Bulletin on Aging & Health

Do Fast Food Restaurants Contribute to Obesity?
Over the past thirty years, the prevalence of obesity and obesity-related diseases in the U.S. has risen sharply. Since the early 1970s, the share of children age 6 to 19 classified as overweight has more than tripled, from 5 percent to 17 percent, while the share of adults classified as overweight or obese rose from half to two-thirds of the population. Over this same period, the number of fast food restaurants more than doubled. Exposes such as "Supersize Me" and "Fast Food Nation" as well as reports in the popular press have frequently suggested that fast food is at least partly to blame for the U.S.'s rising obesity rates.
Despite the popularity of this view, it has been difficult to empirically establish a causal link between fast food and obesity. The simple fact that fast food restaurants and obesity have both increased over time is insufficient proof of this link, as are studies that rely on differences in fast food consumption across individuals, since people who eat more fast food may be prone to other behaviors that affect obesity.
In The Effect of Fast Food Restaurants on Obesity (NBER Working Paper 14721 ), researchers Janet Currie , Stefano DellaVigna , Enrico Moretti , and Vikram Pathania undertake a careful study of the effect of fast food on obesity using the exact geographic location of fast food restaurants. Specifically, the authors examine whether proximity to a fast food restaurant affects the obesity rates of 3 million school children and the weight gain of over 1 million pregnant women.
The authors have several strategies to overcome the concern that children whose school is close to a fast food restaurant may be more prone to obesity for other reasons. First, the authors compute whether the fast food restaurant is within 0.10, 0.25, or 0.50 miles of the school and test whether there is a differential effect by distance. Given that there is a fast food restaurant in the general area, whether the restaurant happens to be very close to the school is arguably random and thus a good way to identify the effect of fast food access on obesity. Second, the authors control for a rich set of school and neighborhood characteristics in their analysis and allow these to vary over time. Finally, the authors estimate models relating changes in obesity to changes in access to fast food restaurants, relying on schools that gain or lose a nearby fast food restaurant during the sample period to identify the effect of fast food access on obesity. The authors estimate their models using data on all California 9th graders for the years 1999 and 2001-2007.
In their analysis of pregnant women, the empirical approach is similar - the authors include women for whom they observe at least two births in their sample, so that they can examine whether changes in fast food access between one pregnancy and the next are associated with changes in the probability of excessive weight gain. The authors use Vital Statistics data from 1989 to 2003 for Michigan, New Jersey, and Texas, the states for which they were able to obtain confidential data with mothers' names and addresses.
Turning to the results, the authors find that proximity to a fast food restaurant significantly increases the risk of obesity. For children, having a fast food restaurant within 0.10 miles of school increases the probability of obesity by 1.7 percentage points, or 5.2 percent. Interestingly, there is no significant effect of having a restaurant 0.25 or 0.50 miles from the school. The effects of fast food access are larger for girls.
Is the magnitude of this finding reasonable? To probe this, the authors estimate how many additional calories would be needed to push enough students over the overweight threshold (85th percentile of the historical BMI, or body mass index, distribution) to generate the estimated 5.2 percent increase in obesity. They estimate this to be 30-100 calories per day (per student). Given the large number of calories in typical items from a fast food restaurant, the magnitudes seem plausible.
For pregnant women, having a restaurant within 0.10 miles increases the probability of gaining over 20 kilos during pregnancy by 0.2 percentage points, or 2.5 percent. Unlike for children, the effects are still discernable at 0.25 and 0.50 miles. The effects are largest for African American mothers and mothers with a high school education or less. For pregnant women, the estimated increase in calories needed to generate this effect is much smaller, only 1-4 additional calories per day.
The authors conduct several other interesting analyses. First, they explore the effect of other types of restaurants and find that only fast food restaurants affect obesity. Second, they ask whether the future presence of a fast-food restaurant is associated with greater obesity today. If that were the case, it would suggest that fast food restaurants locate in areas where obesity is trending up, providing an alternative explanation for the paper's findings. However, the authors find that only current fast food locations matter.
Overall, the study suggests that proximity to fast food significantly increases the risk of obesity. One possible explanation is that proximity to fast food reduces the costs of fast food, principally travel costs. A second explanation is that easier access to fast food tempts consumers who have self-control problems. The fact that the effects are larger for teens and fall off more quickly with distance suggests that travel costs are more important for teens than for pregnant women.
The policy implications of these findings are potentially important. As the authors conclude, "policies restricting access to fast food near schools could have significant effects on obesity among school children, but similar policies restricting the availability of fast food in residential areas are unlikely to have large effects on adults."
Researchers
NBER periodicals and newsletters may be reproduced freely with appropriate attribution.
More from NBER
In addition to working papers , the NBER disseminates affiliates’ latest findings through a range of free periodicals — the NBER Reporter , the NBER Digest , the Bulletin on Retirement and Disability , the Bulletin on Health , and the Bulletin on Entrepreneurship — as well as online conference reports , video lectures , and interviews .
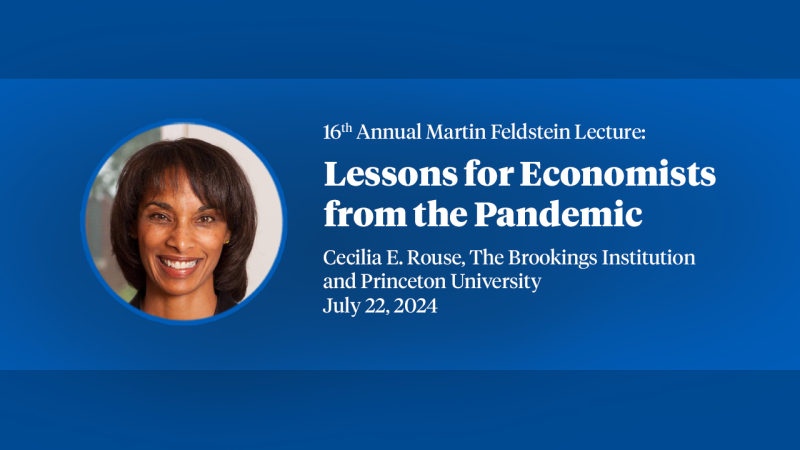
© 2023 National Bureau of Economic Research. Periodical content may be reproduced freely with appropriate attribution.
- Nutritional Medicine
- Food Science
- Nutrition and Dietetics
Examining the Relationship Between Fast Food and Obesity: A Systematic Literature Review and Future Agenda
- December 2021
- Journal of Tourism and Gastronomy Studies 9(4):2441-2461
- 9(4):2441-2461

- Eskisehir Osmangazi University

- Ankara Hacı Bayram Veli University

Discover the world's research
- 25+ million members
- 160+ million publication pages
- 2.3+ billion citations
- Int J Environ Res Publ Health
- Jozaa Z. ALTamimi

- Int J Consum Stud

- INT J OBESITY
- Kenta Okuyama

- Kristina Sundquist

- Dianne Cantali

- Hyunjung Lim

- ECON HUM BIOL

- BRIT FOOD J
- Arzu Kılıçlar

- Elvira Fleury

- Recruit researchers
- Join for free
- Login Email Tip: Most researchers use their institutional email address as their ResearchGate login Password Forgot password? Keep me logged in Log in or Continue with Google Welcome back! Please log in. Email · Hint Tip: Most researchers use their institutional email address as their ResearchGate login Password Forgot password? Keep me logged in Log in or Continue with Google No account? Sign up
24/7 writing help on your phone
To install StudyMoose App tap and then “Add to Home Screen”
Fast Food and Childhood Obesity: A Deeper Look
Save to my list
Remove from my list

