Forecasting the burden of type 2 diabetes in Singapore using a demographic epidemiological model of Singapore
Affiliations.
- 1 Saw Swee Hock School of Public Health, National University of Singapore and National University Health System , Singapore.
- 2 Saw Swee Hock School of Public Health, National University of Singapore and National University Health System , Singapore ; Department of Statistics and Applied Probability , Faculty of Science, National University of Singapore , Singapore.
- 3 Saw Swee Hock School of Public Health, National University of Singapore and National University Health System , Singapore ; Division of Endocrinology , National University Hospital and National University Health System , Singapore ; Yong Loo Lin School of Medicine, National University of Singapore and National University Health System , Singapore.
- 4 Saw Swee Hock School of Public Health, National University of Singapore and National University Health System , Singapore ; Ministry of Health , Singapore.
- 5 Saw Swee Hock School of Public Health, National University of Singapore and National University Health System , Singapore ; Department of Statistics and Applied Probability , Faculty of Science, National University of Singapore , Singapore ; Life Sciences Institute, National University of Singapore , Singapore.
- 6 Saw Swee Hock School of Public Health, National University of Singapore and National University Health System , Singapore ; Department of Ophthalmology , Yong Loo Lin School of Medicine, National University of Singapore and National University Health System , Singapore ; Singapore Eye Research Institute , Singapore ; Center for Quantitative Medicine, Duke-NUS Graduate Medical School , Singapore.
- 7 Department of Ophthalmology , Yong Loo Lin School of Medicine, National University of Singapore and National University Health System , Singapore ; Singapore Eye Research Institute , Singapore.
- 8 Saw Swee Hock School of Public Health, National University of Singapore and National University Health System , Singapore ; Department of Statistics and Applied Probability , Faculty of Science, National University of Singapore , Singapore ; Program in Health Services and Systems Research, Duke-NUS Graduate Medical School , Singapore ; Yale-NUS College , Singapore.
- PMID: 25452860
- PMCID: PMC4212579
- DOI: 10.1136/bmjdrc-2013-000012
Objective: Singapore is a microcosm of Asia as a whole, and its rapidly ageing, increasingly sedentary population heralds the chronic health problems other Asian countries are starting to face and will likely face in the decades ahead. Forecasting the changing burden of chronic diseases such as type 2 diabetes in Singapore is vital to plan the resources needed and motivate preventive efforts.
Methods: This paper describes an individual-level simulation model that uses evidence synthesis from multiple data streams-national statistics, national health surveys, and four cohort studies, and known risk factors-aging, obesity, ethnicity, and genetics-to forecast the prevalence of type 2 diabetes in Singapore. This comprises submodels for mortality, fertility, migration, body mass index trajectories, genetics, and workforce participation, parameterized using Markov chain Monte Carlo methods, and permits forecasts by ethnicity and employment status.
Results: We forecast that the obesity prevalence will quadruple from 4.3% in 1990 to 15.9% in 2050, while the prevalence of type 2 diabetes (diagnosed and undiagnosed) among Singapore adults aged 18-69 will double from 7.3% in 1990 to 15% in 2050, that ethnic Indians and Malays will bear a disproportionate burden compared with the Chinese majority, and that the number of patients with diabetes in the workforce will grow markedly.
Conclusions: If the recent rise in obesity prevalence continues, the lifetime risk of type 2 diabetes in Singapore will be one in two by 2050 with concomitant implications for greater healthcare expenditure, productivity losses, and the targeting of health promotion programmes.
Keywords: Adult Diabetes; Demographics; Simulation; Statistical Methods.
An official website of the United States government
Official websites use .gov A .gov website belongs to an official government organization in the United States.
Secure .gov websites use HTTPS A lock ( Lock Locked padlock icon ) or https:// means you've safely connected to the .gov website. Share sensitive information only on official, secure websites.
- Publications
- Account settings
- Advanced Search
- Journal List


Recognition of diabetes and sociodemographic predictors: results of a cross-sectional nationwide population-based survey in Singapore
Kumarasan roystonn, jue hua lau, pv asharani, fiona devi siva kumar, peizhi wang, chee fang sum, eng sing lee, siow ann chong, mythily subramaniam.
- Author information
- Article notes
- Copyright and License information
Correspondence to Dr Kumarasan Roystonn; [email protected]
Corresponding author.
Series information
Original research
Received 2021 Feb 19; Accepted 2022 Feb 8; Collection date 2022.
This is an open access article distributed in accordance with the Creative Commons Attribution Non Commercial (CC BY-NC 4.0) license, which permits others to distribute, remix, adapt, build upon this work non-commercially, and license their derivative works on different terms, provided the original work is properly cited, appropriate credit is given, any changes made indicated, and the use is non-commercial. See: http://creativecommons.org/licenses/by-nc/4.0/ .
To assess recognition of diabetes among a nationally representative multiethnic sample of Singapore’s general public. Also, to explore the sociodemographic predictors associated with the ability to correctly recognise diabetes.
Design and setting
This was a cross-sectional vignette-based survey. Respondents were instructed to read the vignette, then answer the open-ended question, ‘What do you think the person in the vignette is suffering from?’
Participants
A sample of 2895 household residents aged 18 years and above, of which 436 were persons with diabetes.
