- Skip to Content
- Bulletin Home

- Degree Charts >
- Computational Science and Engineering (PhD)
- Around Campus
- Academic Program
- Administration
- Arts at MIT
- Campus Media
- Fraternities, Sororities, and Independent Living Groups
- Health Services
- Priscilla King Gray Public Service Center
- Religious Organizations
- Student Government
- Work-Life and Family Resources
- Advising and Support
- Digital Learning
- Disability and Access Services
- Information Systems and Technology
- Student Financial Services
- Writing and Communication Center
- Major Course of Study
- General Institute Requirements
- Independent Activites Period
- Undergraduate Research Opportunities Program
- First-Year Advising Seminars
- Interphase EDGE/x
- Edgerton Center
- Grading Options
- Study at Other Universities
- Internships Abroad
- Career Advising and Professional Development
- Teacher Licensure and Education
- ROTC Programs
- Financial Aid
- Medical Requirements
- Graduate Study at MIT
- General Degree Requirements
- Other Institutions
- Registration
- Term Regulations and Examination Policies
- Academic Performance and Grades
- Policies and Procedures
- Privacy of Student Records
- Abdul Latif Jameel Clinic for Machine Learning in Health
- Abdul Latif Jameel Poverty Action Lab
- Art, Culture, and Technology Program
- Broad Institute of MIT and Harvard
- Center for Bits and Atoms
- Center for Clinical and Translational Research
- Center for Collective Intelligence
- Center for Computational Science and Engineering
- Center for Constructive Communication
- Center for Energy and Environmental Policy Research
- Center for Environmental Health Sciences
- Center for Global Change Science
- Center for International Studies
- Center for Real Estate
- Center for Transportation & Logistics
- Computer Science and Artificial Intelligence Laboratory
- Concrete Sustainability Hub
- D-Lab
- Deshpande Center for Technological Innovation
- Division of Comparative Medicine
- Haystack Observatory
- Initiative on the Digital Economy
- Institute for Medical Engineering and Science
- Institute for Soldier Nanotechnologies
- Institute for Work and Employment Research
- Internet Policy Research Initiative
- Joint Program on the Science and Policy of Global Change
- Knight Science Journalism Program
- Koch Institute for Integrative Cancer Research
- Laboratory for Financial Engineering
- Laboratory for Information and Decision Systems
- Laboratory for Manufacturing and Productivity
- Laboratory for Nuclear Science
- Legatum Center for Development and Entrepreneurship
- Lincoln Laboratory
- Martin Trust Center for MIT Entrepreneurship
- Materials Research Laboratory
- McGovern Institute for Brain Research
- Microsystems Technology Laboratories
- MIT Center for Art, Science & Technology
- MIT Energy Initiative
- MIT Environmental Solutions Initiative
- MIT Kavli Institute for Astrophysics and Space Research
- MIT Media Lab
- MIT Office of Innovation
- MIT Open Learning
- MIT Portugal Program
- MIT Professional Education
- MIT Sea Grant College Program
- Nuclear Reactor Laboratory
- Operations Research Center
- Picower Institute for Learning and Memory
- Plasma Science and Fusion Center
- Research Laboratory of Electronics
- Simons Center for the Social Brain
- Singapore-MIT Alliance for Research and Technology Centre
- Sociotechnical Systems Research Center
- Whitehead Institute for Biomedical Research
- Women's and Gender Studies Program
- Architecture (SB, Course 4)
- Architecture (MArch)
- Art and Design (SB, Course 4-B)
- Art, Culture, and Technology (SM)
- Architecture Studies (SMArchS)
- Media Arts and Sciences
- Planning (SB, Course 11)
- Urban Science and Planning with Computer Science (SB, Course 11-6)
- Aeronautics and Astronautics Fields (PhD)
- Aerospace Engineering (SB, Course 16)
- Engineering (SB, Course 16-ENG)
- Biological Engineering (SB, Course 20)
- Biological Engineering (PhD)
- Chemical Engineering (Course 10)
- Chemical-Biological Engineering (Course 10-B)
- Chemical Engineering (Course 10-C)
- Engineering (Course 10-ENG)
- Engineering (Course 1-ENG)
- Computation and Cognition (Course 6-9)
- Computer Science and Engineering (Course 6-3)
- Computer Science and Molecular Biology (Course 6-7)
- Electrical Engineering with Computing (Course 6-5)
- Electrical Engineering and Computer Science (MEng)
- Computer Science and Molecular Biology (MEng)
- Health Sciences and Technology
- Archaeology and Materials (Course 3-C)
- Materials Science and Engineering (Course 3)
- Materials Science and Engineering (Course 3-A)
- Materials Science and Engineering (PhD)
- Mechanical Engineering (Course 2)
- Mechanical and Ocean Engineering (Course 2-OE)
- Engineering (Course 2-A)
- Nuclear Science and Engineering (Course 22)
- Engineering (Course 22-ENG)
- Anthropology (Course 21A)
- Comparative Media Studies (CMS)
- Writing (Course 21W)
- Data, Economics, and Design of Policy (MASc)
- Economics (Course 14-1)
- Economics (PhD)
- Mathematical Economics (Course 14-2)
- Global Studies and Languages (Course 21G)
- History (Course 21H)
- Linguistics and Philosophy (Course 24-2)
- Philosophy (Course 24-1)
- Linguistics (SM)
- Literature (Course 21L)
- Music (Course 21M-1)
- Theater Arts (Course 21M-2)
- Political Science (Course 17)
- Science, Technology, and Society/Second Major (STS)
- Business Analytics (Course 15-2)
- Finance (Course 15-3)
- Management (Course 15-1)
- Biology (Course 7)
- Chemistry and Biology (Course 5-7)
- Brain and Cognitive Sciences (Course 9)
- Chemistry (Course 5)
- Earth, Atmospheric and Planetary Sciences (Course 12)
- Mathematics (Course 18)
- Mathematics (PhD)
- Mathematics with Computer Science (Course 18-C)
- Physics (Course 8)
- Department of Electrical Engineering and Computer Science
- Institute for Data, Systems, and Society
- Chemistry and Biology
- Climate System Science and Engineering
- Computation and Cognition
- Computer Science and Molecular Biology
- Computer Science, Economics, and Data Science
- Humanities and Engineering
- Humanities and Science
- Urban Science and Planning with Computer Science
- African and African Diaspora Studies
- American Studies
- Ancient and Medieval Studies
- Applied International Studies
- Asian and Asian Diaspora Studies
- Biomedical Engineering
- Energy Studies
- Entrepreneurship and Innovation
- Environment and Sustainability
- Latin American and Latino/a Studies
- Middle Eastern Studies
- Polymers and Soft Matter
- Public Policy
- Russian and Eurasian Studies
- Statistics and Data Science
- Women's and Gender Studies
- Advanced Urbanism
- Computational and Systems Biology
Computational Science and Engineering
- Design and Management (IDM & SDM)
- Joint Program with Woods Hole Oceanographic Institution
- Leaders for Global Operations
- Microbiology
- Music Technology and Computation
- Operations Research
- Real Estate Development
- Social and Engineering Systems
- Supply Chain Management
- Technology and Policy
- Transportation
- School of Architecture and Planning
- School of Engineering
- Artificial Intelligence and Decision Making (Course 6-4)
- Nuclear Science and Engineering (PhD)
- School of Humanities, Arts, and Social Sciences
- Humanities (Course 21)
- Humanities and Engineering (Course 21E)
- Humanities and Science (Course 21S)
- Sloan School of Management
- School of Science
- Brain and Cognitive Sciences (PhD)
- Earth, Atmospheric and Planetary Sciences Fields (PhD)
- Interdisciplinary Programs (SB)
- Climate System Science and Engineering (Course 1-12)
- Computer Science, Economics, and Data Science (Course 6-14)
- Interdisciplinary Programs (Graduate)
- Biological Oceanography (PhD)
- Computation and Cognition (MEng)
- Computational Science and Engineering (SM)
- Computer Science, Economics, and Data Science (MEng)
- Engineering and Management (SM)
- Leaders for Global Operations (MBA/SM and SM)
- Music Technology and Computation (SM and MASc)
- Real Estate Development (SM)
- Statistics (PhD)
- Supply Chain Management (MEng and MASc)
- Technology and Policy (SM)
- Transportation (SM)
- Aeronautics and Astronautics (Course 16)
- Aerospace Studies (AS)
- Architecture (Course 4)
- Biological Engineering (Course 20)
- Civil and Environmental Engineering (Course 1)
- Comparative Media Studies / Writing (CMS)
- Comparative Media Studies / Writing (Course 21W)
- Computational and Systems Biology (CSB)
- Computational Science and Engineering (CSE)
- Concourse (CC)
- Data, Systems, and Society (IDS)
- Earth, Atmospheric, and Planetary Sciences (Course 12)
- Economics (Course 14)
- Edgerton Center (EC)
- Electrical Engineering and Computer Science (Course 6)
- Engineering Management (EM)
- Experimental Study Group (ES)
- Global Languages (Course 21G)
- Health Sciences and Technology (HST)
- Linguistics and Philosophy (Course 24)
- Management (Course 15)
- Media Arts and Sciences (MAS)
- Military Science (MS)
- Music (Course 21M)
- Naval Science (NS)
- Science, Technology, and Society (STS)
- Special Programs
- Supply Chain Management (SCM)
- Theater Arts (21T)
- Urban Studies and Planning (Course 11)
- Women's and Gender Studies (WGS)

Doctoral Programs in Computational Science and Engineering
Doctor of philosophy in computational science and engineering, program requirements, programs offered by ccse in conjunction with select departments in the schools of engineering and science.
