- Professuren
- Forschungsschwerpunkte
- Forschungsthemen
- Forschungsprojekte
- Publikationen
- Lehrbereiche
- Bachelorarbeiten
- Masterarbeiten
- Sonstige Arbeiten
- Transferbereiche
- Wissenstransfer
- Transferprojekte
- Weiterbildung
- Stellenangebote

Complex problem solving and intelligence: A meta-analysis
Allgemeines.
Art der Publikation : Journal Article
Veröffentlicht auf / in : Intellligence
Jahr : 2015
Band / Volume : 53
Seiten : 92-101
DOI : https://doi.org/10.1016/j.intell.2015.09.005
ISSN : 0160-2896
Matthias Stadler
Nicolas Becker
Markus Gödker
Detlev Leutner
Samuel Greiff
Zusammenfassung

Zitation kopiert

An official website of the United States government
The .gov means it’s official. Federal government websites often end in .gov or .mil. Before sharing sensitive information, make sure you’re on a federal government site.
The site is secure. The https:// ensures that you are connecting to the official website and that any information you provide is encrypted and transmitted securely.
- Publications
- Account settings
Preview improvements coming to the PMC website in October 2024. Learn More or Try it out now .
- Advanced Search
- Journal List
- Front Psychol
The Role of Motivation in Complex Problem Solving
C. dominik güss.
1 Department of Psychology, University of North Florida, Jacksonville, FL, United States
Madison Lee Burger
Dietrich dörner.
2 Trimberg Research Academy, University of Bamberg, Bamberg, Germany
The role of motivation in complex problem solving
Previous research on Complex Problem Solving (CPS) has primarily focused on cognitive factors as outlined below. The current paper discusses the role of motivation during CPS and argues that motivation, emotion, and cognition interact and cannot be studied in an isolated manner. Motivation is the process that determines the energization and direction of behavior (Heckhausen, 1991 ).
Three motivation theories and their relation to CPS are examined: McClelland's achievement motivation, Maslow's hierarchy of needs, and Dörner's needs as outlined in PSI-theory. We chose these three theories for several reasons. First, space forces us to be selective. Second, the three theories are among the most prominent motivational theories. Finally, they are need theories postulating several motivations and not just one. A thinking-aloud protocol is provided to illustrate the role of motivational and cognitive dynamics in CPS.
Problems are part of all the domains of human life. The field of CPS investigates problems that are complex, dynamic, and non-transparent (Dörner, 1996 ). Complex problems consist of many interactively interrelated variables. Dynamic ones change and develop further over time, regardless of whether the involved people take action. And non-transparent problems have many aspects of the problem situation that are unclear or unknown to the involved people.
CPS researchers focus exactly on such kinds of problems. Under a narrow perspective, CPS can be defined as thinking that aims to overcome barriers and to reach goals in situations that are complex, dynamic, and non-transparent (Frensch and Funke, 1995 ). Indeed, past research has shown the influential role of task properties (Berry and Broadbent, 1984 ; Funke, 1985 ) and of cognitive factors on CPS strategies and performance, such as intelligence (e.g., Süß, 2001 ; Stadler et al., 2015 ), domain-specific knowledge (e.g., Wenke et al., 2005 ), cognitive biases and errors (e.g., Dörner, 1996 ; Güss et al., 2015 ), or self-reflection (e.g., Donovan et al., 2015 ).
Under a broader perspective, CPS can be defined as the study of cognitive, emotional, motivational, and social processes when people are confronted with such complex, dynamic and non-transparent problem situations (Schoppek and Putz-Osterloh, 2003 ; Dörner and Güss, 2011 , 2013 ; Funke, 2012 ). The assumption here is that focusing solely on cognitive processes reveals an incomplete picture or an inaccurate one.
To study CPS, researchers have often used computer-simulated problem scenarios also called microworlds or virtual environments or strategy games. In these situations, participants are confronted with a complex problem simulated on the computer from which they gather information, and identify solutions. These decisions are then implemented into the system and result in changes to the problem situation.
