
An official website of the United States government
The .gov means it’s official. Federal government websites often end in .gov or .mil. Before sharing sensitive information, make sure you’re on a federal government site.
The site is secure. The https:// ensures that you are connecting to the official website and that any information you provide is encrypted and transmitted securely.
- Publications
- Account settings
Preview improvements coming to the PMC website in October 2024. Learn More or Try it out now .
- Advanced Search
- Journal List
- HHS Author Manuscripts


Detecting, Preventing, and Responding to “Fraudsters” in Internet Research: Ethics and Tradeoffs
Jennifer e. f. teitcher.
Research assistant for Dr. Robert Klitzman at Columbia University
Walter O. Bockting
Professor of Medical Psychology (in Psychiatry and Nursing) at Columbia University Medical Center and a Research Scientist with the New York State Psychiatric Institute
José A. Bauermeister
John G. Searle Assistant Professor of Health Behavior and Health Education (HBHE), and Director of the Center for Sexuality & Health Disparities (SexLab) at the University of Michigan School of Public Health
Chris J. Hoefer
Research Project Coordinator at the Program in Human Sexuality within the University of Minnesota Medical School
Michael H. Miner
Professor and Director of Research at the Program in Human Sexuality, Department of Family Medicine and Community Health at the University of Minnesota Medical School
Robert L. Klitzman
Professor of Psychiatry and the Director of the Masters of Bioethics Program at Columbia University
Research that recruits and surveys participants online is increasing, but is subject to fraud whereby study respondents — whether eligible or ineligible — participate multiple times. Online Internet research can provide investigators with large sample sizes and is cost efficient. 1 Internet-based research also provides distance between the researchers and participants, allowing the participant to remain confidential and/or anonymous, and thus to respond to questions freely and honestly without worrying about the stigma associated with their answers. However, increasing and recurring instances of fraudulent activity among subjects raise challenges for researchers and Institutional Review Boards (IRBs). 2 The distance from participants, and the potential anonymity and convenience of online research allow for individuals to participate easily more than once, skewing results and the overall quality of the data.
Duplicate entries not only compromise the quality of the research data, but also impact the studies’ budgets if not caught before participants’ payment — a growing concern with decreasing NIH funding lines. Though reports have begun to explore methods for detecting and preventing fraud, 3 the ethical issues and IRB considerations involved have received little systematic attention. Researchers and IRBs may be unfamiliar with these issues and thus be overly restrictive or lax with Internet research protocols.
In the past, researchers have identified several problematic patterns: 1) eligible individuals who take a study twice, presumably without malicious intent; 2) eligible individuals who take a study repeatedly to receive additional compensation; and 3) ineligible individuals who take a study once or repeatedly to profit from compensation. 4 Despite using methods to detect and prevent fraud, a recent study of transgender and sexual health conducted by Swinburne Romine et al. nonetheless, uncovered more serious fraudulent behavior. Specifically, these researchers found that individuals with IP addresses from China participated in the study by creating fake IP addresses and providing U.S. home addresses that, upon review, were not residential locations. 5 These “fraudsters” may not themselves have been in China, but may have routed these IP addresses through that country in order to avoid detection. Nonetheless, after Swinburne Romine et al. first encountered this problem in 2011–2012, the media has revealed widespread hacking activities from that country. 6 Given these phenomena, we decided to review the literature in light of increasing use of online surveys in academic research and potential fraud by survey participants.
Early studies regarding Internet-based research suggested that multiple submissions were a valid concern but were rare, 7 below 3% in most studies. 8 Reasons given for duplicate responses to surveys were not due to malicious intent, but rather to the respondents’ curiosity of how his or her results may change if s/he gave different answers, 9 entertainment (such as a fun game or intellectual challenge), and beliefs that providing more data — even if duplicate — would aid the researchers. 10 Prevention strategies have been recommended — such as providing a link to allow respondents, if they want, to continue to participate without the responses counting toward the data, and simply requesting respondents not to participate more than once. 11 But these strategies do not deter participants with malicious intent from repeatedly entering a study. Reips mentions that high incentives may increase multiple submissions, 12 and Mustanski states that different forms of compensation (direct, lottery, or a donation to charity of choice) may lead to multiple entries, as well as that current prevention strategies are ineffective deterrences, 13 yet they both fall back on the assumption that fraudulent behavior is “extremely rare.” 14 Birnbaum writes that providing compensation or a prize can lead to multiple entries for additional compensation or higher chances at winning a prize. He suggests that merely stating that participants will only be compensated once for their participation is a possible solution, but he does not take into account sophisticated and/or malicious “fraudsters.” 15
Ten years ago when these articles were written, incentives were rarely used. 16 But over the past decade, as response rates have decreased, incentives have become more frequent. 17 According to a meta-analysis by Göritz, participants receiving an incentive were 19% more likely to respond and 27% more likely to complete an online survey than those who did not receive an incentive. 18 Additionally, incentives have been shown to boost retention rates in longitudinal studies. 19 However, monetary compensation seems to be increasing both response rates and multiple submissions. 20
We have found only five sexual health studies that have examined the frequency of multiple submissions. The percentages of entries that were multiple submissions were, respectively, 10% (of which 55% were from the same person), 21 8% among young men who have sex with men (YMSM), 22 16% among a sample predominantly of heterosexual young adults, 23 and approximately 33% of the submissions (of which 51% of multiple submissions were from subjects who participated between 11–67 times). 24 In a recent study conducted by Bauermeister, of the 2,329 YMSM participants who seemed eligible and completed the study, 15% of entries were multiple submissions. 25 Bowen et al. concluded that participants eligible for reimbursement were six times more likely to engage in repeated responses than those who were not offered compensation. 26
Discussions concerning the ethics of online research often focus on protecting participants’ confidentiality to encourage them to trust the researchers. 27 But critical problems can also arise concerning researchers’ abilities to trust the participants. Methods of detection and prevention of both duplicate submissions and fraudulent behavior are at times the same, while at other times they are different. Hence, we will discuss both duplicate submissions and fraud below, but highlight issues pertaining to “fraudsters” — those who are ineligible for studies and participate solely for compensation.
Methods for Detecting and Preventing Fraud
In brief, as indicated in Table 1 and described below, several possible methods exist for detecting and preventing fraud, each with pros and cons, and logistical and ethical questions and implications. Researchers can detect and prevent Internet research fraud in four broad ways: at the level of the questionnaire/instrument, the participants’ non-questionnaire data and external validation, computer information, and study design. Researchers and IRBs face ethical questions of whether to report “fraudsters” to external authorities, and whether and how to include these methods in an informed consent form.
Methods of Detecting and Preventing Internet Study Duplication and Fraud and Their Implications
Level of Intervention | Type of Intervention | Method of Detection | Method of Prevention | Pros | Cons | Additional Ethical Issues |
---|---|---|---|---|---|---|
Questions in Survey | Inconsistent Reponses | Check for proper/consistent answers | Subjects may skip questions because of discomfort | |||
Include same/similar/strange questions throughout study | Indicates level of attention | Can impact experimental design | ||||
Include questions of social desirability | Possibly help assess personality traits associated with providing inaccurate responses | |||||
Software for Administering Survey | No back button | Subjects can’t easily resubmit survey | ||||
Change order of questions with each administration | ||||||
CAPTCHA | Detects “bots” | |||||
Collect paradata (i.e., subject’s behavior, e.g., time stamp, how mouse moved on the screen) | Examines how subject responding to survey | Programs that allow tracking of paradata are costly | Ethical questions of what we can see with paradata – whether to disclose to participants what we can see of their behavior | |||
Tracking Non-Questionnaire Data | Personal Information | Similar/same email, username, password between “different” participants | Contact participant about “red flag,” and if no response, remove from study | Clears up misunderstandings | email, username, password | Needs to balance protecting integrity of data and subject privacy and confidentiality are particularly important |
Inaccurate/fake address & phone numbers | Researchers request to provide phone number/address to get through registration process | Participants need valid number in order to proceed | “Fraudsters” can create temporary phone numbers | |||
Check whether person, address, phone number is valid (through Facebook, whitepages.com, etc.) | ||||||
Ask participants for a website where they are listed (e.g., Facebook) | May deter “fraudsters” and multiple submissions | |||||
Computer Information | IP Addresses | Same IP as another participant | Check whether IP address is the same or if it is encrypted | Can determine how many times participants took survey and whether participant fulfills location criteria (i.e., living in US) | ||
Block IP address if participant is ineligible | Avoids “fraudsters” from participating | Could be dynamic IP address and not ineligible participant | ||||
Internet Cookies | Cookies detecting completion of study and multiple attempts access study | Enable cookies | Can detect multiple submissions by tracking the progress/completion of study | |||
Tracking Survey URL | URL posted in unintended locations | Tracking/Googling URL on Internet | Can see if website where URL located is targeting proper audience | Doesn’t prevent “frausters” taking study multiple times | ||
Provide link in email to website and track referring URL | Researchers don’t always know the targeted population | |||||
Study Design | Informed Consent | Break up consent online and only provide compensation information at the end of all the forms | May deter eligible pa1rticipants | |||
Compensation | Many gift certificates mailed to same address | Mention that subjects will not be compensated if suspect of fraudulent behavior | Avoids paying “fraudsters” yet keeps incentive | |||
Only inform participants of their eligibility for the study after survey | ||||||
Ask for mailing address (vs. email address) and verify addresses | Deters ineligible participants if researchers have means to verify addresses | May deter eligible participants (because of need to provide personal information) | ||||
Check if multiple gift certificates are being sent to one location | Can avoid paying participants if suspected of fraudulent behavior yet keeps incentive | Linking identification to data can threaten confidentiality | ||||
De-incentivize fraud by paying less and/or emphasizing research and the importance of social/community costs of fraud | Potential “fraudsters” may be persuaded not to skew results | |||||
Provide lottery for compensation (do not pay every person) | Gives researchers time to review and determine “fraudsters” before compensating | |||||
Including Interview | See whether subject already participated and/or is lying on responses | Audio Interview | Needs to balance protecting integrity of data and subject privacy and confidentiality are particularly important | |||
Skype/”face-to-face” Interview | ||||||
IRBs | IRB Structure | Having an online/computer expert as a member of the IRB | Does not deter “fraudsters” from taking survey multiple times | |||
Have PIs Report Information on “Fraudsters” to IRB | IRBs can follow and monitor to make appropriate decisions for current and future studies | May deter “fraudsters” from participating | ||||
Broader Regulatory and other Entities | Reporting Information on “Fraudsters” | PIs create “fraudster” list for other PIs and share information | “Fraudsters” can create new names, emails, IP addresses for each study to avoid detection as a “fraudster” | Possible harm of individuals are incorrectly classified as “fraudsters” and reported externally? Need to ensure that characterization as “fraudster” is accurate | ||
Reporting fraudulent behavior to Internet Crime Complaint Center (IC3.gov), OHRP or funders | May deter “fraudsters” from participating |
Questionnaire/Instrument Level
Questions in survey.
Researchers have suspected fraudulent behavior from the inconsistent responses participants provide. 28 For example, Romine et al. excluded participants whose ages did not match birth dates or whose answers to questions about sex, gender, and sexuality were inconsistent (e.g., I was born with a penis/I have had genital reconstructive surgery/I still have a penis; I have had insertive vaginal sex with multiple female partners/None of my partners have vaginas). 29 Researchers can also check that participants have not answered in an “All or Nothing” manner (i.e., answering all 0s or 6s in a survey, or following other patterns of responding [e.g., 1,2,3,4,1,2,3,4]), 30 or skipped large portions of the survey. However, participants may skip questions due to discomfort answering particular questions, and not necessarily due to fraudulent behavior. Nevertheless, examining the types of questions skipped, and how those questions were answered could be helpful in determining discomfort or lack of attention. Similarly, Nosek et al. suggest including choices to survey questions that are not likely to be true. 31 Participants who are not taking the survey seriously may be more likely to select an odd response, though this strategy should be used sparingly, as it may impact the experimental design.
Including questions about social desirability/sociopathy could potentially identify personality traits correlated with providing inaccurate responses. 32 However, tests of such personality traits may have low, if any, predictability for intentional fraud behavior, as “fraudsters” may not respond to these questions honestly.
Lastly, some entries can be submitted by “bots,” instead of individuals. “Bots,” short for “robots,” are a type of software application that can perform automated tasks over the Internet at a much quicker pace than individuals can. Thus, “bots” can fill out surveys quickly and repeatedly, allowing for the bots’ programmers to complete surveys and receive additional compensation quickly. For example, in 1999, Slashdot.com created an online poll asking which was the best graduate school in computer science. Students at Carnegie Mellon and MIT wrote a voting program using “bots” to complete the ballots, resulting in over 21,000 votes for each of these schools, while every other school submitted fewer than 1,000 votes. 33 Similarly, Bauermeister has conducted studies where their own system detected “bots” after flagging rapid re-entries into the system from the same IP address and randomized answer patterns from these entries. As suggested above, researchers can review inconsistent answers (though often needing to do so by hand) to remove submissions from “bots” as well as “fraudsters.”
Software for Administering Surveys
Online survey software can be engineered to help prevent Internet fraud. Disabling the back button on the web-browser can prevent “fraudsters” from going back through the survey and revising and resubmitting their responses easily. However, legitimate participants may change their mind about an answer upon greater reflection, and may legitimately want to alter a previous response but would be unable to do so. To solve this issue, the survey could be constructed to allow respondents to review answers periodically. Investigators can also construct the survey to change the order of the questions with each administration, so answers that do not match the questions would be flagged as suspicious.
“Bots” are also commonly prevented by Completely Automated Public Turing test to tell Computers and Humans Apart (CAPTCHA), which frequently requires the user to type in letters and numbers from a distorted image that a computer cannot copy, to ensure that the respondent is indeed a person and not a “bot.” This approach, however, may decrease the participation of individuals with low computer literacy, who have visual disabilities (though some CAPTCHA programs offer an audio/sound option), and/or have outdated computer systems that do not work appropriately with CAPTCHA. 34 Additionally, not all CAPTCHA codes are secure, allowing “bots” to invade the system. Some CAPTCHA codes are also used frequently, motivating programmers to create “bots” that can bypass these common CAPTCHAs. 35
Researchers can check other information beyond what participants provide through the survey’s technology. Reviewing the administrative data, also known as paradata, on each subject’s behavior can indicate if participants paid attention to the content of the questions or changed answers, potentially shedding light on whether the participant is confused or deliberately being dishonest. 36 A researcher can look at the timestamp, the length of time it took participants to take the study, the ways their mouse moved on the screen, the deletions or changes in their answers, and more. Miner, Bockting and colleagues removed submissions if participants took fewer than 30 minutes to complete the survey, or fewer than 19 minutes to complete the three most important portions of the survey. 37 These cut-offs were based on the overall distribution of respondents’ completion times. In each case the cut-off was set at greater than two standard deviations from the mean completion time.
It is important to note, however, that paradata are generally included in relatively costly, private survey programs such as Sawtooth Software, 38 and not accessible through other, “free” survey systems, such as SurveyMonkey. 39 With Sawtooth Software, for example, only researchers have access to participants’ information (paradata and other information) as the data may be deposited into the researchers’ own data server. 40 Easily accessible online survey tools like SurveyMonkey, on the other hand, may store the information in their data servers and in their terms of agreement may include their right to review participants’ data. 41 Hence, the researchers are not the only ones with access to this information — raising concerns regarding the survey’s confidentiality when used in these systems. Other public surveys like Qualtrics may store the paradata for free, yet for a fee allow the researchers alone to store and access these data. 42 Consequently, researchers and IRBs must be cautious of which survey service is used to avoid breaches in data safety and security.
Tracking Participants Non-Questionnaire Data
As discussed more fully below, investigators can obtain additional information about participants outside the questionnaire in the form of general information (username, password) or through the computer (IP addresses, cookies). These methods each pose both similar and different ethical issues.
Personal Information
Similar or same email, username, and/or passwords among participants.
Investigators can check for the same or similar email addresses, usernames, or passwords among participants in the study. Effective cross-referencing may reveal that a username in one entry is similar to an email address in another entry. However, certain common usernames or passwords among participants (e.g., 123456) may not indicate suspicious activity, 43 but may, in fact, be a way for subjects to take part in the study without providing personal information. Removing all such frequent usernames and/or passwords as duplicates from the study could thus result in losing important data. Moreover, “fraudsters” may have multiple, dissimilar, valid email addresses that researchers would not be able to detect. Other means of detection would thus need to be used.
To ensure valid entries are counted while avoiding “fraudsters,” researchers can also contact participants about the duplicate entries to clear up any misunderstandings that might have occurred. Bowen et al. deactivated accounts that were identified as multiple submissions and participants were sent a message to contact the project due to a problem with their account, and no subjects asked to be reactivated. 44
However, contacting participants about “red flags” can dissuade eligible participants, and/or yield a response bias, and risk excluding valid data. Additionally, contacting participants can reveal to “fraudsters” the methods researchers use to detect fraud, thus helping the “fraudsters” to cheat the system more effectively. Researchers may find it advantageous not to reveal explicitly what was flagged as suspicious, so that fraudulent participants will not know how researchers detected the fraudulent behavior.
Phony Address/Phone Number/Birth Date — External Validation
Checking the name, address, phone number, and age and birth date of participants to determine whether participants provided accurate and unique information can prevent both ineligible participants from taking part in the study and eligible participants from taking part multiple times. 45 Yet participants can provide friends’ addresses or phone numbers or a work address or phone number to appear as two different participants, or provide fake addresses and phone numbers. 46 Similarly, in Romine et al., phone numbers were required to complete the registration process (an automated robocall to their number of record then provided a PIN that would allow the participant to continue with the registration process), yet “fraudsters” set up and used temporary Google numbers to circumvent this step. 47
Additionally, investigators can confirm subjects’ eligibility through external validation such as looking up the individual through publicly available search engines, or checking websites such as Facebook or LinkedIn. Bauermeister’s study found that using Facebook and MySpace were most helpful in straightening out suspicious data. However, participants did not always have an account for verification, and sometimes privacy restrictions were activated or the profile was associated with a different email address. 48 Researchers can also use Google Earth/Google Maps, whitepages.com, Accurint (which has access to individual’s driver’s licenses and birthdates, among other records), and NCOA (National Change of Address, a database of changes of address that have been filed) to determine and confirm valid addresses and phone numbers. Unfortunately, eligible participants may be discouraged from taking part in the study if researchers look at information beyond what participants provide for the study. A solution to this issue could be to make providing personal information optional. Bowen et al. requested that participants include their phone numbers for follow-up and retention, yet this request was optional. Bowen and colleagues then used “reverse look-up” on the Internet to determine whether the phone number was valid. 49 Providing optional personal information may be a good way to facilitate participation since eligible subjects can remain anonymous and comfortable. But fraudulent participants may also opt-out of providing information that might identify them as ineligible.
To confirm eligibility, investigators can ask participants to provide a website where they are listed. This request can deter ineligible participants from taking the survey and deter eligible participants from taking the survey more than once, since they cannot assume another identity without proof. However, both eligible and ineligible participants can provide fake information (creating a fake Facebook account, for example) which would “confirm” eligibility yet be completely inaccurate. 50 Moreover, eligible participants may also be deterred from participating.
Publicly-available online information about subjects, if collected without interacting with an individual, would presumably not be considered human subject research, and would not require informed consent. Thus, examining outside sources might appear similar to Humphreys’ tearoom trade study, where he collected individuals’ license plates without informing them, obtained their names and addresses and contacted them. However, Humphrey’s study was deemed unethical in part because the researcher collected data on individuals without their consent in order to identify and later contact these individuals. 51 Collecting information on individuals separate from what is collected as part of the survey would not be used to gather identifying information that subjects wish to withhold, as was the case with Humphrey’s study. But questions nevertheless arise as to whether subjects should be told that such information would be collected. Individuals who make information publicly available on the Internet presumably should not have expectations that the information is private and confidential. Nonetheless, these individuals may mistakenly think that information they provide online is private, when that is not in fact the case (e.g., companies may sell data on online customer purchasing behavior). These individuals may also scroll through and unwittingly accept legal agreements that limit their privacy, but not understand these legal statements. Researchers could also include in the consent form that they will be seeking external validation of subject information.
These strategies raise questions of what is considered personal identifiable information. As Ohm points out, providing date of birth, sex, and zip code — three seemingly vague, innocuous descriptions — can accurately identify a person 87% of the time. 52 Participants might be hesitant to provide potentially identifying information, especially if the questions are sensitive or personal; hence researchers must be careful to ensure participants of the confidentiality of information to encourage eligible subjects to participate.
Computer Information
Ip addresses.
Researchers can detect multiple entries through tracking the IP address of the computer used to take the survey. Investigators can see how many times the participant took the survey and whether the participant meets geographic location eligibility (i.e., a survey may only want to study residents of the U.S.; IP addresses would reveal the participants’ geographic location). If researchers see an IP address used by many participants, or an IP address from the wrong geographic location, researchers can identify those participants and block those IP addresses, thus preventing participants from taking the study again. 53
However, problems arise when multiple eligible participants complete the survey from the same computer (e.g., roommates), or a study is being conducted on a large campus where students on the network receive the same IP addresses at different points in time. 54 Some companies that offer internet connectivity at home may also have rotating IP addresses for an area. Consequently, depending on a given day, a home may have two different IP addresses. Without fixed IP addresses, one participant may have different IP addresses, creating problems in determining whether entries are from “fraudsters” or are merely a single individual with an IP address that legitimately rotates. Additionally, eligible participants may be traveling outside a required geographic location while taking the study, in which case a foreign IP address will show up, raising unnecessary red flags. Yet respondents could potentially be asked if the computer they are using is not their usual one, and if so, why. Bauermeister and colleagues, as well as Bowen et al., used other prevention techniques to determine if entries with the same IP address were valid (time completion, asking how many people use the computer, etc.), and concluded that some were indeed valid entries with duplicate IP addresses. 55
In addition, IP addresses can be encrypted, scrambled or even faked; “fraudsters” can obtain a U.S. IP address in a different country, preventing researchers from knowing exactly where the participant is, and whether s/he has taken the survey multiple times. Indeed, after further examination in the Romine study of transgender individuals in the U.S., IP addresses were registered to people from China who fit the study’s category of “fraudsters.” While it was not certain where some of the other “fraudsters” were located, the researchers realized that these individuals were making efforts to produce multiple false records. This realization prompted the researchers to review the demographic information that was provided and determine fake addresses in order to systematically remove these participant records. 56 Similar to paradata, there are costly tracking systems that can determine if someone is re-routing an IP address.
Researchers’ examination of IP addresses poses several ethical questions. Researchers may deem a participant’s first entry valid, and the subsequent entries as duplicates or fraudulent. Yet, researchers should consider whether the first entry should be deemed valid, as it may not be an eligible participant submitting multiple times, but rather an ineligible “fraudster.” By reviewing the results both with and without the first entry, researchers can see how the entries impacted the data.
Additionally, while the United States does not consider IP addresses to be personal information/identification (except for HIPAA purposes), 57 the European Union does. 58 European participants may not want to participate if IP addresses will be tracked, posing problems in conducting research internationally. Researchers may thus be limited in their ability to track IP addresses and face questions of whether to list such tracking in the consent form. Anecdotally, some IRBs have initially been wary of researchers collecting IP addresses, viewing this information as identifying and unnecessary for answering the research questions per se. In a study conducted by Bauermeister, the IRB first discouraged researchers from tracking IP addresses (despite the fact that the U.S. does not consider IP addresses to be personal information/identification). Upon explaining to the IRB the need for this personal data, the IRB agreed but required the researchers to include in the consent form that IP addresses would be tracked. Yet researchers and these committees should consider the possibilities that collection of this information is justified in order to ensure research integrity, and hence scientific and social benefits. A balance of what to track and how to convey this information will be discussed later.
Internet Cookies
Internet cookies are bits of data sent from a website that are stored in an individual user’s web browser while the user is visiting that website. Each time the individual user accesses the site, the browser sends the cookie back to the website with information about the user’s previous activity. Cookies can also detect if an individual has accessed and/or completed a survey, as well as track the URL to determine from where online participants accessed the survey. If the individual attempts to access the website from the same browser, the cookies can detect if the individual has completed the survey and can note additional attempts to complete the survey.
Researchers can also provide a link to the survey exclusively in an email, thereby controlling the number of times participants can access the survey, as cookies can show researchers the number of times on which a link was clicked, and investigators can thus detect “fraudsters.” Van Gelder et al. suggested recruiting a targeted population via email with a link to a password-protected study in the email. 59 A username would be assigned to each individual who received the email, so that multiple entries could be prevented, and recruiting a targeted population would obclude “fraudsters” from participating.
Relying on cookies presents several challenges. Participants can access the survey from different browsers or delete the cookies stored on their computers, preventing researchers from knowing whether participants have taken the study multiple times. Furthermore, if multiple usernames/emails are provided, cookies would not be able to detect multiple submissions from the same user. Cookies can also reveal and identify someone as a participant in a study; for instance, parents may check the cookies of their teen’s computer and see that s/he participated in an LGBT survey. Regarding recruitment via email, Van Gelder et al. suggested that IRBs may be disinclined to recruit participants via individualized email, 60 and/or researchers may not know in advance the email addresses of all the potential participants (e.g., conducting a study on groups that are not easily identified, such as many substance abusers).