Fast Food and Childhood Obesity: A Deeper Look. (2018, Sep 08). Retrieved from https://studymoose.com/fast-food-and-childhood-obesity-essay
"Fast Food and Childhood Obesity: A Deeper Look." StudyMoose , 8 Sep 2018, https://studymoose.com/fast-food-and-childhood-obesity-essay
StudyMoose. (2018). Fast Food and Childhood Obesity: A Deeper Look . [Online]. Available at: https://studymoose.com/fast-food-and-childhood-obesity-essay [Accessed: 8 Sep. 2024]
"Fast Food and Childhood Obesity: A Deeper Look." StudyMoose, Sep 08, 2018. Accessed September 8, 2024. https://studymoose.com/fast-food-and-childhood-obesity-essay
"Fast Food and Childhood Obesity: A Deeper Look," StudyMoose , 08-Sep-2018. [Online]. Available: https://studymoose.com/fast-food-and-childhood-obesity-essay. [Accessed: 8-Sep-2024]
StudyMoose. (2018). Fast Food and Childhood Obesity: A Deeper Look . [Online]. Available at: https://studymoose.com/fast-food-and-childhood-obesity-essay [Accessed: 8-Sep-2024]
- A Deeper Look to Poems and Songs Pages: 4 (1052 words)
- Exploring Dynamics in "The Pearl": A Deeper Look at Juana, Kino, and the Intricate Threads of Fate Pages: 4 (1063 words)
- Unmasking the Elusive American Dream: A Deeper Look through 'The Great Gatsby' Pages: 3 (754 words)
- The Saline Symphony: A Deeper Look at the Boiling Point of Saltwater Pages: 2 (493 words)
- Exploring Equality in the U.S. Constitution: A Deeper Look Pages: 2 (380 words)
- Critiquing "Getting to Yes": A Deeper Look at Negotiation Flaws Pages: 3 (624 words)
- Budweiser Marketing Mastery: A Deeper Look into Persuasion Pages: 3 (664 words)
- The Enigmatic Allure of Feline Companions: A Deeper Look into the Mystique of Cats Pages: 3 (780 words)
- The Psychology of Horror: A Deeper Look into Stephen King's Insights Pages: 3 (751 words)
- A Deeper Look into Gary Soto's Inner Struggles With Guilt Pages: 2 (531 words)
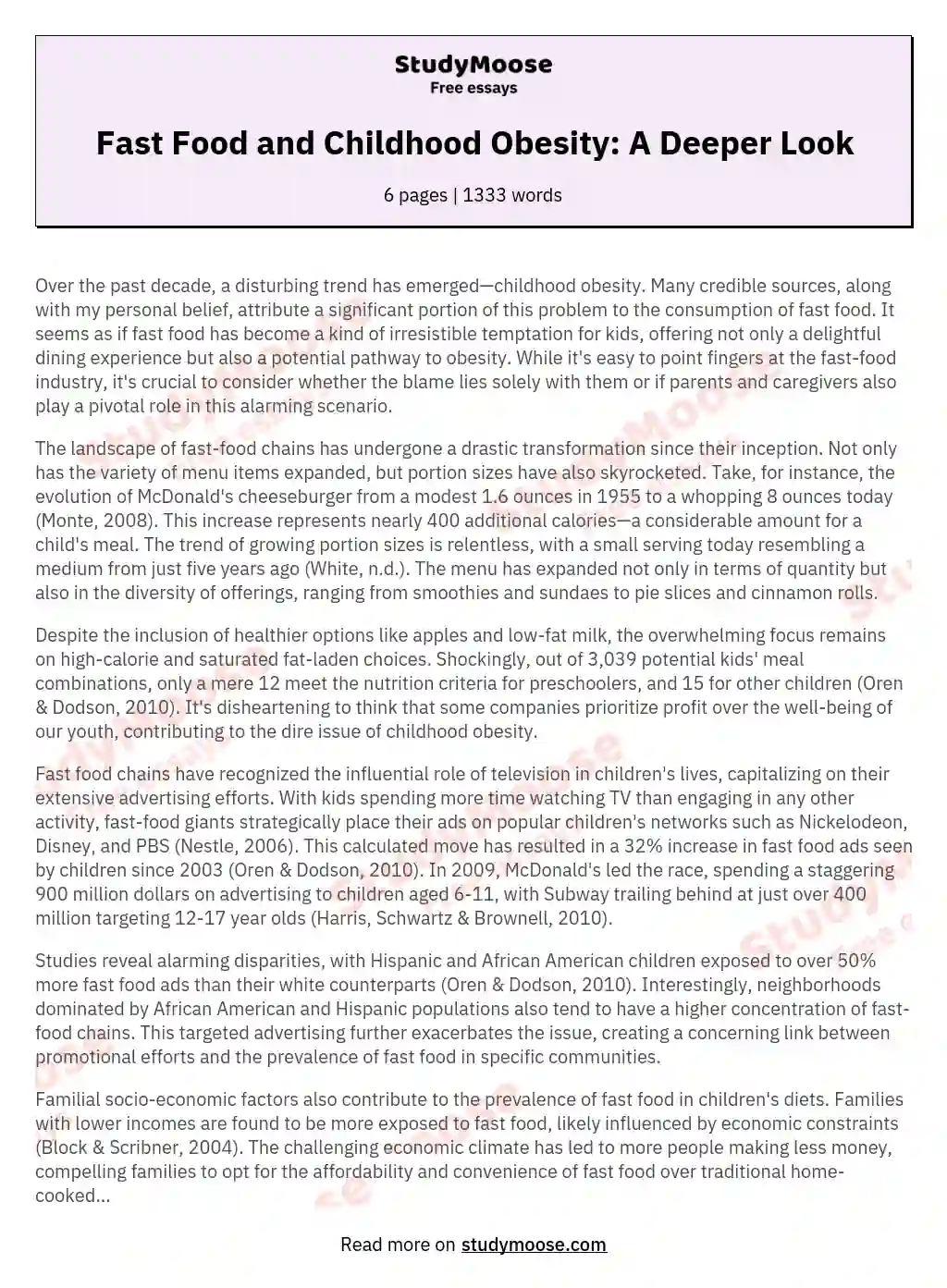
👋 Hi! I’m your smart assistant Amy!
Don’t know where to start? Type your requirements and I’ll connect you to an academic expert within 3 minutes.
How Fast Food Affects Children's Health
- Download PDF Copy
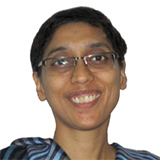
Fast food or junk food is a generic term for all kinds of foods which are rich in energy, because they contain a lot of fat and sugar, as well as salt, but are relatively low in other important nutrients such as protein, fiber, vitamins, and minerals.
However, fast food is extremely attractive to most children because of the taste, comparatively lower price, and convenience (doesn’t require any cooking or preparation). Since children typically do not understand how this kind of food negatively impacts their health, it can be quite addictive.
This article describes some important ways in which junk food harms children’s health.
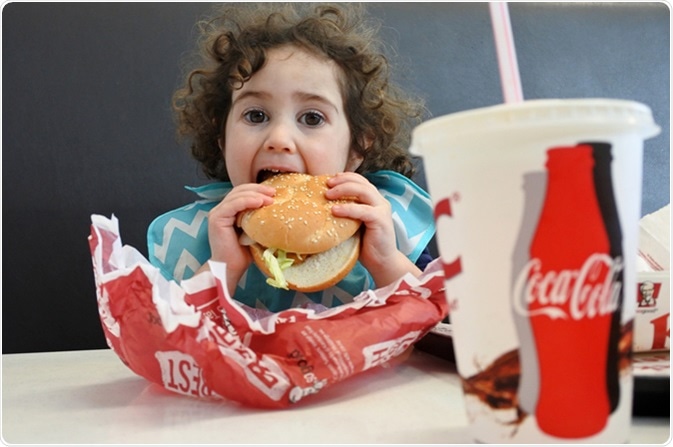
Negative Aspects of Junk Food
Regular junk food intake leads to long-term health problems such as obesity, accompanying emotional and self-esteem problems, and chronic illnesses in later life. A single fast food meal could add 160 and 310 extra kilocalories to the daily caloric intake for teenagers and younger children, respectively.
Lack of vitamins such as A and C, and minerals such as magnesium and calcium, encourage the development of deficiency diseases and osteoporosis, as well as dental caries due to higher sugar intake.
The presence of hazardous food coloring agents and/or unhealthy trans fats in many fast food items, and issues with food preparation safety, often complicate the issue further.
Fast food intake more than three times a week is associated with greater odds of atopic disorders such as asthma, eczema or rhinitis, while asthma severity is almost 40% higher in teenagers and more than 25% in younger children.
Eating junk food 4-6 times a week leads to lower math and reading skills compared with the children who did not eat so much junk food.
Constipation
An overdose of calories, fats, sugars, and other carbohydrates in repeated meals changes the food desires of the child, and makes it less likely that the child will eat fibers, fruits, milk, and vegetables. This can result in greater chances of constipation.
Eating a lot of fast food in childhood makes it hard to eat healthy in later life, even if related medical problems are already evident, because childhood food habits solidify by adulthood. The addictive taste of fast food makes it quite unlikely that the palate will later savor the less complicated and less spicy flavors of ordinary food.
Poor Academics
Fast food can lead to impaired academic performance because high sugar levels followed by sugar crashes and poor concentration levels make it difficult to accomplish tasks which need extended periods of focused attention. Blood sugar fluctuations can also result in mood swings and lack of alertness, lowering classroom participation.
Less Energy
Fast food can inhibit participation in extracurricular activities because it doesn’t provide adequate nutrients for physical activity. Lack of physical activity not only keeps children out of peer groups but also impairs physical and mental health.
Obesity can result in lowered self-esteem, and perhaps depression. Some children who eat junk food are at risk of developing depression even without obesity. Depression in turn affects growth and development parameters, academic performance, and social relationships. It also results in a higher risk of suicide.
Sleep Disturbances
Pop and cola drinks often contain caffeine which can make bedtime an ordeal by postponing normal sleep-wake cycles.
Hyperactivity
Essential fatty acids are typically missing or lacking in fast foods. These include omega-3 and omega-6 polyunsaturated fatty acids which cannot be produced within the body, but are essential for the manufacture of cell membranes, and are also required in high concentrations within the brain and retina. The lack of such nutrients is thought to be associated with increased antisocial behavior, and perhaps with hyperactivity, though more research is needed to prove this.
Fast food intake definitely needs to be strictly controlled in children as it does no good and may do much harm. The antidote? Surprisingly, a simple increase in fruit intake can improve the mood and reduce the severity of atopic diseases. Stopping the marketing of junk foods directed at children with attractive characters and gifts may be one way to help children eat better. Another method is to make healthy food more easily available at affordable prices and in a more appealing format.
- www.mayoclinic.org/.../faq-20058241
- https://www.ncbi.nlm.nih.gov/pubmed/14702458
- www.healthychildren.org/.../...Develop-Unhealthy-Food-Preferences.aspx
- http://thorax.bmj.com/content/early/2013/01/03/thoraxjnl-2012-202285
- medical-clinical-reviews.imedpub.com/...ption-in-children-a-review.php
- https://www.ncbi.nlm.nih.gov/pmc/articles/PMC2664919/
Further Reading
- All Diet Content
- Low Calorie and Very Low Calorie Diets
- Diabetic Diet
- DASH Diet for High Blood Pressure
- High Protein Diet
Last Updated: Aug 23, 2018
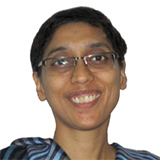
Dr. Liji Thomas
Dr. Liji Thomas is an OB-GYN, who graduated from the Government Medical College, University of Calicut, Kerala, in 2001. Liji practiced as a full-time consultant in obstetrics/gynecology in a private hospital for a few years following her graduation. She has counseled hundreds of patients facing issues from pregnancy-related problems and infertility, and has been in charge of over 2,000 deliveries, striving always to achieve a normal delivery rather than operative.
Please use one of the following formats to cite this article in your essay, paper or report:
Thomas, Liji. (2018, August 23). How Fast Food Affects Children's Health. News-Medical. Retrieved on September 08, 2024 from https://www.news-medical.net/health/How-Fast-Food-Affects-Childrens-Health.aspx.
Thomas, Liji. "How Fast Food Affects Children's Health". News-Medical . 08 September 2024. <https://www.news-medical.net/health/How-Fast-Food-Affects-Childrens-Health.aspx>.
Thomas, Liji. "How Fast Food Affects Children's Health". News-Medical. https://www.news-medical.net/health/How-Fast-Food-Affects-Childrens-Health.aspx. (accessed September 08, 2024).
Thomas, Liji. 2018. How Fast Food Affects Children's Health . News-Medical, viewed 08 September 2024, https://www.news-medical.net/health/How-Fast-Food-Affects-Childrens-Health.aspx.
Suggested Reading

Cancel reply to comment
- Trending Stories
- Latest Interviews
- Top Health Articles

How can microdialysis benefit drug development
Ilona Vuist
In this interview, discover how Charles River uses the power of microdialysis for drug development as well as CNS therapeutics.

Global and Local Efforts to Take Action Against Hepatitis
Lindsey Hiebert and James Amugsi
In this interview, we explore global and local efforts to combat viral hepatitis with Lindsey Hiebert, Deputy Director of the Coalition for Global Hepatitis Elimination (CGHE), and James Amugsi, a Mandela Washington Fellow and Physician Assistant at Sandema Hospital in Ghana. Together, they provide valuable insights into the challenges, successes, and the importance of partnerships in the fight against hepatitis.

Addressing Important Cardiac Biology Questions with Shotgun Top-Down Proteomics
In this interview conducted at Pittcon 2024, we spoke to Professor John Yates about capturing cardiomyocyte cell-to-cell heterogeneity via shotgun top-down proteomics.

Latest News

Newsletters you may be interested in

Your AI Powered Scientific Assistant
Hi, I'm Azthena, you can trust me to find commercial scientific answers from News-Medical.net.
A few things you need to know before we start. Please read and accept to continue.
- Use of “Azthena” is subject to the terms and conditions of use as set out by OpenAI .
- Content provided on any AZoNetwork sites are subject to the site Terms & Conditions and Privacy Policy .
- Large Language Models can make mistakes. Consider checking important information.
Great. Ask your question.
Azthena may occasionally provide inaccurate responses. Read the full terms .
While we only use edited and approved content for Azthena answers, it may on occasions provide incorrect responses. Please confirm any data provided with the related suppliers or authors. We do not provide medical advice, if you search for medical information you must always consult a medical professional before acting on any information provided.
Your questions, but not your email details will be shared with OpenAI and retained for 30 days in accordance with their privacy principles.
Please do not ask questions that use sensitive or confidential information.
Read the full Terms & Conditions .
Provide Feedback


An official website of the United States government
The .gov means it’s official. Federal government websites often end in .gov or .mil. Before sharing sensitive information, make sure you’re on a federal government site.
The site is secure. The https:// ensures that you are connecting to the official website and that any information you provide is encrypted and transmitted securely.
- Publications
- Account settings
Preview improvements coming to the PMC website in October 2024. Learn More or Try it out now .
- Advanced Search
- Journal List
- HHS Author Manuscripts

