

DON'T BEAR WITH THE CHEATERS
Easy anti-cheat is the industry-leading anti-cheat service, countering hacking and cheating in multiplayer pc games through the use of hybrid anti-cheat mechanisms., anti-cheat made easy.
Easy Anti-Cheat™ is the industry-leading anti-cheat service, countering hacking and cheating in multiplayer PC games through the use of hybrid anti-cheat mechanisms.
Pioneering security
Easy Anti-Cheat counters the root cause of cheating with industry-leading prevention techniques. Our approach is constantly evolving, which results in fewer hacking attempts, no false positives, and a healthier, more enjoyable community.
Premium experience
Our mission is to keep games fun. Easy Anti-Cheat is non-invasive and trusted industry-wide. Experience your favourite games the way they were meant to be experienced by complementing your security efforts with established technology and practices.
Expert team
With over a decade of experience in developing anti-malware technology, the team has the industry-leading level of expertise. During the game's entire life cycle, Easy Anti-Cheat helps you keep cheaters at bay.
Easy protected games
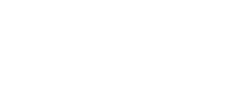
From gamers, for gamers
Being devoted gamers, we strongly believe in the value of clean game experiences. We favor a positive approach in which every player is valued and endless ban lists are not a default.
Believing in the positive
Watch our GDC 2023 video below for further insight in our believes and approach.
Easy is part of epic
With Epic Online Services, we're making it easier and faster for developers to successfully manage and scale high-quality games.
Learn more about Epic Online Services by visiting the link below.
Prevention beats the cure
The traditional mass-penalization approach does not deliver long-term sustainable results and only divides communities. We're strong believers in cheat prevention being the most effective tool to establish a shift in the player's mindset.
With an ongoing focus on prevention, we don't accept anti-cheat solutions should by default be privacy invasive.
License Easy Anti-Cheat
Secure your game with the industry defining anti-cheat solution.
Looking for support?
Browse our Knowledge Base for instant answers to frequently asked questions, or send us your message.
Free AI Detector
Identify AI-generated content, including ChatGPT and Copilot, with Scribbr's free AI detector
Why use Scribbr’s AI Detector
Authority on ai and plagiarism.
Our plagiarism and AI detector tools and helpful content are used by millions of users every month.
Advanced algorithms
Our AI checker is built using advanced algorithms for detecting AI-generated content. It’s also been enhanced to distinguish between human-written, AI-generated, and AI-refined writing.
Unlimited free AI checks
Perform an unlimited number of AI checks for free, with a limit of up to 1,200 words per submission, ensuring all of your work is authentic.
No sign-up required
Start detecting AI-generated content instantly, without having to create an account.
Confidentiality guaranteed
Rest easy knowing your submissions remain private; we do not store or share your data.
Paraphrasing detection
Scribbr’s AI Detector provides insight into whether a piece of writing is fully AI-generated, AI-refined, or completely human-written.
Multilingual support
Our AI Detector supports multiple languages, including German, French, and Spanish. We check and analyze your content at a high level of accuracy across many different languages.
Paragraph level feedback
Our AI Detector identifies specific areas in your text that are likely AI-generated or AI-refined, to provide a detailed analysis of the content.
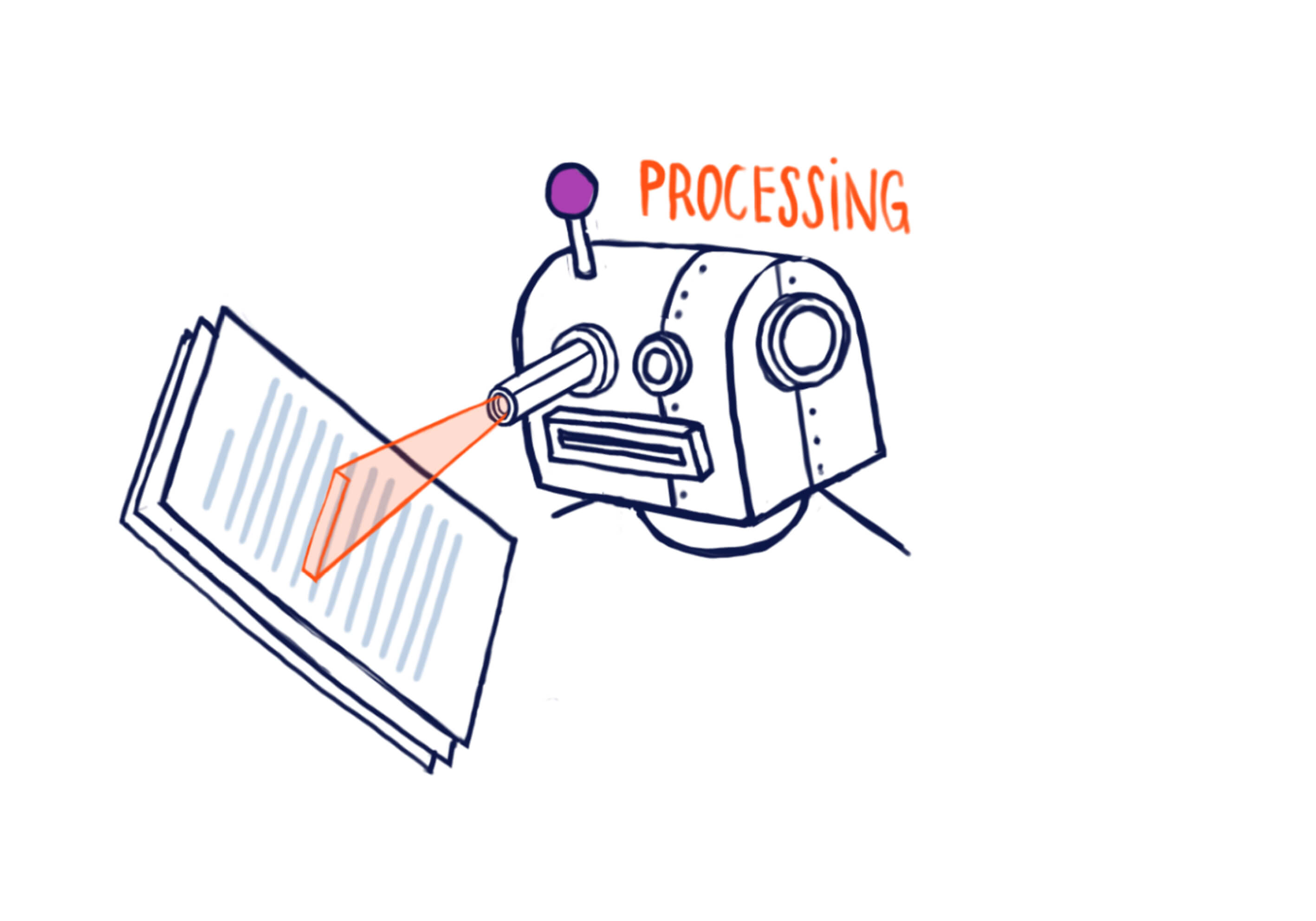
AI Detector for ChatGPT, Copilot, Gemini, and more
Scribbr’s AI Detector accurately detects texts generated by the most popular tools, like ChatGPT, Gemini, and Copilot. It also offers advanced features, such as differentiation between human-written, AI-generated, and AI-refined content and paragraph-level feedback for more detailed analysis of your writing.
Our advanced AI checker tool can detect the latest models, like GPT4 with high accuracy. Note that no AI Detector can provide complete accuracy ( see our research ). As language models continue to develop, detection tools will always have to race to keep up with them.
Our AI Detector is perfect for..
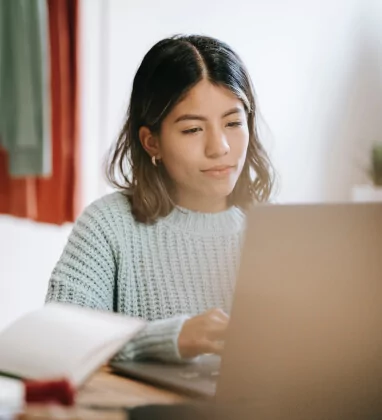
Confidently submit your papers
Scribbr’s AI Detector helps ensure that your essays and papers adhere to your university guidelines.
- Verify the authenticity of your sources, ensuring that you only present trustworthy information.
- Identify any AI-generated content, like that created by ChatGPT, that might need proper attribution.
- Analyze content in English, German, French, and Spanish.
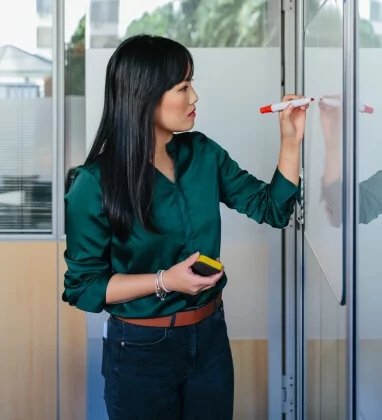
Check the authenticity of your students’ work
More and more students are using AI tools like ChatGPT in their writing process. Our AI checker helps educators detect AI-generated, AI-refined, and human-written content in text.
- Analyze the content submitted by your students to ensure that their work is actually written by them.
- Promote a culture of honesty and originality among your students.
- Receive feedback at the paragraph level for more detailed analysis.
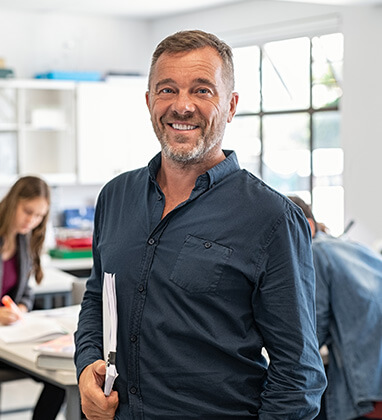
Prevent search algorithm penalties
Using our AI content detector ensures that your content will be indexed by assisting you to publish high-quality and original content.
- Analyze the authenticity of articles written by external contributors or agencies before publishing them.
- Deliver unique content that engages your audience and drives traffic to your website.
- Differentiate between human-written, AI-generated, and AI-refined content for detailed analysis.
AI Detectors vs. Plagiarism Checkers
AI detectors and plagiarism checkers are both used to verify the originality and authenticity of a text, but they differ in terms of how they work and what they’re looking for.
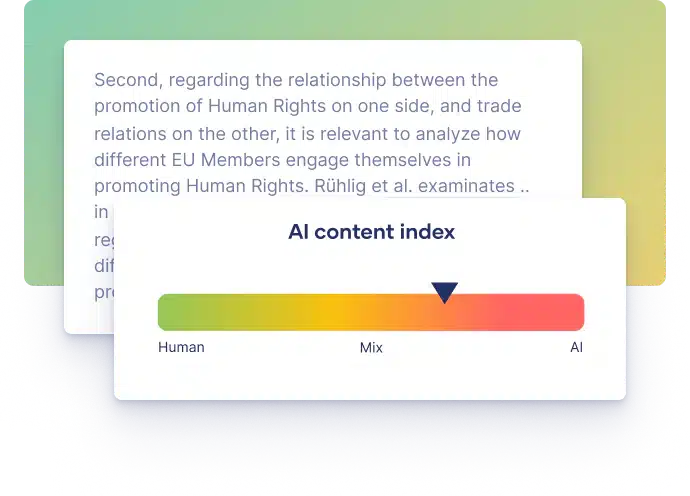
AI Detector or ChatGPT Detector
AI detectors try to find text that looks like it was generated by an AI writing tool, like ChatGPT. They do this by measuring specific characteristics of the text like sentence structure and length, word choice, and predictability — not by comparing it to a database of content.
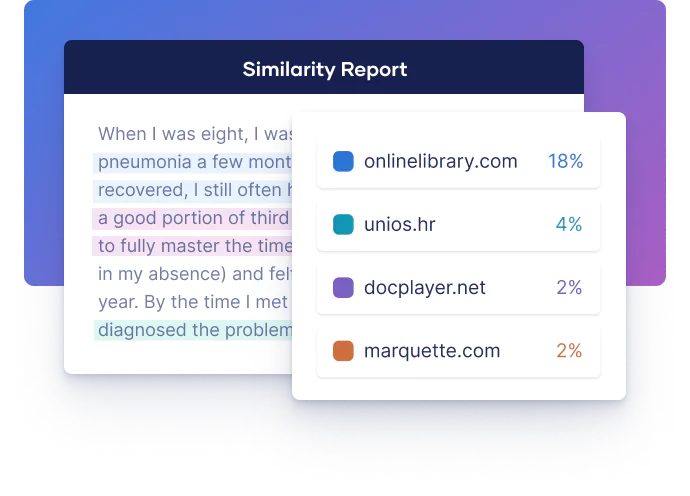
Plagiarism Checker
Plagiarism checkers try to find text that is copied from a different source. They do this by comparing the text to a large database of web pages, news articles, journals, and so on, and detecting similarities — not by measuring specific characteristics of the text.
Scribbr & academic integrity
Scribbr is committed to protecting academic integrity. Our tools, like the AI Detector, Plagiarism Checker , and Citation Generator are designed to help students produce quality academic papers and prevent academic misconduct.
We make every effort to prevent our software from being used for fraudulent or manipulative purposes.
Your questions, answered
Scribbr’s AI Detector can confidently detect most English texts generated by popular tools like ChatGPT, Gemini, and Copilot.
Our free AI Detector can detect texts written using GPT2, GPT3, and GPT3.5 with average accuracy, while our Premium AI Detector has high accuracy and the ability to detect GPT4.
Our AI Detector is carefully trained to detect most texts generated by popular tools like ChatGPT and Bard. These texts often contain certain phrases, patterns, or awkward wording that indicate they were not created by a human. However, no AI model on the market can guarantee 100% accuracy, including ours. To get the best results, we recommend scanning longer pieces of text rather than individual sentences or paragraphs.
Our research into the best AI detectors indicates that no tool can provide complete accuracy; the highest accuracy we found was 84% in a premium tool or 68% in the best free tool.
The AI score is a percentage between 0% and 100%, indicating how much of the text contains content likely written or refined using AI tools.
No—Scribbr’s AI Detector will only give you a percentage between 0% and 100% that indicates the likelihood that your text contains contains AI-generated, AI-refined, or human-written content.
No—our AI content checker can only identify AI-generated, AI-refined, and human-written content. Our Plagiarism Checker can help prevent unintentional plagiarism in your writing.
Yes—our AI Detector can currently analyze text in English, Spanish, German, and French
Detect ChatGPT3.5, GPT4 and Gemini in seconds
Get in touch with questions.
We answer your questions quickly and personally from 9:00 to 23:00 CET
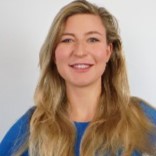
- Start live chat
- Email [email protected]
- Call +1 (510) 822-8066
- WhatsApp +31 20 261 6040
Learn how to use AI tools responsibly
How to cite chatgpt, how to write a paper with chatgpt, how do ai detectors work, university policies on ai writing tools.
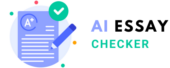
AI Essay Checker
Level up your writing with our free AI Essay Checker 🎯 Refine grammar, detect AI content, and ensure originality 📊
Max 1200 Words is allowed. Visit → RewriterPro AI Checker for higher limits.
Probability Score
Plagiarized
Join thousands of users who have used essay check to refine their writing, remove plagiarism, and eliminate ai content with 100% accuracy..

High School Teacher
Upholding Academic Integrity: The Essential Role of Essay Check for English Teachers
“ Being an English teacher, maintaining academic integrity is paramount. Essay Check has become an indispensable tool in my classroom. It not only aids in detecting plagiarism but also identifies any AI-generated content seamlessly. With its user-friendly interface, Essay Check empowers me to uphold academic honesty effortlessly ”

Content Creator
Content Creation Revolution
“Essay Check transforms my online content creation, ensuring authenticity and originality in scripts and blog posts. It eliminates AI-generated content, empowering me to confidently engage my audience!”
SEO Specialist
Such a life-changing experience. Highly recommended!
“In the competitive world of SEO, Essay Check has truly become my trusted ally. By meticulously ensuring that my content is devoid of any plagiarism or AI-generated elements, it not only maintains but elevates the quality and integrity of my work. This invaluable tool has enabled me to consistently deliver top-notch content, leading to tangible improvements in my clients’ website rankings. With Essay Check by my side, I feel confident in my ability to navigate the competitive landscape of SEO and drive lasting success for my clients.!”

College Student
An overall wonderful and excellent experience
“”Essay Check makes my essays better and original. It catches mistakes check AI content and stops copying so I get good grades.”!”
Patrick Abrams
Transformative Content Creation with Essay Check
“ Essay Check has revolutionized the way I create content for my online platforms. As a content creator, authenticity is everything. This incredible tool not only helps me maintain originality in my scripts and blog posts but also ensures there’s no trace of AI-generated content. With Essay Check, I can confidently produce content that resonates with my audience! ”
Get Rid of Spelling Errors
Once you paste your text into Essay Check, it will identify potential spelling errors and offer suggestions. If our tool flags a word that’s spelled correctly, just select the “Ignore All” option!
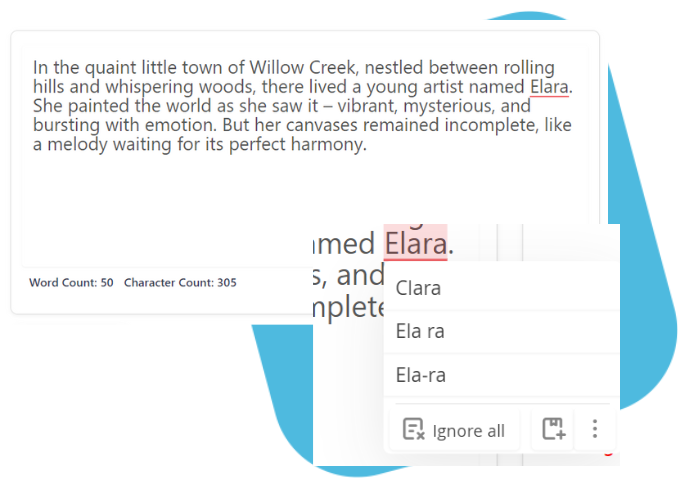
When I first looked at whether it's possible to fight back against AI-generated plagiarism, and how that might work, it was January 2023, just a few months into the world's exploding awareness of generative AI . More than a year later, it feels like we've been exploring generative AI for years, but we've only looked at the issue for about 18 months.
In any case, this is an updated version of that original January 2023 article. When I first tested GPT detectors, I used three: the GPT-2 Output Detector (this is a different URL than we published before), Writer.com AI Content Detector , and Content at Scale AI Content Detection .
Also: How to use ChatGPT: Everything you need to know
The best result was 66% correct, from the GPT-2 Output Detector. I did another test in October 2023 and added three more: GPTZero , ZeroGPT (yes, they're different), and Writefull's GPT Detector . Now, in the summer of 2024, I'm adding QuillBot and a commercial service, Originality.ai , to the mix.
In October 2023, I removed the Writer.com AI Content Detector from our test suite because it failed back in January 2023, it failed again in October, and it failed now. See below for a comment from the company, which their team sent me after the original article was published in January.
Before I go on, though, we should discuss plagiarism and how it relates to our problem. Merriam-Webster defines " plagiarize " as "to steal and pass off (the ideas or words of another) as one's own; use (another's production) without crediting the source."
This definition fits AI-created content well. While someone using an AI tool like Notion AI or ChatGPT isn't stealing content, if that person doesn't credit the words as coming from an AI and claims them as their own, it still meets the dictionary definition of plagiarism.
Also: The best AI image generators to try
In this experimental article, I've asked ChatGPT to help out. My words are in normal and bold text. The AI's words are italicized. After each AI-generated section, I'll show the results of the detectors. At the end of the article, we'll look at how well the detectors performed overall.
Here are the test results for the above text, which I wrote myself:
- GPT-2 Output Detector: 99.98% real
- Content at Scale AI Content Detection: 100% highly likely to be human!
- GPTZero: Your text is likely to be written entirely by a human
- ZeroGPT: 22% AI GPT Your Text is Most Likely Human written
- Writefull GPT Detector: 1% likely this comes from GPT-3, GPT-4 or ChatGPT
- Originality.ai: 84% Original 16% AI
- QuillBot: 45% of text is likely AI-generated
Human-written content: 5-of-7 correct
Before we move on, let's discuss what I'll consider a "correct" analysis. If the score is numeric, anything above 80% will be counted as a correct analysis, and that's being generous. If the score has a summary, I'll look at the summary and make a judgment.
With that, let's dig in some more.
Explain why ChatGPT can be a real problem for teachers and editors when it comes to plagiarism
ChatGPT is a variant of the GPT (Generative Pre-trained Transformer) language model developed by OpenAI. It is designed to generate human-like text by predicting the next word or phrase in a given input sequence. While ChatGPT can be a useful tool for generating text and responding to prompts, it can also be a problem for teachers and editors when it comes to plagiarism.
Also: 4 ways to overcome your biggest worries about generative AI
One of the main reasons why ChatGPT can be a problem for teachers and editors is that it can generate text that is very similar to that of real human writers. This means that students or other individuals could potentially use ChatGPT to generate text that they could then submit as their own work, thereby committing plagiarism.
For teachers and editors, detecting whether a given piece of text was generated by ChatGPT or written by a human can be very difficult, as the generated text may not contain any obvious errors or discrepancies that would reveal its artificial origin. This can make it difficult for teachers and editors to identify and prevent plagiarism, as they may not be able to tell whether a given piece of text is original or copied from another source.
Overall, ChatGPT and other similar language models can be a problem for teachers and editors when it comes to plagiarism because they can generate text that is difficult to distinguish from that written by humans, making it easier for students and other individuals to commit plagiarism without being detected.
- GPT-2 Output Detector: 99.69% real
- Content at Scale AI Content Detection: Hard to tell
- GPTZero: 100% AI
- ZeroGPT: 100% AI
- Writefull GPT Detector: 12% likely this comes from GPT-3, GPT-4 or ChatGPT
- Originality.ai: 100% AI
- QuillBot: 83% of text is likely AI-generated
AI-written content: 4-of-7 correct
Remember, text shown in italics was generated by the AI.
Show me at least three ways that I can detect ChatGPT was used to produce a piece of text
There are several ways that you can potentially detect whether ChatGPT or another language model was used to produce a piece of text. Here are three potential approaches you could use:
- Look for repetitive or unusual patterns in the text: One way to detect whether a language model was used to generate a piece of text is to look for repetitive or unusual patterns in the writing. For example, language models may repeat certain phrases or word combinations, or use unusual or inconsistent sentence structures.
- Check for lack of originality: Another way to detect whether a language model was used to generate a piece of text is to check for a lack of originality in the writing. If a piece of text appears to be copied from another source, or if it contains information that is widely available on the internet or in other sources, it could be a sign that it was generated by a language model.
- Use a plagiarism checker: Finally, you can use a plagiarism checker to check whether a piece of text appears to be copied from another source. While a plagiarism checker may not be able to definitively determine whether a language model was used to generate a piece of text, it can help you identify instances of copying or paraphrasing from other sources.
- Note when the answer is obviously wrong: I talked about this in my Notion AI review , where I spotlighted some text the AI wrote with seeming authority, but which was blatantly wrong. If a student or writer doesn't have full command of the topic being discussed, they may not spot the inaccuracies and leave them in the AI-generated response. Of course, incorrect statements aren't just the purview of an AI writing tool. Many bloggers and commenters, for example, repeat statements made by other bloggers, creating an echo chamber of completely "false facts", showing that while intelligence may be artificial, stupidity is a universal trait.
Keep in mind that it can be difficult to definitively determine whether a language model was used to generate a piece of text, and these methods may not always be effective. However, they can be useful tools to help you identify potential instances of language model-generated text.
In this set of tests, only the italicized content above was run through the checker suite.
- GPT-2 Output Detector: 99.98% fake
- Content at Scale AI Content Detection: Passes as human
- GPTZero: 100% AI
- ZeroGPT: 99.69% AI GPT Your Text is AI/GPT Generated
- Writefull GPT Detector: 79% likely this comes from GPT-3, GPT-4 or ChatGPT
- QuillBot: 100% of text is likely AI-generated
AI-written content: 5-of-7 correct
List three plagiarism checkers that can detect ChatGPT text
It is worth noting that there is currently no plagiarism checker that is specifically designed to detect text generated by ChatGPT or other language models. While some plagiarism checkers may be able to identify certain characteristics of language model-generated text that could indicate its artificial origin, there is no guarantee that they will be able to definitively determine whether a piece of text was generated by a language model.
With that said, here are three plagiarism checkers that you might consider using to help identify potential instances of language model-generated text:
- Turnitin: Turnitin is a popular plagiarism checker that is widely used by schools and universities. It compares a piece of text to a database of other texts to identify instances of copying or paraphrasing. While it may not be able to definitively determine whether a piece of text was generated by a language model, it could potentially identify certain characteristics of language model-generated text that could indicate its artificial origin.
- PlagScan: PlagScan is another plagiarism checker that compares a piece of text to a database of other texts to identify instances of copying or paraphrasing. Like Turnitin, it may be able to identify certain characteristics of language model-generated text that could indicate its artificial origin, but it is not specifically designed to detect language model-generated text.
- Copyleaks: Copyleaks is a plagiarism checker that uses advanced machine learning algorithms to scan a piece of text and compare it to a database of other texts to identify instances of copying or paraphrasing. While it may be able to identify certain characteristics of language model-generated text, it is not specifically designed to detect language model-generated text.
It is worth noting that no plagiarism checker is completely foolproof, and there is always the possibility that a piece of language model-generated text could escape detection. Therefore, it is important to use plagiarism checkers as just one part of a larger strategy to detect and prevent plagiarism.
- GPT-2 Output Detector: 99.58% real
- ZeroGPT: 100% AI GPT
- Writefull GPT Detector: 86% likely this comes from GPT-3, GPT-4 or ChatGPT
Online AI plagiarism checkers
Most plagiarism detectors are used to compare writing against a corpus of other writing. For example, when a student turns in an essay, a product like Turnitin scans the submitted essay against a huge library of essays in its database, and other documents and text on the internet to determine if the submitted essay contains already-written content.
However, the AI-writing tools generate original content, at least in theory. Yes, they build their content from whatever they've been trained on, but the words they construct are somewhat unique for each composition.
Also: OpenAI pulls its own AI detection tool because it was performing so poorly
As such, the plagiarism checkers mentioned above probably won't work because the AI-generated content probably didn't exist in, say, another student's paper.
In this article, we're just looking at GPT detectors. But plagiarism is a big problem, and as we've seen, some choose to define plagiarism as something you claim as yours that you didn't write, while others choose to define plagiarism as something written by someone else that you claim is yours.
That distinction was never a problem until now. Now that we have non-human writers, the plagiarism distinction is more nuanced. It's up to every teacher, school, editor, and institution to decide exactly where that line is drawn.
- GPT-2 Output Detector: 99.56% real
- GPTZero: 98% human
- ZeroGPT: 16.82 AI Your text is human written
- Writefull GPT Detector: 7% likely this comes from GPT-3, GPT-4 or ChatGPT
- Originality.ai: 84% Original 16% AI
- QuillBot: 0% of text is likely AI-generated
Human-written content: 7-of-7 correct
Overall results
Overall, test results this time are dramatically better than they have been with previous tests.
In our previous runs, none of the tests got everything right. This time, three of the seven services tested got the results correct 100% of the time.
Test | Overall | Human | Al | Al | Al | Human |
GPT-2 Output Detector | 60% | Correct | Fail | Correct | Fail | Correct |
Content at Scale Al Content Detection | 40% | Correct | Fail | Fail | Fail | Correct |
GPTZero | 100% | Correct | Correct | Correct | Correct | Correct |
ZeroGPT | 100% | Correct | Correct | Correct | Correct | Correct |
Writefull GPT Detector | 80% | Correct | Fail | Correct | Correct | Correct |
Originality.ai | 100% | Correct | Correct | Correct | Correct | Correct |
QuillBot | 80% | Fail | Correct | Correct | Correct | Correct |
While the overall results have improved dramatically, I would not be comfortable relying solely on these tools to validate a student's content. As has been shown, writing from non-native speakers often gets rated as generated by an AI , and even though my hand-crafted content has no longer been rated as AI, there were a few paragraphs flagged by the testers as possibly being AI-based. So, I would advocate caution before relying on the results of any (or all) of these tools.
Also: Could someone falsely accuse you of using AI-generated text? This could be why
Let's look at the individual testers and see how each performed.
GPT-2 Output Detector (Accuracy 60%)
This first tool was built using a machine-learning hub managed by New York-based AI company Hugging Face . While the company has received $40 million in funding to develop its natural language library, the GPT-2 detector appears to be a user-created tool using the Hugging Face Transformers library. Of the six tests I ran, it was accurate for four of them.
Writer.com AI Content Detector (Accuracy N/A)
Writer.com is a service that generates AI writing, oriented towards corporate teams. Its AI Content Detector tool can scan for generated content. Unfortunately, I found this tool unreliable, and it failed to generate results -- exactly the same way it did in January 2023.
After this article was originally published in January, the folks at Writer.com reached out to ZDNET. CEO May Habib had this comment to share:
Demand for the AI detector has skyrocketed. Traffic has grown 2-3x per week since we launched it a couple months ago. We've now got the necessary scaling behind it to make sure it doesn't go down, and our goal is to keep it free - and up to date to catch the latest models' outputs, including ours. If AI output is going to be used verbatim, it absolutely should be attributed.
Content at Scale AI Content Detection (Accuracy 40%)
The third tool I found was also produced by an AI content generation firm. Content at Scale pitches itself as "We Help SEO-Focused Content Marketers by Automating Content Creation." Its marketing call to action is, "Upload a list of keywords and get 2,600+ word blog posts that bypass AI content detection -- all with no human intervention!" Disturbingly, the results got worse from January -- back then, it was 50% accurate. It has not improved since.
GPTZero (Accuracy 100%)
It's not entirely clear what drives GPTZero . The company is hiring engineers and sales folks, and it runs on AWS, so there are expenses and sales involved. However, all I could find about a service offering was a place where you could register for a free account to scan more than the 5,000 words offered without login. If you're interested in this service for GPT detection, you'll have to see if they'll respond to you with more details. Accuracy has increased since the last time I ran these tests.
ZeroGPT (Accuracy 100%)
ZeroGPT seems to have matured as a service since we last looked at it. When we last looked, no company name was listed, and the site was peppered with Google ads with no apparent strategy for monetization. The service worked fairly well but seemed sketchy as heck.
That sketchy-as-heck feeling is now gone. ZeroGPT presents as any other SaaS service, complete with pricing, company name, contact information, and all the rest. It still performs quite well, so perhaps the developers decided to turn their working code into more of a working business. Accuracy increased as well. Good for them.
Writefull GPT Detector (Accuracy 80%)
Writefull sells writing support services, as well as a free taste of its tools. The GPT detector is fairly new and worked fairly well. Although not fully accurate, it did improve from 60% accurate to 80% accurate with my tests.
Originality.ai (Accuracy 100%, sort of)
Originality.ai is a commercial service that bills itself as both an AI checker and a plagiarism checker. The company sells its services based on usage credits. To give you an idea, all the scans I did for this article used a total of 30 usage credits. The company sells 2,000 credits a month for $12.95 per month. I pumped about 1,400 words through the system and used only 1.5% of the monthly allocation.
Results were great for the AI checker, but they failed 3 out of 5 times when it came to using the service as a plagiarism checker. The following screenshot claims that the text pasted in was 0% plagiarised:
That's wrong since all the text pasted into it was from this article, which has been published online for 18 months. I thought, perhaps, that the plagiarism scanner couldn't read ZDNET content, but that's not the case, as this screenshot shows:
To be fair, I didn't set out to check plagiarism checkers in this article. But since I'm using source material I know I pulled from my existing article, I figured the plagiarism checker would have slammed all of them as 100% plagiarized. In any case, Originality.ai did well on the part we set out to test, the AI checker. They get points for that.
QuillBot (Accuracy 80%-ish)
Nothing is ever easy. The first time I ran my first test through QuillBot , it said that 45% of the text was likely generated by an AI. It wasn't. I wrote it. But then, after completing all the other tests, I returned to QuillBot to grab a screenshot for this section, fed it the same text that generated the 45% score, and, as you can see, it now reports 0% AI.
So, what are we to make of this result? Sadly, I didn't grab a screenshot of the first time I tested this text, but it highlights the concern with relying too much on AI detectors because they are also quite capable of hallucination.
What about OpenAI's own ChatGPT detector?
Well, OpenAI pulled it last year because the detector wasn't particularly accurate. Now, it's supposed to be 99% accurate . That's a little tough to believe because ChatGPT itself is far from 100% accurate.
Also: Will OpenAI's new AI detection tool put an end to student cheating?
But, in any case, as my ZDNET buddy Steven Vaughan-Nichols writes, it's not available yet. We'll give it a spin when it's released for public use. Stay tuned.
Final thoughts
First, I'll let ChatGPT have its say: AI-driven plagiarism is a growing problem, as AI tools like Notion AI and ChatGPT can generate text that is difficult to distinguish from that written by humans. There are several ways to detect whether a piece of text was generated by an AI, such as looking for repetitive or unusual patterns, checking for a lack of originality, or using a plagiarism checker. Plagiarism checkers like Turnitin, PlagScan, and Copyleaks may be able to identify certain characteristics of language model-generated text, but they are not foolproof.
Even the specialty tools I found were woefully unsuited to the task. There was some limited improvement over my tests in January. However, I certainly wouldn't feel comfortable threatening a student's academic standing or accusing them of cheating based on the results of these tools.
Also: Surprise! AI chatbots don't increase student cheating after all, research finds
At this point, I don't think we're ready to let AI fight AI. Instead, we'll have to rely on human perception (which means folks have to pay attention) to identify works generated by an AI.
Don't immediately assume that something is machine-generated. Just because something is stupid, or wildly inaccurate, doesn't mean it was not written by a human. As we all know, humans can write some bad stuff on their own.
Also: Can Perplexity Pro help you code? It aced my programming tests - thanks to GPT-4
So, what do you think? Can you tell if a piece is AI-generated? Have you used these AI writing tools in the months since they suddenly became available? Do you think they're a help or hindrance to education and journalism? Let us know in the comments below. Here's how ChatGPT answered this:
As a language model, I can't see or interact with physical pieces of writing, so I can't tell if a piece is AI-generated or not. However, I can tell you that there are several AI writing tools that are available, and they can be used to generate a wide range of written content, including news articles, fiction, poetry, and more.
I don't have personal experience using these tools since I am just a computer program and don't have the ability to browse the internet or use physical tools. However, I can tell you that some people have found AI writing tools to be helpful in education and journalism, as they can save time and effort by automating certain tasks. Others have expressed concerns about the potential for these tools to be used to produce misleading or biased content or to replace human writers. Ultimately, whether AI writing tools are a help or hindrance depends on how they are used and the context in which they are used.
So, yeah, there's that.
You can follow my day-to-day project updates on social media. Be sure to subscribe to my weekly update newsletter , and follow me on Twitter/X at @DavidGewirtz , on Facebook at Facebook.com/DavidGewirtz , on Instagram at Instagram.com/DavidGewirtz , and on YouTube at YouTube.com/DavidGewirtzTV .
Artificial Intelligence
Wyze is testing a new ai feature that lets you search your video footage by keyword, 1 in 3 workers are using ai multiple times a week - and they're shouting about it, searching through your google photos is about to get a lot easier, thanks to ai.
AI Cheating: Tips to Avoid and Detect
In an era where technology continues to shape the way we learn and assess knowledge, the battle against academic dishonesty has taken on a new form. As students increasingly turn to artificial intelligence for a helping hand, the need to stay one step ahead of AI cheating methods has become more crucial than ever. From exams to assignments and essays, this article unveils the strategies and tools to detect and prevent AI-powered cheating, ensuring a fair and honest educational environment for all.
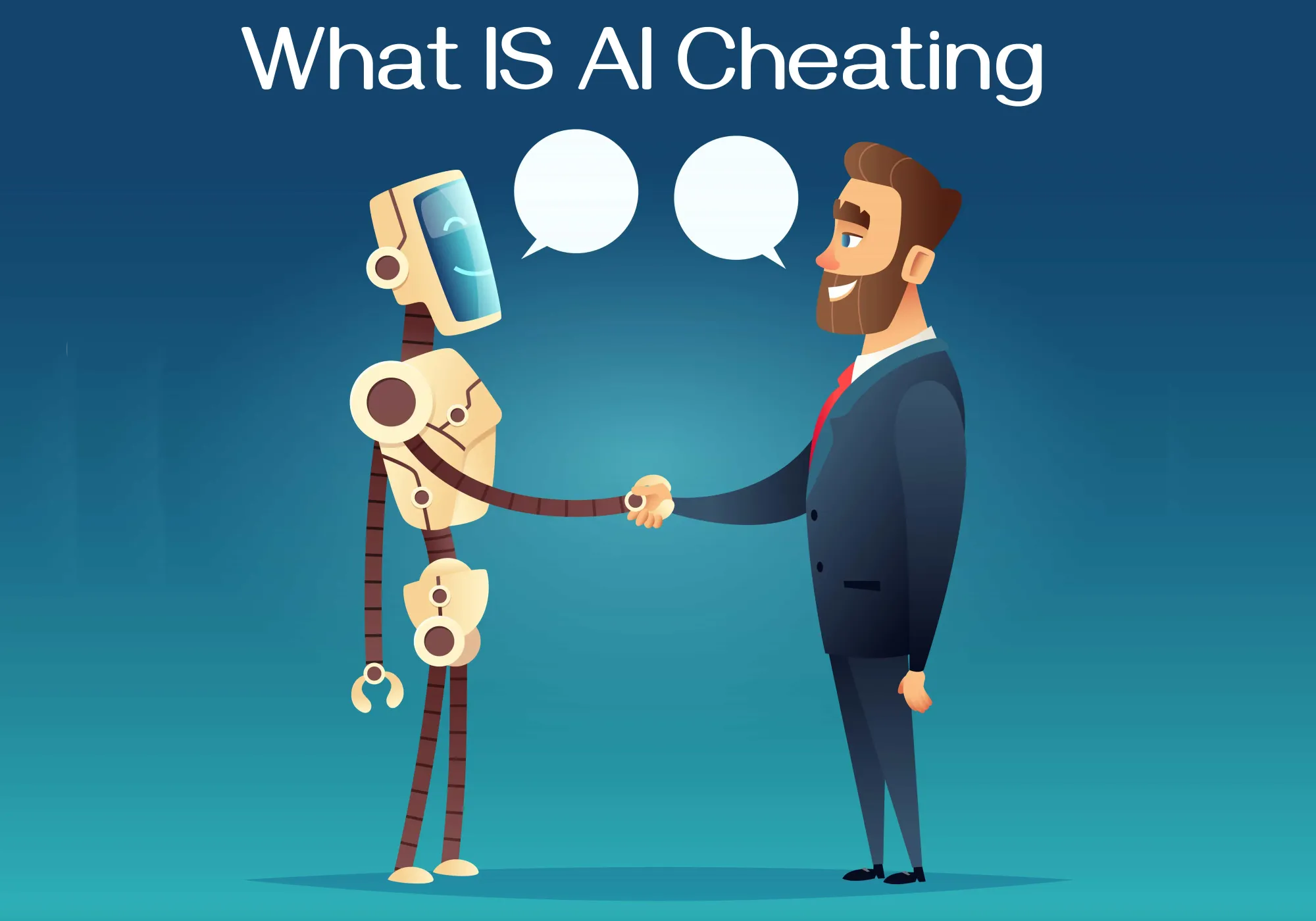
Introduction
As we navigate the digital era, the intersection of education and technology continues to evolve, presenting both opportunities and challenges. One such challenge that has emerged prominently is AI cheating. In this article, we will delve into the complexities of AI cheating, its implications, and strategies for prevention and detection, to ensure the integrity of our academic institutions in the face of this modern challenge.
Brief Overview of AI Cheating
AI cheating involves leveraging sophisticated artificial intelligence tools to unduly gain an advantage in academic settings, undermining the essence of learning and evaluation. It manifests in various forms - from using AI-powered applications to solve complex mathematical problems, employing language models for writing essays or assignments, to even using advanced tools for cheating in online examinations. The ingenuity of these methods makes detection and prevention increasingly difficult.
Scope of the Problem in Academic Settings
The scope of AI cheating is not confined to a specific academic level or field; it's pervasive across high schools, colleges, and universities worldwide. The ease of access to AI tools, coupled with the rapid transition to online learning, has created an environment where AI cheating can thrive if left unchecked. The convenience and anonymity provided by digital platforms have inadvertently fostered a climate where students may be tempted to exploit these advanced tools for dishonest means.
The widespread prevalence of AI cheating not only compromises the integrity of academic evaluations but also undermines the educational process as a whole. It diminishes the value of authentic learning and discourages the development of critical thinking skills, which are vital for academic and professional success.
Addressing this issue requires a comprehensive understanding of its extent and the development of effective strategies tailored to the evolving landscape of AI-empowered cheating. By staying informed and proactive, we can safeguard the sanctity of academic settings in this digital age.
Understanding AI Cheating
What is ai cheating simply.
AI cheating is simply when students use advanced computer programs, also known as artificial intelligence, to dishonestly complete their schoolwork or exams while pretending that the work is their own. In essence, AI cheating refers to the misuse of artificial intelligence technologies or tools to gain an unfair advantage in academic endeavors. This misuse can range from simpler tasks, like using AI-based calculators for complex equations, to more complex applications, such as employing AI-powered language models to generate essays or assignments.
Explanation of How AI Tools Can Be Used for Cheating
Artificial Intelligence, designed to mimic human intellect, can perform tasks usually requiring human intelligence. While this capability opens up numerous beneficial applications, it can also be manipulated for dishonest purposes in educational settings.
AI-powered tools, known as 'essay mills,' can generate well-structured, coherent essays on various topics with minimal human input. Similarly, advanced problem-solving applications can instantly solve complex equations or problems, offering students an unfair advantage. AI-driven language translation tools and 'paraphrasing' tools can present translated or rephrased content as original work, while some AI tools can even manipulate screen sharing or remote proctoring tools during online exams.
Examples of AI Cheating in Exams, Assignments, and Essays
In the context of essay writing, students may use AI tools to generate full essays from a few keywords or a basic outline. These tools can produce content that is well-structured and coherent, potentially bypassing plagiarism detection software.
During exams or while completing assignments , students can use AI problem-solving tools to solve complex equations or problems in subjects like mathematics or physics, gaining an unfair advantage. Using AI translation tools, students can translate text from one language to another and present the translated content as their own work.
Moreover, AI 'paraphrasing' tools can help students rephrase copied content to make it appear unique and bypass plagiarism detection.
In the case of online exams, sophisticated AI tools can offer real-time assistance by solving questions or providing direct answers. Some of these AI tools can manipulate screen sharing or remote proctoring, making cheating hard to detect.
The misuse of these AI tools in various ways underscores the urgent need for comprehensive strategies to tackle AI cheating in academic settings.
The Evolution of AI Cheating
The progression of ai cheating over the years.
AI cheating has not sprung up overnight. Its roots can be traced back to the inception of digital learning tools, but it has evolved significantly over the years, hand in hand with advancements in technology.
AI cheating has grown in tandem with the evolution of technology. In the early days of digital learning tools, instances of cheating were relatively simple. Students might have used basic calculators for tasks meant to be completed manually or turned to the internet to find answers to assignment questions. But as technology advanced, so did the methods of cheating.
Over the years, the emergence of more sophisticated AI tools has allowed the scale and complexity of cheating to escalate. For instance, the development of AI calculators capable of solving complex mathematical equations or AI programs that could generate relatively simple content opened new avenues for academic dishonesty.
B. Recent Advancements in AI That May Contribute to Cheating
In recent years, AI technology has seen exponential growth, becoming more sophisticated and capable. This has inadvertently led to an increase in the potential for AI cheating.
Today, AI can write comprehensive essays, solve intricate problems, and even mimic a student's writing style. AI-powered language models, for instance, can generate complex, high-quality essays on a given topic, making it incredibly difficult for educators to distinguish between a student's original work and AI-generated content.
Moreover, some AI tools have been designed to defeat basic security measures in online examinations. For instance, certain AI-powered applications can provide answers, mimic human-like typing patterns during online tests, and even take the test on behalf of the student, making the cheating process much more covert.
As AI technology continues to evolve, the potential for its misuse in academic settings is a growing concern. It is crucial to understand these risks and develop effective strategies to counteract them.
Major AI Tools Used for Cheating
As we delve deeper into the world of AI-assisted cheating, it's important to understand some of the key tools that students may misuse. Here's an overview of some of the most commonly used AI tools and how they can contribute to academic dishonesty.
AI-Powered Essay Generators
These tools, also known as 'essay mills,' can generate well-structured and coherent essays on various topics based on a few keywords or a simple outline. This allows students to submit high-quality essays without having to do the actual writing.
Examples: EssaySoft, Articoolo, Dr Assignment Auto Writer
Advanced Problem-Solving Applications
AI-powered applications that solve complex equations or problems can give students an unfair advantage during exams or assignments. These tools can handle everything from calculus equations to complex physics problems, providing instant solutions that students are supposed to derive themselves.
Examples: Wolfram Alpha, Microsoft Math Solver, Photomath
AI Translation and Paraphrasing Tools
AI-driven language translation tools can help students translate text from one language to another, and then present the translated content as their own work. Similarly, AI paraphrasing tools can rephrase copied content to make it appear unique, helping students bypass plagiarism detection software.
Examples: Google Translate, Quillbot, SpinnerChief
AI Proctoring Manipulation Tools
In the context of online exams, certain sophisticated AI tools can offer real-time assistance by solving questions or providing direct answers. Some of these AI tools can even manipulate screen sharing or remote proctoring, making it challenging for invigilators to detect any wrongdoing.
AI-Based Writing Style Mimicry Tools
These tools can analyze a student's writing style and then generate content that closely resembles it, making it hard to differentiate between a student's original work and AI-generated content.
AI Screen Sharing and Webcam Manipulation Tools
These AI tools can manipulate screen sharing or webcam feeds during online exams, presenting a pre-recorded feed or altering the shared screen's content in real time.
Advanced Plagiarism Bypassing Tools
AI algorithms can subtly alter copied text by changing words or phrases, making it difficult for plagiarism detection software to identify the original source.
AI-Based Human Behavior Mimicry Tools
Some tools can mimic human behavior during online exams, like typing patterns or mouse movements, making it harder for proctoring software to detect cheating.
Optical Character Recognition (OCR) Tools
OCR tools are designed to recognize text within images or scanned documents, which can then be edited or searched. In the context of academic cheating, students can use OCR tools to extract text from secured PDFs or images, which may not be accessible for copying otherwise.
For instance, a student could use an OCR tool to extract text from a copyrighted textbook or a secured PDF and then use a paraphrasing tool to alter the extracted text, presenting it as their own work. This process could bypass plagiarism detection software, since the original source text may not be easily accessible for comparison.
Examples: Adobe Scan, Google Lens, Tesseract OCR
Understanding these tools and their potential misuse is the first step toward developing effective strategies to combat AI cheating. As AI technology continues to evolve, new tools will undoubtedly emerge, and educators must stay informed to stay ahead of this issue.
V. Impact of AI Cheating
The consequences of AI cheating extend beyond the immediate unfair advantage it provides to individual students. The implications ripple out, impacting academic integrity, educators, and educational institutions as a whole.
A. Undermining Academic Integrity
Academic integrity forms the foundation of any educational institution, fostering an environment that values honesty, trust, fairness, respect, and responsibility. When students resort to AI cheating, they erode these values. The use of AI to generate essays or solve complex problems masks the true academic abilities of students, leading to a lack of trust in the authenticity of their work. Moreover, it fosters a culture where dishonesty is overlooked, which is detrimental to the overall learning environment.
B. Consequences for Students
While AI cheating might offer immediate benefits like higher grades or less study time, it comes with long-term consequences for students. They miss out on the opportunity to learn and develop critical skills that are integral to their academic and professional growth. Over-reliance on AI can also create a dependency that leaves students ill-prepared to handle tasks without AI assistance. Further, if caught, students might face severe penalties including failure in the course, expulsion, or lasting damage to their academic reputation.
C. Consequences for Educators and Institutions
For educators, AI cheating makes it challenging to accurately assess a student's understanding and progress, leading to skewed results that don’t reflect their true capabilities. This can also undermine an educator's efforts to promote and encourage honest academic practices.
For institutions, widespread AI cheating can damage their credibility and the value of the degrees they confer. It can also lead to increased scrutiny from accreditation bodies and potential legal complications in cases of copyright infringement or other violations.
In sum, while AI has tremendous potential to enhance education, its misuse for cheating poses serious challenges that need to be addressed to maintain the sanctity and purpose of education.
VI. Legal and Ethical Implications
The misuse of AI technologies for cheating in academic settings raises significant legal and ethical questions. It's essential to understand and address these implications to ensure a fair and equitable educational landscape.
A. Legal Aspects of AI Cheating
Legally, AI cheating can fall into murky waters. On one hand, the misuse of AI tools can involve copyright infringement, particularly when content from copyrighted sources is copied and rephrased without permission. On the other hand, AI cheating can potentially violate academic integrity policies, leading to disciplinary actions ranging from course failure to expulsion.
Moreover, the use of AI tools to manipulate proctoring software or to create false identities during online exams could potentially lead to legal repercussions under computer fraud and abuse laws. However, the rapidly evolving nature of AI technology often outpaces existing legal frameworks, making it challenging to address these issues adequately.
B. Ethical Considerations
From an ethical standpoint, AI cheating raises concerns about fairness, honesty, and responsibility. Students using AI tools to gain an unfair advantage violate the fundamental principle of equity in education, undermining the efforts of those who work honestly. This dishonesty erodes trust among peers, educators, and institutions.
AI cheating also fosters a culture of dependency and short-termism, where the focus shifts from genuine learning and skill development to immediate results. This approach can be detrimental in the long run, as students may fail to develop critical skills needed for their future careers and personal growth.
Moreover, the misuse of AI tools raises questions about the responsibilities of AI developers and providers. Should they be held accountable for the misuse of their tools? What measures should they take to prevent misuse? These are complex issues that require ongoing dialogue and consideration.
In conclusion, addressing the legal and ethical implications of AI cheating is crucial in maintaining the integrity of educational institutions and ensuring a fair and equitable learning environment.
VIII. Comprehensive Strategies to Prevent AI Cheating
A. honor code.
Establishing an honor code that explicitly forbids the use of AI or any other unauthorized assistance can provide a fundamental ethical guideline for examinations or assignments. This code should clearly define what constitutes cheating and outline the consequences of any violations.
B. Changing Assessment Strategies
Focus on assessment methods where AI assistance would provide little to no benefit. This could include:
- Open-Book Tests: These tests are based on understanding and application rather than memorization. The answers aren't easily accessible by an AI because they require critical thinking and personal interpretation.
- In-Person Proctored Exams: In a supervised environment, it becomes much harder for a student to use AI to cheat. However, this is more difficult to implement in online or distance learning environments.
- Oral Exams: These require real-time interaction between the examiner and the student, making it very difficult for an AI to help.
- Project-Based Assignments: These are long-term tasks that require a significant amount of personal work and creativity, making it hard for AI to effectively provide assistance.
C. Use of Proctoring Software
Proctoring software can monitor a student's activity during an online exam, including keystrokes, mouse movements, and web browsing activities. Advanced proctoring software can also detect the presence of additional software or applications running in the background, like an AI program.
D. Randomize Questions and Answers
Many online learning platforms have the option to randomize the order of questions and answers. This can make it more difficult for AI programs to find and select the correct response.
E. Use Detailed Questions
AI models, as of my knowledge cutoff in 2021, struggle with complex, multi-step problems and questions that require deep understanding or synthesis of information. Therefore, asking detailed, context-heavy questions can make it harder for AI to provide the correct response.
F. Monitor Time Taken
If answers to complex questions are submitted too quickly, this could indicate AI assistance. AIs can generate responses much faster than a human typically would.
G. Plagiarism Detection Software.
These tools can be used to detect if a piece of text produced by a student matches other sources too closely, including AI-generated content.
H. Data Analysis
Unusual patterns in answer submissions, such as many students making the same unusual mistakes or writing in a similar style, could indicate AI use.
These comprehensive strategies can significantly aid in curbing the AI cheating phenomenon in educational settings.
IX. Detecting AI Cheating
A. role of plagiarism detection software.
Plagiarism detection software plays a critical role in identifying AI cheating. These tools can compare a student's work against vast databases of existing content, including AI-generated text. Advanced plagiarism checkers can even identify subtle changes in language or sentence structures, thus helping to flag potential instances of AI-assisted paraphrasing or translation.
B. Importance of Screen Recording and Online Proctoring Tools
Screen recording and online proctoring tools are essential in detecting AI cheating during online exams. By recording a student's screen activity and using advanced algorithms to monitor unusual behavior, these tools can effectively identify potential cheating attempts. For instance, if a student is frequently switching between tabs or applications during an exam, it could indicate the use of AI assistance.
C. Introduction to Stylometry and its Potential for Detecting AI-Generated Text
Stylometry, the study of linguistic style, can be a promising tool in the fight against AI cheating. AI-generated text often has unique linguistic patterns that are discernible from human writing. By analyzing these patterns, stylometry can help identify AI-generated text, even if it has been paraphrased or restructured.
D. Importance of Manual Review and Understanding Student Writing Styles
Despite advancements in technology, manual review remains a crucial tool in detecting AI cheating. Educators who are familiar with their students' writing styles can often detect sudden or unexplained changes in these styles. This approach, however, requires time and effort from educators and is more effective in smaller classrooms where educators can get more familiar with individual students' writing styles. It is important to combine manual review with the other technological tools mentioned for a more effective detection strategy.
IX. The Role of AI in Cheating Prevention and Detection
How ai can be used to prevent and detect cheating.
Ironically, while AI has been a boon for cheaters, it also holds the key to preventing and detecting cheating. With advances in machine learning and data analytics, AI can analyze patterns of behavior and flag suspicious activity. For example, AI can be used in proctoring software to track eye movements, facial expressions, and keyboard strokes to detect anomalies during online exams.
Moreover, AI-based text analysis tools can identify subtle changes in a student's writing style, indicating potential AI-assisted cheating. AI can also be trained to identify the unique patterns in AI-generated text, thus helping to expose students who misuse AI for academic dishonesty.
The Potential Future of AI in Maintaining Academic Integrity
As AI technology continues to evolve, its role in maintaining academic integrity is set to expand. Future AI systems might be able to identify a wider range of cheating tactics, from sophisticated AI-generated essays to the misuse of AI in online exams. AI could also provide personalized learning resources for each student, reducing the need for cheating by addressing individual learning gaps.
X. Role of Educational Institutions
Proactive role of schools, colleges, and universities in curbing ai cheating.
Educational institutions have a crucial role to play in curbing AI cheating. They need to be proactive in educating students about the ethical implications of AI cheating and the potential consequences for academic and professional careers. Regular workshops and awareness campaigns can be organized to address the issue.
Moreover, institutions can invest in advanced cheating detection tools, including AI-based proctoring software and plagiarism checkers, to maintain academic integrity. They should also strive to stay updated with the latest advancements in AI and cheating tactics to ensure their prevention and detection methods remain effective.
Policies and Measures That Educational Institutions Can Implement
Institutions can implement strict policies against AI cheating, with clear consequences for those caught misusing AI. Such policies should be communicated clearly to all students.
Educators can also employ a variety of assessment strategies that make cheating difficult, such as open-book tests, in-person proctored exams, oral exams, and project-based assignments.
Lastly, institutions can collaborate with tech companies to develop more robust methods for detecting AI cheating and ensuring the fair use of technology in education.
XI. Parental and Guardian Involvement
Promoting academic integrity.
Parents and guardians play a pivotal role in shaping a child's character, including their understanding and appreciation of academic integrity. They can instill the values of honesty, hard work, and fairness from an early age. They should discuss the harmful effects of cheating, both on personal development and on academic and professional prospects. Parents can also lead by example, demonstrating ethical behavior in their own actions and decisions.
Keeping a Check on the Misuse of AI Tools
In the digital age, parental oversight extends to the online activities of their children. Parents and guardians can keep a check on the use of AI tools by their children for academic purposes. This includes maintaining open communication about the appropriate use of AI and technology in general.
Moreover, they can monitor their child's study patterns and time spent on assignments or projects. If a task that generally takes several hours is completed in an unusually short time, it could be a sign of AI misuse.
Finally, parents and guardians can make use of parental control software to restrict access to certain AI tools and websites known for facilitating academic dishonesty. They can also encourage the use of AI for positive learning experiences, such as AI-based tutoring programs, thus showing their children the potential benefits of AI when used ethically and responsibly.
XIII. Moving Forward: The Future of AI and Academic Integrity
A. the positive role of ai in education.
While this article has primarily discussed the misuse of AI in academic settings, it's important to highlight the potential benefits of these technologies. From personalized learning programs that adapt to each student's needs to intelligent tutoring systems that provide instant feedback, AI has the potential to revolutionize education. It can facilitate distance learning, diversify teaching methods, and provide accessible education resources to those who may not have had them before. Thus, the goal is not to eliminate AI from education but to ensure its ethical use.
B. Future Developments in AI Cheating and Countermeasures
As AI technology continues to evolve, so too will the methods of AI cheating. AI models will become more sophisticated, and new forms of cheating may emerge. It's therefore crucial for educators, institutions, and software developers to stay ahead of the curve.
This may involve developing more advanced AI proctoring and plagiarism detection tools or leveraging AI itself to detect dishonest practices. For example, machine learning algorithms could be trained to identify AI-generated text or to detect patterns indicative of cheating in student data.
Similarly, assessment strategies may need to evolve. This could involve a greater emphasis on in-person exams, oral exams, or project-based assignments, where AI assistance is less effective.
XIV. Conclusion
In conclusion, tackling AI cheating is crucial in preserving the value of education in this rapidly advancing digital age. While AI offers significant benefits, its misuse in academic dishonesty threatens the integrity of the learning process.
The responsibility of combatting AI cheating is shared by educators, parents, students, and the wider society. Through promoting ethical AI use, creating cheat-resistant assessments, and employing advanced detection methods, we can ensure AI supports, not undermines, the educational journey.
In this era of AI, we must reinforce the importance of human effort and honesty in education. After all, AI can aid the learning process, but it should never replace the quest for knowledge and personal intellectual growth.
AI Cheating FAQs
What is ai cheating.
AI cheating is the use of artificial intelligence technologies to dishonestly gain an advantage in academic settings. It's like having a computer do your homework or take your test for you, and then pretending that you did it yourself.
This could involve using AI tools to complete assignments, generate essays, solve complex problems, or even manipulate online examination systems. AI cheating is a violation of academic integrity as it involves presenting AI-generated work as one's own, and it poses significant challenges to educators and institutions aiming to uphold fair and honest academic practices.
Can students cheat with ChatGPT?
Yes, it's technically possible for students to use ChatGPT to cheat on assignments or exams. ChatGPT can generate detailed responses to prompts, which could include essay questions or other types of assignments. However, using AI tools like ChatGPT in this way is considered a form of academic dishonesty. It's important for students to understand that using AI to complete their work not only undermines their own learning, but it's also likely against their school's code of conduct and could result in serious consequences.
Is the use of AI cheating?
The use of AI itself is not cheating. AI has many legitimate and beneficial uses in education, such as personalized learning, adaptive testing, and helping with accessibility. However, the misuse of AI to dishonestly complete assignments or exams is considered cheating. For example, using an AI tool to write an essay and then submitting it as your own work would be considered academic dishonesty. It's important to use AI responsibly and within the guidelines set by your educational institution.
How schools can stop students cheating with ChatGPT?
Educate Students: Make students aware of what constitutes cheating and the consequences of such actions. This includes using AI tools like ChatGPT to complete assignments.
Promote Academic Integrity : Encourage honest academic practices and create an environment that values original work and critical thinking.
Change Assessment Methods : Design tests and assignments in a way that requires critical thinking and personalized responses, which are harder for AI to generate.
Use Technology : Employ advanced plagiarism detection software and proctoring tools to identify any unusual patterns that might indicate AI use.
Policy and Consequences : Have clear policies regarding AI cheating and ensure they are enforced consistently.
Table of Contents
Try screenapp today, related posts.
You may also be interested in these posts
.webp)
Best Free AI Tools for Students