Additionally, investigators can enable cookies to be stored on subjects’ hard disk on their computers without the subjects’ knowledge or consent. Alternatively, some websites issue a pop-up before the user accesses any of the website’s contents, noting that by continuing to use the website, the individual agrees to accept cookies on the website. While enabling cookies may assist in detecting “fraudsters” and multiple submissions, informing participants of cookies may discourage eligible subjects from participating.
Similar to IP addresses, enabling cookies may prevent eligible participants who live together or share a computer from participating, if the researcher’s software detects that the study has already been conducted from the shared computer. If multiple individuals use the same computer, researchers should decide if cookies should be enabled. If so, the researchers will in effect only be able to include one participant from each shared computer, losing eligible participants.
Tracking Survey URL
Tracking the referring URL and/or searching for the URL online can show researchers if the enrollment site has been posted elsewhere. There are websites that post links to studies for users intending to earn easy money (such as paidsurveysonline.com , onlinejunkie.com , ranksurveys.com and swagbucks.com ), 61 so knowing where the URL has been posted allows researchers to see where participants are hearing about the study and researchers can then act accordingly to have the re-posting taken down. This situation in fact occurred in the Romine study: participants notified the researchers, sending screen captures of a chat room where users were mocking and planning to fraud the study. 62 While this method does not prevent eligible participants from taking the study multiple times, it controls where the study is advertised and can help avoid ineligible participants.
Study Design Level
Elements of the study’s design, such as breaking up the consent form, controlling how participants are compensated, and including a face-to-face, online chat or Skype interview as part of the study, can help prevent Internet research fraud.
Informed Consent
Investigators can provide the informed consent form online not as one long document, but instead as separate sections and webpages requiring the participants’ consent for each section of the form as it appeared on the screen. The compensation component of the informed consent would be listed at the end. Researchers can have the order of consent options (YES, I agree vs. NO, I don’t agree) randomized at each page. This process requires participants to pay more attention to what they are clicking, and creates a longer process to receive the compensation, as opposed to scrolling down quickly through the consent form and “consenting” to the study. These mechanisms can also help reduce “bots” from entering the system. Additionally, not knowing the compensation initially may discourage some “fraudsters” from participating, as they may find that the time is not worth it, given that the amount of compensation is not clear initially, though eligible participants may also be discouraged if the survey is too long and compensation is unknown. While this new structure of the consent form does not detect “fraudsters” or multiple submissions, it can help prevent these situations from initially occurring.
Compensation
Altering the amount, description or type and timing of compensation can also help prevent fraudulent activity. Studies have suggested that lowering incentives would lower fraudulent behavior. 63 Researchers may also be able to de-emphasize the incentive by paying participants less money, or emphasizing the social and community benefits of the study and the costs of fraud. By focusing on the importance of the research and the costs of fraud, some participants may feel less inclined to submit duplicates or falsify results. Bauermeister et al. sent out an email post-survey about the harmful effects of fraudulent behavior in studies to participants suspected of fraudulent behavior and two of the participants apologized. 64 The note stated:
Dear Participant, We appreciate your interest and willingness to complete our survey. Unfortunately, we noticed irregularities during data collection. Specifically, a few individuals chose to provide false data, refer ineligible individuals, and/or create multiple entries so that they may receive one or more incentives. We cannot underscore how disappointing this has been for us. Legally, this behavior constitutes fraud. As public health practitioners, we strive to collect quality and robust data through research that will inform smoking prevention and sex education programs for young women. False data diminishes our ability and actually harms the population that we seek to help through science and social services. We hope that similar events will not occur in future efforts. It is only through the honesty, integrity, and willingness of participants that we can help to contribute to the health of our communities. If you are receiving this message, you will not receive an incentive; however, if you think that this e-mail is a mistake, please feel free to call us during regular business hours.
However, lowering incentive may also lower participation rates. In addition, some “fraudsters” may not care about the costs of fraud.
Instead of paying all participants in the study, researchers can alternatively provide a lottery for compensation, whereby a smaller number of participants are randomly chosen to receive a larger amount of compensation. This mechanism can also give researchers time to review and identify fraudulent participants before sending out compensation. But “fraudsters” may take the survey multiple times to increase their chances of winning. 65
Other prevention methods include stating that participants will not be compensated if they are found by the researchers to have submitted duplicate and/or ineligible entries. Researchers can also monitor whether multiple gift certificates are being sent to one location. In Romine’s study, the sales representative from giftcertificates.com was able to provide redemption reports that allowed research staff to confirm when a single email address redeemed excessive certificates. 66
Investigators can ask participants, too, for a mailing address instead of an email address in order to verify legitimate residential location, detering participants from providing phony email addresses. However, providing personal information, which can also link identification to data, might discourage eligible subjects from participating. Rosser and colleagues allowed participants to choose their method of payment to accommodate respondents’ comfort levels with anonymity, 67 yet this method would make identifying “fraudsters” more difficult.
In addition, investigators can delay compensation for initial or follow up portions of the studies, giving researchers time to review and determine which participants are fraudulent before sending out compensation. Providing compensation at follow-up portions of a study rather, or proportionally more, than at baseline may increase response and retention rates, and delayed gratification of compensation may also de-incentivize people from answering a survey multiple times. As discussed below, empirical research is needed to examine the potential effectiveness of these approaches.
Including Interview
Researchers can include an interview component to the study via online written, audio, or video chat (e.g., Skype). 68 Face-to-face interviews may be difficult to arrange as participants may be spread out geographically and even across different states or countries. Furthermore, Skype/videochat interviews may be more effective than written chat or audio-only interviews not only for potentially facilitating and enhancing qualitative interviews, but perhaps also for screening purposes. Such interviews provide another possible means to deter or detect lying, but may also deter eligible individuals from participating, as anonymity may be less pronounced. Moreover, interviews are not a foolproof system as “good liars” may be hard to detect. 69
Taking Action against “Fraudsters” Outside the Study
Questions arise as to whether researchers and/or IRBs ever need to report cases of fraud to others, and if so, when and to whom. Researchers could, for instance, communicate with other researchers to share information about specific “fraudsters” — i.e., to make a database. Mentioning the possibility of such a database in the informed consent forms might dissuade “fraudsters” but also may dissuade legitimate participants. However, such a database can potentially be useful. On the other hand, “fraudsters” may create unique fictitious online identities for each study, such that the names, emails, and IP addresses they provide may not be repeated among studies. Nonetheless, as more online studies are conducted, the numbers of “fraudsters” will presumably continue to pose problems, and these other methods may be worth studying for effectiveness. Investigators can assess, for instance, how often they detect identical information from “fraudsters” in different studies.
Once researchers identify fraudulent behavior, they face additional decisions. Questions emerge of whether, in extreme circumstances, researchers may want to file a complaint with the Internet Crime Complaint Center (IC3.gov) — a section of the FBI that deals with Internet crimes 70 — and include a warning in the consent form that reporting may occur. Such a warning could powerfully deter fraudulent behavior, but may frighten eligible participants, who may wonder whether researchers may extend government reporting to include other illicit activities (e.g., drug use). Further scholarly discussion and debate is needed to determine what behaviors, if any, might warrant such action (e.g., if individuals went to great lengths to defraud researchers of government funds).
Certificates of confidentiality (CoCs) from the National Institutes of Health (NIH) are intended to help investigators protect data from involuntary disclosure if subpoenaed by a court. Yet the potential usefulness and limitations of CoCs remain unclear since very few have been challenged in court. This certificate does not cover voluntary or intentional disclosure of information by researchers — e.g., in the case of state reporting if a subject divulges child abuse, or reportable communicative diseases, providing these limitations are included in the informed consent. 71 Hence, this certificate may enable researchers to protect data from subpoenas, but allow researchers to divulge information about fraudulent activity if they think that doing so is necessary.
Cross-Cutting Ethical Concerns
Clearly, ethical considerations arise with each of these approaches. These methods differ in the ethical and logistical issues and the specific nature and degree of tradeoffs they present. Yet across individual strategies, researchers and IRBs confront tensions of how to weigh risks and benefits of each approach — how to include in a study means of checking the validity of subjects and their responses without deterring legitimate subjects from participating. Two underlying ethical principles conflict here: maximizing the scientific and social benefits of research vs. respecting the autonomy of subjects (e.g., by decreasing risks of breaches of confidentiality). It is possible that these two goals cannot both be wholly met simultaneously. That is, effective means of reducing “fraudsters” may inevitably deter some potential subjects from enrolling in a study. However, an optimum balance may be possible to achieve. Specifically, vigorous efforts to significantly reduce or eliminate “fraudsters” can ensure the validity of the data, maximizing its scientific and social benefit. The costs may be that some legitimate subjects do not participate, and that researchers thus need to make additional efforts to recruit necessary sample sizes. However, these additional resources appear justified by the result: optimally valid data. Difficult ethical questions emerge, however, as to whether researchers need to disclose to participants all methods the researchers will use to detect and prevent fraud (e.g., collecting IP addresses; searching for subjects online; and enabling cookies on subjects’ computers), and if so, to what degree. On the one hand, such disclosure respects subjects’ rights to be informed of all relevant aspects of the study, and may deter “fraudsters.” However, legitimate participants may then be deterred from participating as well, and such disclosures may alert “fraudsters” to seek strategies to elude these protections — e.g., creating fake Facebook accounts, listing fake names, etc. Creating a fake online presence may seem to require a significant amount of effort for a “fraudster” and thus disincentivize such behavior, but compensation for some studies with multiple stages over a few years can add up to hundreds of dollars. Bockting, Miner, and Hoefer’s study provided each subject a total of $180 if participants successfully completed all tasks, 72 and Rosser et al.’s study provided $80 for completing the pretest, intervention and post-test, and an additional $20–25 for completing each follow-up survey. 73 The overseas currency conversion rate can also attract “fraudsters” abroad more than from the U.S., making foreign “fraudsters” think that these efforts are worthwhile.
Researchers and IRBs have three options here to include in the informed consent documents: 1) all information about these methods, 2) no such information, or 3) general and/or oblique references to such methods. Ethically, disclosing all methods respects subjects’ rights most. Disclosure of collection of IP addresses can also be important since, as in any study, breaches of confidentiality may occur, posing risks to subjects. Yet, for the reasons discussed above, these disclosures may threaten, too, to decrease the scientific and social benefit of the study. Hence, it appears that these competing pros and cons can best be balanced via an intermediary approach: disclosing the fact that certain measures will be taken, without divulging the details involved (i.e., not mentioning the specifics, such as collection of IP addresses). At the same time, since risks in any study should be minimized, security protections, such as use of firewalls and encryption of data, are essential.
While these various methods share certain underlying ethical tensions, other ethical issues differ somewhat between these approaches. Specifically, these methods vary in the amount of personal information they obtain and/or their degree of invasiveness – i.e., how much they may be considered to impinge on subject autonomy and/or raise additional concerns. Reporting “fraudsters” to external authorities (with such action presented in the informed consent) is most invasive, and though it may be intended to serve as a deterrent, it may be seen as punitive. Conducting a face-to-face Skype interview and collecting IP addresses is less invasive, but poses more concerns than storing cookies, which in turn poses more concerns than searching for subjects online.
Given the increased possibility of fraud in Internet research, strategies in the form of detection and prevention of such duplicate and fake responses are increasingly crucial, yet also pose challenges. Considering the limitations of various prevention methods, it is imperative that researchers use multiple methods to compensate for the limitations of any one approach, and also monitor for duplicate entries by hand throughout the study. 74 A critical eye throughout the study will enhance early detection of duplications and fraud as well as ensure the quality of the data.
Researchers conducting online studies face difficult questions and tradeoffs in seeking to prevent duplicate and fraudulent participation while maintaining and encouraging recruitment of valid subjects. It is vital that both researchers and IRBs remain acutely aware of the phenomena of “fraudsters” described here, and of means of detecting and preventing these practices. Investigators have several possible means of detecting and preventing such ineligible responses — including requesting specific personal information in the study or examining outside sources such as Facebook, Google Earth or whitepages.com. For each study, researchers must decide the strategy that will be useful for preventing research fraud, what information about subjects to request, how to convey these methods and information in the consent form, and to what extent these strategies may have undesired consequences in deterring eligible subjects.
When researchers publish articles reporting data from their studies, they should include information on how much and in what ways they compensated participants for online studies, methods used for detecting and preventing fraud, and the success of these efforts — i.e., report rates of “fraudster” activity among participants to enhance the field’s abilities to avoid these problems. This information will increase understanding of the phenomenon of fraudulent participants, provide a better overview of the study, and ensure data quality.
Researchers and IRBs may also need to consider notifying IRBs, the Office for Human Research Protections (OHRP) and/or funders of fraudulent activity, as these involve unjustified use of grant funds (i.e., paying “fraudsters”), and can affect the integrity of the data and thus the scientific and social benefit of the study. Adverse events per se involve harm to subjects, and research integrity problems generally concern misconduct of investigators. However, “fraudsters” threaten the integrity of the research results. The advantage of such reporting is that IRBs and/or federal agencies (e.g., OHRP, the Office of Research Integrity, or NIH) can then readily track the extent and severity of the problem. The NIH should consider developing an organization similar to the IC3, or interface with the IC3 to assist in tracking and controlling fraudulent research behavior. The IC3 issues periodic alerts regarding new internet crimes and preventions, 75 and the NIH or OHRP could have a similar listing of new “fraudster” strategies and possibly the IP addresses of “fraudsters” and/or the common usernames they use. Clear criteria defining fraudulent behavior that would warrant such action would be imperative. Efforts to gauge the full nature and extent of “fraudsters” in these ways can enable researchers, IRBs, and others to then work together as best as possible to detect, prevent, and address this problem in ongoing and future studies.
IRBs need to be flexible concerning detection and prevention of fraudulent behavior. However, IRBs are not designed, either in practice or by statute, to protect researchers, but to protect research subjects. The “fraudster” complicates the definition of human subject in the context of IRB review and human subject research. Researchers cannot always plan in advance how participants will take advantage of an online survey. Kraut et al. suggests that IRBs should have an online/computer expert to assist with Internet research in “both online behavior and technology.” 76 Such an expert could explain to the IRB what is appropriate in the specific study at hand, and can keep the IRB up-to-date on technological advances. As both the Internet and “fraudsters” become more sophisticated and online studies are conducted more frequently, it will indeed be important for the IRB to have online/computer experts to draw on to help facilitate and enhance the conduct of online research, and have IRB members make appropriate decisions to prevent fraud while protecting subjects. Different challenges will emerge over time, and in various kinds of studies aimed at different populations. Researchers and IRBs will need to choose specific strategies for detecting and preventing fraud in individual studies in order to optimally balance protecting both research integrity and subjects.
Future research should test how the structure of online studies and the content of consent forms affect eligible subjects participating in studies, as well as how relevant stakeholders (subjects, researchers, research ethicists and others) view these issues and methods discussed here to prevent “fraudsters,” and the “acceptability and efficacy” of such approaches. 77 Similarly, future studies should build on Bowen et al.’s post-hoc finding that compensation ( vs. no compensation) increases the number of “fraudsters” and the number of entries these “fraudsters” submit. 78 Studies could also examine prospectively how different rates and structures of compensation and informed consent details affect rates of duplications and/or fraud in a study — e.g., how rates of responses and of “fraudsters” vary between longitudinal studies that offer little or no compensation for the completion of initial surveys or offer equal vs. increasing amounts of compensation with completion of subsequent surveys over time. Investigators can examine how participants perceive the methods outlined here (e.g., altering amounts, timing, or types of compensation) and what they feel is an appropriate level of compensation, which could offer important insights. Research could examine, for instance, whether appropriate potential subjects would feel less inclined to participate in studies that used each of the methods mentioned here, and if so, how much so. Future studies could also probe how these decisions might vary based on the population, the research, and the questions posed — e.g., whether a method that proves effective in reducing “fraudsters” by, say, 70% may dissuade 1% or 40% of appropriate subjects. Additional challenges arise since a $20 gift card may be an appropriate amount for U.S. participants, but will be worth a lot more in poorer countries, potentially incentivizing “fraudsters” from abroad. Further investigation on how “fraudsters” identify studies (e.g., through websites such as swagbucks.com) would be valuable as well.
The challenges that researchers and IRBs face in conducting Internet-based research is varied and evolving. As the Internet develops, “fraudsters” too, become more sophisticated. Norms and expectations of web privacy are also changing, highlighting ongoing needs to understanding appropriate and effective means of ensuring privacy, while adequately providing informed consent to a study’s procedures. As the Internet continues to evolve along with online research, so, too, should efforts to detect, prevent, and respond to fraud that may occur. Future research and discussions in this area, and reports on evolving patterns of duplication and fraud, are critical in the growing field of online research.
Acknowledgments
The impetus for this article was an expert meeting about Internet research methods in which all of the authors, with the exception of Jennifer Teitcher, participated. This meeting was held at Columbia University Medical Center, December 14–15, 2012, and was supported by a grant from the National Institute on Child Health and Human Development (9R01HD057595-A1; PI Walter O. Bockting, Ph.D.). The authors would also like to thank Kris Abbate, Patricia Contino, and a National Institute of Mental Health center grant to the HIV Center for Clinical and Behavioral Studies at NY State Psychiatric Institute and Columbia University (P30-MH43520; PI Robert H. Remien, Ph.D.).
Biographies
Jennifer E. F. Teitcher received her B.A. in Criminology from the University of Pennsylvania, Philadelphia, PA.
Walter O. Bockting, Ph.D. is Co-Director of the LGBT Health Initiative in the Division of Gender, Sexuality, and Health. He received his undergraduate and doctoral degree from the Vrije Universiteit, Amsterdam, the Netherlands.
José A. Bauermeister, M.P.H. received his M.P.H. and PhD from the University of Michigan, Ann Arbor, MI, and completed post-doctoral training at Columbia University’s HIV Center for Clinical and Behavioral Studies, New York, NY.
Chris J. Hoefer has a B.S. degree in Family Social Science and Queer Theory from the University of Minnesota, Minneapolis, MN.
Michael H. Miner, Ph.D. received his M.A. in Counseling Psychology from Loyola Marymount University, Los Angeles, CA, and his Ph.D. from St. Louis University, St. Louis, MO.
Robert L. Klitzman, M.D. obtained his B.A. from Princeton University, Princeton, NJ and his M.D. from Yale University, New Haven, CT.
Contributor Information
Jennifer E. F. Teitcher, Research assistant for Dr. Robert Klitzman at Columbia University.
Walter O. Bockting, Professor of Medical Psychology (in Psychiatry and Nursing) at Columbia University Medical Center and a Research Scientist with the New York State Psychiatric Institute.
José A. Bauermeister, John G. Searle Assistant Professor of Health Behavior and Health Education (HBHE), and Director of the Center for Sexuality & Health Disparities (SexLab) at the University of Michigan School of Public Health.
Chris J. Hoefer, Research Project Coordinator at the Program in Human Sexuality within the University of Minnesota Medical School.
Michael H. Miner, Professor and Director of Research at the Program in Human Sexuality, Department of Family Medicine and Community Health at the University of Minnesota Medical School.
Robert L. Klitzman, Professor of Psychiatry and the Director of the Masters of Bioethics Program at Columbia University.
REVIEW article
Theoretical basis and occurrence of internet fraud victimisation: based on two systems in decision-making and reasoning.
- 1 School of Law, Shandong Normal University, Jinan, China
- 2 MBA Education Center, Shandong University of Technology, Zibo, China
- 3 Junde Experimental School, Jinan, China
- 4 School of Law, Jiangsu Normal University, Xuzhou, China
The influencing factors of internet fraud, including demographics, psychology, experience and knowledge of susceptibility, have been widely studied. Research on the psychological mechanism of the victimisation process of internet fraud is relatively scarce but suggests a new research perspective. To summarise and unify the research in this field, this study systematically searched and analysed articles on the psychological decision-making mechanism of online fraud victims. We found that (a) previous researchers consistently believed that the heuristic processing mode was correlated with susceptibility to online fraud and that the systematic processing mode was helpful to detect and identify fraud. From the overall review results, we do not reject this conclusion, but the verification and intrinsic explanation of this relationship need to be further strengthened. (b) Under the heuristic-systematic model (HSM), with the exception of the trait of suspicion, there is no consensus on whether psychological factors (e.g., personality) influence the likelihood of online fraud through the mediating effect of the selection of the two systems. Objective knowledge and experience in specific fields have been found to be able to achieve this path. Information on the influential variables of equipment and habits is emerging, but how they affect network victimisation through the heuristic processing system needs to be further clarified. (c) The measurement of variables is conducted through simulation experiments. There may be a gap between the likelihood of internet fraud victimisation in the simulation experiment and in the real world. (d) The defence strategies under the HSM are intentional explorations, such as content-based cue recognition technology and simulated scene training.
Introduction
Internet fraud is defined as the act of obtaining money through deception using network communication technology or the act of providing fraudulent invitations to potential victims or conducting fraudulent transactions using the internet ( Tade and Aliyu, 2011 ; Whitty, 2015 , 2019 ; Gao, 2021 ). Internet fraud is also called phishing and is typically performed by sending victims an email that is ostensibly from a legitimate organisation or individual ( Frauenstein and Flowerday, 2020 ). With the communication technologies currently available, especially mobile devices, internet fraud occurs not only through email but also through text messages, social networking sites (SNSs), and telephones ( Vishwanath, 2015 ; Aleroud and Zhou, 2017 ; Frauenstein and Flowerday, 2020 ).
Internet fraud, including phishing, is the fifth most common cause of security incidents and has the highest success rate of any threat vector ( Verizon, 2019 ). Facebook and Google were defrauded of more than $100,000 through a phishing scheme that impersonated a large Asian-based manufacturer in 2017 ( United States Department of Justice, 2017 ). A meta-analysis showed that internet fraud in the United States in 2018 caused approximately 2.7 billion dollars in economic losses. Internet fraud is also the fastest growing crime in the United Kingdom, with approximately 3.25 million people becoming victims each year ( Norris et al., 2019 ). At the beginning of the COVID-19 pandemic in 2020, online fraudsters began to take advantage of people’s panic and uncertainty to conduct phishing attacks ( Muncaster, 2020 ). Internet fraud has become an important social governance problem ( Burnes et al., 2017 ) and has attracted increasing attention from scholars ( Vishwanath et al., 2011 ; Modic and Lea, 2013 ; Harrison et al., 2016a ; Modic et al., 2018 ).
Routine activity theory notes that victimisation is caused by motivated criminals, appropriate targets and a lack of effective guardianship ( Cohen and Felson, 2010 ). Motivated criminals use cunning as a means of defrauding victims. Cialdini (2018) has summarised six key principles of persuasion often used by fraudsters: reciprocity, social proof or conformity, commitment or consistency, authority, liking, and scarcity. For example, when confronted with scarcity information, the receiver responds to the information to avoid the loss of opportunities ( Bullée et al., 2015 ). In terms of effective guardianship, all countries attach great importance to combating and preventing internet fraud. Common means include strict legal action against criminals, educating and reminding potential victims, and interception by technical methods ( Chen and Yang, 2022 ). Questioning why many people every day suffer from internet fraud attacks requires a shift of vision to the appropriate target or victim. There are three main directions for research on potential victims of internet fraud.
The first research direction is demographics, which refers to the relationship between the age, income, education, gender, and race of victims of internet fraud ( Cohen et al., 1981 ; Holtfreter et al., 2006 ; Salthouse, 2012 ; Burnes et al., 2017 ; Gavett et al., 2017 ). Carcach et al. (2001) found that men are more likely than women to be victims of personal crimes such as internet fraud. Age has been the focus of many scholars’ attention and research, and the growing ageing phenomenon and the spread of anecdotal evidence, such as news reports, have formed the concept that older adults are more vulnerable to fraud. Many scholars have analysed different factors of internet fraud victims and found that compared with other types of crimes, older adults are more likely to become victims of consumer fraud ( Carcach et al., 2001 ). Burnes et al. (2019) agreed that “the elderly are more easily cheated, which is related to their slow cognitive processing and high experiences of loneliness.” In addition, James et al. (2014) found that vulnerability to fraud is related to victims’ income and education level. Some studies by the Federal Trade Commission (FTC) show that Aboriginal Americans, African Americans, and Hispanic Americans are more likely than non-Hispanic white Americans to be victims of fraud ( Anderson, 2004 ; Anderson, 2013 ).
Second, regarding the direction of psychological characteristics, researchers have mainly studied the influencing factors of susceptibility to online fraud. These include risk perception ( Moody et al., 2017 ), trust ( Wright and Marett, 2010 ), suspicion ( Harrison et al., 2016a ), personality ( Ashton and Lee, 2009 ), and self-control ( Modic and Lea, 2012 ). Holtfreter et al. (2008) proposed that groups with low self-control are more likely to be cheated. This is mainly because people with low self-control attempt to meet their needs immediately. They may follow the instructions of a fraudster to obtain a promise. In research on the relationship between personality and vulnerability to online fraud, researchers found that not all personality traits predict vulnerability to fraud. Alseadoon et al. (2012) simulated fraud against 200 college students and found that openness and extraversion could improve the possibility of replying to emails, although no other personality traits were found to have a predictive effect. In the study of personality differences and susceptibility to online fraud, scholars have also examined the relationship between victims’ online experience, security knowledge and susceptibility to online fraud ( Larcom and Elbirt, 2006 ; Wright and Marett, 2010 ).