The interdisciplinary doctoral program in Computational Science and Engineering ( PhD in CSE + Engineering or Science ) offers students the opportunity to specialize at the doctoral level in a computation-related field of their choice via computationally-oriented coursework and a doctoral thesis with a disciplinary focus related to one of eight participating host departments, namely, Aeronautics and Astronautics; Chemical Engineering; Civil and Environmental Engineering; Earth, Atmospheric and Planetary Sciences; Materials Science and Engineering; Mathematics; Mechanical Engineering; or Nuclear Science and Engineering.
Doctoral thesis fields associated with each department are as follows:
- Aerospace Engineering and Computational Science
- Computational Science and Engineering (available only to students who matriculate in 2023–2024 or earlier)
- Chemical Engineering and Computation
- Civil Engineering and Computation
- Environmental Engineering and Computation
- Computational Materials Science and Engineering
- Mechanical Engineering and Computation
- Computational Nuclear Science and Engineering
- Nuclear Engineering and Computation
- Computational Earth, Science and Planetary Sciences
- Mathematics and Computational Science
As with the standalone CSE PhD program, the emphasis of thesis research activities is the development of new computational methods and/or the innovative application of state-of-the-art computational techniques to important problems in engineering and science. In contrast to the standalone PhD program, however, this research is expected to have a strong disciplinary component of interest to the host department.
The interdisciplinary CSE PhD program is administered jointly by CCSE and the host departments. Students must submit an application to the CSE PhD program, indicating the department in which they wish to be hosted. To gain admission, CSE program applicants must receive approval from both the host department graduate admission committee and the CSE graduate admission committee. See the website for more information about the application process, requirements, and relevant deadlines .
Once admitted, doctoral degree candidates are expected to complete the host department's degree requirements (including qualifying exam) with some deviations relating to coursework, thesis committee composition, and thesis submission that are specific to the CSE program and are discussed in more detail on the CSE website . The most notable coursework requirement associated with this CSE degree is a course of study comprising five graduate subjects in CSE (below).
Computational Concentration Subjects
Note: Students may not use more than 12 units of credit from a "meets with undergraduate" subject to fulfill the CSE curriculum requirements

Print this page.
The PDF includes all information on this page and its related tabs. Subject (course) information includes any changes approved for the current academic year.
- Who’s Teaching What
- Subject Updates
- MEng program
- Opportunities
- Minor in Computer Science
- Resources for Current Students
- Program objectives and accreditation
- Graduate program requirements
- Admission process
- Degree programs
- Graduate research
- EECS Graduate Funding
- Resources for current students
- Student profiles
- Instructors
- DEI data and documents
- Recruitment and outreach
- Community and resources
- Get involved / self-education
- Rising Stars in EECS
- Graduate Application Assistance Program (GAAP)
- MIT Summer Research Program (MSRP)
- Sloan-MIT University Center for Exemplary Mentoring (UCEM)
- Electrical Engineering