82.7% could correctly recognise diabetes in the vignette. Overall, recognition was significantly higher among respondents aged 35–49 years (OR 1.85, 95% CI 1.15 to 2.98), 50–64 years (OR 2.06, 95% CI 1.19 to 3.56), ethnic Malays (OR 1.39, 95% CI 1.02 to 1.89) and persons with diabetes (OR 2.64, 95% CI 1.38 to 5.08). By contrast, male (OR 0.64, 95% CI 0.46 to 0.90), ethnic Others (OR 0.59, 95% CI 0.37 to 0.93) and the unemployed (OR 0.48, 95% CI 0.25 to 0.92) were significantly associated with poor recognition of diabetes.
Overall public recognition of diabetes was high, but the significant gaps in knowledge in certain demographic groups were of concern. Public health interventions aimed at preventing and controlling diabetes should continue to target all members of the population with accurate and appropriate information. Ongoing efforts of diabetes awareness and screening programmes need to be improved, particularly for young adults, males and the unemployed.
Keywords: public health, diabetes & endocrinology, qualitative research, general diabetes
Strengths and limitations of this study.
This is the first study to explore recognition of diabetes in a representative sample of the general adult population in Singapore.
Vignettes were developed in consultation with clinicians and people with diabetes.
Use of a vignette-based design and structured questionnaire ensures reproducibility of the study.
People who were hospitalised, institutionalised or uncontactable during the period of the study were excluded from the survey.
The vignette might have facilitated diabetes identification by clearly describing persons with prototypical symptoms and functional changes, which may be different from real-life scenarios.
Introduction
Diabetes mellitus is a prevalent chronic condition which results in substantial target organ disease and significant complications including blindness, kidney failure, stroke and coronary heart disease. In 2017, it was estimated that there were 451 million adults (8.5%) worldwide with diabetes, with an associated cost of US$850 billion comprising direct and indirect medical costs as well as informal care. 1 2 There is a growing diabetes epidemic in the Asia-Pacific region with more than 50% of people with diabetes being undiagnosed. 2–4 To date, the International Diabetes Federation has estimated that Asia accounts for 60% of the world's diabetic population. 3 In Singapore, as in many developed countries, diabetes is a major public health concern. 5 The cost of diabetes per patient was found to be US$1575.6, which was higher than the costs reported in other Asian countries. 6 In 2017, diabetes became the seventh leading cause of morbidity and premature death in Singapore. 7 An epidemiological study of the resident general population, found the prevalence of diabetes to be approximately 11.3% (one in nine) in those aged 18 years and above with higher rates among men than women. 8 The national health survey data also revealed that 51.4% of individuals in Singapore were unaware that they had diabetes.
Diabetes literacy is herein defined as the knowledge and beliefs regarding diabetes mellitus that aid recognition, management or prevention of this physical disease. This is adapted from ‘mental health literacy’, a term by Jorm 9 with widespread usage that refers to ‘knowledge and beliefs about mental disorders which aid recognition, management or prevention’. Poor knowledge of the signs and symptoms of diabetes may be a particularly important barrier to help-seeking behaviour for this chronic disease. Failure to recognise signs and symptoms associated with diabetes could lead to delays in timely medical attention. 10 Accurate recognition and labelling of the disease are paramount to the prevention of diabetes to facilitate early and appropriate help-seeking to improve long-term health outcomes.
Recent qualitative studies exploring health literacy in diabetes in Asian cultures found that culture shaped understanding and experiences of health literacy. 11–13 There are significant ethnic differences in how physical health conditions are recognised and labelled. For example, in a study with ethnic Chinese, the researcher found that a majority held on to cultural beliefs that ageing or a ‘weak body’ was the cause for diabetes. 14 Much of the available literature tends to focus on the knowledge of patients with diabetes. Few reports are available on evaluating diabetes literacy in the general population, even though diabetes is increasingly becoming a major threat to global public health. A general population study of Australian adult residents found that only 14%–29% of the population were able to correctly recognise diabetes symptoms and warning signs. 15 In the UK, 59.4% of the public were able to correctly identify diabetes symptoms and deemed to have adequate awareness of the disease. 16 On the other hand, research conducted in Sri Lanka reported that 77% of the general public surveyed could correctly recognise diabetes. 17 In Singapore, the only local study evaluating general public’s knowledge of diabetes reported that about 60% were able to recognise the symptoms and complications of the disease. 18 However, recent literature remains sparse and studies were often with smaller samples and the findings are not a valid representation of the overall population of the country.
Singapore is a multicultural, multiethnic and multilingual country where the major ethnic groups of Chinese, Malays and Indians still maintain somewhat separate cultural identities. The population of Singapore comprises about 5.9 million people, of which 3.9 million are Singapore citizens or permanent residents. The resident population comprises four main ethnic groups—Chinese (74.3%), Malay (13.3%), Indian (9.1%) and Others (3.3%). 19 Therefore, Singapore is an ideal location to study diabetes literacy among the multiethnic Asian population. The purpose of this population-based diabetes literacy study was to specifically evaluate the proportion of the Singapore public who are able to recognise diabetes correctly and the factors associated with it, in a nationally representative multiethnic sample. The results of the study may have strategic implications for the reduction of ethnic or socioeconomic disparities in diabetes detection, and better inform future health promotion campaigns.
Setting and study design
This nationwide cross-sectional study of the Singapore population includes citizens and permanent residents aged 18 and above, belonging to the four major ethnic groups, who were literate in English, Chinese, Malay or Tamil and living in Singapore at the time of survey. All residents who were uncontactable due to incomplete or incorrect addresses and those living outside of the country were excluded from the study. The overall response rate of the study was 66.2%. Written informed consent was obtained from all respondents 21 years of age and above, as well as from parents or guardians of those aged 18–20 years.
