to see activity in High FSM schools account for around 11 per cent of total teacher activity (the percentage of all children in High FSM schools). Instead, we see teacher activity in High FSM schools move from being under-represented during the first school closures in Spring 2020 (8.2 per cent and 4 per cent of teacher usage in mid-April 2020 for Tools 1 and 2 respectively), to over-represented by the second school closures in Spring 2021 (16.6 per cent and 16.3 per cent in early February 2021 for Tools 1 and 2 respectively). Overall usage of Tool 1 and 2 grew during the period in all school categories, so we can be confident that this trend reflects an increase of use in High FSM schools rather than a decrease elsewhere.
This change in adoption patterns means children attending schools with higher levels of disadvantage were, on average, less likely to use Tools 1 and 2 for remote learning at the beginning of the pandemic. But, by the time the second set of school closures was introduced in Spring 2021, this was reversed.
3. Trends in device-type data suggest the ‘device disadvantage gap’ has narrowed
Access to appropriate hardware devices has been a major aspect of efforts to support disadvantaged children to learn remotely. The Sutton Trust reported that when school closures were first introduced, just 5 per cent of teachers in state schools reported that all their pupils had access to an appropriate device for remote learning, compared to 54 per cent at private schools .
Analysis of device data for Tool 1 tells a story of progress [Figure 3]. During the first school closures in Spring 2020, children in High FSM schools are less likely to access the platform from a desktop or laptop computer than children in Low or Medium FSM schools, and in July we can see a clear ‘device disadvantage gap’ of 9.9 per cent. However, by the second national school closures in Spring 2021, this gap has narrowed significantly to 0.4 per cent in February 2021.
Figure 3: The proportion of usage on a desktop or laptop computer by school deprivation level
Some of this shift may be the results of the Department for Education’s ‘ Get Help with Technology during Coronavirus ’ effort to dispatch laptops and tablets to children and families without access (rollout indicated by the grey line in Figure 3). As the government’s device distribution accelerates from November 2020 to February 2021, the ‘device disadvantage gap’ among Tool 1 users narrows. We should note that there were many other efforts going on (from individual school fundraising to local campaigns involving community groups and businesses) to help get devices in the hands of children who needed them.
4. But the ‘engagement gap’ looks more persistent
If analysis of the ‘device disadvantage gap’ describes a modest success, investigating measures of engagement with Tools 1 and 2 tell a more complicated story and suggest that there are significant barriers (besides access to devices) that continue to disproportionately impact disadvantaged children. To try and understand relative engagement with platforms, we have measured the amount of pupil or parent (for Tools 1 and 2 respectively) activity per teacher login - ie. the amount of engagement teachers get back for the same amount of platform activity they put in.
Figure 4: Pupil/parents activity per Teacher login by school deprivation level and by Ofsted rating (a proxy for ‘engagement’)
Figure 4 shows lower numbers of student and parent logins (for Tools 1 and 2 respectively) per teacher login in schools with higher levels of deprivation. Importantly, this gap does not close over time and the ‘engagement gap’ remains persistent. We can also see a similar gap (although slightly narrower) when we compare schools by Ofsted ratings. Data from Tool 3 covering January to March 2021 suggests a similar ‘engagement gap’, with parents or carers of pupil premium pupils less likely to make comments through the platform than those of non-pupil premium pupils.
What comes next?
This analysis provides a glimpse of how children’s experiences of EdTech during the pandemic have varied, revealing clear differences. However, there are reasons for cautious optimism - particularly with changes in the relative adoption among schools with higher deprivation levels, and the narrowing of the device disadvantage gap. We can see the results of progress towards getting the fundamental components of more equitable digital learning in place - such as the availability of EdTech platforms for teachers and children who want them, and the devices on which to access them.
However, consistent gaps in measures of engagement confirm that barriers to fairer distribution of the benefits of EdTech remain. We’re exploring these barriers through the EdTech R&D Programme - a partnership with the Department for Education, ImpactEd, 65 schools, the Teacher Development Trust and six widely used EdTech tools to develop and test improvements designed to support cohorts of children with particular barriers to digital learning. It is likely that these further barriers are more challenging, encompassing context-specific improvements to implementation, product design and teacher support. We look forward to sharing our findings from this work in the Autumn.
Also of interest
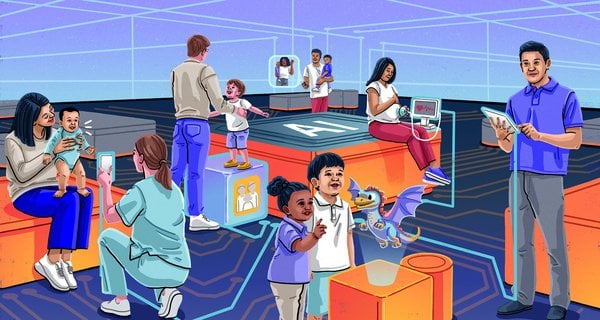
Innovation Sweet Spots: digital technologies for the early years
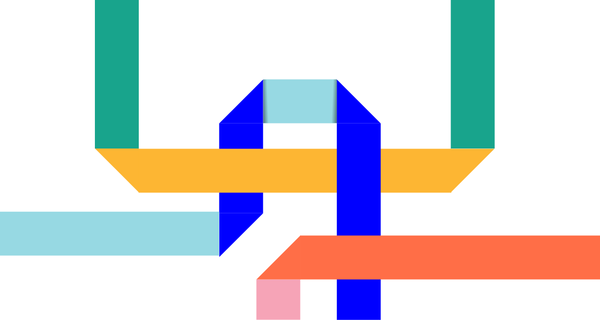
Call for evidence on building a sustainable system of integrated family support
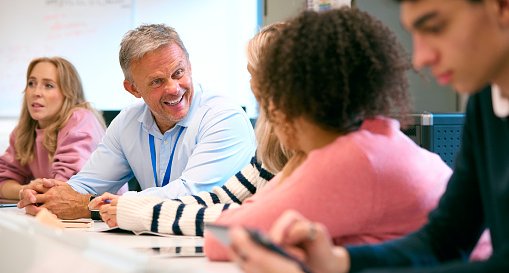
A new era of integration in the early years
Stay up to date.
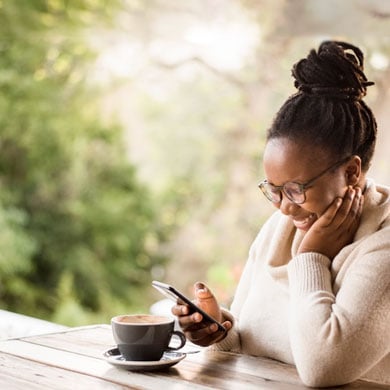
Join our mailing list to receive the Nesta edit: your first look at the latest insights, opportunities and analysis from Nesta and the innovation sector.
* denotes a required field
Sign up for our newsletter
You can unsubscribe by clicking the link in our emails where indicated, or emailing [email protected] . Or you can update your contact preferences . We promise to keep your details safe and secure. We won’t share your details outside of Nesta without your permission. Find out more about how we use personal information in our Privacy Policy .
- #Educational Technology in Schools Infographic
- #Educational Technology Timeline Infographic
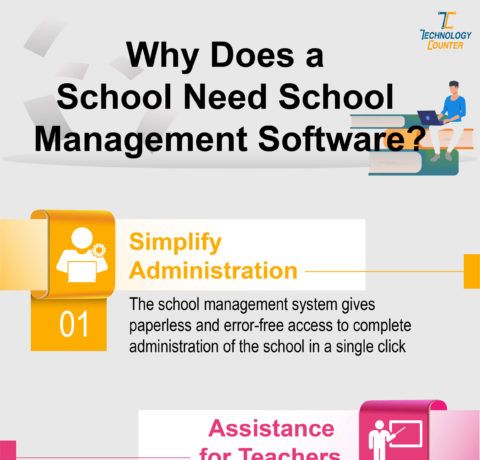
Timeline of Educational Technology in Schools Infographic
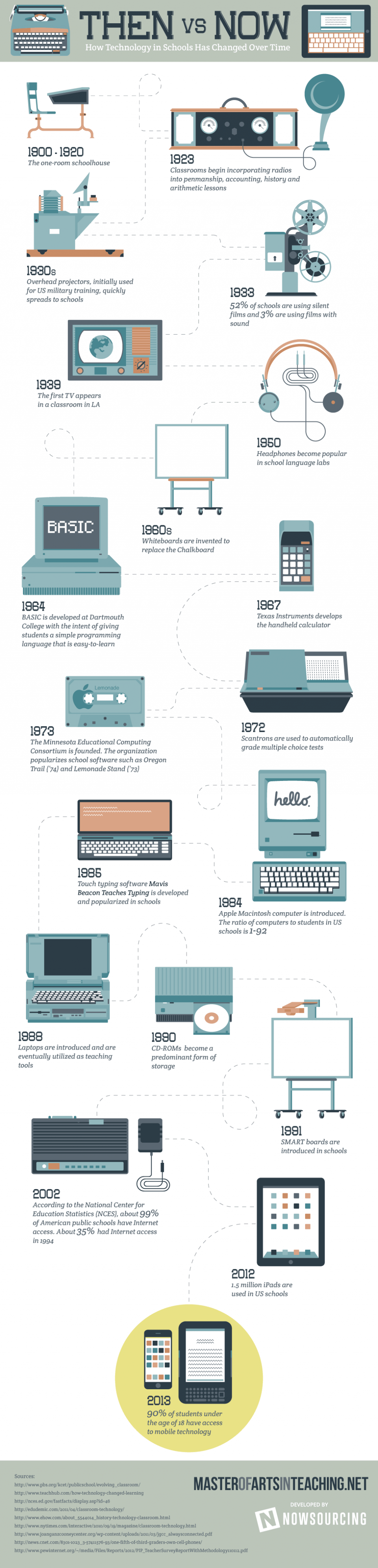
It’s amazing how quickly technology changes, even in the course of six months. But can you imagine how much it has changed over decades? The Timeline of Educational Technology in Schools Infographic shows how educational technology has evolved in schools over the past 114 years.