- Computer Science
- Artificial Intelligence + Decision-making
AI and Society
AI for Healthcare and Life Sciences
- Artificial Intelligence and Machine Learning
Biological and Medical Devices and Systems
Communications Systems
- Computational Biology
Computational Fabrication and Manufacturing
Computer Architecture
Educational Technology
Electronic, Magnetic, Optical and Quantum Materials and Devices
Graphics and Vision
Human-Computer Interaction
Information Science and Systems
Integrated Circuits and Systems
Nanoscale Materials, Devices, and Systems
Natural Language and Speech Processing
- Optics + Photonics
Optimization and Game Theory
Programming Languages and Software Engineering
Quantum Computing, Communication, and Sensing
Security and Cryptography
Signal Processing
Systems and Networking
Systems Theory, Control, and Autonomy
Theory of Computation
- Departmental History
- Departmental Organization
- Visiting Committee
- Faculty AI+D
- Faculty Emeritus
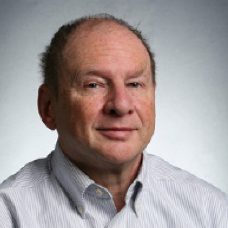
Hal Abelson
Class of 1922 Professor, [CS and AI+D]
- [email protected]
- (617) 253-5856
- Office: 32-G516
Artificial Intelligence + Machine Learning
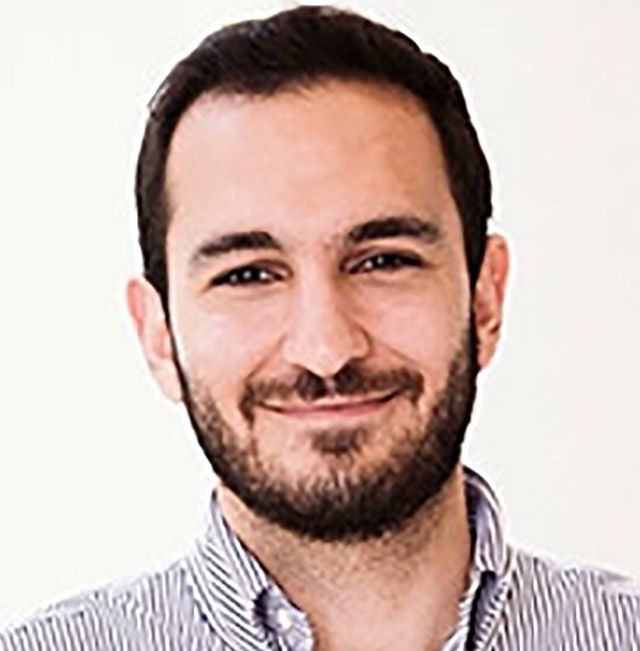
Associate Professor, Media Lab; Joint appointment in EECS, [CS]
- [email protected]
- (617) 715-5956
- Office: E14-474G
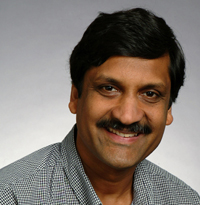
Anant Agarwal
CEO, edX; Professor of EECS; [CS and EE]
- [email protected]
- (617) 253-1448
- Office: NE55-900
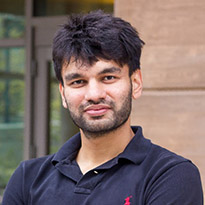
Pulkit Agrawal
Associate Professor [AI+D and CS]
- [email protected]
- (617) 253-5851
- Office: 45-641H
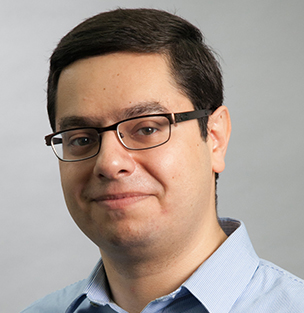
Mohammad Alizadeh
Associate Professor, [CS and AI+D]; Industry Officer; Director, 6-A MEng Thesis Program
- [email protected]
- (617) 253-6042
- Office: 32-G920
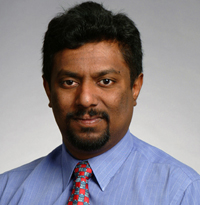
Saman Amarasinghe
Professor of EECS, [CS]
- [email protected]
- (617) 253-8879
- Office: 38-427
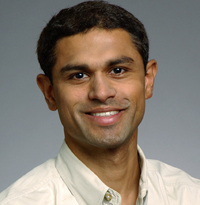
Hari Balakrishnan
Fujitsu Professor in Electrical Engineering and Computer Science, [CS and AI+D]
- [email protected]
- (617) 253-8713
- Office: 32-G940
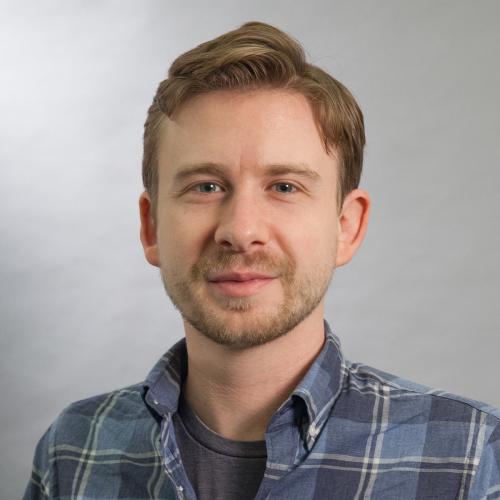
Associate Professor, [CS]
- [email protected]
- (617) 253-0004
- Office: 32-G996
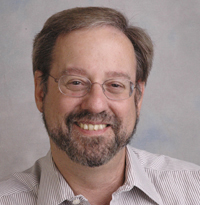
Robert Berwick
Professor of CS and Engineering and Computational Linguistics (Post-Tenure), [AI+D and CS]
- [email protected]
- (617) 253-8918
- Office: 32-D728
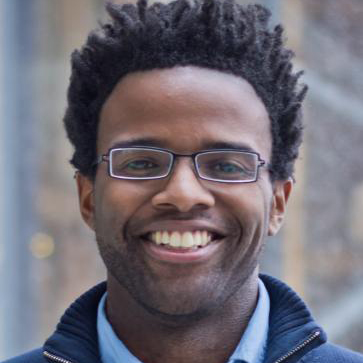
Michael Carbin
Associate Professor, [CS and AI+D]
- [email protected]
- (617) 253-5881
- Office: 32-G782
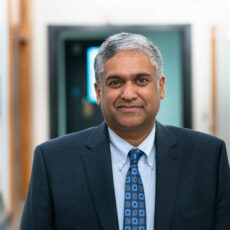
Anantha Chandrakasan
Dean, MIT School of Engineering; Chief Innovation and Strategy Officer, MIT; Vannevar Bush Professor, [EE and CS]
- [email protected]
- (617) 258-0818
- Office: 1-206
Brynmor Chapman
Lecturer, [CS]
- [email protected]
- Office: 38-683
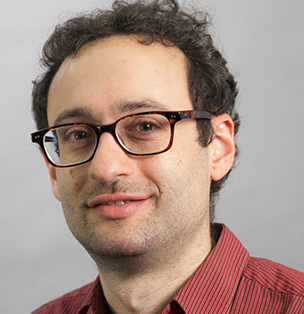
Adam Chlipala
Arthur J. Conner (1888) Professor, [CS]
- [email protected]
- (617) 324-8439
- Office: 32-G842
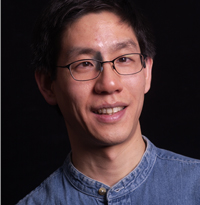
Isaac Chuang
Professor of EECS, [AI+D and EE, CS]
- [email protected]
- (617) 253-1692
- Office: 26-251
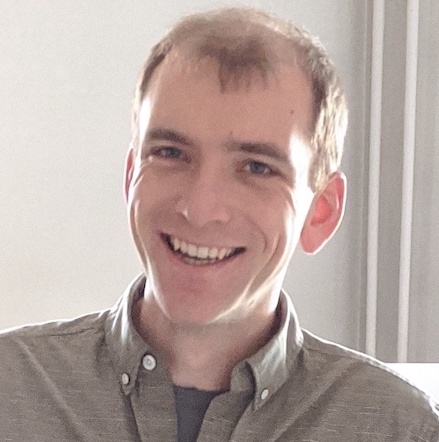
Henry Corrigan-Gibbs
Douglas Ross (1954) Career Development Professor of Software Technology; Assistant Professor, [CS]
- [email protected]
- 617-253-0301
- Office: 32-G970a
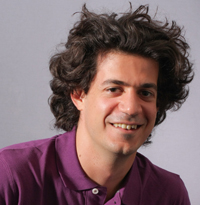
Constantinos Daskalakis
Armen Avanessians (1982) Professor, [AI+D and CS]
- [email protected]
- (617) 253-9643
- Office: 32-G694