Sample size calculation and sampling
Statistical power calculations for binary proportions post-adjusted for design effects determined sample sizes for the population prevalence estimate, as well as for subgroup (age and ethnicity) estimates, with an overall precision of 2.5%. 20 Using 20% as a prevalence estimate based on previously reported prevalence rates of diabetes knowledge in Singapore, 18 a total sample size of 3000 was estimated to be adequate to determine the general knowledge of diabetes in the population. The margin of error for the overall prevalence estimate was found to be 2.5%, while that of the subgroups by age and ethnicity ranged from 4.5% to 5%. The relative standard error (SE) was found to be substantially below the acceptable range (<30%), ranging from 2.1% to 4.2%. Further details of the sampling strategy and processes are published elsewhere. 20
The sampling frame was an administrative database of all residents in Singapore from which the sample was derived. A disproportionate stratified sampling design was used in the study to randomly select a probability sample, based on 12 strata according to ethnicity (Chinese, Indian, Malay) and age groups (18–34, 35–49, 50–64, 65 and above). The study oversampled those of Malay and Indian ethnicities, as well as residents aged 65 and above in order to ensure sufficient sample size and to improve the reliability of parameter estimates for these population subgroups.
Data collection
Data were collected in face-to-face interviews by trained interviewers, using the computer assisted personal interviews on handheld tablets. For quality assurance, at least 10% of the completed interviews were validated through face-to-face and telephone follow-up.
Questionnaire
A structured questionnaire was used to obtain sociodemographic information including age, gender, ethnicity, marital status, personal income, educational and employment status, and self-reported diagnosis of diabetes. The study was introduced as an investigation of Singapore residents’ knowledge, attitudes and practices of a ‘chronic physical condition’. This was done so as to not influence respondents’ responses to the vignette presented at the beginning of the interview. The actual disease of interest, diabetes, was only revealed after the vignette section.
Vignette adaptation
Respondents were presented with a hypothetical vignette describing a person with diabetes mellitus. The vignette was developed and refined by the researchers in consultation with experienced clinicians specialising in diabetes care. The vignette length was approximately 110–120 words and described classic and common symptoms of the disease. Presented in English, Chinese, Malay or Tamil, the vignette was phrased with simple laymen’s terms. Further incorporating elements of the local context such as descriptions of the character’s background and home functioning, facilitated the development of a vignette storyline that was natural and relatable. 21 22 The vignette also described a person of the same gender and ethnicity as the respondent. For instance, a Chinese male participant was presented a vignette about Mr Tan (see online supplemental appendix A ). Respondents were asked what they thought the person described in the vignette was suffering from and to name the condition associated with the vignette description (free response). They were asked to base their considerations on the available information only. The response was coded as correct if the participant was able to correctly label the condition. In cases where the response was a near approximation of the correct answer, three of the investigators including the first author (AP, KR and MS) would come to a consensus on how that response should be coded.
bmjopen-2021-050425supp001.pdf (38.5KB, pdf)
Vignette translation and cognitive testing
The translation procedure undertaken was aimed at achieving conceptual equivalence using an adapted four-step process from WHO: (1) forward translation: the vignette and follow-up questions were translated into the three local languages—Chinese, Malay and Tamil using a professional translating firm, (2) expert panel review: which involved a critical evaluation of expert panel recommendations on issues identified with translations (3) pre-testing and cognitive interviews (CI): further CI were undertaken, with 25 respondents from diverse age groups, ethnicity, gender, and socioeconomic (or education attainment) status. Trained CI systematically probed respondents on what they thought the vignette was about, what came to their mind when they were presented a particular phrase or term and were asked how they decided on their response. Words or expressions that were not easily understood, or deemed offensive or unacceptable were highlighted to interviewers, and where alternative words or expressions existed, respondents were asked which of the alternatives better conformed to their usual language. (4) The development of final translations was achieved after minor changes based on the information gathered from the CI.

Statistical analyses
The survey sample was weighted by age and ethnicity to match the Singapore resident population so that the results could be generalised. Weighted mean and SE of the mean were calculated for continuous variables, and frequencies and percentages for categorical variables. Descriptive statistics were performed to establish the prevalence of diabetes literacy as well as to describe sociodemographic characteristics of the study sample. Univariate analyses (t-test or χ 2 test) was used to investigate differences among age groups (18–34=1; 35–49=2; 50–64=3; 65 and above=4), gender (female=1; male=2), ethnicity (Chinese=1; Malay=2; Indian=3; Others=4), education (degree and above=1; primary and below=2; secondary=3; preuniversity/junior college=4; vocational institute/ITE=5; diploma=6), employment (employed=1; economically inactive*=2; unemployed=3), income (in SGD) (below 2000=1; 2000–5999=2; 6000 and above=3) and diabetes diagnosis (no=1; yes=2). Categories coded as 1 were set as the reference category for all variables. A logistic regression analysis using survey weights to account for complex survey design was conducted to determine the sociodemographic variables significantly associated with correct recognition of diabetes. The level of statistical significance was set at p<0.05 using two-sided tests.
Patient and public involvement
Cognitive testing of survey questionnaires involved diabetic patients and members of the public to improve the quality of the questionnaire and to adapt it for local population use. Patients and/or the public were not involved in the recruitment, conduct, reporting and dissemination plans of this research.