- 1900 - 1920 - Age of the One-room Schoolhouse
- 1923 - Radios were introduced to classrooms; major cities established classroom instruction on radios - penmanship, accounting, history and arithmetic were included
- 1930s - overhead projectors initially used for US military training purposes quickly spread to schools
- 1933 - 52% of schools were using silent films and 3% were using sound films
- 1939 - the first TV appeared in a classroom in LA; now the most widely used technology in schools
- 1950 - Headphones became popular in schools and stations used to listen to audio tapes were dubbed 'language labs'
- 1964 - BASIC developed at Dartmouth College with the intent to give students a simple programming language that was easy-to-learn
- 1967 - Texas Instruments develops the handheld calculator
- 1967 - LOGO programming language developed
- 1972 - Scantron - automatically graded multiple choice examples
- 1973 - The Minnesota Educational Computing Consortium (later Corporation), most commonly known as MECC was founded creators of Lemonade Stand ('73) and Oregon Trail ('74)
- 1984 - The Apple Macintosh computer is developed. The ration of computers to students in US schools is 1 - 92
- 1985 - Touch typing software Mavis Beacon Teaches Typing is developed and popularized in schools
- 1988 - laptops are developed and are eventually utilized as teaching tools
- 1990 - CD-ROM disks became the new kind of storage
- 1992 - Schools are use Gopher servers to provide students with online information
- 1994 - According to the National Center for Education Statistics (NCES), about 35% of American public schools had Internet access
- 1995 - Most CAI is delivered on CD-ROM disks and is growing in popularity
- 1996 - Faculty create instructional web pages
- 1999 - SMART boards introduced in schools
- 2001 - 80% of schools with internet access offered professional development training for teachers for integrating technology into classrooms.
- 2002 - 99% of schools had internet access
- 2009 - 1 computer for every 5.3 students in US schools
- 2010 - 1 wireless device for every 3.4 students in US schools
- 2011 - 80% of children under 5 use internet daily in the US
- 2012 - 1.5 million iPads provided by schools
- 2013 - 90% of students under the age of 18 have access to mobile technology
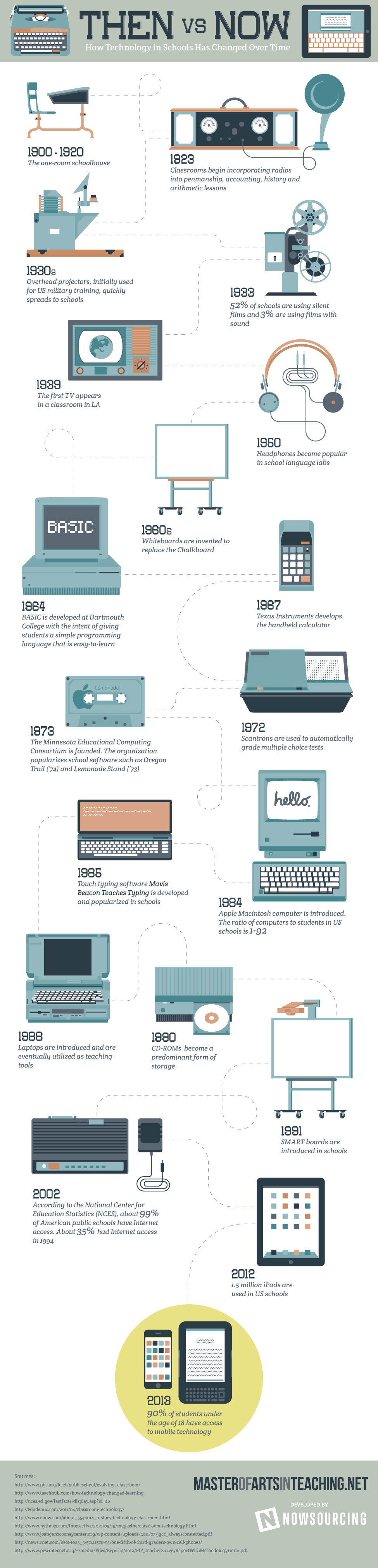
Trends and Topics in Educational Technology, 2024 Edition
- Column: Editorial
- Published: 21 March 2024
- Volume 68 , pages 402–410, ( 2024 )
Cite this article
- Bohdana Allman 1 ,
- Royce Kimmons 1 ,
- Wei Wang 2 ,
- Hanhui Bao 2 ,
- Joshua M. Rosenberg 2 &
- Matthew J. Koehler 3
4047 Accesses
Explore all metrics
Avoid common mistakes on your manuscript.
Introduction
This editorial continues to landscape the trends and popular educational technology topics for 2023. We used the public internet data mining approach from previous years (Allman et al., 2023b ; Kimmons, 2020 ; Kimmons & Rosenberg, 2022 ; Kimmons et al., 2021 ). This year, we extracted and analyzed data from the Scopus research article database, K-12 school and district Facebook pages, and the open publishing platform EdTech Books. We also looked closer at two key terms—“artificial intelligence” and “OER”—using Google Custom Search API to examine patterns in the higher education context and the description of resources from the Teachers Pay Teachers (TPT) website for insights in the K-12 context. This year, we no longer utilized the X (formerly Twitter) #EdTech affinity group as a data source because of the changes to the platform/accessibility of the data. Our analysis represents snapshots of 2023 trends in educational technology from these dataset angles, furthering our understanding of current EdTech community’s attitudes, behaviors, and leanings and underpinning a predictive vision of future trends in the field.
What Were Trends in EdTech Journals in 2023?
For insight into which research topics were trending in the field in 2023, we analyzed article titles published in the top educational technology journals during the year. We utilized a similar methodology as in previous years (Kimmons et al., 2021 ; Kimmons & Rosenberg, 2022 ; Allman et al., 2023b ) and compiled a list of 3,355 articles published in 2023 from the top educational technology journals (n = 18) as identified by Google Scholar and retrieved via the Scopus API. See Table 1 for the list of journals included in the analysis. Following this, we looked at the frequencies of each keyword and n-gram (multi-word phrase) appearing in the titles to identify potential trends.
We then manually categorized top keywords and n-grams into three information types suggested by the data: “Contexts,” “Methods,” and a broader category of “Topics, Tools, and Modalities” (see Table 2 ). Contexts included terms related to the research settings, such as “high school” or “university.” Methods included descriptors of the research methods, such as “systematic review” or “case study.” Topics, Tools, and Modalities included a more comprehensive array of terms, such as “online,” “learning analytics,” and “virtual reality.” Notably, in previous years, we had separated modalities into their own section, but this separation seemed to become increasingly arbitrary and unnecessary (e.g., is VR a topic or a modality?). So, we combined modalities and topics into a common category. We included all relevant n-grams above 0.5% and their comparatively ranked keywords in the table.
Table 2 suggests several noteworthy findings. Regarding contexts, higher education was far more common as a setting for educational technology studies than K-12, and secondary schools were more represented than elementary schools. This suggests an inverted pyramid representation of EdTech research being done at different educational levels, a trend that we saw in previous analyses (Allman et al., 2023b ). Referenced physical locations also focused on school settings, suggesting an emphasis on formal (rather than informal or non-formal) learning. As expected, references to COVID-19 declined from the previous year (3.6% to 2.4%). Relatively high on the list were also references to language learning. Specifically, search terms “language” (n = 169, 5.0%) and “EFL” (n = 95; 2.8%) and related n-grams “language learning” (n = 54; 1.6%), “EFL learner” (n = 37; 1.1%), and “foreign language” (n = 28, 0.8%). Additionally, references to “support” (n = 124, 3.7%), “professional,” and “preservice” (both n = 64; 1.9%) and n-grams “preservice teacher” (n = 49, 1.9%) and “professional development” (n = 22, 0.7%) might be worth noticing as important context keywords for studies carried out in 2023.
The most commonly referenced n-grams related to research methods mentioned in 2023 titles were secondary data analysis methods, specifically “systematic OR scoping OR literature reviews” (n = 194, 5.8%) and “meta-analyses” (n = 49, 1.9%). The most common primary data analysis method n-grams included “machine learning” (n = 43, 1.3%), “case study” (n = 43, 1.3%), “network analysis” (n = 23, 0.7%), and “mixed methods” (n = 24, 0.7%). Additionally, several keywords related to measuring educational success in the titles of 2023 journal articles are noteworthy. Specifically, search terms “effect” (n = 202, 6.0%), “performance” (n = 193, 5.8%), “impact” (n = 148, 4.4%), “evaluation” (n = 82, 2.4%), “effectiveness” and “achievement” (both n = 77, 2.3%), and “outcome” (n = 68, 2.0%).
Regarding modality, the dominant term continues to be “online” (n = 469, 14%) and the n-gram “online teaching OR online learning” (n = 150, 4.5%), outnumbering the next-highest n-gram, “blended learning” (n = 31, 0.9%), at a rate of 5-to-1. Although, from closer investigation of the titles, it appears that “online,” “distance,” “blended,” “remote,” and a variety of other terms are sometimes used interchangeably to describe a broad spectrum of internet-mediated synchronous or asynchronous learning situations. Immersive environments, in the form of “virtual,” “augmented,” and “mixed reality,” in that order, were also of interest. Specifically, the search term “virtual” appeared 188 times (5.6%), and n-grams “virtual reality” were seen 110 times (3.3%), “augmented reality” 63 times (1.9%), and “immersive virtual” 22 times (0.7%). Notably, references to “artificial intelligence” more than doubled from the previous year (n = 91, increase from 1.4% to 2.7%), and “learning analytics” also saw increased attention (n = 72, 1.6% to 2.1%).
What Was Trending among School and School District Facebook Groups in 2023?
The comprehensive analysis of hyperlinks shared on school and district Facebook pages revealed significant trends in technology adoption and usage within K-12 educational settings. Table 3 showcases the top fifteen domains by their prevalence and highlights the evolving landscape of digital tools in education from 2021 to 2023.
To identify the technologies shared on school and district Facebook pages, we scrutinized the domain names of all hyperlinks posted across 16,309 publicly accessible pages, totaling 10,597,076 posts. Executing this analysis involved exploring the homepages of all schools and school districts in the U.S. for links to Facebook pages. Subsequently, we uploaded the identified links to Facebook pages onto the CrowdTangle platform to access publicly available posts for the years 2021–2023 and identified the domains of websites linked within schools' and districts' posts. Additional details on the data collection approach can be found in Rosenberg et al. ( 2022 ). The top fifteen most-shared domains, delineated by year (2021, 2022, and 2023), are presented in Table 3 . The following explanation may help the reader interpret the table. For instance, in 2023, 7049, or 43% of schools or districts with publicly accessible Facebook pages, shared one or more links to docs.google.com , and the domain was shared on average 5.3 times.