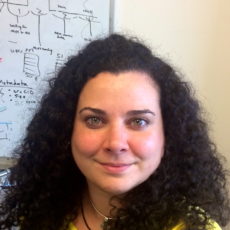
Christina Delimitrou
KDD Career Development Professor in Communications and Technology; Associate Professor, [CS]
- [email protected]
- Office: 32-G738
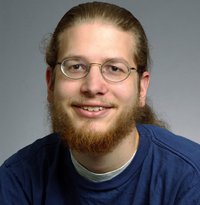
Erik Demaine
Professor, [CS]
- [email protected]
- (617) 253-6871
- Office: 32-G680
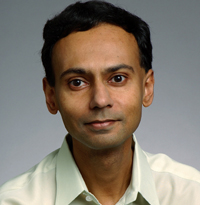
Srini Devadas
Edwin Sibley Webster Professor, [CS]
- [email protected]
- (617) 253-0454
- Office: 32-G844
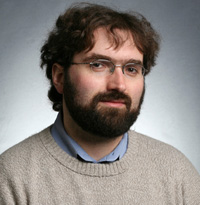
Frederic Durand
Amar Bose Professor of Computing, [AI+D and CS]
- [email protected]
- (617) 253-7223
- Office: 32-D426
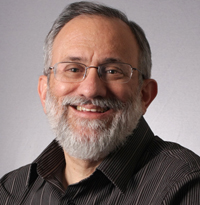
Professor of the Practice in EECS, [CS and EE]
- [email protected]
- (617) 258-9190
- Office: 32-868
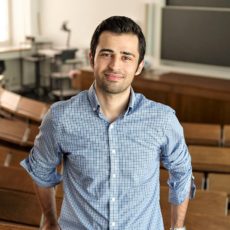
Mohsen Ghaffari
Steven and Renee Finn Career Development Professor; Associate Professor, [CS]
- [email protected]
- (617) 253-4591
- Office: 32-G618
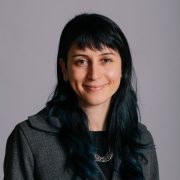
Manya Ghobadi
- [email protected]
- 617-253-5851
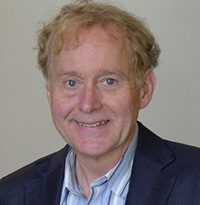
David Gifford
Professor of CS and Engineering (Post-Tenure), [AI+D and CS]
- [email protected]
- (617) 253-6039
- Office: 32-G542
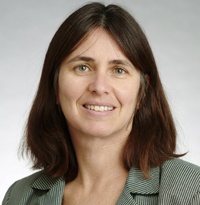
Shafrira Goldwasser
RSA Professor (Post-Tenure) , [CS]
- [email protected]
- (617) 253-5914
- Office: 32-G682
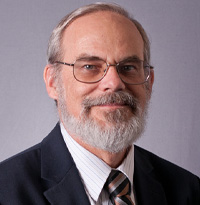
W. Eric L Grimson
Chancellor for Academic Advancement; Interim Vice President for Open Learning; Bernard M. Gordon Professor in Medical Engineering; Professor of Computer Science and Engineering, [CS and AI+D]
- [email protected]
- 617-253-4645
- Office: 3-221
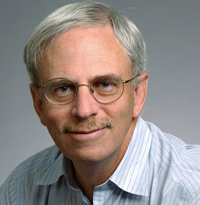
John Guttag
Dugald C. Jackson Professor in Electrical Engineering, [CS]
- [email protected]
- (617) 253-6022
- Office: 32-G966
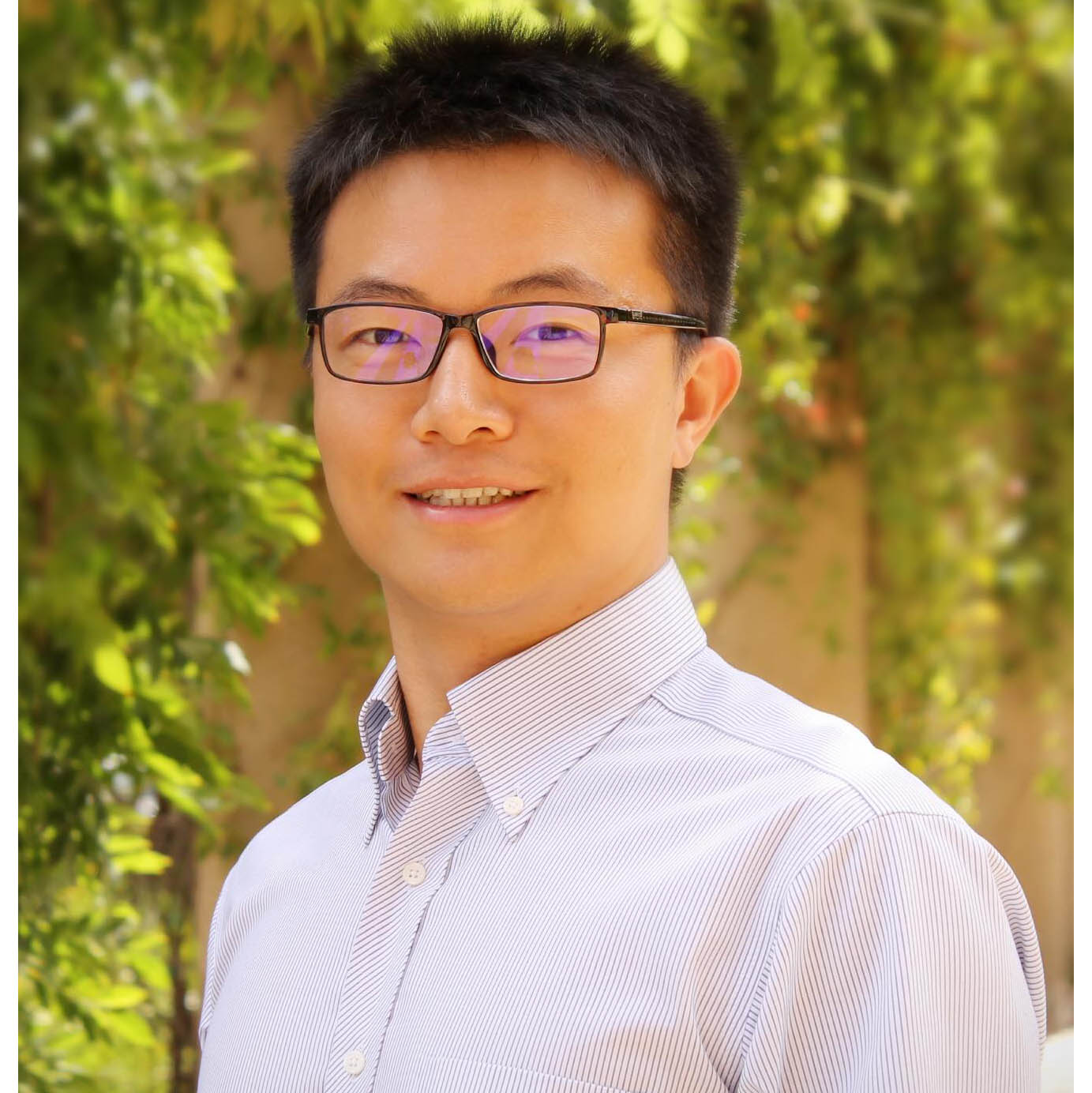
Associate Professor, [EE and CS]
- [email protected]
- (617) 253-0086
- Office: 38-344
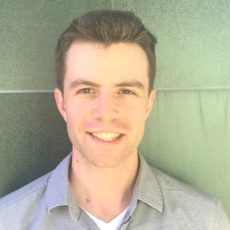
Sam Hopkins
Jamieson Career Development Professor in Electrical Engineering and Computer Science; Assistant Professor, [CS and AI+D]
- [email protected]
- Office: 32-G666
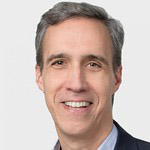
Daniel Huttenlocher
Dean, MIT Stephen A. Schwarzman College of Computing; Henry Ellis Warren (1894) Professor, [CS and AI+D]
- [email protected]
- (617) 253-5388
- Office: 4-212
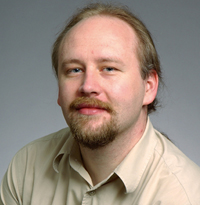
Piotr Indyk
Thomas D. and Virginia W. Cabot Professor, [CS and AI+D]
- [email protected]
- (617) 452-3402
- Office: 32-G642
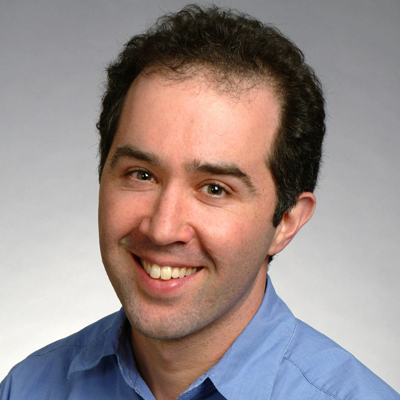
Daniel Jackson
Professor of CS and Engineering, [CS]
- [email protected]
- (617) 258-8471
- Office: 32-G704
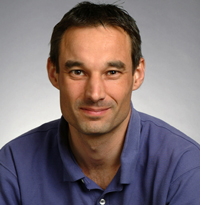
M. Frans Kaashoek
Charles A. Piper (1935) Professor, [CS]
- [email protected]
- (617) 253-7149
- Office: 32-G992
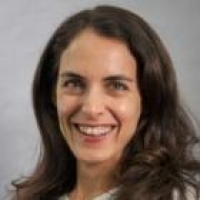
Ellen Swallow Richards (1873) Professor; Professor of EECS, [CS]
- [email protected]
- Office: 32-G594
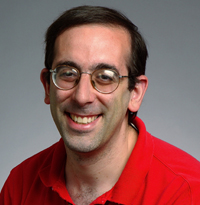
David Karger
- [email protected]
- (617) 258-6167
- Office: 32-G592
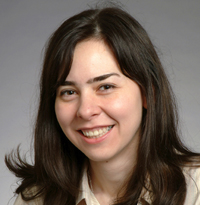
Dina Katabi
Thuan (1990) and Nicole Pham Professor, [CS and AI+D]
- [email protected]
- (617) 324-6027
- Office: 32-G936

Manolis Kellis
Professor of CS, [AI+D and CS]
- [email protected]
- (617) 253-2419
- Office: 32-G564
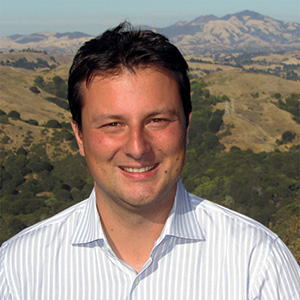
- [email protected]
- (510) 926-5856
- Office: 32-G914
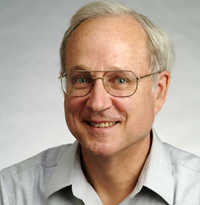
Butler Lampson
Adjunct Professor of CS and Engineering
- [email protected]
- (425) 703-5925
- Office: 32-G924