The demographics of this study population are shown in table 1 . The survey data include 2895 respondents. The mean age of respondents was 45.8 years and 51.6% of the respondents were female. Majority (75.8%) were Chinese, 12.7% were Malays, 8.6% were Indians and 2.9% were from the ethnic group, Others. For education level, 29.5% of the respondents were university graduates, 20.3% of the respondents had completed secondary education and 20.4% had primary education or less.
Sample characteristics (n=2895)
Frequencies and percentages may not tally to 100% due to missing data.
*Economically inactive includes retired, homemaker, student and the physically disabled.
ITE, Institute of Technical Education.
Figure 1 presents the percentage of respondents endorsing each category with respect to recognition of the vignette. In total, 82.7% (n=2418) of the respondents from the general population correctly identified the disease from the vignette, of which 89.9% (n=2036) did not have a diabetes diagnosis. While, 0.7% (n=23) were found to have partly correct recognition (eg, mislabelling and referring to diabetes as ‘high blood sugar’). The 23 responses were as such, included under ‘correct recognition’ in regression analysis. About 7.7% (n=220) incorrectly recognised the condition as other medical problems, 2.9% (n=75) incorrectly recognised the condition as ageing, 1.0% (n=33) of the respondents mislabelled the disease as a non-medical problem, and 4.9% (n=126) did not provide answers, responding with ‘don’t know’ or ‘not sure’.
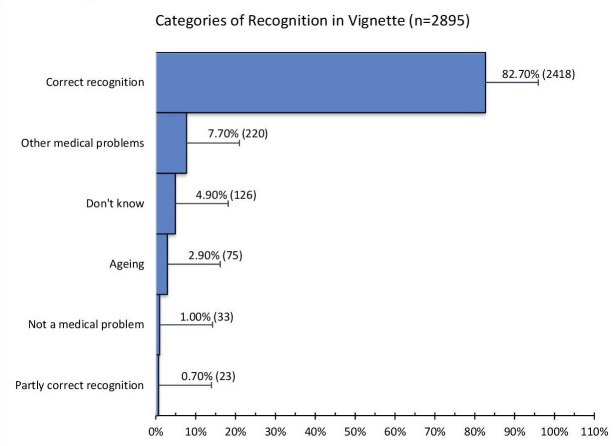
Weighted percentages of respondents by categories of recognition of diabetes. Error bar represents SE.
Online supplemental appendix B presents the weighted percentages of sociodemographic factors of respondents with incorrect recognition of diabetes. Among the 454 respondents who incorrectly recognised diabetes, the majority were male (56.1%, n=262), aged 18–34 years (40.4%, n=172), married (54.4%, n=253) and an equal proportion of them had primary education and below (22.8%, n=119) or had attained a university degree and above (22.8%, n=86); and about 4.3% (n=31) of the respondents diagnosed with diabetes, were unable to recognise the disease (see figure 2 ).
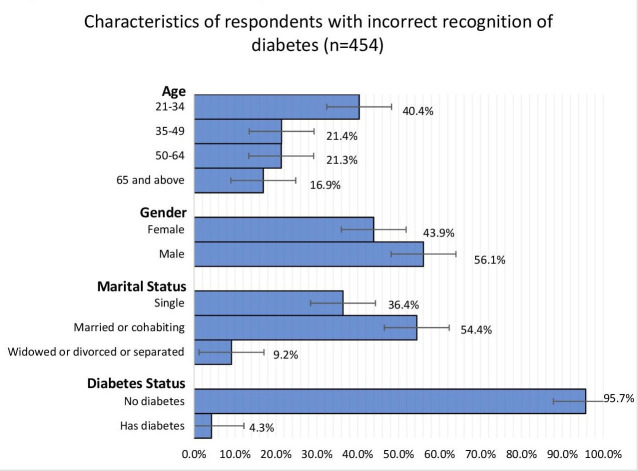
Weighted percentages of respondents by sociodemographic factors for incorrect recognition of diabetes. Error bar represents SE.
bmjopen-2021-050425supp002.pdf (19.7KB, pdf)
Sociodemographic factors associated with correct recognition
Logistic regression modelling was used to determine the significant correlates of the recognition of diabetes. Table 2 reveals that individuals of Malay ethnicity (OR 1.39, 95% CI 1.02 to 1.89; p=0.04) had higher odds of correct recognition for diabetes than the Chinese. Compared with individuals of ages 18–34 years, the recognition of diabetes was significantly higher among the age groups of 35–49 years (OR 1.85; 95% CI 1.15 to 2.98; p=0.01) and 50–64 years (OR 2.06; 95% CI 1.19 to 3.56; p=0.01). Individuals who were diagnosed with diabetes were nearly three times as likely to correctly recognise the condition as those who did not have diabetes (OR 2.64; 95% CI 1.38 to 5.08; p<0.01).
Sociodemographic correlates of correct recognition of diabetes
However, we found that male gender (OR 0.64; 95% CI 0.46 to 0.90; p<0.01) and those from the Others ethnic category (OR 0.59; 95% CI 0.37 to 0.93; p<0.01), had significantly lower odds of recognition for diabetes. Those who were unemployed (OR 0.48; 95% CI 0.25 to 0.92; p=0.03) also had lower odds of correct recognition compared with those who were employed.
This was the first extensive study of diabetes literacy in Singapore to evaluate recognition by using a vignette. The study also identified significant sociodemographic characteristics associated with the correct recognition of diabetes mellitus. This study serves as a baseline measure of diabetes knowledge in Singapore’s general population, and will play an important role to inform future health policies and initiatives as part of ongoing national efforts to tackle diabetes.