Top 10 AI-Powered Video Summarizers

Best Free Online AI Voice Generators Review

Plagiarism Checker
A completely free and accurate online plagiarism detector. Simply copy and paste to detect copied content.
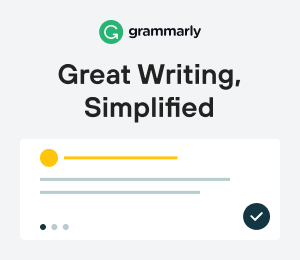
Get your Weekly Plan Only in

The free version does not support more than 1000 words.
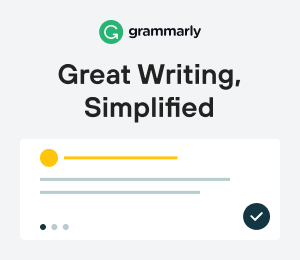
Plagiarism Checker “ PRO ” the most trustworthy plagiarism detection tool
Our mission is to fulfill everyone's NEEDS in plagiarism detection. Most user-friendly interface with plenty of exclusive FEATURES to execute efficiency & reliability mission.
Plagiarism Checker Online for Multiple Languages
Why choose our plagiarism checker.
Our best free plagiarism checker is totally free. It doesn’t charge you anything which means you can conduct as many searches as you like. All you need to do is just copy and paste your content.
This plagiarism checker software offers you a deep and thorough check. With deep search, you get extra levels of plagiarism search and additional result details.
If you don’t want to copy and paste your text, you can alternatively upload the page URL or the file for a quick and free plagiarism check.
Consequences of Plagiarism
Today, copyrighted content has become a constant problem in educational institutions. Students create many assignments using a lot of research in a short period. Many schools/institutions, such as Duke University, have judicial affairs offices. They will review each case of plagiarism and fix penalties.
Most of the outcomes of plagiarism occur once the replication is caught. Institutions have noted that students who duplicate data often fail to learn research and writing skills. Once they leave the institution, all those students lose the ability to create original content because they have only cheated before.
Professional
Countless journalists, academics, writers, and creators have had their reputations damaged by plagiarism accusations. Not only do plagiarists disrupt their ongoing work by getting fired or terminated. They also find it difficult to get work in the future. A particularly egregious instance of plagiarism, or worse, can destroy a person's professional image. It also forces them to change careers to avoid the stigma of plagiarism.
Apart from all the professional and personal difficulties that may arise in certain cases, there are also legal consequences. Copying copyrighted material for profit may result in the plagiarist having to pay some financial losses. In some cases, copying data can subject the plagiarist to criminal prosecution. And the punishment can range from charges to imprisonment.
So, before submitting any content, do a plagiarism check once to see if you have copied it or not. It can protect you from all kinds of harm. You won't have to wait long for results using a duplicate content checker. You can take advantage of this plagiarism checker free and save yourself from embarrassment.
How it detects?
Plagiarism Detector is the free and an intelligent and essay checker software. It doesn't matter if you are a student or a professional, everyone can have benefit from this likewise.
Now, with the help of our plagiarism detector, you can check if your content that you are just seconds away from publishing and considering its uniqueness. If you are web owner, then it is very important to have a plagiarism-free content on your website if you wish to attract maximum traffic. This is the best Free Plagiarism Checker that you will find online as it works the same way as Google.
Built with thorough research on the needs of our customers, all the essential strategies and techniques have been implemented to make results 100% accurate and authentic for everyone.
We analyzed that any anti-plagiarism software is mostly used by students or teacher to check the essays, papers, and reports that they submit or that have been submitted to them. Because plagiarism is unacceptable no matter what type it is . That is why we have particularly designed a system that offers free plagiarism detection which helps students and teachers to check their duplicate content.
Teachers, as well as students, should discourage plagiarism .
You can try our plagiarism detector now, and you will know that it is rightfully the free online plagiarism checker with percentage!
Importance of plagiarism Detector
If you aim at a higher Authority on SERP (Search Engine Results Page) then never take the risk of publishing a heavily duplicated content.
Plagiarized or duplicated content can lead to lower page rank. If you are caught up in a situation where your article, blog or web page content is not entirely unique, then there is a comparatively higher risk of getting blacklisted by search engines especially Google. You can utilize the free plagiarism detection service offered by our similarity checker to check the content from your own website to make sure that no one has stolen the content from your website. Apart from SEO (Search Engine Optimization), our free plagiarism finder can also be utilized by students and teachers for academic uses.
Beware! It is a double-edged sword .
How the plagiarism scanner Works?
It is very simple!
Plagiarism scanner is an intelligent free and most accurate software.
The content you enter is analyzed on the basis of its
- lexical frequencies,,
- word-choice,,
- matching phrases,,
🌐 Multilingual | 150+ Languages |
✔️ Working | Lexical frequencies Contextual Analysis Fuzzy Matching |
💯 Accuracy | 100% |
✈️ Speed | Immediate checking |
🔁 Check Counts | Unlimited |
and many other important factors. Your given text is mapped into our internal network, and then it is compared against different databases and the entire internet. We have designed our algorithm to especially ignore statistically common phrases to provide a better and more valuable search for potential plagiarism in the text. Once the plagiarism test completes, the results will appear below along with the match percentage that our best plagiarism tool has found.
The article you have entered is carefully scanned, and so is the entire internet. If complete plagiarized sentences are found, the plagiarism detector will identify the original source of that content and show to you. All you have to do is copy and paste the content in the text box and then click on “Check Plagiarism” button.
It is as simple as it sounds. (How to check plagiarism)
This plagiarism checker free online for teachers, students, and writers will run your text through its database of millions of sites to show you the best plagiarism free report with the detailed results. Alternatively, you can either enter the URL of the website for which you want to conduct a plagiarism check or you can upload the files. Whether it is an article, essay or blog, a thorough sentence check will be conducted for your text in order to give you the most reliable and accurate results.
Online Plagiarism Checker
Understanding the results.
Beside the text box, you are shown the percentage for Checked, Unique, and Plagiarized.
Phrases or sentences shown in the results below are the ones that our plagiarism detector has identified as plagiarized and already exist online, along with the matched percentage. These are the links and by clicking on the “Match Text” you will be redirected to the original source where you can see yourself the plagiarized text. When the plagiarism test is finished, you’ll also be given an exact percentage of uniqueness and plagiarism of your text. However, if your content is completely unique then you will get a congratulations message:
Plagiarism Checker API
The plagiarism checker API allows everyone to add plagiarism detection functionality to their software or applications. Our flexible system allows you to customize your settings to fit your needs. It can grow with your business or personal needs. Most importantly, our reliable services give you peace of mind knowing your data is safe and protected.
Identify Copied Material with Anti Plagiarism Software
Plagiarism can appear in different forms, but the end outcome is always the same: the plagiarist stole something from another person. Plagiarism refers to using some other person’s ideas and information without acknowledging that specific person as the source. Similar to all other forms of theft, plagiarism also has many disadvantages associated with it.
It can take place in both intentional, when you try to pass someone’s content as your own, or unintentional when you forget to mention the source for your work correctly. In spite of the kind, piracy always has consequences when it is discovered. Mostly, the punishment of accidental copying is temporary. Because ascertaining unintentional plagiarism requires knowledge of intent, the overall prestige of the plagiarist becomes the deciding factor.
Plagiarism can occur in any shape either textual or pictorial. So we not only scan the document for the copyrights but also check the duplication for images. You can also perform Reverse Image Search to look for the best matching photos. By using advance image recognition algorithms, it will bring out the matching results from all over the internet and search engines.
Before jumping to direct consequences, here’s a brief detail of how can you make sure your work is not matching with any source. Loads of information, millions of text documents, is attainable on the internet now. So, if you think you can check manually, then you are wrong. No matter, how many articles you read or how many hours you spend, you can never be sure. Hence, the alternative best way you can take help from is a free plagiarism detector. There are many such tools readily available on the internet, providing best services to users. Majority of them, search your material against billions of contents and underlines each sentence which resembles with another posted article.
GPT Essay Checker for Students
How to Interpret the Result of AI Detection
To use our GPT checker, you won’t need to do any preparation work!
Take the 3 steps:
- Copy and paste the text you want to be analyzed,
- Click the button,
- Follow the prompts to interpret the result.
Our AI detector doesn’t give a definitive answer. It’s only a free beta test that will be improved later. For now, it provides a preliminary conclusion and analyzes the provided text, implementing the color-coding system that you can see above the analysis.
It is you who decides whether the text is written by a human or AI:
- Your text was likely generated by an AI if it is mostly red with some orange words. This means that the word choice of the whole document is nowhere near unique or unpredictable.
- Your text looks unique and human-made if our GPT essay checker adds plenty of orange, green, and blue to the color palette.
- 🔮 The Tool’s Benefits
🤖 Will AI Replace Human Writers?
✅ ai in essay writing.
- 🕵 How do GPT checkers work?
Chat GPT in Essay Writing – the Shortcomings
- The tool doesn’t know anything about what happened after 2021. Novel history is not its strong side. Sometimes it needs to be corrected about earlier events. For instance, request information about Heathrow Terminal 1 . The program will tell you it is functioning, although it has been closed since 2015.
- The reliability of answers is questionable. AI takes information from the web which abounds in fake news, bias, and conspiracy theories.
- References also need to be checked. The links that the tool generates are sometimes incorrect, and sometimes even fake.
- Two AI generated essays on the same topic can be very similar. Although a plagiarism checker will likely consider the texts original, your teacher will easily see the same structure and arguments.
- Chat GPT essay detectors are being actively developed now. Traditional plagiarism checkers are not good at finding texts made by ChatGPT. But this does not mean that an AI-generated piece cannot be detected at all.
🕵 How Do GPT Checkers Work?
An AI-generated text is too predictable. Its creation is based on the word frequency in each particular case.
Thus, its strong side (being life-like) makes it easily discernible for ChatGPT detectors.
Once again, conventional anti-plagiarism essay checkers won’t work there merely because this writing features originality. Meanwhile, it will be too similar to hundreds of other texts covering the same topic.
Here’s an everyday example. Two people give birth to a baby. When kids become adults, they are very much like their parents. But can we tell this particular human is a child of the other two humans? No, if we cannot make a genetic test. This GPT essay checker is a paternity test for written content.
❓ GPT Essay Checker FAQ
Updated: Jul 19th, 2024
- Abstracts written by ChatGPT fool scientists - Nature
- How to... use ChatGPT to boost your writing
- Will ChatGPT Kill the Student Essay? - The Atlantic
- ChatGPT: how to use the AI chatbot taking over the world
- Overview of ChatGPT - Technology Hits - Medium
- Free Essays
- Writing Tools
- Lit. Guides
- Donate a Paper
- Q&A by Experts
- Referencing Guides
- Free Textbooks
- Tongue Twisters
- Editorial Policy
- Job Openings
- Video Contest
- Writing Scholarship
- Discount Codes
- Brand Guidelines
- IvyPanda Shop
- Online Courses
- Terms and Conditions
- Privacy Policy
- Cookies Policy
- Copyright Principles
- DMCA Request
- Service Notice
IvyPanda's free online GPT essay checker is much more effective than traditional plagiarism checkers. Find out if an academic paper was written by a human or a machine. You will also find a detailed guide on how to interpret the analysis results.
AI checker provides insight into writing as the learning process evolves
Turnitin’s AI content checker helps educators, publishers, and researchers identify when an AI writing tool such as ChatGPT or text spinners may have been used in students’ submissions.
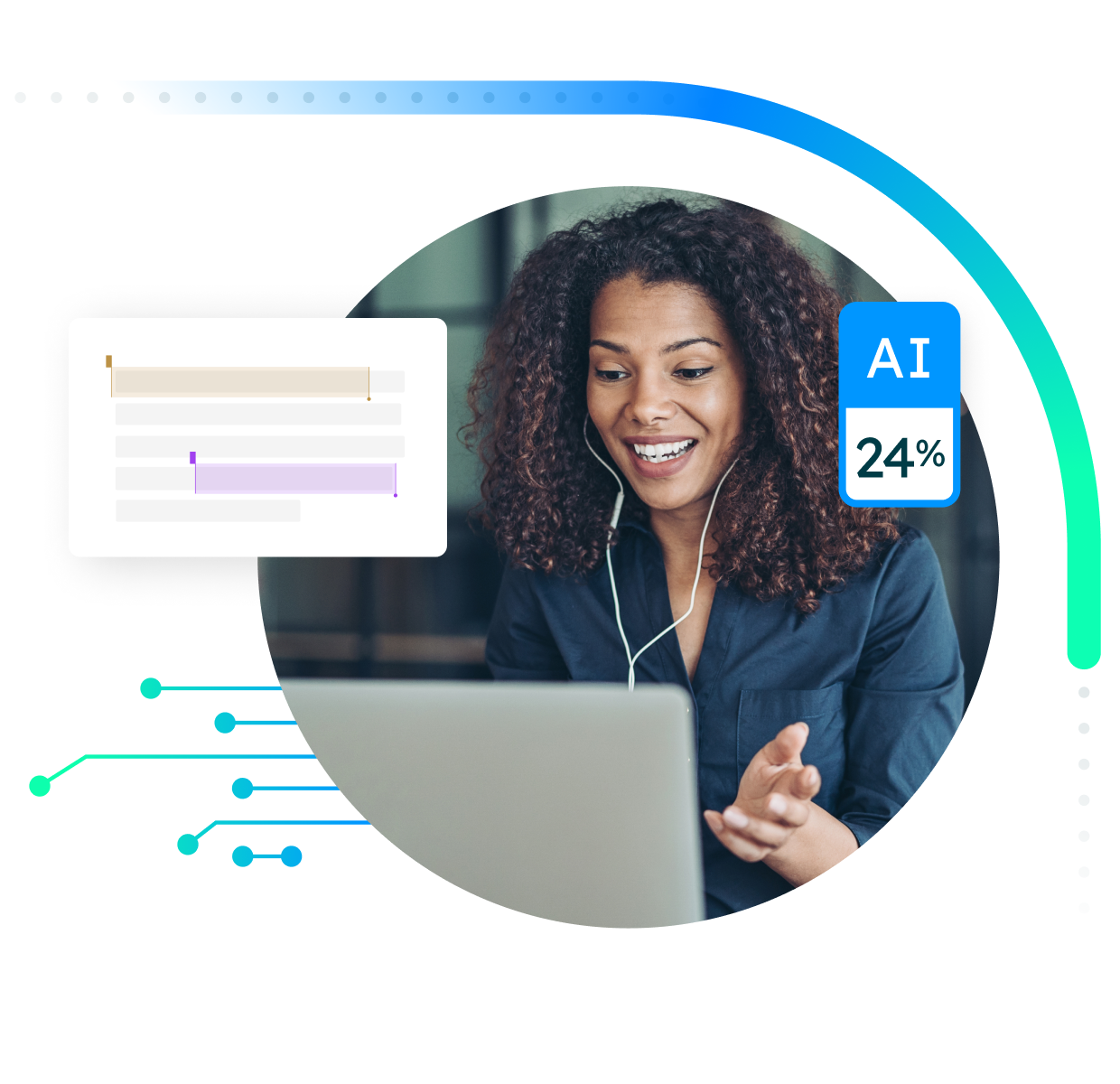
Academic integrity in the age of AI writing
Over the years, technology has supported and tested academic integrity. Today, educators and students face a new frontier with AI-generated text and ChatGPT.
We believe that AI models can be positive forces that, when used responsibly, can support and enhance the learning process. We also believe that equitable access to AI tools is vital. We’re working with educators and students to develop technology that can support and enhance the learning process. However, it’s important to acknowledge new challenges alongside the opportunities.
We recognize that educators need to know when and where students have likely used AI writing tools. That’s why we now offer an AI content detector and more capabilities for educators in our products.
Our AI checker provides valuable insights on how much of a student’s submission is authentic, human-written content versus likely AI-generated from ChatGPT or likely AI-generated and likely AI-paraphrased.
Reporting identifies likely AI-written content or likely AI-paraphrased text and provides information educators need to determine their next course of action. We’ve designed our AI essay checker with educators, for educators.
Our AI essay detector complements our similarity checking workflow and is integrated with your LMS, providing a seamless, familiar experience.
Our AI content detection capability, which is available with Originality, helps educators detect likely AI-generated content in student work while also safeguarding students’ interests.
Turnitin ai innovation lab.
Welcome to the Turnitin AI Innovation Lab, a hub for new and upcoming product developments in the area of AI writing. You can follow our progress on detection initiatives for AI-written content, ChatGPT, and AI paraphrasing.
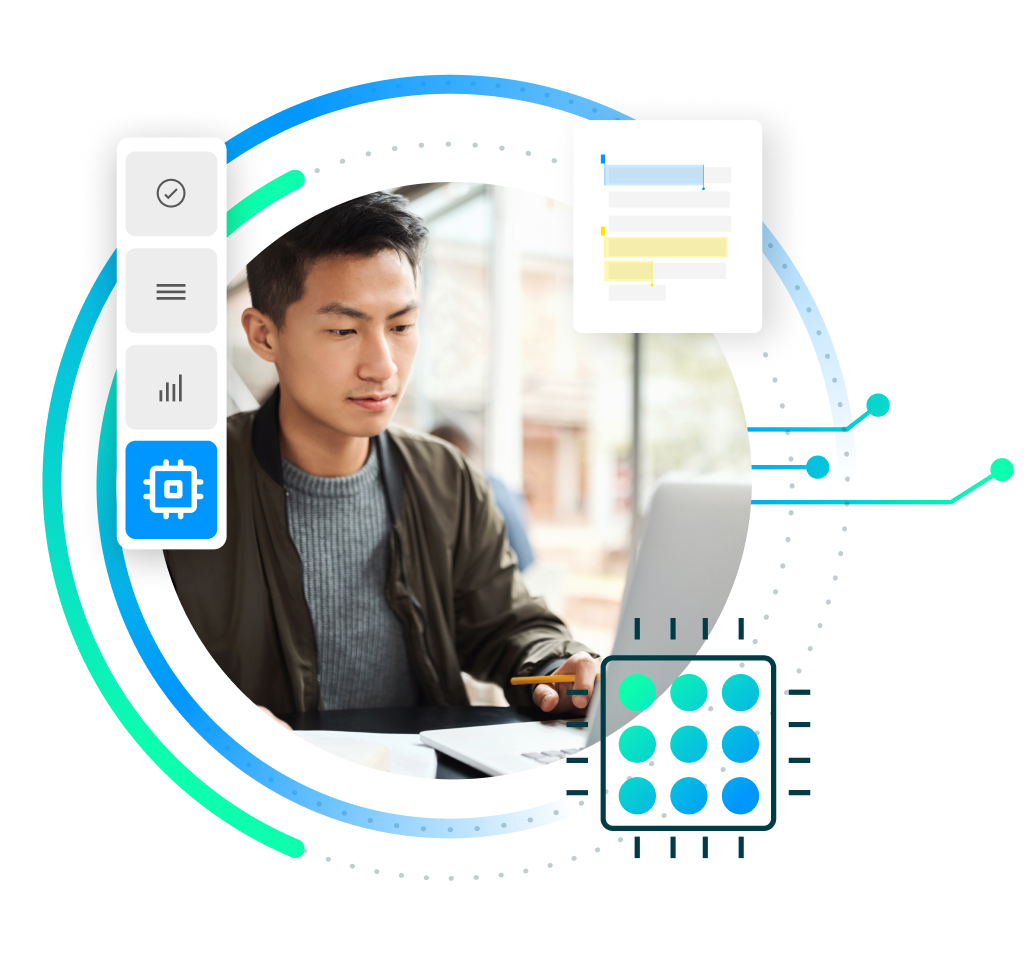
Understanding the false positive rate in our AI sentence detection capabilities
We’d like to share more insight into our false positive rate and tips on using our AI detection tool and data-driven metrics.
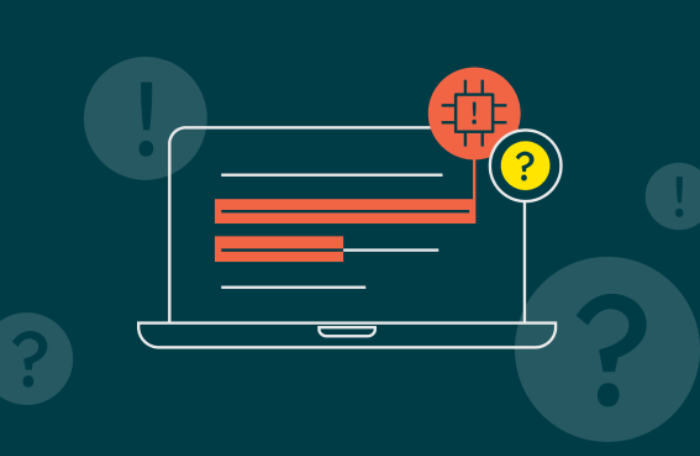
Understanding false positives within our AI checker
We’d like to share some insight into how our AI detection model deals with false positives and what constitutes a false positive.
Have questions? Read these FAQs on our AI writing checker capabilities
Helping solve the ai writing puzzle — one piece at a time.
AI-generated text has transformed every aspect of our lives, including the classroom. However, detecting likely AI-generated content in students’ submissions is just one piece in the broader, complex, ever-evolving AI writing puzzle.
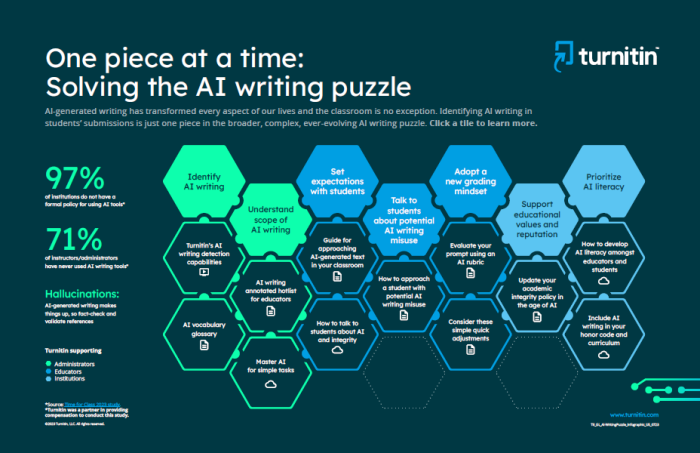
Research corner
Check out the links below if you’re interested in the additional research we performed against English Language Learners and what an independent research study has revealed about Turnitin's AI-writing detection capabilities.
Research shows our AI scanner shows no statistically significant bias against English Language Learners
- In response to customer feedback and papers claiming that AI detector tools are biased against writers whose first language is not English, we expanded our false positive evaluation to include writing samples of English Language Learners (ELL). We tested another nearly 2,000 writing samples of ELL writers.
- We found that in documents meeting the 300-word count requirement, ELL writers received a 0.014 false positive rate, and native English writers received a 0.013.
- This means that there is no statistically significant bias against non-native English speakers.
Independent research shows the “Turnitin [AI writing detector] achieved very high accuracy”
- Two of the 16 detectors, Turnitin and Copyleaks, correctly identified the AI- or human-generated status of all 126 documents, with no incorrect or uncertain responses.
- Three AI text detectors — Turnitin, Originality, and Copyleaks — have very high accuracy with all three sets of documents examined for this study: GPT-3.5 papers, GPT-4 papers, and human-generated papers.
- Of the top three detectors identified in this investigation, Turnitin “achieved very high accuracy” in all five previous evaluations. Copyleaks, included in four earlier analyses, performed well in three of them.
Teaching in the age of AI writing
As AI text generators like ChatGPT quickly evolve, so will educator resources. Curated and created by our team of veteran educators, our resources help educators meet these new challenges. They’re built for professional learning and outline steps educators can take immediately to guide students in maintaining academic integrity when faced with AI writing tools.
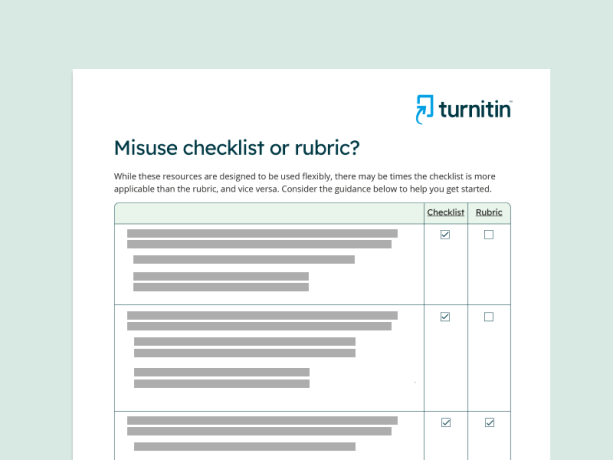
A guide to help educators determine which resource is more applicable to their instructional situation: the AI misuse checklist or the AI misuse rubric.
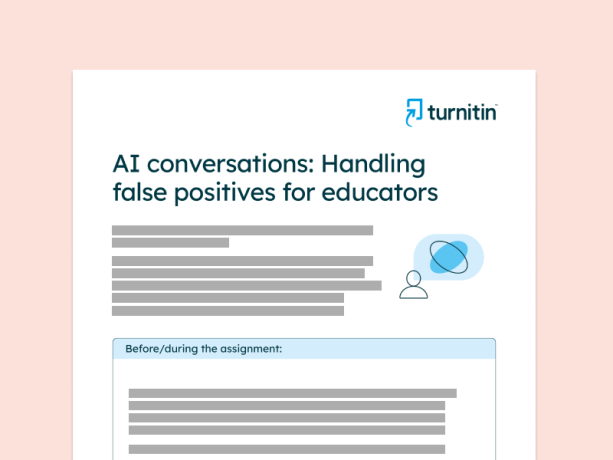
A guide sharing strategies educators can consider to help when confronted with a false positive.
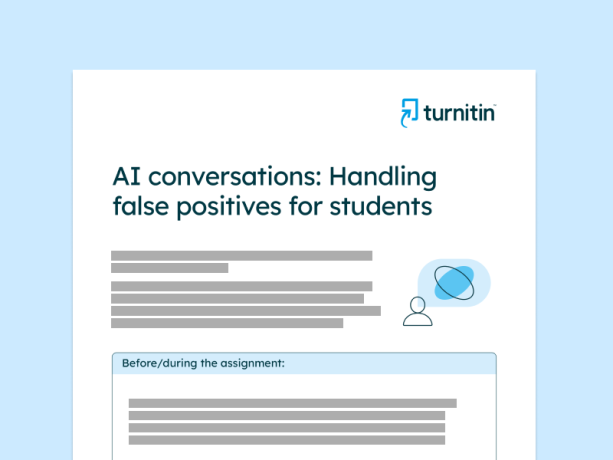
A guide sharing strategies students can consider to help when confronted with a false positive.
The Turnitin Educator Network is a space to meet, discuss and share best practices on academic integrity in the age of AI.
Learn more about ai writing in our blog.
Written by experts in the field, educators, and Turnitin professionals, our blog offers resources and thought leadership in support of students, instructors, and administrators. Dive into articles on a variety of important topics, including academic integrity, assessment, and instruction in a world with machine learning algorithms and artificial intelligence.
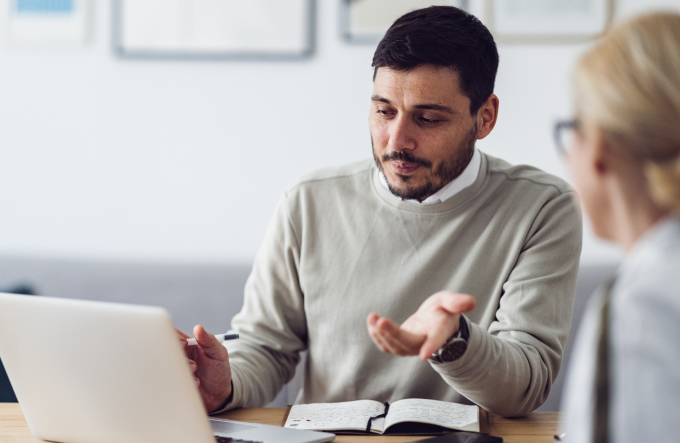
In this blog post, we’re going to address frequently asked questions about AI writing tool misuse for students. Specifically, what does AI writing tool misuse look like? How can you self-check to make sure you’re using AI writing tools properly?
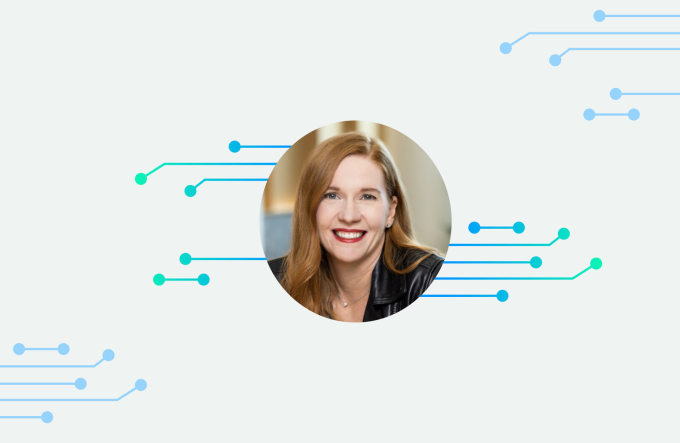
Stay up to date with the latest blog posts delivered directly to your inbox.
Our ai tools in the news.
Never miss an update or announcement. Visit our media center for recent news coverage and press releases.
Cheat GPT? Turnitin CEO Chris Caren weighs in on combating A.I. plagiarism | CNBC Squawk Box
Since the inception of AI-generated writing, educators and institutions are learning how to navigate it in the classroom. Turnitin’s CEO Chris Caren joins ‘Squawk Box’ to discuss how it is being used in the classroom and how educators can identify AI writing in student submissions.
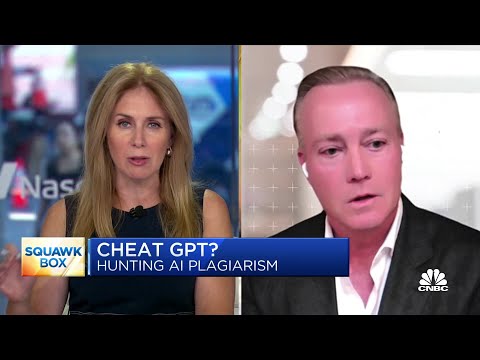
Trouble viewing? View the video on YouTube or adjust your cookie preferences .
Some U.S. schools banning AI technology while others embrace it | NBC Nightly News
ChatGPT, an artificial intelligence program, can write college-level essays in seconds. While some school districts are banning it due to cheating concerns, NBC News’ Jacob Ward has details on why some teachers are embracing the technology.
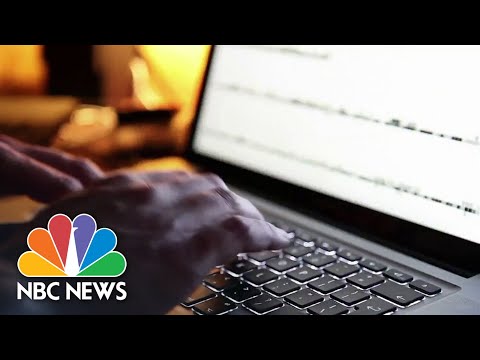
BestColleges
Artificial intelligence, it seems, is taking over the world. At least that's what alarmists would have you believe . The line between fact and fiction continues to blur, and recognizing what is real versus what some bot concocted grows increasingly difficult with each passing week.
ThriveinEDU Podcast
On this episode of the ThriveinEDU podcast, host Rachelle Dené Poth speaks with Turnitin’s Chief Product Officer Annie Chechitelli about her role in the organization, her experience as a parent with school-age children learning to navigate AI writing, and the future of education and original thought.
District Administration
Following the one year anniversary of the public launch of ChatGPT, Chief Product Officer Annie Chechitelli sits down with the publication to discuss Turnitin’s AI writing detection feature and what the educational community has learned.
For press and media inquiries, contact us at [email protected]
Awards & recognition.
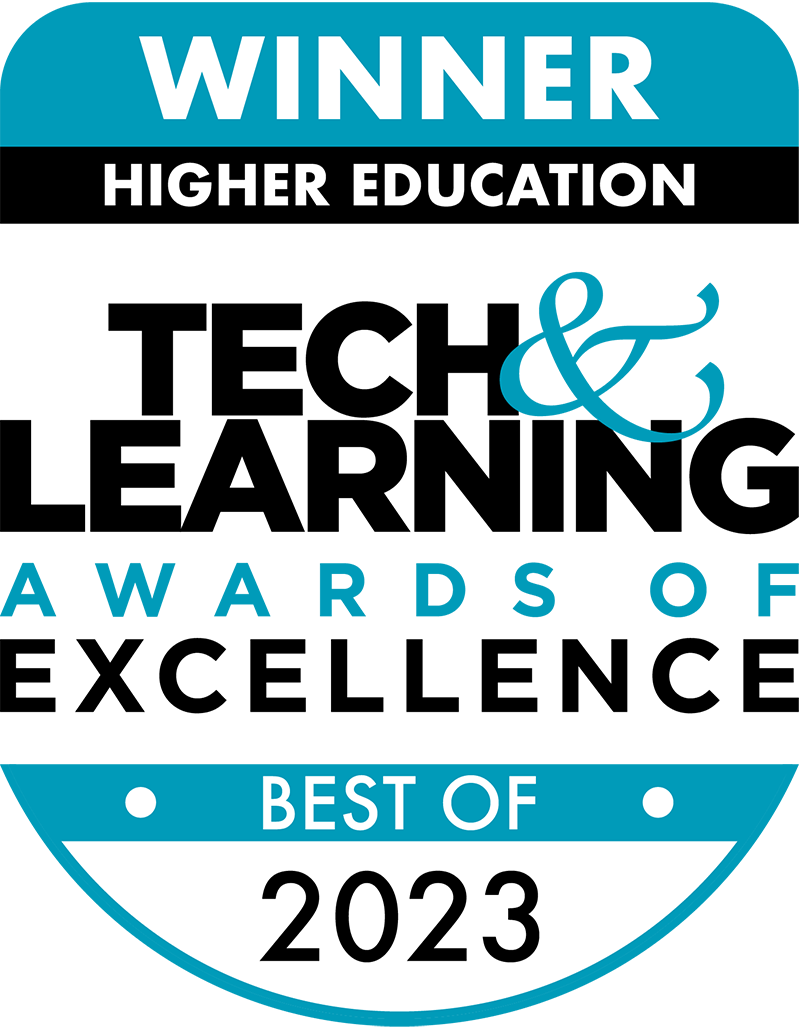
Let’s innovate together
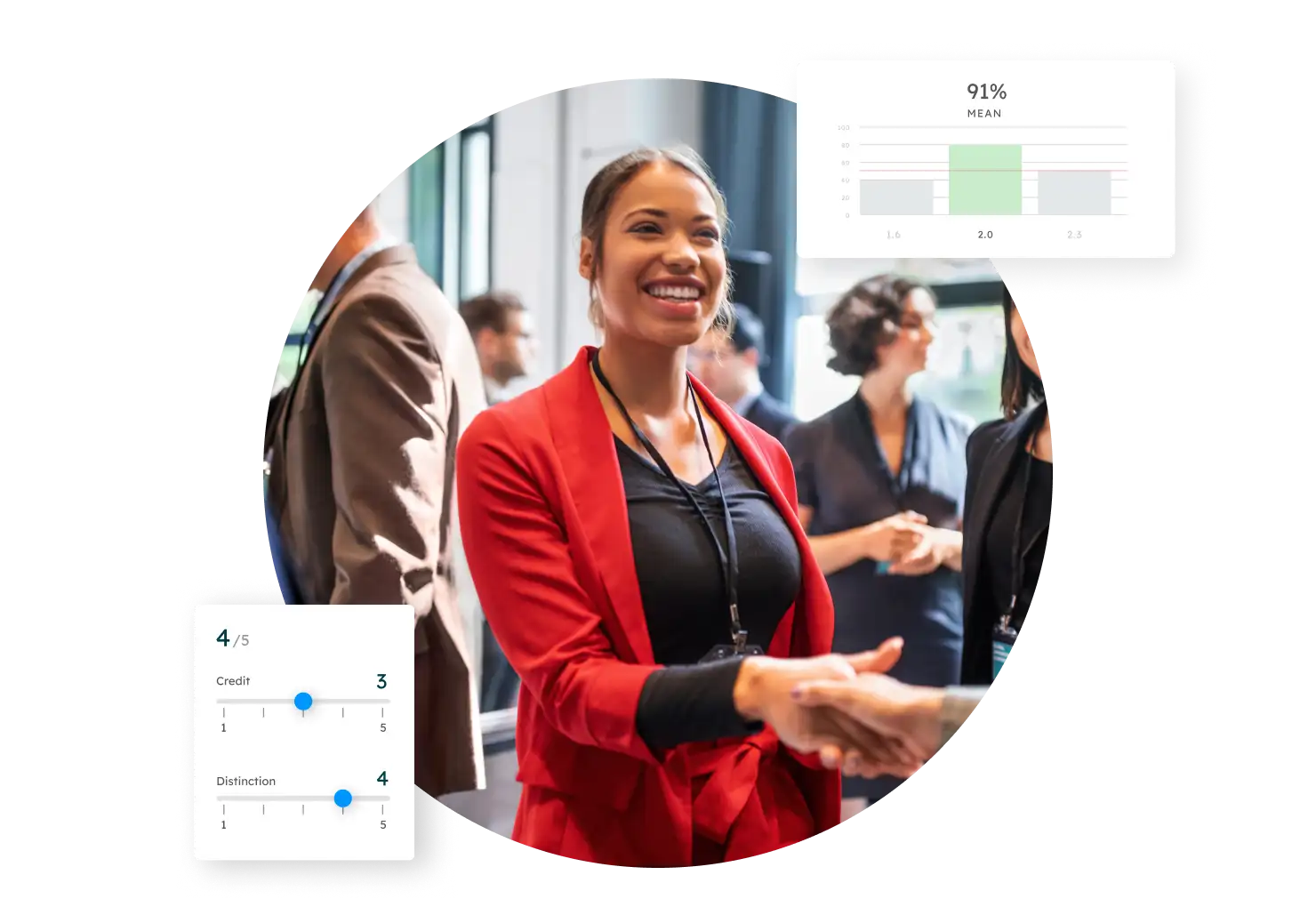
- SYDNEY, NSW
- MELBOURNE, VIC
- HOBART, TAS
- BRISBANE, QLD
- ADELAIDE, SA
- CANBERRA, ACT
- Watch the Paralympic Games closing ceremony here
AI battle lines: How Australia's top anti-cheat software company is prepping for ChatGPT
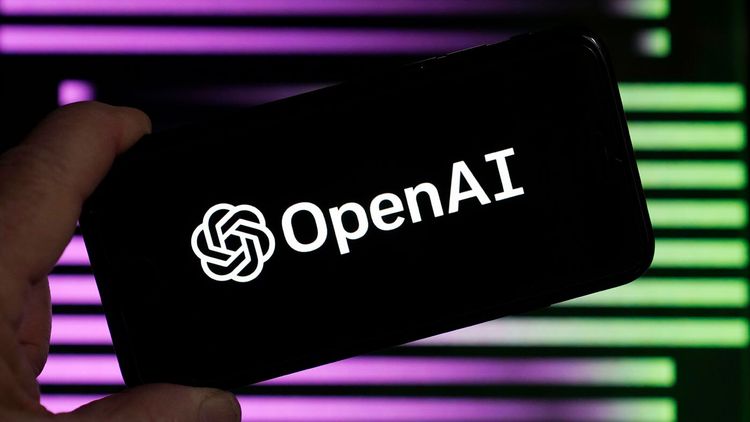
'Greater use of pen and paper assessments'
- Artificial Intelligence
Send your stories to [email protected]
Auto news: Second decline in three months in new car sales Australia.
Top Stories
Paralympic flame blown out but Paris party rages