Does Proximity to Fast Food Cause Childhood Obesity? Evidence from Public Housing
Syracuse University
Amy Ellen Schwartz
Brian elbel.
New York University
Author Statement
Amy Ellen Schwartz : Conceptualization, Methodology, Supervision, Writing - Review & Editing, Data Curation, Funding Acquisition
Brian Elbel : Funding Acquisition, Data Curation, Writing - Review & Editing
Associated Data
We examine the causal link between proximity to fast food and the incidence of childhood obesity among low-income households in New York City. Using individual-level longitudinal data on students living in public housing linked to restaurant location data, we exploit the naturally occurring within-development variation in distance to fast food restaurants to estimate the impact of proximity on obesity. Since the assignment of households to specific buildings is based upon availability at the time of assignment to public housing, the distance between student residence and retail outlets—including fast food restaurants, wait-service restaurants, supermarkets, and corner stores—is plausibly random. Our credibly causal estimates suggest that childhood obesity increases with proximity to fast food, with larger effects for younger children who attend neighborhood schools.
1. Introduction
Does proximity to fast food outlets and “unhealthy” food increase obesity among low-income children? In the United States, nearly one fifth of low-income children are obese, facing elevated risks of adult obesity, hypertension, and cardiovascular disease, among other serious complications that may lead to premature death ( Bridger, 2009 ; Ogden et al., 2018 ; Sahoo et al., 2015 ). One commonly proffered culprit is the high density of fast food and relative scarcity of “healthy” food outlets in low-income neighborhoods, which facilitate consumption of high-calorie, low-nutrient food and spur obesity. Despite persuasive evidence of a correlation between obesity and the food environment in low-income neighborhoods, there is a dearth of credible evidence on the causal effects of proximity to fast food for two key reasons. First, both individual weight and residential location are likely to reflect a set of common underlying individual or family characteristics, such as income or educational attainment, making it difficult to isolate the effects of proximity to fast food per se. Second, individual-level data linking weight measures to proximity to fast food are scarce, typically only available for small samples or in limited detail. In this paper, we leverage the plausibly random within-development location of families living in public housing – a novel strategy – and use longitudinal individual-level data on weight, residential location, and neighborhood food outlets for New York City (NYC) public school students to derive credibly causal estimates of the impact of proximity to fast food on weight outcomes.
The key to our identification strategy is the plausibly random within-development variation in food environment driven by the tenant selection and assignment process of NYC public housing. First, NYC public housing applicants cannot indicate their preference for precise residential location in the assignment process. Second, it takes approximately 38 months on average to get to the top of the waiting list for NYC public housing, and the long wait time discourages applicants from rejecting offers. Thus the unit assignment within a development depends upon the vacancies at the time of assignment and generates the plausibly random variation in proximity to food outlets that we leverage to isolate causal effects of proximity to fast food on weight outcomes.
We draw on a rich individual-level longitudinal dataset on 143,859 K-12 NYC public school students who lived in public housing at some point between academic years 2009 and 2016. In addition to socio-demographic characteristics, the data include residential location and annual height and weight measures. Using data on the locations of food outlets citywide, we then calculate the distance from each student’s residential location to the nearest fast food restaurant (and to other food outlets). The large sizes of public housing developments in NYC yield substantial within-development variation in proximity to food outlets, and we find no statistically significant evidence of selection.
To preview our results, we find that, indeed, proximity to fast food increases the probability a child is obese. More specifically, the probability of being obese increases 0.6 percentage points for every 0.1 mile closer a student lives to the nearest fast food restaurant, and the probability of being overweight (which includes obesity) increases by 1.1 percentage points. In contrast, we find no evidence that proximity to other types of food outlets – wait-service restaurants, supermarkets, or corner stores – has any impact on weight outcomes. Stratifying by grade level reveals the largest effects are among students in grades 3–8, where the incidence of obesity increases 1.4 percentage points and the incidence of overweight increases up to 1.9 percentage points for every 0.1 mile closer to the nearest fast food. Effects are even larger among older elementary and middle school students attending neighborhood schools (located less than a half-mile from home) who are likely to patronize neighborhood food outlets: the incidence of obesity increases 1.7 percentage points, and the incidence of overweight increases 2.1 percentage points for every 0.1 mile closer to the nearest fast food. An average city block is 0.05 mile in NYC, and these estimates respectively represent a 6 percent and a 4.7 percent increase in the obesity and overweight rates from a two-block difference in distance to fast food. Effects among younger students (grades K-2) and older students (grades 9–12) are close to zero and not statistically significant. Results are robust to alternative measures of the food environment, such as variables capturing the presence or number of fast food restaurants within different radiuses from home. In short, we find credibly causal evidence that proximity to fast food increases obesity and overweight among low-income children, and students in grades 3–8 (typically ages 8–13) are the most vulnerable. Our results suggest that place-based interventions to limit access to or consumption of fast food may be effective in reducing the obesity rates among low-income children in urban areas.
This paper is organized as follows. We first review prior literature and the theoretical motivation that inform our approach to examine the relationship between proximity to fast food and childhood obesity. In Section 3 , we describe the institutional setting of public housing in NYC that provides plausibly exogenous variation in student proximity to fast food. Section 4 presents the individual-level weight outcome data, food environment measures, and descriptive statistics of our sample. Most importantly, we show within-development variation in proximity to fast food among students in public housing and test whether the variation in the local food environment is uncorrelated with student demographic characteristics. In Section 5 , we provide the estimating equations for our empirical strategy. We present results and a series of robustness checks in Section 6 and conclude with discussion and implications for policy in Section 7 .
2. Literature review
2.1. the link between obesity and proximity to fast food.
A large body of descriptive studies, from a range of settings, provide compelling evidence on the association between unhealthy food environment and the prevalence of obesity. National studies linking individual weight outcomes with the food environment data at the county-level ( Mehta & Chang, 2008 ), zip-code level ( Gibson, 2011 ), and census tract-level ( Chen et al., 2016 ; Dubowitz et al., 2012 ) find that higher density of fast food restaurants and lower density of supermarkets are associated with increased probability of obesity for individuals in the neighborhood. Studies focusing on southern states also find that individuals in census tracts with more supermarkets are less likely to be obese, while those in census tracts with more fast food restaurants and corner stores are more likely to be obese ( Morland et al., 2006 ; Morland & Everson, 2009 ).
Studies on children further document that micro-neighborhood food environments are associated with obesity risks. A school-level analysis by Davis and Carpenter (2009) finds having a fast food restaurant within a half-mile of school is associated with higher probabilities of being obese among middle and high school students. A more recent study by Elbel et al. (2020) examines the weight outcomes of children attending NYC public schools and the individual-level variation in distance to fast food within census tracts. They find that living more than 0.025 mile (about half of a city block) from the nearest fast food restaurant is associated with lower obesity and overweight risks.
Why might access to fast food affect obesity? Put simply, closer proximity to a fast food outlet lowers the relative travel cost of purchasing fast food, which may lead to increased fast food consumption and, in turn, a high-calorie diet. Previous research on consumption decisions, including McCarthy (1999) and Bellettini and Kempf (2013) , suggests longer distance and travel time increase the opportunity cost and the effective price the consumers pay. The food environment literature (see Anderson & Matsa, 2011 ; Cutler, Glaeser, & Shapiro, 2003 ; Dunn, 2010 ) also builds upon the theory that food purchasing decisions are based on a function of the monetary price of the meal and other disutility, including the value of forgone time used to access or prepare the meal. As the distance to fast food decreases, the time and the cost spent on traveling to purchase fast food decrease, yet the relative time and cost of purchasing groceries and preparing home-cooked meals increase. Thus, among two individuals (if all else equal but their distances to fast food), the person living closer to a fast food restaurant is more likely to purchase fast food on a given day. Athens, Duncan, and Elbel (2016) provide evidence to support the hypothesis that proximity to fast food outlets and supermarkets are predictors of fast food dining frequency. 1
Residential proximity to fast food, however, may be correlated with weight outcomes through other avenues. Families with a higher propensity for obesity – say a taste for fast food – may choose residential locations closer to fast food. More generally, there may be underlying individual or family characteristics that determine both residential location and propensity for obesity. While we are unaware of direct evidence on the underlying factors, a variety of existing studies have documented differences in the demographic characteristics of neighborhoods with different food environments. For example, Lewis et al. (2005) and Galvez et al. (2008) find that neighborhoods with higher density of fast food restaurants are also likely to have higher concentrations of racial minorities and low-income households (see Berger et al., 2019 ; Block, Scribner, & DeSalvo, 2004 ; Powell, Chaloupka, & Bao, 2007 for more). Studies also document that areas with greater access to supermarkets are predominantly white ( Powell, Slater, Mirtcheva, & Chaloupka, 2007 ; Richardson, Boone-Heinonen, Popkin, & Gordon-Larsen, 2012 ). Disparities in weight outcomes across residential locations may reflect underlying differences in individual- or household-level characteristics, apart from the effects of the food environment per se. The key empirical challenge is to isolate the impact of the food environment from these underlying differences.
2.2. Quasi-Experimental Evidence
Despite the abundant descriptive evidence linking obesity to fast food availability, relatively few papers focus on estimating the causal effects. Four key papers use access to highways as an instrument for fast food locations to identify the causal relationship between food environment and obesity outcomes. First, Anderson and Matsa (2011) use distance to interstate highways as an instrument to examine the effect of distance to the nearest fast food restaurant, focusing on rural areas in 11 states. They employ a two-sample instrumental variable technique, using ZIP code centroids for restaurant data and telephone area code centroids for obesity data. They find no significant relationship between distances to the nearest restaurant (both fast food and full-service) and weight outcomes.
Two other studies by Dunn (2010) and Dunn, Sharkey, and Horel (2012) , however, find detrimental effects of living near fast food restaurants on obesity outcomes for racial minorities and female populations. Dunn (2010) uses the number of interstate highway exits in each county as an instrument for county-level variation in the number of fast food restaurants. He categorizes counties into urban, rural, and medium-density counties across the nation and finds that obesity risks increase with the number of fast food restaurants, specifically among non-whites and females in medium-density counties. Dunn, Sharkey, and Horel (2012) focus on households in central Texas and use distance to a major highway – including not only interstates but also Texas highways – as an instrument to identify the effects of distance to the nearest fast food and the number of fast food restaurants near home on obesity outcomes. They find that both living closer to the nearest fast food and having more fast food restaurants within 1 mile and within 3 miles from home results in a statistically significant increase in the probability of being obese for non-white residents.
Potential explanations for heterogeneity in the effects of living near fast food across racial and gender subgroups include differential preferences and travel costs. First, the distance elasticity of fast food demand may be higher for minority groups due to differences in preferences. For example, ethnic cuisines differ in key ingredients that may require further travel to particular food outlets ( Bitler & Haider, 2011 ). Easier access to fast food restaurants increases the opportunity cost of traveling further distances to purchase products for ethnic cuisines. The above studies, however, do not provide evidence for the effects of the availability of other food retails, such as large supermarkets, which is a common data limitation for past studies. Further, Dunn et al. (2012) explain that the travel cost may be higher for racial minorities because they are less likely to own vehicles than their white counterparts. If white residents are highly mobile, their exposure to the food environment near home will only take up a small portion of their total food environment exposure. Dunn (2010) also provides a potential explanation that females may respond differently to the presence of fast food due to differences in household responsibilities. In other words, their opportunity cost for traveling further distances may be higher.
Alviola, Nayga, Thomsen, and Smartt (2014) use distance to a major highway as an instrument to examine the causal relationship between school-level food environment and obesity rates. They examine the number of fast food restaurants near high schools in Arkansas and find each additional fast food restaurant within a mile from school increases school-level obesity rates by 1.23 percentage points.
Another notable study by Currie, DellaVigna, Moretti, and Pathania (2010) uses two different approaches to investigate the impact of proximity to fast food on weight outcomes. First, they examine school-level obesity rates for fifth, seventh, and ninth graders in California and compare schools that have any fast food restaurants within a tenth of a mile, a quarter-mile, and a half-mile from school. Here, the identification assumption is that small differences in proximity do not correlate to unobservable differences between the groups. They find that having a fast food restaurant within one tenth of a mile rather than a quarter of a mile from school increases school-level obesity rates by 1.7 percentage points for ninth graders. They find small and statistically insignificant effects for fifth and seventh graders. Second, using birth certificate data in Michigan, New Jersey, and Texas, they examine the impact of living near fast food on weight gain between pregnancies among women who have at least two children. They find smaller yet significant effects of having a fast food restaurant within a half-mile of a residence.
Currie et al. (2010) also suggest the difference in the magnitude of the results between students and mothers is driven by relative travel costs. If traveling the same distance incurs lower travel costs for adults, students will be more affected by fast food restaurants in the immediate proximity. Following this logic, travel costs are likely to be lower for older students than younger students, implying that younger students will be more sensitive to proximity, conditional on autonomy in food consumption decisions. Put differently, among students old enough to purchase their own food, younger students might be more responsive to fast food availability nearby due to difficulties driving, walking, or using public transportation to travel further distances for food. However, the two existing studies on children – Alviola et al. (2014) and Currie et al. (2010) – focus on high school grade students with little attention on younger children.
Previous studies also do not provide evidence on the causal impact of the residential food environment on childhood obesity. While past studies on student obesity focus on the school food environment, many elementary and middle school students are not allowed to leave school for lunch and are less likely to be affected by the food environment surrounding their school ( Mirtcheva & Powell, 2009 ). This could potentially explain the null effects of fast food restaurants around school on obesity outcomes for younger students in Currie et al. (2010) . After school hours, students can substitute home-prepared meals with fast food near home or consume fast food in addition to home-prepared meals. Especially for younger students, who are more likely to attend a school close to home, fast food near home may take up a larger part of their total food environment exposure. Thus, residential food environment has important implications for student food consumption decisions, and its estimated effects for younger students are likely to differ from that of older students.
Finally, previous quasi-experimental studies typically lack data on non-restaurant food outlets that may also affect food consumption decisions and obesity outcomes. In particular, supermarkets or corner stores may be alternatives to fast food restaurants, and proximity to these food outlets will shape the cost of purchasing fast food. Furthermore, distance to fast food may be correlated with distance to corner stores with low-quality food sources and inversely correlated with distance to high-quality supermarkets, complicating the interpretation of the coefficients on fast food. In this study, we have data on individual-level weight outcomes for school-aged children in all grades and their food environment around home, including distances to different types of food outlets, which we use to estimate the effects of proximity to fast food on childhood obesity.
3. Public Housing and Residential Location
We focus on students living in public housing, because the institutional setting of public housing – and that of NYC public housing, in particular – provides plausibly random variation in individual proximity to fast food within a development. Public housing is a federally funded housing assistance program, administered and managed by local housing authorities like the NYC Housing Authority (NYCHA). A public housing development typically consists of one or more concentrated blocks of standardized high-rise (and sometimes low-rise) apartment buildings. NYCHA is the nation’s largest public housing system, containing 2,418 buildings in 149 developments dispersed across the city’s five boroughs – Manhattan, Bronx, Brooklyn, Queens, and Staten Island ( NYCHA, 2019 ). With roughly 174,000 households living in NYC public housing, an average NYCHA development has more than 16 buildings and approximately 150 residents per building.
To be clear, public housing is a place-based housing assistance program, in which program recipients are assigned to specific units that they can either “take or leave.” It differs from other tenant-based programs like the housing choice vouchers, which allow households to choose their neighborhoods and housing units in the private market. The assignment process into NYC public housing units makes it difficult for public housing applicants to choose the precise residential location of their preference. Furthermore, most inner-city public housing developments are oversubscribed, requiring local housing authorities to have long waiting lists and systemized processes of assigning tenants to public housing units. There is also a long waiting list to get into NYCHA public housing units. In this section, we describe NYCHA’s tenant assignment process that provides tenants little control over their choice of specific buildings, although they can specify some preference over locations.
More specifically, households can list up to two preferred boroughs on the application but are not permitted to list any preference for individual developments or buildings. After receiving applications, NYCHA assigns priority codes to eligible households, based on family size, income, needs (e.g., emergencies), and date of application ( NYCHA, 2020 ). NYCHA then conducts interviews to place households on its waiting list. While the details of the process differ by priority code, all households have limited choice of housing units.
Applicants can select one preferred development during the process, conditional on the development containing an anticipated vacancy. 2 A computer matches applicants to vacant units in the selected development. Applicants can receive up to two offers (i.e., applicants are permitted to reject the initial offer), but applications will be closed if applicants fail to choose a development within 30 days or if applicants reject the second offer ( NYCHA, 2020 ). “Emergency applicants,” while prioritized in the tenant selection process, may only select a preferred borough rather than a particular development. 3 They are matched to vacant units in the selected borough “without regard to any preference by the applicant for a particular development in that borough” ( NYCHA, 2020 ). Emergency applicants can also reject their initial offer, but their application will be closed if they reject the second offer. To summarize, the choice of development is constrained by anticipated vacancies around the time of the initial offer, and the choice of particular units or buildings within a development is more explicitly restricted.
A city-wide oversubscription for NYCHA public housing is likely to further discourage applicants from rejecting offers. From time to time, NYCHA closes its waiting list to control the volume of the applications it receives. Therefore, rejecting the second offer would increase households’ uncertainty around whether they can create new applications to get back on the waiting list. Previous research suggests only a few households turn down the initial set of offers for housing assistance programs with long waiting lists, since starting over the application may entail a substantial wait for and uncertainty regarding the availability of another unit ( Coley, Sullivan, & Kuo, 1997 ; Rosenbaum, 1995 ; DeLuca & Rosenbaum, 2003 ). 4 In the past five years, the average time between “date entered waiting list” and the “admission date” for NYCHA public housing has been more than 38 months ( HUD, 2019 ). This process creates random variation in the precise location of a student’s residence (and the subsequent food environment) within a public housing development, which we leverage to isolate the causal impact of proximity to fast food on children.
A small number of previous studies exploit the assignment process in public housing to identify causal estimates of neighborhood effects on individual outcomes. Two are particularly relevant. Oreopoulos (2003) focuses on the Toronto public housing program, in which applicants cannot specify development preferences, to examine the effects of neighborhoods on long-run labor market outcomes. Gou and Maurin (2007) focus on public housing in France, where public housing managers have a very limited set of units to offer each year to eligible families, to estimate neighborhood effects on academic success. Thus, both studies leverage the resulting quasi-random assignment to a particular public housing development and, therefore, neighborhood to isolate causal estimates of neighborhood effects. We employ a similar methodology but also exploit the within-development variation in proximity to neighborhood (dis)amenities. To summarize, we exploit the institutional setting of public housing that assigns children in different micro-neighborhood food environments to derive credibly causal estimates of living near fast food restaurants.
4. Data and Sample
4.1. student-level data.
Our analyses draw on a rich set of longitudinal, student-level data for NYC public school students, K-12, in AY 2009–2016. Administrative data from the NYC Department of Education (NYCDOE) include student residential location, school attended, socio-demographic variables, such as gender, race/ethnicity, grade, primary language spoken at home, and poverty status, 5 educational program participation (e.g., students with disabilities and English language learners), and critically, student height and weight measures from an annual FitnessGram®. The FitnessGram® measures provide weight and height of students every year, which we use to calculate student body mass index (BMI). We follow the Centers for Disease Control and Prevention guidelines and define students as obese if their BMI is at or above the 95th percentile of their age and sex group and overweight if their BMI is at or above the 85th percentile. In addition to the two binary weight outcome variables, we calculate the z-score of the BMI (zBMI), standardized by age and sex group, to examine the estimated effects on the weight distribution for later robustness checks. We link student residential location to data on the locations of NYCHA developments to create an identifier for each public housing development, which we use to derive a set of development fixed effects.
We also link the student-level data to the locations of restaurants and supermarkets. We follow Elbel et al. (2020) to create four food retail outlet variables derived from two data sources. Specifically, we use data on NYC restaurants from the NYC Department of Health and Mental Hygiene, including information on locations and the type of service provided (fast food or wait-service). We calculate the straight-line distance (in miles) between student residential location and the nearest fast food restaurant (DistFF) and the nearest wait-service restaurant (DistWaitService). 6 We then link to data on the locations and characteristics of food stores from the New York State Department of Agriculture and Markets to calculate distances to the nearest large supermarket (store greater than 6,000 square feet) and the nearest corner store (less than 2,000 square feet), respectively DistSupermarket and DistCornerStore. While our analyses focus on these continuous measures of student distance to food outlets, we create a set of binary variables indicating the presence of each food outlet type within 0.1 mile from home (e.g., AnyFF) as an alternate specification. We also create density measures by counting the total number of food outlets within 0.1 mile and within 0.25 mile from home (e.g., NumFF10 and NumFF25).
Finally, we calculate the straight-line distance between home and school attended in miles (DistSch). Using this, we create an indicator variable, SchNear, that identifies those who attend schools within a half-mile from home. Students in kindergarten, first, and second grade who travel less than a half-mile for school do not qualify for district-provided school buses in NYC and are, therefore, more likely to walk to school in the neighborhood. We also create SchFar to identify those who live far away (half-mile or more) from school and are thus eligible for school buses in the early grades. A second set of variables, SchNear36 and SchFar36, is similarly defined using a one-mile threshold, which determines school bus eligibility for students in grades 3–6. 7