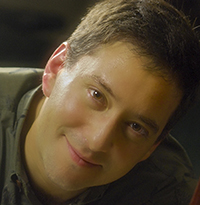
Steven Leeb
Emanuel E. Landsman (1958) Professor, [EE and CS]
- [email protected]
- (617) 253-9360
- Office: 10-069
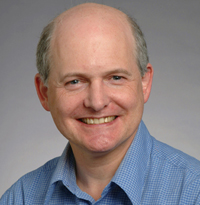
Charles Leiserson
Edwin Sibley Webster Professor; [CS]
- [email protected]
- (617) 253-5833
- Office: 32-G768
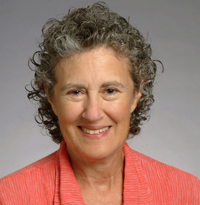
Barbara Liskov
Institute Professor (post tenure)
- [email protected]
- (617) 253-5886
- Office: 32-G942
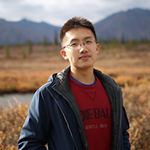
Elting Morison Career Development Professor, Assistant professor, [CS]
- [email protected]
- Office: 32-D632

Nancy Lynch
NEC Professor of Software Science and Engineering (Post-Tenure), [CS]
- [email protected]
- (617) 253-7225
- Office: 32-G668
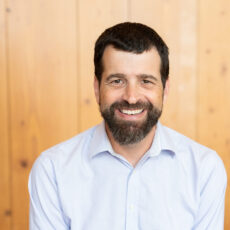
Samuel Madden
Faculty Head, CS (effective Aug 1); Distinguished College of Computing Professor, [CS and AI+D]
- [email protected]
- (617) 258-6643
- Office: 32-G938
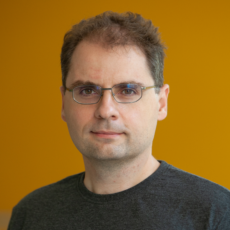
Aleksander Mądry
Cadence Design Systems Professor, [AI+D and CS]
- [email protected]
- (617) 324-6739
- Office: 32-G806
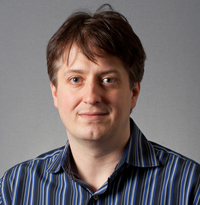
Wojciech Matusik
Joan and Irwin M. (1957) Jacobs Professor, [CS and AI+D]
- [email protected]
- (617) 324-8432
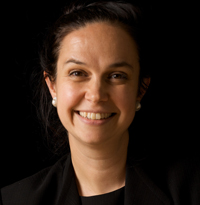
Muriel Médard
NEC Professor of Software Science and Engineering, [EE and CS, AI+D]
- [email protected]
- (617) 253-3167
- Office: 36-512
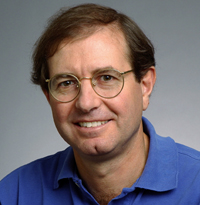

Silvio Micali
Ford Foundation Professor of Engineering (Post-Tenure) , [CS]
- [email protected]
- (617) 253-5949
- Office: 32-G644

Robert Miller
Education Officer for Computer Science, Distinguished Professor in EECS, [CS]
- [email protected]
- (617) 324-6028
- Office: 32-G718
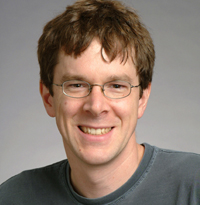
Robert Morris
- [email protected]
- (617) 253-5983
- Office: 32-G972
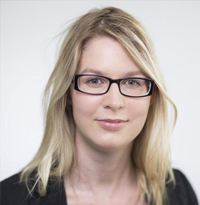
Stefanie Mueller
TIBCO Founders Professor; Associate Professor [CS and EE]
- [email protected]
- Office: 32-211
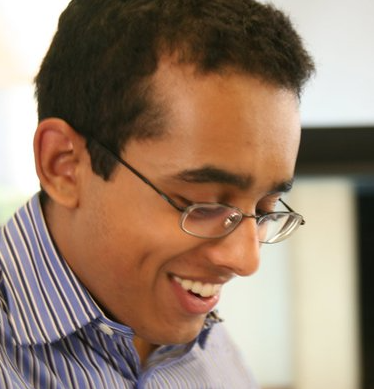
Anand V Natarajan
ITT Career Development Professor in Computer Technology; Assistant Professor, [CS]
- [email protected]
- 408-910-4116
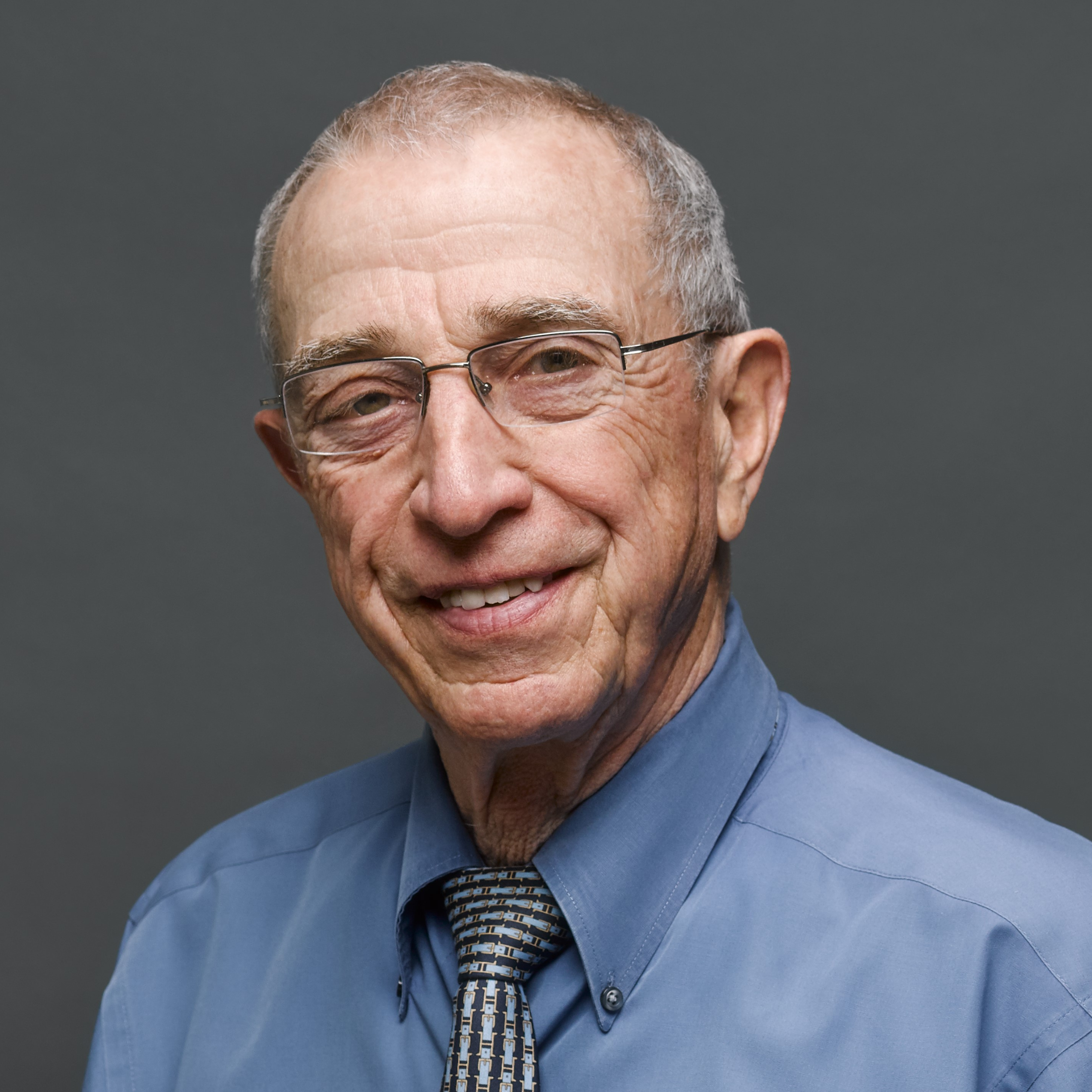
Alan Oppenheim
Ford Professor of Engineering (Post-Tenure), [EE and CS, AI+D]
- [email protected]
- (617) 253-4177
- Office: 36-615D
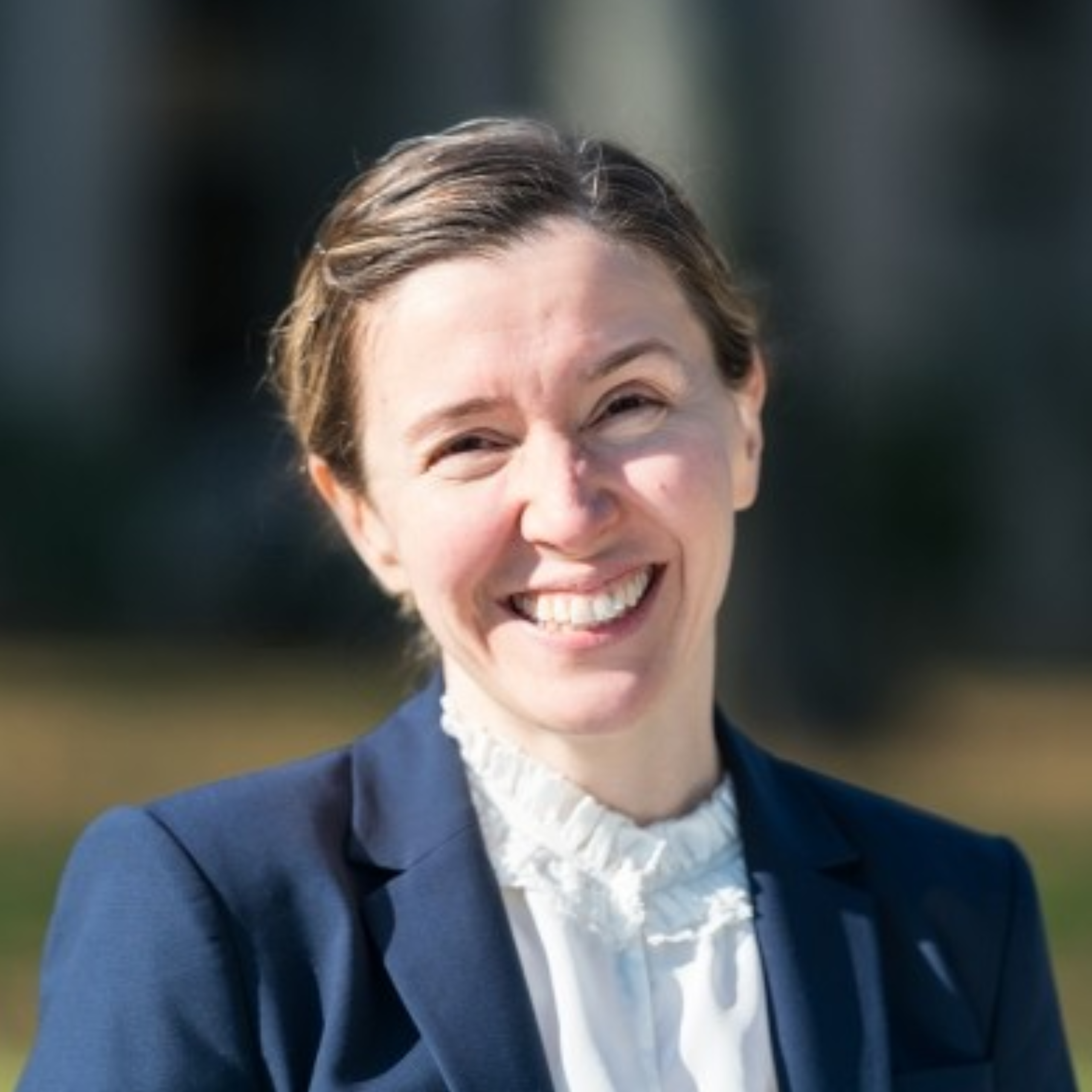
Asu Ozdaglar
EECS Department Head; MIT Schwarzman College of Computing Deputy Dean of Academics; MathWorks Professor, [AI+D and EE, CS]
- [email protected]
- (617) 253-4607
- Office: 38-403
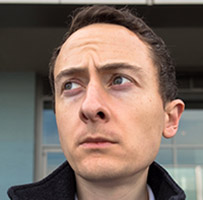
Jonathan Ragan-Kelley
- [email protected]
- 801-913-0101
- Office: 32-D462
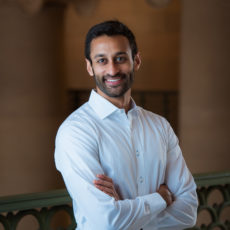
Manish Raghavan
Drew Houston (2005) Professorship; Assistant Professor / Shared Appointment in Sloan School of Management, [CS]
- [email protected]
- (617) 715-5368
- Office: E62-459

Srini Raghuraman
- [email protected]
- Office: 38-688
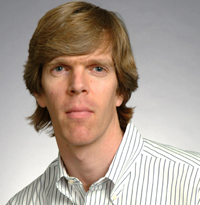
Martin Rinard
Professor of CS and Engineering, [CS and AI+D]
- [email protected]
- (617) 258-6922
- Office: 32-G744
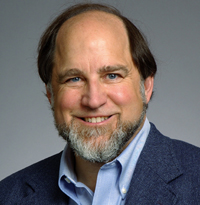
Ronald Rivest
Institute Professor (Post-Tenure); Professor Post-Tenure of Computer Science and Engineering, [CS]
- [email protected]
- (617) 253-5880
- Office: 32-G692
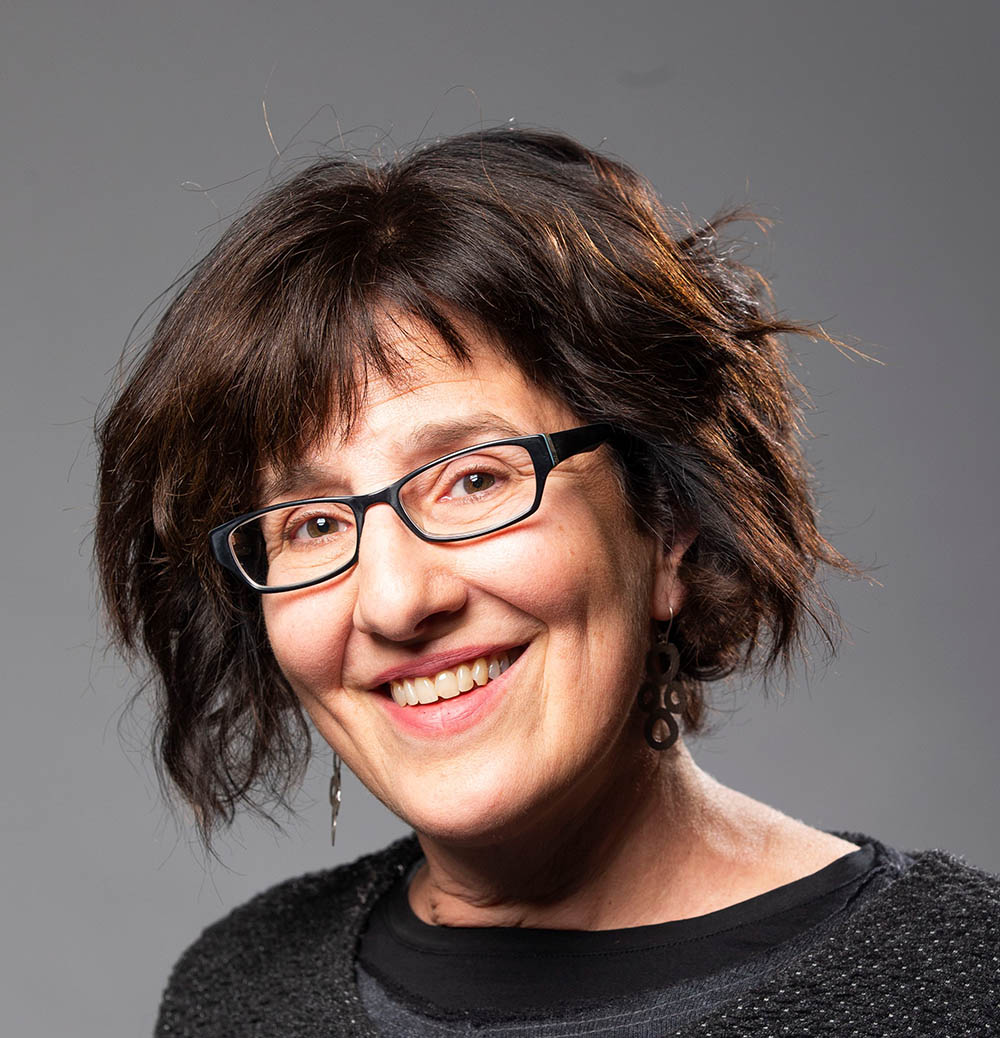
Ronitt Rubinfeld
Edwin Sibley Webster Professor, [CS and AI+D]
- [email protected]
- (617) 253-0884
- Office: 32-G69832-G698
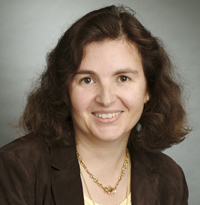
Daniela Rus