Third, with regard to the direction of the psychological mechanism, according to interpersonal deception theory, fraud is essentially antagonistic to social interaction, which requires cognitive resources ( Buller and Burgoon, 1996 ). Deception works because the deceiver takes advantage of the target’s weakness in information processing and takes measures to thwart the target’s cognitive efforts in interaction ( Johnson et al., 2001 ). In other words, the target is victimised because of a weakness in information processing, failure in the cognitive detection of fraudulent information, or both. Previous studies have confirmed that users’ cognitive processing is a key cause of individual online fraud victimisation ( Vishwanath et al., 2011 ). Related theories are the heuristic-systematic model (HSM), the elaboration likelihood model (ELM), and the theory of deception:
The HSM is a model of information processing that includes two information processing modes: the heuristic system based on intuition and the analytic system based on rationality ( Chaiken, 1980 ; Sloman, 1996 ; Evans, 2003 ). The Heuristic system relies more on intuition; parallel processing is fast and does not occupy or occupies little psychological resources. The Analytic System relies more on rationality, serial processing is slow, and occupies more psychological resources. The study also found that heuristic processing leads to lower risk assessment ( Tversky and Kahneman, 1974 ; Trumbo, 2002 ), which makes it difficult for people to identify the traps in the fraudulent information and ultimately leads people to suffer fraud. Phishing attacks usually increase their success rate by misleading the target victim to make a quick but incorrect evaluation of information effectiveness ( Luo et al., 2013 ).
The ELM is also a dual process model; it distinguishes between two ways in which individuals process information. The central processing route involves careful consideration of presented information using comparisons and prior experience, but the peripheral processing route does not consider all elements of the message ( Petty and Cacioppo, 1986 ). Although the HSM is theoretically similar to the ELM, the HSM emphasizes that two distinct modes of thinking about information can occur, and the ELM suggests that information processing occurs on a continuum instead ( Frauenstein and Flowerday, 2020 ). According to Petty and Cacioppo, information processing activities include two subprocesses: attention and elaboration. Attention is the first stage in information processing and indicates the amount of mental focus given to specific elements of an event or object ( Eveland et al., 2003 ; Vishwanath et al., 2011 ). Elaboration is the process through which individuals make conscious connections between the cues they observe and their prior knowledge ( Perse, 1990 ; Vishwanath et al., 2011 ). Jakobsson (2007) found that the target was victimised, probably because certain cues in a phishing e-mail address (e.g., e-mail address) were not noticed. Users who can identify fraud are able to pay attention to irrational clues (ELM’s attention process) and use previous experience and knowledge for evaluation (ELM’s elaboration process).
The theory of deception is also known as the detecting deception model. It refers to individuals identifying fraud by noticing and interpreting inconsistencies between anomalies and their past experience; thus, clue processing is further elaborated ( Johnson et al., 1992 , 2001 ). According to the detection deception model, the process of identifying fraud can be divided into four stages: a. Activation, detecting anomalies of fraud information. b. Hypothesis generation, interpreting abnormal clues and generating suspicion. c. Hypothesis evaluation, comparing the hypotheses developed in the previous stage with certain criteria. d. Global assessment, combination and overall evaluation of known clues. These four stages of cognitive effort are similar to the process of elaboration ( Eveland et al., 2003 ; Vishwanath et al., 2011 ). In 2004, Grazioli tested the authenticity of the trading site on Eighty MBA students and found that competence in evaluating the hypothesis of deception (stage c) was a strong differentiator between successful and unsuccessful detection. Although a large number of previous studies have been conducted on the relationship between demographics, psychological traits and online fraud, there is no consensus on the research conclusions. For example, regarding demographic factors, Button et al. (2009) proposed that no demographic characteristic is necessarily more or less susceptible to internet fraud. Shang et al. (2022) found that elderly people were not a susceptible population, and the influencing factors measured in the past were untenable based on a systematic review of the literature. Regarding psychological factors, there are mutually exclusive research results in relation to trust and other factors ( McKnight et al., 2004 ; Judges et al., 2017 ). Research on online fraud should examine the decision process of victims in the face of fraudulent information ( Norris et al., 2019 ).
A summary of the decision process of network fraud victims shows that although the ELM distinguishes the central processing route (system 1) and the peripheral processing route (system 2) in theory, there is no measurement or classification of these two systems in practice. Researchers mainly focus on the relationship between two subsystems of ELM (attention and elaboration) and online fraud ( Vishwanath et al., 2011 ; Harrison et al., 2016b ). Attention and elaboration are often regarded as indicators of the systematic processing of HSM ( Frauenstein and Flowerday, 2020 ; Gao, 2021 ). Therefore, our research vision should be on ELM. This study searched and analysed the literature on the decision process of online fraud victims using the heuristic systematic model to obtain and discuss previous research conclusions on the victimisation process and promote further exploration in this field.
Materials and method
Systematic review.
This manuscript is a systematic review, and the scope of the review is the literature on the information processing model of internet fraud victims, particularly the heuristic-systematic model. A systematic review is different from a meta-analysis; while the current research literature on fraud victimisation mentions the heuristic or systematic processing mode (in addition to the analytic processing mode), there is little research on the correlation between the two processing modes and fraud susceptibility. Specifically, the previous literature focuses either on the relationship between the information processing mode and trust, doubt and susceptibility or on the relationship between the subprocesses of the cognitive processing mode (attention and elaboration) and the above dependent variables. In other words, published studies on the processing modes of network victimisation differ in terms of the independent variables, dependent variables, intervention methods and research design, which makes it difficult to meet the prerequisite conditions for meta-analysis ( Cheung and Vijayakumar, 2016 ).
Search strategy
This study was conducted using guidelines and checklists outlined by the Preferred Reporting Project for Systematic Review and Meta-Analysis (PRISMA) group ( Moher et al., 2009 ). This search was based on relevant full-text articles selected from multiple database searches of all published documents from the establishment of each database to May 2022 (search process updated on 16 October 2022). The following English databases were used: Web of Science Core Collection, Elsevier, SciELO Citation Index2, ProQuest, and PsycArticles. The English search strategy was as follows: (phishing email OR phishing OR phished OR online OR internet OR cyber OR network OR telemarketing) AND (fraud OR cheat OR swindle OR scam OR deception OR susceptibility to scam OR susceptibility to deception OR susceptibility to persuasion OR susceptibility to fraud OR phishing vulnerability OR phishing susceptibility OR fraud victims OR phishing victims) AND (cognition OR cognitive processing OR information processing OR heuristic model OR systematic model OR HSM OR system processing OR elaboration likelihood model OR ELM OR elaboration OR processing clues OR attention OR suspicion). To more clearly express our search strategies, we have set up Table 1 .
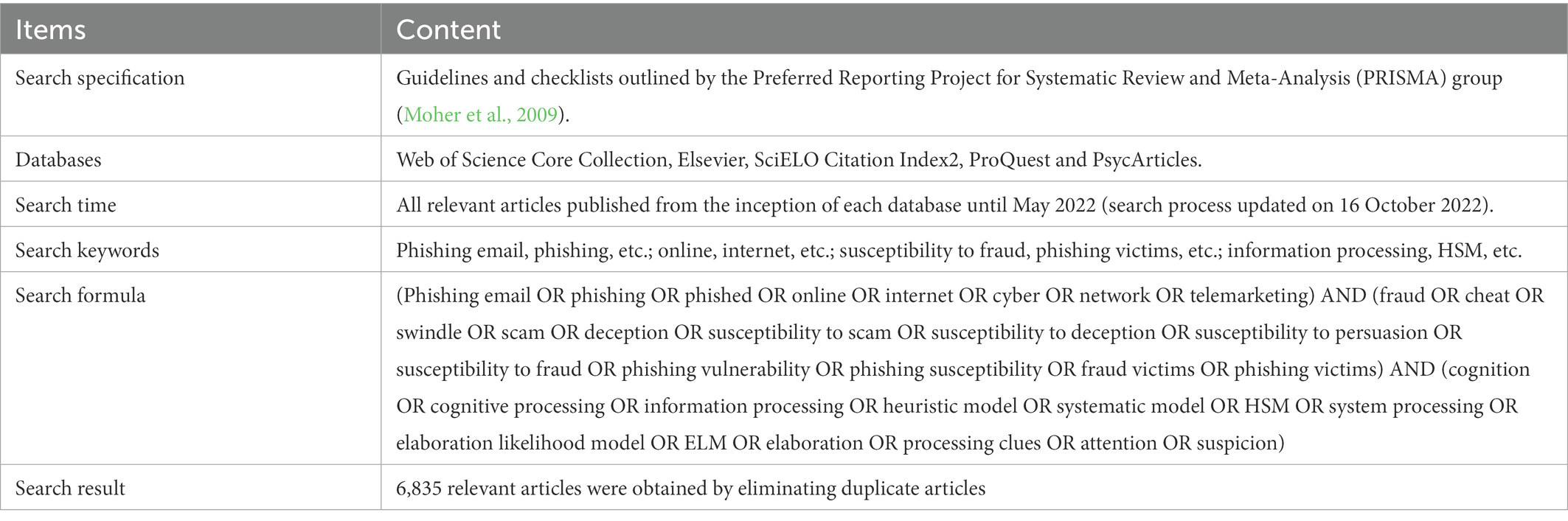
Table 1 . Search Strategy.
Inclusion criteria and exclusion criteria
The topic of this paper is the information processing mode of internet fraud victims. In terms of article types, experimental or measurement studies were preferred, and explanatory phenomenological analysis and anecdotal comments on cases and scams were excluded. For systematic review studies, only the full text of literature that discussed the information processing methods of internet fraud was chosen.
In addition to the investigation of research topics, the following types of studies were excluded: (1) not in a peer-reviewed journal; (2) written in any language other than English; (3) full text could not be accessed through the university library or obtained directly from the corresponding author; (4) published in abstract form (failure to provide enough information to analyse the impact of information processing modes on victims); and (5) used qualitative research methods.
Article screening
Under the guidance of the search strategy, 6,835 relevant articles were obtained by eliminating duplicate articles. The objects of this study were victims of online fraud. Article titles and abstracts were searched, and 6,612 articles were found that did not focus on the subject of online fraud victims. For the remaining 223 articles, the full text and references of these articles were checked. We found that 9 articles were not included in these 233 articles but may be possibly related to network fraud victims. We included these 9 articles and 233 articles previously screened into the analysis. Then, a database of 232 articles was built. According to the inclusion and exclusion criteria, especially the key words of cognitive information processing of network fraud victims, 17 articles were finally included in the analysis. (1) Although most of the literature mentioned the cognitive information processing process of network fraud victims (e.g., the HSM), discussion of the information processing mode was minimal and not the key object of the study in 177 articles, which were excluded. (2) Eleven articles were not published in peer-reviewed journals. (3) Eight articles were published in abstract form. (4) Fourteen systematic review papers did not focus on cognitive processing. (5) Five research papers used qualitative methods. See Figure 1 for the selection process.
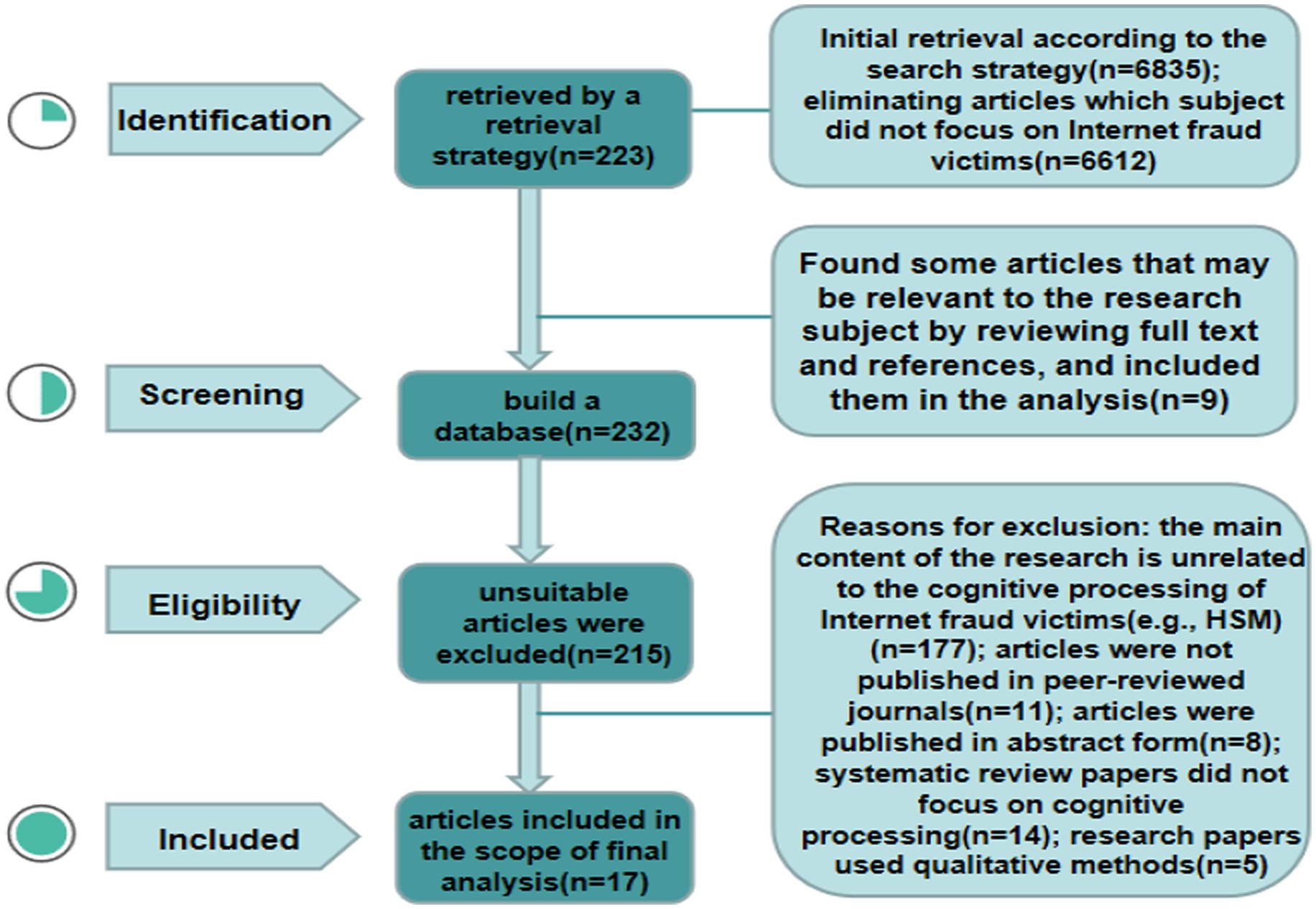
Figure 1 . Identification flowchart of the search for literature on cognitive information processing of online fraud victims (model drawings comes from the author’s construction).
Quality assurance
The entire process of searching and screening was completed by two graduate students independently. To ensure the objectivity and accuracy of the screening, the research team first fully discussed the inclusion and exclusion criteria and unified opinions on the preset divergence. After the screening was finished, the screening tools were used to compare the results and conduct a collective study on the literature with different opinions. The selection and reporting of risks was controlled, to a certain extent, through the above process. Finally, the publishing risk of the final included articles was evaluated. Among the 17 articles included in the analysis, 94% of the published journals ranked in the top 50% of Journal Citation Reports.
The Supplementary Table shows the main characteristics of research on the psychological mechanism of online fraud victims using the HSM framework (N = 17). The following information was selected: source, country, method, sample size, sample description, and main findings. Researchers conducted the studies in the United States ( N = 14; Grazioli and Wang, 2001 ; Johnson et al., 2001 ; Wright and Marett, 2010 ; Vishwanath et al., 2011 , 2016 ; Wang et al., 2012 ; Luo et al., 2013 ; Petty and Briñol, 2014 ; Canfield et al., 2016 ; Vishwanath, 2016 ; Harrison et al., 2016a , b ; Huang et al., 2022 ; Valecha et al., 2022 ), South Africa (N = 1; Frauenstein and Flowerday, 2020 ), China ( N = 1; Chen and Yang, 2022 ), and the UK ( N = 1; Jones et al., 2015 ).
Based on the similarity between the HSM and the ELM as well as the inclusion of the theory of deception, a systematic review of the cognitive processing mechanism of online fraud victims was conducted. We report the relationship between the HSM and susceptibility to online fraud. On this basis, the influencing factors of the decision mode selection of victims are discussed, and the network fraud defence countermeasures proposed by researchers under the HSM are highlighted.
The selection of the heuristic-analytic processing mode and victims of internet fraud
Heuristic processing uses simple factors or messages (i.e., heuristic cues) to conduct rapid effectiveness evaluation, while systematic processing conducts a highly elaborative validity evaluation of the received information by carefully studying the content of the information and comparing the information with previous experience. This tendency to process information in different ways may influence users’ attitudes, judgements and behaviours towards specific information ( Petty and Cacioppo, 1986 ). Studies have shown that individuals prefer heuristic processing rather than effort for information evaluation based on consideration of the cognitive resource economy ( Sundar et al., 2007 ; Sundar, 2008 ). However, studies also show that heuristic processing leads to lower risk assessment, which makes it difficult for individuals to identify traps in fraudulent information and thus exposes people to fraud ( Wang et al., 2012 ; Jones et al., 2015 ; Vishwanath et al., 2016 ).
The HSM argues that when people make a validity evaluation, their confidence in their evaluation must meet or exceed the adequacy threshold (the extent people wish to reach when making decisions) to feel comfortable with their own judgements ( Eagly and Chaiken, 1993 ). When heuristic processing alone cannot guide message receivers to reach the sufficiency threshold, receivers are likely to invoke systematic processing ( Luo et al., 2013 ). Vishwanath et al. (2016) found that systematic processing significantly reduces the chances of fraud victimisation; in contrast, heuristic processing significantly increases the chances of fraud victimisation, doubling the likelihood that people will be victims of email and Facebook phishing attacks. In addition, according to the weakening principle of the HSM, a high level of systematic processing can weaken the impact of heuristic processing and may even produce conclusions that limit or overturn heuristic processing ( Watts and Zhang, 2008 ). When individuals activate system processing to detect and process fraud information, it is easier for them to identify online fraud ( Grazioli and Wang, 2001 ; Grazioli, 2004 ).
The studies included in our analysis consistently indicate that victimisation through online fraud is related to the heuristic decision-making model. Phishing attackers know the weaknesses of human information processing and aim to improve the success rate of fraud by arousing victims’ heuristic thinking and reducing systematic thinking ( Johnson et al., 2001 ; Luo et al., 2013 ; Canfield et al., 2016 ; Vishwanath et al., 2016 ; Chen and Yang, 2022 ). In terms of specific demonstrations, only 3 studies provided data analysis (other documents studied either the relationship between subsystems of the HSM and victimisation or the influencing factors of the HSM), which mainly demonstrated the information processing models (the heuristic processing mode vs. the systematic processing mode) and whether subjects were susceptible to online fraud ( Table 2 ).
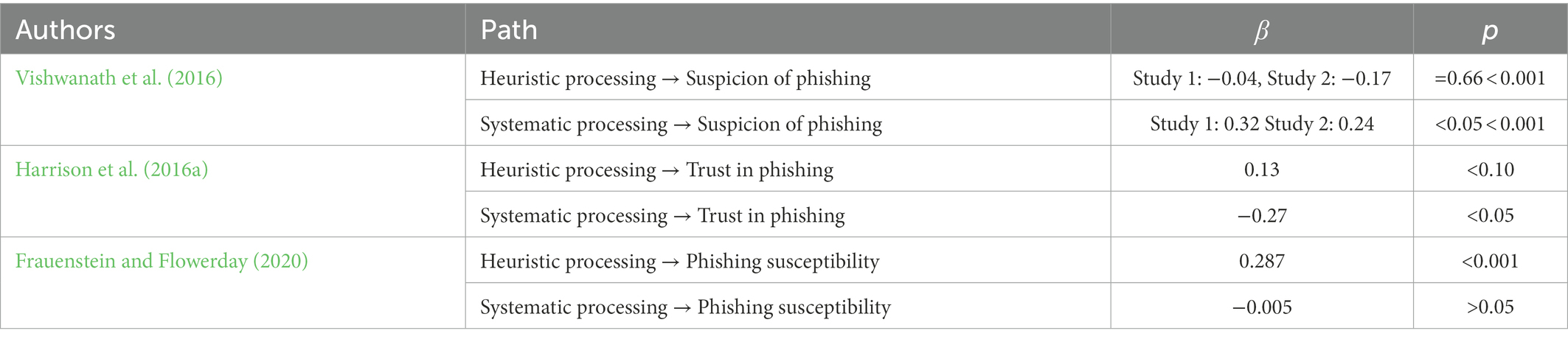
Table 2 . Path analysis of heuristic-systematic processing and data results.
Social psychology research on phishing suggests that an ineffective cognitive process is a major cause of personal victimisation ( Workman, 2008 ; Vishwanath et al., 2011 , 2016 ). How does fraud information make the HSM produce invalid cognition and thus affect people’s vulnerability to fraud? Scholars believe that information processing activities are divided into two discrete subprocesses, attention and elaboration (regarded as indicators of systematic processing, Frauenstein and Flowerday, 2020 ; Gao, 2021 ). Different degrees of attention to and elaboration of information ultimately lead to different susceptibilities to fraud victimisation.
Attention is the first stage of information processing. This initial attention may cause specific individuals to feel compelled to search for further clues in the email, relate these clues to existing knowledge, determine whether the email is relevant and ultimately conclude that the email is a hoax ( Jakobsson, 2007 ). The research shows that there is a significant correlation between the degree of attention and elaboration. Individuals who pay more attention to information elements have a higher degree of elaboration ( Harrison et al., 2016b ). For example, suspicious concerns about typographical errors, grammatical errors, and website addresses in phishing emails may lead to more detailed message elaboration, resulting in systematic processing and reducing the likelihood of being victimised by phishing ( Toma and Hancock, 2012 ). Of course, attention to clues focuses more on quality than quantity. Grazioli (2004) found that successful detection does not heed deception cues more than unsuccessful detection, which is different from conventional perception.
In the second stage of the information processing-elaboration process, elaborate information processing occurs when individuals relate these information elements to prior knowledge and experience by adopting a central (systematic) processing path. In contrast, when the peripheral (heuristic) processing path is adopted, no attention is given to the information elements or no elaboration processing is conducted for the noticed information elements ( Perse, 1990 ; Eveland et al., 2003 ; Gao, 2021 ). People who elaborate on clues are more likely to understand, learn, retain, and subsequently recall information than those who only focus on clues ( Cialdini, 2001 ; Eveland et al., 2003 ). Vishwanath et al. (2011) and Harrison et al. (2016b) found that elaboration is a predictor of individual phishing, which is related to a lower likelihood of being victimised by phishing. The elaboration and processing of information content (i.e., using systematic processing) reduce the likelihood of being cheated.
Factors related to the selection of the heuristic-analytic processing mode
As mentioned in the introduction, research on the influencing factors of online fraud includes demographics, psychological traits and other variables. The discussion in this section explains what factors may influence an individual’s information processing mode and lead to network fraud under the framework of the HSM. Our inductive findings show that psychological factors, knowledge and experience, equipment and habits may influence the cognitive processing mode for internet fraud (the initiation of the heuristic system mode or analytic system mode; Figure 2 ).
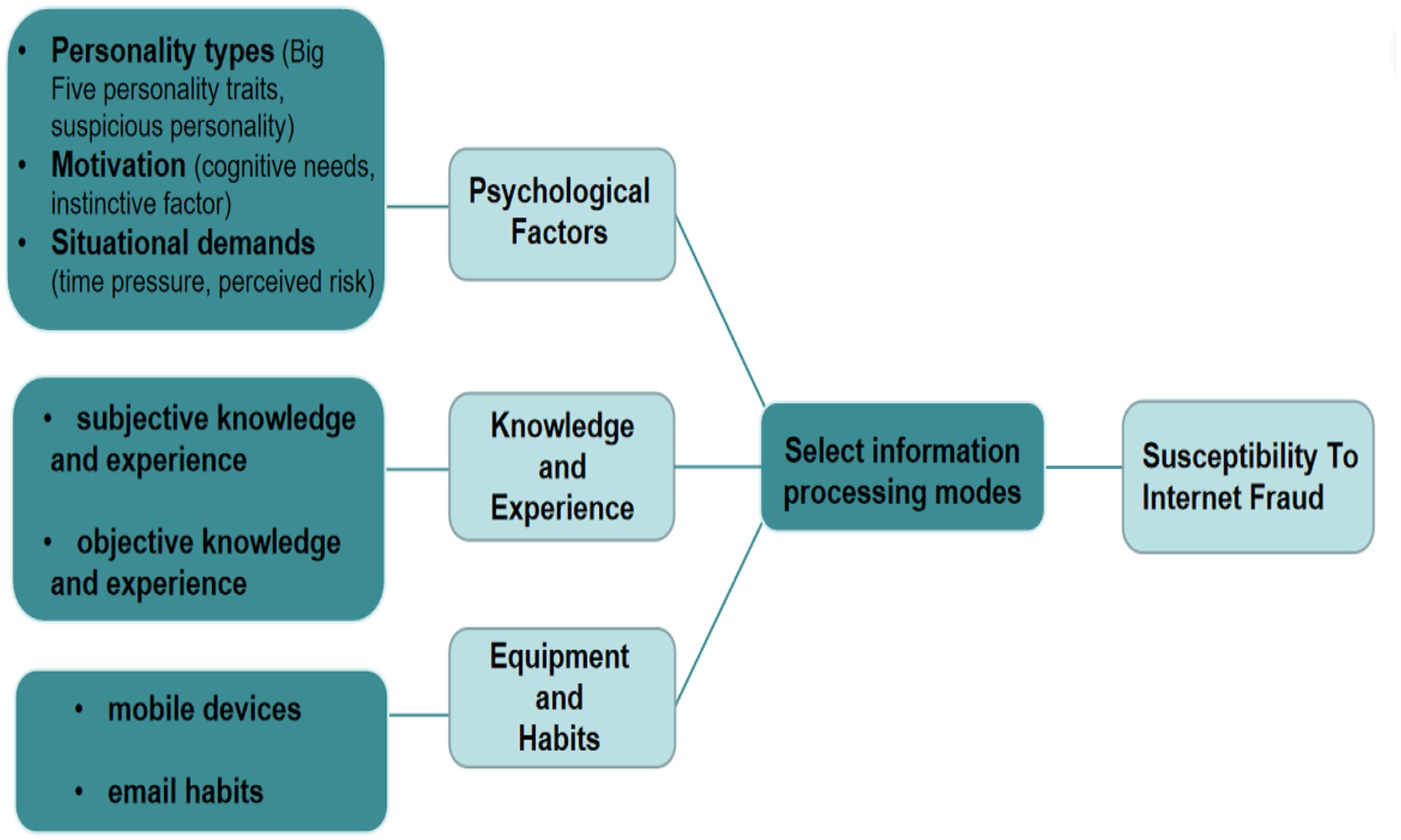
Figure 2 . Influencing factors of susceptibility to internet fraud under a framework of the HSM (model drawing came from the author’s construction, and adapted from Wright and Marett, 2010 ; Norris et al., 2019 ).