After dissident writer died suddenly, doctors found this tiny ball in his leg
Why the pope is visiting Australia's closest neighbour
How do you solve a shark murder mystery?
- Share this —
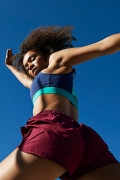
- Watch Full Episodes
- Read With Jenna
- Inspirational
- Relationships
- TODAY Table
- Newsletters
- Start TODAY
- Shop TODAY Awards
- Citi Concert Series
- Listen All Day
Follow today
More Brands
- On The Show
- TODAY Plaza
Teachers sound off on ChatGPT, the new AI tool that can write students’ essays for them
Teachers are talking about a new artificial intelligence tool called ChatGPT — with dread about its potential to help students cheat, and with anticipation over how it might change education as we know it.
On Nov. 30, research lab OpenAI released the free AI tool ChatGPT , a conversational language model that lets users type questions — “What is the Civil War?” or “Who was Leonardo da Vinci?” — and receive articulate, sophisticated and human-like responses in seconds. Ask it to solve complex math equations and it spits out the answer, sometimes with step-by-step explanations for how it got there.
According to a fact sheet sent to TODAY.com by OpenAI, ChatGPT can answer follow-up questions, correct false information, contextualize information and even acknowledge its own mistakes.
Some educators worry that students will use ChatGPT to get away with cheating more easily — especially when it comes to the five-paragraph essays assigned in middle and high school and the formulaic papers assigned in college courses. Compared with traditional cheating in which information is plagiarized by being copied directly or pasted together from other work, ChatGPT pulls content from all corners of the internet to form brand new answers that aren't derived from one specific source, or even cited.
Therefore, if you paste a ChatGPT-generated essay into the internet, you likely won't find it word-for-word anywhere else. This has many teachers spooked — even as OpenAI is trying to reassure educators .
"We don’t want ChatGPT to be used for misleading purposes in schools or anywhere else, so we’re already developing mitigations to help anyone identify text generated by that system," an OpenAI spokesperson tells TODAY.com "We look forward to working with educators on useful solutions, and other ways to help teachers and students benefit from artificial intelligence."
Still, #TeacherTok is weighing in about potential consequences in the classroom.
"So the robots are here and they’re going to be doing our students' homework,” educator Dan Lewer said in a TikTok video . “Great! As if teachers needed something else to be worried about.”
“If you’re a teacher, you need to know about this new (tool) that students can use to cheat in your class,” educational consultant Tyler Tarver said on TikTok .
“Kids can just tell it what they want it to do: Write a 500-word essay on ‘Harry Potter and the Deathly Hallows,’” Tarver said. “This thing just starts writing it, and it looks legit.”
Taking steps to prevent cheating
ChatGPT is already being prohibited at some K-12 schools and colleges.
On Jan. 4, the New York City Department of Education restricted ChatGPT on school networks and devices "due to concerns about negative impacts on student learning, and concerns regarding the safety and accuracy of content," Jenna Lyle, a department spokesperson, tells TODAY.com. "While the tool may be able to provide quick and easy answers to questions, it does not build critical-thinking and problem-solving skills, which are essential for academic and lifelong success."
A student who attends Lawrence University in Wisconsin tells TODAY.com that one of her professors warned students, both verbally and in a class syllabus, not to use artificial intelligence like ChatGPT to write papers or risk receiving a zero score.
And last month, a student at Furman University in South Carolina got caught using ChatGPT to complete a 1,200-word take-home exam on the 18th century philosopher David Hume.
“The essay confidently and thoroughly described Hume’s views on the paradox of horror in (ways) that were thoroughly wrong,” Darren Hick, an assistant professor of philosophy, explained in a Dec. 15 Facebook post . “It did say some true things about Hume, and it knew what the paradox of horror was, but it was just bullsh--ting after that.”
Hick tells TODAY.com that traditional cheating signs — for example, sudden shifts in a person’s writing style — weren’t apparent in the student’s essay.
To confirm his suspicions, Hick says he ran passages from the essay through a separate OpenAI detector, which indicated the writing was AI-generated. Hick then did the same thing with essays from other students. That time around, the detector suggested that the essays had been written by human beings.
Eventually, Hick met with the student, who confessed to using ChatGPT. She received a failing grade for the class and faces further disciplinary action.
“I give this student credit for being updated on new technology,” says Hick. “Unfortunately, in their case, so am I.”
Getting at the heart of teaching
OpenAI acknowledges that its ChatGPT tool is capable of providing false or harmful answers. OpenAI Chief Executive Officer Sam Altman tweeted that ChatGPT is meant for “ fun creative inspiration ” and that “ it’s a mistake to be relying on it for anything important right now.”
Kendall Hartley, an associate professor of educational technology at the University of Las Vegas, Nevada, notes that ChatGPT is "blowing up fast," presenting new challenges for detection software like iThenticate and TurnItIn , which teachers use to cross-reference student work to material published online.
Still, even with all the concerns being raised, many educators say they are hopeful about ChatGPT's potential in the classroom.
When you think about the amazing teachers you’ve had, it’s likely because they connected with you as a student. That won’t change with the introduction of AI.”
Tiffany Wycoff, a former school principal
"I'm excited by how it could support assessment or students with learning disabilities or those who are English language learners," Lisa M. Harrison, a former seventh grade math teacher and a board of trustee for the Association for Middle Level Education , tells TODAY.com. Harrison speculates that ChatGPT could support all sorts of students with special needs by supplementing skills they haven’t yet mastered.
Harrison suggests workarounds to cheating through coursework that requires additional citations or verbal components. She says personalized assignments — such as asking students to apply a world event to their own personal experiences — could deter the use of AI.
Educators also could try embracing the technology, she says.
"Students could write essays comparing their work to what's produced by ChatGPT or learn about AI," says Harrison.
Tiffany Wycoff, a former elementary and high school principal who is now the chief operating officer of the professional development company Learning Innovation Catalyst (LINC), says AI offers great potential in education.
“Art instructors can use image-based AI generators to (produce) characters or scenes that inspire projects," Wycoff tells TODAY.com. "P.E. coaches could design fitness or sports curriculums, and teachers can discuss systemic biases in writing.”
Wycoff went straight to the source, asking ChatGPT, "How will generative AI affect teaching and learning in classrooms?" and published a lengthy answer on her company's blog .
According to ChatGPT's answer, AI can give student feedback in real time, create interactive educational content (videos, simulations and more), and create customized learning materials based on individual student needs.
The heart of teaching, however, can't be replaced by bots.
"When you think about the amazing teachers you’ve had, it’s likely because they connected with you as a student," Wycoff says. "That won’t change with the introduction of AI."
Tarver agrees, telling TODAY.com, "If a student is struggling and then suddenly gets a 98 (on a test), teachers will know."
"And if students can go in and type answers in ChatGPT," he adds, "we're asking the wrong questions.”
Elise Solé is a writer and editor who lives in Los Angeles and covers parenting for TODAY Parents. She was previously a news editor at Yahoo and has also worked at Marie Claire and Women's Health. Her bylines have appeared in Shondaland, SheKnows, Happify and more.
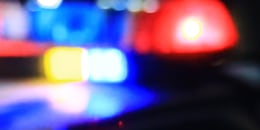
How to talk to children about shootings: An age-by-age guide
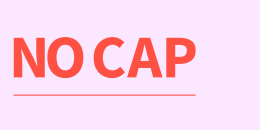
What does ‘no cap’ mean?
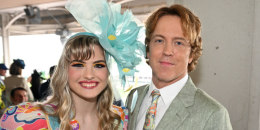
Anna Nicole Smith’s daughter Dannielynn debuts a dark wig in photos shared by her dad
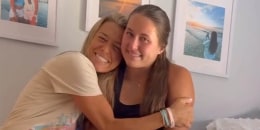
Mom sleeps over in daughter’s dorm room on her first night in college: Sweet or selfish?
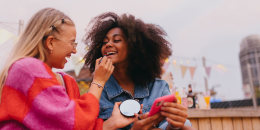
Who exactly is Gen Alpha and Gen Z? A guide to the generation names
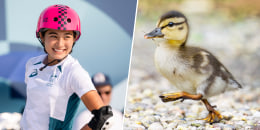
You’ll quack up when you hear what this Olympian asked her parents for
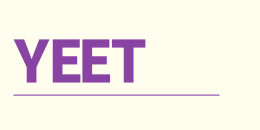
Why are teens yelling ‘yeet!’?
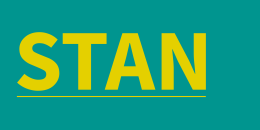
Should you worry if your teen is a ‘stan’?
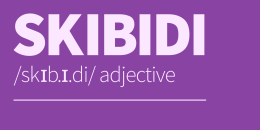
‘Skibidi toilet’ could get made into a movie: What does it mean?
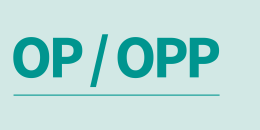
OP and OPP: They might not mean what you think they do. Teen slang, explained
- Staff Directory
- Workshops and Events
- For Students
Combating Academic Dishonesty, Part 6: ChatGPT, AI, and Academic Integrity
by Thomas Keith | Jan 23, 2023 | Instructional design , Services
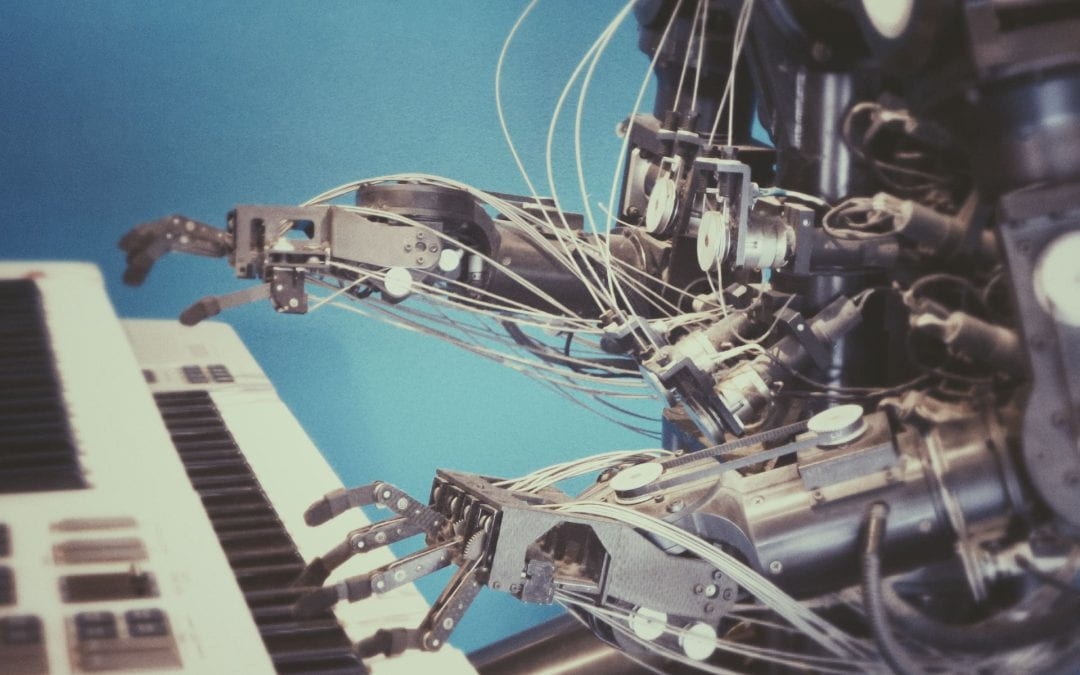
Author’s Note: This is the latest installment in an ongoing series of articles about issues pertaining to academic integrity in higher education. For earlier installments, please see: Part 1 | Part 2 | Part 3 | Part 4 | Part 5
ATS instructional designers Mohammad Ahmed and Michael Hernandez contributed content to this article.
Introduction
The state of the tool, issues for academic integrity, technological prevention, non-technological prevention, creative adaptation, further resources.
There are few current issues in education that have provoked more interest – or sounded more alarms – than artificial intelligence (AI) technology. While the issue has simmered for some time, it burst into the forefront of debate following OpenAI’s public release of ChatGPT . When given a prompt – e.g. “What were the causes of World War I?” or “How does the Krebs cycle work?” – ChatGPT (the acronym stands for “Generative Pretrained Transformer”) can generate text that reads, at least on superficial examination, like that written by a human – the basis of the famed Turing Test for machine intelligence.
Once the tool’s capabilities became known, it did not take long for fears to be voiced that students would turn to ChatGPT to write their assignments for them. Eye-grabbing headlines began to appear, not only in sensationalist newspapers like the New York Post ( which dubbed the tool “CheatGPT” ) but in more sober publications like the Atlantic , where an opinion piece bluntly claimed that “the college essay is dead”. Advocates for the worst-case scenario see a future in which human-generated and computer-generated text are indistinguishable, essay assignments are meaningless, and the very skill of academic writing is lost.
One need not accept this doomsday proposition to recognize that ChatGPT raises legitimate concerns for academic integrity. But if we are to address such concerns, we must first answer several key questions: what is ChatGPT, exactly? What are its affordances and limitations? And, assuming that ChatGPT and tools like it are here to stay (as seems overwhelmingly likely), how should we rethink pedagogy to address this new reality?
At this time, ChatGPT is essentially an information aggregator. It trawls vast quantities of human-produced texts and extracts data, which it then synthesizes into a response to a given prompt. As noted above, its responses on many topics are at least coherent enough that they may be superficially indistinguishable from student writing.
As with all AI tools, though, ChatGPT’s capacity to give responses depends upon what, and how much, it is “fed”. Its lack of data on current events, for example, limits its capacity to respond to prompts such as “How is the war in Ukraine progressing today?” There are also guardrails in place to prevent the tool’s being used for nefarious purposes (though cybercriminals are already seeking to circumvent these ).
There are other significant limitations to the tool as well. It cannot cite sources correctly – any request for a bibliography produces false and/or irrelevant citations. Nor is it error-free: users have run across blatant, even comical, mistakes when ChatGPT is asked a question as straightforward as “How do you work?” Like all AI, it is subject to the biases of those who supply its data . And, most fundamentally, it is not true artificial intelligence. There are no indications that ChatGPT understands the questions it is being asked or what it is producing in answer; simply put, it is not sapient. This is worth keeping in mind as the debate rages over whether such tools are capable of supplanting human creativity.
It is, without question, too early in the game to expound upon all the possible difficulties ChatGPT and similar generative AI tools could pose for academic integrity. Already, however, ChatGPT-generated text has proved itself capable of evading plagiarism checkers such as Turnitin. Plagiarism detection software relies on comparing student work to a database of pre-existing work and identifying identical phrases, sentences, etc. to produce an “originality score”. Because the text generated by ChatGPT is (in some sense, anyway) “original,” it renders this technique useless.
ChatGPT also ties into the broader issue of contract cheating – hiring a third party to do work, such as writing an essay or taking an exam, on a student’s behalf. Contract cheating is already a severe problem worldwide, and with the widespread availability of AI writing tools, students can now generate “original” written work for free, without the need to involve a human agent who might betray the student’s confidence.
How Do We Deal with the Problem?
As the New York Times has noted , many faculty and instructors already feel the need to adjust their pedagogy to account for the existence of ChatGPT. Their strategies, actual and proposed, for coping with the tool can be divided into three categories: technological prevention; non-technological prevention; and creative adaptation. We shall consider each of these in turn below.
It will come as no surprise that technological counters to ChatGPT are already in play. A 22-year-old computer science student at Princeton named Edward Tian has introduced GPTZero , which claims to distinguish human- and computer-generated text with a high degree of accuracy. Meanwhile, other plagiarism tools, such as Turnitin, offer their own AI-detection tools and are rapidly working to detect the newest generation of generative AI text. And finally, the makers of ChatGPT are themselves exploring “watermarking” technology to indicate when a document has been generated by the software.
Some experts foresee an “arms race” between AI writing tools and AI detection tools. If this scenario comes to pass, faculty and instructors will be hard-pressed to keep up with the bleeding-edge software needed to counter the newest writing tools. But more fundamentally, we might ask: is technology always the best solution to the problems it creates? Or are there other, perhaps less involved, means of addressing the questions raised by AI?
At the other end of the spectrum, some faculty and instructors have sought to neutralize ChatGPT entirely. This may entail banning ChatGPT specifically; banning all computers in the classroom; supervising student essay-writing, whether in class or via monitoring software such as Proctorio; or even requiring writing assignments to be handwritten.
The concern that underlies such measures is understandable, and they can be effective in the short-term, but they come at a cost: aside from the anxiety that can be provoked by being under surveillance, accessibility issues that may be raised by requiring handwritten work, and the legal/ethical issues raised by video proctoring, students miss the opportunity to learn about the tool and its implications. As we confront the likelihood of a future with ubiquitous AI technology, those students who have never experienced tools like ChatGPT and who know nothing about their uses may well find themselves at a professional disadvantage.
At this point in time, it seems most productive to take a third path – one that balances the need to safeguard academic integrity with the reality that ChatGPT and its like are here to stay. Here are some suggestions for methods to integrate AI tools like ChatGPT into your pedagogy in a productive, rather than destructive, fashion.
- Clarify expectations at the outset. As early in your course as possible – ideally within the syllabus itself – you should specify whether, and under what circumstances, the use of AI tools is permissible. It may help to think of ChatGPT as similar to peer assistance, group work, or outside tutoring: in all these cases, your students should understand where the boundaries lie, when help is permissible, and when they must rely on their own resources. You might also discuss with your students how they feel about AI and its ability (or lack thereof) to convey their ideas. Emergent research suggests that at least some students feel dissatisfied with the results when they entrust expression of their ideas to AI.
- Craft writing prompts that require creative thought. A tool like ChatGPT can easily respond to a simple prompt such as “What are the causes of inflation?”, but it is likely to have trouble with a prompt such as “Compare and contrast inflation in the present-day American economy with that in the late 1970s”. The more in-depth and thought out the prompt, the more it will demand critical reasoning – not simply regurgitation – to answer.
- Run your prompts through ChatGPT. Related to the point above, actually using ChatGPT on a draft of your writing prompt can be an illuminating exercise. Successive iterations may help you to clarify your thinking and add nuances to your prompt that were not present in the initial draft.
- Scaffold your writing assignments. This is a time-honored technique for combating plagiarism of any kind in academic writing. It will be much harder for a student to submit a final draft generated by AI and get away with it if you have observed that student’s thinking and writing process throughout the course.
- Promote library resources. As mentioned previously, ChatGPT is not presently able to generate an accurate bibliography, nor does it understand the concept of citation. This shortcoming can be a good jumping-off point for you to explain to your students how to cite properly, why citation is important, and how they can use available resources to do their own research.
- Model productive use of AI tools. For all its hazards, ChatGPT also offers promising possibilities. A “dialogue” between the user and the tool can help the user to probe deeper into the subject matter, become familiar with mainstream scholarship on the topic, and push beyond “easy answers” toward original work. To promote such dialogue, you might, for example, assign your students to come up with their own prompts, post them to ChatGPT, and then comment on the answers, finding the strengths and weaknesses of the “argument” that the tool generates.
In a field evolving by the day, no article, this one included, can hope to offer definite answers. What we have presented here are points we hope will contextualize the debate and provide a framework for further discussion. In the end, what AI tools will mean for higher education – and for society as a whole – remains to be seen.
To learn more about AI in the classroom, we recommend the excellent page on AI Guidance from Yale’s Poorvu Center for Teaching and Learning. Turnitin also offers a concise but helpful Guide for approaching AI-generated text in your classroom .
If you have further questions, Academic Technology Solutions is here to help. You can schedule a consultation with us or drop by our office hours (virtual and in-person, no appointment needed). We also offer a range of workshops on topics in teaching with technology.
(Cover Photo by Possessed Photography on Unsplash )
Search Blog
Subscribe by email.
Please, insert a valid email.
Thank you, your email will be added to the mailing list once you click on the link in the confirmation email.
Spam protection has stopped this request. Please contact site owner for help.
This form is protected by reCAPTCHA and the Google Privacy Policy and Terms of Service apply.
Recent Posts
- Make Your Canvas Course Sites More Accessible with PopeTech
- Five Simple Steps to Humanize Your Pedagogy
- Help Students Cultivate Scholarly Habits by Engaging with Art (Even Outside of the Museum!): “Habits of Mind” Blog Series, Part Three
- Make Your Google Docs, Sheets, and Slides More Accessible with Grackle Workspace
- Disassemble Big Ideas for Greater Student Understanding Using Concept Maps: Habits of Mind Series, Part Two
- A/V Equipment
- Accessibility
- Canvas Features/Functions
- Digital Accessibility
- Faculty Success Stories
- Instructional design
- Multimedia Development
- Poll Everywhere
- Surveys and Feedback
- Symposium for Teaching with Technology
- Uncategorized
- Universal Design for Learning
- Visualization
Wipe out plagiarism & grammar errors