Upon reviewing the years 2021 to 2023, we observed the continued dominance of Google services, with Google Docs maintaining its position as the most shared domain for three consecutive years, as highlighted in prior research (Allman et al., 2023b ). YouTube follows closely behind, indicating the sustained prevalence of Google services in the mainstream usage of schools and school districts, underscoring the stability of these technologies within educational institutions. Simultaneously, we noted a significant decline in the percentage of YouTube links from 44% in 2021 and 41% in 2022 to 33% in 2023. This shift might reflect a broader trend towards prioritizing the digital privacy and security of students within the educational community, influencing how schools and districts curate and share content on social media platforms. The trend in Zoom links continues to decline, with the proportion of districts sharing Zoom links decreasing from 21% in 2021 to 11% in 2022 and further dropping to 7% in 2023. This decline aligns with the reduced engagement in remote activities across various schools and school districts. Additionally, tools facilitating event sign-ups, exemplified by SignUpGenius and gofan.co , experienced steady increases, indicating a surge in posts promoting event registrations post-COVID-19 pandemic. Other domains, such as bookfairs.scholastic.com , smore.com , eventbrite.com , and surveymonkey.com , have consistently maintained their presence in the top ten over the past three years. Their similar frequency suggests the sustained importance of tools for school-parent communication, book sales, event management, and survey services within K-12 schools and districts.
What Were Trends in EdTech Open Educational Resources (OER) in 2023?
In addition to Scopus and social media trends, we also examined an EdTech-focused Open Educational Resource (OER) platform EdTech Books ( https://edtechbooks.org ). OER are “teaching, learning, and research materials that reside in the public domain or have been released under an open license that permits their free use and re-purposing by others” (Creative Commons, 2020 ). OER can take various forms and sizes, including textbooks, lessons, courses, learning activities, assessments, technologies, syllabi, images, presentations, videos, and graphics. Being ‘open’ means that OER is freely accessible to anyone with internet access and can be retained, reused, redistributed, revised, and remixed as needed (Wiley, n.d. ), providing significant opportunities for improving “the quality and affordability of education for learners everywhere” (Wiley & Hilton, 2018 , p. 144). Research has repeatedly shown that OER quality is comparable to commercial resources (Clinton & Khan, 2019 ; Kimmons, 2015 ), and their adoption does not negatively impact student learning (Hilton, 2016 , 2019 ) while saving students money (Clinton, 2018 ; Hilton, 2016 ; Ikahihifo et al., 2017 ) and providing a variety of other benefits (Kimmons, 2016 ). In 2023, almost two-thirds (64%) of U.S. higher education faculty are aware of OER, and 29% of faculty require OER in their courses (Seaman & Seaman, 2023b ).
For this year’s OER analysis, we again selected EdTech Books as the authors are most familiar with this platform and have ready access to data. In 2023, ETB provided free OER to more than 1.5 million users worldwide. We believe that as an EdTech-focused platform, EdTech Books analytics may provide valuable insights into user behavior and how OER are developed, adopted, and used in our field.
A perusal of the most popular books (Table 4 ) and chapters (Table 5 ) revealed that readers seemed to be drawn to these resources when they were seeking information on broad theoretical aspects of educational technology (e.g., behaviorism, constructivism, socioculturalism), technology-specific guidance (e.g., how to use a specific tool), or research and evaluation guidelines (e.g., mixed methods or sampling procedures). This is consistent with our findings from last year (Allman et al., 2023b ).
A closer analysis of the most popular books and chapters suggested that the top trending chapters are most influenced by organic traffic via search engines rather than direct links (such as from a course). This underscores the importance of indexing and optimizing OER resources to increase exposure and impact. On the other hand, EdTech books that were most accessed may have been influenced by OER adoption behaviors and instructors’ pedagogical decisions as part of formal access to instructional resources. For example, students might have been instructed to read carefully, which could mean accessing longer chapters several times or downloading them as PDF for annotation or later retrieval. Another instructor may encourage the use of social annotation tools, such as Hypothesis, to complete collaborative classroom assignments, encouraging students to return to a chapter several times and thus increasing overall book views. Additionally, ease of access or anticipation of fees to access may also explain why some books have higher PDF downloads than expected. For example, West’s Foundations of Learning and Instructional Design Technology (highest PDF downloads) is often sought out with search terms like “instructional design pdf,” which suggests that learners are intentionally seeking local copies of these particular resources.
We found that the United States (29.7%), the Philippines (14.1%), and India (6.2%) were again the heaviest users of the platform, with overall use of the platform becoming less centralized to the U.S. We also found an increase in overall mobile device access to the platform, with 39.7% of users accessing on a phone as opposed to 59% on a desktop or laptop. This reveals an increasing trend of globalization of educational-technology-related OER and the need to be attentive to their accessibility with various device configurations and bandwidth limitations.
References to Artificial Intelligence and OER on University Websites and Teachers Pay Teachers
Further exploring how large public data sources might help us identify patterns in the field, we used the Google Custom Search API to scrape data from university websites (cf., such as Kimmons & Veletsianos, 2021 and Veletsianos et al., 2023 ) and descriptions of resources uploaded to the popular curricular sharing site Teachers Pay Teachers (TPT) to understand the frequencies and nature of references to two key terms of particular interest to the authors: “artificial intelligence” and “OER”.
In considering Google indexing results of university websites, it is necessary to limit analyses to a few sets of interesting a priori terms. So, for this analysis, we limited our considerations to AI, given its current interest in the larger social context, and OER, given its attention in educational technology and the topic’s relationship to university missions as public caretakers of knowledge. Results showed that 66.4% of universities mentioned “generative artificial intelligence,” “generative AI,” or “ChatGPT,” and 47.7% referenced “open educational resource” or “open textbook,” with references to generative AI outnumbering references to OER at a rate of nearly 5-to-1 (see Table 6 ). In both cases, politically blue states (Democratic according to the most recent U.S. presidential election) were more likely to reference these technologies than were politically red (Republican) states. However, urban states were more likely to reference “AI,” and rural states were more likely to reference “OER.” Rhode Island, Utah, and Idaho were among the most likely to mention both, and Wyoming was the least likely to mention either. Interestingly, Hawaii was the most likely to mention artificial intelligence but was among the least likely to mention OER. This pattern suggests sociopolitical and economic differences in how educators pay attention to these technologies. Also, it suggests that universities may be more actively playing into the hype of new technologies (e.g., “AI”) in their communication efforts than serving as public distributors of valuable knowledge to their communities (e.g., “OER”).
In a similar vein, data extracted from the TPT website spanning from 2021 to 2023, encompassing 3,936,779 entries, were explored. Specific details regarding the data collection method can be found in (Shelton et al., 2022 ). The analysis revealed a total of 3,303 instances referencing AI-related keywords, including "generative artificial intelligence," "generative AI," "artificial intelligence," "DALL-E," and "ChatGPT." In contrast, mentions of "open educational resource" or "open textbook" numbered 4,285 (see Table 7 for details).
The analysis of the data suggested a growing trend of references to AI-related educational resources on the TPT platform from 2021 to 2023. Notably, despite the proportion of AI-related resources being low before 2023, there has been a remarkable uptick in interest. The number of AI-related resources in 2021 and 2022 were less than 0.05%. Specifically, in 2021, only 521 out of 1,060,241 or 0.049% of total resources and 528 out of 1,268,771 (0.042%) resources in 2022 were related to AI. In 2023, the mentions of AI surged to 2,254 out of 1,607,767 or 0.14% resources, representing almost a threefold increase from the 2022 figures, indicating a burgeoning interest in AI within K-12 educational resources. This surge aligns with the rising interest and integration of AI in educational settings, particularly following the release of generative artificial intelligence tools like ChatGPT in November 2022, reflecting educators' growing curiosity and the pressing need to incorporate AI into their teaching resources.
Compared to the mentions of Open Educational Resources (OER), AI references are fewer in number. However, the ratio of nearly 1-to-1.3 (AI to OER) suggests that AI is also becoming a topic of significant interest within educational resources in the K-12 setting. This is particularly noteworthy given that OERs have been a mainstay in educational discussions for a longer period, emphasizing the rapid ascension of AI as a key area of focus. The increasing mention of specific AI tools like "DALL-E" and "ChatGPT" possibly indicates a shift in the educational resource landscape, where innovative AI tools are starting to play a central role in creating and disseminating educational content. This shift could be attributed to the capabilities of generative AI, offering novel approaches to personalized learning, automated content generation, and interactive learning experiences. The disparity between the growth of AI vs. OER references could also reflect the evolving nature of educational technology, where there is a move from traditional open resources to more dynamic, adaptive, and personalized learning experiences AI offers. Integrating AI in educational resources can represent a transformative step in educational technology, potentially reshaping how educational content is created, distributed, and consumed. However, as AI online educational resources rapidly expand, concerns like academic fraud, information bias, and ethical dilemmas arise and deserve closer attention. Recommendations from educational technology experts are especially relevant and needed since markets often lack the motivation to regulate content under platform capitalism (Rodríguez et al., 2020 ).
Discussion and Conclusion
The analyses of the data from Scopus, Facebook, and EdTech Books, as well as the examination of AI and OER-related terms using Google Custom Search API and Teachers Pay Teachers, represent snapshots from different angles and offer valuable insights into the current state of the educational technology field. Moreover, by comparing some of the 2023 results to previous years, we observed several developmental directions and trends that may guide educational researchers and practitioners for future work.
The Scopus data suggested that studies published in the top EdTech journals in 2023 were predominantly conducted in higher education contexts, and among K-12 studies, secondary contexts were more common than elementary. Not surprisingly, references to COVID-19 declined from previous years. Interestingly, although COVID-19 was less referenced, the terms “online teaching” and “online learning” were frequently mentioned, remaining a dominant learning modality. Secondary data analysis methods, such as literature reviews and meta-analyses, were the most common research methods. However, it is important to mention that this year’s analysis included only titles, not abstracts, as was done in previous years, which may typically include fewer references to primary research methods. Keywords related to emerging technologies, including virtual reality, augmented reality, artificial intelligence, and learning analytics, were also frequently mentioned in the titles.
Through analyzing the hyperlinks on school and school district Facebook pages, we observed that Google-provided services, such as Google Docs, YouTube, and Google search engine, were the most included external links, which seems to be consistent with our findings from previous years (Allman et al., 2023b ; Kimmons et al., 2021 ; Kimmons & Rosenberg, 2022 ). A trend worth mentioning is the consistent decline of Zoom links and increased links to school event planning and registration sites between 2021 and 2023. This suggests a return to in-person learning and an increased school social event activity post-COVID-19.
The analysis of EdTechBooks data as a proxy for OER behavior in the field of educational technology revealed that, similar to last year’s findings, readers continue seeking resources related to theory, educational technology topics, and research and evaluation methods. Closer analysis suggested that chapter access might be more influenced by the organic traffic from search engines. In contrast, book access may be more tied to OER adoption and formal educational setting behaviors, such as course instructional material choices and instructor pedagogical decisions. The increase in global and mobile OER access further emphasizes the importance of technical and design decisions related to accessibility, flexibility, and social justice issues during OER design and development (Allman et al., 2023a ).