Overall, the findings of this study suggest that the general adult population in Singapore has a relatively high rate (82.7%) of diabetes recognition. Our results present a striking contrast with other research 16 and correct recognition was much higher than previously found in local studies, where only about 60% of the general population were able to recognise symptoms and complications of diabetes. 18 In a vignette-based study by Vimalanathan and Furnham, 23 which explored health literacy of different types of diseases, diabetes had a correct recognition rate of 46% among the British adults. Diabetes literacy in our study, in this regard, was considerably higher. The high percentage of recognising diabetes in the general public may be a positive reflection of the recent concerted national efforts and developments surrounding diabetes awareness in the country, which has led to a slew of measures including public health campaigns (‘War on Diabetes’), education initiatives and the portrayal of diabetes-related problems and complications in local mass media. 24 However, some individuals though aware of this disease, may struggle to label it in proper medical terms. This was reflected in our results, with a proportion of answers observed to be ‘partly correct recognition’, wherein diabetes was labelled in non-technical, colloquial language (eg, ‘high blood sugar’). Another interesting finding includes some individuals interpreting the symptoms depicted in the vignette as the presence of other medical conditions. This was reflected in our analysis with 7.7% of the respondents ascribing it to health problems other than diabetes. For example, symptoms of ‘feeling thirsty’ were construed to be a condition of ‘dehydration’. This suggests people may be using their general health knowledge to answer, rather than having specific knowledge about diabetes. While we can appreciate that recognising symptoms as any other physical health condition (not diabetes) might still prove helpful in prompting individuals to seek professional care, ascribing the symptoms to a less serious condition can lead to significant delays in seeking proper and effective treatment. Furthermore, this points out a gap in the public knowledge of diabetes which must be addressed in order to better differentiate its symptoms and treatments, from other health problems. The signs and symptoms of diabetes may have been overlooked because of the slow and chronic progression of the disease; unlike many other physical conditions, the consequences of diabetes may not be manifested immediately. 25
The current study identified a number of sociodemographic factors significantly associated with correct recognition, including age, gender, ethnicity, as well as personal experience with diabetes. Recognition varied by age group, where young individuals (18–34 years) recognised diabetes most poorly, whereas those aged between 35 and 49 years and those aged between 50 and 64 years were significantly better at correctly recognising diabetes. We surmise that these findings may have been a result of several factors. First, the average age of onset of diabetes is about 45 years. 5 Comparably, the average age of respondents in our study was 45 years and a substantial proportion of adults with self-reported diabetes were from the age groups of 35–49 and 50–64, who may have recognised and had experience in dealing with similar problems to those described in the vignette. Unlike mental illnesses which may present with an early onset in younger people, 26 chronic physical diseases are known to be largely present and of greater concern in older individuals. 27 Perhaps the young adults in this study, were less likely to have directly or indirectly encountered diabetes. This theory is consistent with the local national health report 8 which suggests young adults in Singapore were less likely to have attended health screenings for chronic disease and were less likely to seek frequent treatment or follow-up consultations for diabetes than individuals from older age groups. Therefore, it is not surprising that the knowledge and ability to correctly recognise signs and symptoms of diabetes among young adults was poorer than those of older ages. These findings add to the importance of rethinking the diabetes public education efforts in Singapore. There may be a need for different educational strategies, focusing on specific symptoms of the disease, and a consideration of how the content is communicated so as to better target young adults given recognition of diabetes was poorest among them.
This study also observed a significant difference between the ability of males and females to recognise the physical disease. Consistent with the body of literature which reports better rates of diabetes recognition among females than males, 6 28 our study found that males were specifically less able to recognise diabetes. Gender differences in both mental and physical health literacy have previously been explained by greater self-awareness and higher sensitivity to symptoms of illnesses among women compared with men. 29 30 This gender disparity has also been associated with health behaviour paradigms such that, men are less likely to seek professional help for their health problems, 31–33 which could contribute to men’s health gap in terms of disease knowledge and recognition. Therefore, there is a crucial need for policy and health promotion programmes to target males who have a higher burden of the disease, with diabetes education strategies and materials designed to suit their needs and characteristics.
In this study, we found a relationship between ethnicity and recognition of diabetes. That is, ethnic Malays were more likely to identify it correctly, and those from the ethnic group, Others, were less likely to correctly recognise diabetes compared with Chinese. It is possible that respondents who are ethnic Malay, were disproportionately living with diabetes compared with the Chinese majority 34 or more likely to have direct contact with other individuals living with the disease. It can also be reasoned that the national diabetes education campaign since 2016, meant to engage ethnic Malays through community programmes and initiatives, may have been successful. 35 This emphasises utility in encouraging community involvement to support diabetes education by engaging racial/religious community groups in several small-scale activities. On the other hand, individuals from the Others ethnic group demonstrated poor recognition of diabetes, which could be due to the attribution of the symptoms to causes other than the disease. This is unfortunate because recognition of early symptoms can help to get diabetes under control immediately and prevent long-term complications. Content analysis of incorrect responses showed that there was a general tendency to consider symptoms described in the vignette as ‘not a medical problem’ or misinterpreting symptoms as ‘ageing’ or ‘other medical problems’. However, we are unable to offer any definite explanation for this phenomenon just as has been observed in other research. 36 Future studies to elucidate underlying reasons for ethnic differences is warranted.