Director, CSAIL; MIT Schwarzman College of Computing Deputy Dean of Research; Andrew (1956) and Erna Viterbi Professor, [AI+D and CS]
- [email protected]
- (617) 258-7567
- Office: 32-374
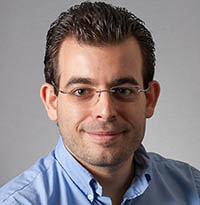
Daniel Sanchez
Professor of EECS, [CS and EE]
- [email protected]
- (617) 715-4886
- Office: 32-G838
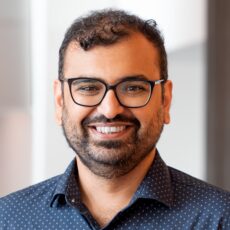
Arvind Satyanarayan
- [email protected]
- (617) 258-0697
- Office: 32-G706
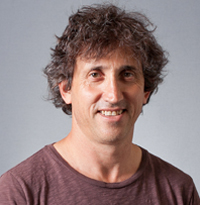
- [email protected]
- (617) 324-8440
- Office: 32-G622
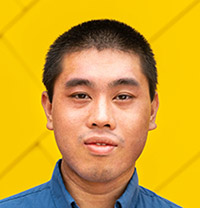
Julian Shun
- [email protected]
- (617) 258-0669
- Office: 32G-736
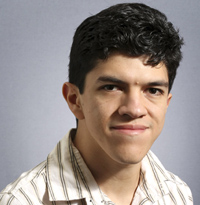
Armando Solar-Lezama
Distinguished Professor of Computing, MIT Schwarzman College of Computing; Professor of EECS, [CS and AI+D]
- [email protected]
- (617) 324-8356
- Office: 32-G840
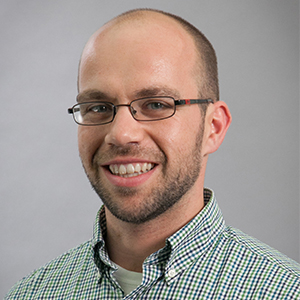
Justin Solomon
Associate Professor of EECS, [AI+D and CS]
- [email protected]
- (617) 324-6738
- Office: 32-D460
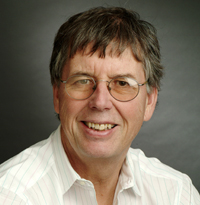
Michael Stonebraker
Adjunct Professor of CS and Engineering, [CS]
- [email protected]
- (617) 253-3538
- Office: 32-G916
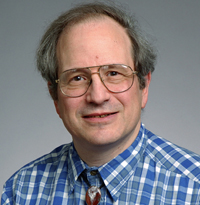
Gerald Sussman
Panasonic Professor, [CS and EE, AI+D]
- [email protected]
- (617) 253-5874
- Office: 32-385
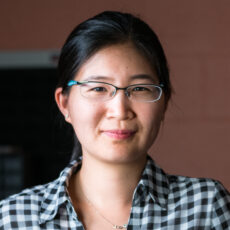
Vivienne Sze
Professor, [EE and CS, AI+D]
- [email protected]
- 617.324.7352
- Office: 38-260
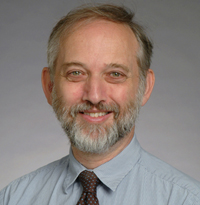
Peter Szolovits
Professor of Computer Science and Engineering; [AI+D and CS]
- [email protected]
- (617) 253-3476
- Office: 32-254
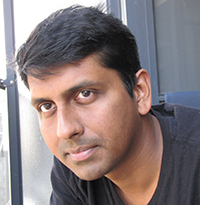
Vinod Vaikuntanathan
Ford Foundation Professor of Engineering, [CS and AI+D]
- [email protected]
- (617) 324-8444
- Office: 32-G696
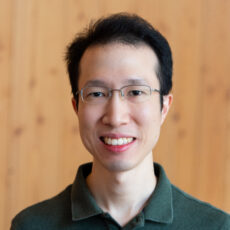
Andrew Wang
- [email protected]
- Office: 38-648
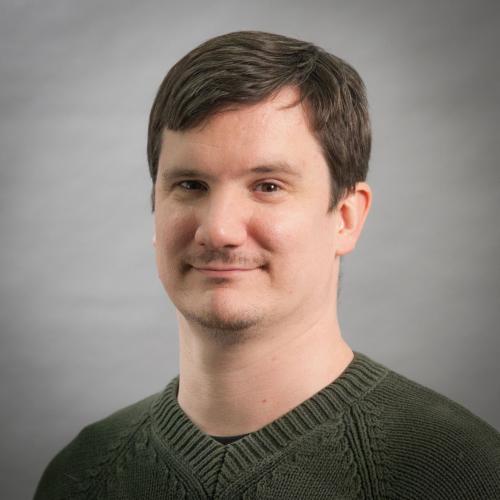
Ryan Williams
Professor of EECS, [CS and AI+D]
- [email protected]
- Office: 32-G638
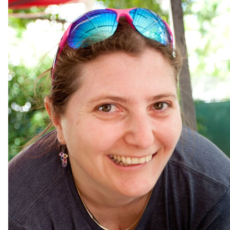
Virginia Vassilevska Williams
Professor, [CS and AI+D]
- [email protected]
- Office: 32-G640
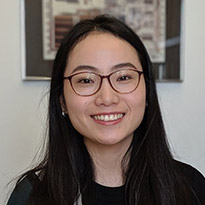
Mengjia Yan
- [email protected]
- 617-258-0719
- Office: 32G-840
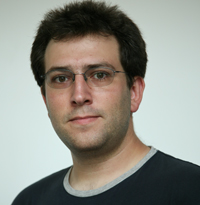
Nickolai Zeldovich
Joan and Irwin M. (1957) Jacobs Professor, [CS]
- [email protected]
- (617) 253-6005
- Office: 32-G994
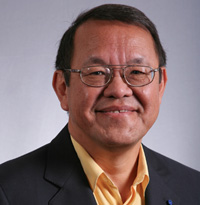
Delta Electronics Professor of EECS (Post-Tenure), [AI+D and CS]
- [email protected]
- (617) 253-8513
- Office: 32-G470
- Dean’s Office
- External Advisory Council
- Computing Council
- Extended Computing Council
- Undergraduate Advisory Group
- Break Through Tech AI
- Building 45 Event Space
- Infinite Mile Awards: Past Winners
- Frequently Asked Questions
- Undergraduate Programs
Graduate Programs
- Educating Computing Bilinguals
- Online Learning
- Industry Programs
- Postdoctoral Fellows Programs
- AI Policy Briefs
- 2024 Winners
- 2023 Winners
- SERC Symposium 2023
- SERC Case Studies
- SERC Scholars Program
- SERC Group Leaders
- Common Ground Subjects
- For First-Year Students and Advisors
- For Instructors: About Common Ground Subjects
- Common Ground Award for Excellence in Teaching
- New & Incoming Faculty
- Shared Faculty
- Faculty Resources
- Faculty Openings
- Newsletter Archives
- Search for: Search
- MIT Homepage
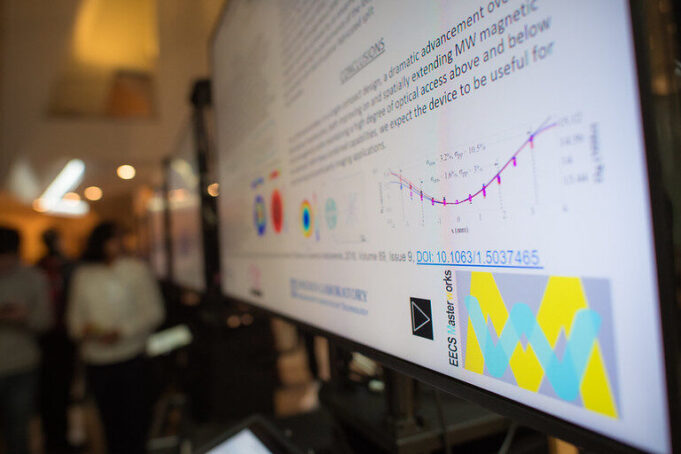
Below is a list of the MIT Schwarzman College of Computing’s graduate degree programs. The Doctor of Philosophy (PhD) degree is awarded interchangeably with the Doctor of Science (ScD).