4.2. Sample and Descriptive Statistics
Our analysis focuses on the students living in NYCHA public housing developments. The sample consists of 486,178 observations of K-12 NYC public school students in public housing for AY 2009–2016. Students missing weight and height data or residential location are not included in the sample. We further exclude outliers of non-poor students, who comprise less than 2 percent of the students living in public housing. Table 1 provides summary statistics of our analytic sample in all grades and by grade level. In addition to stratifying by elementary (K-5), middle (6–8), and high school grades (9–12), we separate students in grades K-2 from those in grades 3–5 to explore plausible heterogeneity within elementary school grade kids.
Mean student characteristics for all students and by grade level
All grades (1) | Grades K-2 (2) | Grades 3–5 (3) | Grades 6–8 (4) | Grades 9–12 (5) | |
---|---|---|---|---|---|
Obese | 0.232 (0.422) | 0.214 (0.410) | 0.270 (0.444) | 0.256 (0.436) | 0.195 (0.396) |
Overweight | 0.409 (0.492) | 0.380 (0.485) | 0.448 (0.497) | 0.445 (0.497) | 0.370 (0.483) |
DistFF | 0.099 (0.056) | 0.099 (0.056) | 0.099 (0.056) | 0.100 (0.057) | 0.099 (0.056) |
DistWaitService | 0.212 (0.168) | 0.213 (0.169) | 0.212 (0.167) | 0.215 (0.172) | 0.211 (0.164) |
DistSupermarket | 0.187 (0.145) | 0.189 (0.147) | 0.187 (0.144) | 0.188 (0.146) | 0.186 (0.143) |
DistCornerStore | 0.096 (0.067) | 0.096 (0.067) | 0.096 (0.067) | 0.097 (0.068) | 0.096 (0.066) |
DistSch | 1.480 (2.211) | 0.613 (1.458) | 0.720 (1.542) | 1.139 (1.706) | 3.053 (2.663) |
SchNear | 0.512 (0.500) | 0.817 (0.387) | 0.770 (0.421) | 0.461 (0.499) | 0.111 (0.314) |
SchNear36 | 0.641 (0.480) | 0.893 (0.309) | 0.854 (0.353) | 0.685 (0.465) | 0.235 (0.424) |
Female | 0.515 (0.500) | 0.508 (0.500) | 0.520 (0.500) | 0.520 (0.500) | 0.513 (0.500) |
Hispanic | 0.474 (0.499) | 0.474 (0.499) | 0.475 (0.499) | 0.477 (0.500) | 0.469 (0.499) |
Asian | 0.047 (0.213) | 0.039 (0.194) | 0.041 (0.199) | 0.047 (0.211) | 0.059 (0.236) |
Black | 0.462 (0.499) | 0.467 (0.499) | 0.466 (0.499) | 0.461 (0.499) | 0.456 (0.498) |
White | 0.017 (0.128) | 0.020 (0.138) | 0.018 (0.131) | 0.015 (0.123) | 0.015 (0.121) |
Grade | 5.895 (3.605) | 1.029 (0.808) | 3.993 (0.818) | 7.035 (0.817) | 10.269 (1.093) |
Student with disability | 0.188 (0.391) | 0.157 (0.364) | 0.201 (0.401) | 0.203 (0.402) | 0.190 (0.392) |
English language learner | 0.075 (0.264) | 0.089 (0.284) | 0.084 (0.278) | 0.069 (0.253) | 0.063 (0.244) |
N | 486,178 | 111,477 | 113,070 | 119,687 | 141,944 |
Notes : Standard deviations are shown in parentheses. Sample consists of NYC public school students, ever eligible for free and reduced-price lunch and living in NYCHA public housing, for AY 2009–2016. All distances are in miles.
Table 1 shows that 23.2 percent of students in our analytic sample are obese and 40.9 percent are overweight. Obesity rates differ across grade levels, where students in grades 3–5 are more likely to be obese (27 percent) and high school students are less likely to be obese (19.5 percent) than students in other grade levels. A majority of our analytic sample are either Hispanic (47.4 percent) or black (46.2 percent), and less than 10 percent, across all grade levels, are Asian or white. Approximately half of the students are female (51.5 percent). Moreover, students in higher grade levels are likely to travel further distances for school. While less than a quarter of elementary school students attend schools more than a half-mile from home, almost 90 percent of high school students attend schools outside a half-mile radius from home. However, there is less, if any, variation in distances to the nearest food outlets across grade levels. On average, students live approximately 0.1 mile (around two city blocks in NYC) from the nearest fast food restaurant regardless of grade level.
Critical to our study is the within-development variation in distances to fast food. To explore this, we plot the distribution of DistFF in each of the 139 public housing developments in Figure 1 . In this figure, each line shows the range of student distance to the nearest fast food at 5% and 95% of the distribution in a given development. The first range plot presented in Figure 1 , for example, shows that one student would have to travel 0.2 mile (around four city blocks) further to reach the nearest fast food restaurant from home compared to another student in the same development. The plotted range of DistFF within developments suggests the within-development distance between buildings can span multiple blocks and place children in substantially different micro-neighborhood food environments. A decomposition of the variation in DistFF, in a one-way analysis of variance (ANOVA), indicates that almost half of the variation (47.8 percent) is within developments and only slightly more (52.2 percent) is between developments.