Get automatic paper help
- Expert Check
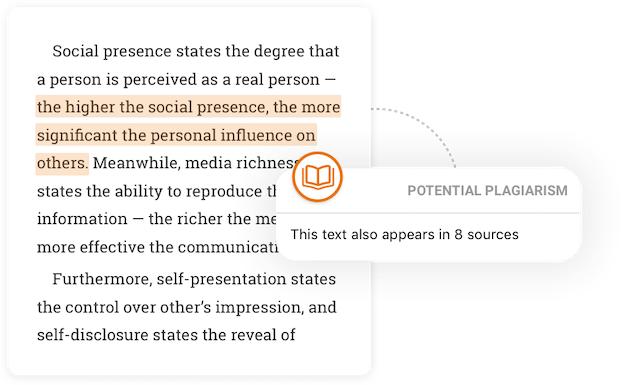
Check for unintentional plagiarism
Check your paper for unintentional plagiarism against thousands of sources.
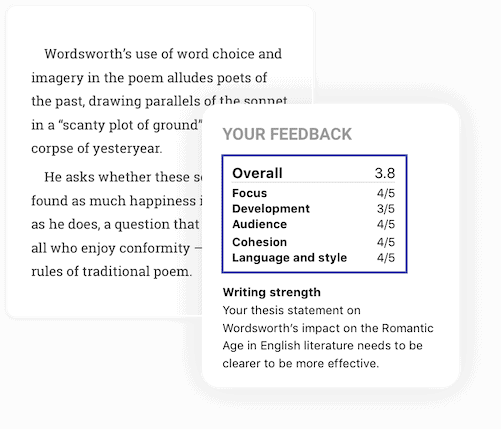
Strengthen your writing
Give your paper an in-depth check. Receive feedback within 24 hours from writing experts on your paper's main idea, structure, conclusion, and more.
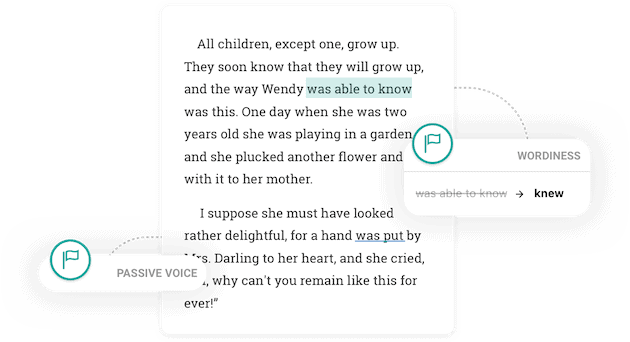
Find and fix grammar errors
Don't lose points over small mistakes. Our smart proofreader checks for grammar and writing mistakes so that you don't have to.
What students are saying about us
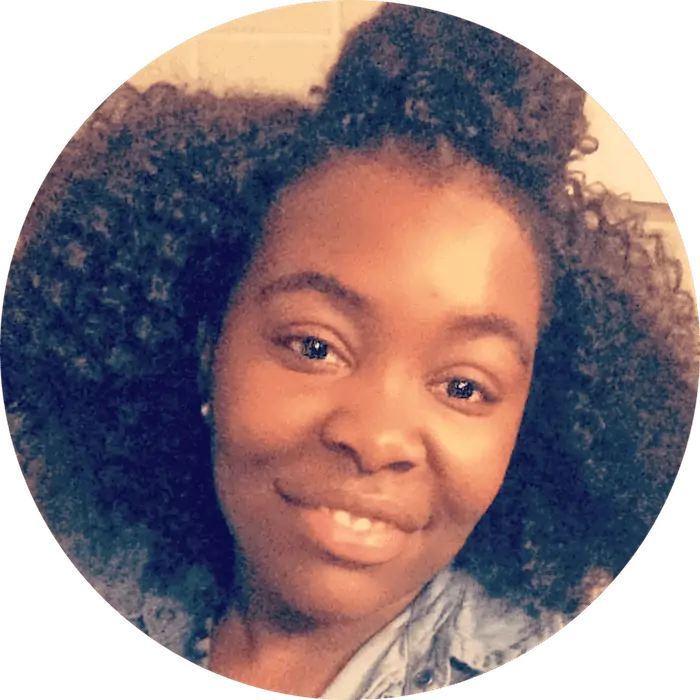
"Caught comma errors that I actually struggle with even after proofreading myself."
- Natasha J.
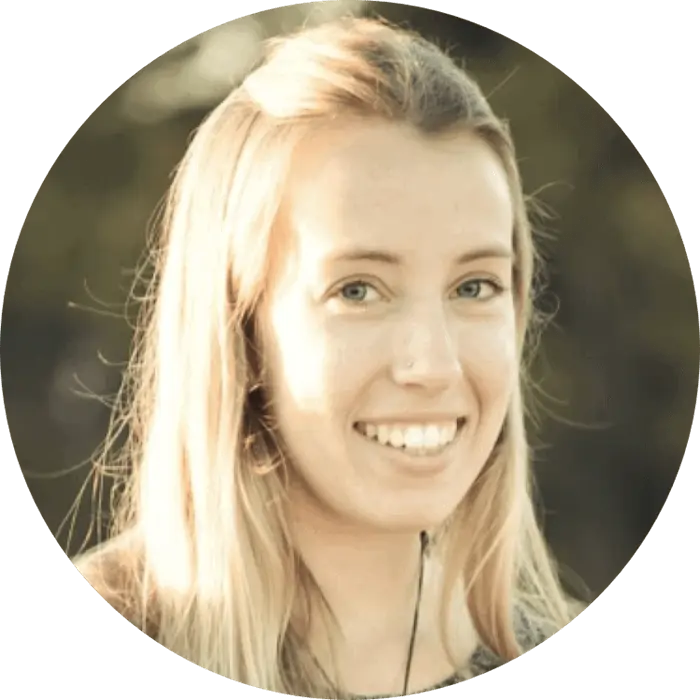
"I find the suggestions to be extremely helpful especially as they can instantly take you to that section in your paper for you to fix any and all issues related to the grammar or spelling error(s)."
- Catherine R.
Write with confidence with...
Features | Free Account | EasyBib Plus |
---|---|---|
Unlimited suggestions to improve your writing style, grammar and sentence structure | Unlimited | Unlimited |
Unlimited checks for unintentional plagiarism | None | Unlimited |
Multiple styles and source type to choose from to create accurate citations | None | Unlimited |
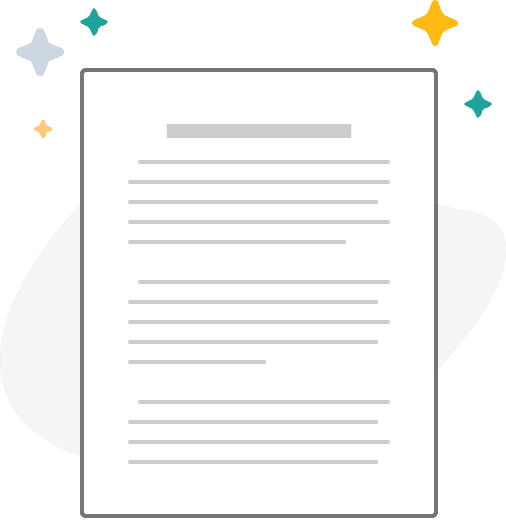
Personalized Writing Help When You Need it
Unintentional plagiarism, grammar mistakes, and uncited sources can turn what you thought was a good paper into a poor one. When you’re writing a paper the last thing you want is for your message to get lost due to incorrect punctuation or confusing sentence structure. You know that the great ideas in your head would make for a standout paper, if only you could get them written clearly on the page. If this struggle sounds familiar, you’re not alone.
Great ideas may be an essential part of high-quality writing, but they’re not the only component. Excellent papers and essays clearly express strong ideas with good grammar, proper punctuation, spot-on spelling, and thorough, careful citations. While this may sound like a lot, your teachers and professors are grading you on your skills as both a writer and a researcher, which means your assignments will require an ethical and attentive approach. Luckily, there is no shortage of available tools to help you along your way.
You could use a plagiarism checker free, though, these tools often lack grammatical support. Given the high stakes and rigorous requirements, the aid of a plagiarism checker without the needed support of a grammar checker could mean the difference between an “A” paper and a “C” or even “D” paper.
Thankfully, the EasyBib Plus plagiarism tool provides all-in-one support to cover all your bases. Our premium essay checker is convenient, easy to use, and includes access to a grammar and spell checker, plus a plagiarism checker. With a single scan, you’ll receive personalized feedback to help identify potentially missing citations and help improve your sentence structure, punctuation, grammar, and more.
Not sure if that noun is spelled correctly, or if the preposition at the end of your sentence is grammatically correct? The EasyBib Plus plagiarism tool is your one-stop shop to help check plagiarism, get grammatical suggestions, correct spelling and punctuation errors, and help create polished papers you can be proud to turn in. And, we haven’t told you the best part yet: you can try our tool free and scan your work for grammar suggestions right now!
Access all the tools today!
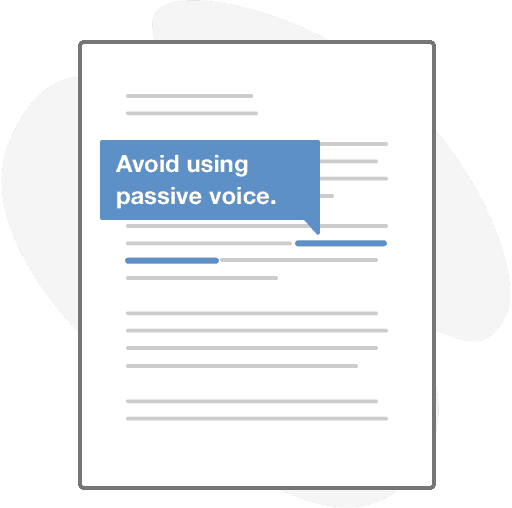
A Grammar Check for Peace of Mind
You know that grammar plays a significant role in your assignments. Not only does it factor into your overall grade, but without clear and precise language, your ideas can lose their impact or might even be misunderstood entirely. Still, with so many different parts of speech and rules to learn and apply, it’s not uncommon to get them mixed up and find yourself questioning your knowledge now and again:
Can you use an adverb to modify a noun? (No. You need an adjective for that.)
Well, can you use it to modify a pronoun ? (Still no.)
What do they modify, then? (Almost everything else. Fun, right?)
Ugh. (That was an interjection .)
Does this have to be so difficult? (Nope!)
Scanning your paper with the EasyBib Plus writing tool delivers more than the basic punctuation and spell check functions that come standard in word processing programs. You’ll receive immediate, targeted feedback that can help improve the sentence structure and style of your writing. Not only can this help ensure that you don’t lose points off your grade for grammatical errors such as mismatched verb tense, but it can also help to clarify your meaning and strengthen your arguments by eliminating confusing punctuation and run-on sentences that confuse readers.
Reviewing your work to correct errors and refine the flow of your writing is a critical part of the revision process for novices and novelists alike. Even the most conscientious of wordsmiths might make mistakes, such as using a conjunctive adverb in place of a coordinating conjunction or pairing a plural determiner with an uncountable word. They likely appreciate a subscription-based or free grammar check as much as a beginning writer. That’s why the EasyBib Plus writing tool is designed for all writers, from students who are still learning the fundamentals to published professionals who get paid by the word.
The EasyBib Plus writing tool provides quick, targeted feedback that you can use to help improve your writing immediately. Also, our free resource library is available 24/7, just like the rest of our tools, to help you brush up on the areas that challenge you the most (Conjunctive what? Uncountable who?) That means you can continue to sharpen your skills and improve your writing over time, which will help make finishing your next project easier.
Ready to give your paper a boost? Sign up for EasyBib Plus or scan a paper right now for a free spell check—it’s easy!Just upload or copy and paste your paper to the online grammar check tool and, in a matter of seconds, you’ll be able to receive up to 5 feedback cards so you can begin polishing your draft.
To learn more about proofreading tools, you can find more info here. Or, for more tips on grammar, punctuation, and style, check out this useful reference .
Why is Grammar So Important, Anyway?
Why is grammar important? As long as others know what you mean, does it really matter if you use proper grammar? These are age-old questions, but the answers remain unchanged. Grammar is important for many reasons:
Communication: Communication is about more than merely listening and talking. We communicate in myriad ways: with our voices, our mannerisms, our facial expressions, our actions, and frequently, our written words. Written communication is just as important as all the other ways we broadcast our thoughts and feelings. Unlike our other communication methods, though, written communication leaves a record. While most of us relax our style when talking to or texting friends, the fact remains that more formal venues require a more formal tone. Proper spelling, grammar, and punctuation are a necessary element of professional and academic writing, so running your work through a spelling and grammar check before you submit it is an absolute must.
Clarity: Expressing your thoughts, ideas, and opinions is an uphill battle when you communicate them with less-than-stellar punctuation, spelling, and grammar. A missing or misplaced comma, for example, can entirely change your meaning:
- Call me Ishmael = My name is Ishmael.
- Call me, Ishmael = I want Ishmael to call me.
Spelling errors can result in similarly muddled meaning:
- Her shoes perfectly complemented her dress. = Her shoes and dress go well together.
- Her shoes perfectly complimented her dress. = Her shoes can both speak and engage in flattery.
Grammar deficiencies such as a dangling modifier yield similarly confusing results:
- I saw the girl’s purple backpack in the library. = The purple backpack belonging to the girl is in the library; I saw it.
- I saw the purple girl’s backpack in the library. = You know the girl who mysteriously turned purple? I saw her backpack in the library. This week has not been easy on her.
Credibility: Establishing your credibility is critical whether you’re writing for school or business. Proving that you can reliably communicate using proper grammar is essential to establishing and maintaining the trust of your teachers, professors, readers, customers, and colleagues.
To learn even more about the importance of English grammar in writing, check here .
What Happens After I Upload My Paper?
Once you upload a paper, the EasyBib Plus grammar checker scans your text and highlights grammar issues within your document so you can see it in context. For users running a grammar check and spell check using the grammar check free tool, up to the first 5 issues are shown. For subscription subscribers, all of the areas that require your review will be highlighted once the scan is complete.
No matter which version of the tool you’re using, your feedback will include detailed explanations so you can understand why the text was flagged. Other highlighted areas will include examples of how the issues can be fixed. Some will include a detailed explanation as to why the issue was flagged. This personalized feedback allows you to make an educated decision about whether to edit your text or dismiss the suggestion, so you’re always in control of your final draft.
Subscription users also have the plagiarism checker at their disposal when scanning their papers for style and grammar suggestions. Just as with our other writing tools, when you use the tool to check plagiarism, changes are never made without your review, so you are always in the driver’s seat. You can review each highlighted area as well as the sources of matching text and will always be given a choice to either accept or ignore both citation and grammar suggestions.
Whether you are a student or a professional, the EasyBib Plus tools are powerful allies that can help you improve your paper, establish credibility as a writer, and maintain an ethical writing process.
Try our checker free for 3 days to see what you think*. Trust us; you’ll wonder how you got along so far without it!
*See Terms and Conditions
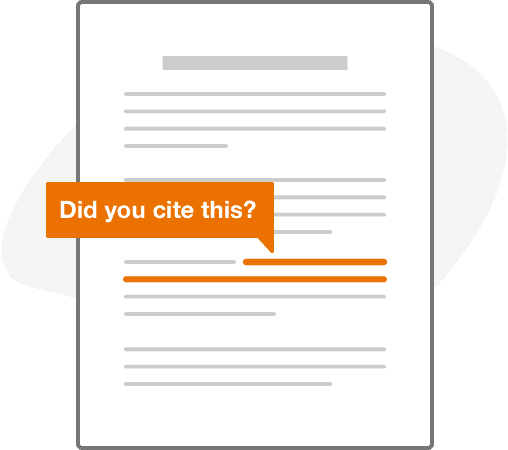
Check for Unintentional Plagiarism
Persistent use of the best grammar checker and spell checker you can find will help to shape up the style and substance of your composition. However, to ensure the integrity of your work and root out unintentional instances of academic dishonesty, you’ll also need to incorporate a subscription-based or free plagiarism checker for students.
The ability to scan your paper and check plagiarism and grammar before submitting your work is an invaluable tool for students. It’s so valuable, in fact, that students are not the only ones who consistently use this type of software.
Many teachers and professors use a service to ensure that student papers are original and include properly formatted and sourced citations for all reference materials. For some, this may be a consistent element of their grading process, while others may utilize one only when they feel there is a reason to do so.
What Exactly Does a Plagiarism Checker Do?
If you’ve used an online plagiarism checker before, you may be familiar with the process that these tools employ. For those who have never used one, it can be helpful to understand how these tools work and what you should expect as a result of using one.
The most basic free tools will scan your paper and provide you with a percentage that indicates how much of your work is original. This feature can be useful to a point, as some instructors and institutions set a percentage for the amount of similar text that is considered acceptable. This policy does not mean that they are lenient about academic dishonesty but, instead, that they recognize that similar or even matching word choice is not always an indicator of copying.
However, some schools do not tolerate any form of incremental plagiarism, period. In these institutions, “check paper for plagiarism” is likely a standard action when grading all papers.
With a free plagiarism checker, the percentage of word-for-word plagiarism in your text may be the only feedback you receive. Moreover, some of these providers may wade in unethical waters, and offer essay writing services in conjunction with their checking services. This is why many students and teachers prefer subscription-based checkers that focus on integrity, such as the EasyBib writing tools.
With our premium tools, you get more than just supporting proof that your paper is original and in your own words. Your writing is scanned for potentially matching text and areas that needs your attention is highlighted. We’ll provide you with the source of the matching text so you can review it and decide how to proceed. If you agree that a citation is needed, our citation tools and resources will provide you with the information you need to help format and insert the new sources in your text as well as your works cited page.
How Does the EasyBib Plus Online Plagiarism Checker Work?
The EasyBib Plus writing tool provides an all-in-one spell check, grammar review, and plagiarism checker that not only helps you with the paper you’re writing now but enables you to gain new knowledge and improve your writing for the future, too.
Our essay checker searches online for phrases, sentences, or passages that are similar to those in your paper. If it returns matching text, this may indicate that you have passages that are missing citations.
Just as with many checkers, our tool will tell you how many instances of matching text it finds in your paper. Our plagiarism tool is designed to do more than most free tools, however.
When a portion of your paper is flagged for review, we provide you with the source that it matches. As with our online spell check tool, you have the power to review each area and choose how to proceed. If you decide to cite the flagged text, you can review the suggested source and access our citation tools to help create a proper citation and start building a bibliography for your paper. Or, you may determine that no citation is needed, in which case it’s simple to dismiss the suggestion and continue to the next section.
If you’re unsure about how to proceed, our library of resources can help you learn more and make an educated decision. In this way, you gain more than just the ability to correct your work; over time, you can learn how to avoid plagiarism altogether.
Reasons Your Teacher May Run Your Paper Through a Plagiarism Detector
As stated previously, your teachers and professors grade papers with a fixed process that includes “check paper for plagiarism” as a standard, across-the-board action. Others may check for plagiarism only when they feel there is cause to do so. There are a few red flags that may stand out to them while reviewing a paper that would lead to their running a plagiarism check, including:
Style/Voice: Most teachers are familiar with the style and voice that you use in your writing. While most will hope that the feedback they provide when grading your work will help you to improve problem areas over time, a paper or essay that has a dramatically different style or voice than the work you’ve previously submitted can be a red flag.
Inconsistency: Changes in the font, font size, formality, formatting, and more may indicate that portions of a paper have been copied and pasted. If these inconsistent passages are not presented as quotations or don’t include citations, this may further indicate to your teacher that there’s plagiarism in the paper.
Old References: Part of a well-researched paper is verifying the legitimacy and relevance of your sources. While some older references may be acceptable depending on the topic, if all of your sources are outdated, it may lead your teacher to believe that you’ve recycled your own work or someone else’s.
Looking for a checker to try? With EasyBib Plus you get unlimited checks to give you peace of mind when turning in your papers!
What is Plagiarism?
If you’re looking for a free online plagiarism checker , you’re already aware that it’s not something you want to be found in your papers. However, you may still be wondering: what does plagiarism mean? It’s a reasonable question and one that merits exploring. After all, some of the actions and circumstances that fall within the plagiarism definition come as a surprise to those who only learn that they’ve committed them after it’s too late.
So what is plagiarism? To define plagiarism in the most basic sense, you might say that it is taking someone else’s ideas and words and passing them off as your own.
If your goal is avoiding plagiarism entirely, you’ll need to go beyond the basics to thoroughly define plagiarism, recognize it, and keep clear of it. Some examples of plagiarism that students find surprising include forgotten citations, poor paraphrasing, and re-submitting your own work in whole or in part for more than one assignment. This useful article provides more help in recognizing and understanding the different forms that academic dishonesty can take.
Of course, using your own words and ideas does not count as plagiarism, nor does using common knowledge . Basically, common knowledge is information that is well known by the average person. Examples of common knowledge:
- there are 12 months in a year
- the freezing point of Celsius is zero degrees
- Socrates was a Greek philosopher
So should you only include your original thoughts and common knowledge in your papers? Of course not! Research-based assignments are meant to demonstrate your skills as a researcher, after all, as well as your ability to build upon the work of others to formulate new ideas. To avoid accidentally committing an act that falls within the plagiarism definition when you’re using another person’s words or ideas, though, you need to give them proper credit. This means you’ll need to clearly identify direct quotations or properly paraphrase them when including them in your paper.
Regardless of your approach, you’ll also need to cite your sources according to the style your professor specifies. Generally, you will use MLA format for the arts and humanities and APA format for the sciences, but it’s always best to check with your instructor when you’re unsure.
If you know what style to use but still aren’t sure how to create your citations, don’t fret! Our library of resources includes free guides to help you learn about various styles so you can properly structure and place them. And our premium tools not only help you check grammar, spelling, and originality in your papers, but subscribers also enjoy access to our citation creation tools!
What are the Different Types of Plagiarism?
In addition to the question “what is plagiarism,” you may also be wondering, “why do students plagiarize?” While some students do intentionally plagiarize because they believe they can pass off someone else’s work as their own to avoid spending time on their assignments, many others do so accidentally. They may not understand how broad the plagiarism definition is or they haven’t learned how to research and cite their sources properly. That is why it is vital to recognize plagiarism in all of its forms if you wish to ensure the integrity of your work.
Examples of plagiarism & How to prevent it
Direct plagiarism:
Intentionally copying another person’s work without including a citation that gives credit to the source. When most students are asked to identify potential plagiarism examples and behaviors, this direct and deliberate act is what they think of first.
- Prevention: If you use an idea or quote from another source, cite it in the text. Make it clear that it was not your own words.
Incremental plagiarism:
Copying parts of another person’s work, such as phrases, sentences, or paragraphs without crediting the source. When deciding which tools to use to check a paper for plagiarism , instructors often seek out those that will identify incremental forms as well as instances of direct copying and similar phrasing.
- Prevention : Decide to either directly quote the phrases or sentences you want to use, or write a good paraphrase. In both cases, be sure to add a citation. Using a plagiarism checker could also help you identify problematic passages.
Self-plagiarism:
Academic self-plagiarism occurs when a student submits the same paper or parts of a paper for more than one assignment. When your instructors are grading your papers, they’re assessing your research and writing skills in the present. When you submit work that you completed in the past, they are both unable to evaluate your current skills and unaware that you haven’t completed the assignment.
- Prevention: Write a new paper for each assignment you’re assigned. If you feel strongly that your past work could enhance your new paper, speak to your instructor and ask for permission first.
Misleading citations:
Including a citation for a quote or idea that misrepresents the source material. This can occur if a student does not understand the reference they are citing; if a student includes a citation for a disreputable source; or the source material simply does not align with the idea or argument that the student has attributed to it.
- Prevention: Carefully review your assignment to understand it. As you research, take the time to evaluate each source notes . Remember, it’s better to have quality citations over an abundance of citations.
Invented sources:
If a reference in a student’s bibliography is found not to exist, it is considered an invented source. This may occur if a student couldn’t find a reputable source to back their argument, or if they needed to include additional references to meet the requirements of the assignment and chose to take an unethical shortcut rather than completing the required amount of research. No matter the reason, this behavior ultimately hurts them in the long run. Not only will they fail to gain the experience they need to conduct research in the future properly, but they’ll also experience significant consequences if they’re caught.
- Prevention: Set aside time to do proper research so you can find enough sources. Start creating a list of sources as you’re researching and take good notes. This will help you keep track of your sources so none are forgotten. If you do end up forgetting where a quote came from, a paper checker could help you pinpoint the original source.
Patchwriting/Spinning:
Patchwriting is often confused with paraphrasing, but there’s a significant difference. When you paraphrase, you demonstrate that you understand the topic well enough to restate it in your own words. When you patchwrite or spin, it is more akin to a word-swapping game; there’s no need to understand the subject, merely to have access to a thesaurus so you can substitute enough synonyms to mask the source material. This can be intentional, but it may also be a result of having a poor understanding of how to paraphrase.
- Prevention: Using your own words, write down the key points of the phrase or idea, and put them together in a sentence. Be sure to include a citation as well.
A good way to test if you’re patchwriting or paraphrasing is to remove your sources from view. If you can write about what you’ve read without looking at the source material, you likely understand it well enough to paraphrase it. If you have to review the source material with each new sentence or consult a thesaurus while writing about it (except when you’re adding direct quotations), you may be spinning your sources instead of paraphrasing them.
Academic Integrity Policies and Statements
If you’re still uncertain about what counts as plagiarism, look for your school’s/ organization’s policies on academic integrity and plagiarism. The policies of academic institutions usually cover what is considered plagiarism, the consequences of committing it, and how to avoid it. One great example is Purdue University’s Academic Integrity statement .
What are the Consequences of Plagiarism?
No matter the setting, plagiarism is not taken lightly, and the consequences can be significant. For a good reason, too! Whether in an academic or professional setting, the plagiarism consequences reflect the seriousness of the act, which is ultimately a form of theft that hurts everyone involved.
Just as with the theft of a tangible object, there can be legal punishments for plagiarism. It is, after all, a form of copyright infringement in many instances. A quick search for plagiarism articles will reveal that professional instances of intellectual theft have resulted in civil lawsuits and can even be criminally prosecuted under rare circumstances. In addition to the possible legal consequences, professionals may lose their jobs or have to start over in a new field after their acts of fraud are uncovered.
As a student, you’re likely to wonder what happens if you plagiarize in college or high school. While there will almost always be consequences for this behavior, there is no one-size-fits-all plagiarism sentence. Depending on the circumstances, academic dishonesty could result in outcomes such as:
- You might get a zero for the assignment in which the infringement occurred.
- You may receive a failing grade for the class. If it is a required course, this could leave you without enough credits to move on to the next level until you can repeat it and, in some instances, postpone graduation.
- You may be expelled from your school or university.
The academic dishonesty may be noted on your transcript, which can lead to you not getting into your preferred college, graduate school, or Ph.D. program in the future.
Nobody wants to be known as a fraud or to have a reputation for dishonesty follow them through their career. And, given the consequences that can extend beyond just their reputation, it’s no wonder that professional and academic writers who wish to avoid them take the time to understand the complete definition of plagiarism and run their work through a plagiarism checker before sending it out into the world.
Even the vigilant can fall prey to inferior tools, unfortunately. Before selecting a plagiarism checker, you should understand how they work and what they can (and cannot) detect.
How We Check for Plagiarism
When exploring how to check for plagiarism, most students and professionals conclude that including a checking tool in their revision process is not only helpful but necessary. When you consider the Herculean task of checking each line of your paper against the text of each of your resources, the benefits of a checker are clear. Moreover, this manual approach would only alert you to matching text in the sources you’re aware of, after all, and leaves the sources you haven’t reviewed untouched.
But, hang on. Why would sources you haven’t reviewed factor into your review? The answer to this lies in the plagiarism definition you learned above. What is plagiarism? It’s presenting someone else’s work or ideas as your own, intentionally or otherwise. It is not uncommon to uncover an idea while you’re researching a topic and later misremember it as your own. This might even occur years after you originally came across it.
You might wonder: how can plagiarism be avoided if you have to account for the source of every thought you include in your work? A few exceptions minimize the scope of this. Common knowledge about your topic does not need to be supported by a citation, nor does knowledge that you gained through your personal experience. Using a subscription-based or free plagiarism checker will help you locate any passages that may fall into these categories so you can review them and decide for yourself whether a citation is needed.
EasyBib Plus writing tools provide easy, convenient, and reliable support to help you find potentially missed citations and can help you improve your paper into a high-quality paper with integrity. Simply upload your paper, select the checker, then sit back and relax as the site scans your document. In mere moments, we’ll search the web for passages of similar text and highlight duplicate content for your review.
Regardless of the tools you use to help you revise and polish your work, it’s ultimately your responsibility to ensure that you’re writing and submitting ethical work. That’s why our tools go beyond the basics and require your participation. The tool never automatically makes changes to your paper, but only flags sections that may need your attention and provides you with the matching source so you can to make an educated decision.
If you find that a citation is needed, our citation tools can help you create properly formatted citations and develop a complete bibliography. And, if you review the passage and determine that the match is coincidental, you can dismiss the alert and move on to the next.
Seamless Citation Creation
Professional writers and students alike can find creating citations incredibly confusing. Nevertheless, they are an integral part of a well-written and researched paper and a requirement in almost all academic settings. But, have you ever wondered why they are so important? Citations really do have a purpose—other than driving us crazy— that make it necessary always to cite our sources.
We cite our sources for a few important reasons:
We need to give credit where credit is due. When you use a quote from someone else’s work, you need to give credit for using their words and ideas. Research is often based on the works and ideas of others. However, to include the words and ideas of another without giving them proper credit is to plagiarize, even if the act was unintentional.
In addition, showing that you’ve done proper research by including in text & parenthetical citations and a comprehensive list of sources to back up your arguments gives your work a layer of credibility that can help you earn the trust of your reader or teacher.
Readers can find the sources you used. When you’re writing high school or undergraduate papers, your only readers may be your instructors and peers. However, including citations at every level of writing helps to ensure that anyone who reads your work can access your sources to check their accuracy, learn more about your topic, and further their own research.
Sources can take your research and learning to the next level, too. When you are conducting research on a topic, checking the works cited or annotated bibliographies prepared by your sources can lead you down new avenues in your research to further develop your ideas and help you write your papers.
Correctly utilizing citations gives strength to your thoughts and opinions. Understanding the value of citations does not, unfortunately, make them any easier to create. We know how confusing it can be, but don’t throw in the towel on learning how to cite websites in MLA , create your APA citation website references, or format your works cited —this is where we come in!
While a free online plagiarism detector may tell you how much of your work is unoriginal and may even identify the passages that meet their plagiarize definition, a premium EasyBib Plus plagiarism checker account also gives you access to our powerful citation resources and generator so you can:
Scan your papers with the plagiarism tool to check for plagiarism-free work before you submit.
Review flagged passages to determine if they meet the plagiarism definition and create unlimited citations in APA format and MLA format for anything fitting the plagiarism meaning. Need more styles ? Check out our regular citation generator for thousands of choices and free resources to help you learn how to create an APA parenthetical citation , APA book citation , APA works cited , and so much more!
Build a full bibliography for your paper right along with your parenthetical or in-text citations, which can save you hours of work along the way compared to manually creating and formatting them.
How Else Do We Help You Improve Your Paper?
The EasyBib Plus plagiarism detector helps you check your content or paper for text that may be missing a citation—which may fall within the definition of plagiarism—to help ensure you don’t accidentally plagiarize. It also includes grammar check and spelling check tools to help identify errors and suggest grammatical tweaks that could help to elevate the level of your writing.
Running a plagiarism check couldn’t be easier! Just upload your paper to the online proofreader, and in a few moments we’ll have your writing and citing suggestions. And, there’s no need to worry about your paper after it’s been scanned.
Run a Grammar and Spell Check for Free!
There’s no denying that your schoolwork can be challenging at times, and your assignments can lead to some late nights even when you’ve started them early. The last thing you want is to submit assignments that don’t reflect your best effort, but it can occasionally be quite the juggling act to get everything done on time.
Why not let our plagiarism checker free up space on your calendar by helping you revise your papers quickly and efficiently? The EasyBib Plus paper checker is your one-stop shop to check for plagiarism, create citations, spot spelling mistakes, and receive feedback on your grammar and style.
When you proofread and do a manual spelling check on work that you’ve written, you’re more likely to overlook mistakes. This is even more likely if you’re pressed for time or trying to complete several different assignments at once. There are tricks to help minimize this, such as reading your work aloud to identify poor grammar or reading each sentence backward to find spelling errors. When time is of the essence, though, these solutions aren’t the most convenient or useful options. That’s why many students seek the assistance of online tools that will run a grammar and plagiarism check on their work.
Are you asking yourself, “ How do I check my grammar online ?” We’ve got the perfect checker for you! Our advanced tools help you find and correct grammar, spelling, and punctuation mistakes. Our targeted feedback and free writing and citing resources help you learn as you go to improve your writing over time.
The best part? You can use our grammar and writing tools any time of the day, any day of the year for! Looking to try it? Just upload or copy and paste your text into our online proofreader for a free grammar check with up to 5 suggestions, or sign up for EasyBib Plus today! EasyBib Plus gives you use of the plagiarism checker and unlimited access to suggestions that can help improve your spelling, sentence structure, punctuation, writing style, and grammar.
Published February 3, 2019. Updated April 9, 2020.
Written and edited by Michele Kirschenbaum and Melissa DeVrieze Meyer . Michele is a school library media specialist and the in-house librarian at EasyBib. You can find her here on Twitter. Melissa is a professional writer and editor based in New Jersey. She writes for academic brands and independent publishers about writing, grammar, and literature, and creates study and curriculum materials for ESL learners. You can find her on Twitter .
Visit our EasyBib Twitter feed to discover more citing tips, fun grammar facts, and the latest product updates.
Advertisement
Supported by
Don’t Use A.I. to Cheat in School. It’s Better for Studying.
Generative A.I. tools can annotate long documents, make flashcards, and produce practice quizzes.
- Share full article

By Brian X. Chen
Hello! We’re back with another bonus edition of On Tech: A.I. , a pop-up newsletter that teaches you about artificial intelligence, how it works and how to use it.
Last week, I went over how to turn your chatbot into a life coach . Let’s now shift into an area where many have been experimenting with A.I. since last year: education.
Generative A.I.’s specialty is language — guessing which word comes next — and students quickly realized that they could use ChatGPT and other chatbots to write essays . That created an awkward situation in many classrooms. It turns out, it’s easy to get caught cheating with generative A.I. because it is prone to making stuff up , a phenomena known as “hallucinating.”
But generative A.I. can also be used as a study assistant. Some tools make highlights in long research papers and even answer questions about the material. Others can assemble study aids, like quizzes and flashcards.
One warning to keep in mind: When studying, it’s paramount that the information is correct, and to get the most accurate results, you should direct A.I. tools to focus on information from trusted sources rather than pull data from across the web. I’ll go over how to do that below.
First, let’s explore one of the most daunting studying tasks: reading and annotating long papers. Some A.I. tools, such as Humata.AI , Wordtune Read and various plug-ins inside ChatGPT, act as research assistants that will summarize documents for you.
We are having trouble retrieving the article content.
Please enable JavaScript in your browser settings.
Thank you for your patience while we verify access. If you are in Reader mode please exit and log into your Times account, or subscribe for all of The Times.
Thank you for your patience while we verify access.
Already a subscriber? Log in .
Want all of The Times? Subscribe .
A systematic review of research on cheating in online exams from 2010 to 2021
- Published: 07 March 2022
- Volume 27 , pages 8413–8460, ( 2022 )
Cite this article
- Fakhroddin Noorbehbahani 1 ,
- Azadeh Mohammadi 1 &
- Mohammad Aminazadeh 1
27k Accesses
84 Citations
20 Altmetric
Explore all metrics
In recent years, online learning has received more attention than ever before. One of the most challenging aspects of online education is the students' assessment since academic integrity could be violated due to various cheating behaviors in online examinations. Although a considerable number of literature reviews exist about online learning, there is no such review study to provide comprehensive insight into cheating motivations, cheating types, cheating detection, and cheating prevention in the online setting. The current study is a review of 58 publications about online cheating, published from January 2010 to February 2021. We present the categorization of the research and show topic trends in the field of online exam cheating. The study can be a valuable reference for educators and researchers working in the field of online learning to obtain a comprehensive view of cheating mitigation, detection, and prevention.
Similar content being viewed by others
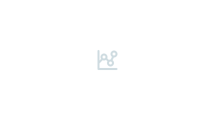
The temptation to cheat in online exams: moving beyond the binary discourse of cheating and not cheating
Online Academic Cheating in the Twenty-First Century
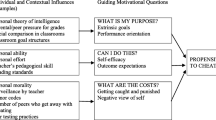
Exam cheating among Quebec’s preservice teachers: the influencing factors
Explore related subjects.
- Artificial Intelligence
- Digital Education and Educational Technology
Avoid common mistakes on your manuscript.
1 Introduction
Today, distance education has been transformed into online settings, and the COVID-19 pandemic has raised online learning significantly across the world. The COVID-19 enforced the closing of traditional learning all over the world, resulting in 1.5 billion students and 63 million educators shifting from face-to-face learning to online learning. This situation has revealed the strengths and weaknesses of the digital transformation of education (Valverde-Berrocoso et al., 2020 ).
In (Martin et al., 2020 ), it has been shown that the online learning publications are continuously being increased from 2009 to 2018, and one of the leading research themes is course assessment. Course assessment is very challenging in online learning due to the lack of direct control over students and educators.
For an educational institution, assessment integrity is essential because it affects institutional reputation. It is necessary to employ traditional cheating detection besides prevention methods and new digital monitoring and validation techniques to support assessment integrity in online exams (Fluck, 2019 ).
The study (Watson & Sottile, 2010 ) has reported that students are remarkably more likely to get answers from others during online exams or quizzes compared to live (face-to-face) ones. Therefore, preserving the integrity of online exams is more challenging. There are some strategies to mitigate online exam cheating, such as getting offline (face-to-face) proctored exam, developing cheat-resistant questions (e.g., using subjective measures instead of objective measures), and lessening the exam score percentage contributing to the overall course grade.
Traditional cheating methods include, hiding notes in a pencil case, behind ruler, or clothes, writing on arms/hands, leaving the room, etc. (Curran et al., 2011 ). Technological advances and online learning have enhanced education, however, they also have facilitated cheating in courses (Turner & Uludag, 2013 ). For instance, an examinee could use a mobile phone to text someone to get the answer. Although this would be difficult in the exam hall, some examinees could text without looking at the mobile phone. Applying scientific calculators, Mp3 players calculator, and wireless equipment such as an earphone and a microphone are other tools that facilitate cheating in offline exams (Curran et al., 2011 ).
Although cheating motivations in online and offline exams are not significantly different (Turner & Uludag, 2013 ), detecting and mitigating online cheating could be more intricate. This is because, in addition to traditional cheating methods that also could be exploited in online exam cheating, there exist various technologies and tools that could be applied for cheating in online exams more easily. For example, using remote desktop and share screen, searching for solutions on Internet, using social networks, etc.
Cheating in an online setting is more convenient than a traditional offline exam. Accordingly, detecting and preventing online cheating is critical for online assessment. Therefore, this issue is one of the biggest challenges that MOOC (Massive Open Online Courses) summative assessment faces.
Recent researches imply that a critical issue in online education is academic dishonesty and cheating. Today, paid services exist that impersonate students in online courses to ensure their identity. In recent years, proctoring technologies such as identity authentication, keystroke recognition, and webcam proctoring will be extended to secure online exams (Xiong & Suen, 2018 ). Apart from direct proctoring, there are some techniques such as controlling the browser, limiting exam time, randomizing questions and choices, etc. However, it seems cheating in online courses is pretty common (Dendir & Maxwell, 2020 ).
Although one of the most critical challenges in online learning is to mitigate and handle cheating, there is no comprehensive literature review and classification in this field. Hence, in this paper, we present a systematic mapping review of researches in online examination cheating. The research questions are as follows:
RQ1: What are the publication trends in online cheating?
RQ2: What are the main reasons for online cheating?
RQ3: What are the cheating types in online exams?
RQ4: How can online cheating be detected?
RQ5: How can online exam cheating be prevented?
The paper is structured as follows. In Section 2 , the research method is described, including study selection criteria, databases and search strategy, and study selection. Section 3 presents review results and provides the answers to research questions. Sections 4 and 5 discuss the results and conclude the paper, respectively.
The current study is a literature review about cheating in online exams. A literature review identifies, selects, and synthesizes primary research studies in order to provide a picture of the topic under investigation. According to (Page et al., 2021 ), a record is the title or abstract (or both) of a report indexed in a database or website, and a report is a document (in paper or electronic format) supplying information about a particular study. It could be a journal article, preprint, conference abstract, study register entry, clinical study report, dissertation, unpublished manuscript, government report, or any other document providing relevant information. The current literature search has been performed based on the well-established PRISMA principles (Page et al., 2021 ).
2.1 Inclusion and exclusion criteria
The main criteria for the articles considered in the current review are as follows.
Inclusion criteria:
Researches should be written in English.
Records should be retrieved utilizing the designed search query.
Studies should be published between January 2010 and February 2021.
In cases where several papers reported the same study, only the most recent ones were included (i.e., theses and papers extracted from theses, extended version of papers published in journals).
Exclusion criteria:
Papers merely related to methods applicable to traditional cheating types, detection, and prevention are eliminated.
Studies not related to research questions are ignored.
Articles only related to cyber-attacks to online exam systems are excluded.
Low-quality researches are discarded (i.e., studies published by non-reputable publishers without peer review, too short review time, and so on, studies with poor theoretical background, experimental evaluation, or structure).
2.2 Databases and search strategy
We applied a wide range of databases as our primary source, including Google Scholar, Web of Science, and Scopus. We also added the publications which had cited the extracted records. Records were searched using the following search terms for the title, keywords, and abstract sections.
(Cheat OR e-Cheating OR Fraud OR Dishonesty OR Anti-cheating OR Cheat-resistant OR Abnormal behavior OR Misconduct OR Integrity OR Plagiarism) AND
(Electronic OR Online OR Digital OR Virtual OR Cyber OR Academic) AND
(Exam OR e-Exam OR Course OR e-Course OR Assessment OR e-Assessment OR Test OR e-Test OR Environment OR e-Environment) AND
(Prevent OR Detect OR Mitigate OR Reduce OR Minimize OR Monitor OR Proctor OR Reason OR Motivation OR Type OR Deter OR Control).
2.3 Study selection
The search result included 289 records, 26 of which were duplicated, and so they were deleted. From 263 screened records, 54 records were excluded by examining either the title or the abstract. In the next step, 12 reports were eliminated because they were not retrieved because were not accessible. Furthermore, after full-text eligibility checking, 144 reports have been excluded according to the inclusion and exclusion criteria as mentioned earlier.
This resulted in 53 reports that along with 5 other reports (obtained from citation searching and assessed for eligibility), were finally selected for literature review about online cheating. The flow of information through different phases of the review is presented in the PRISMA flow diagram depicted in Fig. 1 .
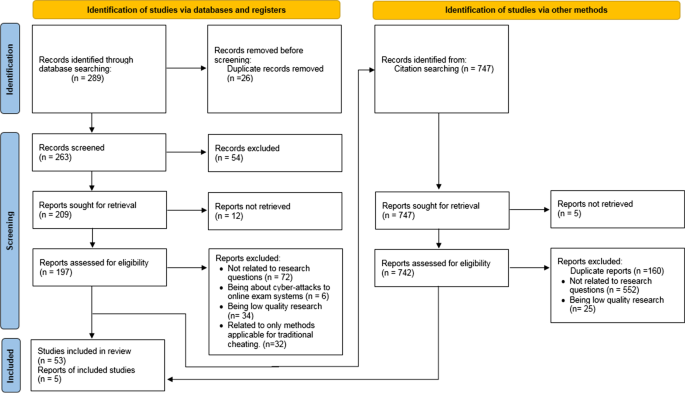
The PRISMA flow diagram
After selecting 58 studies, three domain experts were asked to assign a Credibility Score (CS) to each study. After evaluation of each study, experts agreed on a credibility score ranging from 0 to 5 based on the following criteria: publisher credibility, number of citations per year, theoretical and experimental quality, and organization and structure. CS statistics are as follows: mean = 3.81, SD =0.79, min = 2.5, max =5.
A summary of online cheating research papers and their study themes is presented in Table 1 . (Appendix 1 .)
Several findings emerged as a result of the research synthesis of the selected fifty-eight records on online cheating. The selected studies were categorized into four main topics, namely Cheating reasons, Cheating types, Cheating detection, and Cheating prevention, as shown in Fig. 2 . All subsequent classifications reported in this paper have been provided by the authors. The studies under every four main topics are investigated by three experts, and a list of items is extracted for each category. Notably, some studies were corresponded to multiple main topics. Next, several brainstorming sessions have been conducted to classify each main topic further. To extract the classifications, the XMind tool has been employed, which is a professional and popular mind mapping software.