Psychological factors
Personality type.
Research on personality types mainly focuses on the Big Five personality traits and suspicious personality. Studies have been conducted on the relationship between the Big Five personality traits and the likelihood of online fraud victimisation ( Norris et al., 2019 ). For example, Alseadoon et al. (2012) found that individuals with a high degree of agreeableness, openness and extraversion are highly susceptible to information on the internet, but this study did not reach a consistent conclusion. Cho et al. (2016) found that agreeableness and neuroticism had significant predictive effects on the likelihood of being cheated on the internet. Within the framework of the HSM, only the study by Frauenstein and Flowerday (2020) was found. These authors showed that heuristic processing increased the susceptibility to phishing and examined for the first time the effect of the relationship between the Big Five personality model and the heuristic-systematic model of information processing. They found that extraversion was not statistically correlated with either heuristic or systematic processing; agreeableness, neuroticism and openness all had effects with both heuristic and systematic processing; and conscientiousness was statistically correlated with heuristic processing but had no effect with systematic processing. It should be noted that some personality variables (such as agreeableness and neuroticism) are in the same direction as the effects of heuristic processing and systemic processing, which confirms, to some extent, that heuristic processing and systemic processing may be enabled simultaneously when processing information.
There is a tendency among individuals to be suspicious of the intentions of others, which is a type of persistent personality trait and is defined as generalised communicative suspicion (GCS; Levine and McCornack, 1991 ). Research on the relationship among GCS, the HSM and the susceptibility to network fraud has gone through two stages: in stage 1, GCS and the HSM were regarded as independent dependent variables; in stage 2, the linkage between GCS and the HSM was established. Stage 2 is mainly discussed here. According to the viewpoint of the HSM and internet fraud victimisation, the main reason network users fail to identify fraud and ultimately are victimised is that they start the heuristic system when processing information ( Grazioli and Wang, 2001 ; Wang et al., 2012 ; Luo et al., 2013 ; Huang et al., 2022 ). Harrison et al. (2016a) introduced information insufficiency as a mediator between GCS and the HSM and found that high GCS increases uncertainty and leads to a desire for more information before making a judgement. The desire for more information leads to systematic processing of available information and more accurate detection of phishing deception.
According to the HSM, if people lack motivation, they tend to limit their investments of time and cognitive resources ( Luo et al., 2013 ). Individuals with the motivation to process information pay attention to key information of arguments and then conduct elaborative processing. In contrast, individuals who lack motivation may focus on cues peripheral to the main argument and may be persuaded by noncontent cues ( Petty et al., 1981 ; Petty and Cacioppo, 1986 ; Stamm and Dube, 1994 ). The motivation of receivers to pay attention to information determines the degree of information elaboration. The more motivated network users are to consider a scam, the more likely they are to carefully evaluate the details of the information, which may lead to the discovery of leaked clues about the scam and thus to the avoidance of victimisation ( Langenderfer and Shimp, 2001 ; Wang et al., 2012 ). Langenderfer and Shimp (2001) also found a potentially negative correlation between motivation and vulnerability to fraud victimisation and suggested that a low level of motivation may be one of the reasons for a lack of review. However, some scholars believe that when individuals are in a state of strong motivation, they do not fully elaborate on the advantages and disadvantages of decision-making, neglect possible problems, and reduce the quality of their decision-making and related information processing ( Schwarz et al., 1980 ; Frey, 1986 ; Fischer et al., 2008 ). Experiments by Ariely et al. (2009) confirmed that decision-making deteriorates when the amount involved is large enough to exceed people’s normal experience.
These inconsistent findings require a search for cognitive and instinctive factors in motivation. According to the HSM, the motivation to commit cognitive resources is premised on personal expectations about behaviour (cognitive needs; Chaiken, 1987 ). Perceived information insufficiency significantly predicts system processing, and the greater cognitive needs are, the greater the need to use processing resources ( Vishwanath, 2015 ). People with higher cognitive needs are less affected by heuristic processing, so they are less likely to be cheated ( Luo et al., 2013 ). However, in some cases, even if motivation is high, people may still be subject to fraud. This may be related to instinct, which often produces thoughtless decisions; that is, people affected by instinct usually do not consider the consequences of their own actions ( Loewenstein, 1996 ). When individuals are too eager to obtain a reward promised by fraudulent information or to avoid the danger contained in fraudulent information, they ignore obvious clues to fraud in the attention process ( Langenderfer and Shimp, 2001 ). Therefore, the influence of motivation on the vulnerability to fraud may be moderated by instinctual factors. When instinct has a great influence, individuals with strong motivation are more likely to miss clues in the information and focus more on rewards or avoiding losses, whereas when instinct has little influence, individuals may choose to carefully evaluate the details of the information rather than the reward itself ( Whitty, 2013 ; Jones et al., 2015 ).
Situational demands (time pressure, perceived risk, etc.)
Shah et al. (2004) found that in phishing emails, people focus disproportionately on urgent cues and tend to ignore other elements, such as the source, grammar, and spelling ( Jakobsson, 2007 ). Attention to urgent cues may induce a sense of urgency and pressure, and individuals under time pressure tend to rely more on one of these cues or use fewer product attributes to make choices, eliminating the systematic processing that requires time and cognitive resources ( Wright, 1974 ; Rothstein, 1986 ). Information produced by phishers that contains urgent cues reduces the cognitive processing of information and inhibits the systematic processing of other cues that may indicate illegitimate information sources. Phishers hope that these urgent cues will emphasise emotional responses and guide users away from more rational decision-making processes ( Workman, 2008 ; Vishwanath et al., 2011 ; Harrison et al., 2016b ). Luo et al. (2013) proposed that imposing more time pressure on phishing messages may reduce the impact of argument quality and increase the effect of source credibility and the herd effect, thus priming heuristic processing and influencing susceptibility to fraud victimisation. However, some studies have shown that email characteristics (i.e., the need for timely decision-making) do not influence how web users process phishing emails ( Harrison et al., 2016b ).
Risk-related beliefs have been found to be the most commonly used cognition when individuals examine risk-related actions ( Griffin et al., 2002 ). When people perceive a threat, they adjust their behaviours based on the risk and possible damage caused by the threat ( Grothmann and Reusswig, 2006 ). Individuals anticipate that their behaviours will have serious consequences, which increases their uncertainty, and systematic processing occurs ( Workman, 2008 ). Vishwanath et al. (2016) found that cyber-risk beliefs are negatively related to heuristic processing and positively related to systematic processing. Individuals with strong cyber-risk beliefs are more able to identify online fraud. This is different from the findings of Das et al. (2003) , who suggested that the existence of threat elements in information may have a special impact on information processing; as a result, information processing resources are distributed unevenly, and the acceptability of persuasive information increases. Studies have shown that the perceived risk caused by fear does not influence the elaboration process, and some scholars have also verified that higher perceived risk did not decrease the likelihood that a person would be deceived by a phishing email through experiments. This is because when it comes to online fraud, some people with higher perceived risk may fear the consequences of a wrong judgment, and they may be less motivated to detect deception cues because of possible interpersonal and economic repercussions ( Wright and Marett, 2010 ; Harrison et al., 2016b ).
Knowledge and experience
The stage of information elaboration processing can be predicted by knowledge and experience variables. People who do not have the experience or knowledge necessary to understand an argument usually rely on peripheral clues in the information, which triggers heuristic processing and may lead to incorrect decisions ( Petty and Cacioppo, 1986 ; Chen and Chaiken, 1999 ). Wright and Marett (2010) found that in the context of phishing, security knowledge and network experience can help users more easily find and identify fraudulent clues in phishing emails, increase the possibility of attention to and elaboration of the information, and thus reduce the possibility of victimisation from phishing. Harrison et al. (2016b) also found that elaboration is not influenced by message factors but is predicted by knowledge in specific fields.
With the growth of acquired knowledge and cognitive skills, people are able to critically analyse relevant information, which makes adults less reliant on heuristic processing than children ( Ross, 1981 ; Petty and Cacioppo, 1986 ). Knowledgeable subjects are able to participate in and successfully complete deception detection even under time pressure ( Grazioli, 2004 ). Knowledge of email scams increases attention to phishing scam indicators and directly reduces the likelihood of responses ( Wang et al., 2012 ). A higher level of prior professional knowledge among information receivers increases their ability to understand and process relevant issues, which increases the likelihood of elaboration and reduces reliance on peripheral cues ( Ratneshwar and Chaiken, 1991 ).
Harrison et al. (2016b) distinguished between subjective and objective knowledge and found that only objective phishing knowledge was associated with more attention to emails. More knowledge also means that less attention resources are used to trigger professional knowledge. However, it has also been argued that since stored knowledge is often biased towards the original viewpoint, such prior knowledge may provide a biased view of information provided externally ( Crocker et al., 1984 ). False knowledge (subjective knowledge) may also cause a false sense of confidence and lead to decreased attention and elaboration of the specific nuances in phishing emails that may reveal deception ( Harrison et al., 2016b ).
Equipment and habits
Recent research has shown that the use of mobile devices such as smartphones can make people more likely to fall into online fraud traps by enhancing heuristic processing. If users prefer to process emails on their mobile phones rather than computers, they will be more responsive to the heuristic clues contained in phishing emails ( Kim and Sundar, 2015 ; Vishwanath, 2016 ). Compared with computers, smartphones have smaller screens and are mostly touch based, so content must be displayed in a limited space ( Sundar, 2008 ). The design and layout of smartphones emphasise rich graphical clues rather than text content. Rich presentation exhausts the limited cognitive capacity and resources needed to process persuasive content, thus enhancing heuristic processing ( Kim and Sundar, 2015 ; Vishwanath, 2015 ). Moreover, a multitasking processing mode reduces the available cognitive resources for system processing ( Chaiken, 1987 ; Ratneshwar and Chaiken, 1991 ). Experimental results show that a large screen size and video mode of smartphones promotes heuristic processing, while a small screen size and text mode promotes systematic processing ( Kim and Sundar, 2015 ). However, some studies suggest that email habits and cognitive heuristics jointly and independently affect the possibility of being cheated on the internet. Mobile devices such as smartphones affect vulnerability to fraud by strengthening habits rather than affecting cognitive processing ( Sundar, 2008 ; Vishwanath, 2016 ).
A habit is an automatic response or behaviour pattern that follows a fixed cognitive pattern; it is triggered by environmental stimuli and executed without positive consideration ( Bargh and Gollwitzer, 1994 ; LaRose and Eastin, 2004 ). Studies have reported that responses to phishing emails can be constricted by habitual response patterns (e.g., responding immediately upon waking up in the morning); that is, individuals respond automatically to relevant emails rather than actively paying attention to them ( Vishwanath et al., 2011 ). Based on the definition of habit, habitual email behaviour that is formulated unconsciously is separate from conscious behaviour that involves some degree of thinking ( Aarts et al., 1998 ). In other words, the habit of replying to online fraud information involves a lack of attention and elaboration of the HSM. Within the framework of the HSM, there are three main ways for email habits to influence online fraud victims: habitual patterns of media usage (an extreme value of involvement, which is positively related to the level of elaboration) combined with a high-level email load (which is negatively related to the level of elaboration) have a strong and significant impact on the likelihood of individuals being phished ( Vishwanath et al., 2011 ); email habits are negatively related to suspicion, heuristic processing is also negatively related to suspicion, and systematic processing is positively related to suspicion ( Vishwanath et al., 2011 ); and email habits are parallel to the heuristic-systematic model ( Vishwanath et al., 2016 ).
Measures of the heuristic-analytic processing mode, influencing factors and likelihood of internet fraud victimisation
Measures of the likelihood of internet fraud victimisation under the HSM framework are conducted by the experimental method. During these experiments, experimenters provide victims with fraud materials (the materials may be real fraud materials or may be designed by the researchers according to the research purpose), such as shopping websites ( Grazioli, 2004 ), phishing emails ( Vishwanath et al., 2011 ; Luo et al., 2013 ; Harrison et al., 2016b ), and financial statements ( Grazioli and Wang, 2001 ). The subject’s judgement of the validity of these materials, or whether the subject responds, is used as an assessment of the likelihood of being cheated. For example, Wang et al. (2012) investigated 321 members of a public university community in the northeastern United States with a real phishing email as a stimulus. The researchers claimed that they were an email team, notified users of a website upgrade, asked users to verify their email account information, and required users to provide their user name, password and other information. Users were told that if they did not provide the requested information within 7 days, they would permanently lose their email accounts. The title of the email read “UPGRADE YOUR EMAIL ACCOUNT NOW.” In the study by Wang et al., subjects had the possibility of being cheated if they responded to emails and provided information but not otherwise.
Measures of heuristic processing and systematic processing are mainly carried out through self-reports after experiments. Different researchers have designed different contents and quantities of items; some studies used 3 items ( Vishwanath et al., 2011 ), some studies used 4 items ( Griffin et al., 2002 ), and some studies used 6 items ( Schemer et al., 2008 ). The scale of Vishwanath et al. (2011) has often been cited: heuristic processing includes 4 items, such as “I skimmed (i.e., moved quickly) through the Facebook message” and “I briefly looked at the sender/source of the message”; systematic processing includes 3 items, such as “I thought about the action I took based on what I saw in the Facebook message.” Although different scales had different contents and quantities of items, they all adopted a five-point Likert scale.
Attention to and elaboration of the subsystems of the HSM have also been measured by self-reports, but the specific measurement methods were different. Some studies have referred to the scale of Eveland et al. (2003) or Eveland and Dunwoody (2002) . Some studies have used alternative methods for measures. For example, Harrison et al. (2016b) used response length (word count) as a measure to capture the level of elaboration, while the degree of elaboration was measured by an open-ended item asking participants why they did or did not do something. For attention, the researchers measured attention to email elements by accurately recalling email elements. They found that elaboration and attention were significantly correlated with each other such that individuals who showed more elaboration of the message also showed more attention to the message elements.
Influencing factors can be measured through existing scales, such as the BFI personality trait scale ( John and Srivastava, 1999 ), suspicion scale ( Lyons et al., 2011 ), suspicion of humanity scale ( McKnight et al., 2003 ; Wright and Marett, 2010 ), cyber-risk beliefs scale ( Vishwanath et al., 2016 ), risk beliefs scale ( Jarvenpaa et al., 2000 ; Malhotra et al., 2004 ; Wright and Marett, 2010 ), perceived risk scale ( Drolet and Morrison, 2001 ; Grazioli and Wang, 2001 ), domain-specific knowledge scale ( Vishwanath et al., 2011 ), subjective e-mail knowledge and experience scale ( Harrison et al., 2016b ), web experience scale ( Everard and Galletta, 2005 ; Wright and Marett, 2010 ), and email habits scale ( Verplanken and Orbell, 2003 ), or influencing factors can be controlled through experiments. For example, Wang et al. (2012) gave a time and fear atmosphere (for example, if the requested information was not provided within 7 days, the users would lose their email accounts indefinitely).
Defence strategies under the HSM
Within the theoretical framework of the heuristic-systematic model, countermeasures to susceptibility to online fraud mainly include technology, education and simulated scene training.
Huang et al. (2022) suggested that online fraud may involve inherent human weaknesses, such as lack of attention. Based on eye-tracking data, they developed a human-technical solution that generates adaptive visual aids (ADVERT) to direct users’ attention to the email content instead of peripheral cues. They reported success in a case study based on a human experimental dataset from New York University. Chen and Yang (2022) also developed an advanced deep attention collaborative filter to help users analyse social information directly or indirectly to detect spam, which was tested successfully in a case study based on the context of an educational organisation. In addition, previous studies have found that device affordance may affect heuristic processing by leading users to relax their cognitive participation in information processing, reducing their cognitive resource investment, enabling them to perform heuristic processing on cognitive information, and thus making them vulnerable to online fraud ( Kim and Sundar, 2015 ; Vishwanath, 2016 ). The use of technology to defend against fraud attacks mediated by intelligent devices has also shown positive results, such as spam blockers ( Vishwanath et al., 2011 ), fraud risk identification systems ( Frauenstein and Flowerday, 2020 ), and anti-phishing software and toolbars ( Wright and Marett, 2010 ).
Groups with high vulnerability to online fraud are generally characterised by a lack of relevant network security knowledge and poor risk perception. Education can enrich individuals’ network security knowledge reserves and enhance their risk beliefs. The results of current studies show that education is the most promising way to prevent phishing ( Wright and Marett, 2010 ). To implement educational measures, network security knowledge education should be strengthened, such as targeting training and education on email deception detection ( Harrison et al., 2016a ), legal initiatives to combat internet deception ( Grazioli, 2004 ), and user training efforts ( Luo et al., 2013 ). People who do not have specific domain knowledge are less able to detect deceptive information; they tend to perform peripheral processing and rely on simple clues embedded in emails during information processing and thus make incorrect decisions and suffer from online fraud ( Vishwanath et al., 2011 ). Improved knowledge through education can help people identify fraud clues more easily, increase attention to and elaboration of the information, and reduce the likelihood of being victimised by phishing ( Harrison et al., 2016b ). Additionally, people’s risk perception ability should be improved through education, such as cyber-risk belief education ( Vishwanath et al., 2016 ), security awareness education programmes ( Frauenstein and Flowerday, 2020 ), and scam awareness training ( Wang et al., 2012 ).

Simulated scene training
Simulated scene training is an embedded education method that involves users role-playing on a mocked-up email inbox and being presented with several different scenarios. Participants are exposed to several types of email phishing and are able to experience the results of appropriate and inappropriate responses ( Kumaraguru et al., 2007 ; Sheng et al., 2007 ; Wright and Marett, 2010 ). This measure has been officially recognised; for example, to prevent phishing, institutions such as the New York State government have adopted contextual training in which users are sent simulated phishing emails and are given materials on combating phishing at the end of the research ( Wright and Marett, 2010 ). Through lifelike interaction, network users immersed in the simulated network fraud environment can learn relevant anti-fraud knowledge and experience it actively, intuitively and vividly while effectively improving their sense of network self-efficacy. This makes them more confident when processing information related to network fraud in reality and ultimately reduces the likelihood of responding to network fraud information.
The heuristic system and internet fraud victimisation
The analysed literature seems to agree that network fraud is related to heuristic processing and the analytic processing mode is used to identify fraud. This is because the heuristic system relies on intuition, the parallel processing speed is fast, and decision errors occur easily, whereas the analytic system relies more on rationality, the processing speed is slow, and the error probability is relatively low ( Chaiken, 1980 ; Evans, 2003 ). This conclusion has also been confirmed by interpersonal deception theory and the theory of deception ( Johnson et al., 1992 ; Buller and Burgoon, 1996 ; Johnson et al., 2001 ). However, despite the experimental results of the heuristic-analytic system and vulnerability to online deception, the supporting evidence is not solid.
First, there are few direct empirical studies in the literature (only the three studies reported here: Vishwanath et al., 2016 , Harrison et al., 2016b , and Frauenstein and Flowerday, 2020 ). Second, these three studies do not absolutely support the explanation of online fraud victimisation by the HSM. For example, Frauenstein and Flowerday (2020) did not find that systematic processing has a significant correlation with phishing susceptibility. Third, some researchers do not agree with the division of the two systems in the decision-making and reasoning process. For example, Moshman (2000) suggested that the heuristic system has an implicit nature while the analytic system has an automated nature, and the division of the two systems cannot cover the whole process of decision-making and reasoning. If there is no dual system division, the prediction of the likelihood of network fraud victimisation by heuristic processing is difficult to support. Last but not least, the view that the rational analytic system must be superior to the intuitive heuristic system may be incorrect. On the basis of the assumptions of bounded rationality and ecological rationality, Gigerenzer and the ABC Research Group under his guidance discovered and proposed the “Fast and frugal heuristics” ( Gigerenzer, 1996 , 2008a , b ; Goldstein and Gigerenzer, 2002 ; Gigerenzer et al., 2008 ; Liu, 2009 ). A large number of studies showed that “Fast and frugal heuristics” was reasonable and efficient cognitive strategies to save information. For example, Gigerenzer and Gaissmaier (2011) found that ignoring part of the information could lead to more accurate judgments than weighting and adding all information, for instance for low predictability and small samples. The existence of these uncertain or controversial viewpoints require more effective research to demonstrate the correlation between heuristic information processing mode and network fraud.
According to the views of the scholars in our study, attention and elaboration are regarded as subsystems of the HSM ( Frauenstein and Flowerday, 2020 ; Gao, 2021 ), and scholars regard attention and elaboration as subsystems of the ELM ( Petty and Cacioppo, 1986 ). Empirical studies also confirm the influence of attention and elaboration on the susceptibility to online fraud ( Vishwanath et al., 2011 ; Toma and Hancock, 2012 ). It is important to note that attention is not focused on the number of clues but on the quality of the clues, which is used to judge online fraud. Theoretically, the explanation for the HSM is the use of cognitive busyness or cognitive laziness ( Petty and Wegener, 1999 ), adjustment insufficiency ( Epley and Gilovich, 2004 ), and intuitive confidence ( Simmons and Nelson, 2006 ). However, these mechanisms have not been suggested in current studies that adopt the HSM to explain susceptibility to online fraud. In addition, Gigerenzer (2008a) has summarized 10 kinds of “Fast and frugal heuristics “, such as recognition heuristics (if one of the two or more options is recognized, it is inferred that it has a higher validity value), adoption of the best heuristics (search the clue according to the validity of the clue, and terminate the search once the clue that can distinguish the two options is encountered). More evidence is needed to confirm which “quick thrift heuristic” is associated with online fraud victims.
The above discussion does not aim to deny the relationship between the heuristic-analytic system and online fraud victimisation. Despite research on the relationship between the two processing systems and susceptibility to online fraud or on the relationship between the explanation mechanism (the subsystems) of the two processing systems and susceptibility to online fraud, further demonstration is needed.
In our research, exploration of the influencing factors was conducted within the HSM framework, which is different from simply studying the influencing factors of susceptibility to online fraud. In the process of analysis, psychological factors are unstable variables, and different studies have mutually exclusive results. For example, in the study of motivation, generally speaking, individuals with the motivation to process information pay attention to key information arguments and then carry out elaboration processing ( Luo et al., 2013 ). However, decisions deteriorate when the amount of information involved is large enough to exceed individuals’ normal experience ( Ariely et al., 2009 ). Risk perception under situational demand involves uncertainty, which may be caused by different definitions of risk perception. If risk perception is regarded as a permanent personality, individuals with strong cyber-risk beliefs may be able to activate systematic processing to better identify online fraud ( Vishwanath et al., 2016 ). However, if there is a state of fear caused by threat elements in the information, it may increase vulnerability to deception ( Das et al., 2003 ) or have no influence ( Das et al., 2003 ; Wright and Marett, 2010 ).
In addition, since personality traits have been applied to the HSM framework for the first time ( Frauenstein and Flowerday, 2020 ), their mechanism needs to be further explored. In contrast to the above factors, high GCS increases uncertainty, which leads to the systematic processing of available information and more accurate phishing detection ( Harrison et al., 2016a ). This finding is consistent with previous studies ( Wright and Marett, 2010 ). Are there other psychological factors that influence the selection of the heuristics and analytic systems?
Forgas and East (2008) found that the emotions of online users affect their ability to detect deception. When users feel sad, their detection ability improves. According to the ELM, under relatively low thinking conditions, similar to other variables, emotions can affect attitudes through various low effort processes. However, when the likelihood of thinking is relatively high, these same emotions can affect persuasion through other mechanisms ( Petty and Briñol, 2014 ). Whether emotions affect susceptibility to online fraud by influencing the mediating effect of the heuristic and systematic processing modes needs to be further explored. Building workers often live far from their families, which can lead to loneliness over time ( Schonfeld and Chang, 2017 ). A survey found that to eliminate loneliness and insecurity, they chose to make friends online, which led to online cheating in relationships.