Notes: Each range plot shows student distance to the nearest fast food at the 5% and 95% of the distribution in a given development and the outliers through dots. Sample consists of NYC public school students, ever eligible for free and reduced-price lunch and living in NYCHA public housing, for AY 2009–2016.
4.3. Exploring the Within-Development Variation in Local Food Environments
Before turning to models, we explore the empirical support for the claim that the within-development variation in distance between residence and fast food is plausibly random. To do so, we estimate a series of regression models that examine the correlation between distance to the nearest food outlet and student characteristics, using a set of development and year fixed effects. We use DistFF, DistWaitService, DistSupermarket, and DistCornerStore as the outcome and link them to a vector of student demographic variables, including gender, race/ethnicity (Asian, black, or white, using Hispanic as the reference group) and grade level (grades 3–5, grades 6–8, or grades 9–12, using grades K-2 as the reference group).
The results in Table 2 provide little evidence of a meaningful relationship between distance to food outlets and student characteristics. The magnitudes of all coefficients are substantively unimportant, ranging from −0.002 to 0.001, although some are statistically significant. 8 The coefficient for black, for example, indicates that black students are 0.001 mile, or five feet, further away from the nearest fast food restaurant than Hispanic students living in the same development. This represents one fiftieth of a typical city block in NYC. Similarly, estimates suggest older students (in middle school and high school grades) live 0.001 mile further from the nearest fast food restaurant than younger kids (grades K-2) in the same development. These distances are not economically meaningful and bolster our confidence that the causal interpretation of our estimates is warranted.
Relationship between student demographic characteristics and proximity to food outlets
Dependent variable: | DistFF (1) | DistWaitService (2) | DistSupermarket (3) | DistCornerStore (4) |
---|---|---|---|---|
Female | −0.000 (0.000) | 0.000 (0.000) | −0.000 (0.000) | −0.000 (0.000) |
Hispanic | - | - | - | - |
Asian | 0.000 (0.000) | −0.001 (0.001) | −0.002 (0.001) | 0.000 (0.000) |
Black | 0.001 (0.000) | 0.001 (0.000) | 0.000 (0.000) | 0.001 (0.000) |
White | −0.000 (0.001) | −0.001 (0.001) | −0.001 (0.001) | −0.000 (0.001) |
Grades K-2 | - | - | - | - |
Grades 3–5 | 0.000 (0.000) | 0.000 (0.000) | 0.000 (0.000) | 0.000 (0.000) |
Grades 6–8 | 0.001 (0.000) | −0.000 (0.000) | 0.001 (0.000) | 0.000 (0.000) |
Grades 9–12 | 0.001 (0.000) | −0.001 (0.000) | 0.001 (0.000) | 0.000 (0.000) |
N | 486,178 | 486,178 | 486,178 | 486,178 |
Year FX | Y | Y | Y | Y |
Development FX | Y | Y | Y | Y |
Notes : Robust standard errors are shown in parentheses (*** p<0.01, ** p<0.05, * p<0.1).
Each column is a different regression. Sample consists of NYC public school students, ever eligible for free and reduced-price lunch and living in NYCHA public housing, for AY 2009–2016.
5. Empirical Strategy
5.1. regression models.
As described previously, we exploit the exogenous within-development variation in distance to fast food and identify the effects of proximity to fast food by comparing weight outcomes among students living in the same development but in different buildings (thus with different micro-neighborhood food environments). Our baseline model contains the following elements:
where Y idt represents the weight outcome (obese and overweight) of student i in development d in year t. DistFF idt captures student distance to the nearest fast food restaurant in miles. A vector of student characteristics (shown in Table 1 ) are included in the equation as X idt , and year fixed effects, τ t , control for secular trends. Finally, δ d are development fixed effects, such that our coefficient of interest, β 1 , is identified by the variation in DistFF within developments. An alternate specification includes and controls for student distance to other food outlets (DistWaitService, DistSupermarket, and DistCornerStore), which may also affect the relative travel cost for DistFF and child weight outcomes.
We first estimate this baseline model on our full analytic sample of students in all grades (K-12) and then stratify by grade levels to shed light on heterogeneity across grades, as discussed in earlier sections. We then explore differences in the estimated effects of DistFF between students who live near enough to school to be in the early grades “walk zone” of a half-mile and those who live farther away with the following model:
where we fully interact DistFF with binary indicators of student distance to school, SchNear and SchFar, to allow the estimated impact of DistFF to vary by student distance to school. Again, we first estimate this model on the full analytic sample and then stratify by grade level, with and without controlling for distances to other food outlets. Following previous studies that find stronger effects of proximity to fast food on obesity outcomes among minorities and women, we also examine heterogeneity by student race/ethnicity and gender.
5.2. Robustness Checks
We also explore the robustness of our results to alternative specifications and measures. First, we re-estimate our models using zBMI, instead of the binary indicators obese and overweight. Second, we explore alternative ways of capturing the food environment, substituting continuous distance measures with binary indicators, such as AnyFF . We also control for the density of food outlets by within different radiuses from home, including NumFF10 and NumFF25. Finally, we use alternative measures for distance to school, replacing SchNear and SchFar with SchNear36 and SchFar36, constructed using a one-mile threshold, and using the continuous measure of student distance between home and school in miles, DistSch, instead of the indicator variables.
6.1. Impact of Proximity to Fast Food by Grade Level
Baseline results in Table 3 show the estimated impact of proximity to fast food on student weight outcomes for K-12 students. Consistently negative and statistically significant coefficients for DistFF suggest that proximity to fast food increases student probability of being obese and overweight. Indeed, every additional 0.1 mile (or two city blocks) separating the nearest fast food restaurant from a student’s residence decreases the probability of being obese by approximately 0.6 percentage points. The effects on overweight range between 0.93 to 1.11 percentage point increases, depending on the inclusion of distances to other food outlets in the model. We see little evidence that proximity to other food outlets matters. Coefficients on the distances to other food outlets (see full results in Table A.1 ) are small and statistically insignificant.
Baseline impact of proximity to fast food on weight outcomes, K-12
Dependent variable: | Obese (1) | Obese (2) | Overweight (3) | Overweight (4) |
---|---|---|---|---|
DistFF | −0.058 (0.016) | −0.062 (0.019) | −0.093 (0.018) | −0.111 (0.022) |
N | 486,178 | 486,178 | 486,178 | 486,178 |
Dist. to other food | - | Y | - | Y |
Student characteristics | Y | Y | Y | Y |
Year FX | Y | Y | Y | Y |
Development FX | Y | Y | Y | Y |
Each column is a different regression. Distance to other food include mile-distances to the nearest wait-service restaurant, supermarket, and corner stores. Student characteristics include gender, race/ethnicity, grade, primary language spoken at home, special education, and limited English proficiency status. Sample consists of NYC public school students, ever eligible for free and reduced-price lunch and living in NYCHA public housing, AY 2009–2016.
As described earlier, we estimate the impact of DistFF by student grade level and report separate coefficients for students in grades K-2, grades 3–5, grades 6–8, and grades 9–12. Estimates in Table 4 suggest that the baseline effects are largely driven by older elementary school students (in grades 3–5) and middle school students (in grades 6–8). For every 0.1 mile a student lives further away from the nearest fast food, the probability of being obese decreases by 1.39 to 1.42 percentage points and overweight decreases by 1.66 to 1.86 percentage points for students in grades 3–8 (see Table 4 Columns 2 and 4). To understand the magnitude of the effects, consider the group mean obesity rate of 27 percent for older elementary school students and 25.6 percent for middle school students (see Table 1 ). A 1.39 to 1.42 percentage point increase translates to approximately a 5.4 percent increase in obesity rate for living two blocks closer to the nearest fast food.
Baseline impact of proximity to fast food on weight outcomes by grade level
Dependent variable: | Obese (1) | Obese (2) | Overweight (3) | Overweight (4) |
---|---|---|---|---|
DistFF | 0.000 (0.032) | 0.023 (0.039) | −0.050 (0.037) | −0.065 (0.046) |
N | 111,477 | 111,477 | 111,477 | 111,477 |
DistFF | −0.129 (0.034) | −0.142 (0.042) | −0.149 (0.038) | −0.166 (0.047) |
N | 113,070 | 113,070 | 113,070 | 113,070 |
DistFF | −0.115 (0.032) | −0.139 (0.040) | −0.153 (0.037) | −0.186 (0.045) |
N | 119,687 | 119,687 | 119,687 | 119,687 |
DistFF | −0.003 (0.027) | −0.001 (0.033) | −0.039 (0.033) | −0.044 (0.040) |
N | 141,944 | 141,944 | 141,944 | 141,944 |
Dist. to other food | - | Y | - | Y |
Student characteristics | Y | Y | Y | Y |
Year FX | Y | Y | Y | Y |
Development FX | Y | Y | Y | Y |
Notes: Robust standard errors are shown in parentheses (*** p<0.01, ** p<0.05, * p<0.1).
Each column in each panel is a different regression. See notes in Table 3 for variable and sample descriptions.
In contrast, the estimated effects on K-2 students and high school students are smaller in magnitude and, more importantly, statistically insignificant across all models using different weight outcomes and controls for distance to other food outlets. Students in grades K-2 may not be old enough to exercise independent food consumption decisions regardless of fast food locations near home. High school students, who tend to travel the furthest for school (see Table 1 ), may have exposure to food environment outside their residential neighborhood and, therefore, appear to be less sensitive to the micro-neighborhood food environments near home.
6.2. Does School Proximity Matter?
Estimates in Table 5 show that the impact of proximity to fast food near home differs by distance to school among students in all grade levels, except for K-2 students. Negative coefficients of DistFF for students attending neighborhood schools (coefficient on DistFFxSchNear) are always larger in magnitude than the corresponding coefficient for students attending schools farther away ( DistFFxSchFar ). 9 For example, among students in grade 3–5 (see Table 5 Panel B Columns 1 and 2), the estimated effects of DistFFxSchNear on obesity is larger by approximately 0.6 percentage points for every 0.1-mile increase compared to those of DistFFxSchFar (−0.144 vs. −0.081 and −0.158 vs. −0.095). The impact of living near fast food on obesity outcomes is almost 1.78 times larger for students attending schools nearby. We also find similar patterns for obesity outcomes among middle school students (see Table 5 Panel C). The impact of living 0.1 mile closer to fast food increases probability of obese by 1.43 to 1.68 percentage points for students attending neighborhood schools, approximately 0.5 percentage points larger than those attending schools farther away. These estimates imply that every 0.1 mile closer a student lives to fast food translates into approximately a 6 and 7 percent increase in obesity rates (and a 4.2 and 4.7 percent increase in overweight rates) respectively for older elementary students and middle school students that attend neighborhood schools.
Impact of proximity to fast food on weight outcomes by grade level and whether a student attends a school within 0.5 mile from home
Dependent variable: | Obese (1) | Obese (2) | Overweight (3) | Overweight (4) |
---|---|---|---|---|
DistFFxSchNear | −0.004 (0.032) | 0.019 (0.039) | −0.059 (0.038) | −0.074 (0.046) |
DistFFxSchFar | 0.017 (0.039) | 0.041 (0.045) | −0.014 (0.046) | −0.029 (0.053) |
P-value for joint F-test | 0.451 | 0.446 | 0.179 | 0.180 |
N | 111,477 | 111,477 | 111,477 | 111,477 |
DistFFxSchNear | −0.144 (0.035) | −0.158 (0.043) | −0.174 (0.039) | −0.191 (0.047) |
DistFFxSchFar | −0.081 (0.040) | −0.095 (0.047) | −0.073 (0.045) | −0.090 (0.053) |
P-value for joint F-test | 0.026 | 0.026 | 0.046 | 0.045 |
N | 113,070 | 113,070 | 113,070 | 113,070 |
DistFFxSchNear | −0.143 (0.036) | −0.168 (0.043) | −0.175 (0.041) | −0.208 (0.048) |
DistFFxSchFar | −0.096 (0.034) | −0.120 (0.041) | −0.139 (0.039) | −0.171 (0.047) |
P-value for joint F-test | 0.061 | 0.059 | 0.203 | 0.195 |
N | 119,687 | 119,687 | 119,687 | 119,687 |
DistFFxSchNear | −0.031 (0.043) | −0.029 (0.047) | −0.126 (0.052) | −0.129 (0.057) |
DistFFxSchFar | −0.001 (0.027) | 0.001 (0.034) | −0.032 (0.033) | −0.036 (0.041) |
P-value for joint F-test | 0.389 | 0.390 | 0.031 | 0.031 |
N | 141,944 | 141,944 | 141,944 | 141,944 |
Dist. to other food | - | Y | - | Y |
Student characteristics | Y | Y | Y | Y |
Year FX | Y | Y | Y | Y |
Development FX | Y | Y | Y | Y |
Each column in each panel is a different regression. See notes in Table 3 for variable and sample descriptions. We test whether the coefficients for DistFFxSchNear and DistFFxSchFar are statistically different from each other and present the p-value of the joint F-test.
As for high school students, in Panel D, the coefficient on DistFFxSchNear indicates statistically significant, negative effects on overweight. The estimates suggest living 0.1 mile closer to the nearest fast food increases high school students’ probability of being overweight by approximately 1.3 percentage points, and the effect is statistically different from that of DistFFxSchFar. Thus, even for high school students, those attending neighborhood schools are affected by fast food near home. To understand the magnitude of the effects, consider the base overweight rate of 37 percent for high school students (see Table 1 ). An increase in the probability of being overweight by 1.3 percentage points represents a 3.5 percent increase in overweight rates.
Overall, the detrimental effects of living near fast food are largest among those students who are most likely to have meaningful autonomy in food decisions and, at the same time, are likely to spend a significant amount of their free time in their residential neighborhood. Results are robust to clustering standard errors at the development level (see Table A.2 and more on online appendix ). Although standard errors are slightly larger, our key coefficients are still statistically significant at conventional levels.
6.3. Heterogeneity by Race and Gender and Robustness Checks
In Table 6 , our analyses reveal considerable heterogeneity in impact across demographic groups, consistent with findings from previous research. First, we see negative and statistically significant effects of DistFF for black students, with similar evidence for Hispanic students. For black students, living 0.1 mile closer to fast food increases the probability of being obese by 0.9 to 1.05 percentage points and overweight by 1.08 to 1.17 percentage points. For Hispanic students the effects on overweight ranges between 0.8 to 1.28 percentage points. We see little evidence of the effects on weight outcomes among Asian and white students. 10 In Table 7 , we report separate results by gender and find that boys are more sensitive to proximity to fast food near home than girls. While this may reflect greater autonomy granted to boys than girls, other underlying mechanisms are possible and warrant further research.
Baseline impact of proximity to fast food on weight outcomes by race/ethnicity
Dependent variable: | Obese (1) | Obese (2) | Overweight (3) | Overweight (4) |
---|---|---|---|---|
DistFF | −0.082 (0.060) | −0.058 (0.076) | −0.014 (0.080) | −0.016 (0.102) |
N | 22,912 | 22,912 | 22,912 | 22,912 |
DistFF | −0.016 (0.024) | −0.045 (0.030) | −0.080 (0.028) | −0.128 (0.034) |
N | 230,252 | 230,252 | 230,252 | 230,252 |
DistFF | −0.105 (0.022) | −0.090 (0.027) | −0.117 (0.026) | −0.108 (0.031) |
N | 224,794 | 224,794 | 224,794 | 224,794 |
DistFF | 0.232 (0.121) | 0.225 (0.146) | 0.064 (0.141) | 0.018 (0.170) |
N | 8,090 | 8,090 | 8,090 | 8,090 |
Dist. to other food | - | Y | - | Y |
Student characteristics | Y | Y | Y | Y |
Year FX | Y | Y | Y | Y |
Development FX | Y | Y | Y | Y |
Baseline impact of proximity to fast food on weight outcomes by gender
Dependent variable: | Obese (1) | Obese (2) | Overweight (3) | Overweight (4) |