Finally, the results of further examining AI and OER-related terms on university websites and Teachers Pay Teachers were intriguing. One interesting finding was that universities in politically blue states were more likely to refer to both technologies than universities in politically red states. Additionally, universities in urban states typically referenced AI more often, while rural state universities more likely referenced OER. This suggests that EdTech attention may be associated with social, political, and economic factors, such as available capital and resources. The analysis of resources on the Teachers Pay Teachers platform emphasized a rising interest in AI in K-12 educational resources while the interest in OER resources remained steady. Among the AI tools, references to generative AI tools such as ChatGPT increased the most, suggesting interest in applying these tools in education and educational content creation.
This year’s analyses indicated that the field of educational technology continues to be influenced by the past pandemic as well as emerging technologies. Even though COVID-19 has gradually faded out in people’s lives, online learning has become a widely accepted way of learning, and technology-mediated instruction has become a norm in all educational settings. Digital educational resources replaced, for the most part, traditional print materials both in higher education and K-12 settings (Seaman & Seaman, 2023a , 2023b ). Mobile and digital learning platforms make learning more accessible and facilitate collaboration through cloud-based services across modalities. OER remain an interest in K-12 and higher ed, particularly in rural states. Immersive technologies continue transforming the EdTech landscape, integrating VR, AR, and gamification elements into learning environments for more engaging experiences. We found that AI and generative AI, in particular, are topics that are notably raising interest in the educational technology field. Utilizing generative AI to produce content and instructional resources, provide adaptive and personalized learning experiences, and automate assessment and evaluation are only a few potential applications that could transform the field of educational technology in the near future. Although the inclusion of AI is relevant at the university and K-12 level, social, political, and economic influences and implications need to be considered. Recognizing that many across educational sectors feel unprepared for AI-related changes (Cengage, 2023 ), we should embrace these new technologies with optimistic caution, carefully considering potentials balanced against security, privacy, and other concerns.
Data Availability
Data is available upon request.
Allman, B., Bozkurt, A., Dickson-Deane, C., Kimmons, R., Stefaniak, J., & Warr, M. C. (2023a, October 15–19). EdTechnica: Open educational resource and open educational practice [Panel discussion]. AECT International Convention. Retrieved January 15, 2024, from https://www.youtube.com/watch?v=8GB0V1CGvZY
Allman, B., Kimmons, R., Rosenberg, J., & Dash, M. (2023b). Trends and topics in educational technology, 2023 edition. TechTrends, 67 , 583–591. https://doi.org/10.1007/s11528-023-00840-2
Article Google Scholar
Cengage. (2023). Examining higher ed’s digital future: Infographic of 2023–2024 digital learning pulse survey. Retrieved February 8, 2024, from https://www.bayviewanalytics.com/reports/pulse/infographic-fall2023.pdf
Clinton, V. (2018). Savings without sacrifices: A case study of open-source textbook adoption. Open Learning: The Journal of Distance and Open Learning, 33 (3), 177–189. https://doi.org/10.1080/02680513.2018.1486184
Clinton, V., & Khan, S. (2019). Efficacy of open textbook adoption on learning performance and course withdrawal rates: A meta-analysis. AERA Open, 5 (3), 1–20. https://doi.org/10.1177/2332858419872212
Creative Commons. (2020). Open education. Retrieved December 6, 2023, from https://creativecommons.org/about/program-areas/education-oer
Hilton, J. (2016). Open educational resources and college textbook choices: A review of research on efficacy and perceptions. Educational Technology Research and Development, 64 (4), 573–590. https://doi.org/10.1007/s11423-016-9434-9
Hilton, J. (2019). Open educational resources, student efficacy, and user perceptions: A synthesis of research published between 2015–2018. Educational Technology Research and Development, 68 (3), 853–876. https://doi.org/10.1007/s11423-019-09700-4
Ikahihifo, T. K., Spring, K. J., Rosecrans, J., & Watson, J. (2017). Assessing the savings from open educational resources on student academic goals. The International Review of Research in Open and Distance Learning, 18 (7). https://doi.org/10.19173/irrodl.v18i7.2754
Kimmons, R. (2020). Current trends (and missing links) in educational technology research and practice. TechTrends, 64 (6), 803–809. https://doi.org/10.1007/s11528-020-00549-6
Kimmons, R., & Rosenberg, J. M. (2022). Trends and topics in educational technology, 2022 edition. TechTrends, 66 (2), 134–140. https://doi.org/10.1007/s11528-022-00713-0
Kimmons, R., Rosenberg, J., & Allman, B. (2021). Trends in educational technology: What Facebook, twitter, and Scopus can tell us about current research and practice. TechTrends, 65 (2), 125–136. https://doi.org/10.1007/s11528-021-00589-6
Kimmons, R., & Veletsianos, G. (2021). Proctoring software in higher ed: Prevalence and patterns. Educause Review . Retrieved December 8, 2023, from https://er.educause.edu/articles/2021/2/proctoring-software-in-higher-ed-prevalence-and-patterns
Kimmons, R. (2015). OER quality and adaptation in K-12: Comparing teacher evaluations of copyright-restricted, open, and open/adapted textbooks. The International Review of Research in Open and Distributed Learning, 16 (5). https://doi.org/10.19173/irrodl.v16i5.2341
Kimmons, R. (2016). Expansive openness in teacher practice. Teachers College Record, 118 (9). https://doi.org/10.1177/016146811611800901
Rodríguez, N., Brown, M., & Vickery, A. (2020). Pinning for profit? Examining elementary preservice teachers’ critical analysis of online social studies resources about Black history. Contemporary Issues in Technology and Teacher Education, 20 (3), 497–528. Retrieved January 5, 2024, from https://www.learntechlib.org/primary/p/216743/
Rosenberg, J. M., Borchers, C., Stegenga, S. M., Burchfield, M. A., Anderson, D., & Fischer, C. (2022). How educational institutions reveal students’ personally identifiable information on Facebook. Learning, Media and Technology, 1–17. https://doi.org/10.1080/17439884.2022.2140672
Seaman, J.E., & Seaman J. (2023a). Curricula of many sources: Educational resources in U.S. K-12 education, 2023 . Bay View Analytics. Retrieved February 8, 2024, from https://www.bayviewanalytics.com/reports/curricula-of-many-sources-2023.pdf
Seaman, J.E., & Seaman, J. (2023b). Digitally established: Educational resources in U.S. higher education, 2023 . Bay View Analytics. Retrieved February 8, 2024, from https://www.bayviewanalytics.com/reports/digitallyestablished-2023.pdf
Shelton, C. C., Koehler, M. J., Greenhalgh, S. P., & Carpenter, J. P. (2022). Lifting the veil on TeachersPayTeachers.com: an investigation of educational marketplace offerings and downloads. Learning, Media and Technology , 22(2), 268–287. https://doi.org/10.1080/17439884.2021.1961148
Veletsianos, G., Kimmons, R., & Bondah, F. (2023). ChatGPT and higher education: Initial prevalence and areas of interest. Educause Review . Retrieved February 12, 2024, from https://er.educause.edu/articles/2023/3/chatgpt-and-higher-education-initial-prevalence-and-areas-of-interest
Wiley, D. (n.d.). Defining the “open” in open content and open educational resources. Retrieved February 10, 2024, from https://opencontent.org/definition/
Wiley, D., & Hilton, J. L., III. (2018). Defining OER-enabled pedagogy. The International Review of Research in Open and Distributed Learning, 19 (4). https://doi.org/10.19173/irrodl.v19i4.3601
Download references
Author information
Authors and affiliations.
Brigham Young University, Provo, UT, USA
Bohdana Allman & Royce Kimmons
University of Tennessee, Knoxville, TN, USA
Wei Wang, Hanhui Bao & Joshua M. Rosenberg
Michigan State University, East Lansing, MI, USA
Matthew J. Koehler
You can also search for this author in PubMed Google Scholar
Corresponding author
Correspondence to Bohdana Allman .
Additional information
Publisher's note.
Springer Nature remains neutral with regard to jurisdictional claims in published maps and institutional affiliations.
Rights and permissions
Reprints and permissions
About this article
Allman, B., Kimmons, R., Wang, W. et al. Trends and Topics in Educational Technology, 2024 Edition. TechTrends 68 , 402–410 (2024). https://doi.org/10.1007/s11528-024-00950-5
Download citation
Published : 21 March 2024
Issue Date : May 2024
DOI : https://doi.org/10.1007/s11528-024-00950-5
Share this article
Anyone you share the following link with will be able to read this content:
Sorry, a shareable link is not currently available for this article.
Provided by the Springer Nature SharedIt content-sharing initiative
- Find a journal
- Publish with us
- Track your research
- Technology & Telecommunications ›
- Consumer Electronics
Children and technology - Statistics & Facts
Consumption in the united states, consumption in europe, key insights.
Detailed statistics
Children with internet access at home worldwide 2020, by region
Most visited online content categories among kids worldwide 2023, by browser
Leading YouTube channels made for kids worldwide 2024, by subscribers
Further recommended statistics
- Premium Statistic Revenue of the consumer electronics industry worldwide 2019-2029
- Premium Statistic Consumer electronics market revenue worldwide 2023, by segment
- Premium Statistic Children with internet access at home worldwide 2020, by region
- Basic Statistic Most visited online content categories among kids worldwide 2023, by browser
- Basic Statistic Parents in selected countries who block WhatsApp on their children phones 2021
Revenue of the consumer electronics industry worldwide 2019-2029
Revenue of the consumer electronics market worldwide from 2019 to 2029 (in billion U.S. dollars)
Consumer electronics market revenue worldwide 2023, by segment
Revenue of the global consumer electronics market in 2023, by segment (in billion U.S. dollars)
Share of children with internet access in their household in 2020, by region
Most popular online content categories among children worldwide from May 2022 to May 2023, by browser
Parents in selected countries who block WhatsApp on their children phones 2021
Share of parents in selected countries who blocked WhatsApp on their children mobile devices as of 2021
United States
- Premium Statistic Share of U.S. children with an electronic device in their home 2019-2021, by type
- Premium Statistic Share of U.S. children with a personal electronic device 2021, by type
- Premium Statistic Share of U.S. children owning a smartphone 2015-2021, by age
- Premium Statistic Share of tweens who engaged in media activity in the U.S. 2021, by activity
- Premium Statistic Daily entertainment screen time among children in the U.S. in 2021, by activity
Share of U.S. children with an electronic device in their home 2019-2021, by type
Share of children with an electronic device in their home in the United States in 2019 and 2021, by type
Share of U.S. children with a personal electronic device 2021, by type
Share of children with a personal electronic device in the United States in 2021, by type
Share of U.S. children owning a smartphone 2015-2021, by age
Share of children owning a smartphone in the United States in 2015, 2019 and 2021, by age
Share of tweens who engaged in media activity in the U.S. 2021, by activity
Share of tweens who engaged in media activity in the United States in 2021, by activity
Daily entertainment screen time among children in the U.S. in 2021, by activity
Average daily entertainment screen time among children in the United States in 2021, by activity (in hours.minutes)
United Kingdom
- Premium Statistic Devices available to children in the home in the United Kingdom (UK) 2015-2020
- Premium Statistic Children owning mobile phones in the UK 2023, by age
- Basic Statistic Devices used to browse the web by children in the United Kingdom 2023, by age group
- Basic Statistic Gaming devices used by children in the United Kingdom (UK) 2023, by age group
- Premium Statistic UK teens and parents on the benefits using the internet 2022
Devices available to children in the home in the United Kingdom (UK) 2015-2020
Devices available to children in the home in the United Kingdom (UK) from 2015 to 2020
Children owning mobile phones in the UK 2023, by age
Share of children owning mobile phones in the United Kingdom (UK) in 2023, by age group
Devices used to browse the web by children in the United Kingdom 2023, by age group
Devices used to go online by children in the United Kingdom (UK) as of December 2023, by age group
Gaming devices used by children in the United Kingdom (UK) 2023, by age group
Devices used for gaming by children in the United Kingdom (UK) as of December 2023, by age group
UK teens and parents on the benefits using the internet 2022
Benefits of using the internet according to teenagers and parents in the United Kingdom in 2022
- Premium Statistic Electronic devices in households with children in Germany 2022
- Basic Statistic Smartphone ownership among children and teenagers Germany 2022, by age group
- Premium Statistic Share of children using electronic devices in France 2019-2021, by type
- Premium Statistic Types of social network activities amongst children in France in 2020-2022
- Premium Statistic Italian children who interact with their mobile devices when in company 2021
- Premium Statistic Italian children experiences with online dangers and online safety 2021
Electronic devices in households with children in Germany 2022
Which of the following electronic devices are available in your household?