Online cheating research classification
In the following sub-sections, the detailed analysis of the review results is described according to the five research questions we defined to drive the research.
3.1 Publication trends
In Fig. 3 , the number of publications per year is displayed (in this study, the final publication date is applied). In 2017, the greatest number of studies corresponding to the conducted review have been published. As shown in Fig. 4 , the dominant publication type is journal papers with 53% of the total publications. In terms of the average citations of the selected studies regarding their classes, the maximum average citations belong to the journal papers with an average citation of 19.65 (see Fig. 5 ).
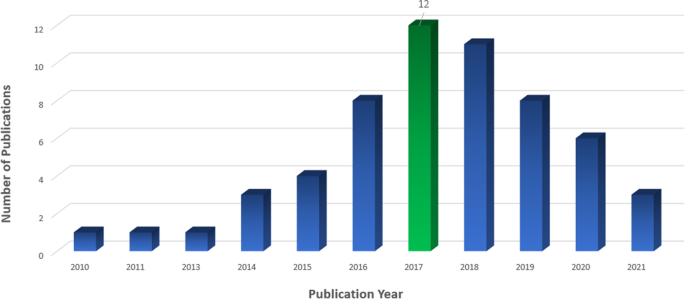
Number of publications per year
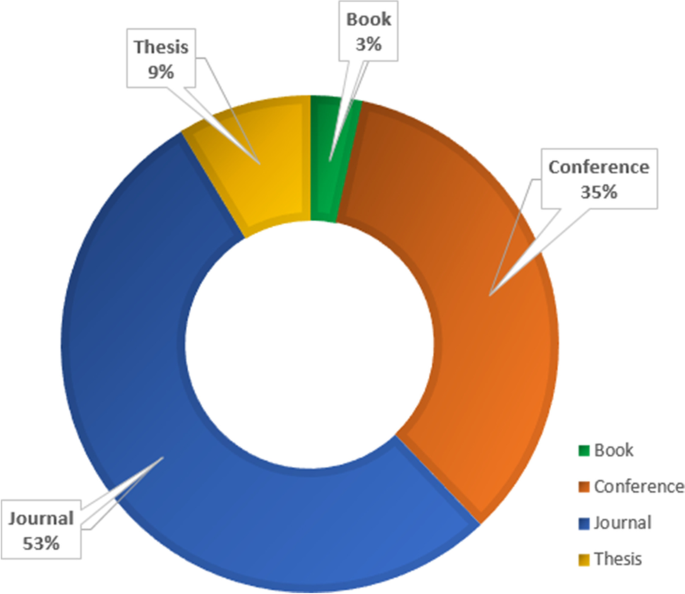
Distribution of publication per types
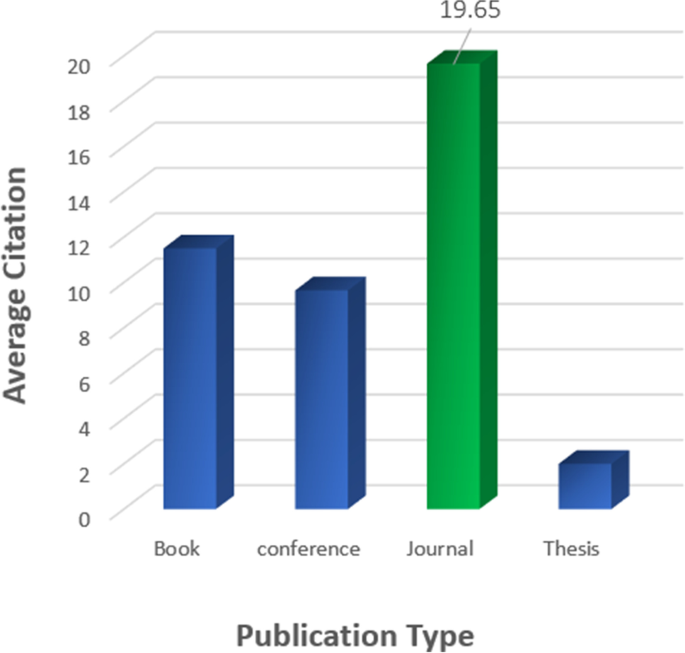
Average citation per publication type
There are 747 works cite the selected studies related to the review. As displayed in Fig. 6 , the greatest and lowest shares of the total citations pertain to the journal articles and the theses, respectively. The number of publications per research theme is shown in Fig. 7 . The cheating prevention and detection themes are the most prevalent research themes in online cheating. In the following four subsections, the studies under each of the four research themes are described and classified thoroughly.
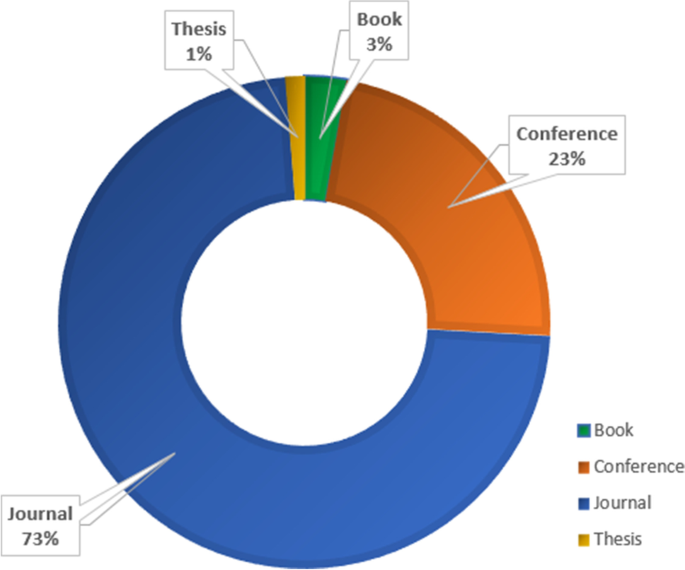
Distribution of publications according to citations
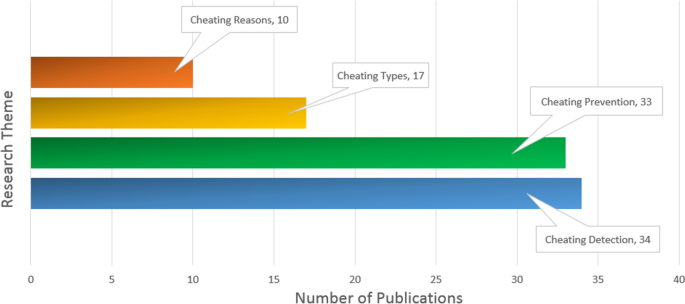
Number of publications per research theme
3.2 Cheating reasons
The primary reason for cheating is that examinees feel the rewards outweigh the risks (Lancaster & Clarke, 2017 ). There exists a wide variety of reasons why candidates decide to commit cheating, still, they could be categorized into four general reasons, namely Teacher-related, Institutional, Internal, and Environmental reasons. The complete classification of the cheating reasons is displayed in Fig. 8 , which is described in the following sections.
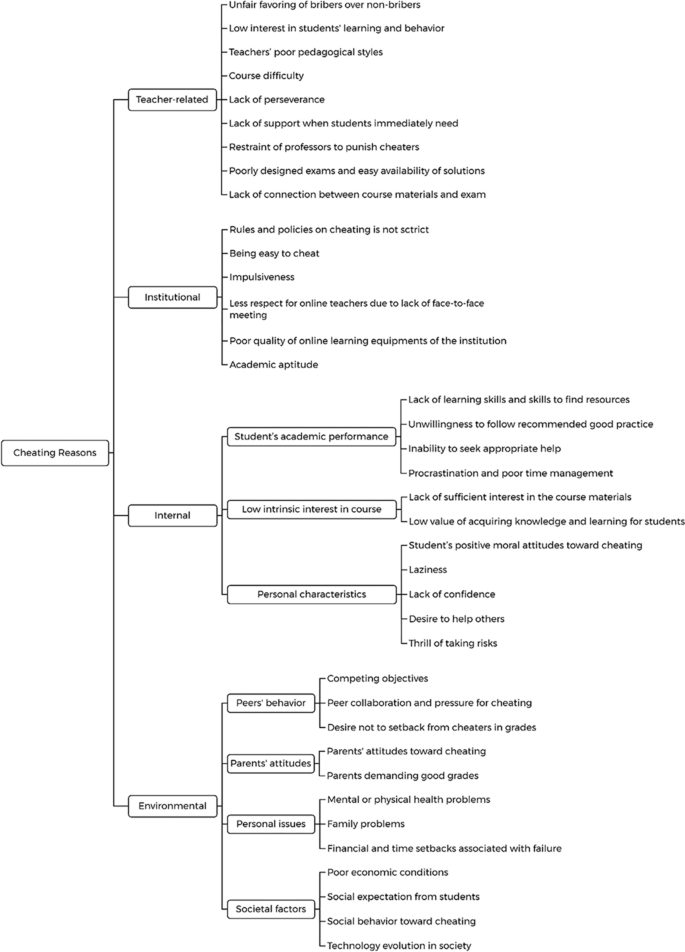
Cheating reasons
3.2.1 Teacher-related reasons
All the reasons related to the teacher or the course instructor are put into this category. Maeda ( 2019 ), has observed that the student’s relationship with the teacher has crucial influences on academic integrity. Teachers’ unethical behaviors, such as favoring those who have bribed over those who have not, or favoring the students who participated in private tutoring sessions, motivate the oppressed students to cheat. The author also found that teachers’ low interest in students’ depth of learning, which also results in a poor pedagogical style, could be an important reason that motivates students to participate in any kind of unethical behavior (Maeda, 2019 ).
Course difficulty could motivate the examinees to cheat. Some students blamed their teachers for complicated and complex course materials. In some specific cases, this reason could be a consequence of students’ lack of perseverance. They find cheating as a way to relieve these difficulties (Amigud & Lancaster, 2019 ).
As a result of distributed learning with online courses and examinations, Moten et al. ( 2013 ), have expressed that students feel isolated in an online environment. They often become frustrated when they do not get the help they immediately need, for instance, the night before an exam. This situation is closely dependent on the presence time of the teacher in online communication environments.
Some teachers restrain from punishing the cheaters appropriately due to ethical issues. This could be due to the sympathy of some teachers with cheaters. After listening to the cheater’s excuses and justifications, the teacher might give them a second chance. Sometimes, teachers are worried about the consequences of punishments and the corresponding pressures that cheaters experience, hence they don’t punish the cheater or the punishment is too mellow.
This increases the students’ courage to cheat during online exams due to decreased risk of being punished after being caught and implies that cheating penalties are insignificant over the long run (Topîrceanu, 2017 ).
Exam design is one of the most important contributing factors that motivates examinees to cheat in the exam. Weakly designed exams such as similar multiple-questions for every examinee or easy accessibility of solutions over the web, can make it easy to cheat. On the other hand, questions being too complex and irrelevant to course materials, forces students to commit cheating during online exams (Srikanth & Asmatulu, 2014 ).
3.2.2 Institutional reasons
In (Maeda, 2019 ), it is observed that the rules and policies of the institution are directly related to the number of unethical behaviors occurrences. It is found that institutions with stricter regulations and better commitment to strengthening academic integrity, face much less cheating behavior between their students. Institutional policies not only create an anti-cheating atmosphere, but also makes dishonest academic behaviors challenging to take place. Also, Backman ( 2019 ) emphasizes that if it becomes easy for students to cheat, they will cheat.
Impulsiveness is a crucial reason why students try to cheat during online examinations. They feel isolated and disconnected, so they may imagine they won’t get caught or the instructor does not care if they commit academic dishonesty. Unethical behaviors have a direct relationship with the student’s impulsiveness (Moten et al., 2013 ).
Moreover, in an isolated environment, due to the lack of face-to-face communications with teachers, students have much less respect for their teachers that leads to increasing misbehaviors. That is why teachers should personalize the online environment for students by calling their names or listening to their voices, so that online classes become more engaging and interactive for students (Moten et al., 2013 ).
Dobrovska ( 2017 ), expressed that the poor quality of the institution’s online learning system discourages students from learning the course materials, and makes it difficult for them to learn, hence, they are more motivated to cheat.
Academic aptitude is one of the most important and underrated reasons leading students to commit misbehaviors. It means educational institutions don’t discriminate between students and ignore their unique abilities, skills, and different levels of preparedness for a specific task. This makes unprepared students feel frustrated about that particular task or course, which leads them to seek help from more talented and prepared students in that specific context (Amigud & Lancaster, 2019 ).
3.2.3 Internal reasons
Another category of cheating reasons is internal motivators. The motivators over which the candidate has complete control, including intrinsic factors, personality and psychological characteristics, lie in this category. The internal reasons are divided into three subcategories as follows.
Student’s academic performance
One significant internal factor is the student’s academic performance. There are several reasons that could result in poor academic performance as follows: lack of learning and skills to find resources, students unwillingness to follow recommended practices, inability to seek appropriate help, procrastination, poor time management (Dobrovska, 2017 ), and lack of confidence in their ability to learn course materials (Norris, 2019 ).
Low intrinsic interest in the course materials
Low intrinsic interest in the course is another reason mentioned in (Dobrovska, 2017 ), which could be caused by a lack of sufficient interest in course materials and subjects or the mindset that these materials and knowledge are unnecessary and unimportant for future life (Norris, 2019 ).
Personal characteristics
There is a strong relationship between students’ moral attitudes toward cheating and their level of participation in academic misbehaviors (Maeda, 2019 ). Therefore, conscientious belief is considered as an internal reason stopping students from unethical behaviors. However, it has been shown that religious beliefs do not necessarily lower cheating behaviors (Srikanth & Asmatulu, 2014 ).
Other reasons included in studies are student’s laziness for sufficient home preparation before the exam (Dobrovska, 2017 ), competition with others and the desire to get ahead (Amigud & Lancaster, 2019 ), desire to help other peers (Moten et al., 2013 ) and the student’s thrill of taking risk (Hylton et al., 2016 ).
3.2.4 Environmental reasons
The reasons mentioned in this section highly depend on the atmosphere and type of environment a student is in, either during the online exam or beforehand in social media or communication with people. We put these reasons in four major categories: Peers’ behavior, Parents’ attitudes, Personal issues and, Social factors.
Peers’ behavior
Peers could influence individuals in a manner that their cheating motivations are increased. In an academic environment, however, it is primarily because of the competing objectives, such as the desire to get ahead in scores. This depends on the amount of competition in the academic environment (Amigud & Lancaster, 2019 ).
Experimental research among Cambodian students, has figured out that being among a group of cheaters, psychologically drives the students to repeat their peers’ actions and commit cheating. In addition, there is high pressure on those who do not collaborate with peers, or reject participating in their group work. It is found that they are blamed for being odd and unkind (Maeda, 2019 ).
According to (Srikanth & Asmatulu, 2014 ), being in an environment where peers’ cheating remains undetected, gives this kind of feeling to non-cheaters that they are setting back in scores and are unfairly disadvantaged compared to those cheaters.
Parents’ attitude
Parents’ acceptance of cheating behaviors, massively affects the student’s mindset toward these behaviors. As expressed in (Maeda, 2019 ), parents’ behaviors toward their child’s cheating, vary from complete unacceptance to active involvement and support. Another reason related to parents’ attitudes is putting their children under pressure to achieve good or higher than average grades (Backman, 2019 ).
Personal issues
Personal issues could be mental and physical health problems (Amigud & Lancaster, 2019 ), problems within the family (e.g., parents arguing, separation and divorce, etc.), and fear of failure in exams and its further consequences like financial and time setbacks (Hylton et al., 2016 ).
Societal factors
Poor economic conditions and the development level of a country are examples of societal factors affecting students’ motivation to cheat and achieve academic success (Maeda, 2019 ).
Countries with various cultures, social expectancies, and people’s attitudes have different behaviors regarding academic performance. In some countries, academic performance and grades are known to be crucial for success in life, whereas, in other countries, academic performance is relatively low valued. This range of different expectations from students leads to various social beliefs and behaviors toward cheating (Maeda, 2019 ). In research presented in (Holden et al., 2020 ), it is shown that a primary reason could be the existence of a cheating culture. Some students may cheat because they desire to portray a better image of themselves to their society (Norris, 2019 ). Another societal factor influencing cheating behaviors is the technology evolution that strengthens cheating motivation (Maeda, 2019 ). This is because technology brings about increased access to cheating resources. The evolution of technology, specifically search engines and social media, makes it easier for students to cheat.
3.3 Cheating types and facilitators
To mitigate cheating behaviors effectively and efficiently, cheating methodologies, types, and facilitators should be known. Cheating is performed either individually or by the cooperation of others (called group cheating). Figure 9 displays the complete classification of cheating types.
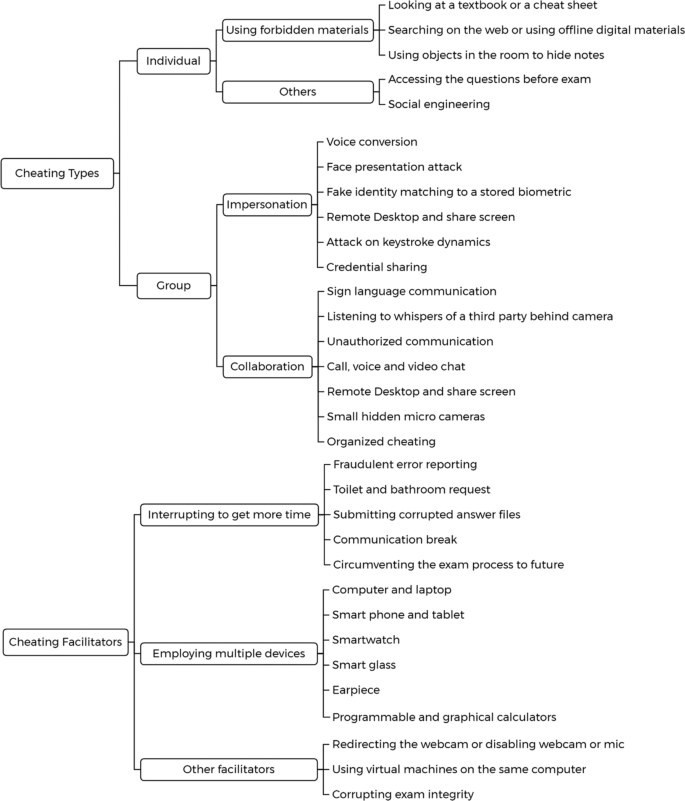
- Cheating types
3.3.1 Individual cheating
Individual cheating is carried out without any assistance from any person. This type of cheating could be categorized as using forbidden materials and other types are described as follows.
Using forbidden materials
Individual cheating can occur by using forbidden materials during the exam, such as looking at a textbook or a cheat sheet (Fontaine et al., 2020 ), (Holden et al., 2020 ), searching the web, using offline electronic resources such as images, voices, etc. (Korman, 2010 ), (Holden et al., 2020 ), or even using objects in the exam room to hide notes.
Other types
Other types of individual cheating include accessing the questions and solutions before the exam, which Korman ( 2010 ) refers to as “unauthorized intelligence”. Another dishonest behavior is social engineering, which is grade negotiation with the teacher through fake facts and exploiting personal sympathy.
3.3.2 Group cheating
Cheating methods through cooperation with others could be categorized as Impersonation, and Collaboration types.
Impersonation
Impersonation means employing someone to take the exam for the examinee, either the whole exam or some parts of it (Korman, 2010 ), (Holden et al., 2020 ). It can occur in forms of voice conversion, face presentation attack and face impersonation, fake identity matching to a stored biometric, and attack on the keystroke dynamics (Chirumamilla & Sindre, 2019 ). These are attacks on the biometric system to bypass the authentication mechanisms. The other impersonation techniques include remote desktop control by a third party (Kasliwal, 2015 ), (Gruenigen et al., 2018 ), sharing the screen with a third party (Gruenigen et al., 2018 ), (Bawarith, 2017 ), and credential sharing, which is impersonation via shared username and password of an academic account or LMS (Learning Management System) (Dobrovska, 2017 ).
Collaboration
Collaboration is defined as getting any kind of help from others to answer the exam questions. It could be in the form of sign language communications that come in numerous forms, such as foot-tapping, pencil or any object dropping during the proctored exam, abnormal coughing, or suspicious actions (Srikanth & Asmatulu, 2014 ).
Listening to a third party’s whispers behind the camera (Chirumamilla & Sindre, 2019 ), any type of communication which is unauthorized such as sending or receiving messages, or voice and video calls (Korman, 2010 ), are also considered as collaborative cheating.
Other cheating methods in this category are remote desktop control (Kasliwal, 2015 ) and sharing the screen with others to collaborate with others about questions (Gruenigen et al., 2018 ), applying small hidden micro cameras to capture images and record videos for sharing with other peers (Bawarith, 2017 ), and finally, organizational cheating which is a result of institution’s personnel corruption (Korman, 2010 ).
The last one, as Korman ( 2010 ) showed, can take place when personnel help candidates to cheat. Changing the exam grade or exam answers after the exam (exam integrity corruption), giving the solutions to the candidate during the exam, or just bribing the proctor not to report the cheating or not to punish after being caught (Kigwana & Venter, 2016 ) are instances of organized cheating.
Contract work is a type of collaboration that means doing work with the help of someone else under the obligations of a contract. Contract workers may provide some or all of the exam answers. In this case, sometimes impersonating the student through the whole academic course is reported (Chirumamilla & Sindre, 2019 ).
3.3.3 Cheating facilitators
Methods discussed here act as cheating facilitators to support the process of cheating. In other words, these facilitators can be applied to perform any kind of cheating. A study presented in (Peytcheva-Forsyth et al., 2018 ), indicates that technology in general, is the leading facilitator of cheating practices. Cheating facilitators are classified as shown in Fig. 10 .
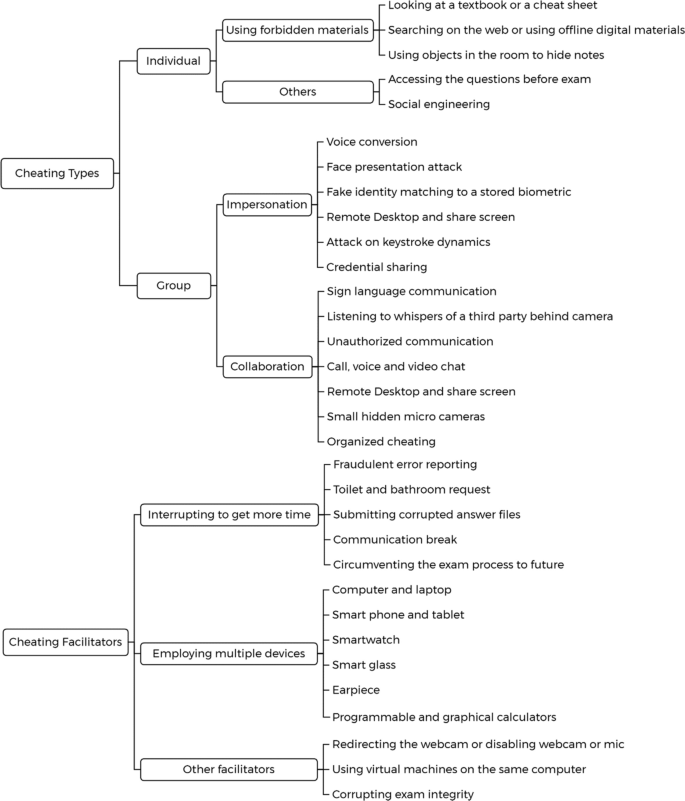
Cheating facilitators
Three different methodologies are used by students to facilitate cheating, either individually or in a group, described as follows.
Interrupting to get more time
Sometimes examinees try to buy more time to work more on the exam answers. For instance, the examinee may report an error about the exam system or exam proctoring software to convince the teacher to restart the exam session. This enables the candidate to get more time for cheating and finding the solutions during this interval when the session is closed (Motenet al., 2013 ). Another interruption method is to submit corrupted answer files by the candidate. In this case, the teacher reports that the files were corrupted and asks the candidate to resubmit the answer files. Most of the time, during the first submission and the second one, there exists at least one day, which implies the candidate gets at least one more day to answer the exam questions (Moten et al., 2013 ).
Other more classical methods to interrupt are toilet requests during the exam (Chirumamilla & Sindre, 2019 ), communication break and delay in answering oral exam right after a question is asked (Chirumamilla & Sindre, 2019 ), circumventing the exam process at a specific time with different excuses, and postponing taking the exam (Fontaine et al., 2020 ), (Korman, 2010 ). By deferring taking the exam, students can buy more time to become more prepared, either by studying more, or getting access to the exam questions and solutions.
Employing multiple devices
In proctored exams, either by a camera or software, students try to use multiple devices and answer the questions with the primary one while cheating via the secondary device. Several types of devices could be employed as the second device, such as computers and laptops (Moten et al., 2013 ), smartwatches (Wong et al., 2017 ), smart glasses such as Google glasses (Srikanth & Asmatulu, 2014 ), smartphones and tablets (Korman, 2010 ), programmable and graphical calculators to store notes and formulas (Kigwana & Venter, 2016 ), and tiny earpieces for remote voice support during the exam (Bawarith, 2017 ).
Other facilitators
Redirecting the webcam to hide something from its field of view (Sabbah, 2017 ), (Srikanth & Asmatulu, 2014 ), or disabling the webcam or microphone completely (Srikanth & Asmatulu, 2014 ) are other tricks used to facilitate cheating.
By using virtual machines on a computer, the user can run a virtual operating system on the primary one. This technique would hide the activities done on the second operating system from the software or the human proctoring the primary operating system. (Kasliwal, 2015 ).
Corrupting the exam system’s integrity to change the exam results after being held (e.g., changing the scores or answers after the examination) is another notable case (Korman, 2010 ). Lastly, in (Parks et al., 2018 ), the authors have investigated that social media and channels operating on them could act as cheating facilitation environments.
3.4 Cheating detection
Cheating detection methods can be categorized into during the exam and after the exam detection methods. Further classification of the cheating detection methods is presented in Fig. 11 .
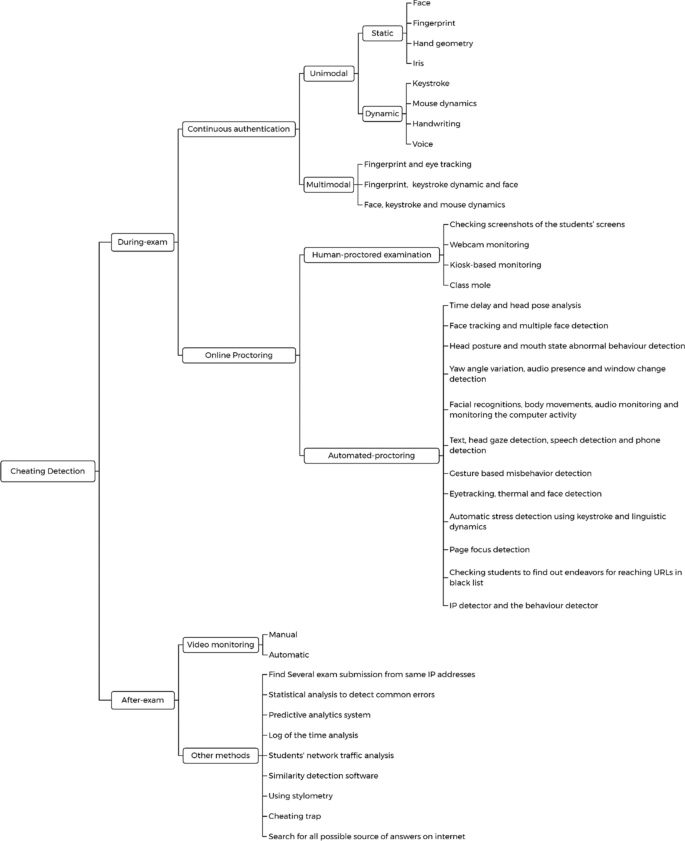
- Cheating detection
3.4.1 Cheating detection during the exam
To ensure academic integrity in online examinations, it is essential to detect cheating during the exam. Cheating detection can be partitioned into two main categories, namely, continuous authentication and online proctoring. Continuous authentication methods verify the identity of test-takers, and online proctoring monitors the examinees to detect any misbehavior during the exam. In the following, we will mention different techniques in each category.
Continuous authentication
One of the main types of cheating is impersonating. Therefore, it is essential to authenticate students before exam registration and prevent unauthorized candidates from taking the examination. In addition, it is necessary to validate the identity of the test-taker during the exam continuously. The continuous authentication systems are mainly based on biometric or behaviometric modalities and can be categorized into unimodal and multimodal schemes.
Unimodal authentication is the automatic recognition and identification of candidates using a unique characteristic. This characteristic could be either static (physiological) such as the face, fingerprint, hand geometry, and iris, or could be dynamic (behavioral) such as voice, handwriting, keystroke, and mouse dynamics (Chirumamilla & Sindre, 2019 ).
As a unimodal authentication system, Arnautovski ( 2019 ) designed a face recognition system, which captures the image of the test-taker at random time intervals. The facial recognition module continuously verifies the examinee’s identity by comparing captured images to the image from the exam registration process. In (Aisyah et al., 2018 ), an Android-based online exam application is implemented that takes photos of the examinee with random intervals and a web-based application lets the admin or supervisor of examination validate pictures of participants. In addition, Idemudia et al. ( 2016 ) proposed a system that tracks and detects faces continuously to verify the candidates. If the authentication failure remains for more than a few seconds, the system will stop the examination.
In (Sabbah, 2017 ), a scheme called ISEEU is proposed, in which each examinee’s session is streamed using a webcam. A proctor monitors the video screens and can generate alerts when any suspicious action is detected. He et al. ( 2018 ) proposed an anti-ghostwriter system using face recognition methods. The ghostwriter merges the student’s photo and their photo to make a fake one, or they change their appearance to mislead the examiners. The experimental results in (He et al., 2018 ), indicate that the proposed framework can detect ghostwriters with an acceptable level of accuracy.
Since some candidates may refuse to use a camera due to privacy concerns, Bilen et al. (2020) suggested that instructors offer their students two options. An examinee can agree to use a camera during the exam. In this situation, the record will be used as evidence if they are accused of cheating. However, if the examinee doesn’t accept using a camera, the instructor can claim cheating without providing evidence to the student.
In (Bawarith, 2017 ), the system authenticates the examinees continuously through an eye tracker. The data obtained from the eye tracker are translated into a set of pixel coordinates so that the presence or absence of eyes in different screen areas can be investigated.
Multimodal biometric authentication systems utilize different biometric or behaviometric traits simultaneously, which makes impersonating more difficult. In this regard, Bawarith et al. ( 2017 ) proposed a system that utilizes fingerprint and eye-tracking for authentication. The eye tribe tracker is used to continuously ensure that test-takers are the ones they are claiming to be. Whenever the system detects the examinee is no longer present in front of the screen, the system is locked, and the test-taker must be authenticated again via fingerprint.
In (Sabbah, 2017 ), a multimodal scheme called SABBAH is proposed, which adds continuous fingerprint and keystroke dynamics to the ISEEU scheme (Sabbah, 2017 ). In contrast to ISEEU, SABBAH uses an automatic system to detect fingerprint, keystroke, or video violations. Traore et al. ( 2017 ) proposed a system that continuously authenticates examinees using three complementary biometric technologies, i.e., face, keystroke, and mouse dynamics. In this system, test-takers are continuously authenticated in the background during the exam, and alarms are created and sent to the instructor through the proctoring panel.
Online proctoring
Online proctoring is essential to promote academic integrity. Alessio et al. ( 2017 ) reported significant grade disparities in proctored versus un-proctored online exams. Online proctoring can be categorized into human and automated proctoring. In human proctoring, a human proctor monitors the students remotely to detect suspicious behavior. In contrast, in automated proctoring, the cheating behaviors are flagged or detected automatically by the proctoring system.
Recently, several technologies have been developed to facilitate proctoring online exams remotely. For example, Kryterion™ Live Video Monitoring and ProctorU allow users to be monitored by a human proctor via a webcam during examination (Hylton et al., 2016 ). In (Reisenwitz, 2020 ), substantial support for online proctoring is provided. The results show a significant difference between the scores of exams that were not proctored and those proctored using ProctorU software.
Some systems can capture screenshots of the candidates’ PCs at random times during the examination (Migut et al., 2018 ). Consequently, if examinees use any forbidden resource on their computer, it will be shown to the proctor. Alessio ( 2018 ) applied video proctoring via a webcam at Miami University. The results demonstrate that students are less likely to cheat when monitored with a webcam during online testing.
In another study, kiosk-based remote online proctored examinations are compared with tests administered under a traditional proctoring environment. In kiosk-based proctoring, the test is taken on special computer kiosks located at accessible places such as libraries. The kiosks are equipped with enhanced webcams and are supervised online by a live remote proctor. The results indicated that examinees’ scores obtained under online kiosk-based proctoring are comparable to examinations taken in test centers with onsite proctors (Weiner & Hurtz, 2017 ).
A different approach for cheating detection is a class mole that means the instructor enrolls in students’ groups under another name as a mole to detect and combat collusion. In this way, they can discover dishonest students when they discuss cheating amongst themselves (Moten et al., 2013 ).
Human proctoring is costly and labor-intensive. Therefore, different automated proctoring systems are proposed to monitor the students during the examination and detect unauthorized behavior. In the following, we discuss several automated methods.
Chuang et al. proposed a semi-automatic proctoring system that employs two factors, namely, time delay in answering the questions and head-pose variation, to detect suspicious behavior. Afterward, a human proctor could use more evidence to decide whether a student has cheated (Chuang et al., 2017 ).
Garg et al. ( 2020 ) proposed a system to detect the candidate’s face using Haar Cascade Classifier and deep learning. If the examinee’s face moves out of the examination frame or multiple faces are detected in the frame, the test will automatically be terminated, and the administrator will receive a notification. In (Fayyoumi & Zarrad, 2014 ), a two-second candidate video is taken during the examination period. The images in the video are analyzed to verify whether the examinee is looking somewhere other than their screen. If the test-taker doesn’t focus on their screen, it may indicate cheating behaviors such as looking at an adjacent PC or reading from an external source.
In (Hu et al., 2018 ), the proposed system uses a webcam to monitor candidates' head posture and mouth state to detect abnormal behavior. Through the rule-based reasoning method, the system can detect suspicious behavior such as turning heads and speaking during the online examination.
Prathish et al. ( 2016 ), developed a multimodal system for online proctoring. The system captures audios and videos of the candidates as well as their active windows. If yaw angle variations, audio presence, or window changes are detected in any time frame, it can be considered an indicator of cheating. Consequently, the captured video, audio, and system usage are fed into a rule-based inference system to detect the possibilities of misbehaviors. ProctorTrack is another automated online exam proctoring product that employs facial and audio recognition, body movements, and computer activity monitoring to detect any suspicious action during examination (Norris, 2019 ).
Atoum et al., ( 2017 ) developed a system that can detect a wide variety of cheating behaviors during an online exam using a webcam, wearcam, and microphone. Using wearcam makes it possible to monitor what the student observes. It helps to detect any phone or text in the testing room that is prohibited. In addition, by using the wearcam, the system can detect another form of cheating that is reading from books, notes, etc. Furthermore, the system can estimate the head gaze of the test-taker by combining the information from the webcam and wearcam. Another form of cheating is getting verbal assistance from another person in the same room, or remotely via a phone call. The system can detect this kind of cheating using the microphone and speech detection. Considering the mentioned aspects, the proposed multimedia system can perform automatic online exam proctoring.
Saba et al. ( 2021 ), developed an automatic exam activity recognition system, which monitors the body movements of the students through surveillance cameras and classifies activities into six categories using a deep learning approach. The action categories are normal performing, looking back, watching towards the front, passing gestures to other fellows, watching towards left or right, and other suspicious actions. Movement recognition based on video images is highly dependent on the quality of images. Therefore, Fan et al. ( 2016 ), employed a Microsoft Kinect device to capture the examinee’s gesture. The duration and frequency of the detected action events are then used to distinguish the misbehavior from the normal behavior.
The system presented in (Mengash, 2019 ) includes a thermal detector attached with a surveillance camera and an eye movement tracker. When examinees intend to cheat, their body will emit a specific range of heat, and the emitted heat will trigger the camera to focus and detect the candidate’s face. Then the eye tracker detects eye movements, and the system detects the cheating intentions of the test-taker. There are other biometric-based methods for cheating detection. For example, keystroke and linguistic dynamics can detect stress, which indicates suspicious behavior (Korman, 2010 ).
Diedenhofen and Musch ( 2017 ), developed a JavaScript application called PageFocus, which can be added to the test page and run in the background. Whenever the examinee switches to a page other than the test page, a defocusing event is registered. The script captures when and how frequently defocusing and refocusing events occur on the test page. Another method is to permit students to get to just a couple of sites that are whitelist. If the examinee tries to open a site that is not allowed (one from blacklist), the instructor will be informed through an Android application or Internet (Kasliwal, 2015 ).
Tiong and Lee ( 2021 ), proposed an e-cheating intelligent agent composed of two modules, namely the internet protocol (IP) detector and the behavior detector. The first module could monitor the examinees’ IP addresses and enable the system to alert if a student changes their device or location. The second module detects abnormal behavior based on the speed of answering questions. Another method for cheating detection is comparing the IP addresses of the examinees to check whether two participants are in the same place (Bawarith, 2017 ).
3.4.2 Cheating detection after the exam
Even though different methods are employed to prevent students from cheating, some will still cheat during the examination. Consequently, a bunch of techniques is proposed to detect cheating students after the exam. This way, the reliability of online assessments will be improved. In the following, we will discuss different methods of cheating detection after the exam.
Video monitoring
The University of Amsterdam has developed a system that records the student’s video screen and the environment during the exam. Later a human proctor views the recording and flags and reports any suspicious behavior (Norris, 2019 ). Proctoring software proposed in (Alessio et al., 2017 ), records everything students do during the examination. After the exam, the recordings can be reviewed by the professor, teaching assistants, or employees of the proctoring vendor to identify cheating behaviors.
Human proctoring is a tedious and time-consuming process. To reduce the time and cost of proctoring, an automatic system can be employed to detect and flag suspicious events using machine learning methods. In this regard, Cote et al. ( 2016 ) proposed a system for the automatic creation of video summaries of online exams. The proposed method employs head pose estimations to model a normal and abnormal examinee’s behavior. Afterward, a video summary is created from sequences of detected abnormal behavior. The video summaries can assist remote proctors in detecting cheating after the exam.
Jalali and Noorbehbahani ( 2017 ), implemented an automatic method for cheating detection using a webcam. During the exam, images are recorded every 30 seconds by a webcam for each candidate. After the exam, the recorded images are compared with reference images of that student. If the difference exceeds a threshold, the image will be labeled as a cheating state.
Li et al. ( 2015 ), proposed a Massive Open Online Proctoring framework that consists of three components. First, the Automatic Cheating Detector (ACD) module uses webcam video to monitor students, and automatically flag suspected cheating behavior. Then, ambiguous cases are sent to the Peer Cheating Detector (PCD) module, which asks students to review videos of their peers. Finally, the list of suspicious cheating behaviors is forwarded to the Final Review Committee (FRC) to make the final decision.
Other methods
There are various ways of cheating, and therefore, different methods are used to detect cheating after the exam. For example, one of the cheating behaviors is to collude and work on tests together. However, most learning management systems allow the instructor to view IP addresses. Therefore, if different students submit their assessments by the same IP address in a short time frame, it could be detected and considered as a sign of collusion (Moten et al., 2013 ).
In addition, statistical methods can be used to analyze student responses to assessments and detect common errors and the similarities of answers (Korman, 2010 ). Mott ( 2010 ) stated that the distribution of identical incorrect responses between examinee pairs is a Polya distribution. The degree of cheating for each examination will follow the skewness or third central moment of the distribution.
Predictive analytics systems implicitly collect data while the students interact with the virtual learning environment. The collected data, which include student’s location, access patterns, learning progress, device characteristics, and performance, is used to predict trends and patterns of student behavior. Consequently, any unusual pattern may indicate suspicious behavior (Norris, 2019 ). Answering an examination takes a reasonable amount of time. Therefore, another indicator of dishonest behavior is an extremely short interval between the access time and the completion of the assessments, which can be detected by log time analysis (Moten et al., 2013 ).
In (Bawarith et al., 2017 ), an E-exam management system is proposed that classifies participants as cheating or non-cheating based on two parameters, namely the total time and the number of times the examinee is out of the screen. The focus of the test-taker is recorded using an eye tracker during the exam.
Kasliwal (Kasliwal, 2015 ), designed an online examination tool that captures the network traffic during the exam using a kismet server. The captured package can then be analyzed to determine the frequency of URLs accessed by students. If one of the URLs is getting accessed more frequently or very rarely, it could be considered suspicious.
To detect plagiarism in papers or essay-type questions, platforms such as DupliChecker.com Footnote 1 or Turnitin.com Footnote 2 can be used. These websites compute a similarity index and show all potential plagiarisms. Based on the similarity index, the instructor decides about further actions (Moten et al., 2013 ).
A weakness of similarity detection software is that it computes the resemblance of a submitted assessment with others' works and cannot detect an original text written by others for the student in question. Stylometry discovers this issue by checking the consistency of the delivered contents with other texts written by the same student. If the style of a text does not match with the previous works of that student, it may indicate complicity (Chirumamilla & Sindre, 2019 ). Opgen-Rhein et al. ( 2018 ) presented an application that employs machine learning methods to learn the programming styles of students. This work is based on the assumption that the programming style of each student is unique, and therefore, the model can be used to verify the author of assignments.
Another way of cheating detection is using a cheating trap, which means creating websites that could be found when the students search for answers. The solutions in trap websites are incorrect, and consequently, dishonest students could be detected (Korman, 2010 ). However, this method contradicts professional ethics.
In addition, the teacher can search the internet by hand periodically and try to find all possible web pages that provide solutions matching the exam questions. This approach could be applied to create a pool of potential solutions from the internet that will be used for plagiarism detection purposes after the exam (Norris, 2019 ).
3.5 Cheating prevention
After discussing and analyzing the examinees’ motivations for cheating and the reasons which directly or indirectly drive them to commit unethical actions during online examinations, a great deal of concern is gathered around how to decrease cheating in online exams and lower the probability of these actions taking place.
We categorized cheating prevention into two major types, namely, before-exam prevention and during-exam prevention. Figure 12 displays the classification of the cheating prevention methods.
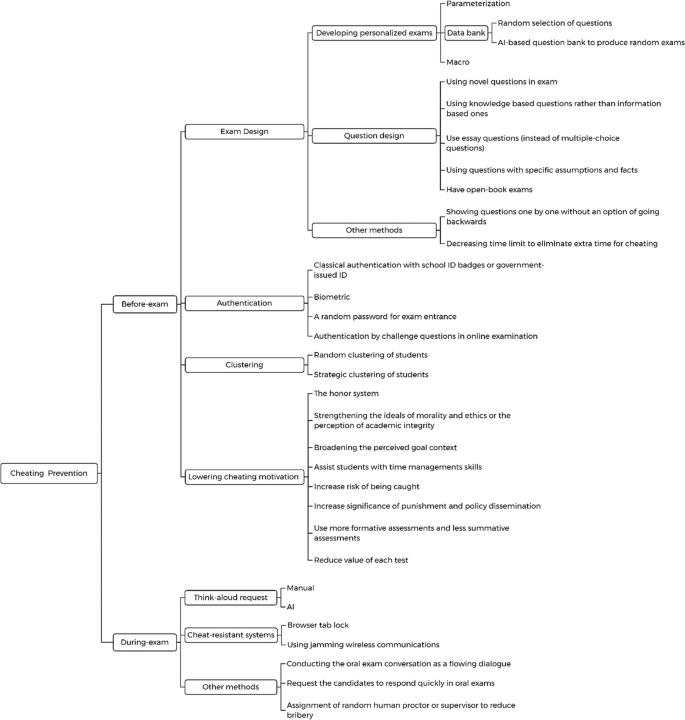
- Cheating prevention
3.5.1 Before-exam prevention
To prevent examinees from cheating, there exist several methods that should be implemented before the exam is held. Each will be discussed in detail as follows.
Exam design
In any situation that prevention is concerned, a proven and low-cost approach is a “cheat-resistant” design -A design that inherently prevents some specific cheating types from happening. This is why exam design is so critical. A cheat-resistant exam design, by its nature, prevents a range of possible forms of cheatings from occurring.
One way of achieving a good design is developing personalized exams for each candidate separately. There are several ways to do so, such as parameterization (Manoharan, 2019 ), which is a set of fixed questions with variable assumption values, using data banks with a large pool of questions to select questions randomly (Manoharan, 2019 ), (Norris, 2019 ) or implementing an AI-based method to produce unique exams (Chua & Lumapas, 2019 ).
Li et al. ( 2020 ) has put effort into designing a method for randomizing the question orders for each candidate. Their general idea is to show the questions one by one, and besides that, each student gets a different question at a time. This research mathematically proves that examinees cannot get much cheating gain.
In (Manoharan, 2019 ), the author has investigated an approach to personalizing multiple-choice examinations using the macro. Macro is a computer program fragment that stores data. It has a set of particular inputs for generating random exams based on a question bank. This method could bring freedom and flexibility to the exam design, but it needs basic programming skills.
Another aspect of exam design concentrates specifically on question design. Some of the most valuable methods are listed below.
Using novel questions: This type of question design is so unique in design and phrasing that it becomes very challenging to be plagiarized even with searching the web (Nguyen et al., 2020 ).
Using knowledge-based questions instead of information-based questions: These questions challenge the level of knowledge. The answers are not on the web or in reference books, and they need critical thinking and reasoning (Nguyen et al., 2020 ).
Using essay questions rather than multiple-choice questions: During an online exam, multiple-choice questions are highly susceptible to cheating. Hence, long essay questions are preferred (Varble, 2014 ).
Using questions with specific assumptions and facts: Although giving extra and not useful facts may mislead any candidate, even those taking the exam honestly, it will reduce the possibility of web-based plagiarism considerably by making it less straightforward to search online (Nguyen et al., 2020 ).
Having an open-book exam: Open-book exam questions should test students’ understanding, critical reasoning, and analytical skills. Since the answers to these questions are not found in any sources directly, open-book exams may reduce the cheating opportunity (Varble, 2014 ), (Backman, 2019 ).
Finally, other methods not placed into the above categories are mentioned below.
Showing questions one by one without the option of going backward is effective in cheating prevention. If it is employed besides strict time limitations and random question series, collaborative cheating will become quite challenging (Chirumamilla & Sindre, 2019 ), (Backman, 2019 ). By setting strict time limitations, the students do not have enough time to handle cheating, therefore, exam cheating efforts are reduced (Backman, 2019 ).
Cluskey et al. ( 2011 ), emphasize low-cost approaches for addressing online exam cheating. They introduce online exam control procedures (OECP) to achieve this target. Taking the exam only at a defined time and avoiding postponing it for any reason, or changing at least one-third of the questions in the next exam, are some instances of these procedures.
Authentication
Authentication is mainly for impersonation prevention before examinations. It could be done classically by checking the school ID badges or government-issued ID by the webcam (Moten et al., 2013 ) or by a more modern approach like biometrics through fingerprint, palm vein scan (Korman, 2010 ), eye vein scan (Kigwana & Venter, 2016 ), voice, and keystroke biometrics (Norris, 2019 ).
An interesting method to prevent cheating has been presented in (Moten et al., 2013 ). Students should call the instructor at a predetermined time to get the password. After the students’ voices are recognized by the instructor, they are authenticated and receive a random password for exam entrance. The password is valid until the end of the exam time limit, thus this method makes cheating more difficult (Moten et al., 2013 ).
The last method of authentication is the one discussed in (Norris, 2019 ) which uses challenge questions. These are the questions only the student will know, for instance, student ID or personal information. In (Ullah, 2016 ), an approach is proposed that creates and consolidates a student’s profile during the learning process. This information is collected in the form of questions and answers. The questions are pre-defined or extracted from a student’s learning activities. A subset of questions is used for authentication, and the students should answer these questions correctly to get access to the online examination. This approach ensures that the person taking the exam is the same one who has completed the course.
Clustering means partitioning students into several groups based on a predefined similarity measure. In (Topîrceanu, 2017 ), random and strategic clustering methods are proposed to break friendships during the exam, as cheating prevention techniques. The advantages of random clustering are time and cost efficiencies; however, it is imprecise, and some clusters may include unbroken friendships.
Breaking friendships through clustering relies on two hypotheses (Topîrceanu, 2017 ):
Students tend to communicate and cheat with the people they know and feel close to.
An individuals’ relationship with others on social networks is closely related to their real-life relationships with people.
Regarding the second hypothesis, social network analysis could find students’ close friends and people they know. After clustering students, a unique set of exam questions are prepared for each cluster. Consequently, the collaboration of friends to cheat during the online exam becomes challenging.
Lowering cheating motivation
Approaches expressed in this section are based on mental and psychological aspects driving students toward academic misbehaviors, and the work being done to reduce these behaviors through controlling mental drivers.
There are several tactics to develop students’ moral beliefs encouraging them to avoid unethical behaviors. For instance, implementing honor systems helps build a healthy and ethical environment (Korman, 2010 ). Another tactic is clarifying academic integrity and morality ideals through establishing educational integrity programs (Korman, 2010 ).
As Korman ( 2010 ) further investigated, changing the students' perception about the goal of studying, could decrease cheating. This could be done by reminding them why learning matters and how it affects their future success. In (Varble, 2014 ), it is stated that emphasizing the actual value of education will lead to the same result.
Varble ( 2014 ), indicates that by improving students’ skills such as time management skills, their academic performance will be highly enhanced; accordingly, their academic misbehaviors will be declined. The risks of being caught and the significance of punishments, are inversely related to students’ motivation for cheating.
Varble ( 2014 ) also mentions that applying formative assessment rather than summative assessment effectively reduces examinees’ desire for cheating due to improving their learning outcomes. Formative assessments aim to enhance the candidates’ learning performance rather than testing them. On the other hand, summative assessments mostly care about measuring candidates’ knowledge and are used to check if they are eligible to pass the course or not.
As an additional description about getting a formative assessment to work, Nguyen et al., ( 2020 ) mention that increasing the exam frequency forces students to study course materials repeatedly, resulting in longer retention of information and knowledge in students’ minds. This brings about alleviating candidates’ motivation for cheating (Nguyen et al., 2020 ). Varble ( 2014 ), also suggests that reducing the value of each test lowers the reward gained by the cheaters over each test; consequently, the motivation for cheating is declined.
A cost-efficient and effective method to lower cheating motivation is to declare the cheating policy for examinees before the exam starts (Moten et al., 2013 ). Warning students of the consequences of being caught makes them nervous and can significantly decrease cheating. It is necessary to have a confirmation button, so that no excuses can be made by cheaters after the exam. It is such effective that in two experiments, it decreased the number of cheatings by 50% (Corrigan-Gibbs et al., 2015 ). It is worth mentioning that in the online environment, having an honor system is much less effective than warning about the consequences of cheating if being caught (Fontaine et al., 2020 ).
3.5.2 During-exam prevention
Most cheating prevention methods were discussed in the before-exam section; still, there exist some during-exam prevention tactics, which are presented in this sub-section.
Think-aloud request
A rarely mentioned method called Think-aloud request was discussed in (Chirumamilla & Sindre, 2019 ). In this method, a request is sent to the student to think aloud about a specific subject (or current question) at random times during the exam. The student has to respond to the request orally, and the voice is recorded for further investigation and cheating detection (e.g., slow response and voice impersonation detection). This mechanism forces students to continuously be ready for responding, which reduces the chance of student cheating. The authors have also mentioned that this system and its questions could be implemented by an AI agent.
Cheat-resistant systems
Using cheat-resistant systems will inherently prevent some kinds of cheatings, although they are costly to be implemented (Korman, 2010 ). Using a browser tab locker (Chua & Lumapas, 2019 ) is one of them that prevents unauthorized movements and also identifies them by sniffing their network packets. Another method is using wireless jammers (Chirumamilla & Sindre, 2019 ) to disrupt any radio signals (Internet) in an area which usually is the examination hall, during semi-online exams.
In (Chirumamilla & Sindre, 2019 ), some valuable suggestions are given for oral exams. One is conducting the oral exam as a flow of short questions and answers, instead of a long initial question and an extended answer afterward. This is because a flowing dialogue significantly reduces the chance of the examinee following someone else’s cues of the solution. They have also suggested that asking the examinee to respond quickly, will facilitate achieving this goal. Besides that, if candidates delay, they may be known suspicious. If a candidate was detected suspicious by the instructor, it is good to interrupt the current question with a new question. This will neutralize the effort made by a third party to help the candidate answer the question.
Another suggestion presented in (Chirumamilla & Sindre, 2019 ), is to prepare a big pool of questions for oral exams to prevent questions repetition. As a result, the candidates cannot adjust themselves to the questions asked from previous candidates.
Bribery is a kind of organizational cheating. In (Kigwana & Venter, 2016 ) it is indicated that by assigning a random human proctor for the exam right before it started, bribery and beforehand contractions between examinee and proctor would be impossible.
4 Discussion
There is no doubt that online education has changed significantly in recent years. One of the main challenges in online education is the validity of the assessment. Specifically, during the COVID19 pandemic, the integrity of online examinations has become a significant concern. Cheating detection and prevention are hot topics in online assessments. In addition, it is needed to conduct more research on cheating motivation and cheating types. In this research, we review and classify online exam cheating comprehensively.
In this review, only publications written in English were investigated. This could result in review bias, however, it is too difficult and infeasible to review studies in all languages. Many systematic mapping researches consider only publications in English, such as (Nikou & Economides, 2018 ) (Martin et al., 2020 ) (Noorbehbahani et al., 2019 ) (Wei et al., 2021 ).
Figure 3 indicates that the publications trend is decreasing, contrary to the hypothesis that online learning is rising, especially with the emergence of the COVID-19. Notably, in this study, online cheating researches have been reviewed. So, Fig. 3 specifically corresponds to online cheating publications not online learning studies in general. However, more investigations of online cheating studies from February 2021 onwards are required to further analyzing the trends.
Several reviewed studies have made no distinction between cheating detection and prevention (Bawarith, 2017 ; Bawarith et al., 2017 ; Korman, 2010 ; Tiong & Lee, 2021 ). They employed detection methods to identify dishonest behaviors. Then preventive actions such as making an alarm to the student, or closing the browser tab are performed to deter student cheating. Regarding this definition of prevention, several studies have applied these terms interchangeably, confusing the reader. In this study, we define cheating prevention as strategies and methods that try to prevent the occurrence of cheating in online exams. Considering the latter definition, we attempted to provide a better review and clearer classification to the readers.
One limitation in this domain is the lack of statistics on the popularity of the types, methods, and tools. In (Sabbah, 2017 ), the most common cheating behaviors and their average risks have been discussed; however, the results are limited to 10 cheating types. Hence, more investigation is required to determine the prevalence of each cheating type and cheating motivation.
An important cheating reason that is overlooked by researchers is learning styles. Students and educators have different preferred learning styles (auditory, visual, kinesthetic and read/write). If teachers and educational institutes don’t consider this issue, the course will not be apprehensible for some students, and consequently, they will be motivated to cheat.
Another issue that should be addressed is to evaluate the feasibility of cheating detection and prevention methods. If the equipment for securing online exams is expensive, the students cannot afford it. Therefore, this factor should be considered when developing detection and prevention methods. Cluskey et al. ( 2011 ), believe that some solutions (e.g., proctors) that detect cheating during online exams are too costly, and their costs outweigh their benefits in some cases. Therefore, cost-effective systems and methods should be implemented.
Privacy and convenience are also vital for examinees. If employed security mechanism for online exams violates privacy and disturbs student convenience, the evaluation will not be practical due to induced stress. Accordingly, these aspects should be considered in cheating detection and prevention systems.
5 Conclusion
In this study, cheating in online exams is reviewed and classified comprehensively. It provides the reader with valuable and practical insights to address online exam cheating. To mitigate students cheating, first, it is necessary to know cheating motivations and cheating types and technologies. Furthermore, cheating detection and prevention methods are needed to combat forbidden actions. Detection methods without applying prevention methods could not be effective. As cheating detection and prevention methods are evolved, new cheating types and technologies emerge as well. Consequently, no system can mitigate all kinds of cheating in online exams, and more advanced methods should be employed. It seems the most efficient strategy for cheating handling is to lower cheating motivation.
It should be mentioned that we have not covered studies related to technical attacks and intrusions to online exam systems and teacher devices. This topic could be considered for conducting another review study.
The impact of COVID-19 on online learning and cheating in online exams could be analyzed in future work.
Another future work is to explore how ignoring students’ learning styles in teaching and assessment could affect cheating motivation.
Privacy issues, user convenience, and enforced costs of cheating detection and prevention technologies need to be examined in other studies.
In this study, publications from 2010 to 2021 have been reviewed. More investigations are required to review accepted but unpublished studies and publications in 2022.
http://www.duplichecker.com
http://www.turnitin.com
Aisyah, S., Bandung, Y., & Subekti, L. B. (2018). Development of Continuous Authentication System on Android-Based Online Exam Application. In 2018 International Conference on Information Technology Systems and Innovation, ICITSI 2018 (pp. 171–176). Padang, Indonesia: IEEE. https://doi.org/10.1109/ICITSI.2018.8695954
Alessio, H. (2018). The Impact of Video Proctoring in Online Courses. Journal on Excellence in Col- Lege Teaching, 29 (3), 1–10.
Google Scholar
Alessio, H. M., Malay, N., Maurer, K., Bailer, A. J., & Rubin, B. (2017). Examining the Effect of Proctoring on Online Test Scores. Online Learning, 2013 (1), 1–16.
Amigud, A., & Lancaster, T. (2019). 246 reasons to cheat: An analysis of students’ reasons for seeking to outsource academic work. Computers and Education, 134 , 98–107. https://doi.org/10.1016/j.compedu.2019.01.017
Article Google Scholar
Arnautovski, L. (2019). Face recognition technology in the exam identity authentication system - implementation concept. In 2nd International Scientific Conference MILCON’19 (pp. 51–56). Olsztyn, Poland.
Atoum, Y., Chen, L., Liu, A. X., Hsu, S. D. H., & Liu, X. (2017). Automated Online Exam Proctoring. IEEE Transactions on Multimedia, 19 (7), 1609–1624. https://doi.org/10.1109/TMM.2017.2656064
Backman, J. (2019). Student s ’ Experiences of Cheating in the Online Exam Environment.
Bawarith, H. R. (2017). Student Cheating Detection System in E-exams . KING ABDULAZIZ UNIVERSITY.
Bawarith, R., Basuhail, A., Fattouh, A., & Gamalel-din, P. S. (2017). E-exam Cheating Detection System. International Journal of Advanced Computer Science and Applications, 8 (4), 176–181.
Bilen, E., & Matros, A. (2021). Online Cheating Amid COVID-19. Journal of Economic Behavior & Organization, 182 , 196–211.
Chirumamilla, A., & Sindre, G. (2019). Mitigation of Cheating in Online Exams: Strengths and Limitations of. In Biometric Authentication in Online Learning Environments (pp. 47–68). IGI Global. https://doi.org/10.4018/978-1-5225-7724-9.ch003
Chua, S. S., & Lumapas, Z. R. (2019). Online Examination System with Cheating Prevention Using Question Bank Randomization and Tab Locking. 2019 4th International Conference on Information Technology (InCIT) , 126–131.
Chuang, C. Y., Craig, S. D., & Femiani, J. (2017). Detecting probable cheating during online assessments based on time delay and head pose. Higher Education Research and Development, 36 (6), 1123–1137. https://doi.org/10.1080/07294360.2017.1303456
Cluskey, G. R. C. Jr, Ehlen, C. R., & Raiborn, M. H. (2011). Thwarting Online Exam Cheating without Proctor Supervision, 4 , 1–7.
Corrigan-Gibbs, H., Gupta, N., Northcutt, C., Cutrell, E., & Thies, W. (2015). Deterring cheating in online environments. ACM Transactions on Computer-Human Interaction , 22 (6). https://doi.org/10.1145/2810239
Cote, M., Jean, F., Albu, A. B., & Capson, D. (2016). Video Summarization for Remote Invigilation of Online Exams. In 2016 IEEE Winter Conference on Applications of Computer Vision (pp. 1–9). NY, USA.
Curran, K., Middleton, G., & Doherty, C. (2011). Cheating in Exams with Technology. International Journal of Cyber Ethics in Education, 1 (2), 54–62. https://doi.org/10.4018/ijcee.2011040105
Dendir, S., & Maxwell, R. S. (2020). Cheating in online courses: Evidence from online proctoring. Computers in Human Behavior Reports, 2 , 100033. https://doi.org/10.1016/j.chbr.2020.100033
Diedenhofen, B., & Musch, J. (2017). PageFocus: Using paradata to detect and prevent cheating on online achievement tests. Behavior Research Methods, 49 (4), 1444–1459. https://doi.org/10.3758/s13428-016-0800-7
Dobrovska, D. (2017). Technical Student Electronic Cheating on Examination. In M. E. Auer, D. Guralnick, & J. Uhomoibhi (Eds.), Interactive Collaborative Learning (pp. 525–531). Springer International Publishing.
Chapter Google Scholar
Fan, Z., Xu, J., Liu, W., & Cheng, W. (2016). Gesture based Misbehavior Detection in Online Examination. In The 11th International Conference on Computer Science & Education (pp. 234–238). NagoyaF, Japan.
Fayyoumi, A., & Zarrad, A. (2014). Novel Solution Based on Face Recognition to Address Identity Theft and Cheating in Online Examination Systems. Advances in Internet of Things, 4 (April), 5–12.
Fluck, A. E. (2019). An international review of eExam technologies and impact. Computers & Education, 132 , 1–15. https://doi.org/10.1016/j.compedu.2018.12.008
Fontaine, S., Frenette, E., & Hébert, M. (2020). Exam cheating among Quebec’s preservice teachers : the influencing factors. International Journal for Educational Integrity, 16 (14), 1–18.
Garg, K., Verma, K., Patidar, K., Tejra, N., & Petidar, K. (2020). Convolutional Neural Network based Virtual Exam Controller. In Proceedings of the International Conference on Intelligent Computing and Control Systems, ICICCS 2020 (pp. 895–899). Secunderabad, India. https://doi.org/10.1109/ICICCS48265.2020.9120966
Gruenigen, D. Von, de Azevedo e Souza, F. B., Pradarelli, B., Magid, A., & Cieliebak, M. (2018). Best practices in e-assessments with a special focus on cheating prevention. In 2018 {IEEE} Global Engineering Education Conference, {EDUCON} 2018, Santa Cruz de Tenerife, Tenerife, Islas Canarias, Spain, April 17-20, 2018 (pp. 893–899). IEEE. https://doi.org/10.1109/EDUCON.2018.8363325
He, H., Zheng, Q., Li, R., & Dong, B. (2018). Using Face Recognition to Detect “ Ghost Writer ” Cheating in Examination. In Edutainment, Lecture Notes in Computer Science (Vol. 11462, pp. 389–397). Springer International Publishing. https://doi.org/10.1007/978-3-030-23712-7
Holden, O., Kuhlmeier, V., & Norris, M. (2020). Academic Integrity in Online Testing: A Research Review. https://doi.org/10.31234/osf.io/rjk7g
Hu, S., Jia, X., & Fu, Y. (2018). Research on Abnormal Behavior Detection of Online Examination Based on Image Information. In 10th International Conference on Intelligent Human-Machine Systems and Cybernetics (IHMSC) (Vol. 02, pp. 88–91). Hangzhou, China: IEEE. https://doi.org/10.1109/IHMSC.2018.10127
Hylton, K., Levy, Y., & Dringus, L. P. (2016). Computers & Education Utilizing webcam-based proctoring to deter misconduct in online exams. Computers & Education, 92–93 , 53–63. https://doi.org/10.1016/j.compedu.2015.10.002
Idemudia, S., Rohani, M. F., Siraj, M., & Othman, S. H. (2016). A Smart Approach of E-Exam Assessment Method Using Face Recognition to Address Identity Theft and Cheating. International Journal of Computer Science and Information Security , 14 (10), 515–522. Retrieved from https://sites.google.com/site/ijcsis/
Jalali, K., & Noorbehbahani, F. (2017). An Automatic Method for Cheating Detection in Online Exams by Processing the Students Webcam Images. In 3rd Conference on Electrical and Computer Engineering Technology (E-Tech 2017), Tehran, Iran (pp. 1–6). Tehran, Iran.
Kasliwal, G. (2015). Cheating Detection in Online Examinations.
Kigwana, I., & Venter, H. (2016). Proposed high-level solutions to counter online examination fraud using digital forensic readiness techniques. Proceedings of the 11th International Conference on Cyber Warfare and Security, ICCWS 2016 , 407–414.
Korman, M. (2010). Behavioral detection of cheating in online examination. Retrieved from https://pure.ltu.se/ws/files/31188849/LTU-DUPP-10112-SE.pdf
Lancaster, T., & Clarke, R. (2017). Rethinking Assessment By Examination in the Age of Contract Cheating. Plagiarism Across Europe and Beyond 2017 .
Li, M., Sikdar, S., Xia, L., & Wang, G. (2020). Anti-cheating Online Exams by Minimizing the Cheating Gain, (May). https://doi.org/10.20944/preprints202005.0502.v1
Li, X., Yueran, K. C., & Alexander, Y. (2015). Massive Open Online Proctor : Protecting the Credibility of MOOCs Certificates, 1129–1137.
Maeda, M. (2019). Exam cheating among Cambodian students : when , how , and why it happens. Compare: A Journal of Comparative and International Education , 1–19. https://doi.org/10.1080/03057925.2019.1613344
Manoharan, S. (2019). Cheat-resistant multiple-choice examinations using personalization. Computers and Education, 130 , 139–151. https://doi.org/10.1016/j.compedu.2018.11.007
Martin, F., Sun, T., & Westine, C. D. (2020). A systematic review of research on online teaching and learning from 2009 to 2018. Computers & Education, 159 , 104009. https://doi.org/10.1016/j.compedu.2020.104009
Mengash, H. (2019). Automated Detection for Student Cheating During Written Exams: An Updated Algorithm Supported by Biometric of Intent. In First International Conference on Computing (pp. 303–3111). Riyadh, Saudi Arabia. https://doi.org/10.1007/978-3-030-36368-0
Migut, G., Koelma, D., Snoek, C. G., & Brouwer, N. (2018). Cheat Me Not: Automated Proctoring Of Digital Exams On Bring-Your-Own-Device. In The 23rd Annual ACM Conference On In- novation And Technology In Computer Science Education (p. 388). New York, NY, USA.
Moten, J. M. Jr, Fitterer, A., Brazier, E., Leonard, J., Brown, A., & Texas, A. (2013). Examining Online College Cyber Cheating Methods and Prevention Measures. Electronic Journal of E-Learning, 11 (2), 139–146.
Mott, J. H. (2010). The Detection and Minimization of Cheating During Concurrent Online Assessments Using Statistical Methods. Collegiate Aviation Review, 28 (2), 32–46.
Nguyen, J. G., Keuseman, K. J., & Humston, J. J. (2020). Minimize Online Cheating for Online Assessments During COVID-19 Pandemic. https://doi.org/10.1021/acs.jchemed.0c00790
Nikou, S. A., & Economides, A. A. (2018). Mobile-based assessment: A literature review of publications in major referred journals from 2009 to 2018. Computers & Education, 125 , 101–119. https://doi.org/10.1016/j.compedu.2018.06.006
Noorbehbahani, F., Salehi, F., & Jafar Zadeh, R. (2019). A systematic mapping study on gamification applied to e-marketing. Journal of Research in Interactive Marketing , 13 (3). https://doi.org/10.1108/JRIM-08-2018-0103
Norris, M. (2019). University online cheating - how to mitigate the damage. Research in Higher Education Journal, 37 , 1–20.
Opgen-Rhein, J., Küppers, B., & Schroeder, U. (2018). An application to discover cheating in digital exams. In ACM International Conference Proceeding Series . Koli, Finland. https://doi.org/10.1145/3279720.3279740
Page, M. J., Moher, D., Bossuyt, P. M., Boutron, I., Hoffmann, T. C., Mulrow, C. D., & Mckenzie, J. E. (2021). PRISMA 2020 explanation and elaboration: Updated guidance and exemplars for reporting systematic reviews. BMJ, 372 ,. https://doi.org/10.1136/bmj.n160
Parks, R. F., Lowry, P. B., Wigand, R. T., Agarwal, N., & Williams, T. L. (2018). Why students engage in cyber-cheating through a collective movement: A case of deviance and collusion. Computers and Education, 125 , 308–326. https://doi.org/10.1016/j.compedu.2018.04.003
Peytcheva-Forsyth, R., Aleksieva, L., & Yovkova, B. (2018). The impact of technology on cheating and plagiarism in the assessment – The teachers’ and students’ perspectives. In AIP Conference Proceedings 2048 (Vol. 020037, pp. 1–11).
Prathish, S., Athi Narayanan, S., & Bijlani, K. (2016). An intelligent system for online exam monitoring. In Proceedings - 2016 International Conference on Information Science, ICIS 2016 (pp. 138–143). Dublin, Ireland. https://doi.org/10.1109/INFOSCI.2016.7845315
Reisenwitz, T. H. (2020). Examining the Necessity of Proctoring Online Exams. Journal of Higher Education Theory and Practice, 20 (1), 118–124.
Saba, T., Rehman, A., Jamail, N. S. M., Marie-Sainte, S. L., Raza, M., & Sharif, M. (2021). Categorizing the Students’ Activities for Automated Exam Proctoring Using Proposed Deep L2-GraftNet CNN Network and ASO Based Feature Selection Approach. IEEE Access, 9 , 47639–47656. https://doi.org/10.1109/ACCESS.2021.3068223
Sabbah, Y. W. (2017). Security of Online Examinations. In Data Analytics and Decision Support for Cybersecurity (pp. 157–200). Springer International Publishing.
Srikanth, M., & Asmatulu, R. (2014). Modern Cheating Techniques, Their Adverse Effects on Engineering Education and preventions. International Journal of Mechanical Engineering Education, 42 (2), 129–140. https://doi.org/10.7227/IJMEE.0005
Tiong, L. C. O., & Lee, H. J. (2021). E-cheating Prevention Measures: Detection of Cheating at Online Examinations Using Deep Learning Approach -- A Case Study, XX (Xx), 1–9. Retrieved from http://arxiv.org/abs/2101.09841
Topîrceanu, A. (2017). Breaking up friendships in exams: A case study for minimizing student cheating in higher education using social network analysis. Computers and Education, 115 , 171–187. https://doi.org/10.1016/j.compedu.2017.08.008
Traore, I., Nakkabi, Y., Saad, S., & Sayed, B. (2017). Ensuring Online Exam Integrity Through Continuous Biometric Authentication. In Information Security Practices (pp. 73–81). Springer International Publishing. https://doi.org/10.1007/978-3-319-48947-6
Turner, S. W., & Uludag, S. (2013). Student perceptions of cheating in online and traditional classes. Proceedings - Frontiers in Education Conference, FIE , (October 2013), 1131–1137. https://doi.org/10.1109/FIE.2013.6685007
Ullah, A. (2016). Security and Usability of Authentication by Challenge Questions in Online Examination . University of Hertfordshire.
Valverde-Berrocoso, J., Garrido-Arroyo, M. del C., Burgos-Videla, C., & Morales-Cevallos, M. B. (2020). Trends in Educational Research about e-Learning: A Systematic Literature Review (2009–2018). Sustainability , 12 (12). https://doi.org/10.3390/su12125153
Varble, D. (2014). Reducing Cheating Opportunities in Online Test Online Tests, 3 (3).
Watson, G., & Sottile, J. (2010). Cheating in the Digital Age: Do Students Cheat More in Online Courses?. Online Journal of Distance Learning Administration , 13 (1).
Wei, X., Saab, N., & Admiraal, W. (2021). Assessment of cognitive, behavioral, and affective learning outcomes in massive open online courses: A systematic literature review. Computers & Education, 163 , 104097. https://doi.org/10.1016/j.compedu.2020.104097
Weiner, J. A., & Hurtz, G. M. (2017). A comparative Study of Online Remote Proctored Vs Onsite Proctored. Journal of Applied Testing Technology, 18 (1), 13–20.
Wong, S., Yang, L., Riecke, B., Cramer, E., & Neustaedter, C. (2017). Assessing the usability of smartwatches for academic cheating during exams. Proceedings of the 19th International Conference on Human-Computer Interaction with Mobile Devices and Services, MobileHCI 2017 . https://doi.org/10.1145/3098279.3098568
Xiong, Y., & Suen, H. K. (2018). Assessment approaches in massive open online courses: Possibilities, challenges and future directions. International Review of Education, 64 (2), 241–263. https://doi.org/10.1007/s11159-018-9710-5
Download references
Author information
Authors and affiliations.
Faculty of Computer Engineering, University of Isfahan, Azadi square, 8174673441, Isfahan, Iran
Fakhroddin Noorbehbahani, Azadeh Mohammadi & Mohammad Aminazadeh
You can also search for this author in PubMed Google Scholar
Corresponding author
Correspondence to Fakhroddin Noorbehbahani .
Ethics declarations
Conflict of interests/competing interests.
The authors declare that they have no competing interests.
Additional information
Publisher's note.
Springer Nature remains neutral with regard to jurisdictional claims in published maps and institutional affiliations.
Rights and permissions
Reprints and permissions
About this article
Noorbehbahani, F., Mohammadi, A. & Aminazadeh, M. A systematic review of research on cheating in online exams from 2010 to 2021. Educ Inf Technol 27 , 8413–8460 (2022). https://doi.org/10.1007/s10639-022-10927-7
Download citation
Received : 26 October 2021
Accepted : 31 January 2022
Published : 07 March 2022
Issue Date : July 2022
DOI : https://doi.org/10.1007/s10639-022-10927-7
Share this article
Anyone you share the following link with will be able to read this content:
Sorry, a shareable link is not currently available for this article.
Provided by the Springer Nature SharedIt content-sharing initiative
- Online exam
- Cheating motivations
- Systematic mapping review.
- Find a journal
- Publish with us
- Track your research