Prospective students apply to the department or program under which they want to register. Application instructions can be found on each program’s website as well as on the MIT Graduate Admissions website.
Center for Computational Science and Engineering
The Center for Computational Science and Engineering (CCSE) brings together faculty, students, and other researchers across MIT involved in computational science research and education. The center focuses on advancing computational approaches to science and engineering problems, and offers SM and PhD programs in computational science and engineering (CSE).
- Computational Science and Engineering, SM and PhD . Interdisciplinary master’s program emphasizing advanced computational methods and applications. The CSE SM program prepares students with a common core of computational methods that serve all science and engineering disciplines, and an elective component that focuses on particular applications. Doctoral program enables students to specialize in methodological aspects of computational science via focused coursework and a thesis which involves the development and analysis of broadly applicable computational approaches that advance the state of the art.
- Computational Science and Engineering, Interdisciplinary PhD. Doctoral program offered jointly with eight participating departments, focusing on the development of new computational methods relevant to science and engineering disciplines. Students specialize in a computation-related field of their choice through coursework and a doctoral thesis. The specialization in computational science and engineering is highlighted by specially crafted thesis fields.
Department of Electrical Engineering and Computer Science
The largest academic department at MIT, the Department of Electrical Engineering and Computer Science (EECS) prepares hundreds of students for leadership roles in academia, industry, government and research. Its world-class faculty have built their careers on pioneering contributions to the field of electrical engineering and computer science — a field which has transformed the world and invented the future within a single lifetime. MIT EECS consistently tops the U.S. News & World Report and other college rankings and is widely recognized for its rigorous and innovative curriculum. A joint venture between the Schwarzman College of Computing and the School of Engineering, EECS (also known as Course 6) is now composed of three overlapping sub-units in electrical engineering (EE), computer science (CS), and artificial intelligence and decision-making (AI+D).
- Computation and Cognition, MEng*. Course 6-9P builds on the Bachelor of Science in Computation and Cognition to provide additional depth in the subject areas through advanced coursework and a substantial thesis.
- Computer Science, PhD
- Computer Science and Engineering, PhD
- Computer Science, Economics, and Data Science, MEng*. New in Fall 2022, Course 6-14P builds on the Bachelor of Science in Computer Science, Economics, and Data Science to provide additional depth in economics and EECS through advanced coursework and a substantial thesis.
- Computer Science and Molecular Biology, MEng*. Course 6-7P builds on the Bachelor of Science in Computer Science and Molecular Biology to provide additional depth in computational biology through coursework and a substantial thesis.
- Electrical Engineering, PhD
- Electrical Engineering and Computer Science, MEng* , SM* , and PhD . Master of Engineering program (Course 6-P) provides the depth of knowledge and the skills needed for advanced graduate study and for professional work, as well as the breadth and perspective essential for engineering leadership. Master of Science program emphasizes one or more of the theoretical or experimental aspects of electrical engineering or computer science as students progress toward their PhD.
- Electrical Engineer / Engineer in Computer Science.** For PhD students who seek more extensive training and research experiences than are possible within the master’s program.
- Thesis Program with Industry, MEng.* Combines the Master of Engineering academic program with periods of industrial practice at affiliated companies.
* Available only to qualified EECS undergraduates. ** Available only to students in the EECS PhD program who have not already earned a Master’s and to Leaders for Global Operations students.
Institute for Data, Systems, and Society
The Institute for Data, Systems, and Society advances education and research in analytical methods in statistics and data science, and applies these tools along with domain expertise and social science methods to address complex societal challenges in a diverse set of areas such as finance, energy systems, urbanization, social networks, and health.
- Social and Engineering Systems, PhD. Interdisciplinary PhD program focused on addressing societal challenges by combining the analytical tools of statistics and data science with engineering and social science methods.
- Technology and Policy, SM . Master’s program addresses societal challenges through research and education at the intersection of technology and policy.
- Interdisciplinary Doctoral Program in Statistics . For students currently enrolled in a participating MIT doctoral program who wish to develop their understanding of 21st-century statistics and apply these concepts within their chosen field of study. Participating departments and programs: Aeronautics and Astronautics, Brain and Cognitive Sciences, Economics, Mathematics, Mechanical Engineering, Physics, Political Science, and Social and Engineering Systems.
Operations Research Center
The Operations Research Center (ORC) offers multidisciplinary graduate programs in operations research and analytics. ORC’s community of scholars and researchers work collaboratively to connect data to decisions in order to solve problems effectively — and impact the world positively.
In conjunction with the MIT Sloan School of Management, ORC offers the following degrees:
- Operations Research, SM and PhD . Master’s program teaches important OR techniques — with an emphasis on practical, real-world applications — through a combination of challenging coursework and hands-on research. Doctoral program provides a thorough understanding of the theory of operations research while teaching students to how to develop and apply operations research methods in practice.
- Business Analytics, MBAn. Specialized advanced master’s degree designed to prepare students for careers in data science and business analytics.
- DACA/Undocumented
- First Generation, Low Income
- International Students
- Students of Color
- Students with disabilities
- Undergraduate Students
- Master’s Students
- PhD Students
- Faculty/Staff
- Family/Supporters
- Career Fairs
- Post jobs, internships, and fellowships
- Build your brand at MIT
- Recruiting Guidelines and Resources
- Connect with Us
- Career Advising
- Distinguished Fellowships
- Employer Relations
- Graduate Student Professional Development
- Prehealth Advising
- Academia & Education
- Architecture, Planning, & Design
- Arts, Communications, & Media
- Business, Finance, & Fintech
- Computing & Computer Technology
- Data Science
- Energy, Environment, & Sustainability
- Life Sciences, Biotech, & Pharma
- Manufacturing & Transportation
- Health & Medical Professions
- Social Impact, Policy, & Law
- Getting Started & Handshake 101
- Exploring careers
- Networking & Informational Interviews
- Connecting with employers
- Resumes, cover letters, portfolios, & CVs
- Finding a Job or Internship
- Post-Graduate and Summer Outcomes
- Professional Development Competencies
- Preparing for Graduate & Professional Schools
- Preparing for Medical / Health Profession Schools
- Interviewing
- New jobs & career transitions
- Career Prep and Development Programs
- Spring Career Night
- Employer Events
- Outside Events for Career and Professional Development
- Events Calendar
- Career Services Workshop Requests
- Early Career Advisory Board
- Peer Career Advisors
- Student Staff
Software Engineer, Data Platform (PhD or Masters)
- Share This: Share Software Engineer, Data Platform (PhD or Masters) on Facebook Share Software Engineer, Data Platform (PhD or Masters) on LinkedIn Share Software Engineer, Data Platform (PhD or Masters) on X
Software Engineer, Data Platform
About the Team/Role: WEX is an innovative global commerce platform and payments technology company looking to forge the way in a rapidly changing environment, to simplify the business of doing business for customers, freeing them to spend more time, with less worry, on the things they love and care about. We are journeying to build a consistent world-class user experience across our products and services and leverage customer-focused innovations across all our strategic initiatives, including big data, AI, and Risk.
Are you ready to make a meaningful impact while advancing your career? WEX is seeking a driven and talented mid-level Engineer to join our Data, AI, and Risk Technology (DART) organization—a dynamic team at the forefront of innovation, solving complex challenges, and delivering transformative business outcomes.
The DART team plays a pivotal role in unlocking the full potential of WEX’s rich and complex data, which powers a diverse range of customer businesses. With AI evolving at an unprecedented pace, this is your chance to harness the synergy of cutting-edge AI technologies and data to revolutionize our products, enhance customer experiences, and transform how we operate.
Our mission spans three critical areas:
Data Empowerment: Building platforms and tools to clean, process, and enrich core data, making it efficient and valuable for customers and internal teams.
AI Enablement: Driving seamless AI application development and operations to stay ahead in a rapidly advancing tech landscape.
Risk Management: Developing technologies that proactively prevent fraud, ensure accurate credit decisions, and safeguard our payment systems.
At WEX, we leverage state-of-the-art technologies and practices—big data, AI, risk management solutions, and agile methodologies—to deliver excellence and innovation at scale.
How you’ll make an impact:
- Meaningful Work: Solve high-impact challenges with real-world implications for our customers and business.
- Supportive Team: Collaborate with a team of talented engineers and leaders dedicated to helping you succeed.
- Career Growth: Accelerate your career by building expertise in cutting-edge tools, technologies, and best practices.
Responsibilities:
- Collaborate to Innovate: Partner with stakeholders to understand customer needs, uncover challenges, and design impactful solutions.
- Deliver Quality Engineering: Take ownership of designing, testing, coding, and monitoring systems with small to medium complexity, ensuring high-quality outcomes.
- Harness Data Insights: Use data to effectively drive informed decisions and measure performance.
- Build Seamless Pipelines: Develop and maintain CI/CD pipelines using tools like GitHub Actions to enable efficient and reliable deployments.
- Transform with IaC: Leverage Infrastructure as Code (IaC) tools such as Terraform to provision and manage scalable cloud-based infrastructure for data, AI, and risk applications.
- Adopt Modern Architectures: Apply TDD, BDD, and microservice or event-driven architectures to build resilient and scalable systems.
- Ensure Operational Excellence: Support live platforms and products, enabling proactive monitoring, rapid incident response, and continuous improvements for data quality and system reliability.
- Drive Optimization: Analyze data, systems, and processes to identify bottlenecks and implement enhancements that improve efficiency and scalability.
- Foster Learning: Mentor and learn from peers, sharing knowledge and cultivating a culture of continuous growth within the team.
