- Skip to main content
- Skip to primary sidebar
- Skip to footer
- QuestionPro

- Solutions Industries Gaming Automotive Sports and events Education Government Travel & Hospitality Financial Services Healthcare Cannabis Technology Use Case AskWhy Communities Audience Contactless surveys Mobile LivePolls Member Experience GDPR Positive People Science 360 Feedback Surveys
- Resources Blog eBooks Survey Templates Case Studies Training Help center

Home Market Research

Experimental vs Observational Studies: Differences & Examples
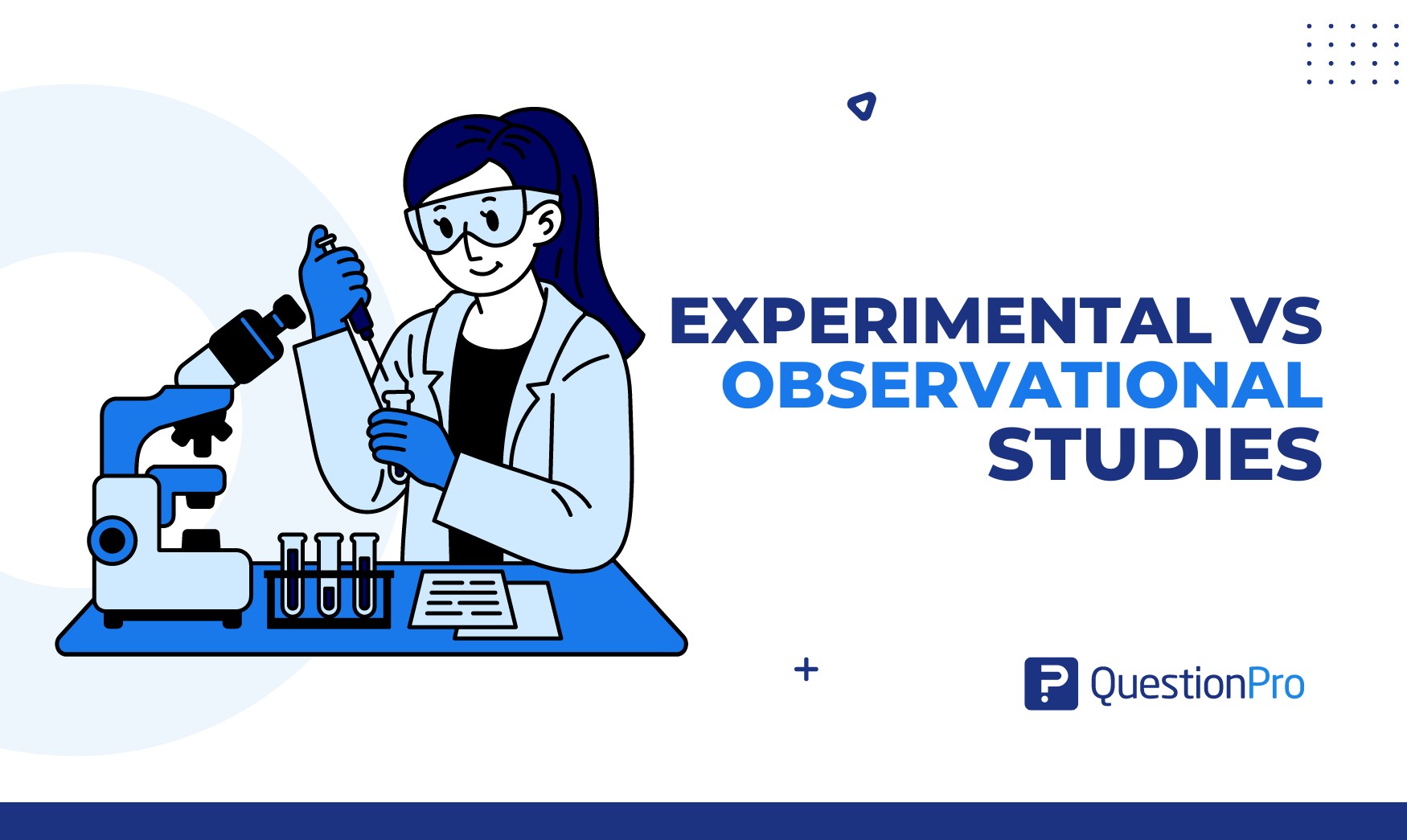
Understanding the differences between experimental vs observational studies is crucial for interpreting findings and drawing valid conclusions. Both methodologies are used extensively in various fields, including medicine, social sciences, and environmental studies.
Researchers often use observational and experimental studies to gather comprehensive data and draw robust conclusions about their investigating phenomena.
This blog post will explore what makes these two types of studies unique, their fundamental differences, and examples to illustrate their applications.
What is an Experimental Study?
An experimental study is a research design in which the investigator actively manipulates one or more variables to observe their effect on another variable. This type of study often takes place in a controlled environment, which allows researchers to establish cause-and-effect relationships.
Key Characteristics of Experimental Studies:
- Manipulation: Researchers manipulate the independent variable(s).
- Control: Other variables are kept constant to isolate the effect of the independent variable.
- Randomization: Subjects are randomly assigned to different groups to minimize bias.
- Replication: The study can be replicated to verify results.
Types of Experimental Study
- Laboratory Experiments: Conducted in a controlled environment where variables can be precisely controlled.
- Field Research : These are conducted in a natural setting but still involve manipulation and control of variables.
- Clinical Trials: Used in medical research and the healthcare industry to test the efficacy of new treatments or drugs.
Example of an Experimental Study:
Imagine a study to test the effectiveness of a new drug for reducing blood pressure. Researchers would:
- Randomly assign participants to two groups: receiving the drug and receiving a placebo.
- Ensure that participants do not know their group (double-blind procedure).
- Measure blood pressure before and after the intervention.
- Compare the changes in blood pressure between the two groups to determine the drug’s effectiveness.
What is an Observational Study?
An observational study is a research design in which the investigator observes subjects and measures variables without intervening or manipulating the study environment. This type of study is often used when manipulating impractical or unethical variables.
Key Characteristics of Observational Studies:
- No Manipulation: Researchers do not manipulate the independent variable.
- Natural Setting: Observations are made in a natural environment.
- Causation Limitations: It is difficult to establish cause-and-effect relationships due to the need for more control over variables.
- Descriptive: Often used to describe characteristics or outcomes.
Types of Observational Studies:
- Cohort Studies : Follow a control group of people over time to observe the development of outcomes.
- Case-Control Studies: Compare individuals with a specific outcome (cases) to those without (controls) to identify factors that might contribute to the outcome.
- Cross-Sectional Studies : Collect data from a population at a single point to analyze the prevalence of an outcome or characteristic.
Example of an Observational Study:
Consider a study examining the relationship between smoking and lung cancer. Researchers would:
- Identify a cohort of smokers and non-smokers.
- Follow both groups over time to record incidences of lung cancer.
- Analyze the data to observe any differences in cancer rates between smokers and non-smokers.
Difference Between Experimental vs Observational Studies
Choosing between experimental and observational studies.
The researchers relied on statistical analysis to interpret the results of randomized controlled trials, building upon the foundations established by prior research.
Use Experimental Studies When:
- Causality is Important: If determining a cause-and-effect relationship is crucial, experimental studies are the way to go.
- Variables Can Be Controlled: When you can manipulate and control the variables in a lab or controlled setting, experimental studies are suitable.
- Randomization is Possible: When random assignment of subjects is feasible and ethical, experimental designs are appropriate.
Use Observational Studies When:
- Ethical Concerns Exist: If manipulating variables is unethical, such as exposing individuals to harmful substances, observational studies are necessary.
- Practical Constraints Apply: When experimental studies are impractical due to cost or logistics, observational studies can be a viable alternative.
- Natural Settings Are Required: If studying phenomena in their natural environment is essential, observational studies are the right choice.
Strengths and Limitations
Experimental studies.
- Establish Causality: Experimental studies can establish causal relationships between variables by controlling and using randomization.
- Control Over Confounding Variables: The controlled environment allows researchers to minimize the influence of external variables that might skew results.
- Repeatability: Experiments can often be repeated to verify results and ensure consistency.
Limitations:
- Ethical Concerns: Manipulating variables may be unethical in certain situations, such as exposing individuals to harmful conditions.
- Artificial Environment: The controlled setting may not reflect real-world conditions, potentially affecting the generalizability of results.
- Cost and Complexity: Experimental studies can be costly and logistically complex, especially with large sample sizes.
Observational Studies
- Real-World Insights: Observational studies provide valuable insights into how variables interact in natural settings.
- Ethical and Practical: These studies avoid ethical concerns associated with manipulation and can be more practical regarding cost and time.
- Diverse Applications: Observational studies can be used in various fields and situations where experiments are not feasible.
- Lack of Causality: It’s easier to establish causation with manipulation, and results are limited to identifying correlations.
- Potential for Confounding: Uncontrolled external variables may influence the results, leading to biased conclusions.
- Observer Bias: Researchers may unintentionally influence outcomes through their expectations or interpretations of data.
Examples in Various Fields
- Experimental Study: Clinical trials testing the effectiveness of a new drug against a placebo to determine its impact on patient recovery.
- Observational Study: Studying the dietary habits of different populations to identify potential links between nutrition and disease prevalence.
- Experimental Study: Conducting a lab experiment to test the effect of sleep deprivation on cognitive performance by controlling sleep hours and measuring test scores.
- Observational Study: Observing social interactions in a public setting to explore natural communication patterns without intervention.
Environmental Science
- Experimental Study: Testing the impact of a specific pollutant on plant growth in a controlled greenhouse setting.
- Observational Study: Monitoring wildlife populations in a natural habitat to assess the effects of climate change on species distribution.
How QuestionPro Research Can Help in Experimental vs Observational Studies
Choosing between experimental and observational studies is a critical decision that can significantly impact the outcomes and interpretations of a study. QuestionPro Research offers powerful tools and features that can enhance both types of studies, giving researchers the flexibility and capability to gather, analyze, and interpret data effectively.
Enhancing Experimental Studies with QuestionPro
Experimental studies require a high degree of control over variables, randomization, and, often, repeated trials to establish causal relationships. QuestionPro excels in facilitating these requirements through several key features:
- Survey Design and Distribution: With QuestionPro, researchers can design intricate surveys tailored to their experimental needs. The platform supports random assignment of participants to different groups, ensuring unbiased distribution and enhancing the study’s validity.
- Data Collection and Management: Real-time data collection and management tools allow researchers to monitor responses as they come in. This is crucial for experimental studies where data collection timing and sequence can impact the results.
- Advanced Analytics: QuestionPro offers robust analytical tools that can handle complex data sets, enabling researchers to conduct in-depth statistical analyses to determine the effects of the experimental interventions.
Supporting Observational Studies with QuestionPro
Observational studies involve gathering data without manipulating variables, focusing on natural settings and real-world scenarios. QuestionPro’s capabilities are well-suited for these studies as well:
- Customizable Surveys: Researchers can create detailed surveys to capture a wide range of observational data. QuestionPro’s customizable templates and question types allow for flexibility in capturing nuanced information.
- Mobile Data Collection: For field research, QuestionPro’s mobile app enables data collection on the go, making it easier to conduct studies in diverse settings without internet connectivity.
- Longitudinal Data Tracking: Observational studies often require data collection over extended periods. QuestionPro’s platform supports longitudinal studies, allowing researchers to track changes and trends.
Experimental and observational studies are essential tools in the researcher’s toolkit. Each serves a unique purpose and offers distinct advantages and limitations. By understanding their differences, researchers can choose the most appropriate study design for their specific objectives, ensuring their findings are valid and applicable to real-world situations.
Whether establishing causality through experimental studies or exploring correlations with observational research designs, the insights gained from these methodologies continue to shape our understanding of the world around us.
Whether conducting experimental or observational studies, QuestionPro Research provides a comprehensive suite of tools that enhance research efficiency, accuracy, and depth. By leveraging its advanced features, researchers can ensure that their studies are well-designed, their data is robustly analyzed, and their conclusions are reliable and impactful.
MORE LIKE THIS
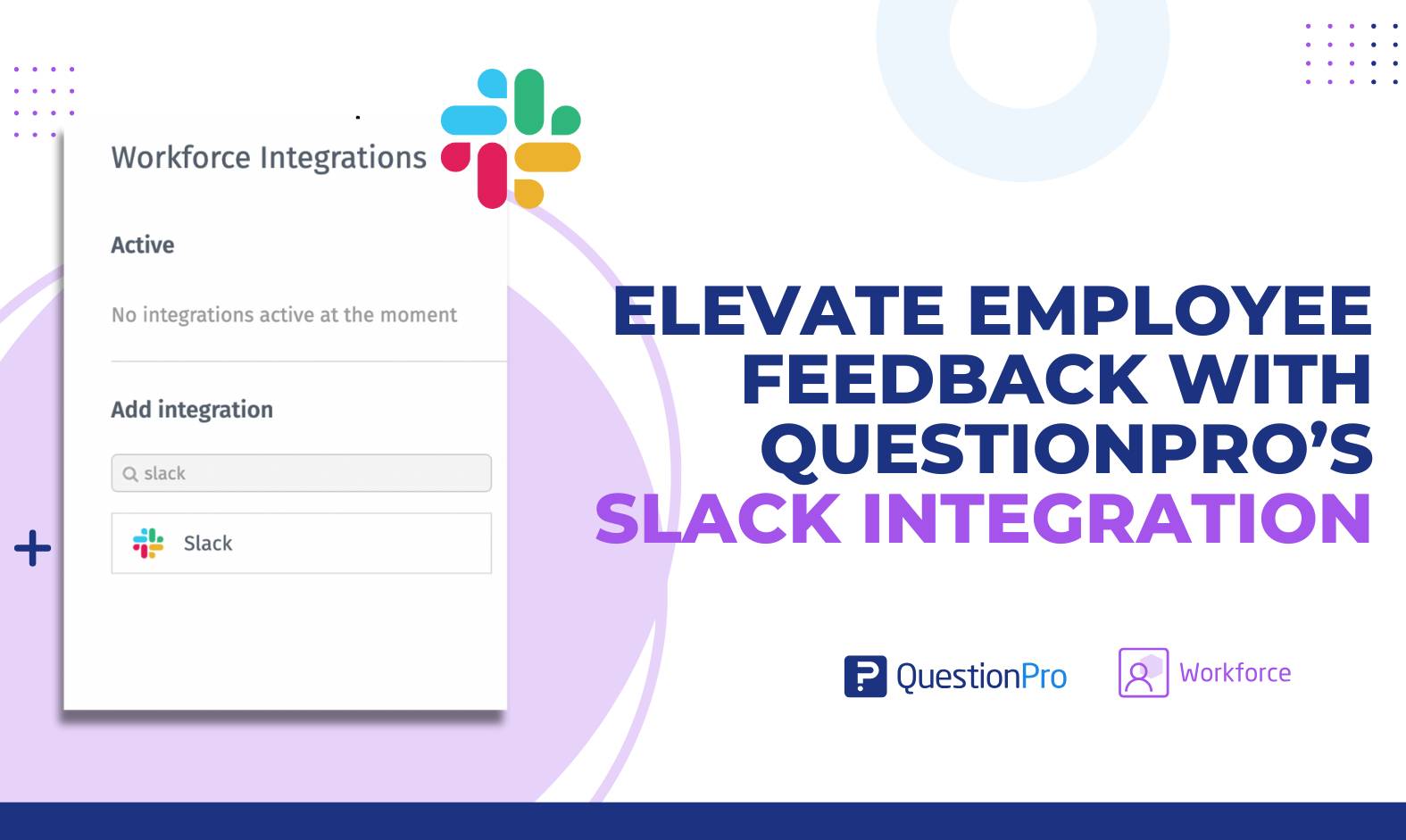
Maximize Employee Feedback with QuestionPro Workforce’s Slack Integration
Nov 6, 2024
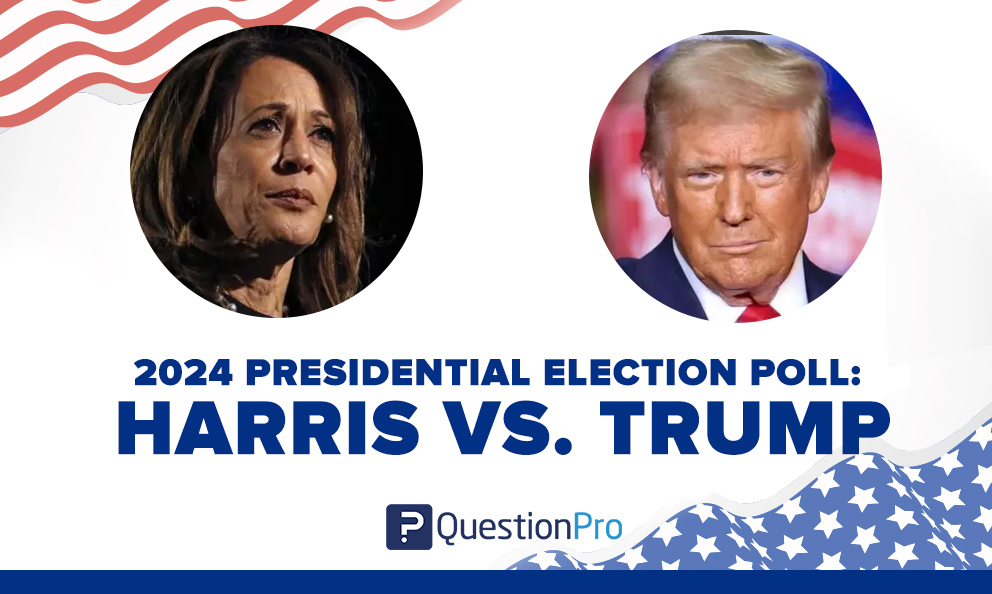
2024 Presidential Election Polls: Harris vs. Trump
Nov 5, 2024
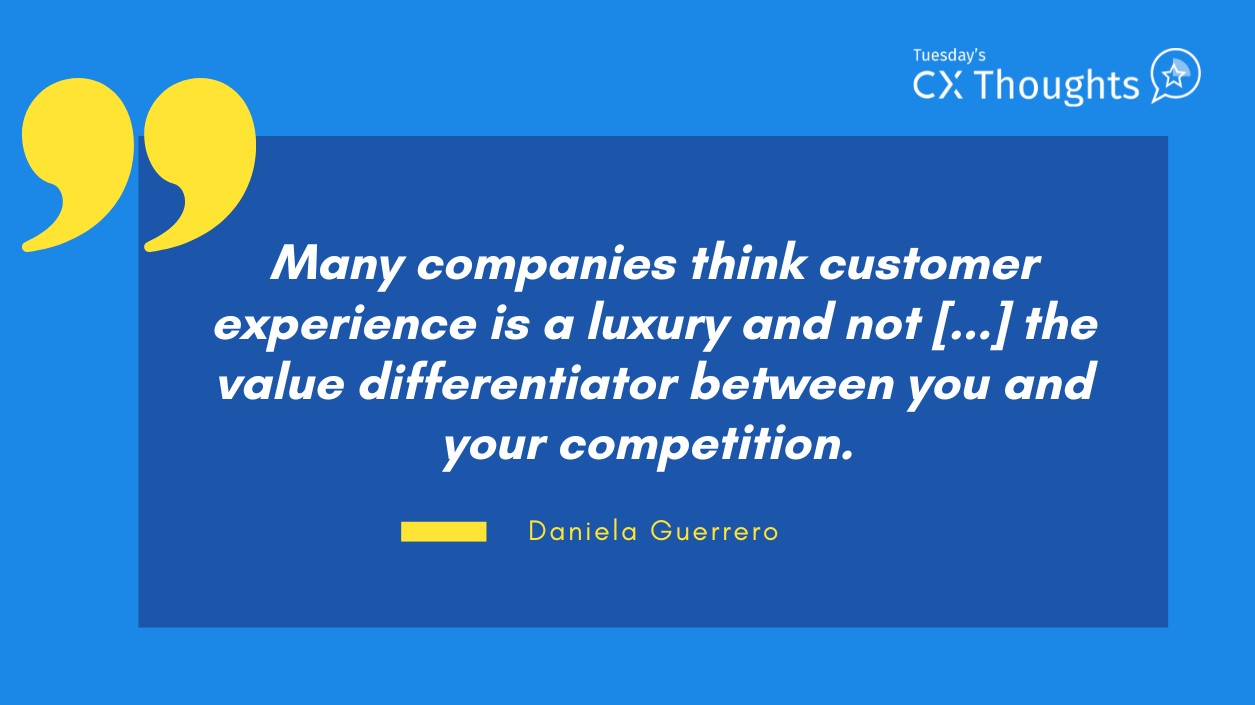
Your First Question Should Be Anything But, “Is The Car Okay?” — Tuesday CX Thoughts
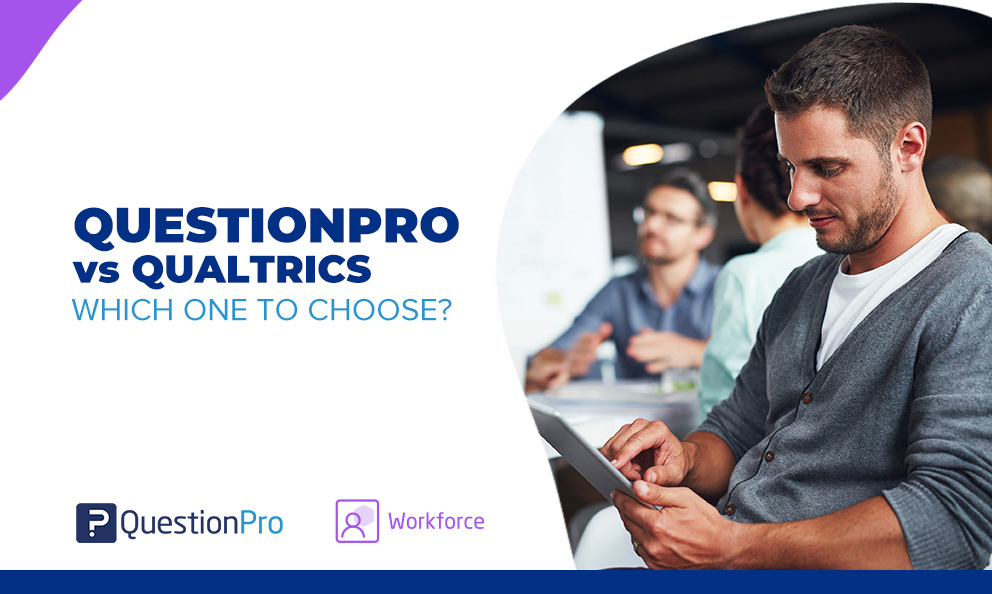
QuestionPro vs. Qualtrics: Who Offers the Best 360-Degree Feedback Platform for Your Needs?
Nov 4, 2024
Other categories
- Academic Research
- Artificial Intelligence
- Assessments
- Brand Awareness
- Case Studies
- Communities
- Consumer Insights
- Customer effort score
- Customer Engagement
- Customer Experience
- Customer Loyalty
- Customer Research
- Customer Satisfaction
- Employee Benefits
- Employee Engagement
- Employee Retention
- Friday Five
- General Data Protection Regulation
- Insights Hub
- Life@QuestionPro
- Market Research
- Mobile diaries
- Mobile Surveys
- New Features
- Online Communities
- Question Types
- Questionnaire
- QuestionPro Products
- Release Notes
- Research Tools and Apps
- Revenue at Risk
- Survey Templates
- Training Tips
- Tuesday CX Thoughts (TCXT)
- Uncategorized
- What’s Coming Up
- Workforce Intelligence
Observational vs. Experimental Study: A Comprehensive Guide
Explore the fundamental disparities between experimental and observational studies in this comprehensive guide by Santos Research Center, Corp. Uncover concepts such as control group, random sample, cohort studies, response variable, and explanatory variable that shape the foundation of these methodologies. Discover the significance of randomized controlled trials and case control studies, examining causal relationships and the role of dependent variables and independent variables in research designs.
This enlightening exploration also delves into the meticulous scientific study process, involving survey members, systematic reviews, and statistical analyses. Investigate the careful balance of control group and treatment group dynamics, highlighting how researchers meticulously assign variables and analyze statistical patterns to discern meaningful insights. From dissecting issues like lung cancer to understanding sleep patterns, this guide emphasizes the precision of controlled experiments and controlled trials, where variables are isolated and scrutinized, paving the way for a deeper comprehension of the world through empirical research.
Introduction to Observational and Experimental Studies
These two studies are the cornerstones of scientific inquiry, each offering a distinct approach to unraveling the mysteries of the natural world.
Observational studies allow us to observe, document, and gather data without direct intervention. They provide a means to explore real-world scenarios and trends, making them valuable when manipulating variables is not feasible or ethical. From surveys to meticulous observations, these studies shed light on existing conditions and relationships.
Experimental studies , in contrast, put researchers in the driver's seat. They involve the deliberate manipulation of variables to understand their impact on specific outcomes. By controlling the conditions, experimental studies establish causal relationships, answering questions of causality with precision. This approach is pivotal for hypothesis testing and informed decision-making.
At Santos Research Center, Corp., we recognize the importance of both observational and experimental studies. We employ these methodologies in our diverse research projects to ensure the highest quality of scientific investigation and to answer a wide range of research questions.
Observational Studies: A Closer Look
In our exploration of research methodologies, let's zoom in on observational research studies—an essential facet of scientific inquiry that we at Santos Research Center, Corp., expertly employ in our diverse research projects.
What is an Observational Study?
Observational research studies involve the passive observation of subjects without any intervention or manipulation by researchers. These studies are designed to scrutinize the relationships between variables and test subjects, uncover patterns, and draw conclusions grounded in real-world data.
Researchers refrain from interfering with the natural course of events in controlled experiment. Instead, they meticulously gather data by keenly observing and documenting information about the test subjects and their surroundings. This approach permits the examination of variables that cannot be ethically or feasibly manipulated, making it particularly valuable in certain research scenarios.
Types of Observational Studies
Now, let's delve into the various forms that observational studies can take, each with its distinct characteristics and applications.
Cohort Studies: A cohort study is a type of observational study that entails tracking one group of individuals over an extended period. Its primary goal is to identify potential causes or risk factors for specific outcomes or treatment group. Cohort studies provide valuable insights into the development of conditions or diseases and the factors that influence them.
Case-Control Studies: Case-control studies, on the other hand, involve the comparison of individuals with a particular condition or outcome to those without it (the control group). These studies aim to discern potential causal factors or associations that may have contributed to the development of the condition under investigation.
Cross-Sectional Studies: Cross-sectional studies take a snapshot of a diverse group of individuals at a single point in time. By collecting data from this snapshot, researchers gain insights into the prevalence of a specific condition or the relationships between variables at that precise moment. Cross-sectional studies are often used to assess the health status of the different groups within a population or explore the interplay between various factors.
Advantages and Limitations of Observational Studies
Observational studies, as we've explored, are a vital pillar of scientific research, offering unique insights into real-world phenomena. In this section, we will dissect the advantages and limitations that characterize these studies, shedding light on the intricacies that researchers grapple with when employing this methodology.
Advantages: One of the paramount advantages of observational studies lies in their utilization of real-world data. Unlike controlled experiments that operate in artificial settings, observational studies embrace the complexities of the natural world. This approach enables researchers to capture genuine behaviors, patterns, and occurrences as they unfold. As a result, the data collected reflects the intricacies of real-life scenarios, making it highly relevant and applicable to diverse settings and populations.
Moreover, in a randomized controlled trial, researchers looked to randomly assign participants to a group. Observational studies excel in their capacity to examine long-term trends. By observing one group of subjects over extended periods, research scientists gain the ability to track developments, trends, and shifts in behavior or outcomes. This longitudinal perspective is invaluable when studying phenomena that evolve gradually, such as chronic diseases, societal changes, or environmental shifts. It allows for the detection of subtle nuances that may be missed in shorter-term investigations.
Limitations: However, like any research methodology, observational studies are not without their limitations. One significant challenge of statistical study lies in the potential for biases. Since researchers do not intervene in the subjects' experiences, various biases can creep into the data collection process. These biases may arise from participant self-reporting, observer bias, or selection bias in random sample, among others. Careful design and rigorous data analysis are crucial for mitigating these biases.
Another limitation is the presence of confounding variables. In observational studies, it can be challenging to isolate the effect of a specific variable from the myriad of other factors at play. These confounding variables can obscure the true relationship between the variables of interest, making it difficult to establish causation definitively. Research scientists must employ statistical techniques to control for or adjust these confounding variables.
Additionally, observational studies face constraints in their ability to establish causation. While they can identify associations and correlations between variables, they cannot prove causality or causal relationship. Establishing causation typically requires controlled experiments where researchers can manipulate independent variables systematically. In observational studies, researchers can only infer potential causation based on the observed associations.
Experimental Studies: Delving Deeper
In the intricate landscape of scientific research, we now turn our gaze toward experimental studies—a dynamic and powerful method that Santos Research Center, Corp. skillfully employs in our pursuit of knowledge.
What is an Experimental Study?
While some studies observe and gather data passively, experimental studies take a more proactive approach. Here, researchers actively introduce an intervention or treatment to an experiment group study its effects on one or more variables. This methodology empowers researchers to manipulate independent variables deliberately and examine their direct impact on dependent variables.
Experimental research are distinguished by their exceptional ability to establish cause-and-effect relationships. This invaluable characteristic allows researchers to unlock the mysteries of how one variable influences another, offering profound insights into the scientific questions at hand. Within the controlled environment of an experimental study, researchers can systematically test hypotheses, shedding light on complex phenomena.
Key Features of Experimental Studies
Central to statistical analysis, the rigor and reliability of experimental studies are several key features that ensure the validity of their findings.
Randomized Controlled Trials: Randomization is a critical element in experimental studies, as it ensures that subjects are assigned to groups in a random assignment. This randomly assigned allocation minimizes the risk of unintentional biases and confounding variables, strengthening the credibility of the study's outcomes.
Control Groups: Control groups play a pivotal role in experimental studies by serving as a baseline for comparison. They enable researchers to assess the true impact of the intervention being studied. By comparing the outcomes of the intervention group to those of survey members of the control group, researchers can discern whether the intervention caused the observed changes.
Blinding: Both single-blind and double-blind techniques are employed in experimental studies to prevent biases from influencing the study or controlled trial's outcomes. Single-blind studies keep either the subjects or the researchers unaware of certain aspects of the study, while double-blind studies extend this blindness to both parties, enhancing the objectivity of the study.
These key features work in concert to uphold the integrity and trustworthiness of the results generated through experimental studies.
Advantages and Limitations of Experimental Studies
As with any research methodology, this one comes with its unique set of advantages and limitations.
Advantages: These studies offer the distinct advantage of establishing causal relationships between two or more variables together. The controlled environment allows researchers to exert authority over variables, ensuring that changes in the dependent variable can be attributed to the independent variable. This meticulous control results in high-quality, reliable data that can significantly contribute to scientific knowledge.
Limitations: However, experimental ones are not without their challenges. They may raise ethical concerns, particularly when the interventions involve potential risks to subjects. Additionally, their controlled nature can limit their real-world applicability, as the conditions in experiments may not accurately mirror those in the natural world. Moreover, executing an experimental study in randomized controlled, often demands substantial resources, with other variables including time, funding, and personnel.
Observational vs Experimental: A Side-by-Side Comparison
Having previously examined observational and experimental studies individually, we now embark on a side-by-side comparison to illuminate the key distinctions and commonalities between these foundational research approaches.
Key Differences and Notable Similarities
Methodologies
- Observational Studies : Characterized by passive observation, where researchers collect data without direct intervention, allowing the natural course of events to unfold.
- Experimental Studies : Involve active intervention, where researchers deliberately manipulate variables to discern their impact on specific outcomes, ensuring control over the experimental conditions.
- Observational Studies : Designed to identify patterns, correlations, and associations within existing data, shedding light on relationships within real-world settings.
- Experimental Studies : Geared toward establishing causality by determining the cause-and-effect relationships between variables, often in controlled laboratory environments.
- Observational Studies : Yield real-world data, reflecting the complexities and nuances of natural phenomena.
- Experimental Studies : Generate controlled data, allowing for precise analysis and the establishment of clear causal connections.
Observational studies excel at exploring associations and uncovering patterns within the intricacies of real-world settings, while experimental studies shine as the gold standard for discerning cause-and-effect relationships through meticulous control and manipulation in controlled environments. Understanding these differences and similarities empowers researchers to choose the most appropriate method for their specific research objectives.
When to Use Which: Practical Applications
The decision to employ either observational or experimental studies hinges on the research objectives at hand and the available resources. Observational studies prove invaluable when variable manipulation is impractical or ethically challenging, making them ideal for delving into long-term trends and uncovering intricate associations between certain variables (response variable or explanatory variable). On the other hand, experimental studies emerge as indispensable tools when the aim is to definitively establish causation and methodically control variables.
At Santos Research Center, Corp., our approach to both scientific study and methodology is characterized by meticulous consideration of the specific research goals. We recognize that the quality of outcomes hinges on selecting the most appropriate method of research study. Our unwavering commitment to employing both observational and experimental research studies further underscores our dedication to advancing scientific knowledge across diverse domains.
Conclusion: The Synergy of Experimental and Observational Studies in Research
In conclusion, both observational and experimental studies are integral to scientific research, offering complementary approaches with unique strengths and limitations. At Santos Research Center, Corp., we leverage these methodologies to contribute meaningfully to the scientific community.
Explore our projects and initiatives at Santos Research Center, Corp. by visiting our website or contacting us at (813) 249-9100, where our unwavering commitment to rigorous research practices and advancing scientific knowledge awaits.
Recent Posts
Join an Alzheimer's clinical trial at Santos Research Center. Discover treatment options, receive expert care, and help advance research. Apply for our paid trial today!
Discover the causes and health risks of obesity, plus explore new clinical trials at Santos Research Center offering innovative treatment options.
Learn about Bipolar I Disorder symptoms, treatments, and clinical trials at Santos Research Center. Explore new treatment options - join our trial today!
At Santos Research Center, a medical research facility dedicated to advancing TBI treatments, we emphasize the importance of tailored rehabilitation...
Learn about COVID-19 rebound after Paxlovid, its symptoms, causes, and management strategies. Join our study at Santos Research Center. Apply now!
Learn everything about Respiratory Syncytial Virus (RSV), from symptoms and diagnosis to treatment and prevention. Stay informed and protect your health with...
Discover key insights on Alzheimer's disease, including symptoms, stages, and care tips. Learn how to manage the condition and find out how you can...
Santos Research Center, Corp. is a research facility conducting paid clinical trials, in partnership with major pharmaceutical companies & CROs. We work with patients from across the Tampa Bay area.
Contact Details
Navigation menu.
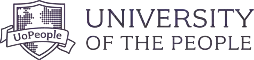
Home > Blog > Tips for Online Students > The Science of Observation: Comparing Observational Study vs Experiment
Tips for Online Students , Tips for Students
The Science of Observation: Comparing Observational Study vs Experiment