Smartphone ownership among children and teenagers Germany 2022, by age group
Smartphone ownership among children and teenagers in Germany in 2022, by age group
Share of children using electronic devices in France 2019-2021, by type
Share of children using electronic devices in France in 2019 and 2021, by type
Types of social network activities amongst children in France in 2020-2022
Preferred social media activities of French children aged 11 to 18 in 2020 and 2022
Italian children who interact with their mobile devices when in company 2021
Share of children in Italy who use smartphones or tablets when in the company of others from May 2021 to October 2021
Italian children experiences with online dangers and online safety 2021
Attitudes and experienced of children in Italy on online dangers and safety from May 2021 to October 2021
Further reports
Get the best reports to understand your industry.
Mon - Fri, 9am - 6pm (EST)
Mon - Fri, 9am - 5pm (SGT)
Mon - Fri, 10:00am - 6:00pm (JST)
Mon - Fri, 9:30am - 5pm (GMT)
Information
- Author Services
Initiatives
You are accessing a machine-readable page. In order to be human-readable, please install an RSS reader.
All articles published by MDPI are made immediately available worldwide under an open access license. No special permission is required to reuse all or part of the article published by MDPI, including figures and tables. For articles published under an open access Creative Common CC BY license, any part of the article may be reused without permission provided that the original article is clearly cited. For more information, please refer to https://www.mdpi.com/openaccess .
Feature papers represent the most advanced research with significant potential for high impact in the field. A Feature Paper should be a substantial original Article that involves several techniques or approaches, provides an outlook for future research directions and describes possible research applications.
Feature papers are submitted upon individual invitation or recommendation by the scientific editors and must receive positive feedback from the reviewers.
Editor’s Choice articles are based on recommendations by the scientific editors of MDPI journals from around the world. Editors select a small number of articles recently published in the journal that they believe will be particularly interesting to readers, or important in the respective research area. The aim is to provide a snapshot of some of the most exciting work published in the various research areas of the journal.
Original Submission Date Received: .
- Active Journals
- Find a Journal
- Proceedings Series
- For Authors
- For Reviewers
- For Editors
- For Librarians
- For Publishers
- For Societies
- For Conference Organizers
- Open Access Policy
- Institutional Open Access Program
- Special Issues Guidelines
- Editorial Process
- Research and Publication Ethics
- Article Processing Charges
- Testimonials
- Preprints.org
- SciProfiles
- Encyclopedia
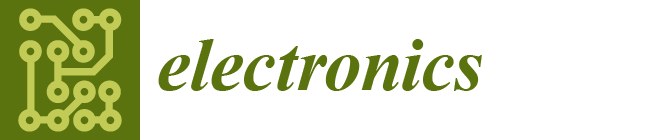
Article Menu
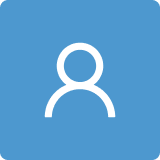
- Subscribe SciFeed
- Recommended Articles
- Google Scholar
- on Google Scholar
- Table of Contents
Find support for a specific problem in the support section of our website.
Please let us know what you think of our products and services.
Visit our dedicated information section to learn more about MDPI.
JSmol Viewer
A survey of knowledge graph approaches and applications in education.
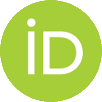
1. Introduction
- What are the patterns of publications on knowledge graphs in education?
- What are the educational contexts of knowledge graph applications?
- What are the objectives, application categories, data sources, technical means, and pedagogical issues for knowledge graph approaches and applications in education?
2. Related Work
3. methodology, 3.1. data collection, 3.2. data analysis, 4.1. overview of the publications, 4.1.1. year of publication, 4.1.2. country/region of authors’ affiliations, 4.1.3. research methods, 4.2. contexts of knowledge graph approaches, 4.2.1. level of education, 4.2.2. subject disciplines, 4.3. implementation of knowledge graph approaches, 4.3.1. types of objectives, 4.3.2. types of application categories, 4.3.3. types of knowledge graph resources, 4.3.4. types of technical means, 4.3.5. types of pedagogies, 5. discussion, 6. conclusions, author contributions, conflicts of interest.
- Kejriwal, M. Knowledge graphs: A practical review of the research landscape. Information 2022 , 13 , 161. [ Google Scholar ] [ CrossRef ]
- Hogan, A.; Blomqvist, E.; Cochez, M.; d’Amato, C.; Melo, G.D.; Gutierrez, C.; Kirrane, S.; Gayo, J.E.L.; Navigli, R.; Neumaier, S.; et al. Knowledge graphs. ACM Comput. Surv. 2021 , 54 , 71. [ Google Scholar ]
- Ji, S.; Pan, S.; Cambria, E.; Marttinen, P.; Philip, S.Y. A survey on knowledge graphs: Representation, acquisition, and applications. IEEE Trans. Neural Netw. Learn. Syst. 2021 , 33 , 494–514. [ Google Scholar ] [ CrossRef ]
- Tiddi, I.; Lécué, F.; Hitzler, P. (Eds.) Knowledge Graphs for Explainable Artificial Intelligence: Foundations, Applications and Challenges ; IOS Press: Amsterdam, The Netherlands, 2020. [ Google Scholar ]
- Singhal, A. Introducing the Knowledge Graph: Things, Not Strings. Official Google Blog. 2012. Available online: https://blog.google/products/search/introducing-knowledge-graph-things-not/ (accessed on 25 May 2024).
- Tiwari, S.; Al-Aswadi, F.N.; Gaurav, D. Recent trends in knowledge graphs: Theory and practice. Soft Comput. 2021 , 25 , 8337–8355. [ Google Scholar ] [ CrossRef ]
- Wu, T.; Qi, G.; Li, C.; Wang, M. A survey of techniques for constructing Chinese knowledge graphs and their applications. Sustainability 2018 , 10 , 3245. [ Google Scholar ] [ CrossRef ]
- Troussas, C.; Krouska, A.; Tselenti, P.; Kardaras, D.K.; Barbounaki, S. Enhancing personalized educational content recommendation through cosine similarity-based knowledge graphs and contextual signals. Information 2023 , 14 , 505. [ Google Scholar ] [ CrossRef ]
- Albreiki, B.; Habuza, T.; Palakkal, N.; Zaki, N. Clustering-based knowledge graphs and entity-relation representation improves the detection of at risk students. Educ. Inf. Technol. 2023 , 29 , 6791–6820. [ Google Scholar ] [ CrossRef ]
- Chung, C.Y.; Hsiao, I.H.; Lin, Y.L. AI-assisted programming question generation: Constructing semantic networks of programming knowledge by local knowledge graph and abstract syntax tree. J. Res. Technol. Educ. 2023 , 55 , 94–110. [ Google Scholar ] [ CrossRef ]
- Limongelli, C.; Lombardi, M.; Marani, A.; Taibi, D. A semantic approach to ranking techniques: Improving web page searches for educational purposes. IEEE Access 2022 , 10 , 68885–68896. [ Google Scholar ] [ CrossRef ]
- Shang, S.; Lyv, W.; Luo, L. Applying lean six sigma incorporated with big data analysis to curriculum system improvement in higher education institutions. Int. J. Syst. Assur. Eng. Manag. 2022 , 13 , 641–656. [ Google Scholar ]
- Zablith, F. Constructing social media links to formal learning: A knowledge graph approach. Educ. Technol. Res. Dev. 2022 , 70 , 559–584. [ Google Scholar ] [ CrossRef ]
- Mzwri, K.; Turcsányi-Szabo, M. Internet wizard for enhancing open-domain question-answering chatbot knowledge base in education. Appl. Sci. 2023 , 13 , 8114. [ Google Scholar ] [ CrossRef ]
- Choi, H.; Lee, H.; Lee, M. Optimal knowledge component extracting model for knowledge-concept graph completion in education. IEEE Access 2023 , 11 , 15002–15013. [ Google Scholar ] [ CrossRef ]
- Yuan, R.; Li, H.; Sun, Z.; Zhang, H. Application of graph convolutional network in the construction of knowledge graph for higher mathematics teaching. Sens. Mater. 2023 , 35 , 4269–4290. [ Google Scholar ] [ CrossRef ]
- Xiao, X.; Fang, Z.; Zou, S.; Zhang, C.; Chen, X. Effects of an intelligent cues recognition-based multilevel knowledge graphs generation method on students in online learning environments. Interact. Learn. Environ. 2023 . [ Google Scholar ] [ CrossRef ]
- Abu-Salih, B.; Alotaibi, S. A systematic literature review of knowledge graph construction and application in education. Heliyon 2024 , 10 , e25383. [ Google Scholar ] [ CrossRef ] [ PubMed ]
- Elkaimbillah, Z.; Rhanoui, M.; Mikram, M.; El Asri, B. Comparative study of knowledge graph models in education domain. In Proceedings of the International Conference on Bigdata, Modelling and Machine Learning, Cox’s Bazar, Bangladesh, 23–25 September 2021. [ Google Scholar ]
- Fettach, Y.; Ghogho, M.; Benatallah, B. Knowledge graphs in education and employability: A survey on applications and techniques. IEEE Access 2022 , 10 , 80174–80183. [ Google Scholar ] [ CrossRef ]
- Chen, X.; Xie, H.; Li, Z.; Cheng, G. Topic analysis and development in knowledge graph research: A bibliometric review on three decades. Neurocomputing 2021 , 461 , 497–515. [ Google Scholar ] [ CrossRef ]
- Wang, G.; He, J. A bibliometric analysis of recent developments and trends in knowledge graph research (2013–2022). IEEE Access 2024 , 12 , 32005–32013. [ Google Scholar ] [ CrossRef ]
- Wang, Q.; Mao, Z.; Wang, B.; Guo, L. Knowledge graph embedding: A survey of approaches and applications. IEEE Trans. Knowl. Data Eng. 2017 , 29 , 2724–2743. [ Google Scholar ] [ CrossRef ]
- Rajabi, E.; Etminani, K. Knowledge-graph-based explainable AI: A systematic review. J. Inf. Sci. 2022 . [ Google Scholar ] [ CrossRef ]
- Tian, L.; Zhou, X.; Wu, Y.P.; Zhou, W.T.; Zhang, J.H.; Zhang, T.S. Knowledge graph and knowledge reasoning: A systematic review. J. Electron. Sci. Technol. 2022 , 20 , 100159. [ Google Scholar ] [ CrossRef ]
- Abu-Salih, B. Domain-specific knowledge graphs: A survey. J. Netw. Comput. Appl. 2021 , 185 , 103076. [ Google Scholar ] [ CrossRef ]