Within the framework of the HSM, a relatively consistent conclusion is that knowledge and experience, especially the specific knowledge and experience related to online fraud, are protective factors against online fraud ( Wright and Marett, 2010 ). Interpersonal deception research puts experience at the centre of the fraud detection process; experience can improve the accuracy of identifying deceptive information ( Feeley et al., 1995 ). When relevant events are stored and easily accessible, it is easier to make connections between the information received and relevant events, so those with relevant knowledge and experience are better able to process new information elaborately. Two points should be noted: (a) knowledge can be divided into subjective knowledge and objective knowledge, with more emphasis on objective knowledge ( Harrison et al., 2016b ), and (b) prior knowledge may involve a biased review of externally provided information ( Crocker et al., 1984 ).
The influence of device affordance and habits on online fraud victimisation is a relatively new area of research. Previous studies have found that a large screen size and video mode of smartphones facilitate heuristic processing, while a small screen size and text mode facilitate systematic processing ( Kim and Sundar, 2015 ). However, Vishwanath (2016) suggested that mobile devices such as smartphones have an impact on the susceptibility of fraud victims by reinforcing habits rather than affecting cognitive processing. This requires consideration of a deeper question of whether habits affect information processing patterns. Regarding online fraud, this research is lacking and needs to be further enhanced.
Measures of the heuristic-analytic processing mode, influencing factors and the likelihood of internet fraud victimisation
The validity and reliability of the scales used were not reported, although there are scales to measure the heuristic and systematic processing modes (including attention and elaboration). Scales to measure the influencing factors were previously available and are not discussed here. We mainly discuss the data collection method used in the research, the simulation experiment. First, this method of data collection is generally agreed upon by experimental subjects in advance, so there are no ethical issues. However, the participants’ environment, the expectation of the stimulating nature of the experiment, the degree of attention, the loss when making incorrect decisions and other factors are very different from real online fraud ( Jones and Towse, 2018 ; Gao, 2021 ). Second, whether users click the link in phishing emails ( Luo et al., 2013 ; Harrison et al., 2016b ) and whether they provide the private information requested in phishing emails ( Wang et al., 2012 ) are used to measure vulnerability to online fraud, which is not equivalent to ultimately being cheated. Third, the subjects used in the experiments were ordinary people ( Vishwanath et al., 2011 ) rather than real victims. Although there may be self-report bias when real victims are used as subjects, this situation is more realistic and objective in terms of influencing factors.
Compared with the defence strategies proposed in the literature that are included in our analysis, previous defence strategies in the non-HSM framework focused on two aspects: technology and education. However, in the framework of the HSM, defence technology for online fraud is more prominent in guiding potential victims to initiate the systematic processing mode (traditional technology emphasises internet fraud information blocking from the government, internet providers, shopping and other related websites). For example, Huang et al. (2022) developed a human-technical solution that generates adaptive visual aids (ADVERT) to direct the user’s attention to the email content instead of peripheral cues. In education, while attaching importance to knowledge and experience, some researchers have proposed simulated scene training ( Kumaraguru et al., 2007 ; Sheng et al., 2007 ). Through lifelike interaction, network users immersed in a simulated network fraud environment can learn relevant anti-fraud knowledge and experience it more actively, intuitively and vividly, effectively improving their sense of network risk perception and self-efficacy and avoiding the cognitive load caused by intensive publicity and education ( Williams and Noyes, 2007 ).
However, simulated scene training also suffers from certain challenges. For example, when simulated phishing studies are used, participants who choose to respond to emails may feel embarrassed and upset because they demonstrate the same vulnerability as real-life victims ( Jones et al., 2015 ). Some scholars have suggested that participants participate in the simulation scenario in an informed manner and conduct the internet fraud attack test after a period of time. However, these problems may still occur if the participants are subjected to an online fraud attack test after they forget they have joined, and the possibility of users responding will be reduced if the participants are fully informed ( Mack, 2014 ). Therefore, in the future, it is necessary to further optimise the simulation scenario and to improve the simulation process and the simulation education effect.
Limitations of this review
The current systematic review is not without limitations. On the one hand, due to keyword selection and database limitations, the number of studies that met the selection criteria was small. Therefore, this study may not cover all the research on online fraud under the HSM framework. The current research only includes articles published in peer-reviewed journals and written in English. Future research could incorporate papers published in other venues (e.g., conference papers) or could further systematically review papers published in other languages on this subject. Nevertheless, this study reviews the relationship between individual information processing modes and online fraud victimisation, influencing factors, heuristic and analytic systems and their explanatory mechanisms, measures of influencing factors, and defence strategy, laying a theoretical foundation for research in this field. In addition, some research gaps were found in this study that provide a direction for future work in this new research area. This study did not conduct a statistical significance level test and effect size determination on the results of previous studies and involved a systematic review rather than a meta-analysis. This is mainly because there were few included articles and different research directions, which could not meet the preconditions for meta-analysis ( Cheung and Vijayakumar, 2016 ). Meta-analytical research is encouraged when a sufficient number of studies share similar research types and variables.
The two systems of decision-making and reasoning are in the initial stages of explaining online fraud victimisation; nevertheless, they show that online fraud victimisation may be related to humans’ inherent weakness in decision-making. When individuals face online fraud information, if they activate the heuristic processing mode to process the information, they may increase the likelihood of victimisation. According to the defence strategy under the HSM, technical application that emphasises directing users’ attention to the content of emails as well as immersive simulated scene training may provide a major breakthrough in combating online fraud in the future. However, the verification of the heuristic and analytic processing modes for the prediction of network fraud victimisation as well as the explanatory mechanism and influencing factors need to be further expanded.
Author contributions
YS: ideas, data collection, writing, and revisions. KW: writing and revisions. YT and YZ: revisions and analysis. BM: writing and data collection. SL: writing, ideas, and data collection. All authors contributed to the article and approved the submitted version.
This work was supported by the Legal Construction and Legal Theory Research Project of China (grant no. 20SFB4038).
Conflict of interest
The authors declare that the research was conducted in the absence of any commercial or financial relationships that could be construed as a potential conflict of interest.
Publisher’s note
All claims expressed in this article are solely those of the authors and do not necessarily represent those of their affiliated organizations, or those of the publisher, the editors and the reviewers. Any product that may be evaluated in this article, or claim that may be made by its manufacturer, is not guaranteed or endorsed by the publisher.
Supplementary material
The Supplementary material for this article can be found online at: https://www.frontiersin.org/articles/10.3389/fpsyg.2023.1087463/full#supplementary-material
Aarts, H., Verplanken, B., and Van Knippenberg, A. (1998). Predicting behavior from actions in the past: repeated decision making or a matter of habit? J. Appl. Soc. Psychol. 28, 1355–1374. doi: 10.1111/j.1559-1816.1998.tb01681.x
CrossRef Full Text | Google Scholar
Aleroud, A., and Zhou, L. (2017). Phishing environments, techniques, and countermeasures: a survey. Comput. Secur. 68, 160–196. doi: 10.1016/j.cose.2017.04.006
Alseadoon, I., Chan, T., Foo, E., and Gonzalez Nieto, J. (2012). Who is more susceptible to phishing emails?: A Saudi Arabian study. ACIS 2012 Proceedings, 21. Available at: https://aisel.aisnet.org/acis2012/21
Google Scholar
Anderson, K. B. (2004). Consumer fraud in the United States: An FTC survey . Washington, DC: Federal Trade Commission.
Anderson, K. B. (2013). Consumer fraud in the United States, 2011: The third FTC survey . Washington, DC: Federal Trade Commission.
Ariely, D., Bracha, A., and Meier, S. (2009). Doing good or doing well? Image motivation and monetary incentives in behaving prosocially. Am. Econ. Rev. 99, 544–555. doi: 10.1257/aer.99.1.544
Ashton, M. C., and Lee, K. (2009). The HEXACO–60: a short measure of the major dimensions of personality. J. Pers. Assess. 91, 340–345. doi: 10.1080/00223890902935878
PubMed Abstract | CrossRef Full Text | Google Scholar
Bargh, J. A., and Gollwitzer, P. M. (1994). “Environmental control of goal-directed action: automatic and strategic contingencies between situations and behavior,” in Integrative views of motivation, cognition, and emotion . eds. W. D. Spaulding and H. A. Simon (London, LON: University of Nebraska Press), 71–124.
Bullée, J. W. H., Montoya, L., Pieters, W., Junger, M., and Hartel, P. H. (2015). The persuasion and security awareness experiment: reducing the success of social engineering attacks. J. Exp. Criminol. 11, 97–115. doi: 10.1007/s11292-014-9222-7
Buller, D. B., and Burgoon, J. K. (1996). Interpersonal deception theory. Commun. Theory 6, 203–242. doi: 10.1111/j.1468-2885.1996.tb00127.x
Burnes, D., Henderson, C. R. Jr., Sheppard, C., Zhao, R., Pillemer, K., and Lachs, M. S. (2017). Prevalence of financial fraud and scams among older adults in the United States: a systematic review and meta-analysis. Am. J. Public Health 107, e13–e21. doi: 10.2105/AJPH.2017.303821
Burnes, D., Sheppard, C., Henderson, C. R. Jr., Wassel, M., Cope, R., Barber, C., et al. (2019). Interventions to reduce ageism against older adults: a systematic review and meta-analysis. Am. J. Public Health 109, e1–e9. doi: 10.2105/AJPH.2019.305123
Button, M., Lewis, C., and Tapley, J. (2009). Fraud typologies and the victims of fraud: Literature review . London: National Fraud Authority.
Canfield, C. I., Fischhoff, B., and Davis, A. (2016). Quantifying phishing susceptibility for detection and behavior decisions. Hum. Factors 58, 1158–1172. doi: 10.1177/0018720816665025
Carcach, C., Graycar, A., and Muscat, G. (2001). The victimisation of older Australians (Vol. 212). Canberra, Australia: Australian Institute of Criminology.
Chaiken, S. (1980). Heuristic versus systematic information processing and the use of source versus message cues in persuasion. J. Pers. Soc. Psychol. 39, 752–766. doi: 10.1037/0022-3514.39.5.752
Chaiken, S. (1987). “The heuristic model of persuasion,” in Social influence: The Ontario symposium , vol. 5. eds. M. P. Zanna, J. M. Olson, and C. P. Herman (Hillsdale, NJ: Psychology Press), 3–39.
Chen, S., and Chaiken, S. (1999). “The heuristic-systematic model in its broader context” in Dual-process theories in social psychology . eds. S. Chaiken and Y. Trope (New York, NY: The Guilford Press), 73–96.
Chen, Y., and Yang, Y. (2022). An advanced deep attention collaborative mechanism for secure educational email services. Comput. Intell. Neurosci. 2022, 1–9. doi: 10.1155/2022/3150626
Cheung, M. W. L., and Vijayakumar, R. (2016). A guide to conducting a meta-analysis. Neuropsychol. Rev. 26, 121–128. doi: 10.1007/s11065-016-9319-z
Cho, J. H., Cam, H., and Oltramari, A. (2016). “Effect of personality traits on trust and risk to phishing vulnerability: Modeling and analysis,” in 2016 IEEE International Multi-Disciplinary Conference on Cognitive Methods in Situation Awareness and Decision Support (CogSIMA) , 7–13.
Cialdini, R. B. (2001). The science of persuasion. Sci. Am. 284, 76–81. doi: 10.1038/scientificamerican0201-76
Cialdini, R. B. (2018). Influence: the psychology of persuasion. Gyan Manag. J. 12, 69–70.
Cohen, L. E., and Felson, M. (2010). “Social change and crime rate trends: a routine activity approach (1979),” in Classics in environmental criminology . eds. M. A. Andresen, P. J. Brantingham, and J. B. Kinney (New York, NY: Routledge), 203–232.
Cohen, L. E., Kluegel, J. R., and Land, K. C. (1981). Social inequality and predatory criminal victimization: An exposition and test of a formal theory. Am. Sociol. Rev. 505–524. doi: 10.2307/2094935
Crocker, J., Fiske, S. T., and Taylor, S. E. (1984). “Schematic bases of belief change,” in Attitudinal judgment . ed. J. Richard Eiser (New York, NY: Springer), 197–226.
Das, E. H., De Wit, J. B., and Stroebe, W. (2003). Fear appeals motivate acceptance of action recommendations: evidence for a positive bias in the processing of persuasive messages. Personal. Soc. Psychol. Bull. 29, 650–664. doi: 10.1177/0146167203029005009
Drolet, A. L., and Morrison, D. G. (2001). Do we really need multiple-item measures in service research? J. Serv. Res. 3, 196–204. doi: 10.1177/109467050133001
Eagly, A. H., and Chaiken, S. (1993). The psychology of attitudes . Fort Worth, TX: Harcourt Brace Jovanovich College Publishers.
Epley, N., and Gilovich, T. (2004). Are adjustments insufficient? Personal. Soc. Psychol. Bull. 30, 447–460. doi: 10.1177/0146167203261889
Evans, J. S. B. (2003). In two minds: dual-process accounts of reasoning. Trends Cogn. Sci. 7, 454–459. doi: 10.1016/j.tics.2003.08.012
Eveland, W. P. Jr., and Dunwoody, S. (2002). An investigation of elaboration and selective scanning as mediators of learning from the web versus print. J. Broadcast. Electron. Media 46, 34–53. doi: 10.1207/s15506878jobem4601_3
Eveland, W. P. Jr., Shah, D. V., and Kwak, N. (2003). Assessing causality in the cognitive mediation model: a panel study of motivations, information processing, and learning during campaign 2000. Commun. Res. 30, 359–386. doi: 10.1177/0093650203253369
Everard, A., and Galletta, D. F. (2005). How presentation flaws affect perceived site quality, trust, and intention to purchase from an online store. J. Manag. Inf. Syst. 22, 56–95. doi: 10.2753/MIS0742-1222220303
Feeley, T. H., de Turck, M. A., and Young, M. J. (1995). Baseline familiarity in lie detection. Commun. Res. Rep. 12, 160–169. doi: 10.1080/08824099509362052
Fischer, P., Schulz-Hardt, S., and Frey, D. (2008). Selective exposure and information quantity: how different information quantities moderate decision makers’ preference for consistent and inconsistent information. J. Pers. Soc. Psychol. 94, 231–244. doi: 10.1037/0022-3514.94.2.94.2.231
Forgas, J. P., and East, R. (2008). On being happy and gullible: mood effects on skepticism and the detection of deception. J. Exp. Soc. Psychol. 44, 1362–1367. doi: 10.1016/j.jesp.2008.04.010
Frauenstein, E. D., and Flowerday, S. (2020). Susceptibility to phishing on social network sites: a personality information processing model. Comput. Secur. 94:101862. doi: 10.1016/j.cose.2020.101862
Frey, D. (1986). Recent research on selective exposure to information. Adv. Exp. Soc. Psychol. 19, 41–80. doi: 10.1016/S0065-2601(08)60212-9
Gao, Y. (2021). Influencing factors of college Students’ susceptibility to online fraud. Master’s thesis. Zhejiang University. Available at: https://kns.cnki.net/KCMS/detail/detail.aspx?dbname=CMFD202102andfifilename=1021610212.nh
Gavett, B. E., Zhao, R., John, S. E., Bussell, C. A., Roberts, J. R., and Yue, C. (2017). Phishing suspiciousness in older and younger adults: the role of executive functioning. PLoS One 12:e0171620. doi: 10.1371/journal.pone.0171620
Gigerenzer, G. (1996). On narrow norms and vague heuristics: a reply to Kahneman and Tversky. Psychol. Rev. 103, 592–596. doi: 10.1037/0033-295X.103.3.592
Gigerenzer, G. (2008a). Why heuristics work. Perspect. Psychol. Sci. 3, 20–29. doi: 10.1111/j.1745-6916.2008.00058.x
Gigerenzer, G. (2008b). “Moral intuition=fast and frugal heuristics?” in Moral psychology . ed. W. E. Sinnott-Armstrong (Cambridge, MA: MIT Press), 1–26.
Gigerenzer, G., and Gaissmaier, W. (2011). Heuristic decision making. Annu. Rev. Psychol. 62, 451–482. doi: 10.1146/annurev-psych-120709-145346
Gigerenzer, G., Hoffrage, U., and Goldstein, D. G. (2008). Fast and frugal heuristics are plausible models of cognition: reply to Dougherty, Franco-Watkins, and Thomas (2008). Psychol. Rev. 115, 230–239. doi: 10.1037/0033-295X.115.1.230
Goldstein, D. G., and Gigerenzer, G. (2002). Models of ecological rationality: the recognition heuristic. Psychol. Rev. 109, 75–90. doi: 10.1037/0033-295x.109.1.75
Grazioli, S. (2004). Where did they go wrong? An analysis of the failure of knowledgeable internet consumers to detect deception over the internet. Group Decis. Negot. 13, 149–172. doi: 10.1023/B:GRUP.0000021839.04093.5d
Grazioli, S., and Wang, A. (2001). “Looking without seeing: understanding unsophisticated consumers’ success and failure to detect internet deception.” in ICIS 2001 Proceedings . 23, 193–204. Available at: https://aisel.aisnet.org/icis
Griffin, R. J., Neuwirth, K., Giese, J., and Dunwoody, S. (2002). Linking the heuristic-systematic model and depth of processing. Commun. Res. 29, 705–732. doi: 10.1177/009365002237833
Grothmann, T., and Reusswig, F. (2006). People at risk of flooding: why some residents take precautionary action while others do not. Nat. Hazards 38, 101–120. doi: 10.1007/s11069-005-8604-6
Harrison, B., Svetieva, E., and Vishwanath, A. (2016b). Individual processing of phishing emails: how attention and elaboration protect against phishing. Online Inf. Rev. 40, 265–281. doi: 10.1108/OIR-04-2015-0106
Harrison, B., Vishwanath, A., and Rao, R. (2016a). “A user-centered approach to phishing susceptibility: the role of a suspicious personality in protecting against phishing.” in 2016 49th Hawaii international conference on system sciences (HICSS). pp. 5628–5634. IEEE.
Holtfreter, K., Reisig, M. D., and Blomberg, T. G. (2006). Consumer fraud victimization in Florida: an empirical study. St. Thomas L. Rev. 18, 761–789.
Holtfreter, K., Reisig, M. D., and Pratt, T. C. (2008). Low self-control, routine activities, and fraud victimization. Criminology 46, 189–220. doi: 10.1111/j.1745-9125.2008.00101.x
Huang, L., Jia, S., Balcetis, E., and Zhu, Q. (2022). Advert: an adaptive and data-driven attention enhancement mechanism for phishing prevention. IEEE Trans. Inf. Forensics Secur. 17, 2585–2597. doi: 10.1109/TIFS.2022.3189530
Jakobsson, M. (2007). The human factor in phishing. Priv. Sec. Cons. Info. 7, 1–19.
James, B. D., Boyle, P. A., and Bennett, D. A. (2014). Correlates of susceptibility to scams in older adults without dementia. J. Elder Abuse Negl. 26, 107–122. doi: 10.1080/08946566.2013.821809
Jarvenpaa, S. L., Tractinsky, N., and Vitale, M. (2000). Consumer trust in an internet store. Inf. Technol. Manag. 1, 45–71. doi: 10.1023/A:1019104520776
John, O. P., and Srivastava, S. (1999). “The big-five trait taxonomy: History, measurement, and theoretical perspectives,” in Handbook of Personality: Theory and Research . eds. L. A. Pervin and O. P. John (New York, NY: Guilford Press.), 102–138.
Johnson, P. E., Grazioli, S., Jamal, K., and Berryman, R. G. (2001). Detecting deception: adversarial problem solving in a low base-rate world. Cogn. Sci. 25, 355–392. doi: 10.1207/s15516709cog2503_2
Johnson, P. E., Grazioli, S., Jamal, K., and Zualkernan, I. A. (1992). Success and failure in expert reasoning. Organ. Behav. Hum. Decis. Process. 53, 173–203. doi: 10.1016/0749-5978(92)90061-b
Jones, H. S., and Towse, J. (2018). “Examinations of email fraud susceptibility: perspectives from academic research and industry practice,” in Psychological and behavioral Examinations in Cyber Security . eds. J. McAlaney, L. A. Frumkin, and V. Benson (Pennsylvania, PA: IGI Global), 80–97.
Jones, H. S., Towse, J. N., and Race, N. (2015). Susceptibility to email fraud: a review of psychological perspectives, data-collection methods, and ethical considerations. Int. J. Cyber Behav. Psychol. Learn. 5, 13–29. doi: 10.4018/IJCBPL.2015070102
Judges, R. A., Gallant, S. N., Yang, L., and Lee, K. (2017). The role of cognition, personality, and trust in fraud victimization in older adults. Front. Psychol. 8:588. doi: 10.3389/fpsyg.2017.00588
Kim, K. J., and Sundar, S. S. (2015). Mobile persuasion: can screen size and presentation mode make a difference to trust? Hum. Commun. Res. 42, 45–70. doi: 10.1111/hcre.12064
Kumaraguru, P., Rhee, Y., Acquisti, A., Cranor, L. F., Hong, J., and Nunge, E. (2007). “Protecting people from phishing: the design and evaluation of an embedded training email system.” in Proceedings of the SIGCHI conference on human factors in computing systems. pp. 905–914.
Langenderfer, J., and Shimp, T. A. (2001). Consumer vulnerability to scams, swindles, and fraud: a new theory of visceral influences on persuasion. Psychol. Mark. 18, 763–783. doi: 10.1002/mar.1029
Larcom, G., and Elbirt, A. J. (2006). Gone phishing. IEEE Technol. Soc. Mag. 25, 52–55. doi: 10.1109/MTAS.2006.1700023
LaRose, R., and Eastin, M. S. (2004). A social cognitive theory of internet uses and gratifications: toward a new model of media attendance. J. Broadcast. Electron. Media 48, 358–377. doi: 10.1207/s15506878jobem4803_2
Levine, T. R., and McCornack, S. A. (1991). The dark side of trust: conceptualizing and measuring types of communicative suspicion. Commun. Q. 39, 325–340. doi: 10.1080/01463379109369809
Liu, Y. F. (2009). Fast and frugal heuristics: the related debates and brief comments. Adv. Psychol. Sci. 17, 885–892.
Loewenstein, G. (1996). Out of control: visceral influences on behavior. Organ. Behav. Hum. Decis. Process. 65, 272–292. doi: 10.1006/obhd.1996.0028
Luo, X. R., Zhang, W., Burd, S., and Seazzu, A. (2013). Investigating phishing victimization with the heuristic-systematic model: a theoretical framework and an exploration. Comput. Secur. 38, 28–38. doi: 10.1016/j.cose.2012.12.003
Lyons, J. B., Stokes, C. K., Eschleman, K. J., Alarcon, G. M., and Barelka, A. J. (2011). Trustworthiness and IT suspicion: an evaluation of the nomological network. Hum. Factors 53, 219–229. doi: 10.1177/0018720811406726
Mack, S. (2014). Reasoning and judgements made in an online capacity. An exploration of how phishing emails influence decision making strategies unpublished dissertation . Lancaster University, Lancaster, UK.
Malhotra, N. K., Kim, S. S., and Agarwal, J. (2004). Internet users’ information privacy concerns (IUIPC): the construct, the scale, and a causal model. Inf. Syst. Res. 15, 336–355. doi: 10.1287/isre.1040.0032
McKnight, D. H., Kacmar, C., and Choudhury, V. (2003). “Whoops—Did I use the wrong construct to predict e-commerce trust? Modeling the risk-related effects of trust versus distrust concepts.” in Proceeding of the thirty-sixth Hawaii international conference on social systems.
McKnight, D. H., Kacmar, C. J., and Choudhury, V. (2004). Dispositional trust and distrust distinctions in predicting high-and low-risk internet expert advice site perceptions. E-Service 3, 35–58. doi: 10.2979/esj.2004.3.2.35
Modic, D., Anderson, R., and Palomäki, J. (2018). We will make you like our research: the development of a susceptibility-to-persuasion scale. PLoS One 13:e0194119. doi: 10.1371/journal.pone.0194119
Modic, D., and Lea, S. E. (2012). How neurotic are scam victims, really? The big five and internet scams. 2448130.
Modic, D., and Lea, S. E. (2013). Scam compliance and the psychology of persuasion. 2364464.
Moher, D., Liberati, A., Tetzlaff, J., and Altman, D. G., PRISMA Group* (2009). Preferred reporting items for systematic reviews and meta-analyses: the PRISMA statement. Ann. Intern. Med. 151, 264–269. doi: 10.7326/0003-4819-151-4-200908180-00135
Moody, G. D., Galletta, D. F., and Dunn, B. K. (2017). Which phish get caught? An exploratory study of individuals’ susceptibility to phishing. Eur. J. Inf. Syst. 26, 564–584. doi: 10.1057/s41303-017-0058-x
Moshman, D. (2000). Diversity in reasoning and rationality: metacognitive and developmental considerations. Behav. Brain Sci. 23, 689–690. doi: 10.1017/S0140525X00483433
Muncaster, P. (2020). #COVID19 drives phishing emails up 667% in under a month. Infosecurity Magazine. Online. Available at: https://www.infosecurity-magazine.com/news/covid19-drive-phishing-emails-667/
Norris, G., Brookes, A., and Dowell, D. (2019). The psychology of internet fraud victimization: a systematic review. J. Police Crim. Psychol. 34, 231–245. doi: 10.1007/s11896-019-09334-5
Perse, E. M. (1990). Audience selectivity and involvement in the newer media environment. Commun. Res. 17, 675–697. doi: 10.1177/009365090017005005
Petty, R. E., and Briñol, P. (2014). Emotion and persuasion: cognitive and meta-cognitive processes impact attitudes. Cognit. Emot. 29, 1–26. doi: 10.1080/02699931.2014.967183
Petty, R. E., and Cacioppo, J. T. (1986). “The elaboration likelihood model of persuasion,” in Communication and persuasion . eds. R. E. Petty and J. T. Cacioppo (New York, NY: Springer), 1–24.