- Solve Real Problems: Understand customer and business challenges to deliver effective, reliable, and scalable solutions with a strong focus on usability.
- Collaborate with Impact: Work closely with team members to tackle complex problems, offering and receiving feedback to improve quality and efficiency.
- Achieve Independence: Take on tasks of small to medium complexity independently while proactively seeking feedback from senior engineers to ensure excellence.
- Engage in Technical Growth: Actively participate in technical discussions, review peer contributions, and share knowledge to advance team expertise.
- Build at Scale: Design secure, reliable, and user-friendly platforms and tools for data, AI, and risk technologies, supporting diverse business needs.
- Master the Stack: Use common technologies, tools, and software packages effectively, ensuring seamless integration with WEX systems and adherence to best practices.
Experience you’ll bring:
- Educational Excellence: PhD in Computer Science (or related field) or a Master’s degree with 1+ years of hands-on software development experience.
- Problem-Solving Expertise: Demonstrated ability to tackle complex challenges and create practical, impactful solutions.
- DevOps Proficiency: Experience in CI/CD automation with tools and workflows for streamlined development.
- Programming Mastery: Strong skills in Java, C#, Golang, or Python, with a solid understanding of TDD, coding best practices, automated testing, and system monitoring.
- Passion for Innovation: Genuine interest in data, AI, risk, and cloud technologies, coupled with a drive to stay ahead of emerging trends.
- Customer-Centric Mindset: Enthusiasm for understanding and addressing customer and business challenges with thoughtful solutions.
- Eager to Learn: Highly motivated and curious, continuously exploring new technologies (e.g., GenAI) to improve productivity, quality, and innovation.
Bonus Skills: Familiarity with data pipelines, quality assurance, SQL, relational and graph databases, ELT, data warehouses, AI/ML, and anomaly detection technologies.

Academic Programs
- CSE PhD Overview
- Dept-CSE PhD Overview
- CSE Doctoral Theses
- Program Overview and Curriculum
- For New CCSE Students
- Terms of Reference
MIT Doctoral Programs in Computational Science and Engineering
The Center for Computational Science and Engineering (CCSE) offers two doctoral programs in computational science and engineering (CSE) – one leading to a standalone PhD degree in CSE offered entirely by CCSE (CSE PhD) and the other leading to an interdisciplinary PhD degree offered jointly with participating departments in the School of Engineering and the School of Science (Dept-CSE PhD).
While both programs enable students to specialize at the doctoral level in a computation-related field via focused coursework and a thesis, they differ in essential ways. The standalone CSE PhD program is intended for students who intend to pursue research in cross-cutting methodological aspects of computational science. The resulting doctoral degree in Computational Science and Engineering is awarded by CCSE via the the Schwarzman College of Computing. In contrast, the interdisciplinary CSE PhD program is intended for students who are interested in computation in the context of a specific engineering or science discipline. For this reason, this degree is offered jointly with participating departments across the Institute; the interdisciplinary degree is awarded in a specially crafted thesis field that recognizes the student’s specialization in computation within the chosen engineering or science discipline.
For more information about CCSE’s doctoral programs, please explore the links on the left. Information about our application and admission process is available via the ‘ Admissions ‘ tab in our menu. MIT Registrar’s Office provides graduate tuition and fee rates as set by the MIT Corporation and the Graduate Admissions section of MIT’s Office of Graduate Education (OGE) website contains additional information about costs of attendance and funding .

Computational Science and Engineering PhD
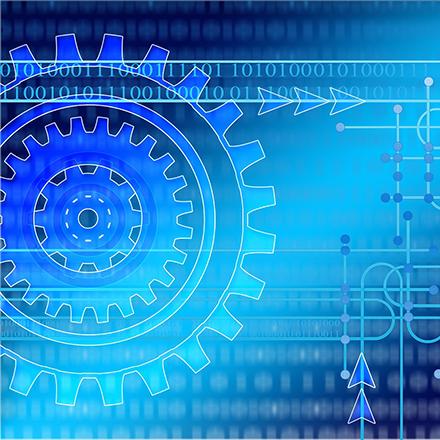
77 Massachusetts Avenue Building 35-434B Cambridge MA, 02139
617-253-3725 [email protected]
Website: Computational Science and Engineering PhD
Application Opens: September 15
Deadline: December 1 at 11:59 PM Eastern Time
Fee: $90.00
Note: Applicants interested in Computer Science must apply to through the Electrical Engineering and Computer Science PhD program .
Terms of Enrollment
Fall Term (September)
Standalone Program:
- Doctor of Philosophy (PhD) in Computational Science and Engineering
Joint Program:
- Doctor of Philosophy (PhD) in Civil Engineering and Computation
- Doctor of Philosophy (PhD) in Environmental Engineering and Computation
- Doctor of Philosophy (PhD) in Mechanical Engineering and Computation
- Doctor of Philosophy (PhD) in Computational Materials Science and Engineering
- Doctor of Philosophy (PhD) in Chemical Engineering and Computation
- Doctor of Philosophy (PhD) in Computational Earth, Atmospheric and Planetary Sciences
- Doctor of Philosophy (PhD) in Aerospace Engineering and Computational Science
- Doctor of Philosophy (PhD) in Mathematics and Computational Science
- Doctor of Philosophy (PhD) in Nuclear Engineering and Computation
- Doctor of Philosophy (PhD) in Computational Nuclear Science and Engineering
Affiliated Departments
- Aeronautics and Astronautics
- Chemical Engineering
- Civil and Environmental Engineering
- Earth, Atmospheric, and Planetary Studies
- Materials Science and Engineering
- Mathematics
- Mechanical Engineering
- Nuclear Science and Engineering
Standardized Tests
Graduate Record Examination (GRE)
- General test not required for Fall 2025 admission cycle
- Institute code: 3514
- Department code: 0000
International English Language Testing System (IELTS)
- Minimum score required: 7
- Electronic scores send to: MIT Graduate Admissions
TOEFL exam may be accepted in special cases. Waivers are not offered.
Financial Support
The CCSE PhD is an interdisciplinary program that collaborates with eight affiliated departments. As financial support may vary by department, CCSE graduate students are encouraged to contact their home department for more information.
Application Requirements
- Online application (including Subjects Taken section)
- Statement of objectives (limited to one page)
- Three letters of recommendation
- Transcripts
- English proficiency exam scores
- CV or resume
- GRE scores (not required for Fall 2023 admission cycle)
Special Instructions
The Computational Science and Engineering (CSE) PhD program allows students to specialize at the doctoral level in a computation-related field of their choice through focused coursework and a doctoral thesis. Applications from candidates who have a strong foundation in core disciplinary areas of mathematics, engineering, physics, or related fields are strongly encouraged.
Applicants interested in Computer Science: Please explore the offerings of the Department of Electrical Engineering and Computer Science.
This site uses cookies to give you the best possible experience. By browsing our website, you agree to our use of cookies.
If you require further information, please visit the Privacy Policy page.

IMAGES
VIDEO
COMMENTS
Doctoral Programs in Computational Science and Engineering Application & Admission Information. The Center for Computational Science and Engineering (CCSE) offers two doctoral programs in computational science and engineering (CSE) - one leading to a standalone PhD degree in CSE offered entirely by CCSE and the other leading to an interdisciplinary PhD degree offered jointly with ...
"Mechanical Engineering Student at MIT" is better, but doesn't tell a recruiter if you have the experience or interest they're looking for. Consider fuller headlines like "MIT Mechanical Engineer with a Passion for Building Medical Devices" or "Software Developer, C++ Expert and MIT Computer Science Grad." 2.
MIT Master of Science Program in Computational Science and Engineering (CSE SM) ... Alumni of CCSE's graduate programs are working throughout the world in both academia and industry, from small start-ups to large global corporations. We encourage our alumni to join our LinkedIn group to stay connected with the CCSE community, on campus and ...
The interdisciplinary doctoral program in Computational Science and Engineering (PhD in CSE + Engineering or Science) offers students the opportunity to specialize at the doctoral level in a computation-related field of their choice via computationally-oriented coursework and a doctoral thesis with a disciplinary focus related to one of eight ...
Artificial Intelligence and Decision-making combines intellectual traditions from across computer science and electrical engineering to develop techniques for the analysis and synthesis of systems that interact with an external world via perception, communication, and action; while also learning, making decisions and adapting to a changing environment.
Graduate Student Professional Development; Prehealth Advising; Career Interests. ... AI in Hiring Trends on Facebook Share MIT Podcasts: AI in Hiring Trends on LinkedIn Share MIT Podcasts: AI in Hiring Trends on X; Copy Link; News & Articles Published on May 18, 2023 ... MIT Computer Science & Artificial Intelligence Lab 6-A Program View All ...
Computer Science and Engineering, PhD; Computer Science, Economics, and Data Science, MEng*. New in Fall 2022, Course 6-14P builds on the Bachelor of Science in Computer Science, Economics, and Data Science to provide additional depth in economics and EECS through advanced coursework and a substantial thesis. Computer Science and Molecular ...
Educational Excellence: PhD in Computer Science (or related field) or a Master's degree with 1+ years of hands-on software development experience. Problem-Solving Expertise: Demonstrated ability to tackle complex challenges and create practical, impactful solutions.
The Center for Computational Science and Engineering (CCSE) offers two doctoral programs in computational science and engineering (CSE) - one leading to a standalone PhD degree in CSE offered entirely by CCSE (CSE PhD) and the other leading to an interdisciplinary PhD degree offered jointly with participating departments in the School of Engineering and the School of Science (Dept-CSE PhD).
77 Massachusetts Avenue Building 35-434B Cambridge MA, 02139. 617-253-3725 [email protected]. Website: Computational Science and Engineering PhD. Apply here