Employment status was another significant predictor of diabetes knowledge. Compared with those employed, unemployed individuals were found to be less likely to correctly recognise diabetes in our study. The majority of diabetes cases occur among working adults. 37 Research has linked diabetes to physical disability in adults worldwide, 38 39 resulting in increased sick days for employees and increased costs for employers. 40 Thus, workplace screening and health talks for diabetes may have led to greater awareness and better recognition among those who are employed. In Singapore, health talks and routine health screenings including comprehensive blood tests offered to employees have become increasingly commonplace. 41 There may be easier affordability to use healthcare services among residents who are gainfully employed compared with their unemployed peers. This is consistent with a recent national survey which found that participation in health screenings rose in tandem with income. 8
It was not unexpected that persons with diabetes had significantly better recognition of the disease than the healthy population in our study. Patients with diabetes would have a deeper personal understanding of symptoms and chronic complications of diabetes when compared with the healthy population given their own experience and the education imparted as part of the management of diabetes.
Strengths and limitations
There were some limitations in our study. The vignette format might have facilitated diabetes identification by clearly describing persons with prototypical symptoms and functional changes. Recognition may be poorer in real life, because one might not notice the slow changes and ignore, minimise, or misattribute symptoms to other causes. The recognition rate found here may not apply to non-typical presentations of diabetes. 42 Moreover, the study did not include those who had language difficulties, and those who were institutionalised, hospitalised or uncontactable during the survey period. The survey was also a part of a larger study aimed at exploring the knowledge differences between those with and without diabetes in the general population, which may have introduced heterogeneity and the results could have hence overestimated diabetes recognition in the population. Nevertheless, the current study has its strengths in that it was a nationwide study with a representative sample who were surveyed ensuring high quality of the data collected. Additionally, the vignette was developed with inputs from experienced clinicians who are experts in the field and further cognitive testing was undertaken before use. Also, the study instruments were translated into the three major local languages ensuring inclusivity.
Given the high prevalence rate of diabetes in high-income countries including Singapore, it is imperative that educational campaigns target all members of the population with accurate and appropriate information. In order to do this, it is important to establish the population’s baseline knowledge and ability to recognise the signs and symptoms of diabetes. This study therefore set out to obtain up-to-date data, which future strategies and national programmes could potentially deploy. Our findings can inform strategic plans to address the growing diabetes epidemic. To prevent diabetes, reduce its economic burden, and improve the quality of life for those with diabetes or at risk of diabetes, public health messages and healthcare system interventions should target specifically, young adults, males and the unemployed with poor knowledge of diabetes and efforts should be made to screen the populations at risk. This should include the development of suitable strategies to communicate more effectively with a deeper understanding of the needs and competencies of the specific demographic. We must additionally acknowledge that often there may be patients with early diabetes with no symptoms. Thus, public health strategies should be directed towards increasing awareness of risk factors such as age, overweight, genetics and gestational diabetes. Research to develop effective ground-up community initiatives to apply diabetes education and screening programmes more widely should be supported to circumvent delays in diagnosis and treatment for persons with diabetes.
Supplementary Material
Contributors: KR led the analysis plan and interpretation of findings and prepared the manuscript. He is guarantor. MS and AP were actively involved in the analysis, interpretation and manuscript content. MS, SAC, ESL and CFS were involved in the conceptualisation of the study. JHL conducted the analysis and assisted in interpretation of findings and manuscript content. FDSK and PW provided significant intellectual inputs into the manuscript.
Funding: This work was supported by the National Medical Research Council of Singapore, grant number NMRC/HSRG/0085/2018.
Competing interests: None declared.
Provenance and peer review: Not commissioned; externally peer reviewed.
Supplemental material: This content has been supplied by the author(s). It has not been vetted by BMJ Publishing Group Limited (BMJ) and may not have been peer-reviewed. Any opinions or recommendations discussed are solely those of the author(s) and are not endorsed by BMJ. BMJ disclaims all liability and responsibility arising from any reliance placed on the content. Where the content includes any translated material, BMJ does not warrant the accuracy and reliability of the translations (including but not limited to local regulations, clinical guidelines, terminology, drug names and drug dosages), and is not responsible for any error and/or omissions arising from translation and adaptation or otherwise.
Data availability statement
Data are available on reasonable request. Data are available on reasonable request to the senior author of the paper.
Ethics statements
Patient consent for publication.
Not applicable.
Ethics approval
The study was approved by the Institutional Research Review Committee and the National Healthcare Group Domain Specific Review Board (Ref no. 2018/00430).
- 1. World Health Organization . Global report on diabetes, 2016. Available: https://www.who.int/diabetes/global-report/en/ [Accessed 2 Dec 2019].