Updated: October 3, 2024
Published: October 1, 2024
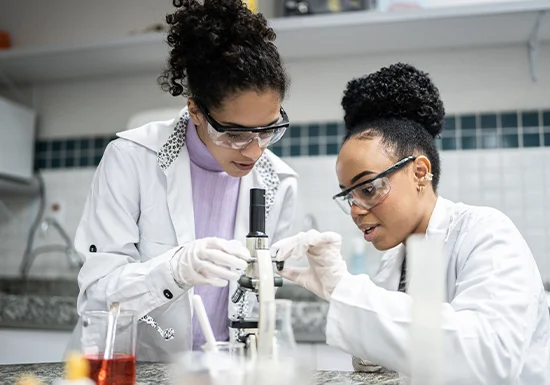
Observation is fundamental in scientific inquiry, helping researchers understand complex phenomena by collecting data and evidence. Observational studies and experiments are two primary research methods for gaining insights and drawing conclusions.
While observational studies involve monitoring subjects without interference, experiments involve manipulating variables to study effects. Each method has its strengths and limitations.
Scientists often use both observational studies and experiments to complement each other, ensuring a thorough understanding of the subject and validation of findings.
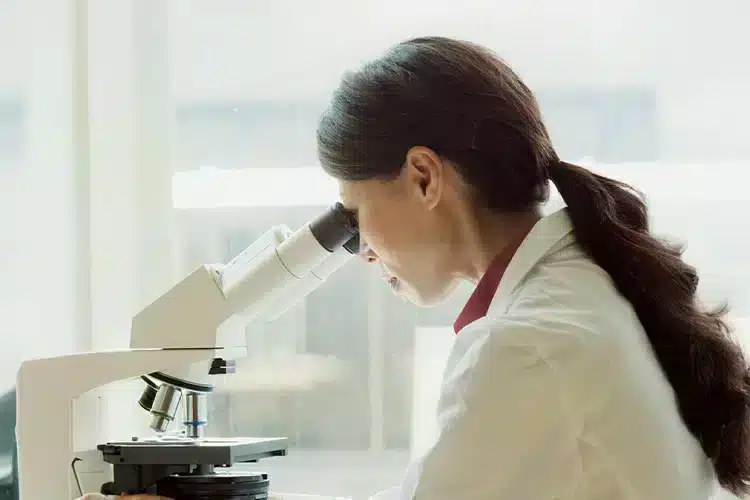
What is an Observational Study?
An observational study is a research method where researchers observe and collect data without manipulating the environment or influencing the subjects. This non-experimental approach allows for the natural occurrence of events and behaviors, providing valuable insights into real-world situations.
Observational studies are commonly used in fields like epidemiology, sociology, and ecology, where ethical or practical constraints make experimental manipulation difficult. Observational studies can reveal patterns and associations that might be missed in controlled settings by focusing on naturally occurring relationships.
What is an Experiment Study?
An experimental study involves deliberately manipulating one or more variables to observe their effects on a dependent variable under controlled conditions. Key components of experiments include the random assignment of participants, the presence of control and experimental groups, and the manipulation of independent variables. This method is designed to establish cause-and-effect relationships by minimizing confounding factors. Experimental studies are particularly valuable in fields like psychology , medicine , and natural sciences , where precise control over variables is crucial for testing hypotheses and understanding specific phenomena.
Observational Study vs Experiment: What are the Key Differences?
Observational studies and experiments differ fundamentally in their approach to data collection and control over variables:
Researcher Control Over Variables
In experiments, researchers have significant control over variables, allowing them to manipulate independent variables and observe the effects on dependent variables. In contrast, observational studies involve no direct manipulation, with researchers observing variables as they naturally occur.
Ability to Establish Causation
Experiments are well-suited to establish causation due to controlled conditions and random assignment, which help isolate the effects of specific variables. Observational studies, on the other hand, are limited in establishing causation because they do not involve direct manipulation of variables and are more prone to confounding factors.
Randomization of Subjects/Participants
Randomization is a key feature of experimental studies, ensuring participants are randomly assigned to different groups (e.g., control or experimental). This process helps eliminate bias and distribute potential confounding factors evenly. In contrast, observational studies typically do not involve randomization, as researchers observe subjects in their natural settings without influencing group assignments.
Manipulation of Independent Variable
In experiments, researchers actively manipulate one or more independent variables to study their effect on a dependent variable. This direct intervention allows for a controlled environment to test specific hypotheses. Observational studies do not involve such manipulation; instead, researchers observe existing conditions and variables without altering them.
Natural vs. Controlled Setting
Observational studies are conducted in natural settings, providing insights into real-world behaviors and interactions. However, experiments are often conducted in controlled environments like laboratories, allowing researchers to isolate specific variables and minimize external influences.
Potential for Confounding Factors
Observational studies are more susceptible to confounding factors because they lack the controlled environment of experiments. Confounding factors can influence both the independent and dependent variables, making it difficult to isolate the cause of observed effects. In experiments, researchers can control or account for these confounding factors, reducing their impact on the results.
Ethical Considerations and Limitations
Ethical concerns can limit the use of experiments, especially when manipulating variables could harm participants or when studying sensitive topics. Observational studies often provide an ethical alternative since they do not involve manipulation. However, they may still face ethical challenges related to privacy and consent, especially in covert observations or studies involving vulnerable populations.
Sample Size Requirements
The sample size needed can vary significantly between observational studies and experiments. Observational studies may require larger sample sizes to detect patterns and account for variability in natural settings. Experiments, with their controlled conditions, may achieve statistically significant results with smaller sample sizes, provided that randomization and proper controls are in place.
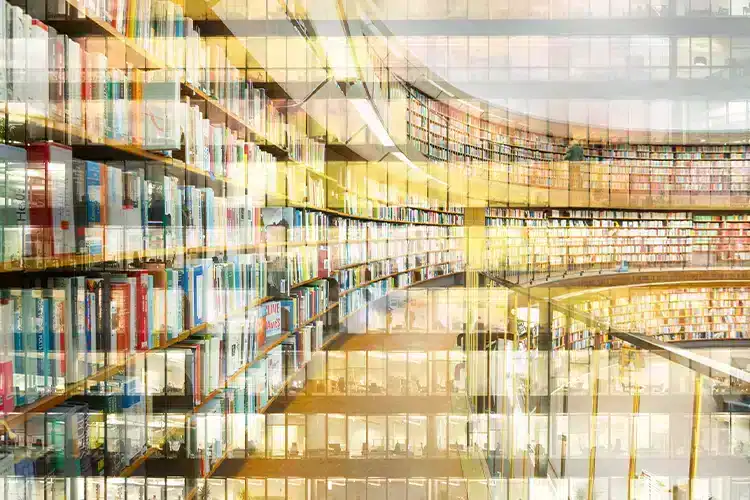
Can Observational Studies and Experiments be Combined in Research?
Combining observational studies and experiments, known as mixed-methods research , leverages the strengths of both approaches for a more comprehensive understanding of complex phenomena.
Researchers might use observational studies to identify patterns or generate hypotheses, then conduct experiments to test those hypotheses under controlled conditions. This integrated approach increases external validity, offers deeper insights, and allows researchers to address a broader range of research questions.
However, combining these methods can also present challenges in study design, data interpretation, and the need for consistent methodologies.
When is it More Appropriate to Conduct an Observational Study?
Observational studies are often more suitable when studying natural phenomena, long-term effects, or large populations where experimental control is impractical or impossible:
Ethical Concerns Prevent Experimentation
Observational studies offer a safer alternative in cases where manipulating variables would be unethical or cause harm, such as studying the impact of certain lifestyle choices on health.
Natural Phenomenon Under Study
Observational studies are particularly useful for investigating natural phenomena, such as animal behavior or environmental changes, where intervention could alter the outcomes being studied.
Rare Events Being Investigated
Observational studies are particularly advantageous for studying rare events that occur infrequently and cannot be easily replicated under controlled conditions, such as natural disasters or rare diseases.
Long-Term Effects Examined
Observational studies are more appropriate when research requires monitoring the long-term effects of specific exposures or behaviors. They allow for continuous data collection over extended periods, providing insights that experiments might not capture due to time and resource constraints.
Large Population-Based Research
Observational studies are well-suited for large-scale population research, where the diversity and variability of the sample make experimental control impractical. They enable the analysis of trends, risk factors, and associations across broad and diverse groups.
When is it More Appropriate to Conduct an Experiment Study?
Experimental studies are preferred when the research goal is to establish causation or test specific hypotheses under controlled conditions:
Causation Needs Establishing
Experiments are the gold standard for establishing clear cause-and-effect relationships between variables. By manipulating one variable and controlling others, researchers can confidently attribute changes in the dependent variable to the manipulated factor.
Variables Can be Controlled
When it’s possible to effectively control and manipulate variables, experiments provide the precision needed to isolate specific effects and reduce the influence of external factors, leading to more reliable results.
Random Assignment is Possible
Experiments allow for the random assignment of participants to different groups, such as control and experimental groups. This randomization minimizes bias and ensures that differences observed between groups are due to the manipulated variables, not pre-existing differences among participants.
Specific Hypotheses Testing
When researchers have specific, well-defined hypotheses they want to test, experimental studies provide a structured environment. Experiments can yield clear, interpretable results by controlling variables and setting up conditions to test these hypotheses.
Short-Term Effects Studied
Experiments are ideal for studying interventions or treatments’ immediate or short-term effects. They allow researchers to observe quick responses to manipulated variables, making them suitable for testing new medical treatments, educational interventions, or psychological responses.
How do Publication Standards Differ for Observational vs. Experimental Studies?
Due to their unique methodologies, publication standards differ significantly between observational and experimental studies. Observational studies emphasize the need for transparency in data collection, including detailing how researchers controlled for confounding factors.
Reporting guidelines like STROBE (Strengthening the Reporting of Observational Studies in Epidemiology) help ensure clarity and reproducibility. In contrast, experimental studies focus on randomization, control group assignment, and detailed descriptions of intervention methods.
Guidelines like CONSORT (Consolidated Standards of Reporting Trials) maintain high standards in experimental research reporting, ensuring results are reliable and can be replicated.
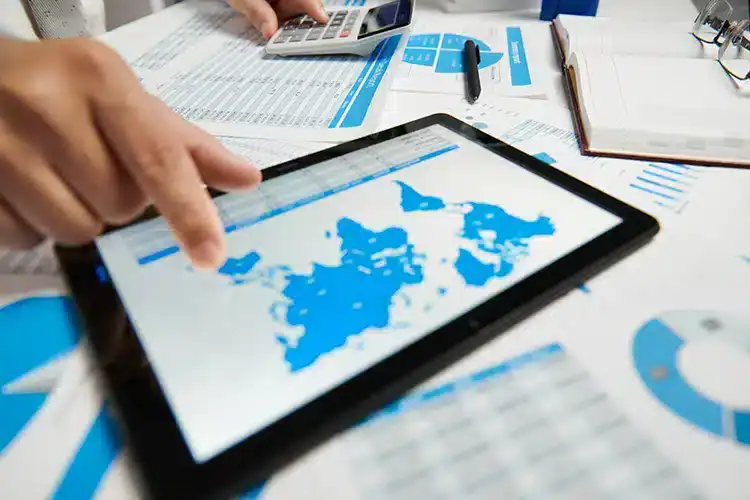
Choosing the Right Scientific Approach
When deciding between observational studies and experiments, researchers should consider the nature of their research question , ethical constraints, practicality, and the desired level of causal inference.
Observational studies are ideal for exploring natural phenomena, long-term effects, and scenarios where intervention is impractical or unethical. In contrast, experiments are best suited for testing specific hypotheses, establishing causation, and studying short-term effects under controlled conditions.
Careful evaluation of the study’s objectives, resources, and potential limitations is essential to selecting the most appropriate and effective research method.
How does an experiment differ from an observational study?
Experiments involve manipulating variables to establish causation, while observational studies involve monitoring variables without interference.
What is the role of a control group in experiments?
Control groups serve as a baseline to compare against the experimental group, helping to isolate the effects of the manipulated variable.
How do researchers control variables in experimental and observational settings?
Experiments control variables through random assignment and manipulation; observational studies control variables by careful selection and statistical adjustments.
What is the importance of randomization in experiments?
Randomization minimizes bias and ensures that the differences observed are due to the intervention, not pre-existing factors.
What statistical analyses are typically used for each method?
Observational studies often use regression analysis and correlation, while experiments may use t-tests, ANOVA, and other inferential statistics to analyze cause and effect.
How do time constraints affect the choice between observation and experimentation?
Experiments are typically suited for short-term studies due to the controlled environment, whereas observational studies can accommodate long-term data collection in natural settings.
In this article
At UoPeople, our blog writers are thinkers, researchers, and experts dedicated to curating articles relevant to our mission: making higher education accessible to everyone. Read More
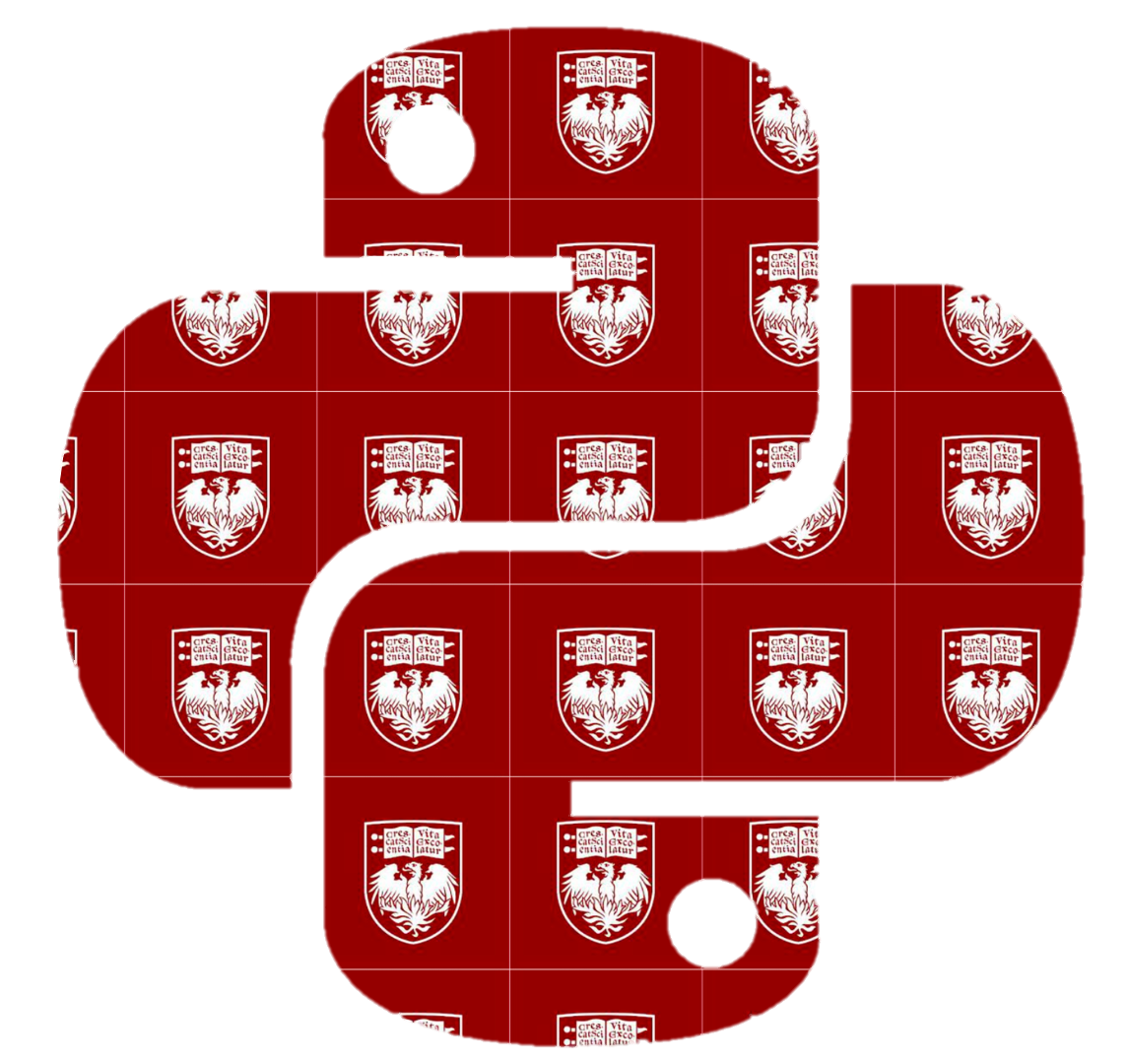
Introduction to Data Science I & II
Observational versus experimental studies, observational versus experimental studies #.
In most research questions or investigations, we are interested in finding an association that is causal (the first scenario in the previous section ). For example, “Is the COVID-19 vaccine effective?” is a causal question. The researcher is looking for an association between receiving the COVID-19 vaccine and contracting (symptomatic) COVID-19, but more specifically wants to show that the vaccine causes a reduction in COVID-19 infections (Baden et al., 2020) 1 .
Experimental Studies #
There are 3 necessary conditions for showing that a variable X (for example, vaccine) causes an outcome Y (such as not catching COVID-19):
Temporal Precedence : We must show that X (the cause) happened before Y (the effect).
Non-spuriousness : We must show that the effect Y was not seen by chance.
No alternate cause : We must show that no other variable accounts for the relationship between X and Y .
If any of the three is not present, the association cannot be causal. If the proposed cause did not happen before the effect, it cannot have caused the effect. In addition, if the effect was seen by chance and cannot be replicated, the association is spurious and therefore not causal. Lastly, if there is another phenomenon that accounts for the association seen, then it cannot be a causal association. These conditions are therefore, necessary to show causality.
The best way to show all three necessary conditions is by conducting an experiment . Experiments involve controllable factors which are measured and determined by the experimenter, uncontrollable factors which are measured but not determined by the experimentor, and experimental variability or noise which is unmeasured and uncontrolled. Controllable factors that the experimenter manipulates in his or her experiment are known as independent variables . In our vaccination example, the independent variable is receipt of vaccine. Uncontrollable factors that are hypothesized to depend on the independent variable are known as dependent variables. The dependent variable in the vaccination example is contraction of COVID-19. The experimentor cannot control whether participants catch the disease, but can measure it, and it is hypothesized that catching the disease is dependent on vaccination status.
Control Groups #
When conducting an experiment, it is important to have a comparison or control group . The control group is used to better understand the effect of the independent variable. For example, if all patients are given the vaccine, it would be impossible to measure whether the vaccine is effective as we would not know the outcome if patients had not received the vaccine. In order to measure the effect of the vaccine, the researcher must compare patients who did not receive the vaccine to patients that did receive the vaccine. This comparison group of patients who did not receive the vaccine is the control group for the experiment. The control group allows the researcher to view an effect or association. When scientists say that the COVID-19 vaccine is 94% effective, this does not mean that only 6% of people who got the vaccine in their study caught COVID-19 (the number is actually much lower!). That would not take into account the rate of catching COVID-19 for those without a vaccine. Rather, 94% effective refers to having 94% lower incidence of infection compared to the control group.
Let’s illustrate this using data from the efficacy trial by Baden and colleagues in 2020. In their primary analysis, 14,073 participants were in the placebo group and 14,134 in the vaccine group. Of these participants, a total of 196 were diagnosed with COVID-19 during the 78 day follow-up period: 11 in the vaccine group and 186 in the placebo group. This means, 0.08% of those in the vaccine group and 1.32% of those in the placebo group were diagnosed with COVID-19. Dividing 0.08 by 1.32, we see that the proportion of cases in the vaccine group was only 6% of the proportion of cases in the placebo group. Therefore, the vaccine is 94% effective.
Chicago has a population of almost 3,000,000. Extrapolating using the numbers from above, without the vaccine, 39,600 people would be expected to catch COVID-19 in the period between 14 and 92 days after their second vaccine. If everyone were vaccinated, the expected number would drop to 2,400. This is a large reduction! However, it is important that the researcher shows this effect is non-spurious and therefore important and significant. One way to do this is through replication : applying a treatment independently across two or more experimental subjects. In our example, researchers conducted many similar experiments for multiple groups of patients to show that the effect can be seen reliably.
Randomization #
A researcher must also be able to show there is no alternate cause for the association in order to prove causality. This can be done through randomization : random assignment of treatment to experimental subjects. Consider a group of patients where all male patients are given the treatment and all female patients are in the control group. If an association is found, it would be unclear whether this association is due to the treatment or the fact that the groups were of differing sex. By randomizing experimental subjects to groups, researchers ensure there is no systematic difference between groups other than the treatment and therefore no alternate cause for the relationship between treatment and outcome.
Another way of ensuring there is no alternate cause is by blocking : grouping similar experimental units together and assigning different treatments within such groups. Blocking is a way of dealing with sources of variability that are not of primary interest to the experimenter. For example, a researcher may block on sex by grouping males together and females together and assigning treatments and controls within the different groups. Best practices are to block the largest and most salient sources of variability and randomize what is difficult or impossible to block. In our example blocking would account for variability introduced by sex whereas randomization would account for factors of variability such as age or medical history which are more difficult to block.
Observational Studies #
Randomized experiments are considered the “Gold Standard” for showing a causal relationship. However, it is not always ethical or feasible to conduct a randomized experiment. Consider the following research question: Does living in Northern Chicago increase life expectancy? It would be infeasible to conduct an experiment which randomly allocates people to live in different parts of the city. Therefore, we must turn to observational data to test this question. Where experiments involve one or more variables controlled by the experimentor (dose of a drug for example), in observational studies there is no effort or intention to manipulate or control the object of study. Rather, researchers collect data without interfering with the subjects. For example, researchers may conduct a survey gathering both health and neighborhood data, or they may have access to administrative data from a local hospital. In these cases, the researchers are merely observing variables and outcomes.
There are two types of observational studies: retrospective studies and prospective studies. In a retrospective study , data is collected after events have taken place. This may be through surveys, historical data, or administrative records. An example of a retrospective study would be using administrative data from a hospital to study incidence of disease. In contrast, a prospective study identifies subjects beforehand and collects data as events unfold. For example, one might use a prospective study to evaluate how personality traits develop in children, by following a predetermined set of children through elementary school and giving them personality assessments each year.
Baden LR, El Sahly HM, Essink B, Kotloff K, Frey S, Novak R, Diemert D, Spector SA, Rouphael N, Creech CB, McGettigan J. Efficacy and safety of the mRNA-1273 SARS-CoV-2 vaccine. New England journal of medicine. 2020 Dec 30.
Experimental Studies and Observational Studies
- Reference work entry
- First Online: 01 January 2022
- pp 1748–1756
- Cite this reference work entry
- Martin Pinquart 3
945 Accesses
1 Citations
Experimental studies: Experiments, Randomized controlled trials (RCTs) ; Observational studies: Non-experimental studies, Non-manipulation studies, Naturalistic studies
Definitions
The experimental study is a powerful methodology for testing causal relations between one or more explanatory variables (i.e., independent variables) and one or more outcome variables (i.e., dependent variable). In order to accomplish this goal, experiments have to meet three basic criteria: (a) experimental manipulation (variation) of the independent variable(s), (b) randomization – the participants are randomly assigned to one of the experimental conditions, and (c) experimental control for the effect of third variables by eliminating them or keeping them constant.
In observational studies, investigators observe or assess individuals without manipulation or intervention. Observational studies are used for assessing the mean levels, the natural variation, and the structure of variables, as well as...
This is a preview of subscription content, log in via an institution to check access.
Access this chapter
Subscribe and save.
- Get 10 units per month
- Download Article/Chapter or eBook
- 1 Unit = 1 Article or 1 Chapter
- Cancel anytime
- Available as PDF
- Read on any device
- Instant download
- Own it forever
- Available as EPUB and PDF
- Durable hardcover edition
- Dispatched in 3 to 5 business days
- Free shipping worldwide - see info
Tax calculation will be finalised at checkout
Purchases are for personal use only
Institutional subscriptions
Atalay K, Barrett GF (2015) The impact of age pension eligibility age on retirement and program dependence: evidence from an Australian experiment. Rev Econ Stat 97:71–87. https://doi.org/10.1162/REST_a_00443
Article Google Scholar
Bergeman L, Boker SM (eds) (2016) Methodological issues in aging research. Psychology Press, Hove
Google Scholar
Byrkes CR, Bielak AMA (under review) Evaluation of publication bias and statistical power in gerontological psychology. Manuscript submitted for publication
Campbell DT, Stanley JC (1966) Experimental and quasi-experimental designs for research. Rand-McNally, Chicago
Carpenter D (2010) Reputation and power: organizational image and pharmaceutical regulation at the FDA. Princeton University Press, Princeton
Cavanaugh JC, Blanchard-Fields F (2019) Adult development and aging, 8th edn. Cengage, Boston
Fölster M, Hess U, Hühnel I et al (2015) Age-related response bias in the decoding of sad facial expressions. Behav Sci 5:443–460. https://doi.org/10.3390/bs5040443
Freund AM, Isaacowitz DM (2013) Beyond age comparisons: a plea for the use of a modified Brunswikian approach to experimental designs in the study of adult development and aging. Hum Dev 56:351–371. https://doi.org/10.1159/000357177
Haslam C, Morton TA, Haslam A et al (2012) “When the age is in, the wit is out”: age-related self-categorization and deficit expectations reduce performance on clinical tests used in dementia assessment. Psychol Aging 27:778–784. https://doi.org/10.1037/a0027754
Institute for Social Research (2018) The health and retirement study. Aging in the 21st century: Challenges and opportunities for americans. Survey Research Center, University of Michigan
Jung J (1971) The experimenter’s dilemma. Harper & Row, New York
Leary MR (2001) Introduction to behavioral research methods, 3rd edn. Allyn & Bacon, Boston
Lindenberger U, Scherer H, Baltes PB (2001) The strong connection between sensory and cognitive performance in old age: not due to sensory acuity reductions operating during cognitive assessment. Psychol Aging 16:196–205. https://doi.org/10.1037//0882-7974.16.2.196
Löckenhoff CE, Carstensen LL (2004) Socioemotional selectivity theory, aging, and health: the increasingly delicate balance between regulating emotions and making tough choices. J Pers 72:1395–1424. https://doi.org/10.1111/j.1467-6494.2004.00301.x
Maxwell SE (2015) Is psychology suffering from a replication crisis? What does “failure to replicate” really mean? Am Psychol 70:487–498. https://doi.org/10.1037/a0039400
Menard S (2002) Longitudinal research (2nd ed.). Sage, Thousand Oaks, CA
Mitchell SJ, Scheibye-Knudsen M, Longo DL et al (2015) Animal models of aging research: implications for human aging and age-related diseases. Ann Rev Anim Biosci 3:283–303. https://doi.org/10.1146/annurev-animal-022114-110829
Moher D (1998) CONSORT: an evolving tool to help improve the quality of reports of randomized controlled trials. JAMA 279:1489–1491. https://doi.org/10.1001/jama.279.18.1489
Oxford Centre for Evidence-Based Medicine (2011) OCEBM levels of evidence working group. The Oxford Levels of Evidence 2. Available at: https://www.cebm.net/category/ebm-resources/loe/ . Retrieved 2018-12-12
Patten ML, Newhart M (2018) Understanding research methods: an overview of the essentials, 10th edn. Routledge, New York
Piccinin AM, Muniz G, Sparks C et al (2011) An evaluation of analytical approaches for understanding change in cognition in the context of aging and health. J Geront 66B(S1):i36–i49. https://doi.org/10.1093/geronb/gbr038
Pinquart M, Silbereisen RK (2006) Socioemotional selectivity in cancer patients. Psychol Aging 21:419–423. https://doi.org/10.1037/0882-7974.21.2.419
Redman LM, Ravussin E (2011) Caloric restriction in humans: impact on physiological, psychological, and behavioral outcomes. Antioxid Redox Signal 14:275–287. https://doi.org/10.1089/ars.2010.3253
Rutter M (2007) Proceeding from observed correlation to causal inference: the use of natural experiments. Perspect Psychol Sci 2:377–395. https://doi.org/10.1111/j.1745-6916.2007.00050.x
Schaie W, Caskle CI (2005) Methodological issues in aging research. In: Teti D (ed) Handbook of research methods in developmental science. Blackwell, Malden, pp 21–39
Shadish WR, Cook TD, Campbell DT (2002) Experimental and quasi-experimental designs for generalized causal inference. Houghton Mifflin, Boston
Sonnega A, Faul JD, Ofstedal MB et al (2014) Cohort profile: the health and retirement study (HRS). Int J Epidemiol 43:576–585. https://doi.org/10.1093/ije/dyu067
Weil J (2017) Research design in aging and social gerontology: quantitative, qualitative, and mixed methods. Routledge, New York
Download references
Author information
Authors and affiliations.
Psychology, Philipps University, Marburg, Germany
Martin Pinquart
You can also search for this author in PubMed Google Scholar
Corresponding author
Correspondence to Martin Pinquart .
Editor information
Editors and affiliations.
Population Division, Department of Economics and Social Affairs, United Nations, New York, NY, USA
Department of Population Health Sciences, Department of Sociology, Duke University, Durham, NC, USA
Matthew E. Dupre
Section Editor information
Department of Sociology and Center for Population Health and Aging, Duke University, Durham, NC, USA
Kenneth C. Land
Department of Sociology, University of Kentucky, Lexington, KY, USA
Anthony R. Bardo
Rights and permissions
Reprints and permissions
Copyright information
© 2021 Springer Nature Switzerland AG
About this entry
Cite this entry.
Pinquart, M. (2021). Experimental Studies and Observational Studies. In: Gu, D., Dupre, M.E. (eds) Encyclopedia of Gerontology and Population Aging. Springer, Cham. https://doi.org/10.1007/978-3-030-22009-9_573
Download citation
DOI : https://doi.org/10.1007/978-3-030-22009-9_573
Published : 24 May 2022
Publisher Name : Springer, Cham
Print ISBN : 978-3-030-22008-2
Online ISBN : 978-3-030-22009-9
eBook Packages : Social Sciences Reference Module Humanities and Social Sciences Reference Module Business, Economics and Social Sciences
Share this entry
Anyone you share the following link with will be able to read this content:
Sorry, a shareable link is not currently available for this article.
Provided by the Springer Nature SharedIt content-sharing initiative
- Publish with us
Policies and ethics
- Find a journal
- Track your research
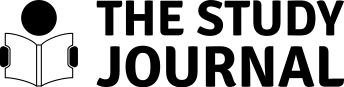
Observational Study vs Experimental Study: Which One is Right for Your Research?
When conducting research, it is essential to carefully consider the study design, whether to opt for an observational or an experimental approach. Observational studies and experimental studies are two main categories of research studies, each with its own strengths and limitations.
In observational studies, researchers observe the effect of a risk factor or intervention without trying to change exposure. This type of study design is useful when it is unethical or impractical to conduct an experimental study . There are different types of observational studies , including cohort studies and case control studies . In cohort studies , researchers compare what happens to members of a group exposed to a variable to those who are not exposed. In case control studies , researchers compare individuals with and without a health problem.
On the other hand, experimental studies involve introducing an intervention and studying its effects. Randomized controlled trials (RCTs) are a type of experimental study where eligible people are randomly assigned to different groups to receive either the intervention or a control. RCTs are considered the gold standard for producing reliable evidence and establishing cause-effect relationships.
In the realm of scientific research, choosing the right methodology can make or break the validity and impact of a study. Two primary approaches dominate the field: observational and experimental studies. While observational studies excel in exploring relationships and correlations, they often grapple with limitations such as confounding variables and a lack of causal inference.
On the other hand, experimental studies stand out for their ability to establish cause-and-effect relationships, thanks to the researcher’s ability to control variables. However, they are not without their drawbacks, including ethical considerations and sometimes limited real-world applicability.
Deciding when to use an experimental vs. observational study design hinges on your research objectives. Are you aiming for generalizability, or is pinpointing causality your main goal? The types of data collection methods you employ, whether quantitative or qualitative, will also depend on the design of your study.
For instance, survey design is often more prevalent in observational research, while experimental studies may involve more controlled data collection techniques. Both approaches have their pros and cons, and understanding these can guide you in achieving robust data analysis and fulfilling your research objectives.
So, whether you’re comparing the validity in observational and experimental research or pondering the ethics involved in each, it’s crucial to weigh the advantages and limitations of your chosen method.
This blog post aims to delve deeper into these aspects, offering a comprehensive guide to help you navigate the intricate landscape of research methods.When it comes to observational studies, they are open to dispute and can contain biases. However, they offer the advantage of studying phenomena as they naturally occur in real-life settings.
Experimental studies, on the other hand, are more controlled and can provide stronger evidence of causal relationships. However, they are often more expensive and may require a smaller sample size compared to observational studies.
Ultimately, the choice between observational and experimental study design depends on the research question and available resources. It is important to carefully assess the objectives of the study, ethical considerations, and the level of control necessary to achieve reliable results.
Image Description1: Types of observational studies, types of experimental studies,differences between observational and experimental studies, pros and cons of observational studies
- Observational studies involve observing the effect of a risk factor or intervention without trying to change exposure.
- Cohort studies and case control studies are types of observational studies .
- Experimental studies involve introducing an intervention and studying its effects.
- Randomized controlled trials (RCTs) are a type of experimental study and considered the gold standard for producing reliable evidence.
- Observational studies are useful when it is unethical or impractical to conduct an RCT.
Observational Study vs Experimental Study
Understanding observational studies.
Observational studies involve observing the effect of a risk factor or intervention without trying to change exposure, providing valuable insights into real-world scenarios. These studies play a crucial role in understanding the relationship between variables and identifying potential associations. Two common types of observational studies are cohort studies and case control studies.
“Observational studies provide an opportunity to examine how certain factors may influence outcomes in a natural setting. They allow researchers to explore a wide range of variables, including genetic, environmental, and lifestyle factors, providing a comprehensive understanding of complex phenomena.”
In cohort studies, researchers follow a group of individuals over time, comparing those exposed to a particular variable with those who are not. This allows for the examination of the development of diseases or outcomes between the two groups. On the other hand, case control studies involve comparing individuals with a specific health problem (cases) to those without the health problem (controls). By examining the exposure history of both groups, researchers can identify potential risk factors.
While observational studies offer numerous advantages, such as their ability to explore a wide range of variables and provide insights into long-term outcomes, they also have limitations. One significant drawback is the potential for bias, as researchers cannot control the exposure or intervention being studied. Additionally, observational studies may lack the ability to establish cause-effect relationships and are subject to confounding variables. Ethical considerations regarding informed consent, privacy, and data collection also play a vital role in observational studies.
Overall, understanding observational studies is essential for researchers and professionals in various fields. They provide valuable data that can inform decision-making and contribute to the advancement of knowledge in many areas. It is crucial to acknowledge the strengths and limitations of observational studies when interpreting their findings and to consider them in conjunction with other study designs to gain a comprehensive understanding of the research question at hand.