---|---|---|---|---|
DistFF | −0.113 (0.023) | −0.112 (0.028) | −0.108 (0.026) | −0.116 (0.032) |
N | 235,740 | 235,740 | 235,740 | 235,740 |
DistFF | −0.004 (0.022) | −0.015 (0.026) | −0.074 (0.025) | −0.104 (0.031) |
N | 250,438 | 250,438 | 250,438 | 250,438 |
Dist. to other food | - | Y | - | Y |
Student characteristics | Y | Y | Y | Y |
Year FX | Y | Y | Y | Y |
Development FX | Y | Y | Y | Y |
The findings from our series of robustness checks suggest the results are not sensitive to alternative measures and specifications. First, results are robust to measuring weight outcomes using zBMI, rather than indicators for obese or overweight (see Table A.3 and more on online appendix ). Living 0.1 mile closer to the nearest fast food increases student BMI by approximately 0.03 standard deviations, or 4.3 percent of the sample’s mean zBMI. Second, results are substantively unchanged by alternative measures of the food environment (see Table A.4 ). The probability of being obese is 0.6 percentage points higher (and overweight is 0.7 percentage points higher) for students who travel less than 0.1 mile to the nearest fast food restaurant. Third, results are substantively unchanged by including controls for density of the fast food restaurants (see Table A.5 ). Finally, we examine whether alternative specifications for distance to school yield similar results. Models with interaction terms using SchNear36 and SchFar36 (see Table A.7 ) and DistSch (see Table A.8 ) in place of SchNear and SchFar consistently show that students who travel further distances to school are less likely to be affected by DistFF. We also see in Table A.6 that including DistSch as a control, instead of interacting DistSch with DistFF, does not change the coefficients for DistFF. In other words, the moderating effects of attending schools nearby on the relationship between proximity to fast food near home and weight outcomes are robust to different specifications for distance to school.
7. Discussion and Policy Implications
A wide range of policymakers, advocates, and “urbanists” blame the ease of access to unhealthy food outlets and particularly fast food as the culprit for high obesity rates among low income, minority children. There are, however, few credibly causal empirical findings on the effects of proximity to fast food on childhood obesity, mainly due to the endogenous nature of fast food locations and scarcity of the requisite micro-data linking children weight to the food environment. In this paper, we overcome the two key empirical obstacles using a detailed set of individual-level data on students living in public housing and exploiting their quasi-random assignment into micro-neighborhood food environments. Specifically, we use administrative data on NYC public school students living in public housing and link their weight outcomes and residential locations with all restaurant locations in NYC. We then leverage the plausibly random within-development variation in distance between residence and fast food generated by NYCHA’s tenant assignment process to derive credibly causal estimates of the effects of living near fast food. To our knowledge, this is the first paper to use this particular identification strategy.
Our results suggest significant deleterious effects of proximity to fast food for student weight outcomes, with the largest effects among students in grades 3–8 attending neighborhood schools. Economic theory predicts that individuals with relatively higher travel costs are more sensitive to fast food availability in close proximity. Currie et al. (2010) , for instance, find that proximity to fast food has larger effects on high school students than pregnant mothers, providing lower travel costs for adults as a potential explanation. In this paper, we exploit a detailed set of data on public housing students in all grades, and our results support the theory that living near fast food has larger impacts on younger students who are likely to have higher travel costs but old enough to make independent food purchasing decisions. In addition to heterogeneity across grade levels, students attending neighborhood schools are also likely to face higher costs traveling outside their residential neighborhood to purchase food, compared to students who attend schools far from home. We also find that students who travel shorter distances to school are more sensitive to fast food proximity in their micro-neighborhood environment. For students in grades 3–8 attending neighborhood schools, the probability of being obese (overweight) increases up to 1.7 (2.1) percentage points for every one tenth mile decrement in distance between home and fast food. These are sizable magnitudes, roughly representing a 12 percent increase in obesity rates (9.4 percent for overweight) for a four-block reduction in distance to the nearest fast food.
We note two key limitations of our study results. First, the location of fast food restaurants may be related to the availability of other neighborhood amenities that may affect student weight outcomes such that our estimates would reflect the combined effects of proximity to fast food and proximity to other unobserved amenities. However, it is reassuring – although not dispositive – that our results are robust to including controls for proximity to other food outlets. Second, our work focuses on public school children living in NYC public housing, a population disproportionately black, Hispanic, and urban. Investigating whether and how proximity to fast food affects higher-income students or those living in lower-density suburban and rural areas with greater reliance on cars remains for future research.
Our study results are particularly relevant to place-based interventions that attempt to limit unhealthy food outlets in an urban context to reduce the prevalence of obesity in low-income, minority neighborhoods. We suggest such interventions might include zoning regulations that restrict openings of fast food outlets in designated areas of a city. In a different vein, school policies regarding the quality or price of school lunch or “open-campus” policies governing student’s ability to exit during school lunch periods might also be relevant. In summary, our findings suggest fast food locations near residence have sizable impacts on childhood obesity and warrant the attention of policymakers hoping to identify policy levers to reduce access to or consumption of fast food among poor, urban children.
- The random micro-variation in residential location created by the tenant assignment process in oversubscribed public housing can be used to identify causal effects of proximity to neighborhood (dis)amenities.
- Proximity to fast food restaurants has economically and statistically significant deleterious effects on weight outcomes for low-income children.
- Effects are largest among students attending neighborhood schools (within a half mile of home) and those in grades 3–8.
Supplementary Material
Acknowledgements.
The authors would like to thank the New York City Department of Education, the New York City Department of Health and Mental Hygiene, and especially Zac McDermott, Joanna Rae Bailey, Meryle Weinstein, and Jeremy Sze, for providing data and support. We also thank seminar participants at the Maxwell School at Syracuse University, the American Real Estate and Urban Economics Association, and the Urban Economics Association.
Funding: This study was supported by the National Institutes of Health, Award Numbers R01HD070739, R01DK097347, and R01DK108682-01. The funder had no role in study design, data collection and analysis, decision to publish, or preparation of the manuscript.
Full results for the impact of proximity to food outlets on weight outcomes
Dependent variable: | Obese (1) | Overweight (2) |
---|---|---|
DistFF | −0.062 (0.019) | −0.111 (0.022) |
DistWaitSerivce | 0.004 (0.008) | −0.004 (0.009) |
DistSupermarket | −0.000 (0.009) | −0.002 (0.011) |
DistCornerStore | 0.003 (0.017) | 0.036 (0.020) |
N | 486,178 | 486,178 |
Student characteristics | Y | Y |
Year FX | Y | Y |
Development FX | Y | Y |
Each column is a different regression. See notes in Table 3 .
Impact of proximity to fast food on weight outcomes, K-12, clustered standard errors
Dependent variable: | Obese (1) | Obese (2) | Overweight (3) | Overweight (4) |
---|---|---|---|---|
DistFF | −0.058 (0.029) | −0.062 (0.033) | −0.093 (0.034) | −0.111 (0.042) |
N | 486,178 | 486,178 | 486,178 | 486,178 |
Dist. to other food | - | Y | - | Y |
Student characteristics | Y | Y | Y | Y |
Year FX | Y | Y | Y | Y |
Development FX | Y | Y | Y | Y |
Notes: Standard errors are clustered at the development level and are shown in parentheses (*** p<0.01, ** p<0.05, * p<0.1). Each column is a different regression. See notes in Table 3 for variable and sample descriptions.
Impact of proximity to fast food on zBMI
Dependent variable: | zBMI (1) | zBMI (2) |
---|---|---|
DistFF | −0.266 (0.043) | −0.245 (0.053) |
N | 486,178 | 486,178 |
Dist. to other food | - | Y |
Student characteristics | Y | Y |
Year FX | Y | Y |
Development FX | Y | Y |
Each column is a different regression. See notes in Table 3 for variable and sample descriptions.
Impact of proximity to fast food on weight outcomes, using binary distance measures
Dependent variable: | Obese (1) | Obese (2) | Overweight (3) | Overweight (4) |
---|---|---|---|---|
AnyFF | −0.006 (0.002) | −0.006 (0.002) | −0.007 (0.002) | −0.007 (0.002) |
N | 486,178 | 486,178 | 486,178 | 486,178 |
Dist. to other food | - | Y | - | Y |
Student characteristics | Y | Y | Y | Y |
Year FX | Y | Y | Y | Y |
Development FX | Y | Y | Y | Y |
Impact of proximity to fast food on weight outcomes, controlling for density measures
Dependent variable: | Obese (1) | Obese (2) | Overweight (3) | Overweight (4) |
---|---|---|---|---|
DistFF | −0.069 (0.017) | −0.069 (0.021) | −0.112 (0.020) | −0.127 (0.024) |
NumFF10 | −0.001 (0.000) | −0.001 (0.000) | −0.001 (0.000) | −0.001 (0.000) |
NumFF25 | 0.000 (0.000) | 0.000 (0.000) | 0.000 (0.000) | −0.000 (0.000) |
N | 486,178 | 486,178 | 486,178 | 486,178 |
AnyFF | −0.008 (0.002) | −0.008 (0.002) | −0.009 (0.002) | −0.009 (0.002) |
NumFF10 | −0.001 (0.000) | −0.001 (0.000) | −0.001 (0.000) | −0.001 (0.000) |
NumFF25 | 0.000 (0.000) | 0.000 (0.000) | 0.000 (0.000) | 0.000 (0.000) |
N | 486,178 | 486,178 | 486,178 | 486,178 |
Dist. to other food | - | Y | - | Y |
Num. of other food | - | Y | - | Y |
Student characteristics | Y | Y | Y | Y |
Year FX | Y | Y | Y | Y |
Development FX | Y | Y | Y | Y |
Impact of proximity to fast food on weight outcomes by grade level, controlling for distance to school
Dependent variable: | Obese (1) | Obese (2) | Overweight (3) | Overweight (4) |
---|---|---|---|---|
DistFF | 0.001 (0.032) | 0.024 (0.039) | −0.049 (0.037) | −0.065 (0.046) |
DistSch | −0.001 (0.001) | −0.001 (0.001) | −0.002 (0.001) | −0.002 (0.001) |
N | 111,477 | 111,477 | 111,477 | 111,477 |
DistFF | −0.128 (0.034) | −0.142 (0.042) | −0.149 (0.038) | −0.166 (0.047) |
DistSch | −0.002 (0.001) | −0.002 (0.001) | −0.001 (0.001) | −0.001 (0.001) |
N | 113,070 | 113,070 | 113,070 | 113,070 |
DistFF | −0.113 (0.032) | −0.138 (0.040) | −0.152 (0.037) | −0.185 (0.045) |
DistSch | −0.002 (0.001) | −0.002 (0.001) | −0.001 (0.001) | −0.001 (0.001) |
N | 119,687 | 119,687 | 119,687 | 119,687 |
DistFF | −0.003 (0.027) | −0.001 (0.033) | −0.039 (0.033) | −0.044 (0.040) |
DistSch | 0.000 (0.000) | 0.000 (0.000) | 0.000 (0.000) | 0.000 (0.000) |
N | 141,944 | 141,944 | 141,944 | 141,944 |
Dist. to other food | - | Y | - | Y |
Student characteristics | Y | Y | Y | Y |
Year FX | Y | Y | Y | Y |
Development FX | Y | Y | Y | Y |
Impact of proximity to fast food on weight outcomes by grade level and whether a student attends a school within 1 mile from home
Dependent variable: | Obese (1) | Obese (2) | Overweight (3) | Overweight (4) |
---|---|---|---|---|
DistFFxSchNear36 | 0.003 (0.032) | 0.026 (0.039) | −0.047 (0.038) | −0.063 (0.046) |
DistFFxSchFar36 | −0.021 (0.044) | 0.002 (0.049) | −0.068 (0.052) | −0.083 (0.058) |
N | 111,477 | 111,477 | 111,477 | 111,477 |
DistFFxSchNear36 | −0.134 (0.035) | −0.148 (0.043) | −0.161 (0.039) | −0.179 (0.047) |
DistFFxSchFar36 | −0.103 (0.044) | −0.116 (0.050) | −0.088 (0.049) | −0.105 (0.056) |
N | 113,070 | 113,070 | 113,070 | 113,070 |
DistFFxSchNear36 | −0.115 (0.034) | −0.139 (0.041) | −0.153 (0.038) | −0.186 (0.046) |
DistFFxSchFar36 | −0.115 (0.036) | −0.139 (0.043) | −0.153 (0.041) | −0.185 (0.049) |
N | 119,687 | 119,687 | 119,687 | 119,687 |
DistFFxSchNear36 | −0.039 (0.033) | −0.038 (0.038) | −0.086 (0.040) | −0.091 (0.047) |
DistFFxSchFar36 | 0.007 (0.028) | 0.008 (0.034) | −0.027 (0.033) | −0.031 (0.041) |
N | 141,944 | 141,944 | 141,944 | 141,944 |
Dist. to other food | - | Y | - | Y |
Student characteristics | Y | Y | Y | Y |
Year FX | Y | Y | Y | Y |
Development FX | Y | Y | Y | Y |
Impact of proximity to fast food on weight outcomes by grade level and distance to school
Dependent variable: | Obese (1) | Obese (2) | Overweight (3) | Overweight (4) |
---|---|---|---|---|
DistFF | −0.002 (0.033) | 0.021 (0.040) | −0.059 (0.039) | −0.075 (0.047) |
DistFFxDistSch | 0.004 (0.012) | 0.004 (0.012) | 0.016 (0.015) | 0.016 (0.015) |
DistSch | −0.001 (0.002) | −0.001 (0.002) | −0.004 (0.002) | −0.004 (0.002) |
N | 111,477 | 111,477 | 111,477 | 111,477 |
DistFF | −0.142 (0.036) | −0.156 (0.043) | −0.159 (0.040) | −0.176 (0.048) |
DistFFxDistSch | 0.018 (0.013) | 0.018 (0.013) | 0.013 (0.015) | 0.013 (0.015) |
DistSch | −0.004 (0.002) | −0.004 (0.002) | −0.002 (0.002) | −0.002 (0.002) |
N | 113,070 | 113,070 | 113,070 | 113,070 |
DistFF | −0.147 (0.035) | −0.171 (0.042) | −0.161 (0.040) | −0.194 (0.048) |
DistFFxDistSch | 0.027 (0.011) | 0.027 (0.011) | 0.007 (0.012) | 0.007 (0.012) |
DistSch | −0.006 (0.001) | −0.006 (0.001) | −0.002 (0.002) | −0.002 (0.002) |
N | 119,687 | 119,687 | 119,687 | 119,687 |
DistFF | −0.039 (0.034) | −0.038 (0.039) | −0.080 (0.041) | −0.085 (0.048) |
DistFFxDistSch | 0.011 (0.007) | 0.011 (0.007) | 0.012 (0.008) | 0.013 (0.008) |
DistSch | −0.001 (0.001) | −0.001 (0.001) | −0.001 (0.001) | −0.001 (0.001) |
N | 141,944 | 141,944 | 141,944 | 141,944 |
Dist. to other food | - | Y | - | Y |
Student characteristics | Y | Y | Y | Y |
Year FX | Y | Y | Y | Y |
Development FX | Y | Y | Y | Y |
Conflict of Interest
The authors have no conflict of interest to declare.
1 Yet a recent empirical study finds that exposing low-income households to the same produces and prices available to high-income households do not change their demand for healthy groceries ( Allcott et al., 2019 ).
2 Development selection should be from one of the two boroughs listed in their initial application form.
3 Emergency applicants are households with children that are either homeless, victims of domestic violence, or intimidated witnesses, and borough selection should also be from one of the two boroughs listed on their initial application form.
4 Drawing on student-level data in England, Weinhardt (2014) finds that precise timing of moving to neighborhoods with oversubscribed social housing is uncorrelated with any observable individual characteristics, suggesting households that apply for housing assistance programs with long waiting lists are likely to accept available offers regardless of their individual taste.
5 Poverty status is defined by whether students were ever eligible for free or reduced-price lunch (household incomes below 185 percent of the federal poverty level) in AY 2001–2016.
6 Street network distances were correlated with straight-line distances at more than 90 percent.
7 Half-mile and one-mile thresholds are used by the NYCDOE to determine school bus eligibility ( NYCDOE, 2020 ). K-2 students are eligible for school buses when they live further than half a mile from school, and students in grades 3–6 are eligible when they live more than a mile from school. Students in grades 7–12 are not eligible for school bus regardless of their distance between home and school; however, students in grades 7–8 living in Staten Island would be eligible for school buses at 1 mile. For other types of pupil transportation, students are eligible for half-fare and full-fare MetroCards. Students in grades K-2 are eligible for half-fare if they travel less than 0.5 mile for school and for full-fare if more than 0.5 mile; students in grades 3–6 are eligible for half-fare if they travel between 0.5 to 1 mile for school and for full-fare if they travel more than 1 mile; students in grades 7–12 are eligible for half-fare if they travel between 0.5 to 1.5 mile for school and for full-fare if they travel more than 1.5 mile.
8 Results are robust to standardizing coefficients (see online appendix ). Differences in distances to food outlets are small and not economically meaningful across student demographic characteristics.