Petty, R. E., Cacioppo, J. T., and Goldman, R. (1981). Personal involvement as a determinant of argument-based persuasion. J. Pers. Soc. Psychol. 41, 847–855. doi: 10.1037/0022-3514.41.5.847
Petty, R. E., and Wegener, D. T. (1999). “The elaboration likelihood model,” in Current status and controversies in dual-process theories in social psychology . eds. S. Chaiken and Y. Trope (New York, NY: Guilford Press), 37–72.
Ratneshwar, S., and Chaiken, S. (1991). Comprehension’s role in persuasion: the case of its moderating effect on the persuasive impact of source cues. J. Consum. Res. 18, 52–62. doi: 10.1086/209240
Ross, L. (1981). “The “intuitive scientist” formulation and its developmental implications” in Social cognitive development: Frontiers andpossible futures . eds. J. H. Flaveil and L. Ross (London and New York: Cambridge University Press)
Rothstein, H. G. (1986). The effects of time pressure on judgment in multiple cue probability learning. Organ. Behav. Hum. Decis. Process. 37, 83–92. doi: 10.1016/0749-5978(86)90045-2
Salthouse, T. (2012). Consequences of age-related cognitive declines. Annu. Rev. Psychol. 63, 201–226. doi: 10.1146/annurev-psych-120710-100328
Schemer, C., Matthes, J., and Wirth, W. (2008). Toward improving the validity and reliability of media information processing measures in surveys. Commun. Methods Meas. 2, 193–225. doi: 10.1080/19312450802310474
Schonfeld, I. S., and Chang, C. H. (2017). Occupational health psychology: Work, stress, and health . eds. D. Wang and Y. Hu (Trans. Shanghai: East China Normal University Press).
Schwarz, N., Frey, D., and Kumpf, M. (1980). Interactive effects of writing and reading a persuasive essay on attitude change and selective exposure. J. Exp. Soc. Psychol. 16, 1–17. doi: 10.1016/0022-1031(80)90032-3
Shah, D. V., Kwak, N., Schmierbach, M., and Zubric, J. (2004). The interplay of news frames on cognitive complexity. Hum. Commun. Res. 30, 102–120. doi: 10.1111/j.1468-2958.2004.tb00726.x
Shang, Y., Wu, Z., Du, X., Jiang, Y., Ma, B., and Chi, M. (2022). The psychology of the internet fraud victimization of older adults: a systematic review. Front. Psychol. 13:912242. doi: 10.3389/fpsyg.2022.912242
Sheng, S., Magnien, B., Kumaraguru, P., Acquisti, A., Cranor, L. F., Hong, J., et al. (2007). “Anti-phishing phil: the design and evaluation of a game that teaches people not to fall for phish.” in Proceedings of the 3rd symposium on usable privacy and security. pp. 88–99.
Simmons, J. P., and Nelson, L. D. (2006). Intuitive confidence: choosing between intuitive and nonintuitive alternatives. J. Exp. Psychol. Gen. 135, 409–428. doi: 10.1037/0096-3445.135.3.409
Sloman, S. A. (1996). The empirical case for two systems of reasoning. Psychol. Bull. 119, 3–22. doi: 10.1037/0033-2909.119.1.3
Stamm, K., and Dube, R. (1994). The relationship of attitudinal components to trust in media. Commun. Res. 21, 105–123. doi: 10.1177/009365094021001006
Sundar, S. S. (2008). The MAIN model: A heuristic approach to understanding technology effects on credibility (pp. 73–100). Cambridge, MA: Mac Arthur Foundation Digital Media and Learning Initiative.
Sundar, S. S., Knobloch-Westerwick, S., and Hastall, M. R. (2007). News cues: information scent and cognitive heuristics. J. Am. Soc. Inf. Sci. Technol. 58, 366–378. doi: 10.1002/asi.20511
Tade, O., and Aliyu, I. (2011). Social organization of internet fraud among university undergraduates in Nigeria. Int. J. Cyber Criminol. 5, 860–875.
Toma, C. L., and Hancock, J. T. (2012). What lies beneath: the linguistic traces of deception in online dating profiles. J. Commun. 62, 78–97. doi: 10.1111/j.1460-2466.2011.01619.x
Trumbo, C. W. (2002). Information processing and risk perception: an adaptation of the heuristic-systematic model. J. Commun. 52, 367–382. doi: 10.1093/joc/52.2.367
Tversky, A., and Kahneman, D. (1974). Judgment under uncertainty: heuristics and biases: biases in judgments reveal some heuristics of thinking under uncertainty. Science 185, 1124–1131. doi: 10.1126/science.185.4157.1124
United States Department of Justice (2017). Lithuanian man arrested for theft of over $100 million in fraudulent email compromise scheme against multinational internet companies [Press release]. Available at: https://www.justice.gov/usao-sdny/pr/lithuanian-man-arrested-theft-over-100-million-fraudulent-email-compromise-scheme
Valecha, R., Mandaokar, P., and Rao, H. R. (2022). Phishing email detection using persuasion cues. IEEE Trans. Dependable Secure Comput. 19, 1–756. doi: 10.1109/TDSC.2021.3118931
Verizon. (2019). 2019 data breach investigations report (DBIR). Available at: https://enterprise.verizon.com/resources/reports/2019-data-breachinvestigations-report.pdf
Verplanken, B., and Orbell, S. (2003). Reflections on past behavior: a self-report index of habit strength. J. Appl. Soc. Psychol. 33, 1313–1330. doi: 10.1111/j.1559-1816.2003.tb01951.x
Vishwanath, A. (2015). Habitual Facebook use and its impact on getting deceived on social media. J. Comput.-Mediat. Commun. 20, 83–98. doi: 10.1111/jcc4.12100
Vishwanath, A. (2016). Mobile device affordance: explicating how smartphones influence the outcome of phishing attacks. Comput. Hum. Behav. 63, 198–207. doi: 10.1016/j.chb.2016.05.035
Vishwanath, A., Harrison, B., and Ng, Y. J. (2016). Suspicion, cognition, and automaticity model of phishing susceptibility. Commun. Res. 45, 1146–1166. doi: 10.1177/0093650215627483
Vishwanath, A., Herath, T., Chen, R., Wang, J., and Rao, H. R. (2011). Why do people get phished? Testing individual differences in phishing vulnerability within an integrated, information processing model. Decis. Support. Syst. 51, 576–586. doi: 10.1016/j.dss.2011.03.002
Wang, J., Herath, T., Chen, R., Vishwanath, A., and Rao, H. R. (2012). Research article phishing susceptibility: an investigation into the processing of a targeted spear phishing email. IEEE Trans. Prof. Commun. 55, 345–362. doi: 10.1109/TPC.2012.2208392
Watts, S. A., and Zhang, W. (2008). Capitalizing on content: information adoption in two online communities. J. Assoc. Inf. Syst. 9, 73–94. doi: 10.17705/1jais.00149
Whitty, M. T. (2013). The scammers persuasive techniques model: development of a stage model to explain the online dating romance scam. Br. J. Criminol. 53, 665–684. doi: 10.1093/bjc/azt009
Whitty, M. T. (2015). Mass-marketing fraud: a growing concern. IEEE Secur. Priv. 13, 84–87. doi: 10.1109/MSP.2015.85
Whitty, M. T. (2019). Predicting susceptibility to cyber-fraud victimhood. J. Financ. Crime 26, 277–292. doi: 10.1108/JFC-10-2017-0095
Williams, D. J., and Noyes, J. M. (2007). How does our perception of risk influence decision-making? Implications for the design of risk information. Theor. Issues Ergon. Sci. 8, 1–35. doi: 10.1080/14639220500484419
Workman, M. (2008). A test of interventions for security threats from social engineering. Inf. Manag. Comput. Secur. 16, 463–483. doi: 10.1108/09685220810920549
Wright, P. (1974). The harassed decision maker: time pressures, distractions, and the use of evidence. J. Appl. Psychol. 59, 555–561. doi: 10.1037/h0037186
Wright, R. T., and Marett, K. (2010). The influence of experiential and dispositional factors in phishing: an empirical investigation of the deceived. J. Manag. Inf. Syst. 27, 273–303. doi: 10.2753/MIS0742-1222270111
Keywords: internet fraud victims, the heuristic-systematic model, influencing factors, measure, defence strategies
Citation: Shang Y, Wang K, Tian Y, Zhou Y, Ma B and Liu S (2023) Theoretical basis and occurrence of internet fraud victimisation: Based on two systems in decision-making and reasoning. Front. Psychol . 14:1087463. doi: 10.3389/fpsyg.2023.1087463
Received: 02 November 2022; Accepted: 12 January 2023; Published: 06 February 2023.
Reviewed by:
Copyright © 2023 Shang, Wang, Tian, Zhou, Ma and Liu. This is an open-access article distributed under the terms of the Creative Commons Attribution License (CC BY) . The use, distribution or reproduction in other forums is permitted, provided the original author(s) and the copyright owner(s) are credited and that the original publication in this journal is cited, in accordance with accepted academic practice. No use, distribution or reproduction is permitted which does not comply with these terms.
*Correspondence: Sanyang Liu, ✉ [email protected]
Disclaimer: All claims expressed in this article are solely those of the authors and do not necessarily represent those of their affiliated organizations, or those of the publisher, the editors and the reviewers. Any product that may be evaluated in this article or claim that may be made by its manufacturer is not guaranteed or endorsed by the publisher.
- Open access
- Published: 13 March 2023
Online payment fraud: from anomaly detection to risk management
- Paolo Vanini ORCID: orcid.org/0000-0003-0391-4847 1 ,
- Sebastiano Rossi 2 ,
- Ermin Zvizdic 3 &
- Thomas Domenig 4
Financial Innovation volume 9 , Article number: 66 ( 2023 ) Cite this article
12k Accesses
13 Citations
Metrics details
Online banking fraud occurs whenever a criminal can seize accounts and transfer funds from an individual’s online bank account. Successfully preventing this requires the detection of as many fraudsters as possible, without producing too many false alarms. This is a challenge for machine learning owing to the extremely imbalanced data and complexity of fraud. In addition, classical machine learning methods must be extended, minimizing expected financial losses. Finally, fraud can only be combated systematically and economically if the risks and costs in payment channels are known. We define three models that overcome these challenges: machine learning-based fraud detection, economic optimization of machine learning results, and a risk model to predict the risk of fraud while considering countermeasures. The models were tested utilizing real data. Our machine learning model alone reduces the expected and unexpected losses in the three aggregated payment channels by 15% compared to a benchmark consisting of static if-then rules. Optimizing the machine-learning model further reduces the expected losses by 52%. These results hold with a low false positive rate of 0.4%. Thus, the risk framework of the three models is viable from a business and risk perspective.
Introduction
Fraud arises in the financial industry via numerous channels, such as credit cards, e-commerce, phone banking, checks, and online banking. Juniper Research ( 2020 ) reports that e-commerce, airline ticketing, money transfer, and banking services will cumulatively lose over $ 200 billion due to online payment fraud between 2020 and 2024. The increased sophistication of fraud attempts and the increasing number of attack vectors have driven these results. We focus on online and mobile payment channels and identity theft fraud (i.e., stealing an individual’s personal information to conduct fraud )(Amiri and Hekmat 2021 ). The aim is to identify external fraudsters who intend to initiate payments in their interests. As fraudsters gain access to the payment systems as if they were the owners of the accounts, they cannot be identified based on the account access process. However, the fraudster behaves differently during a payment transaction than the account owner and/or the payment has unusual characteristics, such as an unusually high payment amount or transfer to an account in a jurisdiction that does not fit the life context and payment behavior of the customer. The assumption is that algorithms can detect anomalies in behavior during payment transactions.
West and Bhattacharya ( 2016 ), Abdallah et al. ( 2016 ), Hilal et al. ( 2021 ), and Ali et al. ( 2022 ) reviewed financial fraud. They found a low number of articles regarding online payment fraud. For example, Ali et al. ( 2022 ) cited 20 articles on financial statement fraud and 32 articles on credit card fraud, see Li et al. ( 2021 ) for credit card fraud detection. Online payment fraud was not listed. The reviews also clarified that many articles utilized aggregated characteristics. However, we emphasize that fraud in online payments can only be detected based on individual data, as such fraud can only be detected through the possible different behavior of the fraudster and the account holder during payments. As fraudsters learn how best to behave undetected over time, they adapt their behavior. Therefore, self-learning defense methods are expected to outperform static-rule-based algorithms. The correctness of the expectation was shown by Abdallah et al. ( 2016 ) and Hilal et al. ( 2021 ). Various machine-learning algorithms for fraud detection have been proposed in the literature, including decision trees, support vector machines, and logistic regression to neural networks. Aggarwal and Sathe ( 2017 ) discussed various methods for outlier ensembles, and Chandola et al. ( 2009 ) provided a taxonomy and overview of anomaly detection methods.
A common feature in many studies is imbalanced data (i.e., the low proportion of fraud events in the dataset, see Wei et al. 2013 ; Carminati et al. 2015 ; Zhang et al. 2022a ; Singh et al. 2022 ). Risk detection involves detecting fraudulent transactions and stopping them before execution.
In addition to the efficiency of the algorithms, the data basis is an important reason for the differences in fraud-detection performance. While many studies have utilized either often less rich synthetic or Kaggle data, we were able to work with real data. Log files, which have substantial information content in our work, are hardly expected in Kaggle data. The difference in the data complexity is also reflected in the number of features. Singh et al. ( 2022 ) showed that the feature space consists of 31 features compared to our 147 features. Moreover, the proportion of fraudulent transactions in Singh et al. ( 2022 ) is more than a hundred times higher than in our case. Consequently, our data are much more unbalanced than any other study we know of, and the task of finding efficient fraud detection algorithms is more difficult.
However, limiting risk management to the optimal detection of anomalies does not ensure that losses caused by fraud are minimal. Optimal fraud detection can be economically suboptimal if, for example, it is efficient for small amounts of money but unsuccessful for large amounts. Thus, the machine learning outputs for risk identification must be optimized from an economic perspective. We call this optimization the triage model. Yet, neither fraud detection nor the triage model can provide an answer to the question of how large the losses in a payment channel are. Therefore, we develop a statistical risk model that considers the effects of countermeasures on loss potential. The risk model provides risk transparency and makes it possible to assess which measures in the fight against fraud in various payment channels make sense from an economic and risk perspective.
Literature on fraud risk models often refers to qualitative or assessment models for assessing fraud risk or risk assessment models (Sabu et al. 2021 ). We are not aware of any quantitative fraud risk management framework that explicitly considers the impact of the fraud detection process statistically in risk modelling. For organizational, procedural, and legal risk aspects, we refer to the literature. Montague’s ( 2010 ) book focuses on fraud prevention in online payments but does not consider machine learning and risk management in detail. The Financial Conduct Authority’s Handbook (FCA 2021 ) provides a full listing of the FCA’s legal instruments, particularly those relating to financial crime in financial institutions. Power ( 2013 ) highlights the difference between fraud and fraud risk from historical and business perspectives. Van Liebergen ( 2017 ) looks at “regtech” applications of machine learning in online banking. Fraud risk events in cryptocurrency payment systems are different from the online banking cases under consideration; see Jung et al. ( 2019 ) for fraud acting on a decentralized infrastructure and the review article of Trozze et al. ( 2022 ).
The development and validation of the three linked models are the main contributions of our work. To our knowledge, this is the first study to develop, validate, and link components of the risk management process. The output of the anomaly detection model (i.e., the ROC curves), is the input for the triage model, which provides economically optimized ROC curves. Fraud statistics data were utilized to calibrate the various components in the risk model. With these three models, the fraud risk management process can be qualitatively implemented at the same level as the risk management of market or counterparty risks (see Bessis ( 2011 ) to describe risk management in banks).
The performance of our risk management framework is the second contribution, although the performance comparison of our fraud detection method with the literature is limited and cautious, due the use of synthetic data instead of real data, a consideration of different channels in payments with different behavioral characteristics of bank customers, and the publication of incomplete statistics. Nevertheless, we compared our work with Wei et al. ( 2013 ) and Carminati et al. ( 2015 ), both of which analyze online banking fraud based, in part, on real data. The true positive rate (TPR) at a false positive rate (FPR) of \(1\%\) was \(45\%\) . In Wei et al. the TPR is between \(49\%\) and \(60\%\) , but unfortunately, the FPR is unknown. In the relevant scenario of Carminati et al. ( 2015 ), the TPR is \(70\%\) with an FPR of \(14\%\) . This FPR is not acceptable to any bank. Processing by specialists leads to high costs. We discuss all these statements in detail in the " Validation results " section. Considering all three models, the theoretical and practical importance of our approach becomes clear. The expected losses in a scenario of CHF 2.023 million, which utilizes the results of machine learning without economic optimization in the triage model, common in the literature, are reduced to CHF 0.800 million with the triage model (i.e., a reduction in the loss potential by more than 60% follows). In addition, if fraud detection is implemented without a risk model, fraud risk can be massively overestimated. Applying our models to three different payment channels, the overestimation of risk ranged from 54% to over 700%.
The remainder of this paper is organized as follows. In " Fraud risk management framework " section, the model selection for the fraud risk management framework is motivated and described. In " Online payment fraud anomaly detection " section, we consider the anomaly-detection model. " Fraud detection triage model " section links fraud detection to an economic perspective utilizing the triage model. " Risk model " presents the statistical risk model. " Conclusion " section concludes.
Fraud risk management framework
We provide an overview of the three interrelated quantitative models in the context of risk management: online payment anomaly detection, triage model, and risk model.
Online payment fraud anomaly detection
The goal of anomaly detection is to detect fraudulent activities in e-banking systems and to maintain the number of false alarms at an acceptable level. The implementation of the model consists of three steps: pre-filter, feature extraction, and machine learning.
Non-learning pre-filters ensure that both obvious fraud and normal transactions are sorted early to reduce the false positive rate. Only transactions that pass the pre-filter step are passed on to the machine-learning model. Banks utilize non-customer-based static if-then rules, such as blacklists or whitelists. Pre-filters free the algorithms from obvious cases. The adaptability and flexibility of the machine-learning model is necessary to counter the ever-improving attacks of fraudsters with effective fraud detection.
Our data face the following general challenges in payment fraud detection (per Wei et al. 2013 ): large transaction volume with the need for real-time fraud detection, a highly imbalanced dataset, dynamic fraud behavior, limited forensic information, and varying customer behavior.
Given the extremely imbalanced data, fully supervised algorithms typically struggle. Aggarwal and Sathe ( 2017 ) proposed unsupervised and weakly supervised approaches based on features that encode deviations from normal behavior. For each customer participating in an e-banking session, we assess whether the agent’s behavior is consistent with the account holder’s normal behavior. The key information for behavioral analysis lies in the sequence of the customer’s clicks during the session. We show that, unlike online e-commerce transactions (see Wei et al. 2013 ), transaction data, customer behavior data, account data, and booking data are also important for the performance of the algorithm. More precisely, these features are divided into behavioral, transactional, and customer-related features. Starting with nearly 800 features, 147 were extracted utilizing a Bagged Decision Tree Model (BDT). These numbers are many times higher than those for credit card fraud with one to two dozen features (see Table 8 in Hilal et al. 2022 ). The high dimensionality of the feature space also arises in machine learning corporate default prediction models, where several steps are needed to extract all noisy features (see Kou et al. 2021 ).
Our e-fraud model operates according to the following principles.
The model learns the “normal” behavior of each customer based on historical payment data and online banking log data.
Each new transaction is checked against the learned “normal” behavior to determine if it is an anomaly by extracting the 147 features from the data.
If an anomaly is detected, it is flagged as suspected fraud.
Detected transactions that are not found to be fraudulent after manual review are reported back to the model for learning purposes.
As there are very few known fraud cases, all base learners are trained on fraud-free data only in step one. Fraud cases are only utilized in step two of ensemble aggregation when the base learners are combined to form the final predictive function. The first step is to define base learners who are rich enough to detect a wide range of suspicious transactions or online user sessions. We consider three base learners: the density-based outlier detection model (unsupervised, Local Outlier Factor (LOF)), the isolation-based outlier detection model (unsupervised, Isolation Forest (IF)), and a model for normal customer behavior (supervised, Bagged Decision Trees (BDT)) as base learners (see Breunig et al. ( 2000 ), Chandola et al. ( 2009 ); Zhang et al. ( 2022b ) for LOF, Liu et al. ( 2012 ); Tokovarov and Karczmarek ( 2022 ) for IF). We refer to individual instances of LOF, IF, or BDT as base learners. The BDT model is not only a base model, but it is also utilized for feature selection in the other two base models: LOF and IF. The LOF method is suitable for outlier detection, where each observation is assigned an outlier level based on its distance from the nearest cluster of neighboring observations. The aim is to detect outliers in inhomogeneous data, for which classical global outlier methods typically do not provide satisfactory results. Conversely, IF explicitly isolates anomalies without capturing all normal instances. These two methods consider the heterogeneity in the data.
In the second stage, the base learner’s fraud score was aggregated. We consider two approaches to determine the weights in the ensembles: a simple averaging and a supervised approach, although our model largely consists of unsupervised procedures because of the limited availability of fraud cases for which we can extract all the required features. However, we introduce supervision where we utilize scarce labelled data to adjust the importance of certain base learners in the voting scheme, ultimately deciding whether an observation is fraudulent. The penalized logistic regression chosen for classification allows for a better interpretation of the model, as the weights can be utilized to identify base learners, subsets of features, and subsets of samples that have been particularly useful in detecting a particular type of fraud.
Triage model
The fraud detection model calculates scores and, in comparison with a threshold value, decides whether a transaction is flagged as an anomaly. This process results in the probability of detection for a given investigation effort, as indicated by the ROC curve. By making the threshold dependent on the transaction size, we can ensure that larger transaction amounts are more likely detected than smaller ones. This gives up part of the true positive rate (TPR) to reduce overall economic losses (i.e., the TPR decreases for a given FPR). This economic optimization that leads to adjusted ROC curves defines the triage model.
To minimize expected cumulative losses, the constant fraud anomaly detection threshold becomes a function of the transaction amount. Here, the transaction amounts are random variables whose distributions are estimated. In the optimization problem, the transaction function is chosen to maximize the average cumulative sum of the detected fraudulent transactions, where the expected FPR must not exceed a certain threshold. Utilizing this optimal threshold function, the fitted ROC curves were obtained.
The optimization problem has a unique solution if the ROC curve is a concave function of the false positive rate function of the threshold and if the acceptance set of the expected false positive function constraint is convex. With the chosen piecewise linear false positive constraint function, the assumptions regarding the existence of an optimum are satisfied. The ROC curves that result when fraud anomalies are detected serve as inputs for the optimization. However, because only a vanishingly small number of fraud cases exist, the TPR values for certain FPR levels are subject to considerable uncertainty. Hence, cubic spline functions were utilized for the ROC curve of the optimization.
The UK Finance (2019) report states that the recovery value for online and mobile banking in the UK is 18% of the potential loss. Therefore, we introduced an extension to the optimization program to include recovery.
Losses from transaction fraud are included in operational risk incurred by banks. As for other operational risks, one of the key questions from a risk-management perspective is whether the allocated resources and countermeasures are adequate. To answer this, one needs some way of quantifying the risk incurred, ideally a Value-at-Risk (VaR)-model that fits the general risk framework of the bank. Simply, the model calculates the loss \(L=E(\lambda )\times E(\tau )\) where \(\lambda\) is the expected event frequency (fraud), and \(\tau\) is the expected loss per event. The challenge is to determine the distributions of these variables in a tractable and plausible manner and define a model while having very scarce data on past events. We chose the path of an aggregated simulation of many scenarios per e-channel to account for the inherent uncertainty in the choice of these parameters.
Unlike market or credit risk, fraud risk is borne by comparatively few individuals or groups who utilize very specific strategies and technologies to overcome vulnerability in the payment process. Simultaneously, defenders analyze attack plans and update their countermeasures. In this constantly changing environment, neither the frequency of attacks nor the transaction amounts can be assumed to be statistically regular with great certainty. Therefore, we propose a simple, flexible stochastic model composed of basic building blocks. With such a model, risk managers can quickly adjust the model as needed, perform what-if analyses, or simulate changes in payment infrastructure.
The basic model structure for the e-fraud risk model consists of (i) independent models for the three channels, whose components and parameters can be flexibly assembled and adjusted, (ii) sub-models in each channel based on three model types, and (iii) a recovery model for each channel. The three model types for the three different online payment channels in this study are a Beta model (restricted distribution of transaction amounts), a Generalized Pareto Distribution (GPD, unrestricted distribution of transaction amounts), and a “mass attack model” (many simultaneous Beta-type attacks).
Countermeasures against fraud and recovery measures after fraud events play an essential role in determining risk potential. Therefore, they were integrated into the risk models. Countermeasures against online fraud can be divided into those that strengthen the general infrastructure of the payment process and those that focus on defense against actual attacks. The former is conceptually part of the risk model described above, as it affects the frequency and possibly the transaction size of the attacks. However, the latter is better understood in the context of recovery and is considered in the triage model.