Image Description: Ethics in observational and experimental studies, when to use experimental vs observational study, observational vs experimental study design
Exploring Experimental Studies
Experimental studies involve introducing an intervention and carefully examining its impact, allowing researchers to establish cause-effect relationships. This study design offers several advantages that make it a powerful tool in scientific research. One major advantage is the level of control that researchers have over the variables in the study. By manipulating the independent variable and controlling for confounding factors, researchers can isolate the effects of the intervention and draw reliable conclusions. This control over variables is crucial in establishing causal relationships between the intervention and the observed outcomes.
Another strength of experimental studies is the ability to randomize participants into different groups. This random assignment helps to minimize bias and ensures that the groups being compared are similar in terms of all potential confounding variables. Randomized controlled trials (RCTs), a type of experimental study, are often considered the gold standard for producing reliable evidence. These studies randomly assign eligible individuals to either the intervention group or a control group, allowing for a more rigorous examination of the intervention’s effects.
When it comes to data collection , experimental studies often employ standardized protocols and procedures. This consistency helps to ensure the reliability and validity of the data collected. By following a predetermined plan, researchers can gather detailed and accurate information on the intervention and its outcomes. This rigorous data collection process contributes to the overall strength and credibility of experimental studies.
Ethics in Observational and Experimental Studies
Ethical considerations play a significant role in both observational and experimental studies. In experimental studies, researchers must ensure that the intervention being introduced is safe and does not pose any harm to the participants. Additionally, informed consent is a critical ethical requirement, as participants must be fully informed about the nature of the study and any potential risks or benefits involved.
In observational studies, ethical concerns arise when researchers are observing subjects without intervening or manipulating any variables. It is essential to consider privacy and confidentiality, ensuring that the participants’ information is protected and used only for research purposes. Ethical guidelines and review boards help to ensure that both observational and experimental studies adhere to ethical standards and prioritize the welfare of the participants.

Image Description: Observational vs experimental data collection
Experimental studies offer several advantages, including the ability to establish cause-effect relationships, rigorous data collection protocols, and the use of random assignment to minimize bias. Researchers have control over the variables being studied and can carefully manipulate the intervention to examine its impact. These strengths contribute to the reliability and validity of the findings obtained from experimental studies.

Types of Observational Studies
Observational studies can be classified into various types, including cohort studies and case control studies, each with its own strengths and limitations. Cohort studies involve comparing what happens to members of a group exposed to a particular variable to those who are not exposed. This type of study allows researchers to study the natural progression of a disease or the impact of an intervention over time.
Case control studies, on the other hand, involve comparing individuals with a specific health problem (cases) to those without the problem (controls). This type of study is useful in investigating rare diseases or conditions with long latency periods. By identifying individuals who already have the health problem, researchers can then look back and determine whether certain factors were more prevalent in the cases compared to the controls.
While observational studies are valuable in providing insights into the association between risk factors or interventions and health outcomes, they do have drawbacks. Generalizability, or the ability to apply the findings to a larger population, can be limited due to the specific characteristics of the study sample. Additionally, observational studies may be prone to biases, such as selection bias or confounding variables, which can affect the accuracy and validity of the results.
Despite these limitations, observational studies play a crucial role in generating hypotheses and informing public health interventions. They provide valuable real-world data and can often be conducted ethically when it is not feasible or ethical to perform experimental studies. However, when establishing cause-effect relationships and minimizing biases are essential, experimental studies, such as randomized controlled trials (RCTs), are the preferred study design.
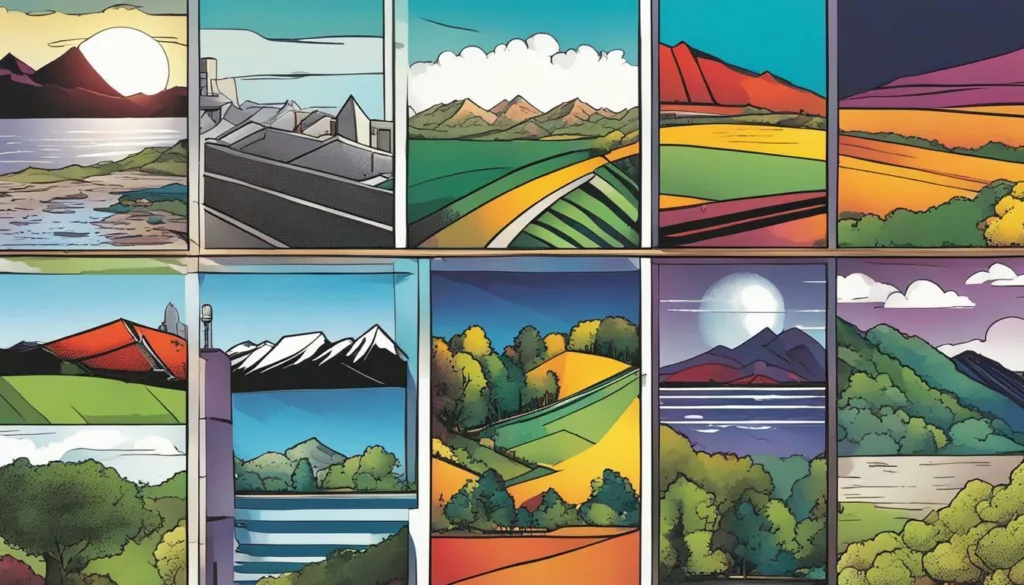
Image Description: Generalizability in observational vs experimental studies
Observational studies serve as a foundation for our understanding of health and disease, providing valuable data on the association between risk factors and outcomes. However, caution must be exercised in interpreting the findings, considering the limitations inherent in these study designs.
The Importance of Randomized Controlled Trials (RCTs)
Randomized controlled trials (RCTs) are considered the gold standard in research, providing rigorous evidence through random assignment of participants into different groups. By randomizing participants, RCTs ensure that any observed differences in outcomes between the intervention and control groups can be attributed to the effects of the intervention itself, rather than other factors.
This design helps eliminate bias and increases the validity of the study findings.
One of the key strengths of RCTs is their ability to establish cause-effect relationships. By comparing outcomes between the intervention and control groups, researchers can determine whether the intervention actually caused the observed effects. This is crucial when evaluating the effectiveness of new treatments or interventions before they are widely implemented.
Another advantage of RCTs is their ability to control for confounding variables.
Through random assignment, RCTs distribute these variables equally among the intervention and control groups, reducing the likelihood of confounding factors affecting the study results. This allows researchers to isolate the effects of the intervention, making the findings more reliable and applicable.
Moreover, RCTs are valuable in assessing the safety and efficacy of interventions.
They provide objective data on the benefits and risks associated with the intervention, enabling informed decision-making in healthcare and policy development. RCTs are particularly important in evaluating the effectiveness of new drugs, medical devices, and treatments, ensuring that they are safe and effective before reaching the market.
However, it is important to acknowledge the limitations of observational research when compared to RCTs.
Observational studies, such as cohort studies and case control studies, can be useful when RCTs are unethical or impractical to conduct. They can provide valuable insights into the natural course of diseases, risk factors, and long-term outcomes. However, they are open to biases and confounding factors, making it challenging to establish causality.
In conclusion , randomized controlled trials (RCTs) play a vital role in research, providing robust evidence through random assignment of participants and controlled experimental conditions.
They are essential for establishing cause-effect relationships and evaluating the safety and efficacy of interventions. While observational studies have their place in research, RCTs are widely regarded as the gold standard for producing reliable evidence. Choosing the appropriate study design based on the research question and available resources is crucial in conducting valid and impactful research.

Image Description: Comparing validity in observational and experimental research
When to Use Observational Studies
Observational studies are particularly useful when ethical or practical constraints make it difficult to conduct experimental studies. These studies involve observing the effect of a risk factor or intervention without trying to change exposure. When it is unethical to intervene or manipulate variables, observational studies provide a valuable approach to gather data and investigate relationships between variables.
One scenario where observational studies are appropriate is when studying the long-term effects of a risk factor. For example, if researchers want to explore the link between smoking and lung cancer, it would be unethical to assign individuals to smoke for an extended period. Instead, they can observe a large group of smokers and non-smokers over time, collecting data on their health outcomes to assess the potential association.
Additionally, observational studies are beneficial when studying rare diseases or events. Since rare conditions have a low occurrence rate, it might be impractical to conduct a controlled experiment specifically targeting those conditions. Observational studies provide an opportunity to investigate such rare occurrences in a more natural setting, enabling researchers to gain insights into potential risk factors or causes.
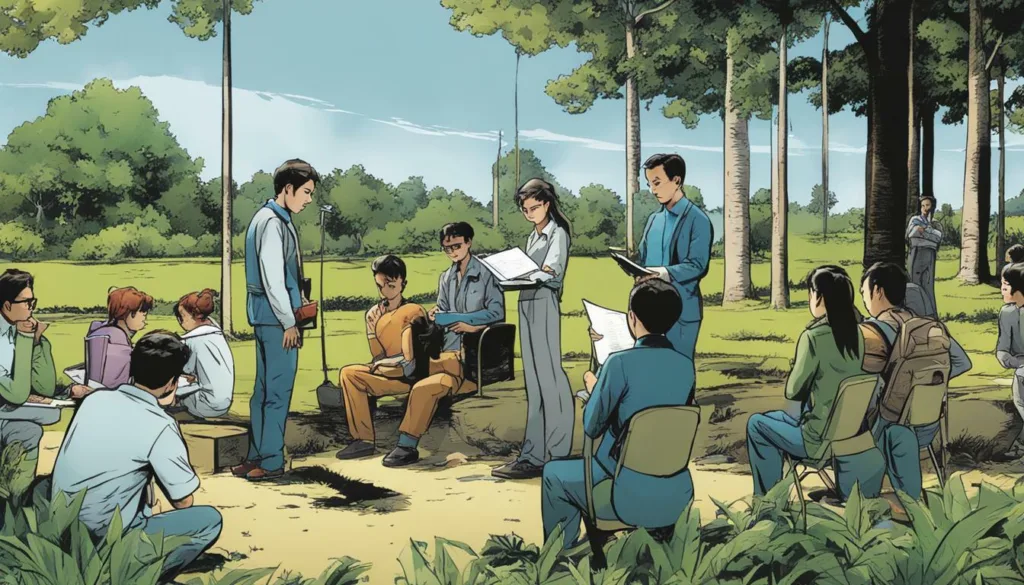
Image Description: Observational study drawbacks, experimental study strengths
While observational studies have their strengths, they also present limitations. There is always a risk of biases and confounding variables influencing the observed associations. Causality cannot be definitively established in observational studies, as other factors may be contributing to the observed relationship. Additionally, data collected in observational studies often relies on self-reporting, which can introduce inaccuracies.
In summary, when ethical or practical constraints limit the feasibility of conducting experimental studies, observational studies offer a valuable alternative. They allow researchers to gather data, observe associations, and explore relationships between variables in a real-world setting. However, it is crucial to be aware of the limitations of observational studies and carefully interpret the results to avoid drawing incorrect conclusions.
When to Use Experimental Studies
Experimental studies are particularly valuable when establishing cause-effect relationships and controlling variables is crucial for achieving research objectives . These studies involve introducing an intervention and carefully studying its effects under controlled conditions. By randomly assigning participants to different groups, researchers can minimize bias and draw conclusions about the impact of the intervention.
One of the primary advantages of experimental studies is their ability to provide strong evidence for causality. By manipulating the independent variable and measuring the dependent variable, researchers can establish a cause-and-effect relationship between the two. This is especially important when evaluating the effectiveness of new treatments or interventions.
Data collection in experimental studies is typically more structured and standardized compared to observational studies. Researchers can control variables, ensuring that any observed effects are directly attributed to the intervention being studied. This level of control allows for more accurate and reliable results.
However, it’s important to note that experimental studies may not always be feasible or ethical. Some research questions may involve interventions that are impractical or unethical to implement in a controlled setting. In such cases, observational studies can provide valuable insights. Additionally, experimental studies can be more time-consuming and expensive, requiring careful planning and sufficient resources.
Example of Experimental Study:
Research Objective: To investigate the effectiveness of a new drug in reducing symptoms of a specific medical condition.
This example highlights how an experimental study can provide valuable insights into the effectiveness of a new treatment. By controlling variables and manipulating the independent variable, researchers are able to establish a clear cause-effect relationship and draw conclusions about the impact of the intervention.

Designing Observational Studies
Designing observational studies requires careful planning, including determining research objectives , selecting appropriate survey designs, and choosing suitable data analysis methods. Observational studies are characterized by their ability to observe and collect data without directly manipulating variables or interventions. This study design is commonly used when it is unethical or impractical to conduct experimental studies, such as in cases where it is not possible to assign participants to different groups or manipulate certain variables.
When designing an observational study , researchers must first establish clear research objectives . This involves identifying the specific research question or hypothesis to be addressed and defining the scope and objectives of the study. By clearly outlining the goals of the research, researchers can ensure that their study design aligns with their objectives and addresses relevant variables or factors.

Image Description: Quantitative research, qualitative research, design of study, survey design, research objectives, data analysis
In terms of survey design , observational studies rely on the collection of data through observations or surveys. Researchers must carefully choose the appropriate survey design based on the nature of their research question and the target population. Common survey designs used in observational studies include cross-sectional studies, cohort studies, and case-control studies. Each design has its strengths and limitations, and researchers must select the most suitable design for their specific research objectives.
Data analysis is a crucial component of observational studies. Researchers must choose appropriate methods to analyze the collected data to draw meaningful conclusions. This may involve statistical analysis techniques, qualitative analysis methods, or a combination of both, depending on the nature of the data and research question. Data analysis methods must be selected in a way that allows for accurate interpretation and provides valuable insights into the observed phenomena.
Designing Experimental Studies
Designing experimental studies involves meticulous planning to ensure research objectives are met, data analysis is accurate, and variables are effectively controlled. In experimental studies, the primary goal is to establish cause-effect relationships by introducing an intervention and studying its effects. To achieve this, researchers must carefully consider several key aspects of study design.
Data Analysis: One of the crucial steps in designing an experimental study is determining the appropriate data analysis methods. Quantitative research methods, such as statistical analysis, are commonly used in experimental studies to analyze numerical data and identify patterns or associations. On the other hand, qualitative research methods, such as content analysis or thematic analysis, can provide valuable insights into participants’ perspectives and experiences. The choice of data analysis approach depends on the research objectives and the type of data collected.
Control Variables: Another important consideration is controlling variables. Experimental studies aim to isolate the effects of the intervention by controlling for other factors that could influence the outcome. This involves identifying and selecting relevant variables that may confound the results and implementing strategies to minimize their impact. By controlling variables, researchers can increase the internal validity of the study and strengthen the causal inference.
Sample Size and Sampling Strategy: Determining an appropriate sample size is crucial to ensure the study’s statistical power and generalizability of the findings. The sample size calculation should take into account the desired effect size, statistical significance level, and the anticipated variability of the outcome measure. Additionally, researchers need to carefully consider the sampling strategy to select a representative sample that accurately reflects the target population. Randomization techniques, such as simple random sampling or stratified random sampling, can help minimize bias and increase the external validity of the study.