Previous research on motivation and CPS
The idea to study the interaction of motivation, emotion, and cognition is not new (Simon, 1967 ). However, in practice this has been rarely examined in the field of CPS. One study assessed the need for cognition (i.e., the tendency to engage in thinking and reflecting) and showed how high need of cognition was related to broader information collection and better performance in a management simulation (Nair and Ramnarayan, 2000 ).
Vollmeyer and Rheinberg ( 1999 , 2000 ) explored in two studies the role of motivational factors in CPS. They assessed mastery confidence (similar to self-efficacy), incompetence fear, interest, and challenge as motivational factors. Their results demonstrated that mastery confidence and incompetence fear were good predictors for learning and for knowledge acquisition.
CPS assessment
Before we describe three theories of motivation and how they might be related and applicable to CPS, we will briefly describe the WINFIRE computer simulation (Gerdes et al., 1993 ; Schaub, 2009 ) and provide a part of a thinking-aloud protocol of one participant while working on WINFIRE. WINFIRE is the simulation of small cities surrounded by forests. Participants take the role of fire-fighting commanders who try to protect cities and forests from approaching fires. Participants can give a series of commands to several fire trucks and helicopters. In WINFIRE quick decisions and multitasking are required in order to avoid fires spreading. In one study, participants were also instructed to think aloud, i.e., to say aloud everything that went through their minds while working on WINFIRE. These thinking-aloud protocols, also called verbal protocols, were audiotaped and transcribed in five countries and compared (see Güss et al., 2010 ).
The following is a verbatim WINFIRE thinking-aloud protocol of a US participant (Güss et al., 2010 ):
Ok, I don't see any fires yet. I'm trying to figure out how the helicopters pick up the water from the ponds. I put helicopters on patrol mode. Not really sure what that does. It doesn't seem to be moving. Oh, there it goes, it's moving…I guess you have to wait till there's a fire showing…Ok, fire just started in the middle, so I have to get some people to extinguish it. Ok, now I have another fire going here. I'm in trouble here. Ok. Ok, when I click extinguish, it don't seem to respond. Guess I'm not clear how to get trucks right to the fire. Ok, one fire has been extinguished, but a new one started in the same area. I'm getting more trucks out there trying to figure out, how to get helicopters to the pond. I still haven't figured that out, because they have to pick up the water. Ok, got a pretty good fire going here, so I'm going to put all the trucks on action, ok, water thing is making me mad. Ok. I'm not sure how it goes? Ok, the forest is burning up now—extinguish! Ok, ok, I'm in big trouble here…
Psychological theories of motivation and their application to CPS
Mcclelland's human motivation theory.
In his Human Motivation theory, McClelland distinguishes three needs (power, affiliation, and achievement) and argues that human motivation is a response to changes in affective states. A specific situation will cause a change in the affective state through the non-specific response of the autonomic nervous system. This response will motivate a person toward a goal to reach a different affective state (McClelland et al., 1953 ). An affective state may either be positive or negative, determining the direction of motivated behavior as either approach oriented, i.e., to maintain the state, or avoidance oriented, i.e., to avoid or discontinue the state (McClelland et al., 1953 ).
Motivation intensity varies among individuals based on perception of the stimulus and the adaptive abilities of the individual. Hence, when a discrepancy exists between expectation and perception, then a person will be motivated to eliminate this discrepancy (McClelland et al., 1953 ). In the statement from the thinking-aloud protocol we can infer the participant's achievement motivation, “ Guess I'm not clear how to get trucks right to the fire. Ok, one fire has been extinguished, but a new one started in the same area.” The participant at first begins to give up and reduce effort, but then achieves a step toward the goal. This achievement causes the reevaluation of the discrepancy between ability and the goal as not too large to overcome. This realization motivates the participant to continue working through the scenario. Whereas, the need for achievement seems to guide CPS, the needs for power and affiliation cannot be observed in the current thinking-aloud protocol.
Based on the previous discussion we can derive the following predictions:
- Prediction 1 : Approach-orientation will lead to greater engagement in CPS compared to avoidance-orientation.
- Prediction 2 : Based on an individual's experience either power, affiliation, or achievement will become dominant and guide the strategic approach in CPS.