Raw data consisted of transaction data, interaction data between customer and e-banking interface, account, booking, and customer reference data. All users with fewer than 10 logged online sessions were removed as input for ensemble learning. The removed cases were handled separately by utilizing a case-back model.
The transaction history in our dataset consists of 140 million transactions over three years. One hundred fraud cases are reported, but only 11 cases can be linked to the recorded 900’000 online session logs: a \(0.0012\%\) fraud rate. Only 900’000 of the 140 million transactions were possible as the log files were only stored in the bank for three months. This change occurred after the project.
A feature vector is created for each e-banking session based on raw data. The interaction pattern features consist of n -grams constructed from customers’ request sequences and normalized deviations from the expected duration between each pair of consecutive requests sent by a customer in an online session. Particular attention was paid to the typical time required to complete a two-step verification process during enrolment. Payment pattern characteristics were calculated for weekday, weekly, and monthly seasonality. These include normalized deviations from the expected payment amount and remaining account balance. Technical data included the IP address of the online session, the HTML agent, and the number of JavaScript scripts executed. Finally, we utilized historically observed confirmed fraudulent transaction identifiers as the ground truth for the weakly monitored part of the pipeline.
Several quality checks were performed. Consistency tests ensure that the session interaction data and transactions match, for example, that the account exists or that a recipient is listed in the transaction. We also checked for missing or non-parsable values, the latter removed.
The data are extracted from several different data sources within the bank in a two-step Python extract, transform, and load (ETL) process, and converted into features for the algorithm. First, we introduce all raw data into a standard structured format for all data sources. Then, we perform the feature engineering described in the following sections to compute the data input for the ensemble.
Our fraud rate \(0.0012\%\) is much lower than that reported in the literature. The figures in the two online banking fraud papers, Wei et al. ( 2013 ) and Carminati et al. ( 2015 ), are \(0.018\%\) and \(1\%\) , respectively. For credit card fraud, the fraud case number is larger, such as \(2\%\) in Piotr et al. ( 2008 ). Outside the banking fraud sector, anomalies account for up to 40% of the observations (see Pang et al. 2020 ). Similar numbers hold in Zhang et al. ( 2022b ), who tested their ensemble-based outlier detection methods on 35 real datasets from various sectors outside the financial sector, with an average fraud rate of 26%.
Feature extraction
For weak supervision, we utilized historically observed confirmed fraudulent transaction identifiers as the ground truth. For training and inference, we created a feature vector for each e-banking session based on the raw data. Each feature aims to encode deviation from expected (“normal”) customer behavior, as observed in historical interactions with the online banking interface and executed transactions. Three types of features are considered.
Behavioral features
The underlying motivation for utilizing features derived from customers’ online session logs is that a large fraction of online payment fraud involves hijacking, where a foreign agent (human or robot fraudster) takes control of the e-banking session. Consequently, it is expected that the timing and sequence of requests posted by the user in a fraudulent session will be significantly different from those in a non-fraudulent session. We utilize the information about user request types (e.g., “get account balance”, “create payment”) and corresponding timestamps to construct the following features:
Normalized n-gram frequency of user’s requests within the online session. We utilized single, pairs and triplets of consecutive requests (1-, 2- and 3-grams) to derive a fixed-size representation. We performed normalization by dividing the number of occurrences for each request with the total number of requests in each session.
Normalized time between consecutive user request n-grams. For each pair of recorded consecutive n-grams, we transformed absolute time between them into deviations by computing z-scores relative to respective historical observations.
Technical attributes of a session (e.g., IP address of the online session, HTML agent, screen size, and the number of executed Javascript scripts) in binary format - 0 if previously observed, otherwise 1.
Transactional features
Transactional features aim to quantify how anomalous aggregate payments scheduled in an online session are compared with previously executed payments and the remaining balance on the account. They are designed to capture attempts to empty a victim’s account through a single large or many small transactions, while being mindful of seasonal patterns (e.g., holidays, travel expenses, bills, etc.).
Normalized ratio of the payment amount relative to remaining account balance. We normalize by computing z-scores relative to historically observed ratios.
Deviation of the scheduled payment amount from the seasonally expected amount. We compute four deviations per session using z-scores relative to historical payments executed in the same hour of the day, day of the week, week of the month, and month of the year, respectively.
Scheduled time for payment execution in binary format - 0 if immediate, 1 if lagged.
A short payment history and many accounts with relatively infrequent transactions proved detrimental to seasonality modelling, hence, these features were omitted from the final model.
Customer-related features
Customer-related features provide insight into peer groups, relationships with other customers, and the service packages they utilize. These include:
Sociodemographic (e.g., age, nationality, profession, and income)
Seniority of client relationship
Product usage (i.e., savings, investment accounts, and mortgages)
Relationship to other customers (i.e., shared accounts, spouses, and family)
These features were not considered within the scope of our study because of data limitations and time constraints.
Functionality and structure of the fraud model
Base learner: bagged decision tree.
Bagged decision trees (BDT) are trained utilizing the concept of transfer learning, which assumes that distinguishing between the behaviors of different clients within their online sessions is a related problem in distinguishing between fraudulent and non-fraudulent sessions. The underlying motivation considers that a large fraction of online payment fraud involves hijacking or when a foreign agent (human or robot fraudster) takes control of the e-banking session. As fraudulent sessions are rare and non-fraudulent sessions abound, the utilization of transfer learning enables the extraction of custom patterns from a much broader dataset and the use of supervised learning. Transfer learning comprises two phases. A learning phase where one discriminates behavioral characteristics of each customer vs. non-customers and a prediction phase where discrimination between non-fraudulent and fraudulent users sessions were considered in this study. The “non-customer” class label is then attributed to fraudulent behavior.
The decision function in BDT base learners is the probability that an observation, a planned transaction x , is a “non-customer behavior.” This value is equal to the average probability observed for all decision trees in the forest.
where M is the number of trees in the bagged forest, \(c_j\) is the corresponding customer behavior class, and \(P_i( Y\ne c_j|X=x)\) is the probability that observation x is a “non-customer behavior” as predicted by the i th tree. Customer behavior class \(c_j\) consists of the collected sessions and transactions, excluding potential fraud. The model was fitted as follows:
For each customer \(c_j\) , we collect the associated sessions, excluding the potential fraud cases. This set represents the “behavior of customer \(c_j\) .”
From the pool of all customer sessions \(C_{-j}\) (excluding \(c_j\) ) we draw a uniform sample of observations to generate a set representing the class “behavior of customer \(c_j\) not,” \(\not c_j\) for short, and equal in size to the \(c_j\) set. Equal sampling is performed to ensure that none of the other customers are overrepresented in \(\not c_j\) .
Each bagged forest in the ensemble is trained on a matching feature subspace utilizing all these observations. The forests consist of 100 decision trees each.
BDTs provide variable selection as an additional benefit, owing to the large amount of data involved in supervised classification. Therefore, they can be utilized to estimate the importance of variables and adequacy of feature engineering. We achieve this by calculating the Gini impurity decreases at each split point within each tree for each feature. Gini impurity is a measure of the likelihood that a randomly selected observation would be incorrectly classified by a specific node m :
where \(p_{mi}\) is the portion of samples classified as i at node m . These impurity decreases were averaged across all decision trees and outputs to estimate the importance of each input variable. The greater the decrease in impurity, the greater the importance. Utilizing these results, we can identify the relevant subsets of input variables.
Relying on the concept of transfer learning (if the problem described in ?? is sufficiently like fraud detection), we use BDT to select a subset of \(N=147\) features. Particularly important were features measuring the deviation from the typical time required to complete a two-step verification process during login. Features that encode the relative time between n -grams and specific user request sequences are important. The following additional base model was built using the features selected by BDT.
Base learner: local outlier factor
The Local Outlier Factor (LOF) detection method assigns an outlier level to each observation based on its distance from the nearest cluster of neighboring observations (Breunig et al. 2000 ). The general intent of the LOF model is to identify outliers in the interior region of data, for which classical global outlier methods and the other considered algorithm, isolation forest, usually do not provide satisfactory results. The LOF decision function is as follows:
with K a k -neighborhood and LD ( x ) the local reachability distance density from x to its k -th nearest neighbor. We fit the model for each customer by collecting the first associated sessions or transactions, excluding potential fraud. Each LOF in the ensemble is created utilizing all these observations on a subspace of the relevant features selected by BDT and the sampled hyper-parameter. Finally, each time a new observation is available, its decision function value is computed regarding the observations from the training set.
Base learner: isolation forest
The IF algorithm recursively splits the data into two parts based on a random threshold, until each data point is isolated. The algorithm randomly selects a feature at each step, and then randomly selects a division value between its minimum and maximum values. The algorithm filters out data points that require fewer steps to be isolated from the entire dataset. In our case, IF separates one observation from the rest of a randomly selected subsample of the original dataset (Fei Tony et al. 2008 ). Anomalies are instances with short average isolation path lengths. The IF decision function is
where E ( H ( x )) is the average number of edges traversed to isolate the node and C is the average number of edges traversed in an unsuccessful search. To fit the model, we first collected each client’s associated sessions or transactions, excluding potential fraud cases. In step two, each isolation forest in the ensemble was created utilizing all these observations in a matching feature subspace. Each forest consisted of 100 isolated trees. Finally, each time a new observation was available, its decision function value was computed regarding the isolation trees created based on the training set.
Base learner scores combination
The decision functions of the base learners produced by our ensembles must be combined into a single fraud score. As these are in different ranges and scales to render the decision functions comparable, we first replace the original scores by their ranks, regarding the non-fraudulent training scores. Rank normalization is more robust and numerical stable as opposed to z-scores, for example. Therefore, we replaced the original scores with their ranks regarding the non-fraudulent training scores for each base learner:
where V is the set of all \(\delta _{\text {Base}}(p )\) over all observations \(p'\) in the learners’ training subsample, with Base being LOF, IF, or BDT.
Owing to the few fraud cases, our model largely consists of unsupervised procedures. However, we introduced supervision utilizing scarce labelled data to readjust the importance of particular base learners in the voting scheme, ultimately deciding whether an observation is fraudulent.
The following score combination procedure was established. First, a training set comprises all fraud cases in the sample, along with healthy transactions uniformly sampled over customers from the ensemble training data. Second, a logistic regression is trained to classify observations as fraudulent or not utilizing the 6 N normalized decision function features and the known fraud status on past transactions as the label.
The following binary-class penalized cost function is minimized:
where \(y_i\) is the fraud label of transaction i , \(X_i'\) is a row of 6 N decision functions describing transaction i , R is the regularization factor, and ( w , c ) is a set of weights defining the decision boundary between the two classes: fraud and non-fraud. To account for the imbalance between fraud and non-fraud transactions in our sample, we assign asymmetric penalties for fraud misclassification, as opposed to non-fraud classification.
Choosing logistic regression to optimize the weights of the base learners ensures that the final score combination \(x'w\) represents the log-odds of an observation to be fraudulent.
where \(\delta (x)\) is the probability that the observation x is fraudulent. Finally, to assign a fraud label to a session x , we compare the output combined score, or equivalently, the probability \(\delta (x)\) , to a threshold y , which is chosen based on ROC curve analysis such that the defined maximum allowed false positive rate is not exceeded. The decision boundary of logistic regression is linear, where each base learner is assigned a weight \(w_i\) to determine its relative importance for fraud classification. This structure simplifies the interpretation of the model because these weights can be utilized to identify the base learners, feature subsets, and sample subsets, which are particularly useful in detecting a particular type of fraud associated with a high weight \(w_i\) . Appendix A provides a detailed description of the ensemble design.
Normal customer behavior model
In summary, we created an ensemble model for each client, which is re-trained with new data at regular intervals and can be described by the following steps:
We consider the disjoint sets of behavioral features on session observations and transactional features on transaction observations.
For each of the two features/observations-pairs, we define \(N=1000\) learners for each of the three model categories as follows.
We fix N random sub-samples of features from the feature set. Each sub-sample remains fixed for all customers.
For each customer, we fix N random observation samples from the customer-specific sessions or transactions observations.
For each of the three model categories, for each customer, and for \(i=1,...,N\) , a base learner is defined by applying the model algorithm to the i -th features sub-sample and i -th observations sub-sample. Thus, this results in 6 N base learners per customer, 3 N for sessions, and another 3 N for transaction data.
The decisions for the three base learners are aggregated utilizing supervision, where the knowledge obtained from existing fraud cases is utilized to adjust the base learner weights.
Utilizing this representation, we train a model that (i) outputs an indicator of how likely a scheduled transaction is fraudulent, (ii) aggregates the overall provided decision functions to derive the unified hypothesis, while assigning more importance to learners that showcase the capability to better distinguish between fraud and non-fraud, and (iii) deals with a large imbalance in class representation.
Validation results
The training, test, and validation sets consisted of data collected from July to October 2017, as dictated by the availability of online session log files. Around 900’000 sessions formed the dataset.
Raw data were processed using ETL to derive customer-specific feature representations of each recorded online session. The data were then split into non-fraud and fraud sets. The fraud set was not utilized to train the unsupervised base learners. Non-fraudulent (“healthy”) sessions were separated into training and test sets utilizing a 3-fold cross-validation split. We then sequentially trained the models on each derived training fold and computed the scores for observations in the corresponding test folds. Following, we obtained an out-of-sample decision function value for each healthy session and each base learner. We then assigned base learner scores to each fraudulent session utilizing base learners trained on all healthy data.
The out-of-sample logistic regression decision function values were aggregated by averaging within their respective ensembles (LOFs, IFs, and BDTs). This step yields a 3-dimensional representation of each customer’s online session. Finally, we utilized leave-one-out cross-validation to report the ROC curve measures. Hence, the logistic regression model is consecutively trained on an all-but-one observation, followed by computing the probability of an observation that was left out. Thus, we again obtain an out-of-sample fraud probability for each observation in the sample. We opted for leave-one-out cross-validation to maximize the number of fraudulent observations in each training set, because these are particularly scarce. Once we have obtained the aforementioned out-of-sample probabilities for each observation, we construct an ROC curve to display the FPR and TPR relationship depending on the decision threshold.
Resultantly, when utilizing no transaction data, the detection rate of the machine learning model was in a realistic range of 18% true positives. These primary results can be easily optimized to increase the TPR and simultaneously reduce the FPR utilizing different measures. This led to an increase in true positives by up to 45%, see Table 1 .
Overall, LOF seems to perform best over the entire dataset compared to IF and BDT. However, BDT has a slightly steeper ROC curve at the beginning, thus showing better pure outlier detection capabilities. Furthermore, because BDT seems to detect frauds, as discussed below, involving larger amounts than those detected by LOF, we cannot conclude that LOF outperforms the other approaches considered. Aggregating the decision functions of the ensembles utilizing simple means outperformed supervised aggregation. Through analysis of logistic regression weights assigned to each ensemble of learners, we determined that significantly higher weights were assigned to the LOF ensemble, most likely due to its best performance over the whole dataset. This dampened the input from the other two ensembles. However, this is not the case when the mean for aggregation is utilized. The results were affected by the small number of frauds and the size of the sample analyzed.
Different ensembles detected different types of fraud, and by observing figures depicting money saved per raised alarm, we see that different ensembles (LOF and BDT) detect different types of fraud cases, displayed by a large difference in saved money per trigger. Logistic regression supervision alarms were affected mainly by LOF, thus making it miss large embezzlement detected by the BDT ensemble. This motivates the triage model described in the next section. As of the restriction of the FPR to no greater than 2%, the entire ROC curve is of less interest. The ROC AUC values for the LOF ensemble is 0.93, for the BDT ensemble 0.82, and for the mean decision function ensemble 0.91.
We compared our results with those of Wei et al. ( 2013 ), and Carminati et al. ( 2015 ). These are two of the few studies dealing with online fraud detection that use real-world data, at least in part. Wei et al. ( 2013 ) utilized an unsupervised approach, whereas Carminati et al. ( 2015 ) utilized a semi-supervised approach. Table 2 compares the performance of our model with those of Wei et al. ( 2013 ) and Carminati et al. ( 2015 ). The results of this table should be interpreted with caution. First, different payment channels were considered. Second, the data of Carminati et al. ( 2015 ) were anonymized and did not include fraud cases. These are artificially added to tune \(1\%\) of the data volume, compared to \(0.018\%\) in Wei et al. ( 2013 ) and \(0.0012\%\) in our dataset. Third, Wei et al. ( 2013 ) did not report the FPR. Finally, Carminati et al. ( 2015 ) published an almost perfect error detection for scenarios I + II, but in scenario III, the false positives are too high; they generate too much manual work for the bank. The former scenarios are simple fraud scenarios that would be blacklisted and filtered out in our data before machine learning engages.
Fraud detection triage model
Formalization.
We formalize the triage model and denote by \(\Omega\) the set of all transactions with \(\omega\) as a single transaction, \(T(\omega )\) as the transaction amount function, and \(\chi _F(\omega )\) as the fraud indicator function, where \(\chi _F(\omega )=1\) represents fraud. Space \(\Omega\) is attributed to a probability distribution P with p ( x ) as the density function of the transaction amounts. The threshold value L of the fraud score function S is a function of the transaction amount x . We define:
If we assume stochastic independence of the transaction amount T , score S and fraud indicator \(\chi _F\) , we obtain the following interpretation:
Note that the assumptions of independence are strong, as transaction sizes are utilized as the input of the machine-learning model underlying the score. Conversely, the independence of \(\chi _f\) and T implies that the transaction amounts of fraudulent transactions have the same distribution as those of non-fraudulent transactions. In the context of the value-at-risk model in the next section, we argue that there is little evidence to support this. This considered, the assumption of independence is theoretically difficult to uphold, but in practice quite necessary to obtain our results.
We formulate our optimization problem as follows:
under the constraint of the integrated FPR
The expectation in ( 11 ) is the average cumulated sum of fraudulent transaction amounts detected by the detection model. By letting \(q_0:=E(\chi _F)\) and utilizing ( 10 ), we can rewrite it as
The constant \(q_0\) is irrelevant to the optimization. Setting \(g(x)=\text {FPR}(L(x))\) , we reformulate the optimization problem in terms of the ROC curve as
under the constraint:
To account for the recovery, we introduce a recovery function \(\theta :\Omega \rightarrow [0,1]\) . This function changes the objective function in the optimization problem, as follows:
whereas this constraint does not change.
Optimization
To put our formal model into practice, we need to fix a distribution for transaction amounts. Utilizing approximately 12 million transactions from online banking and 1.2 million from mobile banking, we approximated the distribution for both channels utilizing lognormal distributions. Although this choice does not particularly focus on the distribution’s tails, it will be seen that the optimal model still places strong emphasis on the detection of anomalies with large transaction amounts. Some basic statistics of the fitted distributions are given in Table 3 .
The ROC curve is conceptually the output of the detection model described in the previous section. However, owing to the limited number of actual fraud cases available, the TPR values for the given FPR levels are tainted with considerable uncertainty. The ROC curve utilized in our optimization was obtained by fitting a cubic spline function to the base points, as presented in Table 4 . The support points were adjusted to avoid unwanted spikes in the cubic interpolation.
As the triage model aims to prevent large losses with a higher probability than smaller ones, the optimal FPR will be an increasing function of the transaction size. To avoid possible optimization problems, we choose a simple form for FPR as a function of transaction size, namely, a piecewise linear function satisfying \(g(0) = 0\) , \(g(T_1) = a\) , \(g(T_2) = 1\) for the parameters \(a > 0\) and \(0< T_1 < T_2\) (see Fig. 1 ).
The optimization problem can be simplified by assuming equality in ( 12 ) and solving a as a function of \(T_1\) and \(T_2\) . For a target integrated FPR of 0.4%, we obtained the solutions listed in Table 5 .
Figure 1 illustrates the results for an online banking channel. The concave shape of the FPR curve up to \(T_2\) shows that the optimal solution emphasizes the detection of large transaction fraud cases, accepting, in turn, the less rigorous testing of small and moderate transactions up to \(T_1\) . For transaction amounts larger than \(T_2\) , FPR and TPR are equal to 1 by construction. Hence, all such transactions are automatically flagged as anomalies.
Total Effectiveness
is the average percentage of integrated fraudulent transaction amounts detected as anomalies. In our optimized case, the rate was 39%.
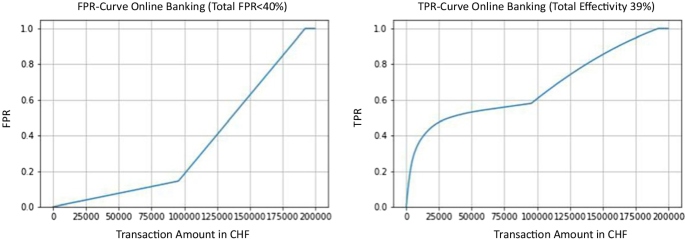
Panel Left: False positive rate as a function of the transaction amount under the constraint that the total false positive rate is smaller than 0.4 percent. Right Panel: True positive rate as a function of the transaction amount. The total effectiveness is 39 percent
Compound Poisson processes were utilized as basic building blocks. We utilize beta marginal distributions for modelling bounded transaction amounts and generalized Pareto marginal distributions (GPD) for unbounded ones. The so-called mass-attack model is formulated as a nested compound Poisson process with a marginal beta distribution. All subprocesses are aggregated independently. Loss statistics, such as value-at-risk or other quantiles of the distribution, are obtained by running Monte Carlo simulations.
Utilizing the limited available fraud data and drawing on discussions with practitioners, we develop the following model for online banking fraud:
Isolated attacks with a moderate transaction size of up to CHF 70’000 are modelled by a compound Poisson process with beta marginal distribution.
Isolated attacks with transaction amounts larger than CHF 70’000 are modelled by a compound Poisson process with GPD marginal.
“Mass attacks” are modelled as a nested compound Poisson process, where the inner Poisson process simulates the individual transactions triggered by the mass attack. The inner process has a beta marginal distribution and generates transaction amounts up to CHF 20’000.
The intensities of the Poisson processes constituting the submodels vary. In our case, isolated attacks of moderate size were by far the most frequent, followed by isolated attacks of large size. Mass attacks were the least frequent.
Mobile banking fraud is modelled analogously, albeit with transaction sizes only up to CHF 20’000, because larger amounts were inadmissible on this channel during our investigation. Hence, there is no Poisson process with GPD marginal in this case. Contrastingly, in the EBICS channel, which is an internet-based payment channel between banks, only the possibility of large fraudulent transactions was of interest. Hence, this model consists of a single compound Poisson process with GPD marginals above CHF 100’000. The details of the parametrization are given in Appendix A .
Countermeasures against fraud and recovery measures after fraud events play an essential role in determining risk potential. Therefore, they were integrated into the risk models. Countermeasures against online fraud fall into two categories: those that strengthen general infrastructure of the payment process to make it harder for attackers to find a weak spot, and those that are geared towards fighting off actual attacks. The first type is conceptually part of the base model described above, as it affects the frequency and possibly the transaction size of attacks. However, the second type is better understood in the context of recovery.
A recovery variable is introduced in the triage model, which accounts for it often being possible to recover money even after it has been transferred to another bank through fraudulent transactions. Conversely, by monitoring transactions utilizing the fraud detection and triage model, a certain percentage of attacks can be identified even before the transactions are released. The ROC curve of the detection model’s ROC curve, in combination with the triage model, allows us to infer the probability of detection from the transaction size: such that this component of the recovery process is readily integrated into the stochastic framework.
Owing to the nonlinearity of the risk statistics, the aggregation of the models was performed at the level of individual scenarios. Thus, for each scenario, \(s_i\) , the loss of the overall model for one-year was calculated from the simulated loss events of the channel models:
For each sub-model, the loss is calculated by pulling the event frequency for the year according to the Poisson intensity, loss magnitude according to the marginal distribution, and stochastic recovery:
where \(\text {Rec}\) denotes recovery function. Simulated loss figures were obtained by simulating the nested overall model, from which the risk statistics could be calculated empirically. Juniper Research ( 2020 ) estimated the recovery rate as \(18\%\) .
The simulation results for online banking are presented in Table 6 . The table shows the simulation results without applying fraud detection utilizing a constant FPR level of 0.4% and the triage model for an integrated FPR of 0.4%, respectively. In this simulation, no additional recovery was applied.
The above table shows the strong mitigation of risk due to fraud detection. The triage model performs better than the constant FPR benchmark in all submodels, particularly for the GPD submodel. Recall that the triage model places strong emphasis on detecting large fraudulent transactions, even flagging all transactions larger than CHF \(192'000\) .
As a second application, we compare the results of this risk model for the three e-channels with the bank’s overall 2019 risk policy. This means that we compare the capital-at-risk (CaR) limits for market and credit risks with operational risk limits, where the e-channel part is now calculated in our model. The following allocation of CaR holds according to the annual report of the bank Footnote 1 : Credit Risk, 69%; operational risk, 11%; market risk trading, 4%; market risk treasury, 11%; market risk real estate, 2%; and investment, 4%.
Approximately 1% of operational risk capital can be attributed to these three channels. Even if we add another 4–5% of the total volume to all payment services, including corporate banking and interbank payments, less than 10% of the operational risk capital is attributed to payment systems. As payment systems account for a significant portion of operational risk, our results confirm serious doubts about the accuracy of the chosen operational risk capital in banks. Without reliable models and data, capital is determined by utilizing dubious business indicators. Our models, which represent a micro-foundation of risk, show that, at least in payment systems, trustworthy risk quantities can be derived by combining machine learning and statistics.
Defense against sophisticated online banking fraud involve several resources and methods. These include risk models, algorithms, human action, knowledge, computer tools, web technology, and online business systems in the context of risk management.