Designing experimental studies requires careful planning and consideration of various factors to ensure the research objectives are achieved. By implementing robust study design and rigorous data analysis methods, researchers can produce reliable evidence and establish cause-effect relationships. However, it is important to note that experimental studies can be resource-intensive and may require specific expertise, making it essential to assess the available resources and feasibility before embarking on such research.
Choosing between observational studies and experimental studies depends on various factors, including research objectives, data collection methods, and the desired outcomes. Observational studies involve observing the effect of a risk factor or intervention without trying to change exposure. They are useful when it is unethical or impractical to conduct an experimental study, such as studying the effects of smoking on lung cancer. However, observational studies are open to dispute and can contain biases, such as selection bias or information bias.
On the other hand, experimental studies involve introducing an intervention and studying its effects. Randomized controlled trials (RCTs) are a type of experimental study that are considered the gold standard for producing reliable evidence. In RCTs, eligible participants are randomly assigned to different groups to receive either the intervention or a control. Experimental studies are more controlled and can establish cause-effect relationships, such as testing the effectiveness of a new drug. However, they are often expensive and may require a smaller sample size compared to observational studies.
It is crucial to select the most appropriate study design based on the research question and the available resources. If the research aims to investigate the association between two variables, and it is not feasible to change the exposure of participants, an observational study would be the preferred choice. However, if the research aims to establish causality and control the exposure, an experimental study, such as an RCT, would be more suitable.
In conclusion , both observational studies and experimental studies have their strengths and limitations. The choice between them depends on the specific research objectives, the ability to control the exposure or intervention, and the resources available. By understanding the differences between these study designs and considering these factors, researchers can make informed decisions to ensure the validity and reliability of their findings.
Q: What are the main differences between observational studies and experimental studies?
A: Observational studies involve observing the effect of a risk factor or intervention without trying to change exposure, while experimental studies involve introducing an intervention and studying its effects.
Q: What types of observational studies exist?
A: Cohort studies and case control studies are types of observational studies.
Q: How do cohort studies and case control studies differ?
A: In cohort studies, researchers compare what happens to members of a group exposed to a variable to those who are not exposed. In case control studies, researchers compare individuals with and without a health problem.
Q: What are randomized controlled trials (RCTs)?
A: Randomized controlled trials (RCTs) are a type of experimental study where eligible people are randomly assigned to different groups to receive either the intervention or a control. RCTs are considered the gold standard for producing reliable evidence.
Q: When are observational studies useful?
A: Observational studies are useful when it is unethical or impractical to conduct a randomized controlled trial (RCT).
Q: What are the advantages of experimental studies?
A: Experimental studies are more controlled and can establish cause-effect relationships. They are considered the gold standard for producing reliable evidence.
Q: What are the limitations of observational studies?
A: Observational studies are open to dispute and can contain biases. They also have limitations in terms of generalizability and establishing cause-effect relationships.
Q: Are observational studies more cost-effective compared to experimental studies?
A: Observational studies are generally more cost-effective compared to experimental studies, as they may require a smaller sample size.
Q: How do I choose the appropriate study design for my research?
A: The choice of study design depends on the specific research question and the available resources. Observational studies are preferred when ethical or practical constraints prevent the use of experimental studies, while experimental studies are suitable for establishing cause-effect relationships.
Frequently asked questions
How does an observational study differ from an experiment.
The key difference between observational studies and experiments is that, done correctly, an observational study will never influence the responses or behaviours of participants. Experimental designs will have a treatment condition applied to at least a portion of participants.
Frequently asked questions: Methodology
Quantitative observations involve measuring or counting something and expressing the result in numerical form, while qualitative observations involve describing something in non-numerical terms, such as its appearance, texture, or color.
To make quantitative observations , you need to use instruments that are capable of measuring the quantity you want to observe. For example, you might use a ruler to measure the length of an object or a thermometer to measure its temperature.
Scope of research is determined at the beginning of your research process , prior to the data collection stage. Sometimes called “scope of study,” your scope delineates what will and will not be covered in your project. It helps you focus your work and your time, ensuring that you’ll be able to achieve your goals and outcomes.
Defining a scope can be very useful in any research project, from a research proposal to a thesis or dissertation . A scope is needed for all types of research: quantitative , qualitative , and mixed methods .
To define your scope of research, consider the following:
- Budget constraints or any specifics of grant funding
- Your proposed timeline and duration
- Specifics about your population of study, your proposed sample size , and the research methodology you’ll pursue
- Any inclusion and exclusion criteria
- Any anticipated control , extraneous , or confounding variables that could bias your research if not accounted for properly.
Inclusion and exclusion criteria are predominantly used in non-probability sampling . In purposive sampling and snowball sampling , restrictions apply as to who can be included in the sample .
Inclusion and exclusion criteria are typically presented and discussed in the methodology section of your thesis or dissertation .
The purpose of theory-testing mode is to find evidence in order to disprove, refine, or support a theory. As such, generalisability is not the aim of theory-testing mode.
Due to this, the priority of researchers in theory-testing mode is to eliminate alternative causes for relationships between variables . In other words, they prioritise internal validity over external validity , including ecological validity .
Convergent validity shows how much a measure of one construct aligns with other measures of the same or related constructs .
On the other hand, concurrent validity is about how a measure matches up to some known criterion or gold standard, which can be another measure.
Although both types of validity are established by calculating the association or correlation between a test score and another variable , they represent distinct validation methods.
Validity tells you how accurately a method measures what it was designed to measure. There are 4 main types of validity :
- Construct validity : Does the test measure the construct it was designed to measure?
- Face validity : Does the test appear to be suitable for its objectives ?
- Content validity : Does the test cover all relevant parts of the construct it aims to measure.
- Criterion validity : Do the results accurately measure the concrete outcome they are designed to measure?
Criterion validity evaluates how well a test measures the outcome it was designed to measure. An outcome can be, for example, the onset of a disease.
Criterion validity consists of two subtypes depending on the time at which the two measures (the criterion and your test) are obtained:
- Concurrent validity is a validation strategy where the the scores of a test and the criterion are obtained at the same time
- Predictive validity is a validation strategy where the criterion variables are measured after the scores of the test
Attrition refers to participants leaving a study. It always happens to some extent – for example, in randomised control trials for medical research.
Differential attrition occurs when attrition or dropout rates differ systematically between the intervention and the control group . As a result, the characteristics of the participants who drop out differ from the characteristics of those who stay in the study. Because of this, study results may be biased .
Criterion validity and construct validity are both types of measurement validity . In other words, they both show you how accurately a method measures something.
While construct validity is the degree to which a test or other measurement method measures what it claims to measure, criterion validity is the degree to which a test can predictively (in the future) or concurrently (in the present) measure something.
Construct validity is often considered the overarching type of measurement validity . You need to have face validity , content validity , and criterion validity in order to achieve construct validity.
Convergent validity and discriminant validity are both subtypes of construct validity . Together, they help you evaluate whether a test measures the concept it was designed to measure.
- Convergent validity indicates whether a test that is designed to measure a particular construct correlates with other tests that assess the same or similar construct.
- Discriminant validity indicates whether two tests that should not be highly related to each other are indeed not related. This type of validity is also called divergent validity .
You need to assess both in order to demonstrate construct validity. Neither one alone is sufficient for establishing construct validity.
Face validity and content validity are similar in that they both evaluate how suitable the content of a test is. The difference is that face validity is subjective, and assesses content at surface level.
When a test has strong face validity, anyone would agree that the test’s questions appear to measure what they are intended to measure.
For example, looking at a 4th grade math test consisting of problems in which students have to add and multiply, most people would agree that it has strong face validity (i.e., it looks like a math test).
On the other hand, content validity evaluates how well a test represents all the aspects of a topic. Assessing content validity is more systematic and relies on expert evaluation. of each question, analysing whether each one covers the aspects that the test was designed to cover.
A 4th grade math test would have high content validity if it covered all the skills taught in that grade. Experts(in this case, math teachers), would have to evaluate the content validity by comparing the test to the learning objectives.
Content validity shows you how accurately a test or other measurement method taps into the various aspects of the specific construct you are researching.
In other words, it helps you answer the question: “does the test measure all aspects of the construct I want to measure?” If it does, then the test has high content validity.
The higher the content validity, the more accurate the measurement of the construct.
If the test fails to include parts of the construct, or irrelevant parts are included, the validity of the instrument is threatened, which brings your results into question.
Construct validity refers to how well a test measures the concept (or construct) it was designed to measure. Assessing construct validity is especially important when you’re researching concepts that can’t be quantified and/or are intangible, like introversion. To ensure construct validity your test should be based on known indicators of introversion ( operationalisation ).
On the other hand, content validity assesses how well the test represents all aspects of the construct. If some aspects are missing or irrelevant parts are included, the test has low content validity.
- Discriminant validity indicates whether two tests that should not be highly related to each other are indeed not related
Construct validity has convergent and discriminant subtypes. They assist determine if a test measures the intended notion.
The reproducibility and replicability of a study can be ensured by writing a transparent, detailed method section and using clear, unambiguous language.
Reproducibility and replicability are related terms.
- A successful reproduction shows that the data analyses were conducted in a fair and honest manner.
- A successful replication shows that the reliability of the results is high.
- Reproducing research entails reanalysing the existing data in the same manner.
- Replicating (or repeating ) the research entails reconducting the entire analysis, including the collection of new data .
Snowball sampling is a non-probability sampling method . Unlike probability sampling (which involves some form of random selection ), the initial individuals selected to be studied are the ones who recruit new participants.
Because not every member of the target population has an equal chance of being recruited into the sample, selection in snowball sampling is non-random.
Snowball sampling is a non-probability sampling method , where there is not an equal chance for every member of the population to be included in the sample .
This means that you cannot use inferential statistics and make generalisations – often the goal of quantitative research . As such, a snowball sample is not representative of the target population, and is usually a better fit for qualitative research .
Snowball sampling relies on the use of referrals. Here, the researcher recruits one or more initial participants, who then recruit the next ones.
Participants share similar characteristics and/or know each other. Because of this, not every member of the population has an equal chance of being included in the sample, giving rise to sampling bias .
Snowball sampling is best used in the following cases:
- If there is no sampling frame available (e.g., people with a rare disease)
- If the population of interest is hard to access or locate (e.g., people experiencing homelessness)
- If the research focuses on a sensitive topic (e.g., extra-marital affairs)
Stratified sampling and quota sampling both involve dividing the population into subgroups and selecting units from each subgroup. The purpose in both cases is to select a representative sample and/or to allow comparisons between subgroups.
The main difference is that in stratified sampling, you draw a random sample from each subgroup ( probability sampling ). In quota sampling you select a predetermined number or proportion of units, in a non-random manner ( non-probability sampling ).
Random sampling or probability sampling is based on random selection. This means that each unit has an equal chance (i.e., equal probability) of being included in the sample.
On the other hand, convenience sampling involves stopping people at random, which means that not everyone has an equal chance of being selected depending on the place, time, or day you are collecting your data.
Convenience sampling and quota sampling are both non-probability sampling methods. They both use non-random criteria like availability, geographical proximity, or expert knowledge to recruit study participants.
However, in convenience sampling, you continue to sample units or cases until you reach the required sample size.
In quota sampling, you first need to divide your population of interest into subgroups (strata) and estimate their proportions (quota) in the population. Then you can start your data collection , using convenience sampling to recruit participants, until the proportions in each subgroup coincide with the estimated proportions in the population.
A sampling frame is a list of every member in the entire population . It is important that the sampling frame is as complete as possible, so that your sample accurately reflects your population.
Stratified and cluster sampling may look similar, but bear in mind that groups created in cluster sampling are heterogeneous , so the individual characteristics in the cluster vary. In contrast, groups created in stratified sampling are homogeneous , as units share characteristics.
Relatedly, in cluster sampling you randomly select entire groups and include all units of each group in your sample. However, in stratified sampling, you select some units of all groups and include them in your sample. In this way, both methods can ensure that your sample is representative of the target population .
When your population is large in size, geographically dispersed, or difficult to contact, it’s necessary to use a sampling method .
This allows you to gather information from a smaller part of the population, i.e. the sample, and make accurate statements by using statistical analysis. A few sampling methods include simple random sampling , convenience sampling , and snowball sampling .
The two main types of social desirability bias are:
- Self-deceptive enhancement (self-deception): The tendency to see oneself in a favorable light without realizing it.
- Impression managemen t (other-deception): The tendency to inflate one’s abilities or achievement in order to make a good impression on other people.
Response bias refers to conditions or factors that take place during the process of responding to surveys, affecting the responses. One type of response bias is social desirability bias .
Demand characteristics are aspects of experiments that may give away the research objective to participants. Social desirability bias occurs when participants automatically try to respond in ways that make them seem likeable in a study, even if it means misrepresenting how they truly feel.
Participants may use demand characteristics to infer social norms or experimenter expectancies and act in socially desirable ways, so you should try to control for demand characteristics wherever possible.
A systematic review is secondary research because it uses existing research. You don’t collect new data yourself.
Ethical considerations in research are a set of principles that guide your research designs and practices. These principles include voluntary participation, informed consent, anonymity, confidentiality, potential for harm, and results communication.
Scientists and researchers must always adhere to a certain code of conduct when collecting data from others .
These considerations protect the rights of research participants, enhance research validity , and maintain scientific integrity.
Research ethics matter for scientific integrity, human rights and dignity, and collaboration between science and society. These principles make sure that participation in studies is voluntary, informed, and safe.
Research misconduct means making up or falsifying data, manipulating data analyses, or misrepresenting results in research reports. It’s a form of academic fraud.
These actions are committed intentionally and can have serious consequences; research misconduct is not a simple mistake or a point of disagreement but a serious ethical failure.
Anonymity means you don’t know who the participants are, while confidentiality means you know who they are but remove identifying information from your research report. Both are important ethical considerations .
You can only guarantee anonymity by not collecting any personally identifying information – for example, names, phone numbers, email addresses, IP addresses, physical characteristics, photos, or videos.
You can keep data confidential by using aggregate information in your research report, so that you only refer to groups of participants rather than individuals.
Peer review is a process of evaluating submissions to an academic journal. Utilising rigorous criteria, a panel of reviewers in the same subject area decide whether to accept each submission for publication.
For this reason, academic journals are often considered among the most credible sources you can use in a research project – provided that the journal itself is trustworthy and well regarded.
In general, the peer review process follows the following steps:
- First, the author submits the manuscript to the editor.
- Reject the manuscript and send it back to author, or
- Send it onward to the selected peer reviewer(s)
- Next, the peer review process occurs. The reviewer provides feedback, addressing any major or minor issues with the manuscript, and gives their advice regarding what edits should be made.
- Lastly, the edited manuscript is sent back to the author. They input the edits, and resubmit it to the editor for publication.
Peer review can stop obviously problematic, falsified, or otherwise untrustworthy research from being published. It also represents an excellent opportunity to get feedback from renowned experts in your field.
It acts as a first defence, helping you ensure your argument is clear and that there are no gaps, vague terms, or unanswered questions for readers who weren’t involved in the research process.
Peer-reviewed articles are considered a highly credible source due to this stringent process they go through before publication.
Many academic fields use peer review , largely to determine whether a manuscript is suitable for publication. Peer review enhances the credibility of the published manuscript.
However, peer review is also common in non-academic settings. The United Nations, the European Union, and many individual nations use peer review to evaluate grant applications. It is also widely used in medical and health-related fields as a teaching or quality-of-care measure.
Peer assessment is often used in the classroom as a pedagogical tool. Both receiving feedback and providing it are thought to enhance the learning process, helping students think critically and collaboratively.
- In a single-blind study , only the participants are blinded.
- In a double-blind study , both participants and experimenters are blinded.
- In a triple-blind study , the assignment is hidden not only from participants and experimenters, but also from the researchers analysing the data.
Blinding is important to reduce bias (e.g., observer bias , demand characteristics ) and ensure a study’s internal validity .
If participants know whether they are in a control or treatment group , they may adjust their behaviour in ways that affect the outcome that researchers are trying to measure. If the people administering the treatment are aware of group assignment, they may treat participants differently and thus directly or indirectly influence the final results.
Blinding means hiding who is assigned to the treatment group and who is assigned to the control group in an experiment .
Explanatory research is a research method used to investigate how or why something occurs when only a small amount of information is available pertaining to that topic. It can help you increase your understanding of a given topic.
Explanatory research is used to investigate how or why a phenomenon occurs. Therefore, this type of research is often one of the first stages in the research process , serving as a jumping-off point for future research.
Exploratory research is a methodology approach that explores research questions that have not previously been studied in depth. It is often used when the issue you’re studying is new, or the data collection process is challenging in some way.
Exploratory research is often used when the issue you’re studying is new or when the data collection process is challenging for some reason.
You can use exploratory research if you have a general idea or a specific question that you want to study but there is no preexisting knowledge or paradigm with which to study it.
To implement random assignment , assign a unique number to every member of your study’s sample .
Then, you can use a random number generator or a lottery method to randomly assign each number to a control or experimental group. You can also do so manually, by flipping a coin or rolling a die to randomly assign participants to groups.
Random selection, or random sampling , is a way of selecting members of a population for your study’s sample.
In contrast, random assignment is a way of sorting the sample into control and experimental groups.
Random sampling enhances the external validity or generalisability of your results, while random assignment improves the internal validity of your study.
Random assignment is used in experiments with a between-groups or independent measures design. In this research design, there’s usually a control group and one or more experimental groups. Random assignment helps ensure that the groups are comparable.
In general, you should always use random assignment in this type of experimental design when it is ethically possible and makes sense for your study topic.
Clean data are valid, accurate, complete, consistent, unique, and uniform. Dirty data include inconsistencies and errors.
Dirty data can come from any part of the research process, including poor research design , inappropriate measurement materials, or flawed data entry.
Data cleaning takes place between data collection and data analyses. But you can use some methods even before collecting data.
For clean data, you should start by designing measures that collect valid data. Data validation at the time of data entry or collection helps you minimize the amount of data cleaning you’ll need to do.
After data collection, you can use data standardisation and data transformation to clean your data. You’ll also deal with any missing values, outliers, and duplicate values.
Data cleaning involves spotting and resolving potential data inconsistencies or errors to improve your data quality. An error is any value (e.g., recorded weight) that doesn’t reflect the true value (e.g., actual weight) of something that’s being measured.
In this process, you review, analyse, detect, modify, or remove ‘dirty’ data to make your dataset ‘clean’. Data cleaning is also called data cleansing or data scrubbing.
Data cleaning is necessary for valid and appropriate analyses. Dirty data contain inconsistencies or errors , but cleaning your data helps you minimise or resolve these.
Without data cleaning, you could end up with a Type I or II error in your conclusion. These types of erroneous conclusions can be practically significant with important consequences, because they lead to misplaced investments or missed opportunities.
Observer bias occurs when a researcher’s expectations, opinions, or prejudices influence what they perceive or record in a study. It usually affects studies when observers are aware of the research aims or hypotheses. This type of research bias is also called detection bias or ascertainment bias .
The observer-expectancy effect occurs when researchers influence the results of their own study through interactions with participants.
Researchers’ own beliefs and expectations about the study results may unintentionally influence participants through demand characteristics .
You can use several tactics to minimise observer bias .
- Use masking (blinding) to hide the purpose of your study from all observers.
- Triangulate your data with different data collection methods or sources.
- Use multiple observers and ensure inter-rater reliability.
- Train your observers to make sure data is consistently recorded between them.
- Standardise your observation procedures to make sure they are structured and clear.
Naturalistic observation is a valuable tool because of its flexibility, external validity , and suitability for topics that can’t be studied in a lab setting.
The downsides of naturalistic observation include its lack of scientific control , ethical considerations , and potential for bias from observers and subjects.
Naturalistic observation is a qualitative research method where you record the behaviours of your research subjects in real-world settings. You avoid interfering or influencing anything in a naturalistic observation.
You can think of naturalistic observation as ‘people watching’ with a purpose.
Closed-ended, or restricted-choice, questions offer respondents a fixed set of choices to select from. These questions are easier to answer quickly.
Open-ended or long-form questions allow respondents to answer in their own words. Because there are no restrictions on their choices, respondents can answer in ways that researchers may not have otherwise considered.
You can organise the questions logically, with a clear progression from simple to complex, or randomly between respondents. A logical flow helps respondents process the questionnaire easier and quicker, but it may lead to bias. Randomisation can minimise the bias from order effects.
Questionnaires can be self-administered or researcher-administered.
Self-administered questionnaires can be delivered online or in paper-and-pen formats, in person or by post. All questions are standardised so that all respondents receive the same questions with identical wording.
Researcher-administered questionnaires are interviews that take place by phone, in person, or online between researchers and respondents. You can gain deeper insights by clarifying questions for respondents or asking follow-up questions.
In a controlled experiment , all extraneous variables are held constant so that they can’t influence the results. Controlled experiments require:
- A control group that receives a standard treatment, a fake treatment, or no treatment
- Random assignment of participants to ensure the groups are equivalent
Depending on your study topic, there are various other methods of controlling variables .
An experimental group, also known as a treatment group, receives the treatment whose effect researchers wish to study, whereas a control group does not. They should be identical in all other ways.
A true experiment (aka a controlled experiment) always includes at least one control group that doesn’t receive the experimental treatment.
However, some experiments use a within-subjects design to test treatments without a control group. In these designs, you usually compare one group’s outcomes before and after a treatment (instead of comparing outcomes between different groups).
For strong internal validity , it’s usually best to include a control group if possible. Without a control group, it’s harder to be certain that the outcome was caused by the experimental treatment and not by other variables.
A questionnaire is a data collection tool or instrument, while a survey is an overarching research method that involves collecting and analysing data from people using questionnaires.
A Likert scale is a rating scale that quantitatively assesses opinions, attitudes, or behaviours. It is made up of four or more questions that measure a single attitude or trait when response scores are combined.
To use a Likert scale in a survey , you present participants with Likert-type questions or statements, and a continuum of items, usually with five or seven possible responses, to capture their degree of agreement.
Individual Likert-type questions are generally considered ordinal data , because the items have clear rank order, but don’t have an even distribution.
Overall Likert scale scores are sometimes treated as interval data. These scores are considered to have directionality and even spacing between them.
The type of data determines what statistical tests you should use to analyse your data.
A research hypothesis is your proposed answer to your research question. The research hypothesis usually includes an explanation (‘ x affects y because …’).
A statistical hypothesis, on the other hand, is a mathematical statement about a population parameter. Statistical hypotheses always come in pairs: the null and alternative hypotheses. In a well-designed study , the statistical hypotheses correspond logically to the research hypothesis.
A hypothesis states your predictions about what your research will find. It is a tentative answer to your research question that has not yet been tested. For some research projects, you might have to write several hypotheses that address different aspects of your research question.
A hypothesis is not just a guess. It should be based on existing theories and knowledge. It also has to be testable, which means you can support or refute it through scientific research methods (such as experiments, observations, and statistical analysis of data).
Cross-sectional studies are less expensive and time-consuming than many other types of study. They can provide useful insights into a population’s characteristics and identify correlations for further research.
Sometimes only cross-sectional data are available for analysis; other times your research question may only require a cross-sectional study to answer it.
Cross-sectional studies cannot establish a cause-and-effect relationship or analyse behaviour over a period of time. To investigate cause and effect, you need to do a longitudinal study or an experimental study .
Longitudinal studies and cross-sectional studies are two different types of research design . In a cross-sectional study you collect data from a population at a specific point in time; in a longitudinal study you repeatedly collect data from the same sample over an extended period of time.
Longitudinal studies are better to establish the correct sequence of events, identify changes over time, and provide insight into cause-and-effect relationships, but they also tend to be more expensive and time-consuming than other types of studies.
The 1970 British Cohort Study , which has collected data on the lives of 17,000 Brits since their births in 1970, is one well-known example of a longitudinal study .
Longitudinal studies can last anywhere from weeks to decades, although they tend to be at least a year long.
A correlation reflects the strength and/or direction of the association between two or more variables.
- A positive correlation means that both variables change in the same direction.
- A negative correlation means that the variables change in opposite directions.
- A zero correlation means there’s no relationship between the variables.
A correlational research design investigates relationships between two variables (or more) without the researcher controlling or manipulating any of them. It’s a non-experimental type of quantitative research .
A correlation coefficient is a single number that describes the strength and direction of the relationship between your variables.
Different types of correlation coefficients might be appropriate for your data based on their levels of measurement and distributions . The Pearson product-moment correlation coefficient (Pearson’s r ) is commonly used to assess a linear relationship between two quantitative variables.
Controlled experiments establish causality, whereas correlational studies only show associations between variables.
- In an experimental design , you manipulate an independent variable and measure its effect on a dependent variable. Other variables are controlled so they can’t impact the results.
- In a correlational design , you measure variables without manipulating any of them. You can test whether your variables change together, but you can’t be sure that one variable caused a change in another.
In general, correlational research is high in external validity while experimental research is high in internal validity .
The third variable and directionality problems are two main reasons why correlation isn’t causation .
The third variable problem means that a confounding variable affects both variables to make them seem causally related when they are not.
The directionality problem is when two variables correlate and might actually have a causal relationship, but it’s impossible to conclude which variable causes changes in the other.
As a rule of thumb, questions related to thoughts, beliefs, and feelings work well in focus groups . Take your time formulating strong questions, paying special attention to phrasing. Be careful to avoid leading questions , which can bias your responses.
Overall, your focus group questions should be:
- Open-ended and flexible
- Impossible to answer with ‘yes’ or ‘no’ (questions that start with ‘why’ or ‘how’ are often best)
- Unambiguous, getting straight to the point while still stimulating discussion
- Unbiased and neutral
Social desirability bias is the tendency for interview participants to give responses that will be viewed favourably by the interviewer or other participants. It occurs in all types of interviews and surveys , but is most common in semi-structured interviews , unstructured interviews , and focus groups .
Social desirability bias can be mitigated by ensuring participants feel at ease and comfortable sharing their views. Make sure to pay attention to your own body language and any physical or verbal cues, such as nodding or widening your eyes.
This type of bias in research can also occur in observations if the participants know they’re being observed. They might alter their behaviour accordingly.
A focus group is a research method that brings together a small group of people to answer questions in a moderated setting. The group is chosen due to predefined demographic traits, and the questions are designed to shed light on a topic of interest. It is one of four types of interviews .
The four most common types of interviews are:
- Structured interviews : The questions are predetermined in both topic and order.
- Semi-structured interviews : A few questions are predetermined, but other questions aren’t planned.
- Unstructured interviews : None of the questions are predetermined.
- Focus group interviews : The questions are presented to a group instead of one individual.
An unstructured interview is the most flexible type of interview, but it is not always the best fit for your research topic.
Unstructured interviews are best used when:
- You are an experienced interviewer and have a very strong background in your research topic, since it is challenging to ask spontaneous, colloquial questions
- Your research question is exploratory in nature. While you may have developed hypotheses, you are open to discovering new or shifting viewpoints through the interview process.
- You are seeking descriptive data, and are ready to ask questions that will deepen and contextualise your initial thoughts and hypotheses
- Your research depends on forming connections with your participants and making them feel comfortable revealing deeper emotions, lived experiences, or thoughts
A semi-structured interview is a blend of structured and unstructured types of interviews. Semi-structured interviews are best used when:
- You have prior interview experience. Spontaneous questions are deceptively challenging, and it’s easy to accidentally ask a leading question or make a participant uncomfortable.
- Your research question is exploratory in nature. Participant answers can guide future research questions and help you develop a more robust knowledge base for future research.
The interviewer effect is a type of bias that emerges when a characteristic of an interviewer (race, age, gender identity, etc.) influences the responses given by the interviewee.
There is a risk of an interviewer effect in all types of interviews , but it can be mitigated by writing really high-quality interview questions.
A structured interview is a data collection method that relies on asking questions in a set order to collect data on a topic. They are often quantitative in nature. Structured interviews are best used when:
- You already have a very clear understanding of your topic. Perhaps significant research has already been conducted, or you have done some prior research yourself, but you already possess a baseline for designing strong structured questions.
- You are constrained in terms of time or resources and need to analyse your data quickly and efficiently
- Your research question depends on strong parity between participants, with environmental conditions held constant
More flexible interview options include semi-structured interviews , unstructured interviews , and focus groups .
When conducting research, collecting original data has significant advantages:
- You can tailor data collection to your specific research aims (e.g., understanding the needs of your consumers or user testing your website).
- You can control and standardise the process for high reliability and validity (e.g., choosing appropriate measurements and sampling methods ).
However, there are also some drawbacks: data collection can be time-consuming, labour-intensive, and expensive. In some cases, it’s more efficient to use secondary data that has already been collected by someone else, but the data might be less reliable.
Data collection is the systematic process by which observations or measurements are gathered in research. It is used in many different contexts by academics, governments, businesses, and other organisations.
A mediator variable explains the process through which two variables are related, while a moderator variable affects the strength and direction of that relationship.
A confounder is a third variable that affects variables of interest and makes them seem related when they are not. In contrast, a mediator is the mechanism of a relationship between two variables: it explains the process by which they are related.
If something is a mediating variable :
- It’s caused by the independent variable
- It influences the dependent variable
- When it’s taken into account, the statistical correlation between the independent and dependent variables is higher than when it isn’t considered
Including mediators and moderators in your research helps you go beyond studying a simple relationship between two variables for a fuller picture of the real world. They are important to consider when studying complex correlational or causal relationships.
Mediators are part of the causal pathway of an effect, and they tell you how or why an effect takes place. Moderators usually help you judge the external validity of your study by identifying the limitations of when the relationship between variables holds.
You can think of independent and dependent variables in terms of cause and effect: an independent variable is the variable you think is the cause , while a dependent variable is the effect .
In an experiment, you manipulate the independent variable and measure the outcome in the dependent variable. For example, in an experiment about the effect of nutrients on crop growth:
- The independent variable is the amount of nutrients added to the crop field.
- The dependent variable is the biomass of the crops at harvest time.
Defining your variables, and deciding how you will manipulate and measure them, is an important part of experimental design .
Discrete and continuous variables are two types of quantitative variables :
- Discrete variables represent counts (e.g., the number of objects in a collection).
- Continuous variables represent measurable amounts (e.g., water volume or weight).
Quantitative variables are any variables where the data represent amounts (e.g. height, weight, or age).
Categorical variables are any variables where the data represent groups. This includes rankings (e.g. finishing places in a race), classifications (e.g. brands of cereal), and binary outcomes (e.g. coin flips).
You need to know what type of variables you are working with to choose the right statistical test for your data and interpret your results .
Determining cause and effect is one of the most important parts of scientific research. It’s essential to know which is the cause – the independent variable – and which is the effect – the dependent variable.
You want to find out how blood sugar levels are affected by drinking diet cola and regular cola, so you conduct an experiment .
- The type of cola – diet or regular – is the independent variable .
- The level of blood sugar that you measure is the dependent variable – it changes depending on the type of cola.
No. The value of a dependent variable depends on an independent variable, so a variable cannot be both independent and dependent at the same time. It must be either the cause or the effect, not both.
Yes, but including more than one of either type requires multiple research questions .
For example, if you are interested in the effect of a diet on health, you can use multiple measures of health: blood sugar, blood pressure, weight, pulse, and many more. Each of these is its own dependent variable with its own research question.
You could also choose to look at the effect of exercise levels as well as diet, or even the additional effect of the two combined. Each of these is a separate independent variable .
To ensure the internal validity of an experiment , you should only change one independent variable at a time.
To ensure the internal validity of your research, you must consider the impact of confounding variables. If you fail to account for them, you might over- or underestimate the causal relationship between your independent and dependent variables , or even find a causal relationship where none exists.
A confounding variable is closely related to both the independent and dependent variables in a study. An independent variable represents the supposed cause , while the dependent variable is the supposed effect . A confounding variable is a third variable that influences both the independent and dependent variables.
Failing to account for confounding variables can cause you to wrongly estimate the relationship between your independent and dependent variables.
There are several methods you can use to decrease the impact of confounding variables on your research: restriction, matching, statistical control, and randomisation.
In restriction , you restrict your sample by only including certain subjects that have the same values of potential confounding variables.
In matching , you match each of the subjects in your treatment group with a counterpart in the comparison group. The matched subjects have the same values on any potential confounding variables, and only differ in the independent variable .
In statistical control , you include potential confounders as variables in your regression .
In randomisation , you randomly assign the treatment (or independent variable) in your study to a sufficiently large number of subjects, which allows you to control for all potential confounding variables.