9 For each model in Table 5 , we test whether the coefficients for the interaction terms are statistically different from each other and report the p-value of the joint F-test.
10 Note that the differences in effects by race/ethnicity may reflect income differences across racial and ethnic subgroups within our low-income populations. Unfortunately, our data do not include information on household income, but future research exploring the heterogeneity across income groups within public housing and the relationship across racial subgroups is clearly warranted.
Publisher's Disclaimer: This is a PDF file of an unedited manuscript that has been accepted for publication. As a service to our customers we are providing this early version of the manuscript. The manuscript will undergo copyediting, typesetting, and review of the resulting proof before it is published in its final form. Please note that during the production process errors may be discovered which could affect the content, and all legal disclaimers that apply to the journal pertain.
Contributor Information
Jeehee Han, Syracuse University.
Amy Ellen Schwartz, Syracuse University.
Brian Elbel, New York University.
- Allcott H, Diamond R, Dube JP, Handbury J, Rahkovsky I, & Schnell M (2019). Food deserts and the causes of nutritional inequality . The Quarterly Journal of Economics , 134 ( 4 ), 1793–1844. [ Google Scholar ]
- Alviola IV PA, Nayga RM Jr, Thomsen MR, Danforth D, & Smartt J (2014). The effect of fast-food restaurants on childhood obesity: a school level analysis . Economics & Human Biology , 12 , 110–119. [ PubMed ] [ Google Scholar ]
- Anderson ML, & Matsa DA (2011). Are restaurants really supersizing America? American Economic Journal : Applied Economics , 3 ( 1 ), 152–88. [ Google Scholar ]
- Athens JK, Duncan DT, & Elbel B (2016). Proximity to fast-food outlets and supermarkets as predictors of fast-food dining frequency . Journal of the Academy of Nutrition and Dietetics , 116 ( 8 ), 1266–1275. [ PMC free article ] [ PubMed ] [ Google Scholar ]
- Bellettini G, & Kempf H (2013). Why not in your backyard? On the location and size of a public facility . Regional Science and Urban Economics , 43 ( 1 ), 22–30. [ Google Scholar ]
- Berger N, Kaufman TK, Bader MD, Rundle AG, Mooney SJ, Neckerman KM, & Lovasi GS (2019). Disparities in trajectories of changes in the unhealthy food environment in New York City: A latent class growth analysis, 1990–2010 . Social Science & Medicine , 234 , 112362. [ PMC free article ] [ PubMed ] [ Google Scholar ]
- Bitler M, & Haider SJ (2011). An economic view of food deserts in the United States . Journal of Policy Analysis and Management , 30 ( 1 ), 153–176. [ Google Scholar ]
- Block JP, Scribner RA, & DeSalvo KB (2004). Fast food, race/ethnicity, and income: a geographic analysis . American Journal of Preventive Medicine , 27 ( 3 ), 211–217. [ PubMed ] [ Google Scholar ]
- Bridger T (2009). Childhood obesity and cardiovascular disease . Pediatrics & Child Health , 14 ( 3 ), 177–182. [ PMC free article ] [ PubMed ] [ Google Scholar ]
- Chen D, Jaenicke EC, & Volpe RJ (2016). Food environments and obesity: household diet expenditure versus food deserts . American Journal of Public Pealth , 106 ( 5 ), 881–888. [ PMC free article ] [ PubMed ] [ Google Scholar ]
- Coley RL, Sullivan WC, & Kuo FE (1997). Where does community grow? The social context created by nature in urban public housing . Environment and Behavior , 29 ( 4 ), 468–494. [ Google Scholar ]
- Currie J, DellaVigna S, Moretti E, & Pathania V (2010). The effect of fast food restaurants on obesity and weight gain . American Economic Journal: Economic Policy , 2 ( 3 ), 32–63. [ Google Scholar ]
- Cutler DM, Glaeser EL, & Shapiro JM (2003). Why have Americans become more obese? Journal of Economic Perspectives , 17 ( 3 ), 93–118. [ Google Scholar ]
- Davis B, & Carpenter C (2009). Proximity of fast-food restaurants to schools and adolescent obesity . American Journal of Public Health , 99 ( 3 ), 505–510. [ PMC free article ] [ PubMed ] [ Google Scholar ]
- DeLuca S, & Rosenbaum JE (2003). If low-income blacks are given a chance to live in white neighborhoods, will they stay? Examining mobility patterns in a quasi-experimental program with administrative data . Housing Policy Debate , 14 ( 3 ), 305–345. [ Google Scholar ]
- Dubowitz T, Ghosh-Dastidar M, Eibner C, Slaughter ME, Fernandes M, Whitsel EA, Bird CE, Jewell A, Margolis KL, Li W, Michael YL, Shih RA, Manson JE, & Escarce JJ (2012). The Women’s Health Initiative: The food environment, neighborhood socioeconomic status, BMI, and blood pressure . Obesity , 20 ( 4 ), 862–871. [ PMC free article ] [ PubMed ] [ Google Scholar ]
- Dunn RA (2010). The effect of fast-food availability on obesity: an analysis by gender, race, and residential location . American Journal of Agricultural Economics , 92 ( 4 ), 1149–1164. [ Google Scholar ]
- Dunn RA, Sharkey JR, & Horel S (2012). The effect of fast-food availability on fast-food consumption and obesity among rural residents: an analysis by race/ethnicity . Economics & Human Biology , 10 ( 1 ), 1–13. [ PubMed ] [ Google Scholar ]
- Elbel B, Tamura K, McDermott ZT, Wu E, & Schwartz AE (2020). Childhood Obesity and the Food Environment: A Population-Based Sample of Public School Children in New York City . Obesity , 28 ( 1 ), 65–72. [ PMC free article ] [ PubMed ] [ Google Scholar ]
- Galvez MP, Morland K, Raines C, Kobil J, Siskind J, Godbold J, & Brenner B (2008). Race and food store availability in an inner-city neighbourhood . Public Health Nutrition , 11 ( 6 ), 624–631. [ PubMed ] [ Google Scholar ]
- Gibson DM (2011). The neighborhood food environment and adult weight status: estimates from longitudinal data . American journal ofpublic health , 101 ( 1 ), 71–78. [ PMC free article ] [ PubMed ] [ Google Scholar ]
- Goux D, & Maurin E (2007). Close neighbours matter: Neighbourhood effects on early performance at school . The Economic Journal , 117 ( 523 ), 1193–1215. [ Google Scholar ]
- Lewis LB, Sloane DC, Nascimento LM, Diamant AL, Guinyard JJ, Yancey AK, & Flynn G (2005). African Americans’ access to healthy food options in South Los Angeles restaurants . American Journal of Public Health , 95 ( 4 ), 668–673. [ PMC free article ] [ PubMed ] [ Google Scholar ]
- McCarthy PS (1999). Public policy and highway safety: a city-wide perspective . Regional Science and Urban Economics , 29 ( 2 ), 231–244. [ Google Scholar ]
- Mehta NK, & Chang VW (2008). Weight status and restaurant availability: a multilevel analysis . American Journal of Preventive Medicine , 34 ( 2 ), 127–133. [ PMC free article ] [ PubMed ] [ Google Scholar ]
- Mirtcheva DM, & Powell LM (2009). Participation in the national school lunch program: Importance of school-level and neighborhood contextual factors . Journal of School Health , 79 ( 10 ), 485–494. [ PubMed ] [ Google Scholar ]
- Morland KB, & Evenson KR (2009). Obesity prevalence and the local food environment . Health & Place , 15 ( 2 ), 491–495. [ PMC free article ] [ PubMed ] [ Google Scholar ]
- Morland K, Roux AVD, & Wing S (2006). Supermarkets, other food stores, and obesity: the atherosclerosis risk in communities study . American Journal of Preventive Medicine , 30 ( 4 ), 333–339. [ PubMed ] [ Google Scholar ]
- New York City Department of Education (2020). Transportation Eligibility . Retrieved from https://www.schools.nyc.gov/school-life/transportation/bus-eligibility .
- New York City Housing Authority (2019). NYCHA 2019 Fact Sheet . Retrieved from https://www1.nyc.gov/assets/nycha/downloads/pdf/NYCHA-Fact-Sheet_2019_08-01.pdf
- New York City Housing Authority (2020). Tenant Selection and Assignment Plan . Retrieved from https://www1.nyc.gov/assets/nycha/downloads/pdf/TSAPlan.pdf
- Ogden CL, Carroll MD, Fakhouri TH, Hales CM, Fryar CD, Li X, & Freedman DS (2018). Prevalence of obesity among youths by household income and education level of head of household-United States 2011–2014 . Morbidity and Mortality Weekly Report , 67 ( 6 ), 186. [ PMC free article ] [ PubMed ] [ Google Scholar ]
- Oreopoulos P (2003). The long-run consequences of living in a poor neighborhood . The Quarterly Journal of Economics , 118 ( 4 ), 1533–1575. [ Google Scholar ]
- Powell LM, Chaloupka FJ, & Bao Y (2007). The availability of fast-food and full-service restaurants in the United States: associations with neighborhood characteristics . American Journal of Preventive Medicine , 33 ( 4 ), S240–S245. [ PubMed ] [ Google Scholar ]
- Powell LM, Slater S, Mirtcheva D, Bao Y, & Chaloupka FJ (2007). Food store availability and neighborhood characteristics in the United States . Preventive Medicine , 44 ( 3 ), 189–195. [ PubMed ] [ Google Scholar ]
- Richardson Andrea S., Janne Boone-Heinonen, Popkin Barry M., and Penny Gordon-Larsen. “ Are neighbourhood food resources distributed inequitably by income and race in the USA? Epidemiological findings across the urban spectrum .” BMJopen 2 , no. 2 ( 2012 ): e000698. [ PMC free article ] [ PubMed ] [ Google Scholar ]
- Rosenbaum JE (1995). Changing the geography of opportunity by expanding residential choice: Lessons from the Gautreaux program . Housing Policy Debate , 6 ( 1 ), 231–269. [ Google Scholar ]
- Sahoo K, Sahoo B, Choudhury AK, Sofi NY, Kumar R, & Bhadoria AS (2015). Childhood obesity: causes and consequences . Journal of Family Medicine and Primary Care , 4 ( 2 ), 187. [ PMC free article ] [ PubMed ] [ Google Scholar ]
- U.S. Department of Housing and Urban Development. (2019). A picture of subsidized households, 2015–2019 [Data file] . Retrieved from https://www.huduser.gov/portal/datasets/assthsg.html .
- Weinhardt F (2014). Social housing, neighborhood quality and student performance . Journal of Urban Economics , 82 , 12–31. [ Google Scholar ]
Kids in the U.S. are eating more fast food, the CDC reports
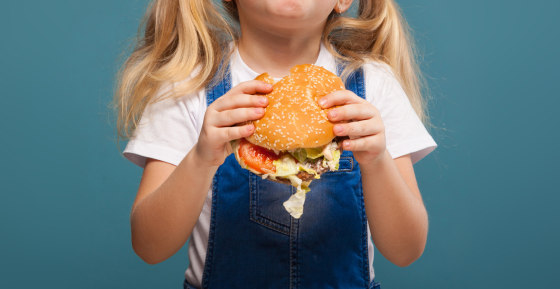
Despite warnings about obesity and unhealthy diets , American kids and adolescents are eating even more fast food. A new report from the Centers for Disease Control and Prevention found that children and adolescents got 13.8 percent of their daily calories from fast food in 2015 to 2018, up from 12.4 percent in 2011 to 2012.
The report, from the CDC’s National Center for Health Statistics, also found that Black and Hispanic kids eat more fast food than their white counterparts, and over one third ate fast food on any given day.
What’s more, the proportion of calories from fast food increased with age, with children ages 2 to 11 getting 11.5 percent of their daily calories from fast food, compared with ages 12 to 19, at 18 percent.
The report included data from the National Health and Nutrition Examination Survey, which is given to a sample of the U.S. population every two years to gather information on health and nutrition.
The rise in consumption among kids and young teens is not unexpected, experts say.
“These are not surprising findings when you think about how strapped families are these days for both time and money, and how fast food can be an easy option for stressed families,” said Dr. Eliana Perrin, professor of pediatrics at Duke University School of Medicine.
The report only included data through 2018, and therefore didn’t touch on how kids and families are eating during the pandemic, but Perrin speculated that it had most likely exacerbated the problem.
While some families are privileged to work and cook from home, “too many families are poorer than they were before, have more food insecurity and have more work to do with less support,” she said. “So fast food once again becomes, I think, a too-easy default for their stressed lives.” And it’s likely that these changes affected people of color more.
Eating too much fast food is bad for childrens’ health because of the high calorie count coupled with too few nutrients, Perrin said. A poor diet can also lead to heart problems and obesity .
Obesity affects an estimated 18.4 percent of U.S. children ages 6 to 11 and 20.9 percent of adolescents ages 12 to 19, according to the CDC . Obesity rates are higher among Black and Hispanic children.
Another factor that may have played a role in the increase in fast-food consumption is advertising, said Frances Fleming-Milici, director of marketing initiatives at the UConn Rudd Center for Food Policy and Obesity. A report from her institution compared the average number of TV advertisements viewed from 2007 to 2016. “We saw a big increase in ad exposure for preschoolers and children for particular fast-food restaurants,” she said.
And many of the ads were targeted at Black and Hispanic children.
“Compared to white youth, Black youth view twice as many ads for fast food and other restaurants,” Fleming-Milici said. On Spanish-language television, “about half of all the food ads that they view are fast-food restaurants,” she added.
Perrin said that targeted marketing contributes to health disparities.
“I think this is a major health equity issue,” she said.
Follow NBC HEALTH on Twitter & Facebook .
Obesity and Fast Food Exploratory Essay
- To find inspiration for your paper and overcome writer’s block
- As a source of information (ensure proper referencing)
- As a template for you assignment
What is so intriguing about fast food?
Studies show that American men and women have realized significant gains in body weight over the years. In fact, a larger proportion of the gains in the body mass arise from the escalating promotional activities carried out by the fast-food producing companies such as McDonalds.
In addition, the companies enjoy massive sales volume of processed and packed food. In this regard, many people continue to consume such foods thereby experiencing significant weight gains.
Based on these factors, several food advocates in the American markets such as Hamburger Chef Jamie Oliver have continuously toiled to make the general society aware of the processes that are utilized by different industries to process fast foods.
Further, the activist exposes the dangerous effects that are attributed to the increased consumption of such foods. Jamie Oliver, through documentaries, television shows as well as interviews has made major strides in explaining to the public the processes that the McDonalds Company’s products undergo before they are sold for consumption (Oliver, 2013).
Jamie’s exposure on the processes used by McDonalds in the manufacture of hamburger has compelled the company to change its production procedures (Peters, 2013).
Further, companies such as Burger King and Taco Bell have ditched the utilization of ammonium hydroxide components in their procedures. Due to the changes in the manufacturing processes by the companies, cases of obesity have been on the decrease across several states in the US (Peters, 2013).
Most importantly, the fast food companies have flooded larger proportions of the American states. As such, the firms are taking advantage of the less cautious territories overseas. For instance, McDonalds Company operates in the UK as well as Ireland where the firm utilizes the meat from the local suppliers at lower price rates.
In order to realize declining levels of overweight, individuals should circumvent the sustenance of companies that exploit populace for profit at the expense of individuals’ health implications. In addition, the families should also divert their eating habits to plant-based edibles. As such, the populace will be reducing the greenhouse gas discharges emanating from the livestock units.
Oliver, J 2013, Unfit for human consumption. Web.
Peters, S 2013, Chef Jamie Oliver Proves McDonald’s Burgers “Unfit for human consumption”. Web.
- Eating Disorders in Adolescent Girls
- Childhood Obesity Causes and Outcomes
- Plant-Based Meat: Controversies
- "Bullied: The Jamie Nabozny Story" Documentary
- Jamie Dodge Company’s Entry into Japanese Market
- Childhood Obesity and Nutrition
- Let's Go Kids Camp
- Influence of Media on Anorexia
- It Is High Time to Become Responsible
- How Can Societal Marketing Concept Be Used to Influence Children to Eat a Healthier Diet?
- Chicago (A-D)
- Chicago (N-B)
IvyPanda. (2019, July 4). Obesity and Fast Food. https://ivypanda.com/essays/obesity-and-fast-food/
"Obesity and Fast Food." IvyPanda , 4 July 2019, ivypanda.com/essays/obesity-and-fast-food/.
IvyPanda . (2019) 'Obesity and Fast Food'. 4 July.
IvyPanda . 2019. "Obesity and Fast Food." July 4, 2019. https://ivypanda.com/essays/obesity-and-fast-food/.
1. IvyPanda . "Obesity and Fast Food." July 4, 2019. https://ivypanda.com/essays/obesity-and-fast-food/.
Bibliography
IvyPanda . "Obesity and Fast Food." July 4, 2019. https://ivypanda.com/essays/obesity-and-fast-food/.
Best Fast Food in Arkhangelsk Oblast, Northwestern District
Fast food restaurants in arkhangelsk oblast, establishment type, traveller rating, restaurant features.