- 2. Cho NH, Shaw JE, Karuranga S, et al. IDF diabetes atlas: global estimates of diabetes prevalence for 2017 and projections for 2045. Diabetes Res Clin Pract 2018;138:271–81. 10.1016/j.diabres.2018.02.023 [ DOI ] [ PubMed ] [ Google Scholar ]
- 3. Baynes HW. Classification, pathophysiology, diagnosis and management of diabetes mellitus. J diabetes metab 2015;6:1–9. [ Google Scholar ]
- 4. Aamir AH, Ul-Haq Z, Mahar SA, et al. Diabetes prevalence survey of Pakistan (DPS-PAK): prevalence of type 2 diabetes mellitus and prediabetes using HbA1c: a population-based survey from Pakistan. BMJ Open 2019;9:e025300. 10.1136/bmjopen-2018-025300 [ DOI ] [ PMC free article ] [ PubMed ] [ Google Scholar ]
- 5. Png ME, Yoong J, Phan TP, et al. Current and future economic burden of diabetes among working-age adults in Asia: conservative estimates for Singapore from 2010-2050. BMC Public Health 2016;16:153. 10.1186/s12889-016-2827-1 [ DOI ] [ PMC free article ] [ PubMed ] [ Google Scholar ]
- 6. Shuyu Ng C, Toh MPHS, Ko Y, et al. Direct medical cost of type 2 diabetes in Singapore. PLoS One 2015;10:e0122795. 10.1371/journal.pone.0122795 [ DOI ] [ PMC free article ] [ PubMed ] [ Google Scholar ]
- 7. Epidemiology & Disease Control Division . The burden of disease in Singapore, 1990–2017: an overview of the global burden of disease study 2017 results. Seattle, WA: IHME, 2019. http://www.Healthdata.Org/sites/default/files/files/policy_report/2019/GBD_2017_Singapore_Report.pdf [ Google Scholar ]
- 8. Ministry of Health . National health survey, 2011. Available: https://www.moh.gov.sg/content/dam/mohweb/Publications/Reports/2011/NHSlow0res.pdf [Accessed 1 July 2020].
- 9. Jorm AF. Mental health literacy. public knowledge and beliefs about mental disorders. Br J Psychiatry 2000;177:396–401. 10.1192/bjp.177.5.396 [ DOI ] [ PubMed ] [ Google Scholar ]
- 10. Vijan S, Stevens DL, Herman WH, et al. Screening, prevention, counseling, and treatment for the complications of type II diabetes mellitus. putting evidence into practice. J Gen Intern Med 1997;12:567–80. 10.1046/j.1525-1497.1997.07111.x [ DOI ] [ PMC free article ] [ PubMed ] [ Google Scholar ]
- 11. Abdullah A, Liew SM, Ng CJ, et al. Health literacy experiences of multi-ethnic patients and their health-care providers in the management of type 2 diabetes in Malaysia: a qualitative study. Health Expect 2020;23:1166–76. 10.1111/hex.13095 [ DOI ] [ PMC free article ] [ PubMed ] [ Google Scholar ]
- 12. Leung AYM, Bo A, Hsiao H-Y, et al. Health literacy issues in the care of Chinese American immigrants with diabetes: a qualitative study. BMJ Open 2014;4:1–12. 10.1136/bmjopen-2014-005294 [ DOI ] [ PMC free article ] [ PubMed ] [ Google Scholar ]
- 13. Prabsangob K, Somrongthong R, Kumar R. Health literacy among Thai elderly population with type-2 diabetes living in rural area of Thailand. PJPH 2018;8:27–31. 10.32413/pjph.v8i1.101 [ DOI ] [ Google Scholar ]
- 14. Soong FS. Beliefs and practices of Chinese diabetic patients concerning the cause and treatment of their ill-health. Singapore Med J 1971;12:309–13. [ PubMed ] [ Google Scholar ]
- 15. Chen A, Chidarikire T, Sarswat D, et al. Diabetes mellitus literacy in a regional community of a developed country. Acta Biomed 2019;90:482–8. 10.23750/abm.v90i4.7687 [ DOI ] [ PMC free article ] [ PubMed ] [ Google Scholar ]
- 16. Kayyali R, Slater N, Sahi A, et al. Type 2 diabetes: how informed are the general public? A cross-sectional study investigating disease awareness and barriers to communicating knowledge in high-risk populations in London. BMC Public Health 2019;19:138. 10.1186/s12889-019-6460-7 [ DOI ] [ PMC free article ] [ PubMed ] [ Google Scholar ]
- 17. Herath HMM, Weerasinghe NP, Dias H, et al. Knowledge, attitude and practice related to diabetes mellitus among the general public in Galle district in southern Sri Lanka: a pilot study. BMC Public Health 2017;17:1–7. 10.1186/s12889-017-4459-5 [ DOI ] [ PMC free article ] [ PubMed ] [ Google Scholar ]
- 18. Wee HL, Ho HK, Li SC. Public awareness of diabetes mellitus in Singapore. Singapore Med J 2002;43:128–34. [ PubMed ] [ Google Scholar ]
- 19. Department of Statistics, Singapore . Census of population, population trends, 2019. Available: https://www.singstat.gov.sg/-/media/files/publications/population/population2018.pdf [Accessed 25 July 2020].
- 20. AshaRani PV, Abdin E, Kumarasan R, et al. Study protocol for a nationwide knowledge, attitudes and practices (KAP) survey on diabetes in Singapore's general population. BMJ Open 2020;10:e037125. 10.1136/bmjopen-2020-037125 [ DOI ] [ PMC free article ] [ PubMed ] [ Google Scholar ]
- 21. Barter C, Renold E. ‘I wanna tell you a story': Exploring the application of vignettes in qualitative research with children and young people. Int J Soc Res Methodol 2000;3:307–23. 10.1080/13645570050178594 [ DOI ] [ Google Scholar ]
- 22. Hughes R. Using vignettes in qualitative research. Sociol Health Illn 1998;20:381–400. [ Google Scholar ]
- 23. Vimalanathan A, Furnham A. Comparing physical and mental health literacy. J Ment Health 2019;28:243–8. 10.1080/09638237.2018.1466050 [ DOI ] [ PubMed ] [ Google Scholar ]
- 24. Ministry of Health . War on diabetes, 2018. Available: https://www.moh.gov.sg/wodcj [Accessed 25 July 2020].