In scientific research, concepts are the abstract ideas or phenomena that are being studied (e.g., educational achievement). Variables are properties or characteristics of the concept (e.g., performance at school), while indicators are ways of measuring or quantifying variables (e.g., yearly grade reports).
The process of turning abstract concepts into measurable variables and indicators is called operationalisation .
In statistics, ordinal and nominal variables are both considered categorical variables .
Even though ordinal data can sometimes be numerical, not all mathematical operations can be performed on them.
A control variable is any variable that’s held constant in a research study. It’s not a variable of interest in the study, but it’s controlled because it could influence the outcomes.
Control variables help you establish a correlational or causal relationship between variables by enhancing internal validity .
If you don’t control relevant extraneous variables , they may influence the outcomes of your study, and you may not be able to demonstrate that your results are really an effect of your independent variable .
‘Controlling for a variable’ means measuring extraneous variables and accounting for them statistically to remove their effects on other variables.
Researchers often model control variable data along with independent and dependent variable data in regression analyses and ANCOVAs . That way, you can isolate the control variable’s effects from the relationship between the variables of interest.
An extraneous variable is any variable that you’re not investigating that can potentially affect the dependent variable of your research study.
A confounding variable is a type of extraneous variable that not only affects the dependent variable, but is also related to the independent variable.
There are 4 main types of extraneous variables :
- Demand characteristics : Environmental cues that encourage participants to conform to researchers’ expectations
- Experimenter effects : Unintentional actions by researchers that influence study outcomes
- Situational variables : Eenvironmental variables that alter participants’ behaviours
- Participant variables : Any characteristic or aspect of a participant’s background that could affect study results
The difference between explanatory and response variables is simple:
- An explanatory variable is the expected cause, and it explains the results.
- A response variable is the expected effect, and it responds to other variables.
The term ‘ explanatory variable ‘ is sometimes preferred over ‘ independent variable ‘ because, in real-world contexts, independent variables are often influenced by other variables. This means they aren’t totally independent.
Multiple independent variables may also be correlated with each other, so ‘explanatory variables’ is a more appropriate term.
On graphs, the explanatory variable is conventionally placed on the x -axis, while the response variable is placed on the y -axis.
- If you have quantitative variables , use a scatterplot or a line graph.
- If your response variable is categorical, use a scatterplot or a line graph.
- If your explanatory variable is categorical, use a bar graph.
A correlation is usually tested for two variables at a time, but you can test correlations between three or more variables.
An independent variable is the variable you manipulate, control, or vary in an experimental study to explore its effects. It’s called ‘independent’ because it’s not influenced by any other variables in the study.
Independent variables are also called:
- Explanatory variables (they explain an event or outcome)
- Predictor variables (they can be used to predict the value of a dependent variable)
- Right-hand-side variables (they appear on the right-hand side of a regression equation)
A dependent variable is what changes as a result of the independent variable manipulation in experiments . It’s what you’re interested in measuring, and it ‘depends’ on your independent variable.
In statistics, dependent variables are also called:
- Response variables (they respond to a change in another variable)
- Outcome variables (they represent the outcome you want to measure)
- Left-hand-side variables (they appear on the left-hand side of a regression equation)
Deductive reasoning is commonly used in scientific research, and it’s especially associated with quantitative research .
In research, you might have come across something called the hypothetico-deductive method . It’s the scientific method of testing hypotheses to check whether your predictions are substantiated by real-world data.
Deductive reasoning is a logical approach where you progress from general ideas to specific conclusions. It’s often contrasted with inductive reasoning , where you start with specific observations and form general conclusions.
Deductive reasoning is also called deductive logic.
Inductive reasoning is a method of drawing conclusions by going from the specific to the general. It’s usually contrasted with deductive reasoning, where you proceed from general information to specific conclusions.
Inductive reasoning is also called inductive logic or bottom-up reasoning.
In inductive research , you start by making observations or gathering data. Then, you take a broad scan of your data and search for patterns. Finally, you make general conclusions that you might incorporate into theories.
Inductive reasoning is a bottom-up approach, while deductive reasoning is top-down.
Inductive reasoning takes you from the specific to the general, while in deductive reasoning, you make inferences by going from general premises to specific conclusions.
There are many different types of inductive reasoning that people use formally or informally.
Here are a few common types:
- Inductive generalisation : You use observations about a sample to come to a conclusion about the population it came from.
- Statistical generalisation: You use specific numbers about samples to make statements about populations.
- Causal reasoning: You make cause-and-effect links between different things.
- Sign reasoning: You make a conclusion about a correlational relationship between different things.
- Analogical reasoning: You make a conclusion about something based on its similarities to something else.
It’s often best to ask a variety of people to review your measurements. You can ask experts, such as other researchers, or laypeople, such as potential participants, to judge the face validity of tests.
While experts have a deep understanding of research methods , the people you’re studying can provide you with valuable insights you may have missed otherwise.
Face validity is important because it’s a simple first step to measuring the overall validity of a test or technique. It’s a relatively intuitive, quick, and easy way to start checking whether a new measure seems useful at first glance.
Good face validity means that anyone who reviews your measure says that it seems to be measuring what it’s supposed to. With poor face validity, someone reviewing your measure may be left confused about what you’re measuring and why you’re using this method.
Face validity is about whether a test appears to measure what it’s supposed to measure. This type of validity is concerned with whether a measure seems relevant and appropriate for what it’s assessing only on the surface.
Statistical analyses are often applied to test validity with data from your measures. You test convergent validity and discriminant validity with correlations to see if results from your test are positively or negatively related to those of other established tests.
You can also use regression analyses to assess whether your measure is actually predictive of outcomes that you expect it to predict theoretically. A regression analysis that supports your expectations strengthens your claim of construct validity .
When designing or evaluating a measure, construct validity helps you ensure you’re actually measuring the construct you’re interested in. If you don’t have construct validity, you may inadvertently measure unrelated or distinct constructs and lose precision in your research.
Construct validity is often considered the overarching type of measurement validity , because it covers all of the other types. You need to have face validity , content validity, and criterion validity to achieve construct validity.
Construct validity is about how well a test measures the concept it was designed to evaluate. It’s one of four types of measurement validity , which includes construct validity, face validity , and criterion validity.
There are two subtypes of construct validity.
- Convergent validity : The extent to which your measure corresponds to measures of related constructs
- Discriminant validity: The extent to which your measure is unrelated or negatively related to measures of distinct constructs
Attrition bias can skew your sample so that your final sample differs significantly from your original sample. Your sample is biased because some groups from your population are underrepresented.
With a biased final sample, you may not be able to generalise your findings to the original population that you sampled from, so your external validity is compromised.
There are seven threats to external validity : selection bias , history, experimenter effect, Hawthorne effect , testing effect, aptitude-treatment, and situation effect.
The two types of external validity are population validity (whether you can generalise to other groups of people) and ecological validity (whether you can generalise to other situations and settings).
The external validity of a study is the extent to which you can generalise your findings to different groups of people, situations, and measures.
Attrition bias is a threat to internal validity . In experiments, differential rates of attrition between treatment and control groups can skew results.
This bias can affect the relationship between your independent and dependent variables . It can make variables appear to be correlated when they are not, or vice versa.
Internal validity is the extent to which you can be confident that a cause-and-effect relationship established in a study cannot be explained by other factors.
There are eight threats to internal validity : history, maturation, instrumentation, testing, selection bias , regression to the mean, social interaction, and attrition .
A sampling error is the difference between a population parameter and a sample statistic .
A statistic refers to measures about the sample , while a parameter refers to measures about the population .
Populations are used when a research question requires data from every member of the population. This is usually only feasible when the population is small and easily accessible.
Systematic sampling is a probability sampling method where researchers select members of the population at a regular interval – for example, by selecting every 15th person on a list of the population. If the population is in a random order, this can imitate the benefits of simple random sampling .
There are three key steps in systematic sampling :
- Define and list your population , ensuring that it is not ordered in a cyclical or periodic order.
- Decide on your sample size and calculate your interval, k , by dividing your population by your target sample size.
- Choose every k th member of the population as your sample.
Yes, you can create a stratified sample using multiple characteristics, but you must ensure that every participant in your study belongs to one and only one subgroup. In this case, you multiply the numbers of subgroups for each characteristic to get the total number of groups.
For example, if you were stratifying by location with three subgroups (urban, rural, or suburban) and marital status with five subgroups (single, divorced, widowed, married, or partnered), you would have 3 × 5 = 15 subgroups.
You should use stratified sampling when your sample can be divided into mutually exclusive and exhaustive subgroups that you believe will take on different mean values for the variable that you’re studying.
Using stratified sampling will allow you to obtain more precise (with lower variance ) statistical estimates of whatever you are trying to measure.
For example, say you want to investigate how income differs based on educational attainment, but you know that this relationship can vary based on race. Using stratified sampling, you can ensure you obtain a large enough sample from each racial group, allowing you to draw more precise conclusions.
In stratified sampling , researchers divide subjects into subgroups called strata based on characteristics that they share (e.g., race, gender, educational attainment).
Once divided, each subgroup is randomly sampled using another probability sampling method .
Multistage sampling can simplify data collection when you have large, geographically spread samples, and you can obtain a probability sample without a complete sampling frame.
But multistage sampling may not lead to a representative sample, and larger samples are needed for multistage samples to achieve the statistical properties of simple random samples .
In multistage sampling , you can use probability or non-probability sampling methods.
For a probability sample, you have to probability sampling at every stage. You can mix it up by using simple random sampling , systematic sampling , or stratified sampling to select units at different stages, depending on what is applicable and relevant to your study.
Cluster sampling is a probability sampling method in which you divide a population into clusters, such as districts or schools, and then randomly select some of these clusters as your sample.
The clusters should ideally each be mini-representations of the population as a whole.
There are three types of cluster sampling : single-stage, double-stage and multi-stage clustering. In all three types, you first divide the population into clusters, then randomly select clusters for use in your sample.
- In single-stage sampling , you collect data from every unit within the selected clusters.
- In double-stage sampling , you select a random sample of units from within the clusters.
- In multi-stage sampling , you repeat the procedure of randomly sampling elements from within the clusters until you have reached a manageable sample.
Cluster sampling is more time- and cost-efficient than other probability sampling methods , particularly when it comes to large samples spread across a wide geographical area.
However, it provides less statistical certainty than other methods, such as simple random sampling , because it is difficult to ensure that your clusters properly represent the population as a whole.
If properly implemented, simple random sampling is usually the best sampling method for ensuring both internal and external validity . However, it can sometimes be impractical and expensive to implement, depending on the size of the population to be studied,
If you have a list of every member of the population and the ability to reach whichever members are selected, you can use simple random sampling.
The American Community Survey is an example of simple random sampling . In order to collect detailed data on the population of the US, the Census Bureau officials randomly select 3.5 million households per year and use a variety of methods to convince them to fill out the survey.
Simple random sampling is a type of probability sampling in which the researcher randomly selects a subset of participants from a population . Each member of the population has an equal chance of being selected. Data are then collected from as large a percentage as possible of this random subset.
Sampling bias occurs when some members of a population are systematically more likely to be selected in a sample than others.
In multistage sampling , or multistage cluster sampling, you draw a sample from a population using smaller and smaller groups at each stage.
This method is often used to collect data from a large, geographically spread group of people in national surveys, for example. You take advantage of hierarchical groupings (e.g., from county to city to neighbourhood) to create a sample that’s less expensive and time-consuming to collect data from.
In non-probability sampling , the sample is selected based on non-random criteria, and not every member of the population has a chance of being included.
Common non-probability sampling methods include convenience sampling , voluntary response sampling, purposive sampling , snowball sampling , and quota sampling .
Probability sampling means that every member of the target population has a known chance of being included in the sample.
Probability sampling methods include simple random sampling , systematic sampling , stratified sampling , and cluster sampling .
Samples are used to make inferences about populations . Samples are easier to collect data from because they are practical, cost-effective, convenient, and manageable.
While a between-subjects design has fewer threats to internal validity , it also requires more participants for high statistical power than a within-subjects design .
Advantages:
- Prevents carryover effects of learning and fatigue.
- Shorter study duration.
Disadvantages:
- Needs larger samples for high power.
- Uses more resources to recruit participants, administer sessions, cover costs, etc.
- Individual differences may be an alternative explanation for results.
In a factorial design, multiple independent variables are tested.
If you test two variables, each level of one independent variable is combined with each level of the other independent variable to create different conditions.
Yes. Between-subjects and within-subjects designs can be combined in a single study when you have two or more independent variables (a factorial design). In a mixed factorial design, one variable is altered between subjects and another is altered within subjects.
Within-subjects designs have many potential threats to internal validity , but they are also very statistically powerful .
- Only requires small samples
- Statistically powerful
- Removes the effects of individual differences on the outcomes
- Internal validity threats reduce the likelihood of establishing a direct relationship between variables
- Time-related effects, such as growth, can influence the outcomes
- Carryover effects mean that the specific order of different treatments affect the outcomes
Quasi-experimental design is most useful in situations where it would be unethical or impractical to run a true experiment .
Quasi-experiments have lower internal validity than true experiments, but they often have higher external validity as they can use real-world interventions instead of artificial laboratory settings.
In experimental research, random assignment is a way of placing participants from your sample into different groups using randomisation. With this method, every member of the sample has a known or equal chance of being placed in a control group or an experimental group.
A quasi-experiment is a type of research design that attempts to establish a cause-and-effect relationship. The main difference between this and a true experiment is that the groups are not randomly assigned.
In a between-subjects design , every participant experiences only one condition, and researchers assess group differences between participants in various conditions.
In a within-subjects design , each participant experiences all conditions, and researchers test the same participants repeatedly for differences between conditions.
The word ‘between’ means that you’re comparing different conditions between groups, while the word ‘within’ means you’re comparing different conditions within the same group.
A confounding variable , also called a confounder or confounding factor, is a third variable in a study examining a potential cause-and-effect relationship.
A confounding variable is related to both the supposed cause and the supposed effect of the study. It can be difficult to separate the true effect of the independent variable from the effect of the confounding variable.
In your research design , it’s important to identify potential confounding variables and plan how you will reduce their impact.
Triangulation can help:
- Reduce bias that comes from using a single method, theory, or investigator
- Enhance validity by approaching the same topic with different tools
- Establish credibility by giving you a complete picture of the research problem
But triangulation can also pose problems:
- It’s time-consuming and labour-intensive, often involving an interdisciplinary team.
- Your results may be inconsistent or even contradictory.
There are four main types of triangulation :
- Data triangulation : Using data from different times, spaces, and people
- Investigator triangulation : Involving multiple researchers in collecting or analysing data
- Theory triangulation : Using varying theoretical perspectives in your research
- Methodological triangulation : Using different methodologies to approach the same topic
Experimental designs are a set of procedures that you plan in order to examine the relationship between variables that interest you.
To design a successful experiment, first identify:
- A testable hypothesis
- One or more independent variables that you will manipulate
- One or more dependent variables that you will measure
When designing the experiment, first decide:
- How your variable(s) will be manipulated
- How you will control for any potential confounding or lurking variables
- How many subjects you will include
- How you will assign treatments to your subjects
Exploratory research explores the main aspects of a new or barely researched question.
Explanatory research explains the causes and effects of an already widely researched question.
An observational study could be a good fit for your research if your research question is based on things you observe. If you have ethical, logistical, or practical concerns that make an experimental design challenging, consider an observational study. Remember that in an observational study, it is critical that there be no interference or manipulation of the research subjects. Since it’s not an experiment, there are no control or treatment groups either.
These are four of the most common mixed methods designs :
- Convergent parallel: Quantitative and qualitative data are collected at the same time and analysed separately. After both analyses are complete, compare your results to draw overall conclusions.
- Embedded: Quantitative and qualitative data are collected at the same time, but within a larger quantitative or qualitative design. One type of data is secondary to the other.
- Explanatory sequential: Quantitative data is collected and analysed first, followed by qualitative data. You can use this design if you think your qualitative data will explain and contextualise your quantitative findings.
- Exploratory sequential: Qualitative data is collected and analysed first, followed by quantitative data. You can use this design if you think the quantitative data will confirm or validate your qualitative findings.
Triangulation in research means using multiple datasets, methods, theories and/or investigators to address a research question. It’s a research strategy that can help you enhance the validity and credibility of your findings.
Triangulation is mainly used in qualitative research , but it’s also commonly applied in quantitative research . Mixed methods research always uses triangulation.
Operationalisation means turning abstract conceptual ideas into measurable observations.
For example, the concept of social anxiety isn’t directly observable, but it can be operationally defined in terms of self-rating scores, behavioural avoidance of crowded places, or physical anxiety symptoms in social situations.
Before collecting data , it’s important to consider how you will operationalise the variables that you want to measure.
Hypothesis testing is a formal procedure for investigating our ideas about the world using statistics. It is used by scientists to test specific predictions, called hypotheses , by calculating how likely it is that a pattern or relationship between variables could have arisen by chance.
There are five common approaches to qualitative research :
- Grounded theory involves collecting data in order to develop new theories.
- Ethnography involves immersing yourself in a group or organisation to understand its culture.
- Narrative research involves interpreting stories to understand how people make sense of their experiences and perceptions.
- Phenomenological research involves investigating phenomena through people’s lived experiences.
- Action research links theory and practice in several cycles to drive innovative changes.
There are various approaches to qualitative data analysis , but they all share five steps in common:
- Prepare and organise your data.
- Review and explore your data.
- Develop a data coding system.
- Assign codes to the data.
- Identify recurring themes.
The specifics of each step depend on the focus of the analysis. Some common approaches include textual analysis , thematic analysis , and discourse analysis .
In mixed methods research , you use both qualitative and quantitative data collection and analysis methods to answer your research question .
Methodology refers to the overarching strategy and rationale of your research project . It involves studying the methods used in your field and the theories or principles behind them, in order to develop an approach that matches your objectives.
Methods are the specific tools and procedures you use to collect and analyse data (e.g. experiments, surveys , and statistical tests ).
In shorter scientific papers, where the aim is to report the findings of a specific study, you might simply describe what you did in a methods section .
In a longer or more complex research project, such as a thesis or dissertation , you will probably include a methodology section , where you explain your approach to answering the research questions and cite relevant sources to support your choice of methods.
The research methods you use depend on the type of data you need to answer your research question .
- If you want to measure something or test a hypothesis , use quantitative methods . If you want to explore ideas, thoughts, and meanings, use qualitative methods .
- If you want to analyse a large amount of readily available data, use secondary data. If you want data specific to your purposes with control over how they are generated, collect primary data.
- If you want to establish cause-and-effect relationships between variables , use experimental methods. If you want to understand the characteristics of a research subject, use descriptive methods.
Ask our team
Want to contact us directly? No problem. We are always here for you.
- Chat with us
- Email [email protected]
- Call +44 (0)20 3917 4242
- WhatsApp +31 20 261 6040
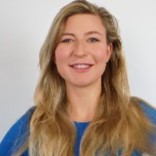
Our support team is here to help you daily via chat, WhatsApp, email, or phone between 9:00 a.m. to 11:00 p.m. CET.
Our APA experts default to APA 7 for editing and formatting. For the Citation Editing Service you are able to choose between APA 6 and 7.
Yes, if your document is longer than 20,000 words, you will get a sample of approximately 2,000 words. This sample edit gives you a first impression of the editor’s editing style and a chance to ask questions and give feedback.
How does the sample edit work?
You will receive the sample edit within 24 hours after placing your order. You then have 24 hours to let us know if you’re happy with the sample or if there’s something you would like the editor to do differently.
Read more about how the sample edit works
Yes, you can upload your document in sections.
We try our best to ensure that the same editor checks all the different sections of your document. When you upload a new file, our system recognizes you as a returning customer, and we immediately contact the editor who helped you before.
However, we cannot guarantee that the same editor will be available. Your chances are higher if
- You send us your text as soon as possible and
- You can be flexible about the deadline.
Please note that the shorter your deadline is, the lower the chance that your previous editor is not available.
If your previous editor isn’t available, then we will inform you immediately and look for another qualified editor. Fear not! Every Scribbr editor follows the Scribbr Improvement Model and will deliver high-quality work.
Yes, our editors also work during the weekends and holidays.
Because we have many editors available, we can check your document 24 hours per day and 7 days per week, all year round.
If you choose a 72 hour deadline and upload your document on a Thursday evening, you’ll have your thesis back by Sunday evening!
Yes! Our editors are all native speakers, and they have lots of experience editing texts written by ESL students. They will make sure your grammar is perfect and point out any sentences that are difficult to understand. They’ll also notice your most common mistakes, and give you personal feedback to improve your writing in English.
Every Scribbr order comes with our award-winning Proofreading & Editing service , which combines two important stages of the revision process.
For a more comprehensive edit, you can add a Structure Check or Clarity Check to your order. With these building blocks, you can customize the kind of feedback you receive.
You might be familiar with a different set of editing terms. To help you understand what you can expect at Scribbr, we created this table:
View an example
When you place an order, you can specify your field of study and we’ll match you with an editor who has familiarity with this area.
However, our editors are language specialists, not academic experts in your field. Your editor’s job is not to comment on the content of your dissertation, but to improve your language and help you express your ideas as clearly and fluently as possible.
This means that your editor will understand your text well enough to give feedback on its clarity, logic and structure, but not on the accuracy or originality of its content.
Good academic writing should be understandable to a non-expert reader, and we believe that academic editing is a discipline in itself. The research, ideas and arguments are all yours – we’re here to make sure they shine!
After your document has been edited, you will receive an email with a link to download the document.
The editor has made changes to your document using ‘Track Changes’ in Word. This means that you only have to accept or ignore the changes that are made in the text one by one.
It is also possible to accept all changes at once. However, we strongly advise you not to do so for the following reasons:
- You can learn a lot by looking at the mistakes you made.
- The editors don’t only change the text – they also place comments when sentences or sometimes even entire paragraphs are unclear. You should read through these comments and take into account your editor’s tips and suggestions.
- With a final read-through, you can make sure you’re 100% happy with your text before you submit!
You choose the turnaround time when ordering. We can return your dissertation within 24 hours , 3 days or 1 week . These timescales include weekends and holidays. As soon as you’ve paid, the deadline is set, and we guarantee to meet it! We’ll notify you by text and email when your editor has completed the job.
Very large orders might not be possible to complete in 24 hours. On average, our editors can complete around 13,000 words in a day while maintaining our high quality standards. If your order is longer than this and urgent, contact us to discuss possibilities.
Always leave yourself enough time to check through the document and accept the changes before your submission deadline.
Scribbr is specialised in editing study related documents. We check:
- Graduation projects
- Dissertations
- Admissions essays
- College essays
- Application essays
- Personal statements
- Process reports
- Reflections
- Internship reports
- Academic papers
- Research proposals
- Prospectuses
Calculate the costs
The fastest turnaround time is 24 hours.
You can upload your document at any time and choose between four deadlines:
At Scribbr, we promise to make every customer 100% happy with the service we offer. Our philosophy: Your complaint is always justified – no denial, no doubts.
Our customer support team is here to find the solution that helps you the most, whether that’s a free new edit or a refund for the service.
Yes, in the order process you can indicate your preference for American, British, or Australian English .
If you don’t choose one, your editor will follow the style of English you currently use. If your editor has any questions about this, we will contact you.
Section 1.2: Observational Studies versus Designed Experiments
- 1.1 Introduction to the Practice of Statistics
- 1.2 Observational Studies versus Designed Experiments
- 1.3 Random Sampling
- 1.4 Bias in Sampling
- 1.5 The Design of Experiments
By the end of this lesson, you will be able to...
- distinguish between an observational study and a designed experiment
- identify possible lurking variables
- explain the various types of observational studies
For a quick overview of this section, watch this short video summary:
To begin, we're going to discuss some of the ways to collect data. In general, there are a few standards:
- existing sources
- survey sampling
- designed experiments
Most of us associate the word census with the U.S. Census, but it actually has a broader definition. Here's typical definition:
A census is a list of all individuals in a population along with certain characteristics of each individual.
The nice part about a census is that it gives us all the information we want. Of course, it's usually impossible to get - imagine trying to interview every single ECC student . That'd be over 10,000 interviews!
So if we can't get a census, what do we do? A great source of data is other studies that have already been completed. If you're trying to answer a particular question, look to see if someone else has already collected data about that population. The moral of the story is this: Don't collect data that have already been collected!
Observational Studies versus Designed Experiments
Now to one of the main objectives for this section. Two other very common sources of data are observational studies and designed experiments . We're going to take some time here to describe them and distinguish between them - you'll be expected to be able to do the same in homework and on your first exam.
The easiest examples of observational studies are surveys. No attempt is made to influence anything - just ask questions and record the responses. By definition,
An observational study measures the characteristics of a population by studying individuals in a sample, but does not attempt to manipulate or influence the variables of interest.
For a good example, try visiting the Pew Research Center . Just click on any article and you'll see an example of an observational study. They just sample a particular group and ask them questions.
In contrast, designed experiments explicitly do attempt to influence results. They try to determine what affect a particular treatment has on an outcome.
A designed experiment applies a treatment to individuals (referred to as experimental units or subjects ) and attempts to isolate the effects of the treatment on a response variable .
For a nice example of a designed experiment, check out this article from National Public Radio about the effect of exercise on fitness.
So let's look at a couple examples.
Visit this link from Science Daily , from July 8th, 2008. It talks about the relationship between Post-Traumatic Stress Disorder (PTSD) and heart disease. After reading the article carefully, try to decide whether it was an observational study or a designed experiment
What was it?
This was a tricky one. It was actually an observational study . The key is that the researchers didn't force the veterans to have PTSD, they simply observed the rate of heart disease for those soldiers who have PTSD and the rate for those who do not.
Visit this link from the Gallup Organization , from June 17th, 2008. It looks at what Americans' top concerns were at that point. Read carefully and think of the how the data were collected. Do you think this was an observational study or a designed experiment? Why?
Think carefully about which you think it was, and just as important - why? When you're ready, click the link below.
If you were thinking that this was an observational study , you were right!The key here is that the individuals sampled were just asked what was important to them. The study didn't try to impose certain conditions on people for a set amount of time and see if those conditions affected their responses.
This last example is regarding the "low-carb" Atkins diet, and how it compares with other diets. Read through this summary of a report in the New England Journal of Medicine and see if you can figure out whether it's an observational study or a designed experiment.
As expected, this was a designed experiment , but do you know why? The key here is they forced individuals to maintain a certain diet, and then compared the participants' health at the end.
Probably the biggest difference between observational studies and designed experiments is the issue of association versus causation . Since observational studies don't control any variables, the results can only be associations . Because variables are controlled in a designed experiment, we can have conclusions of causation .
Look back over the three examples linked above and see if all three reported their results correctly. You'll often find articles in newspapers or online claiming one variable caused a certain response in another, when really all they had was an association from doing an observational study.
The discussion of the differences between observational studies and designed experiments may bring up an interesting question - why are we worried so much about the difference?
We already mentioned the key at the end of the previous page, but it bears repeating here:
Observational studies only allow us to claim association ,not causation .
The primary reason behind this is something called a lurking variable (sometimes also termed a confounding factor , among other similar terms).
A lurking variable is a variable that affects both of the variables of interest, but is either not known or is not acknowledged.
Consider the following example, from The Washington Post:
Coffee may have health benefits and may not pose health risks for many people
By Carolyn Butler Tuesday, December 22, 2009
Of all the relationships in my life, by far the most on-again, off-again has been with coffee: From that initial, tentative dalliance in college to a serious commitment during my first real reporting job to breaking up altogether when I got pregnant, only to fail miserably at quitting my daily latte the second time I was expecting. More recently the relationship has turned into full-blown obsession and, ironically, I often fall asleep at night dreaming of the delicious, satisfying cup of joe that awaits, come morning.
[...] Rest assured: Not only has current research shown that moderate coffee consumption isn't likely to hurt you, it may actually have significant health benefits. "Coffee is generally associated with a less health-conscious lifestyle -- people who don't sleep much, drink coffee, smoke, drink alcohol," explains Rob van Dam, an assistant professor in the departments of nutrition and epidemiology at the Harvard School of Public Health. He points out that early studies failed to account for such issues and thus found a link between drinking coffee and such conditions as heart disease and cancer, a link that has contributed to java's lingering bad rep. "But as more studies have been conducted-- larger and better studies that controlled for healthy lifestyle issues --the totality of efforts suggests that coffee is a good beverage choice."
Source: Washington Post
What is this article telling us? If you look at the parts in bold, you can see that Professor van Dam is describing a lurking variable: lifestyle. In past studies, this variable wasn't accounted for. Researchers in the past saw the relationship between coffee and heart disease, and came to the conclusion that the coffee was causing the heart disease.
But since those were only observational studies, the researchers could only claim an association . In that example, the lifestyle choices of individuals was affecting both their coffee use and other risks leading to heart disease. So "lifestyle" would be an example of a lurking variable in that example.
For more on lurking variables, check out this link from The Math Forum and this one from The Psychology Wiki . Both give further examples and illustrations.
With all the problems of lurking variables, there are many good reasons to do an observational study. For one, a designed experiment may be impractical or even unethical (imagine a designed experiment regarding the risks of smoking). Observational studies also tend to cost much less than designed experiments, and it's often possible to obtain a much larger data set than you would with a designed experiment. Still, it's always important to remember the difference in what we can claim as a result of observational studies versus designed experiments.
Types of Observational Studies
There are three major types of observational studies, and they're listed in your text: cross-sectional studies, case-control studies, and cohort studies.
Cross-sectional Studies
This first type of observational study involves collecting data about individuals at a certain point in time. A researcher concerned about the effect of working with asbestos might compare the cancer rate of those who work with asbestos versus those who do not.
Cross-sectional studies are cheap and easy to do, but they don't give very strong results. In our quick example, we can't be sure that those working with asbestos who don't report cancer won't eventually develop it. This type of study only gives a bit of the picture, so it is rarely used by itself. Researchers tend to use a cross-sectional study to first determine if their might be a link, and then later do another study (like one of the following) to further investigate.
Case-control Studies
Case-control studies are frequently used in the medical community to compare individuals with a particular characteristic (this group is the case )with individuals who do not have that characteristic (this group is the control ). Researchers attempt to select homogeneous groups, so that on average, all other characteristics of the individuals will be similar, with only the characteristic in question differing.
One of the most famous examples of this type of study is the early research on the link between smoking and lung cancer in the United Kingdom by Richard Doll and A. Bradford Hill. In the 1950's, almost 80% of adults in the UK were smokers, and the connection between smoking and lung cancer had not yet been established. Doll and Hill interviewed about 700 lung cancer patients to try to determine a possible cause.
This type of study is retrospective ,because it asks the individuals to look back and describe their habits(regarding smoking, in this case). There are clear weaknesses in a study like this, because it expects individuals to not only have an accurate memory, but also to respond honestly. (Think about a study concerning drug use and cognitive impairment.) Not only that, we discussed previously that such a study may prove association , but it cannot prove causation .
Cohort Studies
A cohort describes a group of individuals, and so a cohort study is one in which a group of individuals is selected to participate in a study. The group is then observed over a period of time to determine if particular characteristics affect a response variable.
Based on their earlier research, Doll and Hill began one of the largest cohort studies in 1951. The study was again regarding the link between smoking and lung cancer. The study began with 34,439 male British doctors, and followed them for over 50 years. Doll and Hill first reported findings in 1954 in the British Medical Journal , and then continued to report their findings periodically afterward. Their last report was in 2004,again published in the British Medical Journal . This last report reflected on 50 years of observational data from the cohort.
This last type of study is called prospective , because it begins with the group and then collects data over time. Cohort studies are definitely the most powerful of the observational studies,particularly with the quantity and quality of data in a study like the previous one.
Let's look at some examples.
A recent article in the BBC News Health section described a study concerning dementia and "mid-life ills". According to the article, researches followed more than 11,000 people over a period of 12-14 years. They found that smoking, diabetes, and high blood pressure were all factors in the onset of dementia.
What type of observational study was this? Cross-sectional, case-control,or cohort?
Because the researchers tracked the 11,000 participants, this is a cohort study .
In 1993, the National Institute of Environmental Health Sciences funded a study in Iowa regarding the possible relationship between radon levels and the incidence of cancer. The study gathered information from 413 participants who had developed lung cancer and compared those results with 614 participants who did not have lung cancer.
What type of study was this?
This study was retrospective - gathering information about the group of interest (those with cancer) and comparing them with a control group(those without cancer). This is an example of a case-control study .
Thought his may seem similar to a cross-sectional study, it differs in that the individuals are "matched" (with cancer vs. without cancer)and the individuals are expected to look back in time and describe their time spent in the home to determine their radon exposure.
In 2004, researchers published an article in the New England Journal of Medicine regarding the relationship between the mental health of soldiers exposed to combat stress. The study collected information from soldiers in four combat infantry units either before their deployment to Iraq or three to four months after their return from combat duty.
Since this was simply a survey given over a short period of time to try to examine the effect of combat duty, this was a cross-sectional study. Unlike the previous example, it did not ask the participants to delve into their history, nor did it explicitly "match" soldiers with a particular characteristic.
<< previous section | next section >>