- Chiu, B.; See-To, W.K.E.; Ngai, E.W.T. Knowledge graph construction and applications in e-retailing: A review of literature. In Proceedings of the Pacific Asian Conference on Information Systems, Virtual, 12 July 2021; p. 244. [ Google Scholar ]
- Wang, S.; Lin, M.; Ghosal, T.; Ding, Y.; Peng, Y. Knowledge graph applications in medical imaging analysis: A scoping review. Health Data Sci. 2022 , 2022 , 9841548. [ Google Scholar ] [ CrossRef ] [ PubMed ]
- Abu-Salih, B.; Al-Qurishi, M.; Alweshah, M.; AL-Smadi, M. Healthcare knowledge graph construction: State-of-the-art, open issues, and opportunities. arXiv 2022 , arXiv:2207.03771. [ Google Scholar ]
- Li, K.C.; Wong, B.T.M. Review of smart learning: Patterns and trends in research and practice. Australas. J. Educ. Technol. 2021 , 37 , 189–204. [ Google Scholar ] [ CrossRef ]
- Jardim, J.; Bártolo, A.; Pinho, A. Towards a global entrepreneurial culture: A systematic review of the effectiveness of entrepreneurship education programs. Educ. Sci. 2021 , 11 , 398. [ Google Scholar ] [ CrossRef ]
- Alonso, R.K.; Vélez, A.; Martínez-Monteagudo, M.C.; Rico-González, M. Flipped learning in higher education for the development of intrinsic motivation: A systematic review. Educ. Sci. 2023 , 13 , 1226. [ Google Scholar ] [ CrossRef ]
- Paneque, M.; del Mar Roldán-García, M.; García-Nieto, J. e-LION: Data integration semantic model to enhance predictive analytics in e-Learning. Expert Syst. Appl. 2023 , 213 , 118892. [ Google Scholar ] [ CrossRef ]
- Zheng, L.; Kinshuk; Fan, Y.; Long, M. The impacts of the comprehensive learning analytics approach on learning performance in online collaborative learning. Educ. Inf. Technol. 2023 , 28 , 16863–16886. [ Google Scholar ] [ CrossRef ]
- Zheng, L.; Niu, J.; Long, M.; Fan, Y. An automatic knowledge graph construction approach to promoting collaborative knowledge building, group performance, social interaction and socially shared regulation in CSCL. Br. J. Educ. Technol. 2023 , 54 , 686–711. [ Google Scholar ] [ CrossRef ]
- Zheng, L.; Long, M.; Chen, B.; Fan, Y. Promoting knowledge elaboration, socially shared regulation, and group performance in collaborative learning: An automated assessment and feedback approach based on knowledge graphs. Int. J. Educ. Technol. High. Educ. 2023 , 20 , 46. [ Google Scholar ] [ CrossRef ]
- Han, D.; Kim, D.; Kim, M.; Han, K.; Yi, M.Y. Temporal enhanced inductive graph knowledge tracing. Appl. Intell. 2023 , 53 , 29282–29299. [ Google Scholar ] [ CrossRef ]
- Troussas, C.; Krouska, A. Path-based recommender system for learning activities using knowledge graphs. Information 2023 , 14 , 9. [ Google Scholar ] [ CrossRef ]
- Yang, Y.; Chen, S.; Zhu, Y.; Zhu, H.; Chen, Z. Knowledge graph empowerment from knowledge learning to graduation requirements achievement. PLoS ONE 2023 , 18 , e0292903. [ Google Scholar ] [ CrossRef ] [ PubMed ]
- Ain, Q.U.; Chatti, M.A.; Bakar, K.G.C.; Joarder, S.; Alatrash, R. Automatic Construction of Educational Knowledge Graphs: A Word Embedding-Based Approach. Information 2023 , 14 , 526. [ Google Scholar ] [ CrossRef ]
- Sin, Z.P.; Jia, Y.; Wu, A.C.; Zhao, I.D.; Li, R.C.; Ng, P.H.; Baciu, G.; Cao, J.; Li, Q. Towards an edu-metaverse of knowledge: Immersive exploration of university courses. IEEE Trans. Learn. Technol. 2023 , 16 , 1096–1110. [ Google Scholar ] [ CrossRef ]
- Hu, Y.; Xu, B. Analysis of the dilemma of higher vocational thinking education in China under the background of “Internet+”. Appl. Math. Nonlinear Sci. 2024 , 9 , 1–15. [ Google Scholar ] [ CrossRef ]
- Zhang, W.; Chung Ee, J.Y. An Intelligent Knowledge Graph-Based Directional Data Clustering and Feature Selection for Improved Education. Int. J. Recent Innov. Trends Comput. Commun. 2023 , 11 , 22–33. [ Google Scholar ] [ CrossRef ]
- Liu, C.; Zhang, J.; Zhang, H.; Li, X.; Zhang, E. Group Cooperative Teaching Design with Knowledge Graphs in Project-Driven Learning. Int. J. Inf. Commun. Technol. Educ. 2023 , 19 , 1–11. [ Google Scholar ] [ CrossRef ]
- Nguyen, H.D.; Truong, D.; Vu, S.; Nguyen, D.; Nguyen, H.; Tran, N.T. Knowledge management for information querying system in education via the combination of rela-ops model and knowledge graph. J. Cases Inf. Technol. 2023 , 25 , 1–17. [ Google Scholar ] [ CrossRef ]
- Nguyen, H.D.; Huynh, H.; Mai, T. Design an Ontology-based model for Intelligent Querying system in Mathematics Education. J. Interdiscip. Math. 2023 , 26 , 449–473. [ Google Scholar ] [ CrossRef ]
- Coccoli, M.; Torre, I.; Galluccio, I. User experience evaluation of Edurell interface for video augmentation. Multimed. Tools Appl. 2024 , 83 , 36695–36717. [ Google Scholar ] [ CrossRef ]
- Liu, J.Y.; Wang, F.; Ma, H.P.; Huang, Z.Y.; Liu, Q.; Chen, E.H.; Su, Y. A Probabilistic Framework for Temporal Cognitive Diagnosis in Online Learning Systems. J. Comput. Sci. Technol. 2023 , 38 , 1203–1222. [ Google Scholar ] [ CrossRef ]
- Agrawal, G.; Deng, Y.; Park, J.; Liu, H.; Chen, Y.C. Building knowledge graphs from unstructured texts: Applications and impact analyses in cybersecurity education. Information 2022 , 13 , 526. [ Google Scholar ] [ CrossRef ]
- Weichselbraun, A.; Waldvogel, R.; Fraefel, A.; van Schie, A.; Kuntschik, P. Building Knowledge Graphs and Recommender Systems for Suggesting Reskilling and Upskilling Options from the Web. Information 2022 , 13 , 510. [ Google Scholar ] [ CrossRef ]
- Li, N.; Shen, Q.; Song, R.; Chi, Y.; Xu, H. MEduKG: A deep-learning-based approach for multi-modal educational knowledge graph construction. Information 2022 , 13 , 91. [ Google Scholar ] [ CrossRef ]
- Keshan, N.; Fontaine, K.; Hendler, J.A. Semiautomated process for generating knowledge graphs for marginalized community doctoral-recipients. Int. J. Web Inf. Syst. 2022 , 18 , 413–431. [ Google Scholar ] [ CrossRef ]
- Yang, S.; Cai, X. Bilateral knowledge graph enhanced online course recommendation. Inf. Syst. 2022 , 107 , 102000. [ Google Scholar ] [ CrossRef ]
- Ezaldeen, H.; Bisoy, S.K.; Misra, R.; Alatrash, R. Semantics-aware context-based learner modelling using normalized PSO for personalized E-learning. J. Web Eng. 2022 , 21 , 1187–1224. [ Google Scholar ] [ CrossRef ]
- Wu, Z.; Jia, F. Construction and Application of a Major-Specific Knowledge Graph Based on Big Data in Education. Int. J. Emerg. Technol. Learn. 2022 , 17 , 64–79. [ Google Scholar ] [ CrossRef ]
- Zhong, M.; Ding, R. Design of a personalized recommendation system for learning resources based on collaborative filtering. Int. J. Circuits Syst. Signal Process. 2022 , 16 , 122–131. [ Google Scholar ] [ CrossRef ]
- Nafa, F.; Babour, A.; Melton, A. Prerequisite relations among knowledge units: A case study of computer science domain. Comput. Model. Eng. Sci. 2022 , 133 , 639–652. [ Google Scholar ] [ CrossRef ]
- Hou, Q. Design of a Visual Training System for Software Engineering Education Based on Knowledge Graphs. Int. J. Emerg. Technol. Learn. 2022 , 17 , 114–130. [ Google Scholar ] [ CrossRef ]
- Liu, P.; Cao, Y.; Wang, L. A Multimodal Fusion Online Music Education System for Universities. Comput. Intell. Neurosci. 2022 , 2022 , 6529110. [ Google Scholar ] [ CrossRef ]
- Badawy, A.; Fisteus, J.A.; Mahmoud, T.M.; Abd El-Hafeez, T. Topic extraction and interactive knowledge graphs for learning resources. Sustainability 2021 , 14 , 226. [ Google Scholar ] [ CrossRef ]
- Martin, A.J.; Dominic, M.M. Personalization of learning objects according to the skill set of the learner using knowledge graph. Turk. J. Comput. Math. Educ. 2021 , 12 , 3974–3987. [ Google Scholar ]
- Yang, Z.; Wang, Y.; Gan, J.; Li, H.; Lei, N. Design and research of intelligent question-answering (Q&A) system based on high school course knowledge graph. Mob. Netw. Appl. 2021 , 26 , 1884–1890. [ Google Scholar ]
- Hur, Y.; Jo, J. Development of Intelligent Information System for Digital Cultural Contents. Mathematics 2021 , 9 , 238. [ Google Scholar ] [ CrossRef ]
- Lv, P.; Wang, X.; Xu, J.; Wang, J. Intelligent personalised exercise recommendation: A weighted knowledge graph-based approach. Comput. Appl. Eng. Educ. 2021 , 29 , 1403–1419. [ Google Scholar ] [ CrossRef ]
- Xu, G.; Jia, G.; Shi, L.; Zhang, Z. Personalized course recommendation system fusing with knowledge graph and collaborative filtering. Comput. Intell. Neurosci. 2021 , 2021 , 9590502. [ Google Scholar ] [ CrossRef ]
- Zhang, Z. A method of recommending physical education network course resources based on collaborative filtering technology. Sci. Program. 2021 , 2021 , 9531111. [ Google Scholar ] [ CrossRef ]
- Cui, J.; Yu, S. Fostering deeper learning in a flipped classroom: Effects of knowledge graphs versus concept maps. Br. J. Educ. Technol. 2019 , 50 , 2308–2328. [ Google Scholar ] [ CrossRef ]
- Telnov, V.; Korovin, Y. Semantic web and knowledge graphs as an educational technology of personnel training for nuclear power engineering. Nucl. Energy Technol. 2019 , 5 , 273–280. [ Google Scholar ] [ CrossRef ]
- Chi, Y.; Qin, Y.; Song, R.; Xu, H. Knowledge graph in smart education: A case study of entrepreneurship scientific publication management. Sustainability 2018 , 10 , 995. [ Google Scholar ] [ CrossRef ]
- Chen, P.; Lu, Y.; Zheng, V.W.; Chen, X.; Yang, B. Knowedu: A system to construct knowledge graph for education. IEEE Access 2018 , 6 , 31553–31563. [ Google Scholar ] [ CrossRef ]
- Shen, T.; Nagai, Y.; Zhao, J.; Shen, T. Hotspots and trends in knowledge graph and concept generation based on bibliometric analysis. In Proceedings of the 2020 International Conference on Intelligent Design (ICID), Xi’an, China, 11–13 December 2020; IEEE: Piscataway, NJ, USA, 2020; pp. 124–127. [ Google Scholar ]
- State Council. New Generation of Artificial Intelligence Development Plan. 2017. Available online: https://flia.org/wp-content/uploads/2017/07/A-New-Generation-of-Artificial-Intelligence-Development-Plan-1.pdf (accessed on 25 May 2024).