We show that anomaly detection is not only useful per se, identifying a significant proportion of fraud while controlling false alarms, but that linking anomaly detection with statistical risk management methods can significantly reduce risk. A bank equipped with an anomaly detection system will be exposed to orders of magnitude of higher risks in payments than a bank implementing our end-to-end risk management framework with the three components of fraud detection, fraud detection optimization, and risk modelling.
As fraud is part of regulated operational risk, our model allows us to analytically capture these operational risks without crude benchmarking. This also provides a microeconomic foundation for capital adequacy. In the area of operational risk, these results put internal models that are not risk sensitive or difficult to verify on a solid footing.
A complicated problem, such as online payment fraud detection, requires a comprehensive understanding. A prerequisite for this is access to a large dataset. To evaluate our method, we utilized a real dataset from a private bank. Regardless of the chosen algorithm, feature extraction is an essential part of developing an effective fraud detection method. We utilized historically observed and confirmed fraudulent transaction identifiers as the ground truth. Each feature in the feature vectors for each e-banking session aims to encode deviations from normal customer behavior. Thus, behavioral, transactional, and customer-specific features are important.
Our framework opens interesting directions for future research. Roughly speaking, the framework goes in only one direction, from machine learning methods in fraud detection to statistical risk modelling. The feedback process from the risk model to the triage model and from the triage model back to the fraud detection model is a challenging task that can be addressed utilizing reinforcement-learning methods. With such a feedback loop, the entire risk-management framework becomes a learning system. Another research direction is to extend the optimization of fraud detection (triage model) by considering transaction-dependent loss risks and other features such as customer segmentation. More emphasis is placed on segments that are known or suspected to be less alert or more vulnerable to fraudulent attacks. This resulted in a higher-dimensional triage model.
Availability of data and materials
The bank provided real transaction data and data on transactions (“raw data”) of the customers. The legal basis of the Swiss Federal Data Protection Act (2020) prevents the raw data from leaving the bank in any form or being accessible to any party other than the bank.
CaR for credit risk is VaR on the bank’s quantile level and for market risk CaR was in the past chosen on an annual basis and a risk budgeting process was defined to align present risk with the annual risk budget.
Abbreviations
Swiss Franc
Receiver operating curve
Area under the ROC curve
True positive rate
False poistive rate
Bagged decision tree
Local outlier factor
Isolation forest
Value-at-risk
Generalized pareto distribution
Payment channel for corporate banking clients
Extract, transform, load is a three-phase process where data is extracted, transformed and loaded into an output data container
Abdallah A, Maarof MA, Zainal A (2016) Fraud detection system: a survey. J Netw Comput Appl 68:90–113
Article Google Scholar
Ali A, Shukor AR, Siti HO, Abdu S (2022) Financial fraud detection based on machine learning: a systematic literature review. Review Appl Sci 12:9637
Amiri M, Hekmat S (2021) Banking fraud: a customer-side overview of categories and frameworks of detection and prevention. J Appl Intell Syst Inf Sci 2(2):58–68
Google Scholar
Aggarwal CC, Sathe S (2017) Outlier ensembles: an introduction. Springer
Bessis J (2011) Risk management in banking. Wiley, New York
Bolton RJ, Hand DJ (2002) Statistical fraud detection: a review. Stat Sci 17(3):235–249
Bolton RJ, Hand DJ (2001) Unsupervised profiling methods for fraud detection, Credit Scoring and Credit Control VII, pp 235–255
Breunig MM, Kriegel H-P, Ng RT, Sander J (2000) LOF: Identifying density based local outliers. In: Proceedings of the 2000 ACM SIGMOD international conference on management of data, pp 93–104
Carminati M, Caron R, Maggi F, Epifani I, Zanero S (2015) BankSealer: a decision support system for online banking fraud analysis and investigation. Comput Secur 53:175–186
Chandola V, Banerjee A, Kumar V (2009) Anomaly detection: a survey. ACM Comput Surveys 41(3):1–58
Embrechts P, Klüppelberg C, Mikosch T (2013) Modelling extremal events: for insurance and finance (Vol 33). Springer Science & Business Media
FCA (2021) Financial conduct authority handbook. www.handbook.fca.org.uk
Fei Tony L, Kai T, Zhi-Hua Z (2008) Isolation forest. In: 2008 Eighth IEE E International Conference on Data Mining, IEEE, pp. 413-422
Haixiang G, Yijing L, Shang J, Mingyun G, Yuanyue H, Bing G (2017) Learning from class-imbalanced data: review of methods and applications. Expert Syst Appl 73:220–2
Hilal W, Gadsden SA, Yawney J (2021) A review of anomaly detection techniques and applications in financial fraud. Exp Syste Appl 116429.
Hilal W, Gadsden SA, Yawney J (2022) Financial fraud: a review of anomaly detection techniques and recent advances. Expert Syst Appl 193:11
Jung E, Le Tilly M, Gehani A, Ge Y (2019, July) Data mining-based ethereum fraud detection. In: 2019 IEEE international conference on blockchain (Blockchain) (pp 266-273). IEEE
Juniper Research (2020) Online payment fraud: Emerging threats, segment analysis and market forecasts 2020-2024. www.juniperresearch.com
KPMG (2019) Global banking fraud survey, KPMG International
Kou G, Xu Y, Peng Y, Shen F, Chen Y, Chang K, Kou S (2021) Bankruptcy prediction for SMEs using transactional data and two-stage multiobjective feature selection. Decis Support Syst 140:113429
Krawczyk B (2016) Learning from imbalanced data: open challenges and future directions. Prog Artif Intell 5(4):221–232
Li T, Kou G, Peng Y, Philip SY (2021) An integrated cluster detection, optimization, and interpretation approach for financial data. IEEE Trans Cybern 52(12):13848–13861
Liu FT, Ting KM, Zhou ZH (2008) Isolation forest. In: 2008 eighth IEEE international conference on data mining, pp 413-422. IEEE
Liu FT, Ting KM, Zhou ZH (2012) Isolation-based anomaly detection. ACM Trans Knowl Discov Data (TKDD) 6(1):1–39
McNeil AJ, Frey R, Embrechts P (2015) Quantitative risk management: concepts, techniques and tools-revised edition. Princeton University Press, Princeton
Montague DA (2010) Essentials of online payment security and fraud prevention, vol 54. Wiley, New York
Molloy I, Chari S, Finkler U, Wiggerman M, Jonker C, Habeck T, Schaik RV (2016) Graph analytics for real-time scoring of cross-channel transactional fraud. In: International conference on financial cryptography and data security, pp 22–40. Springer, Berlin, Heidelberg
Pang G, Shen C, Cao L, Hengel AVD (2020) Deep learning for anomaly detection: a review. arXiv preprint arXiv:2007.02500
Piotr J, Niall AM, Hand JD, Whitrow C, David J (2008) Off the peg and bespoke classifiers for fraud detection. Comput Stat Data Anal 52:4521–4532
Power M (2013) The apparatus of fraud risk. Account Organ Soc 38(6–7):525–543
Sabu AI, Mare C, Safta IL (2021) A statistical model of fraud risk in financial statements. Case for Romania companies. Risks 9(6):116
Shen H, Kurshan E (2020) Deep Q-network-based adaptive alert threshold selection policy for payment fraud systems in retail banking. arXiv preprint arXiv:2010.11062
Singh A, Ranjan RK, Tiwari A (2022) Credit card fraud detection under extreme imbalanced data: a comparative study of data-level algorithms. J Exper Theor Artif Intell 34(4):571–598
Tokovarov M, Karczmarek P (2022) A probabilistic generalization of isolation forest. Inform Sci 584:433–449
Trozze A, Kamps J, Akartuna EA, Hetzel FJ, Kleinberg B, Davies T, Johnson SD (2022) Cryptocurrencies and future financial crime. Crime Sci 11(1):1–35
Van Liebergen B (2017) Machine learning: a revolution in risk management and compliance? J Financ Trans 45:60–67
Vanini P (2022) Reinforcement Learning in Fraud Detection, Preprint University of Basel
Wei W, Li J, Cao L, Ou Y, Chen J (2013) Effective detection of sophisticated online banking fraud on extremely imbalanced data. World Wide Web 16(4):449–475
West J, Bhattacharya M (2016) Intelligent financial fraud detection: a comprehensive review. Comput Secur 57:47–66
Zhang W, Xie R, Wang Q, Yang Y, Li J (2022a) A novel approach for fraudulent reviewer detection based on weighted topic modelling and nearest neighbors with asymmetric Kullback-Leibler divergence. Decis Support Syst 157:113765
Zhang G, Li Z, Huang J, Wu J, Zhou C, Yang J, Gao J (2022b) efraudcom: An ecommerce fraud detection system via competitive graph neural networks. ACM Trans Inform Syst (TOIS) 40(3):1-29.
Zhou ZH (2012) Ensemble methods: foundations and algorithms. CRC Press, Boca Raton
Book Google Scholar
Download references
Acknowledgements
The authors are thankful to P. Senti, B. Zanella, A. Andreoli and R. Brun all from Zurich Cantonal Bank for the discussions and for providing us with the resources to perform his study. The authors are grateful to P. Embrechts (ETH Zurich) for the model discussions.
This research received no specific grant from any funding agency in the public, commercial, or not-for-profit sectors.
Author information
Authors and affiliations.
University of Basel, Basel, Switzerland
Paolo Vanini
Novartis AG, Basel, Switzerland
Sebastiano Rossi
swissQuant Group, Zurich, Switzerland
Ermin Zvizdic
IT Couture, Zurich, Switzerland
Thomas Domenig
You can also search for this author in PubMed Google Scholar
Contributions
Sebastiano Rossi and Ermin Zvizdic designed the fraud detection model and analysed the data. Thomas Domenig and Paolo Vanini designed the triage model. Thomas Domenig designed the risk model and did the calculations for the triage and risk model. Paolo Vanini wrote the manuscript with contributions from all authors. Paolo Vanini was involved in the analysis of the triage and risk model. Ermin Zvizdic led the project in the bank. All authors read and approved the final manuscript.
Corresponding author
Correspondence to Paolo Vanini .
Ethics declarations
Competing interests.
The authors declare that they have no competing interests.
Additional information
Publisher’s note.
Springer Nature remains neutral with regard to jurisdictional claims in published maps and institutional affiliations.
The structure of our ensemble results from several design decisions based on both insights from the field (online banking) and experience in building machine learning models. Our ensemble model was built according to the following guidelines:
Customer-specific models The features used in our approach encode patterns in customers’ session behaviour and transactions. These patterns vary widely from client to client, which is why we chose to use a client-specific model rather than a global model.
Global feature space Although behaviours vary, we have chosen to use the same feature representation for each session/transaction, which allows us to assign weights to specific (model, feature) pairs based on their performance across all clients. This in turn allows for consistent scoring across all clients and information sharing between clients when fraudulent activity occurs. In other words, our approach makes it easy for learners to adjust their weights.
Separation of models based on feature type We have chosen to form separate ensembles, one based on behavioural features and one based on transactional features, rather than concatenating all features into a single vector and forming a single ensemble based on concatenation. This ensures better interpretability and reduces the likelihood of constructing nonsensical feature subspaces during feature bagging.
Modified Bootstrap aggregation (Bagging) To build an ensemble of weak learners, we use a modification of bootstrap aggregation (bagging). Bagging is a meta-algorithm for ensembles that is used to reduce the variance and improve the stability of the prediction as well as to avoid overfitting.
Bagging Pipeline
Observational sampling (bagging): Bagged ensembles for classification generate additional data for training by resampling with replacement from the initial training data to produce multiple sets of the same size of initial training data, one for each base learner. This is done to reduce the prediction variance. For the two outlier detection ensembles, we used variable subsampling (without replacement) to avoid problems associated with repeated data and to mimic random selection of the neighbourhood count hyperparameter (cf. Aggarwal and Sathe 2017 ).
Feature bagging: An important task in outlier detection is to identify the appropriate features on which to base the analysis. However, these features may differ depending on the fraud mechanism. Therefore, instead of pre-selecting features, a more robust approach is to create an ensemble of models that focus on different feature sets and assign different weights to the models that use different features depending on their performance. The procedure is applied to each base learner \(b_j\) as follows:
Randomly select a number \(r_{b_j }\) in range \([ d/10,d-1]\) , where d denotes the feature dimension.
Sample a subspace of features of size \(r_{b_j }\)
Train the base learner \(b_j\) on the sampled subspace.
No Hyperparameter bagging: Due to limited fraud, tuning the hyperparameters via validation may lead to overfitting. For this reason, similar to feature selection, we could instead randomly select a set of different hyperparameters. In our case, however, IF and BDT are not expected to be sensitive to the choice of hyperparameters, and resampling the hyperparameter from LOF would be redundant to the subsampling of the data performed. We therefore set all hyperparameters to reasonable ones found in the literature.
Sharing bagged features and parameters across customers : Subspaces for sampled features and parameters for local outlier factors are shared between all client models and all types of base models (manifested in the respective weak learners). This allows, for example, the introduction of supervision in the aggregation step and increased interpretability of the model, as it is easier to identify features relevant to the detection of certain types of fraud.
Model aggregation: Each base model provides a decision function \(\delta (x)\) for a given observation x . The base model ensemble directly aggregates (majority voting or averaging) the weak learner results based on different subsamples to form a single hypothesis that determines the class membership of an observation. Usually, this aggregation is directly extended after a normalisation step to include models of different types, parameters or feature groups. In our approach, however, this final aggregation is performed based on a monitoring step that uses knowledge of available frauds to assign different weights to each pair (model, feature set). Essentially, these weights quantify how appropriate each model and feature pair is for fraud detection.
We refer to a composite Poisson process whose marginal distribution corresponds to a beta distribution as a beta model or as a GPD model if the marginal distribution corresponds to a generalised Pareto distribution. The mass attack model is a nested compound Poisson process. The outer Poisson process models the mass attack event, while an inner Poisson process models the number of affected transactions. The extent of damage of the individual affected transactions is modelled with a beta distribution.
Online banking:
Beta model: Intensity 35, \(\alpha =0.42, \beta =2.4\) and \(\text {scale}: 71.000.\) By shifting and scaling, explicitly by the transformation \(x\rightarrow \alpha + (\beta -\alpha )x\) , the beta distribution is shifted from [0, 1] to the interval \([\alpha ,\beta ]\) . The parameter \(\alpha\) is called location, and \(\beta -\alpha\) scale.
GPD model: Intensity 3, \(\text {shape}=0.25, \text {location}=60.000, \text {scale}=100.000\) .
Mass Attack model: Intensity 0.1, intensity nested model 1000, Beta model \(\alpha =0.42, \beta =2.4\) and \(\text {scale}: 20.000.\)
Mobile banking:
Beta model: Intensity 45, \(\alpha =0.42, \beta =2.4\) and \(\text {scale}: 20'000.\)
Mass Attack model: Intensity 0.1, intensity nested model 1000, Beta model \(\alpha =0.42, \beta =2.4\) and \(\text {scale}: 20'000.\)
GPD model: \(\text {shape}=0.25, \text {location}=100'000, \text { scale}=300'000\) .
Recovery model:
The recovery model models the percentage recovery in a fraud case. It has the following form:
With a probability \(p_1=65\%\) , a complete recovery is simulated, i.e. no damage remains. This resulted from the fact that in the 159 fraud cases considered, it was actually possible to reduce the loss amount to zero even in \(80\%\) of the cases.
With a probability \(p_2=18\%\) , a recovery of zero is simulated, i.e. the damage corresponds to the full amount of the offence.
With probability \(1-p_1-p_2\) , a recovery between 0 and 1 is simulated. A beta distribution is chosen as the distribution of these partial recoveries.
The beta distribution parameters for the online banking channel were fitted on the fraud cases recorded from 13/03/2013 to 13/03/2018. These are 159 fraud cases, of which both the initial fraud transaction amounts and the effective loss amount, i.e. the residual amount after recovery, were recorded. Of the 159 cases, 152 have a fraud amount between CHF 0 and 60,000, while the remaining 7 fraud amounts range between CHF 100,000 and 300,000. A beta distribution was fitted on the 152 cases with fraud amounts up to 60,000 CHF, whereby the scaling parameter, i.e. the upper limit of the distribution, was defined as a free parameter of the fitting procedure and estimated by it to be 71,000 CHF. Similar procedures apply to the marginal distribution fits of the GPD and mass attack models.
There exists significant statistical uncertainty and variability in the driving forces of the defined models. Putting the intended flexibility of the model structure into practice, we distinguish between ’easily accessible’ parameters, which should be subject to discussion at any time in the context of risk assessments, and ’deeper’ parameters, whose mode of action is less obvious and whose adjustment is subject to the process of model reviews. Roughly speaking, Poisson intensities, which determine the expected frequency of events, as well as upper and lower boundaries of the marginal distributions belong to the former category, while shape parameters for the Beta and GPD marginal distributions belong to the latter.
Rights and permissions
Open Access This article is licensed under a Creative Commons Attribution 4.0 International License, which permits use, sharing, adaptation, distribution and reproduction in any medium or format, as long as you give appropriate credit to the original author(s) and the source, provide a link to the Creative Commons licence, and indicate if changes were made. The images or other third party material in this article are included in the article's Creative Commons licence, unless indicated otherwise in a credit line to the material. If material is not included in the article's Creative Commons licence and your intended use is not permitted by statutory regulation or exceeds the permitted use, you will need to obtain permission directly from the copyright holder. To view a copy of this licence, visit http://creativecommons.org/licenses/by/4.0/ .
Reprints and permissions
About this article
Cite this article.
Vanini, P., Rossi, S., Zvizdic, E. et al. Online payment fraud: from anomaly detection to risk management. Financ Innov 9 , 66 (2023). https://doi.org/10.1186/s40854-023-00470-w
Download citation
Received : 22 March 2022
Accepted : 18 February 2023
Published : 13 March 2023
DOI : https://doi.org/10.1186/s40854-023-00470-w
Share this article
Anyone you share the following link with will be able to read this content:
Sorry, a shareable link is not currently available for this article.
Provided by the Springer Nature SharedIt content-sharing initiative
- Payment fraud risk management
- Anomaly detection
- Ensemble models
- Integration of machine learning and statistical risk modelling
- Economic optimization machine learning outputs
To read this content please select one of the options below:
Please note you do not have access to teaching notes, an analysis of fraud on the internet.
Internet Research
ISSN : 1066-2243
Article publication date: 1 December 1999
This paper examines the issue of fraud on the Internet and discusses three areas with significant potential for misleading and fraudulent practices, namely: securities sales and trading; electronic commerce; and the rapid growth of Internet companies. The first section of the paper discusses securities fraud on the Internet. Activities that violate US securities laws are being conducted through the Internet, and the US Securities and Exchange Commission has been taking steps to suppress these activities. The second section of the paper discusses fraud in electronic commerce. The rapid growth of electronic commerce, and the corresponding desire on the part of consumers to feel secure when engaging in electronic commerce, has prompted various organizations to develop mechanisms to reduce concerns about fraudulent misuse of information. It is questionable, however, whether these mechanisms can actually reduce fraud in electronic commerce. The third section of the paper discusses the potential for fraud arising from the rapid growth of Internet companies, often with little economic substance and lacking traditional management and internal controls. The paper examines the three areas of potential Internet fraud mentioned above and suggest ways in which these abuses may be combated.
Baker, C.R. (1999), "An analysis of fraud on the Internet", Internet Research , Vol. 9 No. 5, pp. 348-360. https://doi.org/10.1108/10662249910297750
Copyright © 1999, MCB UP Limited
Related articles
All feedback is valuable.
Please share your general feedback
Report an issue or find answers to frequently asked questions
Contact Customer Support

IMAGES
VIDEO
COMMENTS
Introduction. Internet fraud is defined as the act of obtaining money through deception using network communication technology or the act of providing fraudulent invitations to potential victims or conducting fraudulent transactions using the internet (Tade and Aliyu, 2011; Whitty, 2015, 2019; Gao, 2021).Internet fraud is also called phishing and is typically performed by sending victims an ...
Introduction. Internet-based fraud targeting older adults is an emerging public health problem and a critical social problem in modern society (Ross et al., 2014; Lichtenberg et al., 2016; Yan et al., 2021).The reported financial losses of people over 55 years old, who are less well educated, more socially isolated and particularly vulnerable to scams, are nearly double those of the youngest ...
The majority of previous research conducted in this area predominantly focus on the persuasive influence of the scam message employed by the fraudster (see Chang and Chong 2010) or the knowledge of scams held by the potential victim (see Harrison et al. 2016a).The purpose of this systematic review is to extend that focus to incorporate variables related to individual psychological differences ...
In this paper, researchers have examined. online fraud cases through web applications and how fraudulent websites affect financial lo ss. Researchers also highlighted. the current situation ...
Stories about scams are a weekly occurrence in the popular media, and scams have become one of the most common crimes globally. One report estimated the financial cost of fraud to the global economy at over $5 trillion per year ( Gee & Button, 2019 ), almost 50% higher than the 2019 U.S. budget (about $3.5 trillion).
The paper explores why victims fall for online scams. It identifies a range of reasons including: the diversity of frauds, small amounts of money sought, authority and legitimacy displayed by scammers, visceral appeals, embarrassing frauds, pressure and coercion, grooming, fraud at a distance and multiple techniques.
Building off interdisciplinary discussions within science and technology studies (STS), this special issue expands research on the underside, illicit, and irregular forms of digital behavior. Our focus is on how scams, fakes, and frauds are embedded in the digital economy. In particular, we look at the institutions shaping online scams, the ...
This systematic review will provide a timely synthesis of the leading psychologically based literature to establish the key theories and empirical research that promise to impact on anti-fraud policies and campaigns. Relevant databases and websites were searched using terms related to psychology and fraud victimisation.
Prior studies on online fraud during the Covid-19 pandemic The advent of the internet and related technologies has led cyber-enabled frauds to become a global issue (Button & Cross, 2017; Pandey ...
The 2022 fraud loss estimate by the FTC represented a 30% increase over 2021. Footnote 1 Global losses related to these scams are currently estimated in the trillions. Footnote 2 Researchers have observed that USA residents are more likely to be the victims of these scams than people from other global regions (Hummer & Byrne, Citation 2023a ...
The advent of the internet and proliferation of its use in the 1990s makes it an attractive medium for communicating the fraud, enabling a worldwide reach. This paper aims to explain how advance ...
In this paper, the experiment data are from police notification data obtained from the Internet, as well as fraud case from media data and case document data from multiple judgment websites. After the screening of fraud-related keywords, a total of 3504 texts are obtained. The data distribution and labeling are displayed in Table 1.
Using data from depth interviews with 15 online fraud victims, 6 focus groups with a further 48 online fraud victims and interviews with 9 professional stakeholders involved in combating this problem. The paper explores why victims fall for online scams. It identifies a range of reasons including: the diversity of frauds, small amounts of money ...
More recently, phishers take advantage of the Coronavirus pandemic (COVID-19) to fool their prey. Many Coronavirus-themed scam messages sent by attackers exploited people's fear of contracting COVID-19 and urgency to look for information related to Coronavirus (e.g., some of these attacks are related to Personal Protective Equipment (PPE) such as facemasks), the WHO stated that COVID-19 has ...
As both the Internet and "fraudsters" become more sophisticated and online studies are conducted more frequently, it will indeed be important for the IRB to have online/computer experts to draw on to help facilitate and enhance the conduct of online research, and have IRB members make appropriate decisions to prevent fraud while protecting ...
Introduction. Internet fraud is defined as the act of obtaining money through deception using network communication technology or the act of providing fraudulent invitations to potential victims or conducting fraudulent transactions using the internet (Tade and Aliyu, 2011; Whitty, 2015, 2019; Gao, 2021).Internet fraud is also called phishing and is typically performed by sending victims an ...
Fraud arises in the financial industry via numerous channels, such as credit cards, e-commerce, phone banking, checks, and online banking. Juniper Research reports that e-commerce, airline ticketing, money transfer, and banking services will cumulatively lose over $ 200 billion due to online payment fraud between 2020 and 2024.The increased sophistication of fraud attempts and the increasing ...
ABSTRACT. The fraud on the Internet became a serious issue in the age of technology. Some areas of the usage of the World. Wide Web have an especially high potential for the implementation of ...
Abstract. This paper examines the issue of fraud on the Internet and discusses three areas with significant potential for misleading and fraudulent practices, namely: securities sales and trading; electronic commerce; and the rapid growth of Internet companies. The first section of the paper discusses securities fraud on the Internet.
In this article, we outline the Reflect, Expect, Analyze, and Label (REAL) Framework, developed for researchers to identify suspected online survey fraud, especially when respondents collect incentives for participation, and make decisions about including or excluding suspicious responses.We first provide a brief background on survey fraud and identify frameworks for addressing fraud as a gap ...
This research paper examines internet fraud, including its causes and types. Internet fraud occurs due to a combination of factors that make up the "fraud triangle": a perceived financial or emotional need motivates fraudsters, while the internet provides both opportunity and means of rationalizing unlawful behavior. From the victim's perspective, fraudsters exploit basic human tendencies like ...
PDF | On Jan 1, 2020, Dr Padmalatha N a published E-Commerce Frauds and the role of fraud Detection Tools in managing the risks associated with the frauds | Find, read and cite all the research ...
This paper highlights problems, i.e., banking industry fraud, unethical activities through the use of secondary data such as literature review, and case studies covering all people involved in ...