Helping math teachers bring statistics to life

Video Resources

In this video, Luke or Lindsey takes students through a full Stats Medic EFFL lesson.
Observational Studies vs. Experiments
Learning targets.
Explain the concept of confounding and how it limits the ability to make cause-and-effect conclusions.
Distinguish between an observational study and an experiment, and identify the explanatory and response variables in each type of study.
Identify the experimental units and treatments in an experiment.
- How It Works
- PhD thesis writing
- Master thesis writing
- Bachelor thesis writing
- Dissertation writing service
- Dissertation abstract writing
- Thesis proposal writing
- Thesis editing service
- Thesis proofreading service
- Thesis formatting service
- Coursework writing service
- Research paper writing service
- Architecture thesis writing
- Computer science thesis writing
- Engineering thesis writing
- History thesis writing
- MBA thesis writing
- Nursing dissertation writing
- Psychology dissertation writing
- Sociology thesis writing
- Statistics dissertation writing
- Buy dissertation online
- Write my dissertation
- Cheap thesis
- Cheap dissertation
- Custom dissertation
- Dissertation help
- Pay for thesis
- Pay for dissertation
- Senior thesis
- Write my thesis
Experiment vs Observational Study: A Deeper Look
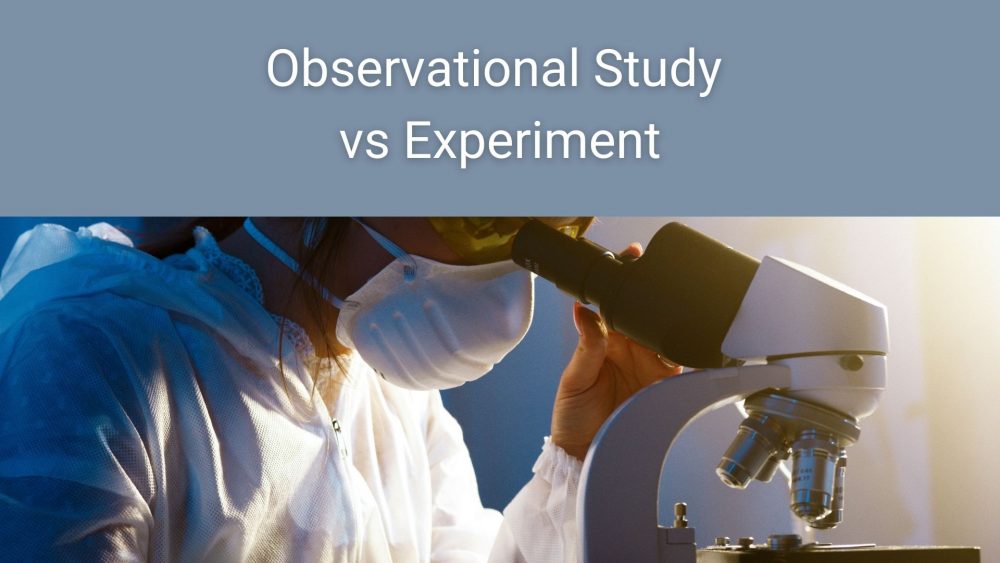
When we read about research studies and reports, many are times that we fail to pay attention to the design of the study. For you to know the quality of the research findings, it is paramount to start by understanding some basics of research/study design.
The primary goal of doing a study is to evaluate the relationship between several variables. For example, does eating fast food result in teenagers being overweight? Or does going to college increase the chances of getting a job? Most studies fall into two main categories, observational and experimental studies, but what is the difference? Other widely accepted research types are cohort studies, randomized controls, and case-control studies, but these three are part of either experimental or observational study. Keep reading to understand the difference between observational study and experiment.
What Is An Observational Study?
To understand observational study vs experiment, let us start by looking at each of them.
So, what is an observational study ? This is a form of research where the measurement is done on the selected sample without running a control experiment. Therefore, the researcher observes the impact of a specific risk factor, such as treatment or intervention, without focusing on who is not exposed. It is simply a matter of observing what is happening.
When an observational report is released, it indicates that there might be a relationship between several variables, but this cannot be relied on. It is simply too weak or biased. We will demonstrate this with an example.
A study asking people how they liked a new film that was released a few months ago is a good example of an observational study. The researcher in the study does not have any control over the participants. Therefore, even if the study point to some relationship between the main variables, it is considered too weak. For example, the study did not factor in the possibility of viewers watching other films.
The main difference between an observational study and an experiment is that the latter is randomized . Again, unlike the observational study statistics, which are considered biased and weak, evidence from experimental research is stronger.
Advantages of Observational Studies
If you are thinking of carrying a research and have been wondering whether to go for randomized experiment vs observational study, here are some key advantages of the latter.
- Because the observational study does not require the use of control, it is inexpensive to undertake. Suppose you take the example of a study looking at the impact of introducing a new learning method into a school. In that case, all you need is to ask any interested students to participate in a survey with questions, such as “yes” and “no.”
- Doing observational research can also be pretty simple because you do not have to keep looking into multiple variables, and trying to control some groups.
- Sometimes the observational method is the only way to study some things, such as exposure to specific threats. For example, it might not be ethical to expose people to harmful variables, such as radiation. However, it is possible to study the exposed population living in affected areas using observational studies.
While the advantages of observational research might appear attractive, you need to weigh them against the cons. To run conclusive observational research, you might require a lot of time. Sometimes, this might run for years or decades.
The results from observational studies are also open to a lot of criticism because of confounding biases. For example, a cohort study might conclude that most people who love to meditate regularly suffer less from heart issues. However, this alone might not be the only cause of low cases of heart problems. The people who medicate might also be following healthy diets and doing a lot of exercises to stay healthy.
Types of Observational Studies
Observational studies further branches into several categories, including cohort study, cross-sectional, and case-control. Here is a breakdown of these different types of studies:
- Cohort Study
For study purposes, a “cohort” is a team or group of people who are somehow linked. Example, people born within a specific period might be referred to as a “birth cohort.”
The concept of cohort study edges close to that of experimental research. Here, the researcher records whether every participant in the cohort is affected by the selected variables. In a medical setting, the researcher might want to know whether the cohort population in the study got exposed to a certain variable and if they developed the medical condition of interest. This is the most preferred method of study when urgent response, especially to a public health concern, such as a disease outbreak is reported.
It is important to appreciate that this is different from experimental research because the investigator simply observes but does not determine the exposure status of the participants.
- Case Control Study
In this type of study, the researcher enrolls people with a health issue and another without the problem. Then, the two groups are compared based on exposure. The control group is used to generate an estimate of the expected exposure in the population.
- Cross-Sectional Research
This is the third type of observational type of study, and it involves taking a sample from a population that is exposed to health risk and measuring them to establish the extent of the outcome. This study is very common in health settings when researchers want to know the prevalence of a health condition at any specific moment. For example, in a cross-sectional study, some of the selected persons might have lived with high blood pressure for years, while others might have started seeing the signs recently.
Experimental Studies
Now that you know the observational study definition, we will now compare it with experiment research. So, what is experimental research?
In experimental design, the researcher randomly assigns a selected part of the population some treatment to make a cause and effect conclusion. The random selection of samples is largely what makes the experiment different from the observational study design.
The researcher controls the environment, such as exposure levels, and then checks the response produced by the population. In science, the evidence generated by experimental studies is stronger and less contested compared to that produced by observational studies.
Sometimes, you might find experimental study design being referred to as a scientific study. Always remember that when using experimental studies, you need two groups, the main experiment group (part of the population exposed to a variable) and the control (another group that does not get exposed/ treatment by the researcher).
Benefits of Using Experimental Study Design
Here are the main advantages to expect for using experimental study vs observational experiment.
- Most experimental studies are shorter and smaller compared to observational studies.
- The study, especially the selected sample and control group, is monitored closely to ensure the results are accurate.
- Experimental study is the most preferred method of study when targeting uncontested results.
When using experimental studies, it is important to appreciate that it can be pretty expensive because you are essentially following two groups, the experiment sample and control. The cost also arises from the factor that you might need to control the exposure levels and closely follow the progress before drawing a conclusion.
Observational Study vs Experiment: Examples
Now that we have looked at how each design, experimental and observational, work, we will now turn to examples and identify their application.
To improve the quality of life, many people are trying to quit smoking by following different strategies, but it is true that quitting is not easy. So the methods that are used by smokers include:
- Using drugs to reduce addiction to nicotine.
- Using therapy to train smokers how to stop smoking.
- Combining therapy and drugs.
- Cold turkey (neither of the above).
The variable in the study is (I, II, III, IV), and the outcome or response is success or failure to quit the problem of smoking. If you select to use an observational method, the values of the variables (I, ii, iii, iv) would happen naturally, meaning that you would not control them. In an experimental study, values would be assigned by the researcher, implying that you would tell the participants the methods to use. Here is a demonstration:
- Observational Study: Here, you would imagine a population of people trying to quit smoking. Then, use a survey, such as online or telephone interviews, to reach the smokers trying to stop the habit. After a year later, you reach the same persons again, to enquire whether they were successful. Note that you do not run any control over the population.
- Experimental study: In this case, a representative sample of smokers trying to stop the habit is selected through a survey. Say you reach about 1000. Now, the number is divided into four groups of 250 persons, and each group is allocated one of the four methods above (i, ii, iii, or iv).
The results from the experimental study might be as shown below:
From the results of the experimental study, we can say that combining therapy and drugs method helped most smokers to quit the habit successfully. Therefore, a policy can be developed to adopt the most successful method for helping smokers quit the problem.
It is important to note that both studies commence with a random sample. The difference between an observational study and an experiment is that the sample is divided in the latter while it is not in the former. In the case of the experimental study, the researcher is controlling the main variables and then checking the relationship.
A researcher picked a random sample of learners in a class and asked them about their study habits at home. The data showed that students who used at least 30 minutes to study after school scored better grades than those who never studied at all.
This type of study can be classified as observational because the researcher simply asked the respondents about their study habits after school. Because there was no group given a particular treatment, the study cannot qualify as experimental.
In another study, the researcher randomly picked two groups of students in school to determine the effectiveness of a new study method. Group one was asked to follow the new method for a period of three months, while the other was asked to simply study the way they were used. Then, the researcher checked the scores between the two groups to determine if the new method is better.
So, is this an experimental or observational study? This type of study can be categorized as experimental because the researcher randomly picked two groups of respondents. Then, one group was given some treatment, and the other one was not.
In one of the studies, the researcher took a random sample of people and looked at their eating habits. Then, every member was classified as either healthy or at risk of developing obesity. The researcher also drew recommendations to help people at risk of developing overweight issues to avoid the problem.
This type of study is observational because the researcher took a random sample but did no accord any group a special treatment. The study simply observed the people’s eating habits and classified them.
In one of the studies done in Japan, the researcher wanted to know the levels of radioactive materials in people’s tissues after the bombing of Hiroshima and Nagasaki in 1945. Therefore, he took a random sample of 1000 people in the region and asked them to get checked to determine the levels of radiation in their tissues.
After the study, the researcher concluded that the level of radiation in people’s tissues is still very high and might be associated with different types of diseases being reported in the region. Can you determine what type of study design this is?
The research is an example observational study because it did not have any control. The researcher only observed the levels but did not have any type of control group. Again, there was no special treatment to one of the study populations.
Get Professional Help Whenever You Need It
If you are a researcher, it is very important to be able to define observational study and experiment research before commencing your work. This can help you to determine the different parameters and how to go about the study. As we have demonstrated, observational studies mainly involve gathering the findings from the field without trying to control the variables. Although this study’s results can be contested, it is the most recommended method when using other studies such as experimental design, is unfeasible or unethical.
Experimental studies giving the researcher greater control over the study population by controlling the variables. Although more expensive, it takes a relatively shorter time, and results are less biased.
Now, go ahead and design your study. Always remember that you can seek help from either your lecturer or an expert when designing the study. Once you understand the concept of observational study vs experiment well, research can become so enjoyable and fun.
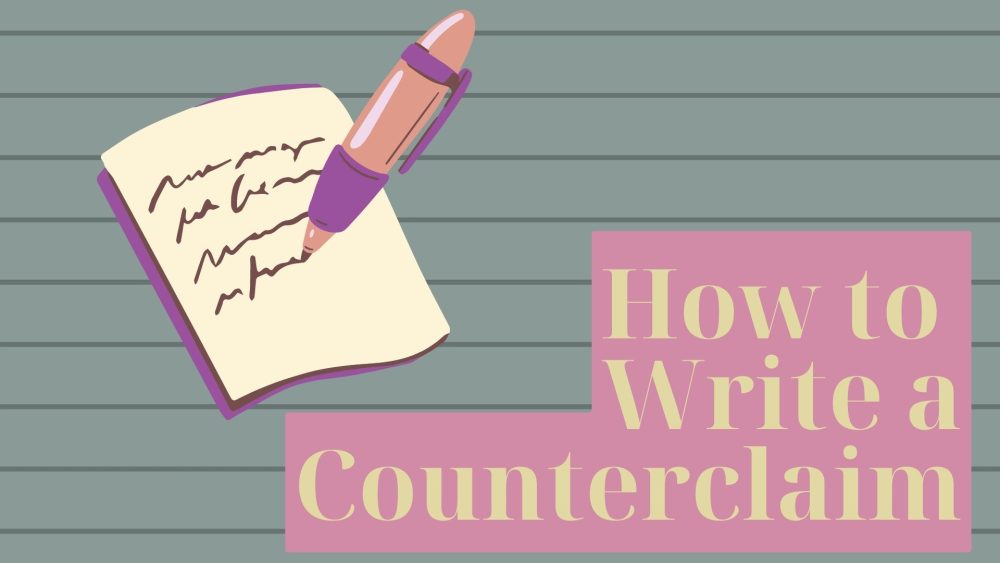
Leave a Reply Cancel reply
Your email address will not be published. Required fields are marked *
Comment * Error message
Name * Error message
Email * Error message
Save my name, email, and website in this browser for the next time I comment.
As Putin continues killing civilians, bombing kindergartens, and threatening WWIII, Ukraine fights for the world's peaceful future.
Ukraine Live Updates
- Open access
- Published: 11 November 2024
Evaluation of the causal effect of maternal education on the utilisation of maternal health services in Bangladesh using an observational study - a comparison of different propensity score methods and covariate adjustment
- Ema Akter ORCID: orcid.org/0000-0003-4772-5431 1 , 2 ,
- M. Shafiqur Rahman 2 ,
- Aniqa Tasnim Hossain 1 ,
- Abu Bakkar Siddique 1 ,
- Abu Sayeed 1 ,
- Promit Barua Chowdhury 3 ,
- Shams El Arifeen 1 ,
- Ahmed Ehsanur Rahman 1 &
- Anisuddin Ahmed 1 , 4
Archives of Public Health volume 82 , Article number: 207 ( 2024 ) Cite this article
Metrics details
Assessing maternal health care utilisation is imperative for the health of both mother and her child. Maternal education is an important determinant in subsequent maternal health care usage, according to research. There is a dearth of research on the causal relationship between maternal education and maternal health services as well as examining the performance of different propensity score methods for estimating absolute effects. Therefore, this study aims to estimate the effect of maternal education on usage of maternal health services minimising the confounding effect and to compare different propensity score approaches to estimate absolute effects of maternal education on usage of maternal health services.
We used data from a cross-sectional study conducted by icddr,b in Bangladesh. A total of 1300 recently delivered mothers were included in this study. We used different propensity score (PS) methods to estimate the causal effect of maternal education on receiving maternal health services, including PS matching, PS weighting, covariate adjustment using PS, as well as used modified log-Poisson regression with and without multiple covariates.
The study revealed highly imbalanced possible confounding factors for mother's educational level, which might lead to erroneous estimates. All methods indicated a significant effect of higher maternal education, whether measured as a continuous scale or a binary variable (secondary or higher vs. below secondary) on maternal health services, significantly increased the likelihood of receiving four or more ANC, delivered at facility, PNC within 42 days and receiving all maternal health services compared to the mothers with lower level of education. The PS weighting provided precise estimates with a low range of confidence interval.
Conclusions
The results provided important insights on how well these techniques worked to reduce effect of confounding variables and achieve precise estimates. Propensity score weighting method performed better in terms of providing more precise estimates with a narrower range of confidence intervals, indicating that this method may be a reliable approach for estimating the causal effect of maternal education on maternal health service utilisation. However, careful consideration should be given to selecting the most appropriate method.
Peer Review reports
Introduction
Globally, approximately 810 women died every day from avoidable causes associated with pregnancy and delivery in 2017 [ 1 ]. Bangladesh maternal mortality and Health Care Survey (BMMS) 2016 reported that maternal mortality ratio (MMR) is 196 per 100,000 live births [ 2 ]. Maternal deaths are caused by a variety of factors that vary by location. Maternal mortality is high as a result of low levels of maternal health care seeking behavior, particularly in developing countries [ 3 ]. Maternal health has become a global priority since maternal health care services can save the lives of millions of women of reproductive age [ 3 ]. It refers to the mother’s health throughout her pregnancy, childbirth, and postpartum period. Maternal health care services are antenatal care (ANC), delivery care and postnatal care (PNC) services. However, maternal health care utilisation among women is low [ 4 ]. For maternal survival to improve, women’s use of health services during pregnancy, childbirth, and early childhood must increase [ 5 ].
Bangladesh Demographic and Health Survey (BDHS) 2017-18 which includes estimates for the three years prior to the survey, documented that about 47%, 49%, and 52% of women who had a live birth had four or more ANC visits throughout their pregnancy, delivered at a health facility, and had postnatal care from a medically qualified provider within two days of delivery, respectively [ 4 ]. On the other hand, the most recent Sustainable Development Goals (SDGs) aimed to reduce MMR to 70 per 100,000 live births worldwide by 2030 [ 6 ].
Evidence from the previous study indicates that maternal education is a significant predictor of health care utilisation, even when controlled for the household’s socioeconomic status [ 7 , 8 ]. However, these findings are based on different observational studies. These studies include those conducted in Latin America [ 9 , 10 ], Africa [ 11 , 12 , 13 , 14 ], and Asia [ 15 , 16 , 17 , 18 ]. A randomised controlled trial (RCT) would ensure whether these associations are causal [ 19 ]. However, due to the complexity of such a design, observational studies are common in practice, where the researcher has no control over the background characteristics or the random allocation of treatments [ 20 ]. Therefore, using observational studies to assess the causal treatment effect is not feasible unless confounding factors are controlled, at least to a certain extent. Pan et al. [ 21 ] highlighted that propensity score methods are widely recognised and effective statistical tools for reducing selection bias in observational data, thereby enhancing the validity of causal inferences drawn from such studies. A study applied propensity score method in a cross-sectional study conducted by Sekine et al. [ 22 ] to evaluate causal effect of child marriage on unmet needs for modern contraception, and unintended pregnancy.
Propensity score (PS) is a very practical and widely used tool, was first proposed by Rosenbaum and Rubin [ 23 , 24 , 25 ] has been used for strengthening causal effect estimates using observational data by minimising the confounding that occurs frequently in observational studies. There are several approaches that utilise the PS for minimising confounding: matching on the PS, inverse probability of treatment weighting using the PS, covariate adjustment using the PS and stratification [ 23 ]. When the outcome is binary, the treatment’s effect can be expressed using relative measures of effect (the odds ratio and the relative risk). Moreover, maternal mortality varies considerably throughout Bangladesh, with hard-to-reach places having high rates [ 26 ]. There is a dearth of research on the causal association between maternal education and maternal health services in these areas as well as examining the performance of different propensity score methods for estimating causal effect. There is a lack of clear guidance on how to make a sensible choice between the various PS methods and conventional covariate adjustment for this type of database. A study documented that while PS methods have theoretical advantages over conventional covariate adjustment, their relative performance in real-world scenarios is poorly characterised [ 27 ]. The authors compared different PS methods and traditional covariate adjustment based on precision (standard error) using four large-scale cardiovascular observational studies [ 27 ]. Therefore, this study describes and applies the PS methods to estimate the effect of maternal education on maternal health services using observational data with mothers from hard-to-reach areas in Bangladesh and compares the results from different approaches for estimating the causal effect. It is beneficial to conduct this study as there is no study that estimates the causal relationship between maternal education and maternal health services using appropriate methods i.e., propensity score methods in case of observational study.
Study design and setting
We used data from a cross-sectional study conducted by icddr,b from October – November 2019 in Bangladesh. The study was conducted in 13 sub-districts located adjacent to 12 different hard-to-reach coastal areas of Bangladesh (Additional file 1: Appendix 1).
Study population, sampling technique and sample size
The study included mothers who delivered six months prior to the survey. The survey’s inclusion criteria were recently delivered mothers with a child aged 0 to 6 months. A simple cluster sampling procedure was implemented to select the mothers. A total of 1300 recently delivered mothers were finally interviewed.
Data collection
The data was collected through a face-to-face interview with the help of structured questionnaires. The questionnaire consisted of socio-demographic characteristics, ANC history, PNC history, delivery history. It specifically asked the information on the number of ANC visits, PNC visits, and the place of delivery. A team of field research managers and six female data collectors were trained in research ethics and questionnaire administration. Face to face interviews were conducted to collect the data.
Outcome variables
This study employed four outcome variables: receiving four or more ANC, facility-based delivery, PNC within 42 days, and receiving all these three above-mentioned maternal health services. These four variables were considered being binary variables in conducting the analysis. The first outcome variable, four or more ANC visits was recorded as a 1 for mothers who had four or more ANC visits, whereas those who had less than 4 visits were coded as 0. The place of the delivery is indicated by the second outcome variable, facility-based delivery. Respondents were asked about whether the delivery was at home or health facilities, including medical college hospital, district hospital, upazilla health complex, family welfare center, NGO clinic, private medical college hospital/clinic. The place of delivery had been grouped into two categories, home and facility-based delivery and coded as 0 for home delivery and 1 for facility-based delivery. A response to the third outcome variable, PNC within 42 days, was recorded as 1 if the respondents received PNC within 42 days, otherwise, it was coded as 0. The fourth outcome variable receiving all maternal health services (four or more ANC, facility-based delivery and PNC within 42 days) coded as 1 if the respondents received all above-mentioned maternal health services otherwise coded as 0.
In this study, we considered maternal educational level as the exposure, analysing it both as a continuous variable and as a binary categorised scale. The education system in Bangladesh has three levels: primary, secondary and higher education [ 28 ]. Aside from that, as indicated by the National Education Policy (2010), the primary education level has been extended to the eighth grade [ 29 ]. This study categorised the mother’s education level into two groups. An education level with > 8 was used to classify the individual as exposed to secondary or higher education level, coded as 1, otherwise introduced as having below the secondary level of education, coded as 0.
Several socioeconomic and demographic characteristics were considered as potential covariates in this study based on the literature review and the design of the study. These included religion (Islam and Others), husband education (> 8 and < = 8), distance to health centre in km (0–1, 2–3, and 4 or more), age at marriage in years (less than 18, 18 or more), pregnancy number (1, 2, 3, and 4 or more) and wealth quintile (poorest, poorer, middle, richer and richest). Wealth quintile was calculated based on ownership of household goods (such as housing materials, type of latrines, availability of electricity, and ownership of radio and/or television, etc.) using the principal component analysis (PCA) method.
Figure 1 presents the relationships between the variables to illustrate the hypothesised causal relationships between maternal education and the utilisation of maternal health services. We identified maternal education as exposure and utilisation of maternal health services as outcome variables, and rest of the variables as covariates as we aimed to isolate the total effect of maternal education on receiving maternal health services without any decomposition, by adjusting for all measured covariates.
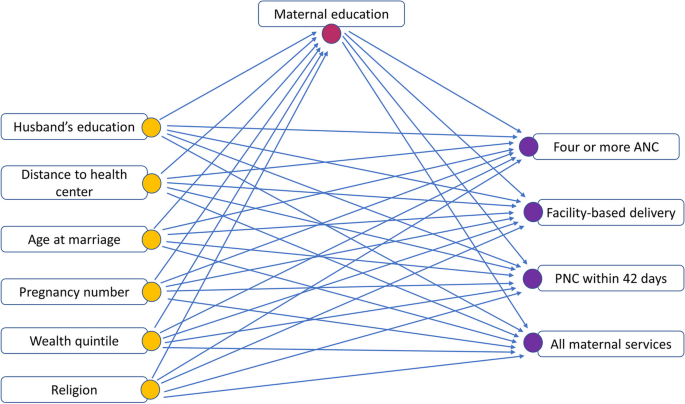
Causal diagram indicating relationship among covariates, maternal education and the utilisation of maternal health services
Statistical analysis
We used the statistical software package Stata 14 [ 30 ] and R 4.3.3 [ 31 ] to perform the analysis. Descriptive statistics, including frequency and percentage were used to illustrate the distribution of the mothers receiving maternal health services across a set of socioeconomic and demographic characteristics. To show the distribution of maternal education within the same set of characteristics, we reported frequency and percentage for categorised maternal education, and mean and standard deviation (SD) for continuous scale of maternal education. The p-value from the chi-square test in case of categorical variables and two sample t-test (covariates with two categories) or ANOVA (covariates with more than two categories) for continuous variables were reported to show the association or difference, respectively. We used the propensity score (PS) methods to reduce the confounding effect and assess the causal effect of exposure considering both binary and continuous exposure to investigate whether the effects of maternal education on receiving maternal health services (ANC, facility-based delivery and PNC) exists. We fitted modified log-Poisson regression models employing generalised estimating equations (GEE) framework to account for clustered data obtained from 13 sub-districts for our four binary outcome variables. The modified log-Poisson approach is generally preferred to estimate risk ratio (RR) in case of binary outcome variables [ 32 ]. In the context of GEE to analyse clustered outcome data, we have used an exchangeable working correlation matrix. It is natural to use such a working correlation matrix when the outcome data are measured at a single point in time and the clustering arises through some natural grouping of individuals (e.g., in different areas) [ 32 ]. We reported crude RR, adjusted RR, RR after incorporating PS and their corresponding 95% confidence interval (CI) from the modified log-Poisson regression models under GEE approach. A study conducted by Elze et al. (2017) using four observational cardiovascular studies, reported standard error (SE) on the effect (i.e., log hazrd ratio) scale, and compared which method provides precise estimates based on SE. We compared the commonly used PS methods (matching, weighting, covariate adjustment), along with unadjusted and multivariate adjusted method in terms of CI range. We considered the usual sandwich variance estimator when employing weighting approach. Absolute standardised mean differences (SMDs) before and after incorporating PS were used to assess the balance in covariates. For binary variable, SMDs are calculated as difference between two sample percentage derived from exposed and unexposed groups divided by the pooled standard deviation, \(\:{SMD}_{j}=\:\frac{{p}_{1j}-{p}_{2j}}{\sqrt{\frac{{p}_{1j}\left(1-{p}_{1j}\right)+{p}_{2j}(1-{p}_{2j})}{2}}}\) , where \(\:{p}_{1j}\:\) and \(\:{p}_{2j}\) are the weighted prevalence of covariate j in exposed and unexposed group. Absolute SMDs values of less than 0.1 are considered negligible [ 33 ].
Propensity score methods for binary exposure
Propensity score.
The probability of receiving a treatment conditional on a set of observed covariates is known as the propensity score [ 23 ]. It can be defined as, \(\:{\varvec{\pi\:}}_{\varvec{i}}=\varvec{p}({\varvec{Z}}_{\varvec{i}}=1|{\varvec{X}}_{\varvec{i}})\) ; \(\:\varvec{i}=\text{1,2},\dots\:\varvec{n}\) , where \(\:{Z}_{i}\) is the treatment indicator (exposure: maternal education, taking 1 if mothers exposed to secondary or higher level of education), \(\:{X}_{i}^{{\prime\:}}\text{s}\) (religion, husband education, distance to health centre, age at marriage, pregnancy number and wealth quintile) are the given covariates and \(\:{\pi\:}_{i}\) is the propensity scores (PS) [ 23 ]. The propensity scores ( \(\:{\pi\:}_{i}\) ) were estimated using modified log-Poisson under GEE approach, where maternal education status as the dependent variable and all other given covariates serving as covariates in the model.
PS matching
PS matching is the process of producing matched sets of exposed and unexposed subjects who have a propensity score that is similar in value to one another [ 34 ]. Pair matching (one-to-one matching) is the most common application of PS matching [ 33 ]. Only one untreated subject (below the secondary level of education) is matched to one treated subject (exposed to secondary or higher education level) who have similar estimated PS value to produce matching pairs in pair matching. As a result, this method may not utilise data from all participants. This matching can be improved by persisting that pairs cannot differ by more than a fixed value which is called caliper. We considered a caliper of width equal to 0.2 of the standard deviation of the logit of the propensity score as this caliper width has been found to perform well in a wide variety of settings [ 35 ]. We also considered two-to-one matching in our analysis to have a larger sample in PS matching analysis, where two untreated subjects are matched to one treated subject. PS matching provides an estimation of the average treatment effect among the treated (ATT) parameter [ 21 ], focusing on the mothers who received secondary or higher education.
PS weighting
This method utilise data from all participants. There are various types of weighting methods, including inverse probability of treatment weighting (IPTW), standardised mortality ratio weighted (SMRW) and stabilised weighting (SW) method. This present study samples are weighted using commonly used inverse probability of treatment (exposed to secondary or higher education level or not) weighting [ 33 , 36 ] to ensure that they are representative of specific populations [ 37 ]. The IPTW that documents estimation of the average treatment effect (ATE) parameter can be defined as, \(W_1=\frac z{\pi_i}+\frac{1-z}{1-\pi_i}\) . An alternate weight that documents estimation of the ATT can be defined as, \(W_2=Z+\left(1-Z\right)\frac{{\mathrm\pi}_i}{1-{\mathrm\pi}_i}\) (Z is the indicator of maternal education status and \({\mathrm\pi}_i\) is the estimated propensity score) [ 34 ]. These weights are then incorporated into the outcome model. Marginal computations was conducted to determine the treatment effect (maternal education) on the outcome (usage of maternal health services) [ 38 ].
PS covariate adjustment
This method utilise data from all participants. In the covariate adjustment approach, an indicator variable denoting exposure condition (exposed to secondary or higher education level or not) and the estimated PS is used to model the outcome variable (usage of maternal health services) [ 33 ]. This approach provides us with the estimation of ATE parameter [ 21 ].
Generalised propensity score methods for continuous exposure
Austin et al. (2019) [ 39 ] considers \(\:r\left(t,x\right)\) to designate the conditional density of the continuous exposure given the observed baseline covariates, using the terms \(\:r\left(t,x\right)=\:{f}_{T|X}\left(t\right|x)\) [ 40 ]. In our case, considered T as the continuous exposure, where \(\:R=\:r\left(t,x\right)\) is the generalised propensity score. It is suggested that one may set out that, the continuous exposure T given covariate X , is normally distributed with mean \(\:{\beta\:}^{T}X\) and variance \(\:{\sigma\:}^{2}\) [ 41 ]. Thus, we estimated \(\:{f}_{T|X}\) by the normal density \(\:\frac{1}{\sqrt{2\pi\:{\sigma\:}^{2}}}{e}^{-\frac{{(t-{\beta\:}^{T}X)}^{2}}{{2\sigma\:}^{2}}}\) . This is a two-step process where a regression model is first fitted to the data, and in the second step, the value of the conditional density function is determined at the value of quantitative exposure. To estimate the GPS, we fitted linear model under GEE approach where continuous maternal education is the dependent variable and baseline covariates worked as covariate in the model. However, in the context of a binary exposure, matching is a well-established approach in causal inference. Though, in the context of a continuous treatment or exposure, matching is not [ 42 ].
GPS weighting
A stabilising factor is considered in practice in GPS to discard the large weights [ 43 ]. We derived weights from the GPS and that is defined as \(\frac{W\left(T_i\right)}{r\left(T_i\left|X_i\right.\right)}\) [ 39 , 44 , 45 ], where the numerator is included to make stable the weights, final estimated stabilised IPW. A reasonable choice for W has been suggested as an estimate of the marginal density function of T [ 45 ]. This density function was determined by computing the mean and the variance of the continuous exposure in the overall sample [ 39 ], \(W\left(T_i\right)=\frac1{\sqrt{2\pi\sigma_{sample}^2}}e^{-\frac{\left(t-\mu_{sample}\right)^2}{2\sigma_{sample}^2}}\) . These weights then were applied in a weighted outcome regression in order to estimate a causal effect. This approach provides the ATE estimation parameter [ 41 ].
GPS covariate adjustment
To perform the covariate adjustment using the GPS, the outcome is regressed on the quantitative exposure and the estimated GPS [ 40 ]. This approach yields the estimated parameter for the average treatment effect (ATE) [ 41 ].
Simple and multiple regressions
For both cases (binary exposure and continuous exposure), simple and multiple modified log-Poisson regressions within GEE framework were used to estimate the effect of maternal education on maternal health services. Simple model that is unadjusted method estimates the crude association between maternal education and the utilisation of maternal health services without controlling for confounders. Multiple adjusted method performs regression model adjusting for potential confounders, shared adjusted estimate of association.
Around 33%, 47%, 41%, and 17% of mothers received four or more ANC, facility-based delivery, PNC within 42 days, and all three maternal health services, respectively (Fig. 2 ).
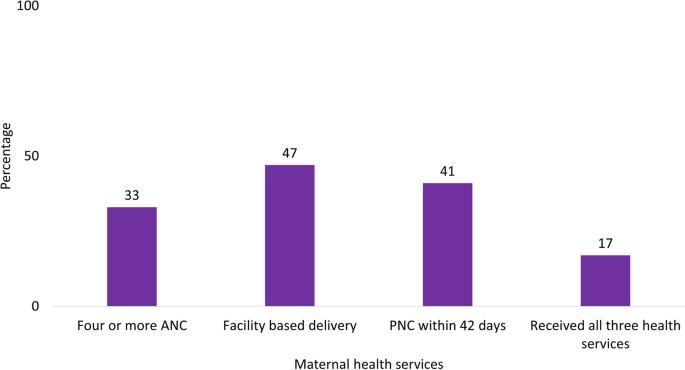
Percentage of mothers receiving maternal health services
Maternal education, wealth index, religion, husband education, and pregnancy number were associated with attending maternal health services (four or more ANC, facility-based delivery, PNC within 42 days, and receiving all health services) (Table 1 ). Around 49%, 64%, 58%, and 31% of the mothers with secondary or higher levels of education attended four or more ANC, facility-based delivery, PNC within 42 days, and received all health services, respectively. About 28% of the mothers from the richest group received all health services as compared to about 12% of the mothers from the poorest group. A small proportion of mothers (11%) received all maternal health services whose husband’s education was below secondary level. Considering the distance to the health care centres, 38% of the mothers attended four or more ANC and about half of the mothers had facility-based delivery as well as PNC within 42 days when the distance was between 0 and 1 km. Around 22% of the mothers whose age was 18 or more at marriage received all health services as compared to around 14% of the mothers whose age was below 18 at marriage. About 20% of the mothers with their first pregnancy received all health services as compared to about 12% of the mothers with four or more pregnancies.
Maternal education was significantly associated with various socioeconomic and demographic characteristics including wealth index, religion, husband education, age at marriage and pregnancy number (Table 2 ). We considered both binary and continuous scale of maternal education. In the poorest group, 24% of mothers had secondary or higher education, while in the richest group, this figure was 41%. Among mothers practicing Islam, 27% had attained secondary education or higher, while among mothers of other religions, this percentage was 51%. For mothers whose husbands had an education level of 8 years or less, 16% had secondary or higher education, compared to 66% when husbands had more than 8 years of education. Among mothers married at less than 18 years of age, average grade level is close to 6, in contrast to, for those married at 18 years or older, it is close to 8th grade. For mothers with their first pregnancy, 43% had secondary or higher education, compared to 9% for those with four or more pregnancies.
All methods including multivariable adjusted, PS-covariate adjustment, PS-weighting, and PS-matching indicate a significant effect of maternal education (employing both binary and continuous exposure) on maternal health services where mothers exposed to secondary or higher levels of education were more likely to have four or more ANC, delivered at the facility, PNC within 42 days as well as receiving all maternal health services compared to the mothers who have below secondary level of education., Alternatively, a one-unit increase in educational grade leads to significant improvements in receiving maternal health services (Table 3 ). The effect estimates resulting from different methods to control for confounding were different. In case of binary exposure, considering the maternal health service named four or more ANC, all methods resulted in an estimated risk ratio less than the unadjusted risk ratio of 1.74 (95% CI: 1.48–2.05). The PS weighting (ATE and ATT) yielded the smallest estimated range of CI (ATE CI: 1.12–1.56 and ATT CI: 1.06–1.49). In the case of facility-based delivery, PNC within 42 days and receiving all maternal health services, the PS weighting (ATE and ATT) provided the smallest estimated range of CI. Regarding continuous exposure, estimates and the range of its corresponding CI were lower than those observed with binary exposure. In this case, PS-weighting also provided the smallest estimated range of CI.
The Love plot [ 46 ] was used to visualise the absolute standardised mean difference (SMDs) in education level before and after PS weighting for assessing covariate balance (Fig. 3 ). A lower absolute value denotes a more balanced state. As can be observed, for unadjusted observational data, educational level exposure varies by covariates (wealth index, religion, husband education, distance to health centre, age at marriage and pregnancy number). After PS weighting adjustment, the majority of adjusted SMDs fall inside the 0.1 thresholds (dash-line), indicating substantial improvement in covariate balance across the exposure status (mothers’ educational level), resulting the satisfactory balance (Additional file 1: Appendix 2).
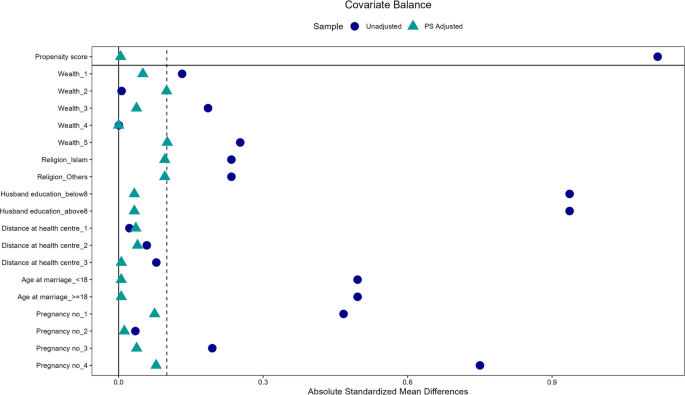
Absolute standardised mean differences for educational level before and after PS weighting
The findings of this study revealed that maternal education is related to maternal health services in Bangladesh. By analysing maternal education as both a binary (secondary or higher vs. below secondary) and a continuous variable, we consistently found that higher educational levels were associated with increased use of maternal health services. After adjusting the PS, all methods including PS-covariate adjustment, PS-weighting, and PS-matching showed that mothers with a secondary or higher level of education had a higher likelihood of receiving health services (four or more ANC, delivered at the facility, PNC within 42 days as well as receiving all maternal health services) compared to the mothers with below secondary level of education. Similarly, when education was treated as a continuous variable, a higher educational level was consistently associated with an increased likelihood of receiving these maternal health services.
Under the causal inference concept, this study provides a rigorous estimation of the causal contribution of maternal education on the utilisation of maternal health services. The study’s findings showed that educational attainment is an important determinant for the proper use of maternal health services, women with secondary or higher education having a higher number of ANC visits, facility-based delivery, and PNC within 42 days. A low percentage (17%) of mothers received all three maternal health services. Around 30% of mothers with secondary or higher levels of education received all three maternal health services, whereas only around 10% of mothers below the secondary level of education received all three maternal health services. According to a study conducted in Bangladesh, the educational level of women has a significant impact on the optimal adoption of ANC services [ 47 ]. Studies in Bangladesh and elsewhere have revealed a strong association between the education of women and their utilisation of health facility delivery and other maternal health care [ 48 , 49 , 50 ]. Another study conducted in Bangladesh by Mosiur et al. [ 51 ] reported that mothers with a higher level of education have a higher likelihood of receiving PNC.
Education may have an impact on female reproductive health by causing late marriage, deferred childbearing, a lower fertility rate, increased independence in reproductive health decisions, the freedom to choose when and where to seek medical assistance without approval from anybody, and economic empowerment [ 52 ]. Regardless of the fact that education is linked to personal and family income levels, it is also related to the use of prenatal and natal services when income and wealth family index are controlled, as they were in this study. Similar findings have been found in Nigeria [ 53 , 54 ], Kenya [ 55 , 56 ], Netherlands [ 57 ] as well as several more countries [ 58 , 59 ]. In addition, several studies, have found that illiterates had the highest risk of maternal and newborn illness, mortality, and disability, as well as the lowest utilisation of maternity services [ 56 , 57 , 59 , 60 ].
In this study, we conducted an in-depth assessment of many of the available PS and covariate-adjustment approaches. Different techniques of estimation were shown to have varying estimates of the associated risk ratios in the study. In case of binary exposure, of the different propensity score methods examined, matching method matched sets of treated (exposed to secondary or higher level of education) and untreated (below secondary level of education) subjects who have a propensity score that is similar in value to one another. The number of untreated subjects in this study is many times greater than the number of treated subjects, PS matching usually resulted in all or nearly all treated patients being successfully matched, while many untreated patients remained unmatched and be excluded from the analysis (which may lead to slightly reduced efficiency). PS-covariate adjustment showed similar results like multivariable adjusted in estimating effect of maternal education on receiving maternal health services. Moreover, the standard deviation of the estimates was higher than that obtained by PS-weighting approaches. We examined two different IPTW approaches for assessing absolute effects of maternal education on usage of maternal health services. PS weighting (ATE and ATT) provides narrower range of CI among all the methods to estimate effect of maternal education on maternal health services. The weighted analysis provides the theoretical advantage of utilising data from all samples of the study and is not affected by additional uncontrolled confounding due to the inability to locate an exact match for each treatment subject [ 61 ]. A study conducted by Jiaqi et al. (2016) [ 62 ] performed simulation studies and reported that when PS is accurately modeled, indicate that weighting produces unbiased estimates.
Binary categorisation of education can lead to information loss, resulting in less precise estimates with larger standard errors. Conversely, treating education as a continuous variable preserves information, leading to more precise estimates with smaller standard errors. While binary models are simpler and easier to interpret, they may oversimplify the exposure-outcome relationship. Nevertheless, PS-weighting performed better in terms of precision in both approaches. In summary, much of the difference between the adjustment procedures arose. It may vary depending on the different settings of different types of study. However, in the evaluation and interpretation of the influence of maternal education on receiving maternal health care services in case of using observational study, the weighted estimates are preferred.
What insights can we have from these experiences to make recommendations for the future applications of propensity score methods and covariate adjustment? As with any study, the main analysis strategy should be defined in advance. A useful approach is to review the baseline covariates before accessing outcome data to identify the most suitable PS method or covariate adjustment, considering factors like the overlap in PS between treatment and control groups. Relying on a single method (which may have its flaws) might be too limiting, so it is wise to predefine several analyses using alternative approaches. This helps assess whether findings on the estimated treatment effect are consistent, thereby enhancing confidence in the primary results.
Strengths and limitations
We believe this is the first study conducted in Bangladesh, providing a rigorous estimation of the causal contribution of maternal education on maternal health services. The use of propensity score approaches is one way of reducing bias in observational studies. PS approaches may be more suited in situations when the event is infrequent (compared to the number of observed baseline variables) and the sample size is insufficient to take into account the multiple covariates [ 63 ]. However, in this study, the analysis was carried with a relatively large sample size, which was considered to be a strength. Another key strength of this study is its comprehensive evaluation of several propensity score methods (PS matching, PS weighting, and covariate adjustment) and covariate-adjustment approach. Additionally, we considered maternal education as both a binary and continuous exposure, which allowed us to capture more nuanced effects of educational attainment. The consistent findings across these methods and exposure measures enhance the robustness of our conclusions regarding the impact of maternal education on maternal health services. From the density plot of PS, we observed that there is a common support region where the propensity scores of both groups overlapped as well as small SMDs values after PS adjustment indicating balancing of the covariates (Additional file 1: Appendices 2–3). We assumed that all relevant confounders were measured and included in the propensity score model. We observed that mothers with higher education levels were more likely to receive four or more ANC visits, opt for facility delivery, and obtain PNC services within 42 days. These observed outcomes reflect the potential outcomes if those mothers indeed had those education levels. This assumption was supported by the alignment of our findings with previous studies [ 47 , 48 , 49 , 50 , 51 ], suggesting that the observed relationships between maternal education and health service utilisation are valid and consistent with the causal effect of education.
Despite our efforts to adjust for measured confounders, unmeasured confounding remains a possibility. PS approaches do not account for unknown variables that might influence whether or not subjects receive treatment i.e., being exposed to secondary or higher level of education (this is why a randomised controlled trial is desirable) [ 63 ]. As a result, there may still be unmeasured confounding, which should be considered as a limitation. In addition, to ensure that our findings can be applied to other communities, research in other parts of Bangladesh are required because the study participants were from riverine regions. Therefore, our findings need to be evaluated with caution, and more studies need to be conducted to validate findings.
Conclusion and recommendations
Educational status was found to be significantly associated with ANC, delivery and PNC services. The findings provided important insights into the efficiency of various PS approaches for reducing the influence of confounding variables and achieving accurate estimates. All methods indicated a significant causal effect of maternal education on the usage of maternal health services. However, the propensity score weighting method demonstrated better results in terms of providing more precise estimates with a narrower range of confidence intervals. This indicates that this method may be a robust approach for estimating the causal effect that maternal education has on the utilisation of maternal health services. PS weighting can be employed to evaluate the causal influence in a scenario discussed in this study. These findings may be utilised by policymakers and healthcare practitioners for the purpose of establishing targeted initiatives that are aimed at increasing maternal education, particularly in places that are difficult to reach. An education campaign for women is required; regular knowledge-enhancing seminars for pregnant mothers may play an important role in raising awareness of the need for having access to maternal health care facilities. For future positive health outcomes, government efforts should be targeted to improve women’s education at least to the secondary level.
Data availability
Summary statistic of the data analysed in this work are all provided in the main manuscript document and its supplements.
World Health Organization (WHO). Maternal mortality:fact-sheets. 2019. Available from: https://wwww.hoint/news-room/fact-sheets/detail/maternal-mortality .
National Institute of Population Research and Training (NIPORT), International Centre for Diarrhoeal Disease Research, Bangladesh (icddr,b), and MEASURE Evaluation. Bangladesh Maternal Mortality and Health Care Survey 2016: Final Report. Dhaka, Bangladesh, and Chapel Hill, NC, USA: NIPORT, icddr,b, and MEASURE Evaluation. 2019. https://niport.portal.gov.bd/sites/default/files/files/niport.portal.gov.bd/miscellaneous_info/4c534fbe_dc9c_491e_89d7_1f85ab298e93/2020-08-23-18-28-35970449b61ba2439986d9e68dfd83b4.pdf .
Kifle D, Azale T, Gelaw YA, et al. Maternal health care service seeking behaviors and associated factors among women in rural Haramaya District, Eastern Ethiopia: a triangulated community-based cross-sectional study. Reprod Health. 2017;14:1–11.
Article Google Scholar
National Institute of Population Research and Training (NIPORT), and ICF. 2020. Bangladesh Demographic and Health Survey 2017-18. Dhaka, Bangladesh, and Rockville, Maryland, USA: NIPORT and ICF. https://dhsprogram.com/pubs/pdf/FR344/FR344.pdf .
Greenaway ES, Leon J, Baker DP. Understanding the association between maternal education and use of health services in Ghana: exploring the role of health knowledge. J Biosoc Sci. 2012;44:733–47.
Article PubMed PubMed Central Google Scholar
United Nations. Sustainable Development Goals. https://unstats.un.org/sdgs/metadata/?Text=&Goal=3&Target .
Cleland JG, Van Ginneken JK. Maternal education and child survival in developing countries: the search for pathways of influence. Soc Sci Med. 1988;27:1357–68.
Article PubMed CAS Google Scholar
Raghupathy S. Education and the use of maternal health care in Thailand. Soc Sci Med. 1996;43:459–71.
Bicego GT and Boerma J. Maternal education, use of health services, and child survival: An analysis of data from the Bolivia DHS Survey. Columbia: Institute for Resource Development/Macro Systems, Inc.; 1990. https://dhsprogram.com/pubs/pdf/wp1/wp1.pdf .
Elo IT. Utilization of maternal health-care services in Peru: the role of women’s education. Health Transition Rev. 1992;2:49–69.
Fosu GB. Access to health care in urban areas of developing societies. J Health Soc Behav 1989;30:398–411.
Caldwell JC. Education as a factor in mortality decline an examination of Nigerian data. Popul Stud 1979;33:395–413.
Farah A-A, Preston SH. Child mortality differentials in Sudan. Popul Dev Rev 1982;8:365–83.
Fosu GB. Maternal influences on preventive health behavior in children. Int Q Community Health Educ. 1991;12:1–19.
Caldwell JC. Routes to low mortality in poor countries. Popul Dev Rev. 1986;36:171–220.
Caldwell JC. Cultural and social factors influencing mortality levels in developing countries. Annals Am Acad Political Soc Sci. 1990;510:44–59.
Caldwell JC, Reddy PH, Caldwell P. The social component of mortality decline: an investigation in South India employing alternative methodologies. Popul Stud. 1983;37:185–205.
Article CAS Google Scholar
Sushama P. N. Social context of health behaviour in Kerala. In What We Know About Health Transition; The Cultural, Social and Behavioural Determinants of Health (Edited by Caldwell J. et al.). Canberra: The Australian National University Press; 1989. p. 77.
Coffman DL, Balantekin KN, Savage JS. Using propensity score methods to assess causal effects of mothers’ dieting behavior on daughters’ early dieting behavior. Child Obes. 2016;12:334–40.
Talukder A, Akter N, Sazzad Mallick T. Exploring association between individuals’ stature and type 2 diabetes status: propensity score analysis. Environ Health Insights. 2019;13:1178630219836975.
Pan W, Bai H. Propensity score methods for causal inference: an overview. Behaviormetrika. 2018;45:317–34.
Sekine K, Carandang RR, Ong KIC, et al. Identifying the causal effect of child marriage on unmet needs for modern contraception and unintended pregnancy in Nepal: a cross-sectional study using propensity score matching. BMJ open. 2021;11: e043532.
Rosenbaum PR, Rubin DB. The central role of the propensity score in observational studies for causal effects. Biometrika. 1983;70:41–55.
Rosenbaum PR, Rubin DB. Reducing bias in observational studies using subclassification on the propensity score. J Am Stat Assoc. 1984;79:516–24.
Rosenbaum PR, Rubin DB. Constructing a control group using multivariate matched sampling methods that incorporate the propensity score. Am Stat. 1985;39:33–8.
Biswas A, Halim A, Rahman F, et al. Factors associated with maternal deaths in a hard-to-reach marginalized rural community of Bangladesh: a cross-sectional study. Int J Environ Res Public Health. 2020;17: 1184.
Elze MC, Gregson J, Baber U, et al. Comparison of propensity score methods and covariate adjustment: evaluation in 4 cardiovascular studies. J Am Coll Cardiol. 2017;69:345–57.
Article PubMed Google Scholar
Rahman AE, Ameen S, Hossain AT, et al. Success and time implications of SpO2 measurement through pulse oximetry among hospitalised children in rural Bangladesh: variability by various device-, provider-and patient-related factors. J Global Health. 2022;12:12.
National Education Policy. 2010. Available from: https://www.reliefwebint/report/bangladesh/national-education-policy-2010-enbn .
StataCorp. Stata statistical software: release 14. College Station: StataCorp LP; 2015.
Google Scholar
R Core Team. (2024). R: a language and environment for statistical computing. Vienna, Austria: R Foundation for Statistical Computing. https://www.R-project.org/ .
Gallis JA, Turner EL. Relative measures of association for binary outcomes: challenges and recommendations for the global health researcher. Ann Global Health. 2019;85:137.
Austin PC. An introduction to propensity score methods for reducing the effects of confounding in observational studies. Multivar Behav Res. 2011;46:399–424.
Austin PC, Schuster T. The performance of different propensity score methods for estimating absolute effects of treatments on survival outcomes: a simulation study. Stat Methods Med Res. 2016;25:2214–37.
Austin PC. Optimal caliper widths for propensity-score matching when estimating differences in means and differences in proportions in observational studies. Pharm Stat. 2011;10:150–61.
Brookhart MA, Wyss R, Layton JB, et al. Propensity score methods for confounding control in nonexperimental research. Circulation: Cardiovasc Qual Outcomes. 2013;6:604–11.
Morgan SL, Todd JJ. 6. A diagnostic routine for the detection of consequential heterogeneity of Causal effects. Sociol Methodol. 2008;38:231–82.
Austin PC, Stuart EA. Estimating the effect of treatment on binary outcomes using full matching on the propensity score. Stat Methods Med Res. 2017;26:2505–25.
Austin PC. Assessing the performance of the generalized propensity score for estimating the effect of quantitative or continuous exposures on binary outcomes. Stat Med. 2018;37:1874–94.
Hirano K, Imbens G. The propensity score with continuous treatments. Applied bayesian modeling and causal inference from incomplete-data perspectives. John Wiley & Sons, Ltd; 2004.
Austin PC. Assessing covariate balance when using the generalized propensity score with quantitative or continuous exposures. Stat Methods Med Res. 2019;28:1365–77.
Wu X, Mealli F, Kioumourtzoglou M-A, et al. Matching on generalized propensity scores with continuous exposures. J Am Stat Assoc. 2024;119:757–72.
Brown DW, Greene TJ, Swartz MD, et al. Propensity score stratification methods for continuous treatments. Stat Med. 2021;40:1189–203.
Imbens GW. The role of the propensity score in estimating dose-response functions. Biometrika. 2000;87:706–10.
Zhang Z, Zhou J, Cao W, et al. Causal inference with a quantitative exposure. Stat Methods Med Res. 2016;25:315–35.
Ahmed A, Husain A, Love TE, et al. Heart failure, chronic diuretic use, and increase in mortality and hospitalization: an observational study using propensity score methods. Eur Heart J. 2006;27:1431–9.
Islam MM, Masud MS. Determinants of frequency and contents of antenatal care visits in Bangladesh: assessing the extent of compliance with the WHO recommendations. PLoS ONE. 2018;13: e0204752.
Colombara DV, Hernández B, Schaefer A, et al. Institutional delivery and satisfaction among indigenous and poor women in Guatemala, Mexico, and Panama. PLoS ONE. 2016;11: e0154388.
Kamal SM, Hassan CH, Alam GM. Determinants of institutional delivery among women in Bangladesh. Asia Pac J Public Health. 2015;27:NP1372-1388.
Tekelab T, Yadecha B, Melka AS. Antenatal care and women’s decision making power as determinants of institutional delivery in rural area of Western Ethiopia. BMC Res Notes. 2015;8:1–8.
Mosiur Rahman M, Haque SE, Sarwar Zahan M. Factors affecting the utilisation of postpartum care among young mothers in Bangladesh. Health Soc Care Commun. 2011;19:138–47.
Umar AS. Does female education explain the disparity in the use of antenatal and natal services in Nigeria? Evidence from demographic and health survey data. Afr Health Sci. 2017;17:391–9.
Fawole A, Shah A, Fabanwo A, et al. Predictors of maternal mortality in institutional deliveries in Nigeria. Afr Health Sci. 2012;12:32–40.
PubMed PubMed Central CAS Google Scholar
Ononokpono DN, Odimegwu CO. Determinants of maternal health care utilization in Nigeria: a multilevel approach. Pan Afr Med J. 2014;17:17.
Desai M, Phillips-Howard PA, Odhiambo FO, et al. An analysis of pregnancy-related mortality in the KEMRI/CDC health and demographic surveillance system in western Kenya. PLoS ONE. 2013;8: e68733.
Article PubMed PubMed Central CAS Google Scholar
Yego F, D’este C, Byles J, et al. Risk factors for maternal mortality in a Tertiary Hospital in Kenya: a case control study. BMC Pregnancy Childbirth. 2014;14:1–9.
Ory BE, Van Poppel FW. Trends and risk factors of maternal mortality in late-nineteenth-century Netherlands. History Family. 2013;18:481–509.
Ahmed S, Creanga AA, Gillespie DG, et al. Economic status, education and empowerment: implications for maternal health service utilization in developing countries. PLoS ONE. 2010;5:e11190.
Wang, Wenjuan, Soumya Alva, Shanxiao Wang, and Alfredo Fort. Levels and Trends in the Use of Maternal Health Services in Developing Countries. DHS Comparative Reports No. 26. Calverton: ICF Macro; 2011. https://dhsprogram.com/pubs/pdf/CR26/CR26.pdf .
Folaranmi OO. Women empowerment as a determinant of investments in children in selected rural communities in Nigeria. Afr Res Rev. 2013;7:138–61.
Rosenbaum PR, Rubin DB. The bias due to incomplete matching. Biometrics. 1985;41:103–16.
Li J, Handorf E, Bekelman J, et al. Propensity score and doubly robust methods for estimating the effect of treatment on censored cost. Stat Med. 2016;35:1985–99.
Barnieh L, James MT, Zhang J, et al. Propensity score methods and their application in nephrology research. J Nephrol. 2011;24:256–62.
Download references
Acknowledgements
This research study was funded by the Department of Foreign Affairs, Trade and Development (DFATD), through Advancing Sexual and Reproduction Health and Rights (AdSEARCH), Grant number: SGDE-EDRMS-#9926532, Purchase Order 7428855, Project P007358. icddr,b acknowledges with gratitude the commitment of Grand Challenges Canada to its research efforts. icddr,b is also grateful to the Governments of Bangladesh, Canada, Sweden and the UK for providing core/unrestricted support. We want to humbly pay our gratitude to the mothers from hard-to-reach areas, that we have included in our paper. The authors would like to express their sincere gratitude to StatReader training sessions organized by MCHD, icddr,b, as the insights and skills acquired during these sessions were instrumental in the design and execution of this research.
This research study was funded by the Department of Foreign Affairs, Trade and Development (DFATD).
Author information
Authors and affiliations.
Maternal and Child Health Division, International Centre for Diarrhoeal Disease Research, Bangladesh (icddr,b), Dhaka, Bangladesh
Ema Akter, Aniqa Tasnim Hossain, Abu Bakkar Siddique, Abu Sayeed, Shams El Arifeen, Ahmed Ehsanur Rahman & Anisuddin Ahmed
Institute of Statistical Research and Training (ISRT), University of Dhaka, Dhaka, Bangladesh
Ema Akter & M. Shafiqur Rahman
Health Science and Population Studies Division, International Centre for Diarrhoeal Disease Research, Bangladesh (icddr,b), Dhaka, Bangladesh
Promit Barua Chowdhury
Department of Women’s and Children’s Health, Uppsala University, Uppsala, Sweden
Anisuddin Ahmed
You can also search for this author in PubMed Google Scholar
Contributions
EA as first author and AA as senior author conceptualised, developed, incorporated feedback from all authors, and finalised the manuscript. MSR, ATH, ABS and PBC assisted EA in data analysis and interpretation. MSR, AS, SEA, and AER reviewed the first draft and provided their input. All authors read and approved the final manuscript.
Corresponding authors
Correspondence to Ema Akter or Anisuddin Ahmed .
Ethics declarations
Ethics approval and consent to participate.
Ethical approval for this study was obtained from the institutional review board (IRB) of International Centre for Diarrhoeal Disease Research, Bangladesh (icddr,b). Participants were informed about the study’s goal, benefits, and risks. Everyone who took part in the study did so of their own free will and received a data protection declaration in line with the Helsinki Declaration. Prior to conducting the interview, written informed consent was obtained from participants.
Consent for publication
Not applicable.
Competing interests
The authors declare no competing interests.
Additional information
Publisher’s note.
Springer Nature remains neutral with regard to jurisdictional claims in published maps and institutional affiliations.
Supplementary Information
Supplementary material 1., rights and permissions.
Open Access This article is licensed under a Creative Commons Attribution-NonCommercial-NoDerivatives 4.0 International License, which permits any non-commercial use, sharing, distribution and reproduction in any medium or format, as long as you give appropriate credit to the original author(s) and the source, provide a link to the Creative Commons licence, and indicate if you modified the licensed material. You do not have permission under this licence to share adapted material derived from this article or parts of it. The images or other third party material in this article are included in the article’s Creative Commons licence, unless indicated otherwise in a credit line to the material. If material is not included in the article’s Creative Commons licence and your intended use is not permitted by statutory regulation or exceeds the permitted use, you will need to obtain permission directly from the copyright holder. To view a copy of this licence, visit http://creativecommons.org/licenses/by-nc-nd/4.0/ .
Reprints and permissions
About this article
Cite this article.
Akter, E., Rahman, M.S., Hossain, A.T. et al. Evaluation of the causal effect of maternal education on the utilisation of maternal health services in Bangladesh using an observational study - a comparison of different propensity score methods and covariate adjustment. Arch Public Health 82 , 207 (2024). https://doi.org/10.1186/s13690-024-01423-0
Download citation
Received : 23 July 2023
Accepted : 13 October 2024
Published : 11 November 2024
DOI : https://doi.org/10.1186/s13690-024-01423-0
Share this article
Anyone you share the following link with will be able to read this content:
Sorry, a shareable link is not currently available for this article.
Provided by the Springer Nature SharedIt content-sharing initiative
- Maternal education
- Health services
- Observational study
- Confounding
Archives of Public Health
ISSN: 2049-3258
- General enquiries: [email protected]
Log in using your username and password
- Search More Search for this keyword Advanced search
- Latest content
- Current issue
- BMJ Journals More You are viewing from: Google Indexer
You are here
- Online First
- Dynamics of the gut microbiome in individuals at risk of rheumatoid arthritis: a cross-sectional and longitudinal observational study
- Article Text
- Article info
- Citation Tools
- Rapid Responses
- Article metrics