- 25. Ramachandran A. Know the signs and symptoms of diabetes. Indian J Med Res 2014;140:579–81. [ PMC free article ] [ PubMed ] [ Google Scholar ]
- 26. Kessler RC, Berglund P, Demler O, et al. Lifetime prevalence and age-of-onset distributions of DSM-IV disorders in the National comorbidity survey replication. Arch Gen Psychiatry 2005;62:593–602. 10.1001/archpsyc.62.6.593 [ DOI ] [ PubMed ] [ Google Scholar ]
- 27. Chang J, Mosenifar Z. Differentiating COPD from asthma in clinical practice. J Intensive Care Med 2007;22:300–9. 10.1177/0885066607304445 [ DOI ] [ PubMed ] [ Google Scholar ]
- 28. Lemes Dos Santos PF, Dos Santos PR, Ferrari GSL, et al. Knowledge of diabetes mellitus: does gender make a difference? Osong Public Health Res Perspect 2014;5:199–203. 10.1016/j.phrp.2014.06.004 [ DOI ] [ PMC free article ] [ PubMed ] [ Google Scholar ]
- 29. Bianchin M, Angrilli A. Gender differences in emotional responses: a psychophysiological study. Physiol Behav 2012;105:925–32. 10.1016/j.physbeh.2011.10.031 [ DOI ] [ PubMed ] [ Google Scholar ]
- 30. Gijsbers van Wijk CM, van Vliet KP, Kolk AM, et al. Symptom sensitivity and sex differences in physical morbidity: a review of health surveys in the United States and the Netherlands. Women Health 1991;17:91–124. 10.1300/J013v17n01_06 [ DOI ] [ PubMed ] [ Google Scholar ]
- 31. Redondo-Sendino A, Guallar-Castillón P, Banegas JR, et al. Gender differences in the utilization of health-care services among the older adult population of Spain. BMC Public Health 2006;6:155. 10.1186/1471-2458-6-155 [ DOI ] [ PMC free article ] [ PubMed ] [ Google Scholar ]
- 32. Jonsson PM, Sterky G, Gåfvels C, et al. Gender equity in health care: the case of Swedish diabetes care. Health Care Women Int 2000;21:413–31. 10.1080/07399330050082245 [ DOI ] [ PubMed ] [ Google Scholar ]
- 33. Chang H-Y, Hsu C-C, Pan W-H, et al. Gender differences in trends in diabetes prevalence from 1993 to 2008 in Taiwan. Diabetes Res Clin Pract 2010;90:358–64. 10.1016/j.diabres.2010.09.032 [ DOI ] [ PubMed ] [ Google Scholar ]
- 34. Phan TP, Alkema L, Tai ES, et al. Forecasting the burden of type 2 diabetes in Singapore using a demographic epidemiological model of Singapore. BMJ Open Diabetes Res Care 2014;2:e000012. 10.1136/bmjdrc-2013-000012 [ DOI ] [ PMC free article ] [ PubMed ] [ Google Scholar ]
- 35. Campaign launched to help Malays fight diabetes, 2016. Available: https://www.todayonline.com/singapore/six-month-nationwide-campaign-be-rolled-out-ccs-fight-diabetes-oct [Accessed 4 Jan 2021].
- 36. Chinnappan S, Sivanandy P, Sagaran R, et al. Assessment of knowledge of diabetes mellitus in the urban areas of Klang district, Malaysia. Pharmacy 2017;5:11. 10.3390/pharmacy5010011 [ DOI ] [ PMC free article ] [ PubMed ] [ Google Scholar ]
- 37. Nixon H, Robertson D. The role of occupational health in diabetes management. Occup Health 2008;60:29. [ Google Scholar ]
- 38. Szosland D. Shift work and metabolic syndrome, diabetes mellitus and ischaemic heart disease. Int J Occup Med Environ Health 2010;23:287. 10.2478/v10001-010-0032-5 [ DOI ] [ PubMed ] [ Google Scholar ]
- 39. Wong E, Backholer K, Gearon E, et al. Diabetes and risk of physical disability in adults: a systematic review and meta-analysis. Lancet Diabetes Endocrinol 2013;1:106–14. 10.1016/S2213-8587(13)70046-9 [ DOI ] [ PubMed ] [ Google Scholar ]
- 40. Gulley T, Boggs D, Mullins R, et al. Diabetes screening in the workplace. Workplace Health Saf 2014;62:444–6. 10.3928/21650799-20141014-01 [ DOI ] [ PubMed ] [ Google Scholar ]
- 41. HPB Health at Work . Healthy workplace ecosystem, 2019. Available: https://www.hpb.gov.sg/workplace/healthy-workplace-ecosystem [Accessed 04 Jan 2021].
- 42. Sjöholm Åke. Atypical diabetes: a diagnostic challenge. BMJ Open Diabetes Res Care 2020;8:e0014. 10.1136/bmjdrc-2020-001470 [ DOI ] [ PMC free article ] [ PubMed ] [ Google Scholar ]
Associated Data
This section collects any data citations, data availability statements, or supplementary materials included in this article.
Supplementary Materials
Data availability statement.
- View on publisher site
- PDF (713.3 KB)
- Collections
Similar articles
Cited by other articles, links to ncbi databases.
- Download .nbib .nbib
- Format: AMA APA MLA NLM
Add to Collections

IMAGES