Sign Up or Log In
Share on hiking project.

Taking other people's content (text, photos, etc) without permission is a copyright violation and NOT OKAY!
Flag Inappropriate Post
Spam? Being a jerk / offensive? This is about an injury or accident Something else? Please explain.
If it's not super-obvious, tell us why:
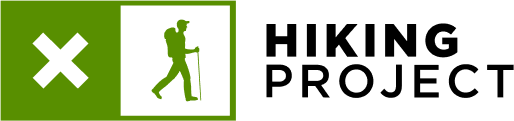
Arkhangelsk Oblast
Local tips or suggestions? Tell us!
Photos of Arkhangelsk Oblast - 1
Hiking Project is built by hikers like you. Add Your Photos

Arkhangelsk Oblast Hiking
- 0 Miles 0 Kilometers of Trail
- 0 Recommended Routes
Weather Averages
Days w/ |
J | F | M | A | M | J | J | A | S | O | N | D |
Join the Community! It's FREE
Already have an account? Login to close this notice.
- onX products are built by adventurers, for adventurers. We believe that every adventurer needs to know where to go, to know where they stand, and to be able to share their experiences.
- Top Contributors
- Share Your Adventures & Photos
- Mountain Project
- MTB Project
- Hiking Project
- Trail Run Project
- Powder Project
- National Parks
Please Confirm

1 Dodo Pizza

2 McDonald's
Navigate forward to interact with the calendar and select a date. Press the question mark key to get the keyboard shortcuts for changing dates.
Navigate backward to interact with the calendar and select a date. Press the question mark key to get the keyboard shortcuts for changing dates.
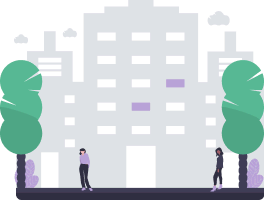
All related maps of Arkhangelsk Oblast
- Map of Arkhangelsk Oblast
- Map of Kholmogorsky District
- Map of Bereznik
- Map of Kotlas
- Map of Kholmogory
- Map of Kargopol
- Map of Krasnaya Gorka
- Map of Verkola
- Map of Novodvinsk
- Map of Nizhnezolotilovo
- Map of Katunino
- Map of Pinega
- Map of Shenkursk
- Map of Solovetskiy
- Map of Severodvinsk
- Map of Sura
- Map of Nyandoma
- Map of Malye Karely
- Map of Arkhangelsk
- Map of Oktyabrsky
- Map of Kimzha
- Map of Lampozhnya
- Map of Mitinskaya
- Map of Velsk
- Map of Krasnoborsk
- Map of Mezen
- Map of Gorodishchenskaya
- Map of Mirny
- Map of Konosha
- Map of Verkhovazhye
- Map of Tarnogsky Gorodok
Arkhangelsk Oblast throughout the year
- Arkhangelsk Oblast in January
- Arkhangelsk Oblast in February
- Arkhangelsk Oblast in March
- Arkhangelsk Oblast in April
- Arkhangelsk Oblast in May
- Arkhangelsk Oblast in June
- Arkhangelsk Oblast in July
- Arkhangelsk Oblast in August
- Arkhangelsk Oblast in September
- Arkhangelsk Oblast in October
- Arkhangelsk Oblast in November
- Arkhangelsk Oblast in December
Best fast food in nearby cities
- The best fast food restaurants in Nizhny Novgorod
- The best fast food restaurants in Yaroslavl
- The best fast food restaurants in Tver
- The best fast food restaurants in Ivanovo
- The best fast food restaurants in Cheboksary
- The best fast food restaurants in Yoshkar-Ola
- The best fast food restaurants in Mytishchi
- The best fast food restaurants in Khimki
Best attractions in nearby cities
- Top things to do and attractions in Kazan
- Top things to do and attractions in Nizhny Novgorod
- Top things to do and attractions in Yaroslavl
- Top things to do and attractions in Tver
- Top things to do and attractions in Arkhangelsk
- Top things to do and attractions in Kostroma
- Top things to do and attractions in Kirov
- Top things to do and attractions in Vologda
- Top things to do and attractions in Ivanovo
- Top things to do and attractions in Cheboksary
- Top things to do and attractions in Suzdal
- Top things to do and attractions in Sergiyev Posad
- Top things to do and attractions in Torzhok
- Top things to do and attractions in Rybinsk
- Top things to do and attractions in Khimki
- Top things to do and attractions in Rostov
- Top things to do and attractions in Ples
Best restaurants in nearby cities
- Where to eat: the best restaurants in Kazan
- Where to eat: the best restaurants in Nizhny Novgorod
- Where to eat: the best restaurants in Yaroslavl
- Where to eat: the best restaurants in Tver
- Where to eat: the best restaurants in Kostroma
- Where to eat: the best restaurants in Ivanovo
- Where to eat: the best restaurants in Cheboksary
- Where to eat: the best restaurants in Yoshkar-Ola
- Where to eat: the best restaurants in Suzdal
- Where to eat: the best restaurants in Mytishchi
- Where to eat: the best restaurants in Dmitrov
- Where to eat: the best restaurants in Sergiyev Posad
- Where to eat: the best restaurants in Torzhok
- Where to eat: the best restaurants in Rybinsk
- Where to eat: the best restaurants in Cherepovets
- Where to eat: the best restaurants in Khimki
- Where to eat: the best restaurants in Dubna
- Where to eat: the best restaurants in Rostov
- Where to eat: the best restaurants in Istra
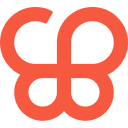
- Itinerary + map in one view
- Live collaboration
- Auto-import hotels and reservations
- Optimize your route
- Offline access on mobile
- See time and distance between all your places

IMAGES
VIDEO
COMMENTS
Fast food consumption and overweight/obesity prevalence ...
2015), as have inequalities in childhood obesity (Bann et al., 2018). 1In 2018, the BBC Shared Data Unitreporteda 34% increase in fast food outlets from 2010 to 2018 in the UK, and an increase in the average number of fast food outlets per 100,000 people from 47 in 2010 to 61 by 2018 (BBC, 2018) Figures from Public Health England (PHE) reveal
The prevalence of obesity among US children increased significantly during the past 3 decades, with ∼1 in 3 overweight or obese by 2009-2010 ().Concurrent with these trends, children's fast food intake has increased markedly since the 1970s ().The percentage of children's total energy intake consumed from fast food restaurants increased from 2% in 1977-1978 to 13% in 2003-2006 (6, 7).
2.1. Study selection criteria. Studies that met all of the following criteria were included in the review: (a) study subjects (children and adolescents aged younger than 18); (b) study outcomes (weight‐related behaviours [eg, diet, physical activity, and sedentary behaviour] and/or outcomes [eg, overweight and obesity measured by body mass index (BMI, kg/m 2), waist circumference, waist‐to ...
The above studies examine adult populations. Two studies analyze fast food restaurants around schools and find fast food restaurants to significantly affect childhood obesity rates. Currie et al. (2010) uses a cross-sectional sample of students from grade 9 in public schools in California. In their study, the effect is identified by comparing ...
Do Fast Food Restaurants Contribute to Obesity?
Following the searches to reduce the positive relationship between fast food and obesity, traditional fast foods and consumption should be increased. Discover the world's research 25+ million members
The prevalence of childhood obesity continues to rise despite decades of clinical and public health efforts. Early identification of children at risk of developing obesity is essential using newer electronic health systems, which move beyond traditional growth charts to provide a wealth of information about body mass index and other relevant parameters such as social determinants of health and ...
Numerous studies link poor dietary choices, such as those prevalent in fast food, to cognitive and behavioral issues in children. The excessive consumption of high-sugar, high-fat meals has been associated with attention deficits and hyperactivity, affecting a child's ability to focus and learn (Smith et al., 2015).
How Fast Food Affects Children's Health
In recent times, there is a growing tendency in advertising and marketing of unhealthy food to children, which is considered to be one of the causes of obesity increased rates. Fast food restaurant owners do not deny this fact, but they do not accept themselves as culprits, admitting that this food consumption is admissible in moderate portions ...
For example, the prevalence of fast food advertising and the rise of screen time have made it easier for children to consume unhealthy foods and lead sedentary lifestyles. These various factors interact in a multifaceted way, making it essential to address child obesity from a holistic perspective. ... Child Obesity Essay Outline. (2024, March ...
134 Childhood Obesity Essay Topics & Examples. Updated: Mar 2nd, 2024. 15 min. If you're writing an academic paper or speech on kids' nutrition or weight loss, you will benefit greatly from our childhood obesity essay examples. Besides, our experts have prepared a list of original topics for your work.
1. Introduction. Does proximity to fast food outlets and "unhealthy" food increase obesity among low-income children? In the United States, nearly one fifth of low-income children are obese, facing elevated risks of adult obesity, hypertension, and cardiovascular disease, among other serious complications that may lead to premature death (Bridger, 2009; Ogden et al., 2018; Sahoo et al., 2015).
Kumanyika (2008) identifies that these factors alter physiological systems within the children's bodies, causing overweight, obesity, and associated health conditions and diseases. Fast foods are associated with the increased risk of acquiring metabolic syndrome, type II diabetes (Kumanyika, 2008). However, the study shows that not all persons ...
This prevalence has tripled since the 1970s. Childhood obesity is associated with several negative health consequences, including diabetes, heart disease, and high blood pressure, as well as emotional and psychological impacts, such as low self-esteem and bullying. This essay will explore the causes of child obesity, its effects, and possible ...
Obesity affects an estimated 18.4 percent of U.S. children ages 6 to 11 and 20.9 percent of adolescents ages 12 to 19, according to the CDC. Obesity rates are higher among Black and Hispanic children.
Due to the changes in the manufacturing processes by the companies, cases of obesity have been on the decrease across several states in the US (Peters, 2013). Most importantly, the fast food companies have flooded larger proportions of the American states. As such, the firms are taking advantage of the less cautious territories overseas.
Search for more papers by this author. Miyang Luo, Miyang Luo. Department of Reproductive Immunology, Affiliated Shenzhen Maternity & Child Healthcare Hospital, Southern Medical University, Shenzhen, China ... physical activity, and family factors. 17 Therefore, the association between fast-food consumption and childhood obesity is less easier ...
Arkhangelsk Oblast
Best Fast Food in Arkhangelsk Oblast: See Tripadvisor traveller reviews of Fast Food Restaurants in Arkhangelsk Oblast.
Next Generation Hiking Trail Maps
We've collected the most-often-mentioned 10 places from other articles, including favorites like Dodo Pizza, McDonald's, and Subway