- http://orcid.org/0000-0002-3640-853X Christopher M Rooney 1 ,
- Ian B Jeffery 2 ,
- http://orcid.org/0000-0002-7945-6582 Kulveer Mankia 3 , 4 ,
- Mark H Wilcox 1 ,
- http://orcid.org/0000-0002-7429-8482 Paul Emery 3 , 4
- 1 Leeds Institute of Medical Research , University of Leeds , Leeds , UK
- 2 4D Pharma PLC , Cork , Ireland
- 3 University of Leeds, Leeds Institute of Rheumatic and Musculoskeletal Medicine , Leeds , UK
- 4 NIHR Leeds Musculoskeletal Biomedical Research Centre , Leeds , UK
- Correspondence to Dr Christopher M Rooney; c.rooney{at}leeds.ac.uk
Objectives This work aimed to resolve the conflicting reports on Prevotellaceae abundance in the development of rheumatoid arthritis (RA) and to observe structural, functional and temporal changes in the gut microbiome in RA progressors versus non-progressors.
Methods Individuals at risk of RA were defined by the presence of anticyclic citrullinated protein (anti-CCP) antibodies and new musculoskeletal symptoms without clinical synovitis. Baseline sampling included 124 participants (30 progressed to RA), with longitudinal sampling of 19 participants (5 progressed to RA) over 15 months at five timepoints. Gut microbiome taxonomic alterations were investigated using 16S rRNA amplicon sequencing and confirmed with shotgun metagenomic DNA sequencing on 49 samples.
Results At baseline, CCP+ at risk progressors showed significant differences in Prevotellaceae abundance compared with non-progressors, contingent on intrinsic RA risk factors and time to progression. Longitudinal sampling revealed gut microbiome instability in progressors 10 months before RA onset, a phenomenon absent in non-progressors. This may indicate a late microbial shift before RA onset, with Prevotellaceae contributing but not dominating these changes. Structural changes in the gut microbiome during arthritis development were associated with increased amino acid metabolism.
Conclusion These data suggest conflicting reports on Prevotellaceae overabundance are likely due to sampling within a heterogeneous population along a dynamic disease spectrum, with certain Prevotellaceae strains/clades possibly contributing to the establishment and/or progression of RA. Gut microbiome changes in RA may appear at the transition to clinical arthritis as a late manifestation, and it remains unclear whether they represent a primary or secondary phenomenon.
- Rheumatoid Arthritis
- Anti-Citrullinated Protein Antibodies
- Risk Factors
Data availability statement
Raw sequencing data available upon reasonable request from the corresponding author.
This is an open access article distributed in accordance with the Creative Commons Attribution 4.0 Unported (CC BY 4.0) license, which permits others to copy, redistribute, remix, transform and build upon this work for any purpose, provided the original work is properly cited, a link to the licence is given, and indication of whether changes were made. See: https://creativecommons.org/licenses/by/4.0/ .
https://doi.org/10.1136/ard-2024-226362
Statistics from Altmetric.com
Request permissions.
If you wish to reuse any or all of this article please use the link below which will take you to the Copyright Clearance Center’s RightsLink service. You will be able to get a quick price and instant permission to reuse the content in many different ways.
WHAT IS ALREADY KNOWN ON THIS TOPIC
Individuals with rheumatoid arthritis (RA) and those at risk possess distinct gut microbiomes when compared with healthy controls; however, detailed insights into the temporal and compositional changes in the gut microbiome as individuals progress to RA are lacking.
Prevotellaceae, and particularly Prevotella copri , have been inconsistently associated with RA development, and while early studies showed an overabundance more recent cohorts that question these observations have emerged.
WHAT THIS STUDY ADDS
We identified a phenomenon where specific strains/clades of Prevotellaceae are enriched, while others are depleted, depending on an individual’s risk profile and time to arthritis progression.
Anticyclic citrullinated protein antibody-positive individuals at risk of RA are characterised by a low-diversity gut microbiome that may become more unstable in the 10 months preceding the transition from preclinical RA to established disease (ie, the development of clinical arthritis).
This instability is marked by accumulation of multiple bacteria, including but not limited to Prevotellaceae.
HOW THIS STUDY MIGHT AFFECT RESEARCH, PRACTICE OR POLICY
These findings illustrate the dynamic nature of the gut microbiome, reflecting an individual’s underlying risk profile and transition from being at risk to developing clinical arthritis.
This evolving understanding could significantly impact approaches to RA prediction, prevention and personalised treatment strategies, emphasising the need for further research into microbiome-based diagnostics and therapeutics.
Introduction
Rheumatoid arthritis (RA) is a chronic autoimmune disorder affecting over half a million individuals in the UK. The hallmark of RA is progressive joint disease, with potential for systemic involvement. Understanding the RA disease spectrum with recognition of at risk individuals has propelled RA research into prevention strategies. The generation of IgA class anticitrullinated protein antibodies (ACPAs) in individuals at risk of RA, 1 combined with epidemiological links with smoking 2 and periodontal disease, 3 points to a mucosal origin of inflammation. The mucosal origin hypothesis proposes that localised inflammation at mucosal sites can initiate a broader immune response, 1 via T cell activation and a subsequent inflammatory cytokine cascade, leading to B cell antibody production. Supporting this, an immunoglobulin class switch from IgA ACPAs to IgG ACPAs 4 5 indicates potential triggering of systemic autoimmunity by diverse antigenic stimuli at mucosal sites. This shift, accompanied with broadening of antibody targets, 6 7 suggests that mucosal barrier deterioration and the ensuing spread of an IgG ACPA response might be more significant in the initial stages of RA than the loss of tolerance to self-antigens.
Profiling of the gut microbiome in individuals at risk of RA and people diagnosed with RA consistently demonstrates a dysbiotic microbiome when compared with healthy controls. 8–21 However, there remains little consensus on the bacterial constituent members of an RA-related dysbiosis. Subsequently, a variety of gut bacteria have been implicated as a potential impetus in the development of RA, none more so than Prevotella copri. Prevotella species have been demonstrated to be overabundant in new-onset rheumatoid arthritis (NORA), 9 11 22 in at risk individuals 8 and especially those with genetic risk. 10 Their abundance decreases after disease-modifying antirheumatic drug (DMARD) therapy, with reversion to a eubiotic state on treatment. 22 Furthermore, mouse models support a role for Prevotellaceae strains derived from patients with RA in RA development. 23 However, Prevotellaceae overabundance does not appear to be an ubiquitous finding across all RA gut microbiome studies, 16–21 including our own previous work. 24 Additionally, researchers have failed to replicate their findings of Prevotellaceae overabundance in larger cohorts. 25 Increasingly, numerous other bacteria have also been associated with RA development, including Subdoligranulum , 26 Lactobacillus , 11 20 Bacteroides species 14 17 and Streptococcaceae , 20 21 among others.
EULAR, the European Alliance of Associations for Rheumatology, defines at risk populations for RA based on a combination of genetic, environmental and immunological factors that predispose individuals to RA. 27 Therefore, heterogeneous RA at risk populations and confounding within microbiome studies further complicate the RA gut microbiome debate. This work examines a comprehensively phenotyped cohort of anticyclic citrullinated protein-positive (anti-CCP+) at risk individuals without clinical arthritis. We combined cross-sectional and longitudinal investigations of the gut microbiome with extensive clinical data, adjusting for potential confounding, to uncover microbial associations in the early RA continuum, with particular attention to Prevotellaceae strains.
Patient and public involvement statement
Before starting this research, patients contributed to the study’s design and feasibility, influencing recruitment, sample return and dissemination of outputs through a patient-focused discussion group at Chapel Allerton Hospital (CAH), Leeds. During the study, participant feedback on the stool collection kit was gathered and led to its redesign.
Study design and participants
This study used both cross-sectional and longitudinal approaches, involving participants from three groups: CCP+ at risk (n=124), newly diagnosed RA (NORA, n=7) and healthy controls (n=22). The study aimed to differentiate gut microbiome bacterial shifts due to confounding factors from those associated with RA progression, using comprehensive phenotypic data and serial stool samples as CCP+ at risk individuals progressed towards arthritis development.
The research was conducted at CAH, Leeds, UK. Individuals at risk of RA were selected from the Leeds CCP+ at risk cohort, as previously outlined. 28 These participants were recruited nationally from both primary and secondary care settings, presenting with recent musculoskeletal pain (onset <3 months) and positive for anti-CCP antibodies (CCP2 BioPlex 2200 Kit >2.99 IU/mL), but with no evidence of clinical synovitis/arthritis.
Within the CCP research clinic at CAH, individuals undergo longitudinal assessment for RA progression. An individual’s risk of arthritis development was calculated using a validated RA risk severity score, based on symptoms, joint ultrasound, human leucocyte antigen (HLA) status and antibody titre, 28 enabling comparison of gut microbiomes across varying RA risk. Comprehensive data on demographics, medical history, medications, diet, bowel habit and lifestyle were also collected ( figure 1 ).
- Download figure
- Open in new tab
- Download powerpoint
Study design and methodological flow chart. The figure depicts the recruitment of healthy controls, CCP+ at risk individuals and those with NORA, with corresponding criteria and subsequent sequential steps from sampling through molecular profiling and data analysis. Incorporates stool and blood analyses, sequencing methods and clinical assessments, culminating in the evaluation of microbial composition and its correlation with RA progression. CCP, cyclic citrullinated protein; csDMARDs, conventional synthetic disease-modifying antirheumatic drugs; GI, gastrointestinal; HLA, human leucocyte antigen; IA, inflammatory arthritis; IBD, inflammatory bowel disease; Log2 FC; Log2 fold change; MSK, musculoskeletal; NORA, new-onset rheumatoid arthritis; RA, rheumatoid arthritis.
As a comparison, individuals with NORA were recruited from general rheumatology clinics at CAH. Those diagnosed with RA and meeting the EULAR criteria, irrespective of CCP antibody status, were invited to participate in the cross-sectional study as participants with NORA. These individuals provided a single stool sample within 48 hours of their RA diagnosis, prior to starting DMARD therapy. Local healthy controls, free of musculoskeletal symptoms and representing diverse socioeconomic backgrounds, were also included, providing a single stool sample for the cross-sectional study. These controls were approximately matched with the CCP+ at risk cohort in terms of age, gender and smoking status.
Assessments
CCP+ at risk individuals underwent a baseline assessment, followed by quarterly follow-up appointments for the first year, with annual visits thereafter. The study endpoint was the development of clinical arthritis. A research helpline enabled prompt reporting of symptom changes for timely clinical evaluation. At baseline, demographic, medical, drug, social, stool and dietary histories 29 were collected. Clinical assessments at each visit included symptom review and joint assessment (78-joint count for tenderness and a 44-joint count for swelling).
Blood samples were collected at each visit for antibody testing, with the BioPlex 2200 machine used for anti-CCP2 testing and nephelometry for rheumatoid factor (RF). During the course of the study, a small number (n=11) of low titre CCP+ individuals transitioned to a negative CCP+ titre at the time of gut microbiome sampling. High-resolution joint ultrasonography using power Doppler (PD) was performed at baseline, 6 monthly for the first year, then annually thereafter and intermittently on symptom exacerbation. PD assessment included scanning of 38 joints bilaterally (metacarpophalangeal, proximal interphalangeal and metatarsophalangeal joints). HLA-DR (Human Leukocyte Antigen-DR isotype) typing occurred during the baseline visit. T cell subpopulations were quantified using flow cytometry, adjusting counts for age. T cell counts were taken at baseline, 6 months and then annually.
Participants were given in-house stool collection kits for home sample collection, returning the kit at their subsequent clinic visit. Samples were kept at room temperature at home before being frozen at −80°C for long-term storage.
Molecular and bioinformatic methods
DNA was extracted from stool samples using the QIAamp DNA Stool Mini Kit (Qiagen, Germany). The University of Leeds’ Next Generation Sequencing Facility at St James’s University Hospital campus conducted sequencing for both 16S rRNA amplicon and shotgun metagenomic analysis. For 16S rRNA sequencing, the V4 region was amplified with specific primers and sequenced using Illumina MiSeq 2500, generating 2×250 base pair (bp) paired-end reads. Data were analysed using the DADA2 method 30 and SILVA reference database 31 within the QIIME2 32 framework.
Shotgun metagenomic libraries were prepared with the Nextera XT DNA Library Prep Kit and sequenced on Illumina NextSeq 2000 using a 2×150 bp paired-end approach. The bioBakery workflow 33 was used for taxonomic and functional analysis of the shotgun sequencing data (see online supplemental materials , pages 1–3 for additional details).
Supplemental material
All samples underwent 16S rRNA amplicon sequencing. In addition, 49 baseline samples, including CCP+ at risk individuals (n=27), individuals with NORA (n=7) and healthy controls (n=15), also underwent shotgun sequencing. This dual approach was employed to validate the findings from the differential analysis of the 16S data, particularly to confirm the observed trends in Prevotella abundance and to explore any inferred functional differences arising from altered microbiome composition.
Cross-sectional
In both sequencing methodologies, analyses were conducted in R (V.3.6.2). 34 Diversity was calculated using phyloseq 35 and vegan 36 packages. For the 16S approach, a rooted phylogenetic tree was generated in QIIME2, 32 with alpha and beta diversity metrics calculated and Benjamini-Hochberg (BH)-adjusted p values (<0.05) determined via pairwise Wilcoxon rank-sum tests.
Permutational multivariate analysis of variance (MANOVA) was performed to assess the impact of metadata variables on beta diversity using three ecological metrics: Bray-Curtis, logged Bray-Curtis (Bray-Curtis was applied to scalar normalised counts (SNC) and logged data via the formula log 10 (SNC+10 −6 )+6) and unweighted UniFrac. Logged Bray-Curtis was used as an attempt to curtail outlying samples which might artificially amplify a signal within the Bray-Curtis index. Significant variables (p<0.05) were ranked by effect size and incorporated into a multivariate model to calculate cumulative variance explained.
For the shotgun method, a phyloseq object 35 was constructed using the enzyme commission number data set, and principal component analysis was performed to assess functional diversity of the microbiome. MaAsLin2 37 was used for functional pathway analysis on prenormalised MetaCyc 38 annotated pathway abundance files, using an false discovery rate (FDR) of <0.05 as a significance threshold.
Differential bacterial abundance between clinically relevant groups was investigated across both sequencing methods using DESeq2, 39 adjusted for age and sequencing run. BLAST analyses supported further taxonomic annotation of significant Prevotellaceae strains. Differential abundance testing was performed at the genus level to determine higher taxonomic changes comparable to previous studies and at the strain level data to infer potential strain/subspecies information. In both methodologies, ggplot2 40 facilitated data visualisation. Only taxa reaching a BH-adjusted p value of <0.05 were labelled. Prevotella strains of interest were identified based on Prevotella strains that reached a p value of <0.05 in the DESeq2 models from the cross-sectional study. The Prevotella strains of interest from the DESeq2 models and their associations were plotted using a chord plot.
Longitudinal
Longitudinal gut microbiome changes were analysed using 16S rRNA amplicon sequencing. Pairwise Bray-Curtis dissimilarities were constructed, and comparisons were limited to within-participant timepoints. These pairwise timepoints were categorised based on the time elapsed from RA diagnosis for progressors or from baseline for non-progressors. A logged Bray-Curtis distance matrix was used to construct a hierarchical tree using the ‘dendrogram’ and ‘hclust’ functions, applying the ‘ward.D2’ clustering algorithm. Mixed-effects linear models were created using the MaAsLin2 37 package. Participants were assigned as the random-effect source in all models. To explore bacterial taxa, a centred log-ratio transformed data set was used at both the genus and strain levels.
The cross-sectional study encompassed 153 participants; their characteristics are detailed in table 1 . In the RA at risk cohort, 70% were female, with a median anti-CCP antibody titre of 73 IU/mL. During the study period, 30 individuals progressed to RA, with a median interval of 9.6 months between stool collection and RA progression. The clinical attributes of progressors, non-progressors and NORA cohorts are outlined in table 2 . Microbiome summary statistics are available in the online supplemental materials , page 4-5.
- View inline
Characteristics of the cross-sectional study participants
Baseline clinical characteristics of the cross-sectional musculoskeletal cohorts
CCP+ at risk individuals are characterised by a low diversity state, which is associated with CCP antibody titre, RF positivity and HLA shared epitope status
Shannon diversity was notably reduced among CCP+ at risk individuals compared with healthy controls (Wilcoxon, p=0.012; figure 2A ). Diversity was reduced in both progressors and non-progressors, and appeared stratified by anti-CCP titre levels ( figure 2A ). Conversely, individuals with low or negative anti-CCP titres maintained diversity levels comparable to healthy controls. Both RF and HLA shared epitope (SE) positivity, known risk factors for arthritis development, were significantly linked to diminished diversity, as was steroid use (see online supplemental material , page 6). When examining the effects of these variables collectively in a multivariate model, none reached statistical significance (see online supplemental materials , page 6), suggesting that the individual contributions of these factors are less clear when considering the combined influence of all variables. Alpha diversity for measured lifestyle factors is presented in the online supplemental materials , page 6. Linear regression exploring variable effects on alpha diversity is presented in online supplemental materials , page 6-8.
Gut microbiome diversity, variance and differential abundance from 16S rRNA amplicon sequencing. (A) Cross-sectional alpha diversity plots. Boxplots of alpha diversity as measured using observed and Shannon diversity metrics. BH-adjusted p values generated by pairwise Wilcoxon rank-sum test. (B) Microbial variance plots. Univariate analysis of significant (p<0.05) metadata variables using permutational ANOVA on three measures of beta diversity: Bray-Curtis, logged Bray-Curtis and unweighted UniFrac. Variables ordered by decreasing effect size. Total variance ring plot demonstrating the total known and unknown variance present within the gut microbiome. Individual multivariate permutational ANOVA, adjusted for significant variables identified in the univariate models, with significance reached in at least two ecological distances. Variables ordered by effect size. (C) Differentially abundant taxa between CCP+ at risk, healthy controls and NORA and between progressors and non-progressors. Pairwise volcano plots of plotting log2 fold change against −log10 p value, coloured according to significance. Vertical line denotes adjusted p value threshold; horizontal line denotes 0.5 log2 fold change. The shape denotes PVT or NPVT taxa. Taxonomic labels were generated to give the lowest taxonomic rank available in SILVA. *Denotes ASV2058. +Denotes ASV1867. (B) Outcome refers to progressor, non-progressor, NORA and healthy individuals. Run refers to sequencing run. ANOVA, analysis of variance; BH, Benjamini-Hochberg; CCP, cyclic citrullinated protein; CRP, C reactive protein; HLA, human leucocyte antigen; NORA, new-onset rheumatoid arthritis; NPVT, not Prevotellaceae; PVT, Prevotellaceae; RA, rheumatoid arthritis; RF, rheumatoid factor; ZFP, zonulin family peptide.
Beta diversity analysis with respect to RA outcome status indicated no association between progression and enterotype (see online supplemental materials , page 9). Participants clustered according to dominant genera, primarily differentiating between Prevotella -dominated and Bacteroides -dominated microbiota. Progressors displayed both Prevotella and non- Prevotella predominant gut microbiome profiles. The analysis of 49 metadata variables using permutational analysis of variance ( online supplemental materials , page 10-13) identified 15 variables with statistical significance (p<0.05) in at least one ecological metric ( figure 2B ), and subsequent multivariate models revealed sequencing run, age and vegetable intake as consistently significant factors across all three ecological distances, explaining microbial variance ranging from 8% to 10% ( figure 2B ). Alpha and beta diversity was calculated using 16S rRNA amplicon sequencing.
Progression from the at risk phase to arthritis development is associated with strain-specific changes in Prevotellaceae
A specific Prevotellaceae strain, ASV2058 (likely P. copri ; BLAST analysis can be found in online supplemental materials , page 30-31), was enriched in the at risk population compared with healthy controls. This same strain (ASV2058) was also found to be overabundant in individuals with NORA compared with healthy controls, with no significant difference in this putative P. copri strain between at risk and NORA (16S rRNA amplicon sequencing; see figure 2C ).
Models were constructed to investigate a baseline RA progression signature within the CCP+ at risk cohort by comparing progressors with non-progressors. Strain-level analysis demonstrates concordance with genus-level results (see online supplemental materials, page 14). Strain-specific phenomena are noted with both enrichment (three strains) and depletion (five strains) of Prevotellaceae-specific strains associated with progression (16S rRNA amplicon sequencing; see figure 2C ). ASV2058 is included as one of those five depleted strains.
A phenomenon of strain-specific enrichment and concomitant depletion of Prevotellaceae strains was identified across multiple factors associated with higher risk of arthritis development, including anti-CCP antibody titre, RF positivity, HLA SE positivity, early immune dysregulation (T-naïve cells) and RA risk score (see online supplemental materials , page 15-19). All Prevotellaceae reaching nominal significance are detailed in online supplemental materials , page 20-29. Figure 3 provides a summary of Prevotellaceae strains found to be increased or decreased within the RA at risk population. Table 3 shows the proportion of each group containing Prevotellaceae and the proportion of Prevotellaceae strains of interest per group. From figure 3 , Prevotellaceae strains ASV2058 and ASV1867 both had eight connections. RA risk category showed the highest number of associations with Prevotellaceae. Among all Prevotellaceae, putative P. copri strains had the most associations with RA clinical variables; however, other strains were also implicated, such as Alloprevotella , Paraprevotella clara , Prevotella stercorea , Prevotellamassilia timonensis and Prevotella shahii .
Prevotellaceae chord plot. Associations of Prevotellaceae strains reaching nominal significance from adjusted DESeq2 models from 16S rRNA amplicon sequencing. Metadata variables are depicted in grey and bacterial strains designated by colour. Chord links are coloured according to increased abundance (red) or decreased abundance (blue). The width of each chord is scaled according to the log2 fold changes in abundance. CCP, cyclic citrullinated protein; HLA, human leucocyte antigen; NORA, new-onset rheumatoid arthritis; RA, rheumatoid arthritis; RF, rheumatoid factor; V, versus.
Proportion of Prevotellaceae presence
Within the shotgun metagenomic data set, we assessed for the presence of the P. copri transposon described by Nii et al 41 but found no significant association with CCP positivity, disease progression or HLA status ( online supplemental materials , page 50-51). However, we observed a higher number of transposon matches in individuals with specific Prevotella strains.
The shotgun sequencing data set comprising 27 CCP+ at risk (17 progressors), 7 NORA and 15 healthy controls corroborated an increase in Prevotellaceae species among at risk individuals relative to healthy controls (online online supplemental materials , page 32-33) and strain-specific changes of Prevotellaceae associated with clinical variables (see online supplemental materials , page 35-39).
In CCP+ at risk individuals, gut microbiome alterations can begin 10 months before arthritis development
Due to the observed decrease in alpha diversity preceding RA onset (see online supplemental materials , page 44), pairwise dissimilarities between timepoints were assessed to examine gut microbiome stability using 16S rRNA amplicon sequencing. These dissimilarities were categorised into intervals related to arthritis development (ie, progression), either retrospective for progressors or prospective from baseline for non-progressors, and presented in figure 4A . Time to progression spanned 0–15 months preceding arthritis onset; the time for non-progressor from baseline to final follow-up was at least 12 months.
Temporal microbial analysis of CCP+ at risk individuals. (A) Pairwise Bray-Curtis dissimilarity boxplots from 16S rRNA amplicon sequencing. Boxplot of pairwise Bray-Curtis dissimilarities grouped according to time from progression: 0–10 months (n=4) and 11–15 months (n=3); or time from baseline for non-progressors: 0–4 months (n=14), 4–8 months (n=6) and 8–12 months (n=6) of follow-up. (B) Circulated dendrogram of longitudinal samples. Constructed using logged Bray-Curtis dissimilarity index and clustered using Ward D2 methodology. Samples coloured according to outcome: non-progressors (green), progressors (orange) and progression (red). (C) Mixed-effects linear modelling with bacterial profiles. Linear model of top 50 strains with significant associations in heatmap, plotted with coloured according to coefficient; p values are indicated as follows: *p<0.25, **p<0.05, ***p<0.01, ****p<0.001. Progression denotes sampling at diagnosis of RA. Progressor denotes sampling prior to progression. All plots constructed using 16S RNA amplicon sequencing. CCP, cyclic citrullinated protein; RA, rheumatoid arthritis.
CCP+ progressors (ie, those who developed arthritis) exhibited the most significant instability (p=0.044, BH-adjusted t-test), with median Bray-Curtis dissimilarities exceeding 0.6 in the period immediately leading up to arthritis onset. This pattern indicates that gut microbiome structural changes commence around 10 months before clinical arthritis development and may extend beyond diagnosis. In contrast, the period from 10 to 15 months prior to RA onset and among non-progressors demonstrated relative stability, with lower Bray-Curtis dissimilarities suggesting more consistent microbiome structures over time and less variability between samples. Clinical, serological and radiological findings from the longitudinal participants are provided in the online supplemental materials , page 40-43.
Hierarchical clustering demonstrates modest microbial divergence prior to RA onset
To determine if samples at the progression timepoint were structurally more similar to each other or to their respective preceding samples (16S rRNA amplicon sequencing), a hierarchical tree was constructed. The resulting circular dendrogram showed higher structural similarity within individual successive samples than between different individuals. However, a notable divergence was observed between progression samples and their immediate predecessors, indicated by increased branch lengths in the dendrogram, such as seen with participant 8 and participant 3 ( figure 4B ). This was further explored using a multidimensional scaling analysis ( online supplemental materials , page 44-45), which corroborates dispersion of the coordinates prior to RA diagnosis.
The gut microbiome of individuals progressing to arthritis accumulates pathobionts over time
Mixed-effects linear models on 16S rRNA amplicon sequencing data were used to analyse temporal bacterial fluctuations in the gut microbiome as some individuals progressed to arthritis. Each participant was defined as the random effect to accommodate correlations across multiple timepoints. The models analysed data at both the genus and strain levels ( figure 4C ).
At the genus-level analysis, six taxa were associated with samples collected at the time of RA diagnosis, termed progression ( figure 4C ), while three taxa were associated to samples preceding RA onset, termed progressors. Significantly, two taxa from the Lachnospiraceae family showed consistent depletion in both progression and progressors samples ( figure 4C ). Strain-level analysis corroborated these findings, demonstrating a decrease in ASV3244, a specific Lachnospiraceae strain, in both progressors and at progression. In contrast, certain bacterial strains were found to increase in the gut microbiome of RA progressors, especially at the progression point ( figure 4C ). Detailed outputs are enumerated in the online supplemental materials , page 46-49. Notably, ASV1026, identified as Prevotella and enriched in the progression samples, was identified as P. copri through BLAST analysis.
NORA gut microbiome is associated with enhanced amino acid and energy metabolism
MaAsLin linear modelling (FDR q value <0.25) using shotgun metagenomic sequencing identified differential abundance in 18 MetaCyc pathways when comparing at risk individuals (n=27), individuals with NORA (n=7) and healthy controls (n=15), with healthy controls serving as the reference group for all comparisons. Eleven pathways exhibited elevation in the NORA gut microbiome, while six showed reduction in healthy controls, as presented in figure 5A . There were no differentially significant pathways identified in CCP+ at risk group when compared with NORA or healthy controls. The MaAsLin analysis also identified key microbial features, including MetaCyc pathways, enzymes and gene families ( figure 5B ), that differ between individuals in various CCP categories (very high, high, low) when compared with healthy controls ( online supplemental materials , pages 50-51). Notably, some enzymes, such as cobalt precorrin-5B (C.1) methyltransferase, were significantly associated with more than one CCP category (see figure 5B ). Functional analysis of HLA, RF and RA risk category did not yield significant results.
Functional analysis of enzymes, gene families and MetaCyc pathways from shotgun metagenomic sequencing. (A) Heatmap of MetaCyc pathways according to group. Heatmap represents MetaCyc pathways differentially abundant in NORA and healthy controls. (B) Heatmap of enzyme commission numbers, gene families and MetaCyc pathways of anti-CCP categories. Asterisks indicate the level of statistical significance: *p<0.25, **p<0.05, ***p<0.01. The colour gradient represents the coefficient values. Constructed using shotgun metagenomic data. CCP, cyclic citrullinated protein; NORA, new-onset rheumatoid arthritis.
This study presents a detailed examination of the gut microbiome’s alterations before the onset of RA, revealing community-wide changes that extend beyond key organisms, both confirming and expanding on current literature. The work presented here represents, to the best of our knowledge, the largest, most homogenous RA at risk cohort, combining unique longitudinal and microbial variance analyses. Our study provides several novel insights, including decreased alpha diversity in the at risk phase, confirmation of Prevotella overabundance in at risk individuals and that Prevotella abundance appears to be associated with the underlying risk profile of the individual, which is consistent despite adjustment for common microbiome confounders. Finally, we propose a potential timeline for when gut microbiome changes occur as individuals develop RA.
Notably, we identified significantly decreased alpha diversity in CCP+ at risk individuals compared with healthy controls ( figure 2A ). This was previously unidentified in three similar studies. 8 24 25 These differences between our previous work and this study could be explained by considerable different cohort size (25 vs 124 CCP+ at risk, respectively). Additionally, our initial study relied on older operational taxonomic unit (OTU) clustering, which is less sensitive than newer denoising methodologies. Both Alpizar-Rodriguez et al 8 and Gilbert et al 25 have previously investigated the at risk phase of RA but there are considerable differences between the RA at risk populations included in these studies. RA risk can be identified through a combination of factors, including antibody status, symptoms and genetics. The cohort examined by Alpizar-Rodriguez et al 8 was characterised by positivity for anti-CCP and/or RF, along with the presence of symptoms. These symptoms were either assessed through a disease screening questionnaire or identified via a diagnosis of undifferentiated arthritis (UA). This approach results in a more diverse at risk population, potentially more advanced in the RA disease continuum due to the inclusion of individuals with UA. Furthermore, it also relied on older OTU analysis. Gilbert et al 25 used the same population; however, risk was subdivided into four categories, with varying risks including genetics, autoantibody presence and/or symptoms, again representing a heterogeneous population.
Interestingly, we showed alpha diversity was stratified according to anti-CCP antibody level ( figure 2A ), where individuals with high and very high anti-CCP levels had significantly lower alpha diversity, as defined by both observed and Shannon diversity index. In contrast, Gilbert et al 25 did not identify reduced alpha diversity in those at risk of RA. However, within the symptomatic and asymptomatic autoimmune groups, 55% and 70%, respectively, were anti-CCP antibody-negative, reflecting a very different population from our study. While eligibility for the Leeds at risk cohort has been defined through anti-CCP antibody positivity, longitudinal follow-up has revealed a small number of individuals (n=11) transition from low titre positive (anti-CCP2 >2.99 IU/mL and <9 IU/mL) to a negative anti-CCP antibody status. Low titre individuals and those converting to negative titre show preserved alpha diversity, similar to healthy controls ( figure 2A ), which corresponds with the findings of Gilbert et al . 25 Comparison of the NORA group with healthy controls did not show significantly decreased alpha diversity, and this may be reflective of the small numbers within this cohort and the heterogeneity in terms of antibody positivity (NORA 73% anti-CCP antibody-positive, 100% RF-positive). However, this result is in keeping with previous work, where others have failed to identify decreased alpha diversity, as measured by Shannon diversity index in NORA compared with healthy controls. 9 11 It is worth noting that we also observed decreased Shannon diversity with steroid use. However, given steroid use is pronounced in the symptomatic group (which tends to have higher CCP titres, HLA and RF positivity), it is impossible to know what portion of change is disease-related versus drug-related.
To understand the factors influencing microbial variability, a permutational MANOVA was conducted. This analysis identified age, vegetable intake and sequencing run as potential confounders (refer to figure 2B ). Age stratification, ranging from 22 to 80 years in this cohort, aligns with existing literature 42–44 as a critical factor in microbiome–disease correlations and was therefore incorporated into the statistical model. However, vegetable intake, while identified as a potential confounder, was not adjusted for in the model to avoid overcorrection that may obscure significant microbiome–disease associations. Considering the hypothesis that diet, including vegetable consumption, might influence the gut microbiome’s role in RA-related autoimmunity, adjusting for vegetable intake could potentially conceal key bacterial associations that are either protective or harmful. Consequently, the study refrained from adjusting for vegetable intake in the analyses. The same rationale was applied to adjustment of intestinal barrier integrity (zonulin family peptide; see figure 2B ). Given the relatively high frequencies of non-steroidal anti-inflammatory drugs, proton pump inhibitors and steroid use within the at risk phases of RA, we investigated their effect on the gut microbiome. None of these variables showed a significant effect in the permutational multivariate analysis, likely due to the pro re nata use of medications coded as ‘ever used’ rather than ‘current use’.
The study highlights a distinct pattern of strain-specific enrichment and concurrent depletion of Prevotellaceae in the RA at risk phase, aligning with previous findings of Prevotellaceae overabundance in CCP+ at risk individuals compared with healthy controls ( figure 2C ). A specific strain of Prevotellaceae, ASV2058, showed significant enrichment in the CCP+ at risk group compared with healthy controls. BLAST analysis suggested this strain is likely P. copri . Notably, this enrichment was also observed in individuals with NORA compared with healthy controls. Intriguingly, ASV2058 was found to be less abundant in progressors versus non-progressors. Its abundance increased with higher CCP antibody titres and HLA positivity, but decreased in individuals with abnormal T-naïve cells. ASV1867, a second putative P. copri strain, was increased in progressors at baseline, positively correlated with CCP titres and abnormal T cells, but was negatively associated with HLA SE positivity. These findings might suggest that different strains of P. copri may have diverse roles in RA progression. One interpretation of these findings could be that ASV2058 is an additional risk factor for RA, associated with known serological and genetic risk markers, and in this context a lower threshold is required to induce early immune dysregulation and progression. Conversely, ASV1867 is not associated with genetic risk, and in this context relatively higher amounts are required for early immune dysregulation and RA progression.
Longitudinal analysis revealed that RA progressors tend to have a more unstable gut microbiome ( figure 4A ) compared with non-progressors. A median Bray-Curtis dissimilarity exceeding 0.6 was observed in progressors within 10 months prior to RA diagnosis ( figure 4A ), indicating a considerable shift in the gut microbiome during this period. When comparing the time periods of 0–10 months before RA and 11–15 months before RA, the gut microbiome appears relatively stable until 10 months before RA onset ( figure 4A ). The median Bray-Curtis index for the period of 11–15 months (0.3) aligns with values observed in non-progressors, suggesting significant changes may occur closer to RA onset. This increase in Bray-Curtis values during the period of 0–10 months may reflect the influence of RA development and associated inflammatory responses on the gut microbiome. However, for participant 3, both samples taken within 9 months before RA diagnosis showed a Bray-Curtis dissimilarity index of 0.8 ( figure 4A ), despite clinical reviews excluding RA progression at 9 and 6 months prior. This indicates that changes in the gut microbiome were occurring before the onset of clinical arthritis, although environmental influences cannot be ruled out. Similar patterns of beta diversity volatility have been noted in patients with inflammatory bowel disease around flare-ups, where increased instability correlated with heightened inflammation. 45
Our finding of increased putrescine degradation and threonine synthesis in the NORA cohort ( figure 5A ) is consistent with previous work. 9 11 Pathways such as L-lysine degradation, L-threonine metabolism and the tricarboxylic acid (TCA) cycle (acetate producers) were notably more active in the NORA group compared with healthy controls, suggesting an upregulation of amino acid metabolism and energy production in these individuals. Additionally, pathways involved in glycol metabolism and degradation, as well as phenylacetate degradation, were also elevated in individuals with NORA, indicating potential alterations in energy utilisation and aromatic compound processing. These findings suggest that individuals with NORA exhibit distinct metabolic shifts, particularly in pathways related to amino acid and energy metabolism, which could reflect underlying differences in metabolic demands or stress responses associated with inflammation.
Investigation of gut microbiome function in relation to anti-CCP antibody titre identified associations between several enzymes, gene families and metabolic pathways, aligning with literature showing that ACPA antibody titre affects microbiome function. 46 For instance, the increase of type IV secretory system conjugative DNA transfer family protein, XRE family transcriptional regulator, DNA topoisomerase and excisionase in CCP+ high and low individuals suggests a coordinated alteration in microbial gene expression. Additionally, enzymes such as cobalt precorrin-5B (C.1) methyltransferase, also elevated in both CCP+ high and low individuals, responsible for the biosynthesis in cobalamin (vitamin B 12 ), indicate potential adjustment in microbial vitamin synthesis. The activity of other enzymes, such as cadmium-exporting ATPase and pyridoxal kinase, points to altered metal ion homeostasis and coenzyme metabolism. Histidine degradation, inosine 5'-phosphate biosynthesis and folate transformations reveal alterations in amino acid metabolism, nucleotide synthesis and one carbon metabolism (oxidative stress), reflective of our findings in the NORA group. It is worth noting, although comparable dietary quality scores suggest that there were no major deviations in diet quality, including protein intake. We do recognise that direct quantification of protein consumption could provide more precise insights into its potential impact on amino acid metabolism.
We specifically assessed for the presence of the transposon described by Nii et al 41 in our shotgun metagenomics data. Our results did not show any significant relationship between the presence of this transposon and clinical factors such as CCP positivity, disease progression or HLA status ( online supplemental materials , page 51-52). The lack of association with these variables suggests that while Prevotella strains with this transposon are indeed present in the cohort (including healthy controls) and potentially relevant, their presence alone does not correlate with these specific clinical outcomes in our cohort. We did identify a higher number of transposon matches in individuals who possessed our identified Prevotella strains of interest. This suggests an interesting association between the presence of specific Prevotellaceae strains which are associated with RA clinical variables and progression and an increased number of matches to a P. copri transposon sequence, and raises the question of whether this is merely a reflection of overall Prevotella abundance or if it points to a more specific biological relationship; this warrants further work beyond the scope of this paper.
The limitations of this research, particularly the small longitudinal sample size and the lack of 1:1 longitudinal comparison between CCP+ at risk and healthy controls, temper the generalisability of the results and increase the chance of spurious associations. This should be considered when interpreting the findings and calls for further research with larger longitudinal cohorts. The heterogeneity in the NORA cohort reflects the practical constraints of recruitment from standard of care clinics, which may limit the interpretability of direct comparisons. Moreover, extending the analysis beyond the bacterial microbiome to include viromes, mycobiomes and archaeomes could unveil additional facets of microbial influence on RA pathogenesis. The absence of integrated transcriptomic or metabolomic data restricts our interpretation to potential rather than confirmed metabolic activity.
In summary, we have shown that individuals at risk of RA harbour a distinctive gut microbial composition, including but not limited to an overabundance of Prevotellaceae species. This microbial signature is consistent and correlates with traditional RA risk factors. Longitudinal examination shows a dynamic microbial environment preceding RA onset. Further research into this late phase of disease development is merited, especially given the potential of the gut microbiome as a target for prevention, including in high-risk individuals with imminent arthritis.
Ethics statements
Patient consent for publication.
Not required.
Ethics approval
This study involves human participants and was conducted at Chapel Allerton Hospital (CAH), Leeds, UK, with ethical approval granted by the Leeds, West Yorkshire Research Ethics Committee (reference: 06/Q1205/169). Participants gave informed consent to participate in the study before taking part.
Acknowledgments
We extend our gratitude to the participants of the Leeds at risk cohort and the patient discussion groups. We also wish to acknowledge the work of Professor F Ponchel with T cell subset analysis and Professor A Kastbom with ZFP quantification. Figure 1 was created with BioRender.com.
- Holers VM ,
- Demoruelle MK ,
- Kuhn KA , et al
- Sugiyama D ,
- Nishimura K ,
- Tamaki K , et al
- Do T , et al
- Sokolova MV ,
- Hartmann F ,
- Sieghart D , et al
- Kastbom A ,
- Roos Ljungberg K ,
- Ziegelasch M , et al
- van der Woude D ,
- Rantapää-Dahlqvist S ,
- Ioan-Facsinay A , et al
- Schellekens GA ,
- De Jong BAW , et al
- Alpizar-Rodriguez D ,
- Lesker TR ,
- Gronow A , et al
- Sczesnak A ,
- Longman RS , et al
- Adebayo AS ,
- Bowyer RCE , et al
- Jia H , et al
- Li J , et al
- Rodrigues GSP ,
- Cayres LCF ,
- Gonçalves FP , et al
- You HJ , et al
- Kumanogoh A ,
- Leboime A , et al
- Vaahtovuo J ,
- Munukka E ,
- Korkeamäki M , et al
- Muñiz Pedrogo DA ,
- Hillmann B , et al
- Chiang H-I ,
- Liu C-C , et al
- Picchianti-Diamanti A ,
- Panebianco C ,
- Salemi S , et al
- Forbes JD ,
- Knox NC , et al
- Danckert NP ,
- Freidin MB ,
- Smith IG , et al
- Kurakawa T ,
- Umemoto E , et al
- Rooney CM ,
- Mitra S , et al
- Gilbert BTP ,
- Tadeo RYT ,
- Lamacchia C , et al
- Chriswell ME ,
- Lefferts AR ,
- Clay MR , et al
- Gerlag DM ,
- van Baarsen LGM , et al
- Hunt L , et al
- Cleghorn CL ,
- Harrison RA ,
- Ransley JK , et al
- Callahan BJ ,
- McMurdie PJ ,
- Rosen MJ , et al
- Pruesse E ,
- Knittel K , et al
- Rideout JR ,
- Dillon MR , et al
- Beghini F ,
- McIver LJ ,
- Blanco-Míguez A , et al
- Mallick H ,
- Rahnavard A ,
- McIver LJ , et al
- Billington R , et al
- Motooka D , et al
- Candela M , et al
- Jeffery IB ,
- Jeffery IB , et al
- Halfvarson J ,
- Brislawn CJ ,
- Lamendella R , et al
Supplementary materials
Supplementary data.
This web only file has been produced by the BMJ Publishing Group from an electronic file supplied by the author(s) and has not been edited for content.
- Data supplement 1
Handling editor Josef S Smolen
X @DrCRooney
Contributors The study was designed by PE, KM, MHW and CMR. Sample and data collection was conducted by KM and CMR. Sample processing was completed by CMR. Data analysis was completed by CMR and supervised by IBJ. All authors contributed to writing the manuscript and approved it for publication. CMR is the guarantor.
Funding CMR was funded to complete this work by a personal fellowship from Versus Arthritis (22294) and Leeds Cares (9R01-A1210) and is currently funded by a National Institute for Health Research (NIHR) Clinical Lectureship. The Leeds CCP clinic is supported by the NIHR Leeds Biomedical Research Unit.
Competing interests CMR has received funding from Versus Arthritis and Leeds Cares and in-kind support from 4D Pharma PLC. KM has received grants from Gilead, Lilly, Serac Healthcare, AstraZeneca and DeepCure; consulting fees from AbbVie, UCB, Lilly, Galapagos, Serac Healthcare, Zura Bio and DeepCure; and honoraria from AbbVie, UCB, Lilly, Galapagos, Serac Healthcare, Zura Bio and DeepCure. PE has received grants from AbbVie, BMS, Lilly, Novartis, Pfizer and Samsung; and consulting fees from AbbVie, Activia, AstraZeneca, BMS, Boehringer Ingelheim, Galapagos, Gilead, Immunovant, Janssen, Lilly and Novartis.
Patient and public involvement Before starting this research, patients contributed to the study's design and feasibility, influencing recruitment, sample return and dissemination of outputs through a patient-focused discussion group at Chapel Allerton Hospital, Leeds. During the study, participant feedback on the stool collection kit was gathered and led to its redesign.
Provenance and peer review Not commissioned; externally peer reviewed.
Supplemental material This content has been supplied by the author(s). It has not been vetted by BMJ Publishing Group Limited (BMJ) and may not have been peer-reviewed. Any opinions or recommendations discussed are solely those of the author(s) and are not endorsed by BMJ. BMJ disclaims all liability and responsibility arising from any reliance placed on the content. Where the content includes any translated material, BMJ does not warrant the accuracy and reliability of the translations (including but not limited to local regulations, clinical guidelines, terminology, drug names and drug dosages), and is not responsible for any error and/or omissions arising from translation and adaptation or otherwise.
Read the full text or download the PDF:

COMMENTS
Observational studies can be prospective or retrospective studies.On the other hand, randomized experiments must be prospective studies.. The choice between an observational study vs experiment hinges on your research objectives, the context in which you're working, available time and resources, and your ability to assign subjects to the experimental groups and control other variables.
Experiment vs Observational Study 1. Experiment. An experiment is a research method characterized by a high degree of experimental control exerted by the researcher. In the context of academia, it allows for the testing of causal hypotheses (Privitera, 2022).
Psychology. Experimental Study: Conducting a lab experiment to test the effect of sleep deprivation on cognitive performance by controlling sleep hours and measuring test scores. Observational Study: Observing social interactions in a public setting to explore natural communication patterns without intervention.
Experiment vs. observational study Experiments and observational studies are both methods of research, but they also have some important differences, including: Purpose The purpose of experiments is typically to test a hypothesis that a researcher has about the reason for an event or the effects of a particular action. Therefore, experiments ...
Observational study vs. experiment. The key difference between observational studies and experiments is that a properly conducted observational study will never attempt to influence responses, while experimental designs by definition have some sort of treatment condition applied to a portion of participants.
Observational research studies involve the passive observation of subjects without any intervention or manipulation by researchers. These studies are designed to scrutinize the relationships between variables and test subjects, uncover patterns, and draw conclusions grounded in real-world data. Researchers refrain from interfering with the ...
Observational studies and experiments are two primary research methods for gaining insights and drawing conclusions. While observational studies involve monitoring subjects without interference, experiments involve manipulating variables to study effects. Each method has its strengths and limitations. Scientists often use both observational ...
Observational Study Definition. In an observational study, the researchers only observe the subjects and do not interfere or try to influence the outcomes. In other words, the researchers do not control the treatments or assign subjects to experimental groups. Instead, they observe and measure variables of interest and look for relationships ...
Some of the key points about experimental studies are as follows: Experimental studies are closely monitored. Experimental studies are expensive. Experimental studies are typically smaller and shorter than observational studies. Now, let us understand the difference between the two types of studies using different problems.
In these cases, the researchers are merely observing variables and outcomes. There are two types of observational studies: retrospective studies and prospective studies. In a retrospective study, data is collected after events have taken place. This may be through surveys, historical data, or administrative records.
Observational Studies. In observational (non-experimental) studies, investigators observe individuals without experimental manipulation or intervention. There is an inadequacy about the term "observational study" because the outcome variable of an experiment could also be observed. Observational studies can be further categorized into ...
Attrition refers to participants leaving a study. It always happens to some extent—for example, in randomized controlled trials for medical research. Differential attrition occurs when attrition or dropout rates differ systematically between the intervention and the control group.As a result, the characteristics of the participants who drop out differ from the characteristics of those who ...
A: The choice of study design depends on the specific research question and the available resources. Observational studies are preferred when ethical or practical constraints prevent the use of experimental studies, while experimental studies are suitable for establishing cause-effect relationships.
How does an observational study differ from an experiment? The key difference between observational studies and experiments is that, done correctly, an observational study will never influence the responses or behaviours of participants. Experimental designs will have a treatment condition applied to at least a portion of participants.
The two main types of research are observational studies and experiments. An observational study is when the researcher observes the effect of a specific variable as it occurs naturally, without ...
Observational studies also tend to cost much less than designed experiments, and it's often possible to obtain a much larger data set than you would with a designed experiment. Still, it's always important to remember the difference in what we can claim as a result of observational studies versus designed experiments. Types of Observational Studies
Observational Studies vs. Experiments. Learning Targets. Explain the concept of confounding and how it limits the ability to make cause-and-effect conclusions. Distinguish between an observational study and an experiment, and identify the explanatory and response variables in each type of study.
Observational studies. Observational studies are ones where researchers observe the effect of a risk factor, diagnostic test, treatment or other intervention without trying to change who is or isn't exposed to it. Cohort studies and case control studies are two types of observational studies. Cohort study: For research purposes, a cohort is ...
Again, unlike the observational study statistics, which are considered biased and weak, evidence from experimental research is stronger. Advantages of Observational Studies. If you are thinking of carrying a research and have been wondering whether to go for randomized experiment vs observational study, here are some key advantages of the latter.
The weaknesses of an observational study. The results of an observational study are frequently open to dispute. The lack of control usually means there are many factors that could have influenced the result. For instance, examining the positive impact of a healthy diet could also be down to the fact that those people will likely exercise too.
Background Assessing maternal health care utilisation is imperative for the health of both mother and her child. Maternal education is an important determinant in subsequent maternal health care usage, according to research. There is a dearth of research on the causal relationship between maternal education and maternal health services as well as examining the performance of different ...
The cross-sectional study encompassed 153 participants; their characteristics are detailed in table 1. In the RA at risk cohort, 70% were female, with a median anti-CCP antibody titre of 73 IU/mL. During the study period, 30 individuals progressed to RA, with a median interval of 9.6 months between stool collection and RA progression.