An official website of the United States government
The .gov means it’s official. Federal government websites often end in .gov or .mil. Before sharing sensitive information, make sure you’re on a federal government site.
The site is secure. The https:// ensures that you are connecting to the official website and that any information you provide is encrypted and transmitted securely.
- Publications
- Account settings
Preview improvements coming to the PMC website in October 2024. Learn More or Try it out now .
- Advanced Search
- Journal List
- Springer Nature - PMC COVID-19 Collection

A systematic review of research on cheating in online exams from 2010 to 2021
Fakhroddin noorbehbahani.
Faculty of Computer Engineering, University of Isfahan, Azadi square, 8174673441 Isfahan, Iran
Azadeh Mohammadi
Mohammad aminazadeh.
In recent years, online learning has received more attention than ever before. One of the most challenging aspects of online education is the students' assessment since academic integrity could be violated due to various cheating behaviors in online examinations. Although a considerable number of literature reviews exist about online learning, there is no such review study to provide comprehensive insight into cheating motivations, cheating types, cheating detection, and cheating prevention in the online setting. The current study is a review of 58 publications about online cheating, published from January 2010 to February 2021. We present the categorization of the research and show topic trends in the field of online exam cheating. The study can be a valuable reference for educators and researchers working in the field of online learning to obtain a comprehensive view of cheating mitigation, detection, and prevention.
Introduction
Today, distance education has been transformed into online settings, and the COVID-19 pandemic has raised online learning significantly across the world. The COVID-19 enforced the closing of traditional learning all over the world, resulting in 1.5 billion students and 63 million educators shifting from face-to-face learning to online learning. This situation has revealed the strengths and weaknesses of the digital transformation of education (Valverde-Berrocoso et al., 2020 ).
In (Martin et al., 2020 ), it has been shown that the online learning publications are continuously being increased from 2009 to 2018, and one of the leading research themes is course assessment. Course assessment is very challenging in online learning due to the lack of direct control over students and educators.
For an educational institution, assessment integrity is essential because it affects institutional reputation. It is necessary to employ traditional cheating detection besides prevention methods and new digital monitoring and validation techniques to support assessment integrity in online exams (Fluck, 2019 ).
The study (Watson & Sottile, 2010 ) has reported that students are remarkably more likely to get answers from others during online exams or quizzes compared to live (face-to-face) ones. Therefore, preserving the integrity of online exams is more challenging. There are some strategies to mitigate online exam cheating, such as getting offline (face-to-face) proctored exam, developing cheat-resistant questions (e.g., using subjective measures instead of objective measures), and lessening the exam score percentage contributing to the overall course grade.
Traditional cheating methods include, hiding notes in a pencil case, behind ruler, or clothes, writing on arms/hands, leaving the room, etc. (Curran et al., 2011 ). Technological advances and online learning have enhanced education, however, they also have facilitated cheating in courses (Turner & Uludag, 2013 ). For instance, an examinee could use a mobile phone to text someone to get the answer. Although this would be difficult in the exam hall, some examinees could text without looking at the mobile phone. Applying scientific calculators, Mp3 players calculator, and wireless equipment such as an earphone and a microphone are other tools that facilitate cheating in offline exams (Curran et al., 2011 ).
Although cheating motivations in online and offline exams are not significantly different (Turner & Uludag, 2013 ), detecting and mitigating online cheating could be more intricate. This is because, in addition to traditional cheating methods that also could be exploited in online exam cheating, there exist various technologies and tools that could be applied for cheating in online exams more easily. For example, using remote desktop and share screen, searching for solutions on Internet, using social networks, etc.
Cheating in an online setting is more convenient than a traditional offline exam. Accordingly, detecting and preventing online cheating is critical for online assessment. Therefore, this issue is one of the biggest challenges that MOOC (Massive Open Online Courses) summative assessment faces.
Recent researches imply that a critical issue in online education is academic dishonesty and cheating. Today, paid services exist that impersonate students in online courses to ensure their identity. In recent years, proctoring technologies such as identity authentication, keystroke recognition, and webcam proctoring will be extended to secure online exams (Xiong & Suen, 2018 ). Apart from direct proctoring, there are some techniques such as controlling the browser, limiting exam time, randomizing questions and choices, etc. However, it seems cheating in online courses is pretty common (Dendir & Maxwell, 2020 ).
Although one of the most critical challenges in online learning is to mitigate and handle cheating, there is no comprehensive literature review and classification in this field. Hence, in this paper, we present a systematic mapping review of researches in online examination cheating. The research questions are as follows:
- RQ1: What are the publication trends in online cheating?
- RQ2: What are the main reasons for online cheating?
- RQ3: What are the cheating types in online exams?
- RQ4: How can online cheating be detected?
- RQ5: How can online exam cheating be prevented?
The paper is structured as follows. In Section 2 , the research method is described, including study selection criteria, databases and search strategy, and study selection. Section 3 presents review results and provides the answers to research questions. Sections 4 and 5 discuss the results and conclude the paper, respectively.
The current study is a literature review about cheating in online exams. A literature review identifies, selects, and synthesizes primary research studies in order to provide a picture of the topic under investigation. According to (Page et al., 2021 ), a record is the title or abstract (or both) of a report indexed in a database or website, and a report is a document (in paper or electronic format) supplying information about a particular study. It could be a journal article, preprint, conference abstract, study register entry, clinical study report, dissertation, unpublished manuscript, government report, or any other document providing relevant information. The current literature search has been performed based on the well-established PRISMA principles (Page et al., 2021 ).
Inclusion and exclusion criteria
The main criteria for the articles considered in the current review are as follows.
Inclusion criteria:
- Researches should be written in English.
- Records should be retrieved utilizing the designed search query.
- Studies should be published between January 2010 and February 2021.
- In cases where several papers reported the same study, only the most recent ones were included (i.e., theses and papers extracted from theses, extended version of papers published in journals).
Exclusion criteria:
- Papers merely related to methods applicable to traditional cheating types, detection, and prevention are eliminated.
- Studies not related to research questions are ignored.
- Articles only related to cyber-attacks to online exam systems are excluded.
- Low-quality researches are discarded (i.e., studies published by non-reputable publishers without peer review, too short review time, and so on, studies with poor theoretical background, experimental evaluation, or structure).
Databases and search strategy
We applied a wide range of databases as our primary source, including Google Scholar, Web of Science, and Scopus. We also added the publications which had cited the extracted records. Records were searched using the following search terms for the title, keywords, and abstract sections.
(Cheat OR e-Cheating OR Fraud OR Dishonesty OR Anti-cheating OR Cheat-resistant OR Abnormal behavior OR Misconduct OR Integrity OR Plagiarism) AND
(Electronic OR Online OR Digital OR Virtual OR Cyber OR Academic) AND
(Exam OR e-Exam OR Course OR e-Course OR Assessment OR e-Assessment OR Test OR e-Test OR Environment OR e-Environment) AND
(Prevent OR Detect OR Mitigate OR Reduce OR Minimize OR Monitor OR Proctor OR Reason OR Motivation OR Type OR Deter OR Control).
Study selection
The search result included 289 records, 26 of which were duplicated, and so they were deleted. From 263 screened records, 54 records were excluded by examining either the title or the abstract. In the next step, 12 reports were eliminated because they were not retrieved because were not accessible. Furthermore, after full-text eligibility checking, 144 reports have been excluded according to the inclusion and exclusion criteria as mentioned earlier.
This resulted in 53 reports that along with 5 other reports (obtained from citation searching and assessed for eligibility), were finally selected for literature review about online cheating. The flow of information through different phases of the review is presented in the PRISMA flow diagram depicted in Fig. Fig.1 1 .