- Grad-Gyenge, L.; Kiss, A.; Filzmoser, P. Graph embedding based recommendation techniques on the knowledge graph. In Proceedings of the Adjunct Publication of the 25th Conference on User Modeling, Adaptation and Personalization, Bratislava, Slovakia, 9–12 July 2017; pp. 354–359. [ Google Scholar ]
Click here to enlarge figure
Ref. | Objectives | Application Categories | Knowledge Graph Resources | Technical Means | Pedagogies | Limitations |
---|---|---|---|---|---|---|
[ ] | Enhance personalised educational content recommendation. | Educational recommendation | Learning resources, learner attributes, and preferences | Cosine similarity | Personalised learning | |
[ ] | Facilitate question generation for instructors. | Question generation | Textbooks | Semantic networks | N/A | |
[ ] | Find a meaningful knowledge-concept path. | Concept instruction | Student log data | Feature selection using Elastic Net (LASSO) + RF algorithm | Personalised learning | |
[ ] | Empower a question-answering Chabot to respond to queries. | Question answering | Wikipedia | The Wit.ai NLP model | N/A | |
[ ] | Enable semantic querying, predictive modelling, and reasoning for student behaviour analysis. | Prediction of educational outcomes | LMSs (Moodle, COCO Udemy, Open University) | OWL 2 ontology | N/A | |
[ ] | Support collaborative knowledge building. | Educational recommendations and educational assessment and feedback | Online discussion transcripts | BERT-BiLSTM-CRF | Collaborative learning | |
[ ] | Improve collaborative learning performance. | Educational recommendations and educational assessment and feedback | Online discussion transcripts | BERT-BiLSTM-CRF and BERT-Random Forest | Collaborative learning | |
[ ] | Promote knowledge elaboration. | Educational assessment and feedback | Online discussion transcripts | BERT-BiLSTM-CRF | Collaborative learning | |
[ ] | Predict students’ mastery of knowledge based on their learning activity. | Prediction of educational outcomes | English problem-solving record data from EdNet and ASSIST2017 | Graph neural network | Personalised learning | |
[ ] | Improve the recommendation of learning activities. | Educational recommendations | Textbooks, student learning activities | Similarity measures | Personalised learning | |
[ ] | Help students find out courses and knowledge related to graduation requirements. | Learning resources searching | Syllabi, teachers’ lesson plans, and webpages | Ontology construction, Large Language Models | Personalised learning | |
[ ] | Offer learners a semantic representation of domain concepts. | Concept instruction | Learning materials, Wikipedia, and Dbpedia | SqueezeBERT, word and sentence embeddings | Personalised learning | |
[ ] | Visualise the knowledge construction process. | Concept instruction | Lecture slides, Wikipedia, and videos | N/A | Immersive learning, collaborative learning | |
[ ] | Identify students at risk of failing a course. | Prediction of educational outcomes | Course information, student historical features and performance | Ontology mapping | N/A | |
[ ] | Help learners efficiently memorise and learn concepts. | Concept instruction | Textbooks, Baidupedia, and students’ classroom responses (collected using sensors) | Graph convolutional network (GCN), BiLSTM-CRF | Personalised learning | |
[ ] | Support knowledge sharing and learning in groups. | Concept instruction | DBpedia, Wikidata, and YAGO3 | Embedding-based knowledge map fusion algorithm | Collaborative learning | |
[ ] | Predict and analyse student educational outcomes. | Prediction of educational outcomes | The Linked Data for Education dataset (learning resources), the Open Academic Graph dataset, DBpedia, and MOOC platforms | Feature selection using LSTM_GOA algorithm | N/A | |
[ ] | Show the logic between knowledge. | Concept instruction | Individually constructed knowledge graphs by teachers and students | Knowledge fusion | Project-based learning, collaborative learning | |
[ ] | Assist students in reviewing lectures and comprehending course material. | Learning resources searching | Textbooks | Ontology Rela-Ops model | N/A | |
[ ] | Represent relations of knowledge components and retrieve contents of queries. | Learning resources searching | Textbooks and workbooks | Ontology Rela-Ops model | N/A | |
[ ] | Enables learners to perform non-linear navigation of learning contents. | Concept instruction | Video lectures | Speech-to-text techniques and semantic analysis | N/A | |
[ ] | Help students quickly and systematically grasp the framework and key content of video lectures. | Concept instruction | Video lectures | BERT, name entity recognition, and YOLOv3 | N/A | |
[ ] | Estimate students’ proficiency in knowledge concepts. | Prediction of educational outcomes | Online tutoring system and E-learning platform | Recurrent neural network | N/A | |
[ ] | Improve the curriculum system in higher education institutions. | Curriculum design and management | Course syllabi in the current university and the benchmarking top universities, teacher information | Latent Dirichlet Allocation | N/A | |
[ ] | Construct meaningful connections between social media and formal learning. | Curriculum design and management | Course information, social media (Facebook, Twitter) | Semantic mediawiki | Collaborative learning | |
[ ] | Provide concept visualisation and promote cognitive engagement. | Concept instruction | Course materials | Named-entity recognition and NLP | Problem-based learning | |
[ ] | Provide semantic search for reskilling and upskilling options. | Educational recommendations | Education providers’ Webpages | Resource Description Framework, slot filling | N/A | |
[ ] | Manage and present various modes of educational resources. | Knowledge management | Online education resources (e.g., Baidu entries), offline education resources (e.g., PowerPoints and class audios) | BERT-BiLSTM-CRF | N/A | |
[ ] | Minimise the time instructors have to spend looking for teaching material. | Educational recommendations | DBpedia Knowledge Graphs, instructor’s teaching plans | Semantic similarity | N/A | |
[ ] | Provide a comprehensive resource for students. | Learning resources searching | National Science Foundation, Survey of Earned Doctorates Restricted Data Analysis System, and Wikidata | Semantic Extract Transform and Load-er | N/A | |
[ ] | Enhance online course recommendations to address user characteristics. | Educational recommendations | Two public datasets (Movielens-20M, Book-Crossing) and an industrial dataset | Graph convolutional network, Collaborative filtering algorithms | Personalised learning | |
[ ] | Predict appropriate resources with the highest ranking linked to the learner’s interests. | Educational recommendations | E-content (e.g., E-Library, Coursera), user selections out of these materials | NLP | Personalised learning | |
[ ] | Help students build complex knowledge structures. | Concept instruction | Educational resources, learning behaviour | Node feature extraction method | N/A | |
[ ] | Help students access learning resources accurately and efficiently. | Educational recommendations | Learning behaviours, course information | Collaborative filtering algorithms, similarity measures | Personalised learning | |
[ ] | Present knowledge units in a semantically well-organised manner. | Concept instruction | Textbooks | NLP | N/A | |
[ ] | Communicate knowledge logically and coherently. | Concept instruction | N/A | Entity extraction, relation extraction, and attribute extraction | N/A | |
[ ] | Improve the course recommendation accuracy for music education | Educational recommendations | Audio, sheet music, chants, and metadata | Resource Description Framework | Personalised learning | |
[ ] | Enable students to seek out and examine educational resources that align with their interests. | Learning resources searching | Textbooks | Wikipedia Miner, NLP | Networked learning | |
[ ] | Provide personalised learning content according to the skill set of learners. | Educational recommendations | Learning assessment, course materials | Named-entity recognition | Personalised learning | |
[ ] | Predict students’ learning behaviour in order to provide feedback on the teaching effect. | Question answering | Subject materials and syllabi | Conditional Random Fields, TF-IDF | Problem-based learning, cognitive learning | |
[ ] | Effectively provide information in response to searches for content that is useful to learners. | Learning resources searching | Webpages | Bi-LSTM model | N/A | |
[ ] | Recommend personalised exercises to students in an appropriate order. | Educational recommendations | Textbook, Wikipedia, and testing behaviour of students | Collaborative filtering | Personalised learning | |
[ ] | Provide personalised content for learners. | Educational recommendations | N/A | Collaborative filtering | Personalised learning | |
[ ] | Effectively recommend learning resources to learners. | Educational recommendations | Webpages | Collaborative filtering | Personalised learning | |
[ ] | Support students in constructing and expanding their knowledge structure. | Concept instruction | Student-generated knowledge graphs | N/A | Collaborative learning | |
[ ] | Provide a well-structured overview of knowledge in nuclear power engineering. | Concept instruction | DBpedia | Semantic Similarity Measure, Resource Description Framework | N/A | |
[ ] | Enhance scientific retrieval efficiency. | Knowledge management | Three scientific databases: Web of Science, Engineering Village, and EBSCO | Machine-learning algorithms | Problem-based learning | |
[ ] | Support personalised teaching services and adaptive learning solutions. | Concept instruction | Standard curriculum data and learning assessment data | Gated recurrent unit network, probabilistic association rule mining algorithm | Personalised learning |
The statements, opinions and data contained in all publications are solely those of the individual author(s) and contributor(s) and not of MDPI and/or the editor(s). MDPI and/or the editor(s) disclaim responsibility for any injury to people or property resulting from any ideas, methods, instructions or products referred to in the content. |
Share and Cite
Qu, K.; Li, K.C.; Wong, B.T.M.; Wu, M.M.F.; Liu, M. A Survey of Knowledge Graph Approaches and Applications in Education. Electronics 2024 , 13 , 2537. https://doi.org/10.3390/electronics13132537
Qu K, Li KC, Wong BTM, Wu MMF, Liu M. A Survey of Knowledge Graph Approaches and Applications in Education. Electronics . 2024; 13(13):2537. https://doi.org/10.3390/electronics13132537
Qu, Kechen, Kam Cheong Li, Billy T. M. Wong, Manfred M. F. Wu, and Mengjin Liu. 2024. "A Survey of Knowledge Graph Approaches and Applications in Education" Electronics 13, no. 13: 2537. https://doi.org/10.3390/electronics13132537
Article Metrics
Article access statistics, further information, mdpi initiatives, follow mdpi.