Maslow's hierarchy of needs
Maslow's Hierarchy of Needs (Maslow, 1943 , 1954 ) suggests that everyone has five basic needs that act as motivating forces in a person's life. Maslow's hierarchy takes the form of a pyramid in which needs lower in the pyramid are primary motivators. They have to be met before higher needs can become motivating forces. At the bottom of the pyramid are the most basic needs beginning with physiological needs, such as hunger, and followed by safety needs. Then follow the psychological needs of belongingness and love, and then esteem. Once these four groups of needs have been met, a person may reach the self-fulfillment stage of self-actualization at which time a person can be motivated to achieve ones full potential (Maslow, 1943 ).
The first four groups of needs are external motivators because they motivate through both deficiency and fulfillment. In essence, a person fulfills a need which then releases the next unsatisfied need to be the dominant motivator (Maslow, 1943 , 1954 ). The safety need is often understood as seeking shelter, but Maslow also understands safety also as wanting “a predictable, orderly world” (Maslow, 1943 , p. 377), “an organized world rather than an unorganized or unstructured one” (Maslow, 1943 , p. 377). Safety refers to the “common preference for familiar rather than unfamiliar things” (Maslow, 1943 , p. 379).
In this sense the safety need becomes active when the person does not understand what is happening in the microworld, as the following passage of the thinking-aloud protocol illustrates. “ I put helicopters on patrol mode. Not really sure what that does. It doesn't seem to be moving.” The safety need is demonstrated in the person's desire for organization, since unknown and unexpected events are seen as threats to safety.
The esteem need as a motivator becomes evident through the statement, “ Guess I'm not clear how to get trucks right to the fire.” The participant becomes aware of his inability to control the situation which affects his self-esteem. The esteem need is never fulfilled in the described situation and remains the primary motivator. The following statements show how affected the participant's esteem need is by the inability to control the burning fires. “ Ok. I'm not sure how it goes? Ok, the forest is burning up now—extinguish! Ok, ok, I'm in big trouble .”
- Prediction 3 : A strong safety need will be related to elaborate and detailed information collection in CPS compared to low safety need.
- Prediction 4 : People with high esteem needs will be affected more by difficulties in CPS and engage more often in behaviors to protect their esteem compared to people with low esteem needs.
Dörner's theory of motivation as part of PSI-theory
PSI-theory described the interaction of cognitive, emotional, and motivational processes (Dörner, 2003 ; Dörner and Güss, 2011 ). Only a small part of the theory is examined here. Briefly, the theory encompasses five basic human needs: the existential needs (thirst, hunger, and pain avoidance), the sexuality need, and the social need for affiliation (group binding), the need for certainty (predictability), and the need for competence (mastery). If the environment is unpredictable, the certainty need becomes active. If we are not able to cope with problems, the competence need becomes active. The need for competence also becomes active when any other need becomes activated. With an increase in needs, the arousal increases.
The first three needs cannot be observed or inferred from the thinking-aloud protocol provided. Statements like, “I'm trying to figure out how the helicopters pick up the water from the ponds.” and “Guess I'm not clear how to get trucks right to the fire,” demonstrate the needs for certainty and competence, i.e., to make the environment predictable and controllable.
The following statements reflect the participant's need for competence, i.e., the inefficacy or incapability of coping with problems. “ I'm in trouble here…ok, water thing is making me mad .” Not being able to extinguish the fires that are approaching cities and are destroying forests is experienced as anger. The arousal rises as the resolution level of thinking decreases. So, the participant does not think about different options in an elaborate manner. Yet, the participant becomes aware of his failure. The competence need then causes the participant to search for possible solutions, “ I still haven't figured that out because they have to pick up the water…” The need for competence is satisfied when the problem solver is able to change either the environment or ones views of the environment.
- Prediction 5 : A strong certainty need is positively related to a strong competence need.
- Prediction 6 : High need for certainty paired with high need for competence can lead to safeguarding behavior, i.e., background monitoring.
- Prediction 7 : An increase in the competence and uncertainty needs leads to increased arousal and a lower resolution level of thinking. CPS becomes one-dimensional and possible long-term and side-effects are not considered adequately.
Summary and evaluation
We have briefly discussed three motivation theories and their relation to CPS referring to one thinking-aloud protocol: McClelland's achievement motivation, Maslow's hierarchy of needs, and Dörner's needs as outlined in PSI-theory.