The PRISMA flow diagram
After selecting 58 studies, three domain experts were asked to assign a Credibility Score (CS) to each study. After evaluation of each study, experts agreed on a credibility score ranging from 0 to 5 based on the following criteria: publisher credibility, number of citations per year, theoretical and experimental quality, and organization and structure. CS statistics are as follows: mean = 3.81, SD =0.79, min = 2.5, max =5.
A summary of online cheating research papers and their study themes is presented in Table Table1. 1 . (Appendix (Appendix1 1 .)
Online cheating studies
No. | Reference | Subject | Pub Type | Study Theme | No. of Citations | CS | Research Purpose | |||
---|---|---|---|---|---|---|---|---|---|---|
Cheating Reasons | Cheating Prevention | Cheating Detection | Cheating Types | |||||||
1 | (H. R. Bawarith, ) | Student Cheating Detection System in E-exams | Thesis | - | ✓ | ✓ | ✓ | 0 | 3 | Investigating cheating methods and designing an e-exam management system. |
2 | (Migut et al., ) | Cheat me not: Automated Proctoring of Digital Exams on Bring-Your-Own-Device | Conference | - | - | ✓ | - | 5 | 2.5 | Presenting preliminary results on automated video proctoring, which can reduce manual effort and scale-up digital assessment. |
3 | (Amigud & Lancaster, ) | 246 Reasons to Cheat: an Analysis of Students’ Reasons for Seeking to Outsource Academic Work | Journal | ✓ | - | - | - | 33 | 5 | Examination of the reasons cause outsourcing academic work. |
4 | (Weiner & Hurtz, ) | A Comparative Study of Online Remote Proctored vs. Onsite Proctored | Journal | - | - | ✓ | - | 21 | 4.5 | Comparing test scores between kiosk-based remote online proctored and onsite proctored exams. |
5 | (Idemudia et al., ) | A Smart Approach of E-Exam Assessment Method Using Face Recognition to Address Identity Theft and Cheating | Journal | - | - | ✓ | - | 3 | 3.5 | Proposed an authentication system using face recognition. |
6 | (Holden et al., ) | Academic Integrity in Online Testing A Research Review | Journal | ✓ | ✓ | ✓ | - | 4 | 3 | Provided a research review with a focus on methods used to improve academic integrity. |
7 | (Opgen-Rhein et al., ) | An Application to Discover Cheating in Digital Exams | Conference | - | - | ✓ | - | 9 | 3 | Proposed a system to verify the author of assignments using programming style. |
8 | (Prathish et al., ) | An Intelligent System for Online Exam Monitoring | Conference | - | - | ✓ | - | 12 | 3.5 | Designed a multimodal system for online exam proctoring and automated cheating detection. |
9 | (Jalali & Noorbehbahani, ) | An Automatic Method for Cheating Detection in Online Exams by Processing the Student's Webcam Images | Conference | - | - | ✓ | - | 6 | 4 | Automatic cheating detection during online exams through processing webcam images. |
10 | (Li et al., ) | Anti-cheating Online Exams by Minimizing the Cheating Gain | Journal | - | ✓ | - | - | 1 | 3.5 | Cheating prevention by minimizing cheating gain with the help of question order randomization. |
11 | (Wong et al., ) | Assessing the Usability of Smartwatches for Academic Cheating During Exams | Journal | - | - | - | ✓ | 11 | 3.5 | Examining the usability of smartwatches for cheating in various exam designs. |
12 | (Atoum et al., ) | Automated Online Exam Proctoring | Conference | - | - | ✓ | - | 54 | 5 | Presented a multimedia (image and voice) analytics system for cheating detection during exams. |
13 | (Korman, ) | Behavioral Detection of cheating | Thesis | ✓ | ✓ | ✓ | ✓ | 5 | 5 | Studies of online examination cheating detection through human-computer interaction dynamics. |
14 | (Gruenigen et al., ) | Best practices in e-assessments with a special focus on cheating prevention | Conference | - | ✓ | ✓ | - | 8 | 2.5 | Discussed methods of cheating prevention during e-assessments. |
15 | (Topîrceanu, ) | Breaking up Friendships in Exams: a Case Study for Minimizing Student Cheating in Higher Education Using Social Network Analysis | Journal | - | ✓ | - | - | 16 | 5 | Methods are discussed for identifying students’ friends via their social network analysis, to divide friends into different groups. |
16 | (Saba et al., ) | Categorizing the Students' Activities for Automated Exam Proctoring Using Proposed Deep L2-GraftNet CNN Network and ASO Based Feature Selection Approach | Journal | - | - | ✓ | - | 0 | 5 | Designed an automated exam proctor that categorizes students’ body movements. |
17 | (Kasliwal, ) | Cheating Detection in Online Examinations | Thesis | - | ✓ | ✓ | ✓ | 2 | 5 | Developed and analyzed a tool for monitoring students’ browsing activities. |
18 | (Manoharan, ) | Cheat-resistant Multiple-choice Examinations Using Personalization | Journal | - | ✓ | ✓ | - | 15 | 5 | Preventing cheating in multiple-choice questions via personalized exams (each student gets a different set of questions). |
19 | (Lancaster & Clarke, ) | Rethinking Assessment By Examination in the Age of Contract Cheating | Conference | - | ✓ | - | ✓ | 22 | 4 | Proposing different techniques of contract cheating and a discussion around the exam design to address these issues. |
20 | (Garg et al., ) | Convolutional Neural Network based Virtual Exam Controller | Conference | - | ✓ | ✓ | - | 2 | 3 | Automated cheating detection via webcam recording. |
21 | (Corrigan-Gibbs et al., ) | Deterring Cheating in Online Environments | Journal | - | ✓ | ✓ | ✓ | 53 | 5 | Measuring the amount of cheating after employing three distinct methods including, honor codes, controlling, and warning. |
22 | (Chuang et al., ) | Detecting Probable Cheating During Online Assessments Based on Time Delay and Head Pose | Journal | - | - | ✓ | - | 8 | 4 | Identifying test takers’ behaviors for detecting cheating, with a focus on time delay and head pose. |
23 | (Aisyah et al., ) | Development of Continuous Authentication System on Android-Based Online Exam Application | Conference | - | ✓ | ✓ | - | 2 | 3 | Developed a continuous authentication system for an android-based online learning application. |
24 | (Diedenhofen & Musch, ) | Pagefocus: Using Paradata to Detect and Prevent Cheating on Online Achievement Tests | Journal | - | ✓ | ✓ | - | 31 | 5 | Developed a system called pageFocus, which detects unauthorized activities such as opening another window or tab beside the exam window. |
25 | (Tiong & Lee, ) | E-cheating Prevention Measures: Detection of Cheating at Online Examinations Using Deep Learning Approach-A Case Study | Journal | - | ✓ | ✓ | - | 0 | 4 | Developed an intelligent cheating detector based on two modules: 1) IP detector, 2) Behavior detector. |
26 | (R. Bawarith et al., ) | E-exam Cheating Detection System | Journal | - | ✓ | ✓ | ✓ | 17 | 4 | Investigates the methods used to detect cheating in online exams, mostly through continuous authentication and online proctoring. |
27 | (Traore et al., ) | Ensuring Online Exam Integrity Through Continuous Biometric Authentication | Book | - | - | ✓ | - | 21 | 5 | Proposed a system that continuously authenticates examinees using face, keystroke and mouse dynamics. |
28 | (Maeda, ) | Exam Cheating Among Cambodian Students: When, How, and Why It Happens | Journal | ✓ | - | - | - | 6 | 4 | Studied Cambodian students’ cheating practices and the reasons behind them. |
29 | (Fontaine et al., ) | Exam Cheating Among Quebec’s Preservice Teachers | Journal | ✓ | - | - | ✓ | 0 | 4 | Presented the results of a search that aimed to examine the phenomenon of student cheating on exams in faculties of education in Quebec university. |
30 | (Moten et al., ) | Examining Online College Cyber Cheating Methods and Prevention Measures | Journal | - | ✓ | - | ✓ | 73 | 4.5 | Mentioning some types of cheating practices and their curtailment techniques. |
31 | (H. M. Alessio et al., ) | Examining the Effect Of Proctoring On Online Test Scores | Journal | - | ✓ | - | - | 33 | 3.5 | Compared test results (scores) between proctored and non-proctored online exams. |
32 | (Reisenwitz, ) | Examining the Necessity of Proctoring Online Exams | Journal | - | ✓ | - | - | 5 | 3 | Investigated the differences between non-proctored and proctored online exam scores. |
33 | (Fan et al., ) | Gesture Based Misbehavior Detection in Online Examination | Conference | - | - | ✓ | - | 7 | 3.5 | Introduced a gesture-based solution for misbehavior detection using Microsoft Kinect device. |
34 | (Cluskey et al., ) | Thwarting Online Exam Cheating Without Proctor Supervision | Journal | - | ✓ | - | - | 96 | 4.5 | Examines the control issues related to online exams. |
35 | (X. Li et al., ) | Massive Open Online Proctor Protecting the Credibility of MOOCS Certificates | Conference | - | - | ✓ | - | 32 | 4 | Proposed a massive open online proctoring (MOOP) framework, which combines both automatic and collaborative approaches to detect cheating behaviors in online tests. |
36 | (Nguyen et al., ) | Minimize Online Cheating for Online Assessments During COVID-19 Pandemic | Journal | - | ✓ | - | - | 6 | 3.5 | Presented strategies that effectively minimize cheating while addressing learning outcomes. |
37 | (Chirumamilla & Sindre, ) | Mitigation of Cheating in Online Exams Strengths and Limitations of Biometric Authentication | Journal | - | ✓ | ✓ | ✓ | 3 | 3.5 | Delivered a categorization of different types of high-stakes assessments, different ways of cheating, and what types of cheating are most relevant for what types of assessments. |
38 | (Srikanth & Asmatulu, ) | modern Cheating Techniques, Their Adverse Effects on Engineering Education and Preventions | Journal | - | ✓ | - | - | 21 | 4 | Evaluated some of the high-tech cheating systems and devices that have been a major threat to engineering education. |
39 | (Fayyoumi & Zarrad, ) | Novel Solution Based on Face Recognition to Address Identity Theft and Cheating in Online Examination Systems | Journal | - | - | ✓ | - | 24 | 4 | Provided a solution for detecting cheating behaviors such as looking at an adjacent PC or reading from an external source using video capturing. |
40 | (Bilen & Matros, ) | Online Cheating Amid COVID-19 | Journal | ✓ | - | - | - | 6 | 3.5 | Presented evidence of cheating that had taken place in online exams during COVID-19 lockdowns and proposed a solution based on the experience accumulated by online chess communities. |
41 | (Chua & Lumapas, ) | Online Examination System with Cheating Prevention Using Question Bank Randomization and Tab Locking | Conference | - | ✓ | - | - | 2 | 3 | Presented the results of interviews with a group of online exam proctors to identify the root causes of academic malpractice. |
42 | (Arnautovski, ) | Face Recognition Technology in the Exam Identity Authentication System. Implementation Concept | Conference | - | ✓ | - | - | 0 | 2.5 | Proposed a unimodal authentication system, which captures the image of the test-taker at random time intervals. |
43 | (Sabbah, ) | Security of Online Examinations | Book | - | ✓ | ✓ | - | 2 | 4 | Proposed two major schemes for continuous authentication. |
44 | (Mengash, ) | Automated Detection for Student Cheating During Written Exams: An Updated Algorithm Supported by Biometric of Intent | Conference | - | - | ✓ | - | 2 | 2.5 | Proposed a system that detects cheating intentions using a thermal detector, a surveillance camera and an eye movement tracker |
45 | (Dobrovska, ) | Technical Student Electronic Cheating on Examination | Conference | ✓ | - | - | ✓ | 0 | 3 | The study gauged the forms, frequency, and variety of electronic cheating of university students, and the teacher attitudes toward cheating. |
46 | (Kigwana & Venter, ) | Proposed High-Level Solutions to Counter Online Examination Fraud Using Digital Forensic Readiness Techniques | Conference | - | ✓ | ✓ | ✓ | 1 | 2.5 | Explored the various ways of student cheating and proposed a high-level digital forensic readiness techniques. |
47 | (Varble, ) | Reducing Cheating Opportunities in Online Tests | Journal | - | ✓ | - | - | 20 | 3.5 | Focused on reducing cheating opportunities of online test assessments. |
48 | (Hu et al., ) | Research on abnormal behavior detection of online examination based on image information | Conference | - | - | ✓ | - | 3 | 3 | Proposed a system that uses a webcam to monitor candidates' head posture and mouth state to detect abnormal behavior during online exams. |
49 | (Ullah, ) | Security and Usability of Authentication by Challenge Questions in Online Examination | Thesis | - | ✓ | ✓ | - | 2 | 4 | Proposed a profile-based challenge question approach to create and consolidate a student’s profile during the learning prcess to be used for authentication in the examination process. |
50 | (Mott, ) | The Detection and Minimization of Cheating During Concurrent Online Assessments Using Statistical Methods | Journal | - | - | ✓ | - | 8 | 4 | Developed a statistical algorithm to detect group cheating by investigating identical incorrect responses. |
51 | (Peytcheva-Forsyth et al., ) | The Impact of Technology on Cheating and Plagiarism in the Assessment, the Teachers' and Students' Perspectives | Conference | - | ✓ | - | ✓ | 12 | 3.5 | Investigated the impact of technology on cheating and plagiarism from the perspective of teachers and students of Sofia university related to both aspects of facilitation and prevention of such behaviors. |
52 | (H. Alessio, ) | The Impact of Video Proctoring in Online Courses | Journal | - | ✓ | - | - | 4 | 3 | Analyzed the change in grade distributions across 29 courses and instructors on a college campus before and after video proctoring. |
53 | (Backman, ) | Students' Experiences of Cheating in the Online Exam Environment | Thesis | ✓ | ✓ | - | ✓ | 1 | 4 | Produced recommendations to teachers who instruct online courses on how to teach courses to mitigate online cheating. |
54 | (Norris, ) | University Online Cheating - How to Mitigate the Damage | Journal | ✓ | ✓ | - | ✓ | 2 | 3 | Discussed the history and motivations for cheating, and the proliferating number of entrepreneurs and products that assist students in completing their courses in ways that compromise academic integrity. |
55 | (He et al., ) | Using Face Recognition to Detect “Ghost Writer” Cheating in Examination | Conference | - | - | ✓ | - | 1 | 3.5 | Proposed a three layers architecture to detect the ghostwriter who takes the exam for others. |
56 | (Hylton et al., ) | Utilizing Webcam-Based Proctoring to Deter Misconduct in Online Exams | Journal | - | ✓ | - | - | 74 | 5 | Investigated the deterrent effect of webcam-based proctoring on misconduct during online exams. |
57 | (Cote et al., ) | Video Summarization for Remote Invigilation of Online Exams | Conference | - | - | ✓ | - | 13 | 4 | Focused on video summarization of abnormal behavior for remote proctoring. |
58 | (Parks et al., ) | Why Students Engage in Cyber-Cheating Through a Collective Movement a Case of Deviance and Collusion | Journal | ✓ | - | - | ✓ | 15 | 5 | Conducted a case study of “Tasribat”, a Facebook page that facilitates cyber cheating among certain social groups of students in Morocco. |
Several findings emerged as a result of the research synthesis of the selected fifty-eight records on online cheating. The selected studies were categorized into four main topics, namely Cheating reasons, Cheating types, Cheating detection, and Cheating prevention, as shown in Fig. Fig.2. 2 . All subsequent classifications reported in this paper have been provided by the authors. The studies under every four main topics are investigated by three experts, and a list of items is extracted for each category. Notably, some studies were corresponded to multiple main topics. Next, several brainstorming sessions have been conducted to classify each main topic further. To extract the classifications, the XMind tool has been employed, which is a professional and popular mind mapping software.

Online cheating research classification
In the following sub-sections, the detailed analysis of the review results is described according to the five research questions we defined to drive the research.
Publication trends
In Fig. Fig.3, 3 , the number of publications per year is displayed (in this study, the final publication date is applied). In 2017, the greatest number of studies corresponding to the conducted review have been published. As shown in Fig. Fig.4, 4 , the dominant publication type is journal papers with 53% of the total publications. In terms of the average citations of the selected studies regarding their classes, the maximum average citations belong to the journal papers with an average citation of 19.65 (see Fig. Fig.5 5 ).

Number of publications per year

Distribution of publication per types

Average citation per publication type
There are 747 works cite the selected studies related to the review. As displayed in Fig. Fig.6, 6 , the greatest and lowest shares of the total citations pertain to the journal articles and the theses, respectively. The number of publications per research theme is shown in Fig. Fig.7. 7 . The cheating prevention and detection themes are the most prevalent research themes in online cheating. In the following four subsections, the studies under each of the four research themes are described and classified thoroughly.

Distribution of publications according to citations

Number of publications per research theme
Cheating reasons
The primary reason for cheating is that examinees feel the rewards outweigh the risks (Lancaster & Clarke, 2017 ). There exists a wide variety of reasons why candidates decide to commit cheating, still, they could be categorized into four general reasons, namely Teacher-related, Institutional, Internal, and Environmental reasons. The complete classification of the cheating reasons is displayed in Fig. Fig.8, 8 , which is described in the following sections.

Teacher-related reasons
All the reasons related to the teacher or the course instructor are put into this category. Maeda ( 2019 ), has observed that the student’s relationship with the teacher has crucial influences on academic integrity. Teachers’ unethical behaviors, such as favoring those who have bribed over those who have not, or favoring the students who participated in private tutoring sessions, motivate the oppressed students to cheat. The author also found that teachers’ low interest in students’ depth of learning, which also results in a poor pedagogical style, could be an important reason that motivates students to participate in any kind of unethical behavior (Maeda, 2019 ).
Course difficulty could motivate the examinees to cheat. Some students blamed their teachers for complicated and complex course materials. In some specific cases, this reason could be a consequence of students’ lack of perseverance. They find cheating as a way to relieve these difficulties (Amigud & Lancaster, 2019 ).
As a result of distributed learning with online courses and examinations, Moten et al. ( 2013 ), have expressed that students feel isolated in an online environment. They often become frustrated when they do not get the help they immediately need, for instance, the night before an exam. This situation is closely dependent on the presence time of the teacher in online communication environments.
Some teachers restrain from punishing the cheaters appropriately due to ethical issues. This could be due to the sympathy of some teachers with cheaters. After listening to the cheater’s excuses and justifications, the teacher might give them a second chance. Sometimes, teachers are worried about the consequences of punishments and the corresponding pressures that cheaters experience, hence they don’t punish the cheater or the punishment is too mellow.
This increases the students’ courage to cheat during online exams due to decreased risk of being punished after being caught and implies that cheating penalties are insignificant over the long run (Topîrceanu, 2017 ).
Exam design is one of the most important contributing factors that motivates examinees to cheat in the exam. Weakly designed exams such as similar multiple-questions for every examinee or easy accessibility of solutions over the web, can make it easy to cheat. On the other hand, questions being too complex and irrelevant to course materials, forces students to commit cheating during online exams (Srikanth & Asmatulu, 2014 ).
Institutional reasons
In (Maeda, 2019 ), it is observed that the rules and policies of the institution are directly related to the number of unethical behaviors occurrences. It is found that institutions with stricter regulations and better commitment to strengthening academic integrity, face much less cheating behavior between their students. Institutional policies not only create an anti-cheating atmosphere, but also makes dishonest academic behaviors challenging to take place. Also, Backman ( 2019 ) emphasizes that if it becomes easy for students to cheat, they will cheat.
Impulsiveness is a crucial reason why students try to cheat during online examinations. They feel isolated and disconnected, so they may imagine they won’t get caught or the instructor does not care if they commit academic dishonesty. Unethical behaviors have a direct relationship with the student’s impulsiveness (Moten et al., 2013 ).
Moreover, in an isolated environment, due to the lack of face-to-face communications with teachers, students have much less respect for their teachers that leads to increasing misbehaviors. That is why teachers should personalize the online environment for students by calling their names or listening to their voices, so that online classes become more engaging and interactive for students (Moten et al., 2013 ).
Dobrovska ( 2017 ), expressed that the poor quality of the institution’s online learning system discourages students from learning the course materials, and makes it difficult for them to learn, hence, they are more motivated to cheat.
Academic aptitude is one of the most important and underrated reasons leading students to commit misbehaviors. It means educational institutions don’t discriminate between students and ignore their unique abilities, skills, and different levels of preparedness for a specific task. This makes unprepared students feel frustrated about that particular task or course, which leads them to seek help from more talented and prepared students in that specific context (Amigud & Lancaster, 2019 ).
Internal reasons
Another category of cheating reasons is internal motivators. The motivators over which the candidate has complete control, including intrinsic factors, personality and psychological characteristics, lie in this category. The internal reasons are divided into three subcategories as follows.
Student’s academic performance
One significant internal factor is the student’s academic performance. There are several reasons that could result in poor academic performance as follows: lack of learning and skills to find resources, students unwillingness to follow recommended practices, inability to seek appropriate help, procrastination, poor time management (Dobrovska, 2017 ), and lack of confidence in their ability to learn course materials (Norris, 2019 ).
Low intrinsic interest in the course materials
Low intrinsic interest in the course is another reason mentioned in (Dobrovska, 2017 ), which could be caused by a lack of sufficient interest in course materials and subjects or the mindset that these materials and knowledge are unnecessary and unimportant for future life (Norris, 2019 ).
Personal characteristics
There is a strong relationship between students’ moral attitudes toward cheating and their level of participation in academic misbehaviors (Maeda, 2019 ). Therefore, conscientious belief is considered as an internal reason stopping students from unethical behaviors. However, it has been shown that religious beliefs do not necessarily lower cheating behaviors (Srikanth & Asmatulu, 2014 ).
Other reasons included in studies are student’s laziness for sufficient home preparation before the exam (Dobrovska, 2017 ), competition with others and the desire to get ahead (Amigud & Lancaster, 2019 ), desire to help other peers (Moten et al., 2013 ) and the student’s thrill of taking risk (Hylton et al., 2016 ).
Environmental reasons
The reasons mentioned in this section highly depend on the atmosphere and type of environment a student is in, either during the online exam or beforehand in social media or communication with people. We put these reasons in four major categories: Peers’ behavior, Parents’ attitudes, Personal issues and, Social factors.
Peers’ behavior
Peers could influence individuals in a manner that their cheating motivations are increased. In an academic environment, however, it is primarily because of the competing objectives, such as the desire to get ahead in scores. This depends on the amount of competition in the academic environment (Amigud & Lancaster, 2019 ).
Experimental research among Cambodian students, has figured out that being among a group of cheaters, psychologically drives the students to repeat their peers’ actions and commit cheating. In addition, there is high pressure on those who do not collaborate with peers, or reject participating in their group work. It is found that they are blamed for being odd and unkind (Maeda, 2019 ).
According to (Srikanth & Asmatulu, 2014 ), being in an environment where peers’ cheating remains undetected, gives this kind of feeling to non-cheaters that they are setting back in scores and are unfairly disadvantaged compared to those cheaters.
Parents’ attitude
Parents’ acceptance of cheating behaviors, massively affects the student’s mindset toward these behaviors. As expressed in (Maeda, 2019 ), parents’ behaviors toward their child’s cheating, vary from complete unacceptance to active involvement and support. Another reason related to parents’ attitudes is putting their children under pressure to achieve good or higher than average grades (Backman, 2019 ).
Personal issues
Personal issues could be mental and physical health problems (Amigud & Lancaster, 2019 ), problems within the family (e.g., parents arguing, separation and divorce, etc.), and fear of failure in exams and its further consequences like financial and time setbacks (Hylton et al., 2016 ).
Societal factors
Poor economic conditions and the development level of a country are examples of societal factors affecting students’ motivation to cheat and achieve academic success (Maeda, 2019 ).
Countries with various cultures, social expectancies, and people’s attitudes have different behaviors regarding academic performance. In some countries, academic performance and grades are known to be crucial for success in life, whereas, in other countries, academic performance is relatively low valued. This range of different expectations from students leads to various social beliefs and behaviors toward cheating (Maeda, 2019 ). In research presented in (Holden et al., 2020 ), it is shown that a primary reason could be the existence of a cheating culture. Some students may cheat because they desire to portray a better image of themselves to their society (Norris, 2019 ). Another societal factor influencing cheating behaviors is the technology evolution that strengthens cheating motivation (Maeda, 2019 ). This is because technology brings about increased access to cheating resources. The evolution of technology, specifically search engines and social media, makes it easier for students to cheat.
Cheating types and facilitators
To mitigate cheating behaviors effectively and efficiently, cheating methodologies, types, and facilitators should be known. Cheating is performed either individually or by the cooperation of others (called group cheating). Figure Figure9 9 displays the complete classification of cheating types.

Cheating types
Individual cheating
Individual cheating is carried out without any assistance from any person. This type of cheating could be categorized as using forbidden materials and other types are described as follows.
Using forbidden materials
Individual cheating can occur by using forbidden materials during the exam, such as looking at a textbook or a cheat sheet (Fontaine et al., 2020 ), (Holden et al., 2020 ), searching the web, using offline electronic resources such as images, voices, etc. (Korman, 2010 ), (Holden et al., 2020 ), or even using objects in the exam room to hide notes.
Other types
Other types of individual cheating include accessing the questions and solutions before the exam, which Korman ( 2010 ) refers to as “unauthorized intelligence”. Another dishonest behavior is social engineering, which is grade negotiation with the teacher through fake facts and exploiting personal sympathy.
Group cheating
Cheating methods through cooperation with others could be categorized as Impersonation, and Collaboration types.
Impersonation
Impersonation means employing someone to take the exam for the examinee, either the whole exam or some parts of it (Korman, 2010 ), (Holden et al., 2020 ). It can occur in forms of voice conversion, face presentation attack and face impersonation, fake identity matching to a stored biometric, and attack on the keystroke dynamics (Chirumamilla & Sindre, 2019 ). These are attacks on the biometric system to bypass the authentication mechanisms. The other impersonation techniques include remote desktop control by a third party (Kasliwal, 2015 ), (Gruenigen et al., 2018 ), sharing the screen with a third party (Gruenigen et al., 2018 ), (Bawarith, 2017 ), and credential sharing, which is impersonation via shared username and password of an academic account or LMS (Learning Management System) (Dobrovska, 2017 ).
Collaboration
Collaboration is defined as getting any kind of help from others to answer the exam questions. It could be in the form of sign language communications that come in numerous forms, such as foot-tapping, pencil or any object dropping during the proctored exam, abnormal coughing, or suspicious actions (Srikanth & Asmatulu, 2014 ).
Listening to a third party’s whispers behind the camera (Chirumamilla & Sindre, 2019 ), any type of communication which is unauthorized such as sending or receiving messages, or voice and video calls (Korman, 2010 ), are also considered as collaborative cheating.
Other cheating methods in this category are remote desktop control (Kasliwal, 2015 ) and sharing the screen with others to collaborate with others about questions (Gruenigen et al., 2018 ), applying small hidden micro cameras to capture images and record videos for sharing with other peers (Bawarith, 2017 ), and finally, organizational cheating which is a result of institution’s personnel corruption (Korman, 2010 ).
The last one, as Korman ( 2010 ) showed, can take place when personnel help candidates to cheat. Changing the exam grade or exam answers after the exam (exam integrity corruption), giving the solutions to the candidate during the exam, or just bribing the proctor not to report the cheating or not to punish after being caught (Kigwana & Venter, 2016 ) are instances of organized cheating.
Contract work is a type of collaboration that means doing work with the help of someone else under the obligations of a contract. Contract workers may provide some or all of the exam answers. In this case, sometimes impersonating the student through the whole academic course is reported (Chirumamilla & Sindre, 2019 ).
Cheating facilitators
Methods discussed here act as cheating facilitators to support the process of cheating. In other words, these facilitators can be applied to perform any kind of cheating. A study presented in (Peytcheva-Forsyth et al., 2018 ), indicates that technology in general, is the leading facilitator of cheating practices. Cheating facilitators are classified as shown in Fig. Fig.10 10 .

Three different methodologies are used by students to facilitate cheating, either individually or in a group, described as follows.
Interrupting to get more time
Sometimes examinees try to buy more time to work more on the exam answers. For instance, the examinee may report an error about the exam system or exam proctoring software to convince the teacher to restart the exam session. This enables the candidate to get more time for cheating and finding the solutions during this interval when the session is closed (Motenet al., 2013 ). Another interruption method is to submit corrupted answer files by the candidate. In this case, the teacher reports that the files were corrupted and asks the candidate to resubmit the answer files. Most of the time, during the first submission and the second one, there exists at least one day, which implies the candidate gets at least one more day to answer the exam questions (Moten et al., 2013 ).
Other more classical methods to interrupt are toilet requests during the exam (Chirumamilla & Sindre, 2019 ), communication break and delay in answering oral exam right after a question is asked (Chirumamilla & Sindre, 2019 ), circumventing the exam process at a specific time with different excuses, and postponing taking the exam (Fontaine et al., 2020 ), (Korman, 2010 ). By deferring taking the exam, students can buy more time to become more prepared, either by studying more, or getting access to the exam questions and solutions.
Employing multiple devices
In proctored exams, either by a camera or software, students try to use multiple devices and answer the questions with the primary one while cheating via the secondary device. Several types of devices could be employed as the second device, such as computers and laptops (Moten et al., 2013 ), smartwatches (Wong et al., 2017 ), smart glasses such as Google glasses (Srikanth & Asmatulu, 2014 ), smartphones and tablets (Korman, 2010 ), programmable and graphical calculators to store notes and formulas (Kigwana & Venter, 2016 ), and tiny earpieces for remote voice support during the exam (Bawarith, 2017 ).
Other facilitators
Redirecting the webcam to hide something from its field of view (Sabbah, 2017 ), (Srikanth & Asmatulu, 2014 ), or disabling the webcam or microphone completely (Srikanth & Asmatulu, 2014 ) are other tricks used to facilitate cheating.
By using virtual machines on a computer, the user can run a virtual operating system on the primary one. This technique would hide the activities done on the second operating system from the software or the human proctoring the primary operating system. (Kasliwal, 2015 ).
Corrupting the exam system’s integrity to change the exam results after being held (e.g., changing the scores or answers after the examination) is another notable case (Korman, 2010 ). Lastly, in (Parks et al., 2018 ), the authors have investigated that social media and channels operating on them could act as cheating facilitation environments.
Cheating detection
Cheating detection methods can be categorized into during the exam and after the exam detection methods. Further classification of the cheating detection methods is presented in Fig. Fig.11 11 .

Cheating detection during the exam
To ensure academic integrity in online examinations, it is essential to detect cheating during the exam. Cheating detection can be partitioned into two main categories, namely, continuous authentication and online proctoring. Continuous authentication methods verify the identity of test-takers, and online proctoring monitors the examinees to detect any misbehavior during the exam. In the following, we will mention different techniques in each category.
Continuous authentication
One of the main types of cheating is impersonating. Therefore, it is essential to authenticate students before exam registration and prevent unauthorized candidates from taking the examination. In addition, it is necessary to validate the identity of the test-taker during the exam continuously. The continuous authentication systems are mainly based on biometric or behaviometric modalities and can be categorized into unimodal and multimodal schemes.
Unimodal authentication is the automatic recognition and identification of candidates using a unique characteristic. This characteristic could be either static (physiological) such as the face, fingerprint, hand geometry, and iris, or could be dynamic (behavioral) such as voice, handwriting, keystroke, and mouse dynamics (Chirumamilla & Sindre, 2019 ).
As a unimodal authentication system, Arnautovski ( 2019 ) designed a face recognition system, which captures the image of the test-taker at random time intervals. The facial recognition module continuously verifies the examinee’s identity by comparing captured images to the image from the exam registration process. In (Aisyah et al., 2018 ), an Android-based online exam application is implemented that takes photos of the examinee with random intervals and a web-based application lets the admin or supervisor of examination validate pictures of participants. In addition, Idemudia et al. ( 2016 ) proposed a system that tracks and detects faces continuously to verify the candidates. If the authentication failure remains for more than a few seconds, the system will stop the examination.
In (Sabbah, 2017 ), a scheme called ISEEU is proposed, in which each examinee’s session is streamed using a webcam. A proctor monitors the video screens and can generate alerts when any suspicious action is detected. He et al. ( 2018 ) proposed an anti-ghostwriter system using face recognition methods. The ghostwriter merges the student’s photo and their photo to make a fake one, or they change their appearance to mislead the examiners. The experimental results in (He et al., 2018 ), indicate that the proposed framework can detect ghostwriters with an acceptable level of accuracy.
Since some candidates may refuse to use a camera due to privacy concerns, Bilen et al. (2020) suggested that instructors offer their students two options. An examinee can agree to use a camera during the exam. In this situation, the record will be used as evidence if they are accused of cheating. However, if the examinee doesn’t accept using a camera, the instructor can claim cheating without providing evidence to the student.
In (Bawarith, 2017 ), the system authenticates the examinees continuously through an eye tracker. The data obtained from the eye tracker are translated into a set of pixel coordinates so that the presence or absence of eyes in different screen areas can be investigated.
Multimodal biometric authentication systems utilize different biometric or behaviometric traits simultaneously, which makes impersonating more difficult. In this regard, Bawarith et al. ( 2017 ) proposed a system that utilizes fingerprint and eye-tracking for authentication. The eye tribe tracker is used to continuously ensure that test-takers are the ones they are claiming to be. Whenever the system detects the examinee is no longer present in front of the screen, the system is locked, and the test-taker must be authenticated again via fingerprint.
In (Sabbah, 2017 ), a multimodal scheme called SABBAH is proposed, which adds continuous fingerprint and keystroke dynamics to the ISEEU scheme (Sabbah, 2017 ). In contrast to ISEEU, SABBAH uses an automatic system to detect fingerprint, keystroke, or video violations. Traore et al. ( 2017 ) proposed a system that continuously authenticates examinees using three complementary biometric technologies, i.e., face, keystroke, and mouse dynamics. In this system, test-takers are continuously authenticated in the background during the exam, and alarms are created and sent to the instructor through the proctoring panel.
Online proctoring
Online proctoring is essential to promote academic integrity. Alessio et al. ( 2017 ) reported significant grade disparities in proctored versus un-proctored online exams. Online proctoring can be categorized into human and automated proctoring. In human proctoring, a human proctor monitors the students remotely to detect suspicious behavior. In contrast, in automated proctoring, the cheating behaviors are flagged or detected automatically by the proctoring system.
Recently, several technologies have been developed to facilitate proctoring online exams remotely. For example, Kryterion™ Live Video Monitoring and ProctorU allow users to be monitored by a human proctor via a webcam during examination (Hylton et al., 2016 ). In (Reisenwitz, 2020 ), substantial support for online proctoring is provided. The results show a significant difference between the scores of exams that were not proctored and those proctored using ProctorU software.
Some systems can capture screenshots of the candidates’ PCs at random times during the examination (Migut et al., 2018 ). Consequently, if examinees use any forbidden resource on their computer, it will be shown to the proctor. Alessio ( 2018 ) applied video proctoring via a webcam at Miami University. The results demonstrate that students are less likely to cheat when monitored with a webcam during online testing.
In another study, kiosk-based remote online proctored examinations are compared with tests administered under a traditional proctoring environment. In kiosk-based proctoring, the test is taken on special computer kiosks located at accessible places such as libraries. The kiosks are equipped with enhanced webcams and are supervised online by a live remote proctor. The results indicated that examinees’ scores obtained under online kiosk-based proctoring are comparable to examinations taken in test centers with onsite proctors (Weiner & Hurtz, 2017 ).
A different approach for cheating detection is a class mole that means the instructor enrolls in students’ groups under another name as a mole to detect and combat collusion. In this way, they can discover dishonest students when they discuss cheating amongst themselves (Moten et al., 2013 ).
Human proctoring is costly and labor-intensive. Therefore, different automated proctoring systems are proposed to monitor the students during the examination and detect unauthorized behavior. In the following, we discuss several automated methods.
Chuang et al. proposed a semi-automatic proctoring system that employs two factors, namely, time delay in answering the questions and head-pose variation, to detect suspicious behavior. Afterward, a human proctor could use more evidence to decide whether a student has cheated (Chuang et al., 2017 ).
Garg et al. ( 2020 ) proposed a system to detect the candidate’s face using Haar Cascade Classifier and deep learning. If the examinee’s face moves out of the examination frame or multiple faces are detected in the frame, the test will automatically be terminated, and the administrator will receive a notification. In (Fayyoumi & Zarrad, 2014 ), a two-second candidate video is taken during the examination period. The images in the video are analyzed to verify whether the examinee is looking somewhere other than their screen. If the test-taker doesn’t focus on their screen, it may indicate cheating behaviors such as looking at an adjacent PC or reading from an external source.
In (Hu et al., 2018 ), the proposed system uses a webcam to monitor candidates' head posture and mouth state to detect abnormal behavior. Through the rule-based reasoning method, the system can detect suspicious behavior such as turning heads and speaking during the online examination.
Prathish et al. ( 2016 ), developed a multimodal system for online proctoring. The system captures audios and videos of the candidates as well as their active windows. If yaw angle variations, audio presence, or window changes are detected in any time frame, it can be considered an indicator of cheating. Consequently, the captured video, audio, and system usage are fed into a rule-based inference system to detect the possibilities of misbehaviors. ProctorTrack is another automated online exam proctoring product that employs facial and audio recognition, body movements, and computer activity monitoring to detect any suspicious action during examination (Norris, 2019 ).
Atoum et al., ( 2017 ) developed a system that can detect a wide variety of cheating behaviors during an online exam using a webcam, wearcam, and microphone. Using wearcam makes it possible to monitor what the student observes. It helps to detect any phone or text in the testing room that is prohibited. In addition, by using the wearcam, the system can detect another form of cheating that is reading from books, notes, etc. Furthermore, the system can estimate the head gaze of the test-taker by combining the information from the webcam and wearcam. Another form of cheating is getting verbal assistance from another person in the same room, or remotely via a phone call. The system can detect this kind of cheating using the microphone and speech detection. Considering the mentioned aspects, the proposed multimedia system can perform automatic online exam proctoring.
Saba et al. ( 2021 ), developed an automatic exam activity recognition system, which monitors the body movements of the students through surveillance cameras and classifies activities into six categories using a deep learning approach. The action categories are normal performing, looking back, watching towards the front, passing gestures to other fellows, watching towards left or right, and other suspicious actions. Movement recognition based on video images is highly dependent on the quality of images. Therefore, Fan et al. ( 2016 ), employed a Microsoft Kinect device to capture the examinee’s gesture. The duration and frequency of the detected action events are then used to distinguish the misbehavior from the normal behavior.
The system presented in (Mengash, 2019 ) includes a thermal detector attached with a surveillance camera and an eye movement tracker. When examinees intend to cheat, their body will emit a specific range of heat, and the emitted heat will trigger the camera to focus and detect the candidate’s face. Then the eye tracker detects eye movements, and the system detects the cheating intentions of the test-taker. There are other biometric-based methods for cheating detection. For example, keystroke and linguistic dynamics can detect stress, which indicates suspicious behavior (Korman, 2010 ).
Diedenhofen and Musch ( 2017 ), developed a JavaScript application called PageFocus, which can be added to the test page and run in the background. Whenever the examinee switches to a page other than the test page, a defocusing event is registered. The script captures when and how frequently defocusing and refocusing events occur on the test page. Another method is to permit students to get to just a couple of sites that are whitelist. If the examinee tries to open a site that is not allowed (one from blacklist), the instructor will be informed through an Android application or Internet (Kasliwal, 2015 ).
Tiong and Lee ( 2021 ), proposed an e-cheating intelligent agent composed of two modules, namely the internet protocol (IP) detector and the behavior detector. The first module could monitor the examinees’ IP addresses and enable the system to alert if a student changes their device or location. The second module detects abnormal behavior based on the speed of answering questions. Another method for cheating detection is comparing the IP addresses of the examinees to check whether two participants are in the same place (Bawarith, 2017 ).
Cheating detection after the exam
Even though different methods are employed to prevent students from cheating, some will still cheat during the examination. Consequently, a bunch of techniques is proposed to detect cheating students after the exam. This way, the reliability of online assessments will be improved. In the following, we will discuss different methods of cheating detection after the exam.
Video monitoring
The University of Amsterdam has developed a system that records the student’s video screen and the environment during the exam. Later a human proctor views the recording and flags and reports any suspicious behavior (Norris, 2019 ). Proctoring software proposed in (Alessio et al., 2017 ), records everything students do during the examination. After the exam, the recordings can be reviewed by the professor, teaching assistants, or employees of the proctoring vendor to identify cheating behaviors.
Human proctoring is a tedious and time-consuming process. To reduce the time and cost of proctoring, an automatic system can be employed to detect and flag suspicious events using machine learning methods. In this regard, Cote et al. ( 2016 ) proposed a system for the automatic creation of video summaries of online exams. The proposed method employs head pose estimations to model a normal and abnormal examinee’s behavior. Afterward, a video summary is created from sequences of detected abnormal behavior. The video summaries can assist remote proctors in detecting cheating after the exam.
Jalali and Noorbehbahani ( 2017 ), implemented an automatic method for cheating detection using a webcam. During the exam, images are recorded every 30 seconds by a webcam for each candidate. After the exam, the recorded images are compared with reference images of that student. If the difference exceeds a threshold, the image will be labeled as a cheating state.
Li et al. ( 2015 ), proposed a Massive Open Online Proctoring framework that consists of three components. First, the Automatic Cheating Detector (ACD) module uses webcam video to monitor students, and automatically flag suspected cheating behavior. Then, ambiguous cases are sent to the Peer Cheating Detector (PCD) module, which asks students to review videos of their peers. Finally, the list of suspicious cheating behaviors is forwarded to the Final Review Committee (FRC) to make the final decision.
Other methods
There are various ways of cheating, and therefore, different methods are used to detect cheating after the exam. For example, one of the cheating behaviors is to collude and work on tests together. However, most learning management systems allow the instructor to view IP addresses. Therefore, if different students submit their assessments by the same IP address in a short time frame, it could be detected and considered as a sign of collusion (Moten et al., 2013 ).
In addition, statistical methods can be used to analyze student responses to assessments and detect common errors and the similarities of answers (Korman, 2010 ). Mott ( 2010 ) stated that the distribution of identical incorrect responses between examinee pairs is a Polya distribution. The degree of cheating for each examination will follow the skewness or third central moment of the distribution.
Predictive analytics systems implicitly collect data while the students interact with the virtual learning environment. The collected data, which include student’s location, access patterns, learning progress, device characteristics, and performance, is used to predict trends and patterns of student behavior. Consequently, any unusual pattern may indicate suspicious behavior (Norris, 2019 ). Answering an examination takes a reasonable amount of time. Therefore, another indicator of dishonest behavior is an extremely short interval between the access time and the completion of the assessments, which can be detected by log time analysis (Moten et al., 2013 ).
In (Bawarith et al., 2017 ), an E-exam management system is proposed that classifies participants as cheating or non-cheating based on two parameters, namely the total time and the number of times the examinee is out of the screen. The focus of the test-taker is recorded using an eye tracker during the exam.
Kasliwal (Kasliwal, 2015 ), designed an online examination tool that captures the network traffic during the exam using a kismet server. The captured package can then be analyzed to determine the frequency of URLs accessed by students. If one of the URLs is getting accessed more frequently or very rarely, it could be considered suspicious.
To detect plagiarism in papers or essay-type questions, platforms such as DupliChecker.com 1 or Turnitin.com 2 can be used. These websites compute a similarity index and show all potential plagiarisms. Based on the similarity index, the instructor decides about further actions (Moten et al., 2013 ).
A weakness of similarity detection software is that it computes the resemblance of a submitted assessment with others' works and cannot detect an original text written by others for the student in question. Stylometry discovers this issue by checking the consistency of the delivered contents with other texts written by the same student. If the style of a text does not match with the previous works of that student, it may indicate complicity (Chirumamilla & Sindre, 2019 ). Opgen-Rhein et al. ( 2018 ) presented an application that employs machine learning methods to learn the programming styles of students. This work is based on the assumption that the programming style of each student is unique, and therefore, the model can be used to verify the author of assignments.
Another way of cheating detection is using a cheating trap, which means creating websites that could be found when the students search for answers. The solutions in trap websites are incorrect, and consequently, dishonest students could be detected (Korman, 2010 ). However, this method contradicts professional ethics.
In addition, the teacher can search the internet by hand periodically and try to find all possible web pages that provide solutions matching the exam questions. This approach could be applied to create a pool of potential solutions from the internet that will be used for plagiarism detection purposes after the exam (Norris, 2019 ).
Cheating prevention
After discussing and analyzing the examinees’ motivations for cheating and the reasons which directly or indirectly drive them to commit unethical actions during online examinations, a great deal of concern is gathered around how to decrease cheating in online exams and lower the probability of these actions taking place.
We categorized cheating prevention into two major types, namely, before-exam prevention and during-exam prevention. Figure Figure12 12 displays the classification of the cheating prevention methods.