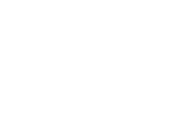
Subscribe to receive issue release notifications and newsletters from MDPI journals
Adopting Knowledge graph in STEM pre-service teacher education: a mix-method study based on learning behaviors and technology acceptance
New citation alert added.
This alert has been successfully added and will be sent to:
You will be notified whenever a record that you have chosen has been cited.
To manage your alert preferences, click on the button below.
New Citation Alert!
Please log in to your account
Information & Contributors
Bibliometrics & citations, recommendations, building pre-service teacher interest in computer science education through mentoring experiences (abstract only).
K-12 teachers have an important role to play in introducing children to computer science. However, most teachers lack explicit training in computer science. This project explored questions related to the experiences of pre-service teachers as they ...
Blended learning at pre-service teacher education in Turkey: A systematic review
The literature shows an increase in blended learning implementations (Ný=ý74) at faculties of education in Turkey whereas pre-service and in-service teachers' ICT competencies have been identified as one of the areas where they are in need of ...
Technology workshops by in-service teachers for pre-service teachers
This project was an initiative through university courses to have graduate in-service teachers, who have learned the use of technology for classroom instruction, offer workshops to undergraduate pre-service teachers. The goals of the project were two-...
Information
Published in.

Association for Computing Machinery
New York, NY, United States
Publication History
Permissions, check for updates, author tags.
- knowledge graph
- pre-service teacher education
- Research-article
- Refereed limited
Funding Sources
- the Major Project of Social Science in South China Normal University
- Ministry of Education in China Project of Humanities and Social Sciences
Contributors
Other metrics, bibliometrics, article metrics.
- 0 Total Citations
- 3 Total Downloads
- Downloads (Last 12 months) 3
- Downloads (Last 6 weeks) 3
View Options
Login options.
Check if you have access through your login credentials or your institution to get full access on this article.
Full Access
View options.
View or Download as a PDF file.
View online with eReader .
HTML Format
View this article in HTML Format.
Share this Publication link
Copying failed.
Share on social media
Affiliations, export citations.
- Please download or close your previous search result export first before starting a new bulk export. Preview is not available. By clicking download, a status dialog will open to start the export process. The process may take a few minutes but once it finishes a file will be downloadable from your browser. You may continue to browse the DL while the export process is in progress. Download
- Download citation
- Copy citation
We are preparing your search results for download ...
We will inform you here when the file is ready.
Your file of search results citations is now ready.
Your search export query has expired. Please try again.

IMAGES
COMMENTS
The promise of technology in the classroom is great: enabling personalized, mastery-based learning; saving teacher time; and equipping students with the digital skills they will need for 21st-century careers. Indeed, controlled pilot studies have shown meaningful improvements in student outcomes through personalized blended learning. 1 John F. Pane et al.,
addresses the use of technology in education around the world through the lenses of relevance, equity, scalability and sustainability. It argues that education systems should always ensure that learners' interests are placed at the center and that digital technologies are used to support an education based on human interaction rather than ...
Education Technology in 10 Charts. 1. Education will be a $7T+ industry by 2025. Education is one of the world's single largest industries, making up more than 6% of GDP. We expect total global expenditure from governments, companies and consumers together to reach $7.3T by 2025.
Arianna Prothero covers technology, student well-being, and the intersection of the two for Education Week. A version of this article appeared in the April 05, 2023 edition of Education Week as ...
About 63 percent of K-12 teachers use technology in the classroom daily.A new survey from the University of Phoenix College of Education found that daily classroom tech use is up from 55 percent in 2016.. While a whopping 86 percent of educators indicate they use laptops in classrooms the most, the use of other tools is on the rise. About 58 percent of teachers use educational apps, 41 ...
To address this need, J-PAL North America recently released a new publication summarizing 126 rigorous evaluations of different uses of education technology. Drawing primarily from research in developed countries, the publication looks at randomized evaluations and regression discontinuity designs across four broad categories: (1) access to ...
There is little robust evidence on digital technology's added value in education. Technology evolves faster than it is possible to evaluate it: Education technology products change every 36 months, on average. Most evidence comes from the richest countries. In the United Kingdom, 7% of education technology companies had conducted randomized
It provides the mid-term assessment of progress towards SDG 4, which was summarized in a brochure and promoted at the 2023 SDG Summit. The 2023 GEM Report and 200 PEER country profiles on technology and education were launched on 26 July. A recording of the global launch event can be watched here and a south-south dialogue between Ministers of ...
The Education Technology Use in Schools report reflects the views of students, teachers, principals and district administrators on digital learning tools. Including the perspectives of those most familiar with the impact of these tools in schools can lend important clarity for those seeking
As of 2023, many higher education courses in the U.S. remain at least partially online and in K-12 schools, the average number of edtech tools accessed per school district reached over 2,500, a ...
Survey results from public school teachers suggest that educational technology professional development is reasonably widespread. In a 2009 survey, only 18% of teachers reported having completed no educational technology training over the previous year; the majority (53%) reported completing 1 to 8 hours training (Gray et al., 2010). Further, they
In the United States, technology exacerbated what is now the largest income gap in the world. Between the years 2000 and 2009, average employees gained 2.3% in their wages, managers gained 9% in their wages and board members saw their wages skyrocket by 19%. This led to record levels of income inequality. One product of this technological boom ...
Existing technology like videogames can aid in the development of education. Strategy games such as Sid Meier's Civilization V, teach an understanding of the intricate relationships among the geographical, historical and economic systems that shape civilizations.Sandbox games like Minecraft can boost SEL skills like collaboration, creativity and problem-solving.
Educational technology Exhibit 1 of 6 Some student-based technologies are associated with lower student outcomes. Impact of using technology in the classroom, points change in PISA score between "No" and "Yes and use technology in classroom" in a regression (40 points ≈ 1 year of learning)¹ Data projector Internet-connected computer ...
In 2016, self-paced elearning industry made up $46.67 billion of the global market share and, by 2022, that number is projected to exceed $243 billion ( Statista ). As of August 2020, the global education and training market is expected to reach $7.3 trillion by 2025 ( Holon IQ ). In 2019, the global education technology market was estimated at ...
This number was around 244 million in 2023. Many children who attend primary school drop out and do not attend secondary school. That means many more children or adolescents are missing from secondary school than primary education. Globally, until recently, boys were more likely to attend school than girls.
Today, the U.S. Department of Education's Office of Educational Technology (OET) released a new report, "Artificial Intelligence (AI) and the Future of Teaching and Learning: Insights and Recommendations" that summarizes the opportunities and risks for AI in teaching, learning, research, and assessment based on public input. This report is part of the Biden-Harris Administration's ongoing ...
As part of a wider partnership with the Department for Education to support more effective use of technology, Nesta and SchoolDash have collaborated to analyse usage data in England from three EdTech industry partners, spanning from January 2020 (before the first national school closures) to March 2021. We set out to understand more about how children's experience of the pandemic and EdTech ...
The Timeline of Educational Technology in Schools Infographic shows how educational technology has evolved in schools over the past 114 years. 1984 - The Apple Macintosh computer is developed. The ration of computers to students in US schools is 1 - 92. 2001 - 80% of schools with internet access offered professional development training for ...
This editorial continues to landscape the trends and popular educational technology topics for 2023. We used the public internet data mining approach from previous years (Allman et al., 2023b; Kimmons, 2020; Kimmons & Rosenberg, 2022; Kimmons et al., 2021).This year, we extracted and analyzed data from the Scopus research article database, K-12 school and district Facebook pages, and the open ...
Children and technology - Statistics & Facts. With technology playing a major role in people's lives, children are becoming exposed and learning how to use it at increasingly younger ages. In ...
This paper presents a comprehensive survey of knowledge graphs in education. It covers the patterns and prospects of research in this area. A total of 48 relevant publications between 2011 and 2023 were collected from the Web of Science, Scopus, and ProQuest for review. The findings reveal a sharp increase in recent years in the body of research into educational knowledge graphs which was ...
Modern Educational Technology 29, 12 (2019), 89-95. Google Scholar [6] ... Knowedu: A system to construct knowledge graph for education. Ieee Access 6, (2018), 31553-31563. Google Scholar [12] Furong Dang, Jintao Tang, and Shasha Li. 2019. MOOC-KG: A MOOC knowledge graph for cross-platform online learning resources. In 2019 IEEE 9th ...