A Comparison of Three Need Theories in the Context of CPS.
Scope/ Breadth | + | ++ | ++ |
Applicability to CPS | + | + | ++ |
Adaptability of needs | − | − | + |
Incorporation of emotion | ++ | − | ++ |
Individual differences | ++ | − | ++ |
. |
Comparing the scope of the three theories and referring to the scope and different needs covered in the three theories, McClelland's theory describes three needs (power, affiliation, and achievement), Maslow's theory describes five groups of needs (physiological, safety, love and belonging, esteem, self-actualization), and Dörner's theory describes five different needs (existential, sexuality, affiliation, certainty, and competence).
All three theories can be applied to CPS. McClelland's need for achievement, Maslow's needs for esteem and safety, and Dörner's needs for certainty and competence could be inferred from the thinking–aloud passage. The need for affiliation which is a part of each of the three theories could play an important role when groups solve complex problems.
The existential needs and the need for affiliation outlined in PSI-theory can also be found in Maslow's hierarchy of needs. These two theories differ in the adaptability of the needs. However, Maslow's esteem needs are only activated as the primary motivator as the physiological needs, belongingness, and love needs are met. The needs are more fluidly described as motivators in PSI-theory. One need becomes the dominant motive according to the expectancy–value principle. Expectancy stands for the estimated likelihood of success. The value of a motive stands for the strength of the need. According to McClelland's theory, the role of three motivations develops through life experience in a specific culture; and often times, one of the three becomes the main driving force for a person, almost like a personality trait. In that sense, there is not much flexibility.
Motivation and emotion are closely related as became partially clear in the discussion of McClelland's theory. Emotions are discussed in detail in PSI-theory, but space does not allow us to discuss those in detail here (see Dörner, 2003 ). Emotions are not described in detail in Maslow's Hierarchy of Needs.
Individual differences in motivation and needs are discussed in two of the three theories. According to McClelland, a person develops an individual achievement motive by learning one's own abilities from past achievements and failures. Based on different learning histories, different persons will have a different dominant motivation guiding behavior in a given situation. Learning history also influences the competence need in PSI-theory. Additionally PSI-theory assumes individual differences that are simulated through different individual motivational parameters in the theory. The certainty need, for example, becomes active when there is a deviation from a given set point. Individual differences are related to different set points and how sensitive the deviations are (e.g., deviation starts quickly vs. deviation starts slowly).
The thinking-aloud example from the WINFIRE microworld described earlier demonstrates that a person's CPS process is influenced by the person's needs. We have focused in our discussion on motivational processes that are considered in the framework of need theories. Beyond that, other motivational theories exist that focus on the importance of motivation for learning and achievement (e.g., expectancy, reasons for engagement, see Eccles and Wigfield, 2002 ). Thus, the applicability of these theories to CPS could be explored in future studies as well.
We discussed the three motivational theories of McClelland's Achievement Motivation, Maslow's Hierarchy of Need, and Dörner's Theory of Motivation as part of PSI-Theory. Although, the theories differ our discussion has shown that the three theories can be applied to CPS. Problem solving is a motivated process and determined by human motivations and needs.
Author contributions
The first author CG conceptualized the manuscript, selected the thinking-aloud passage, the second author MB primarily summarized McClellands and Maslow's theories. All authors contributed to writing up the manuscript.
Conflict of interest statement
The authors declare that the research was conducted in the absence of any commercial or financial relationships that could be construed as a potential conflict of interest.