Before-exam prevention
To prevent examinees from cheating, there exist several methods that should be implemented before the exam is held. Each will be discussed in detail as follows.
Exam design
In any situation that prevention is concerned, a proven and low-cost approach is a “cheat-resistant” design -A design that inherently prevents some specific cheating types from happening. This is why exam design is so critical. A cheat-resistant exam design, by its nature, prevents a range of possible forms of cheatings from occurring.
One way of achieving a good design is developing personalized exams for each candidate separately. There are several ways to do so, such as parameterization (Manoharan, 2019 ), which is a set of fixed questions with variable assumption values, using data banks with a large pool of questions to select questions randomly (Manoharan, 2019 ), (Norris, 2019 ) or implementing an AI-based method to produce unique exams (Chua & Lumapas, 2019 ).
Li et al. ( 2020 ) has put effort into designing a method for randomizing the question orders for each candidate. Their general idea is to show the questions one by one, and besides that, each student gets a different question at a time. This research mathematically proves that examinees cannot get much cheating gain.
In (Manoharan, 2019 ), the author has investigated an approach to personalizing multiple-choice examinations using the macro. Macro is a computer program fragment that stores data. It has a set of particular inputs for generating random exams based on a question bank. This method could bring freedom and flexibility to the exam design, but it needs basic programming skills.
Another aspect of exam design concentrates specifically on question design. Some of the most valuable methods are listed below.
- Using novel questions: This type of question design is so unique in design and phrasing that it becomes very challenging to be plagiarized even with searching the web (Nguyen et al., 2020 ).
- Using knowledge-based questions instead of information-based questions: These questions challenge the level of knowledge. The answers are not on the web or in reference books, and they need critical thinking and reasoning (Nguyen et al., 2020 ).
- Using essay questions rather than multiple-choice questions: During an online exam, multiple-choice questions are highly susceptible to cheating. Hence, long essay questions are preferred (Varble, 2014 ).
- Using questions with specific assumptions and facts: Although giving extra and not useful facts may mislead any candidate, even those taking the exam honestly, it will reduce the possibility of web-based plagiarism considerably by making it less straightforward to search online (Nguyen et al., 2020 ).
- Having an open-book exam: Open-book exam questions should test students’ understanding, critical reasoning, and analytical skills. Since the answers to these questions are not found in any sources directly, open-book exams may reduce the cheating opportunity (Varble, 2014 ), (Backman, 2019 ).
Finally, other methods not placed into the above categories are mentioned below.
Showing questions one by one without the option of going backward is effective in cheating prevention. If it is employed besides strict time limitations and random question series, collaborative cheating will become quite challenging (Chirumamilla & Sindre, 2019 ), (Backman, 2019 ). By setting strict time limitations, the students do not have enough time to handle cheating, therefore, exam cheating efforts are reduced (Backman, 2019 ).
Cluskey et al. ( 2011 ), emphasize low-cost approaches for addressing online exam cheating. They introduce online exam control procedures (OECP) to achieve this target. Taking the exam only at a defined time and avoiding postponing it for any reason, or changing at least one-third of the questions in the next exam, are some instances of these procedures.
Authentication
Authentication is mainly for impersonation prevention before examinations. It could be done classically by checking the school ID badges or government-issued ID by the webcam (Moten et al., 2013 ) or by a more modern approach like biometrics through fingerprint, palm vein scan (Korman, 2010 ), eye vein scan (Kigwana & Venter, 2016 ), voice, and keystroke biometrics (Norris, 2019 ).
An interesting method to prevent cheating has been presented in (Moten et al., 2013 ). Students should call the instructor at a predetermined time to get the password. After the students’ voices are recognized by the instructor, they are authenticated and receive a random password for exam entrance. The password is valid until the end of the exam time limit, thus this method makes cheating more difficult (Moten et al., 2013 ).
The last method of authentication is the one discussed in (Norris, 2019 ) which uses challenge questions. These are the questions only the student will know, for instance, student ID or personal information. In (Ullah, 2016 ), an approach is proposed that creates and consolidates a student’s profile during the learning process. This information is collected in the form of questions and answers. The questions are pre-defined or extracted from a student’s learning activities. A subset of questions is used for authentication, and the students should answer these questions correctly to get access to the online examination. This approach ensures that the person taking the exam is the same one who has completed the course.
Clustering means partitioning students into several groups based on a predefined similarity measure. In (Topîrceanu, 2017 ), random and strategic clustering methods are proposed to break friendships during the exam, as cheating prevention techniques. The advantages of random clustering are time and cost efficiencies; however, it is imprecise, and some clusters may include unbroken friendships.
Breaking friendships through clustering relies on two hypotheses (Topîrceanu, 2017 ):
- Students tend to communicate and cheat with the people they know and feel close to.
- An individuals’ relationship with others on social networks is closely related to their real-life relationships with people.
Regarding the second hypothesis, social network analysis could find students’ close friends and people they know. After clustering students, a unique set of exam questions are prepared for each cluster. Consequently, the collaboration of friends to cheat during the online exam becomes challenging.
Lowering cheating motivation
Approaches expressed in this section are based on mental and psychological aspects driving students toward academic misbehaviors, and the work being done to reduce these behaviors through controlling mental drivers.
There are several tactics to develop students’ moral beliefs encouraging them to avoid unethical behaviors. For instance, implementing honor systems helps build a healthy and ethical environment (Korman, 2010 ). Another tactic is clarifying academic integrity and morality ideals through establishing educational integrity programs (Korman, 2010 ).
As Korman ( 2010 ) further investigated, changing the students' perception about the goal of studying, could decrease cheating. This could be done by reminding them why learning matters and how it affects their future success. In (Varble, 2014 ), it is stated that emphasizing the actual value of education will lead to the same result.
Varble ( 2014 ), indicates that by improving students’ skills such as time management skills, their academic performance will be highly enhanced; accordingly, their academic misbehaviors will be declined. The risks of being caught and the significance of punishments, are inversely related to students’ motivation for cheating.
Varble ( 2014 ) also mentions that applying formative assessment rather than summative assessment effectively reduces examinees’ desire for cheating due to improving their learning outcomes. Formative assessments aim to enhance the candidates’ learning performance rather than testing them. On the other hand, summative assessments mostly care about measuring candidates’ knowledge and are used to check if they are eligible to pass the course or not.
As an additional description about getting a formative assessment to work, Nguyen et al., ( 2020 ) mention that increasing the exam frequency forces students to study course materials repeatedly, resulting in longer retention of information and knowledge in students’ minds. This brings about alleviating candidates’ motivation for cheating (Nguyen et al., 2020 ). Varble ( 2014 ), also suggests that reducing the value of each test lowers the reward gained by the cheaters over each test; consequently, the motivation for cheating is declined.
A cost-efficient and effective method to lower cheating motivation is to declare the cheating policy for examinees before the exam starts (Moten et al., 2013 ). Warning students of the consequences of being caught makes them nervous and can significantly decrease cheating. It is necessary to have a confirmation button, so that no excuses can be made by cheaters after the exam. It is such effective that in two experiments, it decreased the number of cheatings by 50% (Corrigan-Gibbs et al., 2015 ). It is worth mentioning that in the online environment, having an honor system is much less effective than warning about the consequences of cheating if being caught (Fontaine et al., 2020 ).
During-exam prevention
Most cheating prevention methods were discussed in the before-exam section; still, there exist some during-exam prevention tactics, which are presented in this sub-section.
Think-aloud request
A rarely mentioned method called Think-aloud request was discussed in (Chirumamilla & Sindre, 2019 ). In this method, a request is sent to the student to think aloud about a specific subject (or current question) at random times during the exam. The student has to respond to the request orally, and the voice is recorded for further investigation and cheating detection (e.g., slow response and voice impersonation detection). This mechanism forces students to continuously be ready for responding, which reduces the chance of student cheating. The authors have also mentioned that this system and its questions could be implemented by an AI agent.
Cheat-resistant systems
Using cheat-resistant systems will inherently prevent some kinds of cheatings, although they are costly to be implemented (Korman, 2010 ). Using a browser tab locker (Chua & Lumapas, 2019 ) is one of them that prevents unauthorized movements and also identifies them by sniffing their network packets. Another method is using wireless jammers (Chirumamilla & Sindre, 2019 ) to disrupt any radio signals (Internet) in an area which usually is the examination hall, during semi-online exams.
In (Chirumamilla & Sindre, 2019 ), some valuable suggestions are given for oral exams. One is conducting the oral exam as a flow of short questions and answers, instead of a long initial question and an extended answer afterward. This is because a flowing dialogue significantly reduces the chance of the examinee following someone else’s cues of the solution. They have also suggested that asking the examinee to respond quickly, will facilitate achieving this goal. Besides that, if candidates delay, they may be known suspicious. If a candidate was detected suspicious by the instructor, it is good to interrupt the current question with a new question. This will neutralize the effort made by a third party to help the candidate answer the question.
Another suggestion presented in (Chirumamilla & Sindre, 2019 ), is to prepare a big pool of questions for oral exams to prevent questions repetition. As a result, the candidates cannot adjust themselves to the questions asked from previous candidates.
Bribery is a kind of organizational cheating. In (Kigwana & Venter, 2016 ) it is indicated that by assigning a random human proctor for the exam right before it started, bribery and beforehand contractions between examinee and proctor would be impossible.
There is no doubt that online education has changed significantly in recent years. One of the main challenges in online education is the validity of the assessment. Specifically, during the COVID19 pandemic, the integrity of online examinations has become a significant concern. Cheating detection and prevention are hot topics in online assessments. In addition, it is needed to conduct more research on cheating motivation and cheating types. In this research, we review and classify online exam cheating comprehensively.
In this review, only publications written in English were investigated. This could result in review bias, however, it is too difficult and infeasible to review studies in all languages. Many systematic mapping researches consider only publications in English, such as (Nikou & Economides, 2018 ) (Martin et al., 2020 ) (Noorbehbahani et al., 2019 ) (Wei et al., 2021 ).
Figure Figure3 3 indicates that the publications trend is decreasing, contrary to the hypothesis that online learning is rising, especially with the emergence of the COVID-19. Notably, in this study, online cheating researches have been reviewed. So, Fig. Fig.3 3 specifically corresponds to online cheating publications not online learning studies in general. However, more investigations of online cheating studies from February 2021 onwards are required to further analyzing the trends.
Several reviewed studies have made no distinction between cheating detection and prevention (Bawarith, 2017 ; Bawarith et al., 2017 ; Korman, 2010 ; Tiong & Lee, 2021 ). They employed detection methods to identify dishonest behaviors. Then preventive actions such as making an alarm to the student, or closing the browser tab are performed to deter student cheating. Regarding this definition of prevention, several studies have applied these terms interchangeably, confusing the reader. In this study, we define cheating prevention as strategies and methods that try to prevent the occurrence of cheating in online exams. Considering the latter definition, we attempted to provide a better review and clearer classification to the readers.
One limitation in this domain is the lack of statistics on the popularity of the types, methods, and tools. In (Sabbah, 2017 ), the most common cheating behaviors and their average risks have been discussed; however, the results are limited to 10 cheating types. Hence, more investigation is required to determine the prevalence of each cheating type and cheating motivation.
An important cheating reason that is overlooked by researchers is learning styles. Students and educators have different preferred learning styles (auditory, visual, kinesthetic and read/write). If teachers and educational institutes don’t consider this issue, the course will not be apprehensible for some students, and consequently, they will be motivated to cheat.
Another issue that should be addressed is to evaluate the feasibility of cheating detection and prevention methods. If the equipment for securing online exams is expensive, the students cannot afford it. Therefore, this factor should be considered when developing detection and prevention methods. Cluskey et al. ( 2011 ), believe that some solutions (e.g., proctors) that detect cheating during online exams are too costly, and their costs outweigh their benefits in some cases. Therefore, cost-effective systems and methods should be implemented.
Privacy and convenience are also vital for examinees. If employed security mechanism for online exams violates privacy and disturbs student convenience, the evaluation will not be practical due to induced stress. Accordingly, these aspects should be considered in cheating detection and prevention systems.
In this study, cheating in online exams is reviewed and classified comprehensively. It provides the reader with valuable and practical insights to address online exam cheating. To mitigate students cheating, first, it is necessary to know cheating motivations and cheating types and technologies. Furthermore, cheating detection and prevention methods are needed to combat forbidden actions. Detection methods without applying prevention methods could not be effective. As cheating detection and prevention methods are evolved, new cheating types and technologies emerge as well. Consequently, no system can mitigate all kinds of cheating in online exams, and more advanced methods should be employed. It seems the most efficient strategy for cheating handling is to lower cheating motivation.
It should be mentioned that we have not covered studies related to technical attacks and intrusions to online exam systems and teacher devices. This topic could be considered for conducting another review study.
The impact of COVID-19 on online learning and cheating in online exams could be analyzed in future work.
Another future work is to explore how ignoring students’ learning styles in teaching and assessment could affect cheating motivation.
Privacy issues, user convenience, and enforced costs of cheating detection and prevention technologies need to be examined in other studies.
In this study, publications from 2010 to 2021 have been reviewed. More investigations are required to review accepted but unpublished studies and publications in 2022.
Table Table1Table 1
Declarations
The authors declare that they have no competing interests.
1 http://www.duplichecker.com
2 http://www.turnitin.com
Publisher's note
Springer Nature remains neutral with regard to jurisdictional claims in published maps and institutional affiliations.
Contributor Information
Fakhroddin Noorbehbahani, Email: ri.ca.iu.gne@inahabhebroon .
Azadeh Mohammadi, Email: [email protected] .
Mohammad Aminazadeh, Email: [email protected] , Email: [email protected] .
- Aisyah, S., Bandung, Y., & Subekti, L. B. (2018). Development of Continuous Authentication System on Android-Based Online Exam Application. In 2018 International Conference on Information Technology Systems and Innovation, ICITSI 2018 (pp. 171–176). Padang, Indonesia: IEEE. 10.1109/ICITSI.2018.8695954
- Alessio H. The Impact of Video Proctoring in Online Courses. Journal on Excellence in Col- Lege Teaching. 2018; 29 (3):1–10. [ Google Scholar ]
- Alessio HM, Malay N, Maurer K, Bailer AJ, Rubin B. Examining the Effect of Proctoring on Online Test Scores. Online Learning. 2017; 2013 (1):1–16. [ Google Scholar ]
- Amigud A, Lancaster T. 246 reasons to cheat: An analysis of students’ reasons for seeking to outsource academic work. Computers and Education. 2019; 134 :98–107. doi: 10.1016/j.compedu.2019.01.017. [ CrossRef ] [ Google Scholar ]
- Arnautovski, L. (2019). Face recognition technology in the exam identity authentication system - implementation concept. In 2nd International Scientific Conference MILCON’19 (pp. 51–56). Olsztyn, Poland.
- Atoum Y, Chen L, Liu AX, Hsu SDH, Liu X. Automated Online Exam Proctoring. IEEE Transactions on Multimedia. 2017; 19 (7):1609–1624. doi: 10.1109/TMM.2017.2656064. [ CrossRef ] [ Google Scholar ]
- Backman, J. (2019). Student s ’ Experiences of Cheating in the Online Exam Environment.
- Bawarith, H. R. (2017). Student Cheating Detection System in E-exams . KING ABDULAZIZ UNIVERSITY.
- Bawarith R, Basuhail A, Fattouh A, Gamalel-din PS. E-exam Cheating Detection System. International Journal of Advanced Computer Science and Applications. 2017; 8 (4):176–181. doi: 10.14569/IJACSA.2017.080425. [ CrossRef ] [ Google Scholar ]
- Bilen E, Matros A. Online Cheating Amid COVID-19. Journal of Economic Behavior & Organization. 2021; 182 :196–211. doi: 10.1016/j.jebo.2020.12.004. [ PMC free article ] [ PubMed ] [ CrossRef ] [ Google Scholar ]
- Chirumamilla, A., & Sindre, G. (2019). Mitigation of Cheating in Online Exams: Strengths and Limitations of. In Biometric Authentication in Online Learning Environments (pp. 47–68). IGI Global. 10.4018/978-1-5225-7724-9.ch003
- Chua, S. S., & Lumapas, Z. R. (2019). Online Examination System with Cheating Prevention Using Question Bank Randomization and Tab Locking. 2019 4th International Conference on Information Technology (InCIT) , 126–131.
- Chuang CY, Craig SD, Femiani J. Detecting probable cheating during online assessments based on time delay and head pose. Higher Education Research and Development. 2017; 36 (6):1123–1137. doi: 10.1080/07294360.2017.1303456. [ CrossRef ] [ Google Scholar ]
- Cluskey GR, Jr, Ehlen CR, Raiborn MH. Thwarting Online Exam Cheating without Proctor Supervision. 2011; 4 :1–7. [ Google Scholar ]
- Corrigan-Gibbs, H., Gupta, N., Northcutt, C., Cutrell, E., & Thies, W. (2015). Deterring cheating in online environments. ACM Transactions on Computer-Human Interaction , 22 (6). 10.1145/2810239
- Cote, M., Jean, F., Albu, A. B., & Capson, D. (2016). Video Summarization for Remote Invigilation of Online Exams. In 2016 IEEE Winter Conference on Applications of Computer Vision (pp. 1–9). NY, USA.
- Curran K, Middleton G, Doherty C. Cheating in Exams with Technology. International Journal of Cyber Ethics in Education. 2011; 1 (2):54–62. doi: 10.4018/ijcee.2011040105. [ CrossRef ] [ Google Scholar ]
- Dendir S, Maxwell RS. Cheating in online courses: Evidence from online proctoring. Computers in Human Behavior Reports. 2020; 2 :100033. doi: 10.1016/j.chbr.2020.100033. [ CrossRef ] [ Google Scholar ]
- Diedenhofen B, Musch J. PageFocus: Using paradata to detect and prevent cheating on online achievement tests. Behavior Research Methods. 2017; 49 (4):1444–1459. doi: 10.3758/s13428-016-0800-7. [ PubMed ] [ CrossRef ] [ Google Scholar ]
- Dobrovska D. Technical Student Electronic Cheating on Examination. In: Auer ME, Guralnick D, Uhomoibhi J, editors. Interactive Collaborative Learning. Springer International Publishing; 2017. pp. 525–531. [ Google Scholar ]
- Fan, Z., Xu, J., Liu, W., & Cheng, W. (2016). Gesture based Misbehavior Detection in Online Examination. In The 11th International Conference on Computer Science & Education (pp. 234–238). NagoyaF, Japan.
- Fayyoumi A, Zarrad A. Novel Solution Based on Face Recognition to Address Identity Theft and Cheating in Online Examination Systems. Advances in Internet of Things. 2014; 4 (April):5–12. doi: 10.4236/ait.2014.42002. [ CrossRef ] [ Google Scholar ]
- Fluck AE. An international review of eExam technologies and impact. Computers & Education. 2019; 132 :1–15. doi: 10.1016/j.compedu.2018.12.008. [ CrossRef ] [ Google Scholar ]
- Fontaine S, Frenette E, Hébert M. Exam cheating among Quebec’s preservice teachers : the influencing factors. International Journal for Educational Integrity. 2020; 16 (14):1–18. [ Google Scholar ]
- Garg, K., Verma, K., Patidar, K., Tejra, N., & Petidar, K. (2020). Convolutional Neural Network based Virtual Exam Controller. In Proceedings of the International Conference on Intelligent Computing and Control Systems, ICICCS 2020 (pp. 895–899). Secunderabad, India. 10.1109/ICICCS48265.2020.9120966
- Gruenigen, D. Von, de Azevedo e Souza, F. B., Pradarelli, B., Magid, A., & Cieliebak, M. (2018). Best practices in e-assessments with a special focus on cheating prevention. In 2018 {IEEE} Global Engineering Education Conference, {EDUCON} 2018, Santa Cruz de Tenerife, Tenerife, Islas Canarias, Spain, April 17-20, 2018 (pp. 893–899). IEEE. 10.1109/EDUCON.2018.8363325
- He, H., Zheng, Q., Li, R., & Dong, B. (2018). Using Face Recognition to Detect “ Ghost Writer ” Cheating in Examination. In Edutainment, Lecture Notes in Computer Science (Vol. 11462, pp. 389–397). Springer International Publishing. 10.1007/978-3-030-23712-7
- Holden, O., Kuhlmeier, V., & Norris, M. (2020). Academic Integrity in Online Testing: A Research Review. 10.31234/osf.io/rjk7g
- Hu, S., Jia, X., & Fu, Y. (2018). Research on Abnormal Behavior Detection of Online Examination Based on Image Information. In 10th International Conference on Intelligent Human-Machine Systems and Cybernetics (IHMSC) (Vol. 02, pp. 88–91). Hangzhou, China: IEEE. 10.1109/IHMSC.2018.10127
- Hylton K, Levy Y, Dringus LP. Computers & Education Utilizing webcam-based proctoring to deter misconduct in online exams. Computers & Education. 2016; 92–93 :53–63. doi: 10.1016/j.compedu.2015.10.002. [ CrossRef ] [ Google Scholar ]
- Idemudia, S., Rohani, M. F., Siraj, M., & Othman, S. H. (2016). A Smart Approach of E-Exam Assessment Method Using Face Recognition to Address Identity Theft and Cheating. International Journal of Computer Science and Information Security , 14 (10), 515–522. Retrieved from https://sites.google.com/site/ijcsis/
- Jalali, K., & Noorbehbahani, F. (2017). An Automatic Method for Cheating Detection in Online Exams by Processing the Students Webcam Images. In 3rd Conference on Electrical and Computer Engineering Technology (E-Tech 2017), Tehran, Iran (pp. 1–6). Tehran, Iran.
- Kasliwal, G. (2015). Cheating Detection in Online Examinations.
- Kigwana, I., & Venter, H. (2016). Proposed high-level solutions to counter online examination fraud using digital forensic readiness techniques. Proceedings of the 11th International Conference on Cyber Warfare and Security, ICCWS 2016 , 407–414.
- Korman, M. (2010). Behavioral detection of cheating in online examination. Retrieved from https://pure.ltu.se/ws/files/31188849/LTU-DUPP-10112-SE.pdf
- Lancaster, T., & Clarke, R. (2017). Rethinking Assessment By Examination in the Age of Contract Cheating. Plagiarism Across Europe and Beyond 2017 .
- Li, M., Sikdar, S., Xia, L., & Wang, G. (2020). Anti-cheating Online Exams by Minimizing the Cheating Gain, (May). 10.20944/preprints202005.0502.v1
- Li, X., Yueran, K. C., & Alexander, Y. (2015). Massive Open Online Proctor : Protecting the Credibility of MOOCs Certificates, 1129–1137.
- Maeda, M. (2019). Exam cheating among Cambodian students : when , how , and why it happens. Compare: A Journal of Comparative and International Education , 1–19. 10.1080/03057925.2019.1613344
- Manoharan S. Cheat-resistant multiple-choice examinations using personalization. Computers and Education. 2019; 130 :139–151. doi: 10.1016/j.compedu.2018.11.007. [ CrossRef ] [ Google Scholar ]
- Martin F, Sun T, Westine CD. A systematic review of research on online teaching and learning from 2009 to 2018. Computers & Education. 2020; 159 :104009. doi: 10.1016/j.compedu.2020.104009. [ PMC free article ] [ PubMed ] [ CrossRef ] [ Google Scholar ]
- Mengash, H. (2019). Automated Detection for Student Cheating During Written Exams: An Updated Algorithm Supported by Biometric of Intent. In First International Conference on Computing (pp. 303–3111). Riyadh, Saudi Arabia. 10.1007/978-3-030-36368-0
- Migut, G., Koelma, D., Snoek, C. G., & Brouwer, N. (2018). Cheat Me Not: Automated Proctoring Of Digital Exams On Bring-Your-Own-Device. In The 23rd Annual ACM Conference On In- novation And Technology In Computer Science Education (p. 388). New York, NY, USA.
- Moten JM, Jr, Fitterer A, Brazier E, Leonard J, Brown A, Texas A. Examining Online College Cyber Cheating Methods and Prevention Measures. Electronic Journal of E-Learning. 2013; 11 (2):139–146. [ Google Scholar ]
- Mott JH. The Detection and Minimization of Cheating During Concurrent Online Assessments Using Statistical Methods. Collegiate Aviation Review. 2010; 28 (2):32–46. [ Google Scholar ]
- Nguyen, J. G., Keuseman, K. J., & Humston, J. J. (2020). Minimize Online Cheating for Online Assessments During COVID-19 Pandemic. 10.1021/acs.jchemed.0c00790
- Nikou SA, Economides AA. Mobile-based assessment: A literature review of publications in major referred journals from 2009 to 2018. Computers & Education. 2018; 125 :101–119. doi: 10.1016/j.compedu.2018.06.006. [ CrossRef ] [ Google Scholar ]
- Noorbehbahani, F., Salehi, F., & Jafar Zadeh, R. (2019). A systematic mapping study on gamification applied to e-marketing. Journal of Research in Interactive Marketing , 13 (3). 10.1108/JRIM-08-2018-0103
- Norris M. University online cheating - how to mitigate the damage. Research in Higher Education Journal. 2019; 37 :1–20. [ Google Scholar ]
- Opgen-Rhein, J., Küppers, B., & Schroeder, U. (2018). An application to discover cheating in digital exams. In ACM International Conference Proceeding Series . Koli, Finland. 10.1145/3279720.3279740
- Page, M. J., Moher, D., Bossuyt, P. M., Boutron, I., Hoffmann, T. C., Mulrow, C. D., & Mckenzie, J. E. (2021). PRISMA 2020 explanation and elaboration: Updated guidance and exemplars for reporting systematic reviews. BMJ, 372 ,. 10.1136/bmj.n160 [ PMC free article ] [ PubMed ]
- Parks RF, Lowry PB, Wigand RT, Agarwal N, Williams TL. Why students engage in cyber-cheating through a collective movement: A case of deviance and collusion. Computers and Education. 2018; 125 :308–326. doi: 10.1016/j.compedu.2018.04.003. [ CrossRef ] [ Google Scholar ]
- Peytcheva-Forsyth, R., Aleksieva, L., & Yovkova, B. (2018). The impact of technology on cheating and plagiarism in the assessment – The teachers’ and students’ perspectives. In AIP Conference Proceedings 2048 (Vol. 020037, pp. 1–11).
- Prathish, S., Athi Narayanan, S., & Bijlani, K. (2016). An intelligent system for online exam monitoring. In Proceedings - 2016 International Conference on Information Science, ICIS 2016 (pp. 138–143). Dublin, Ireland. 10.1109/INFOSCI.2016.7845315
- Reisenwitz TH. Examining the Necessity of Proctoring Online Exams. Journal of Higher Education Theory and Practice. 2020; 20 (1):118–124. [ Google Scholar ]
- Saba T, Rehman A, Jamail NSM, Marie-Sainte SL, Raza M, Sharif M. Categorizing the Students’ Activities for Automated Exam Proctoring Using Proposed Deep L2-GraftNet CNN Network and ASO Based Feature Selection Approach. IEEE Access. 2021; 9 :47639–47656. doi: 10.1109/ACCESS.2021.3068223. [ CrossRef ] [ Google Scholar ]
- Sabbah, Y. W. (2017). Security of Online Examinations. In Data Analytics and Decision Support for Cybersecurity (pp. 157–200). Springer International Publishing.
- Srikanth M, Asmatulu R. Modern Cheating Techniques, Their Adverse Effects on Engineering Education and preventions. International Journal of Mechanical Engineering Education. 2014; 42 (2):129–140. doi: 10.7227/IJMEE.0005. [ CrossRef ] [ Google Scholar ]
- Tiong, L. C. O., & Lee, H. J. (2021). E-cheating Prevention Measures: Detection of Cheating at Online Examinations Using Deep Learning Approach -- A Case Study, XX (Xx), 1–9. Retrieved from http://arxiv.org/abs/2101.09841
- Topîrceanu A. Breaking up friendships in exams: A case study for minimizing student cheating in higher education using social network analysis. Computers and Education. 2017; 115 :171–187. doi: 10.1016/j.compedu.2017.08.008. [ CrossRef ] [ Google Scholar ]
- Traore, I., Nakkabi, Y., Saad, S., & Sayed, B. (2017). Ensuring Online Exam Integrity Through Continuous Biometric Authentication. In Information Security Practices (pp. 73–81). Springer International Publishing. 10.1007/978-3-319-48947-6
- Turner, S. W., & Uludag, S. (2013). Student perceptions of cheating in online and traditional classes. Proceedings - Frontiers in Education Conference, FIE , (October 2013), 1131–1137. 10.1109/FIE.2013.6685007
- Ullah, A. (2016). Security and Usability of Authentication by Challenge Questions in Online Examination . University of Hertfordshire.
- Valverde-Berrocoso, J., Garrido-Arroyo, M. del C., Burgos-Videla, C., & Morales-Cevallos, M. B. (2020). Trends in Educational Research about e-Learning: A Systematic Literature Review (2009–2018). Sustainability , 12 (12). 10.3390/su12125153
- Varble, D. (2014). Reducing Cheating Opportunities in Online Test Online Tests, 3 (3).
- Watson, G., & Sottile, J. (2010). Cheating in the Digital Age: Do Students Cheat More in Online Courses?. Online Journal of Distance Learning Administration , 13 (1).
- Wei X, Saab N, Admiraal W. Assessment of cognitive, behavioral, and affective learning outcomes in massive open online courses: A systematic literature review. Computers & Education. 2021; 163 :104097. doi: 10.1016/j.compedu.2020.104097. [ CrossRef ] [ Google Scholar ]
- Weiner JA, Hurtz GM. A comparative Study of Online Remote Proctored Vs Onsite Proctored. Journal of Applied Testing Technology. 2017; 18 (1):13–20. [ Google Scholar ]
- Wong, S., Yang, L., Riecke, B., Cramer, E., & Neustaedter, C. (2017). Assessing the usability of smartwatches for academic cheating during exams. Proceedings of the 19th International Conference on Human-Computer Interaction with Mobile Devices and Services, MobileHCI 2017 . 10.1145/3098279.3098568
- Xiong Y, Suen HK. Assessment approaches in massive open online courses: Possibilities, challenges and future directions. International Review of Education. 2018; 64 (2):241–263. doi: 10.1007/s11159-018-9710-5. [ CrossRef ] [ Google Scholar ]
Two professors who say they caught students cheating on essays with ChatGPT explain why AI plagiarism can be hard to prove
- Two philosopher professors said they caught their students submitting essays written by ChatGPT.
- They said certain red flags alerted them to the use of AI.
- If students don't confess to using the program, professors say it can be hard to prove.

A few weeks after the launch of the AI chatbot ChatGPT , Darren Hick, a philosophy professor at Furman University, said he caught a student turning in an AI-generated essay .
Hick said he grew suspicious when the student turned in an on-topic essay that included some well-written misinformation.
After running it through Open AI's ChatGPT detector , the results said it was 99% likely the essay had been AI-generated.
Antony Aumann, a religious studies and philosophy professor at Northern Michigan University, told Insider he had caught two students submitting essays written by ChatGPT .
After the writing style set off alarm bells, Aumann submitted them back to the chatbot asking how likely it was that they were written by the program. When the chatbot said it was 99% sure the essays were written by ChatGPT, he forwarded the results to the students.
Both Hick and Aumann said they confronted their students, all of whom eventually confessed to the infraction. Hick's student failed the class and Aumann had his students rewrite the essays from scratch.
'It was really well-written wrong'
There were certain red flags in the essays that alerted the professors to the use of AI. Hick said the essay he found referenced several facts not mentioned in class, and made one nonsensical claim.
"Word by word it was a well-written essay," he said, but on closer inspection, one claim about the prolific philosopher, David Hume "made no sense" and was "just flatly wrong."
"Really well-written wrong was the biggest red flag," he said.
For Aumann, the chatbot just wrote too perfectly. "I think the chat writes better than 95% of my students could ever," he said.
Related stories
"All of a sudden you have someone who does not demonstrate the ability to think or write at that level, writing something that follows all the requirements perfectly with sophisticated grammar and complicated thoughts that are directly related to the prompt for the essay," he said.
Christopher Bartel, a professor of philosophy at Appalachian State University, said that while the grammar in AI-generated essays is almost perfect, the substance tends to lack detail.
He said: "They are really fluffy. There's no context, there's no depth or insight."
Hard-to-prove plagiarism
If students don't confess to using AI for essays, it can leave academics in a tough spot.
Bartel said that some institutions' rules haven't evolved to combat this kind of cheating. If a student decided to dig their heels in and deny the use of AI, it can be difficult to prove.
Bartel said the AI detectors on offer were "good but not perfect."
"They give a statistical analysis of how likely the text is to be AI-generated, so that leaves us in a difficult position if our policies are designed so that we have to have definitive and demonstrable proof that the essay is a fake," he said. "If it comes back with a 95% likelihood that the essay is AI generated, there's still a 5% chance that it wasn't."
In Hick's case, although the detection site said it was "99% certain" the essay had been generated by an AI, he said it wasn't enough for him without a confession.
"The confession was important because everything else looks like circumstantial evidence," he said. "With AI-generated content, there is no material evidence, and material evidence has a lot more weight to it than circumstantial evidence."
Aumann said although he thought the analysis by the chatbot would be good enough proof for disciplinary action, AI plagiarism was still a new challenge for colleges.
He said: "Unlike plagiarism cases of old where you can just say, 'hey, here's the paragraph from Wikipedia.' There is no knockdown proof that you can provide other than the chat says that's the statistical likelihood."
- Main content

IMAGES
VIDEO
COMMENTS
Easy Anti-Cheat™ is the industry-leading anti-cheat service, countering hacking and cheating in multiplayer PC games through the use of hybrid anti-cheat mechanisms. Pioneering security Our approach is constantly evolving, which results in fewer hacking attempts, no false positives, and a healthier, more enjoyable community.
Free AI Detector - Gemini, GPT4 and ChatGPT Detector
The Best AI Detection Tools to Catch Cheating and ...
AI Essay Checker: Detect AI Content, Plagiarism & Fix Grammar
Free AI Detector
For example, when a student turns in an essay, a product like Turnitin scans the submitted essay against a huge library of essays in its database, and other documents and text on the internet to ...
AI Cheating: Tips to Avoid and Detect
Free AI-Powered Essay and Paper Checker ...
When comparing the same two ChatGPT-written essays on OpenAI's text classifier and Tian's GPTZero — three paragraphs on the dangers of plagiarism — the text classifier said the text was ...
Plagiarism Checker Free | Accurate with Percentage
GPT Essay Checker - Detect AI-Generated Content Easily ...
While anti-cheating software makes it easier for schools to conduct digital proctoring through computer cameras used to observe students while taking exams, essay mills continue to exist. These allow students to pay others to write papers for them and turn in the work as their own.
AI Writing Detection | AI Tools
Exclusive: Australian schools and universities will be armed with new tech to catch students trying to cheat with ChatGPT, the advanced artificial intelligence chatbot which can write essays on ...
"The essay confidently and thoroughly described Hume's views on the paradox of horror in (ways) that were thoroughly wrong," Darren Hick, an assistant professor of philosophy, explained in a ...
Five high school students helped our tech columnist test a ChatGPT detector coming from Turnitin to 2.1 million teachers. It missed enough to get someone in trouble. Lucy Goetz, a student at ...
Advocates for the worst-case scenario see a future in which human-generated and computer-generated text are indistinguishable, essay assignments are meaningless, and the very skill of academic writing is lost. One need not accept this doomsday proposition to recognize that ChatGPT raises legitimate concerns for academic integrity.
Essay Checker: Free Grammar & Plagiarism Check
There's a Tool to Catch Students Cheating With ChatGPT. OpenAI Hasn't Released It. Technology that can detect text written by artificial intelligence with 99.9% certainty has been debated ...
That created an awkward situation in many classrooms. It turns out, it's easy to get caught cheating with generative A.I. because it is prone to making stuff up, a phenomena known as ...
In cases where several papers reported the same study, only the most recent ones were included (i.e., theses and papers extracted from theses, extended version of papers published in journals). ... (Cheat OR e-Cheating OR Fraud OR Dishonesty OR Anti-cheating OR Cheat-resistant OR Abnormal behavior OR Misconduct OR Integrity OR Plagiarism) AND ...
A systematic review of research on cheating in online ...
Professors Caught Students Cheating on College Essays ...