- Berry D. C., Broadbent D. E. (1984). On the relationship between task performance and associated verbalizable knowledge . Q. J. Exp. Psychol. 36A , 209–231. 10.1080/14640748408402156 [ CrossRef ] [ Google Scholar ]
- Donovan S., Güss C. D., Naslund D. (2015). Improving dynamic decision making through training and self-reflection . Judgm. Decis. Making 10 , 284–295. [ Google Scholar ]
- Dörner D. (1996). The logic of failure. Recognizing and Avoiding Error in Complex Situations . New York, NY: Basic Books. [ Google Scholar ]
- Dörner D. (2003). Bauplan für eine Seele [Blueprint for a soul] . Reinbek: Rowohlt. [ Google Scholar ]
- Dörner D., Güss C. D. (2011). A psychological analysis of Adolf Hitler's decision making as Commander in Chief: summa confidentia et nimius metus . Rev. Gen. Psychol. 15 , 37–49. 10.1037/a0022375 [ CrossRef ] [ Google Scholar ]
- Dörner D., Güss C. D. (2013). PSI: a computational architecture of cognition, motivation, and emotion . Rev. Gen. Psychol. 17 , 297–317. 10.1037/a0032947 [ CrossRef ] [ Google Scholar ]
- Eccles J. S., Wigfield A. (2002). Motivational beliefs, values, and goals . Annu. Rev. Psychol. 53 , 109–132. 10.1146/annurevsych.53.100901.135153 [ PubMed ] [ CrossRef ] [ Google Scholar ]
- Frensch P., Funke J. (eds.) (1995). Complex Problem Solving: The European Perspective . Hillsdale, NJ: Lawrence Erlbaum Associates Inc. [ Google Scholar ]
- Funke J. (1985). Steuerung dynamischer Systeme durch Aufbau und Anwendung subjektiver Kausalmodelle [Control of dynamic systems via Construction and Application of subjective causal models] . Zeitschrift für Psychol. 193 , 443–465. [ Google Scholar ]
- Funke J. (2012). Complex problem solving , in Encyclopedia of the Sciences of Learning , ed Seel N. M. (Heidelberg: Springer; ), 682–685. [ Google Scholar ]
- Gerdes J., Dörner D., Pfeiffer E. (1993). Interaktive Computersimulation “Winfire.” [The Interactive Computer Simulation “Winfire”] . Otto-Friedrich-Universität Bamberg: Lehrstuhl Psychologie, II. [ Google Scholar ]
- Güss C. D., Tuason M. T., Gerhard C. (2010). Cross-national comparisons of complex problem-solving strategies in two microworlds . Cogn. Sci. 34 , 489–520. 10.1111/j.1551-6709.2009.01087.x [ PubMed ] [ CrossRef ] [ Google Scholar ]
- Güss C. D., Tuason M. T., Orduña L. V. (2015). Strategies, tactics, and errors in dynamic decision making . J. Dyn. Decis. Making 1 , 1–14. 10.11588/jddm.2015.1.13131 [ CrossRef ] [ Google Scholar ]
- Heckhausen H. (1991). Motivation and Action . New York, NY: Springer. [ Google Scholar ]
- Maslow A. H. (1943). A theory of human motivation . Psychol. Rev. 50 , 370–396. 10.1037/h0054346 [ CrossRef ] [ Google Scholar ]
- Maslow A. H. (1954). Motivation and Personality . New York, NY: Harper and Row. [ Google Scholar ]
- McClelland D. C., Atkinson J. W., Clark R. A., Lowell E. L. (1953). The Achievement Motive . East Norwalk, CT: Appleton-Century-Crofts. [ Google Scholar ]
- Nair K. U., Ramnarayan S. (2000). Individual differences in need for cognition and complex problem solving . J. Res. Pers. 34 , 305–328. 10.1006/jrpe.1999.2274 [ CrossRef ] [ Google Scholar ]
- Schaub H. (2009). Fire Simulation . Ottobrunn: IABG. [ Google Scholar ]
- Schoppek W., Putz-Osterloh W. (2003). Individuelle Unterschiede und die Bearbeitung komplexer Probleme . Zeitschrift für Differentielle und Diagnostische Psychol. 24 , 163–173. 10.1024/0170-1789.24.3.163 [ CrossRef ] [ Google Scholar ]
- Simon H. A. (1967). Motivational and emotional controls of cognition . Psychol. Rev. 74 , 29–39. 10.1037/h0024127 [ PubMed ] [ CrossRef ] [ Google Scholar ]
- Süß H.-M. (2001). Die Rolle von Intelligenz und Wissen für erfolgreiches Handeln in komplexen Problemsituationen [The role of intelligence and knowledge for successful performance in complex problem solving] , in Komplexität und Kompetenz: Ausgewählte Fragen der Kompetenzforschung , ed Franke G. (Bielefeld: Bertelsmann; ), 249–275. [ Google Scholar ]
- Stadler M., Becker N., Gödker M., Leutner D., Greiff S. (2015). Complex problem solving and intelligence: a meta-analysis . Intelligence 53 , 92–101. 10.1016/j.intell.2015.09.005 [ CrossRef ] [ Google Scholar ]
- Vollmeyer R., Rheinberg F. (1999). Motivation and metacognition when learning a complex system . Eur. J. Psychol. Educ. 14 , 541–554. 10.1007/BF03172978 [ CrossRef ] [ Google Scholar ]
- Vollmeyer R., Rheinberg F. (2000). Does motivation affect performance via persistence? Learn. Instruct. 10 , 293–309. 10.1016/S0959-4752(99)00031-6 [ CrossRef ] [ Google Scholar ]
- Wenke D., Frensch P. A., Funke J. (2005). Complex problem solving and intelligence: empirical relation and causal direction , in Cognition and Intelligence: Identifying the Mechanisms of the Mind , eds Sternberg R. J., Pretz J. E. (New York, NY: Cambridge University Press; ), 160–187. [ Google Scholar ]
Intelligence, Creativity, and Wisdom: A Case for Complex Problem Solving?
- First Online: 28 June 2023
Cite this chapter
- Florian Krieger 4 &
- Samuel Greiff 5
465 Accesses
This chapter covers the roles that intelligence, creativity, and wisdom play during problem solving as an integral competency that is needed to master challenges in the twenty-first century. We suggest that problem solving requires a set of skills that are strongly intertwined with all three concepts: intelligence, creativity, and wisdom.
This is a preview of subscription content, log in via an institution to check access.
Access this chapter
Subscribe and save.
- Get 10 units per month
- Download Article/Chapter or eBook
- 1 Unit = 1 Article or 1 Chapter
- Cancel anytime
- Available as PDF
- Read on any device
- Instant download
- Own it forever
- Available as EPUB and PDF
- Compact, lightweight edition
- Dispatched in 3 to 5 business days
- Free shipping worldwide - see info
- Durable hardcover edition
Tax calculation will be finalised at checkout
Purchases are for personal use only
Institutional subscriptions
Ardelt, M. (2004). Wisdom as expert knowledge system: A critical review of a contemporary operationalization of an ancient concept. Human Development, 47 (5), 257–285.
Article Google Scholar
Autor, D. H., Levy, F., & Murnane, R. J. (2003). The skill content of recent technological change: An empirical exploration. The Quarterly Journal of Economics. https://doi.org/10.1162/003355303322552801
Azevedo, R. (2009). Theoretical, conceptual, methodological, and instructional issues in research on metacognition and self-regulated learning: A discussion. Metacognition and Learning. https://doi.org/10.1007/s11409-009-9035-7
Baker, R., & Yacef, K. (2009). Journal of Educational Data Mining JEDM. JEDM | Journal of Educational Data Mining, 1 (1), 3–17.
Google Scholar
Baltes, P. B., & Smith, J. (2008). The fascination of wisdom: Its nature, ontogeny, and function. Perspectives on Psychological Science: A Journal of the Association for Psychological Science, 3 (1), 56–64.
Article PubMed Google Scholar
Berardi-Coletta, B., Buyer, L. S., Dominowski, R. L., & Rellinger, E. R. (1995). Metacognition and problem solving: A process-oriented approach. Journal of Experimental Psychology. Learning, Memory, and Cognition, 21 (1), 205–223.
Blech, C., & Funke, J. (2010). You cannot have your cake and eat it, too: How induced goal conflicts affect complex problem solving. The Open Psychology Journal, 3 (1), 42–53.
Boring, E. G. (1923). Intelligence as the tests test it. New Republic , 210–214.
Care, E., Griffin, P., & Wilson, M. (2018). Assessment and teaching of 21st century skills research and applications. Educational Assessment in an Information Age, January. https://doi.org/10.1007/978-3-319-65368-6
Cascio, W. F. (1995). Whither industrial and organizational psychology in a changing world of work? The American Psychologist, 50 (11), 928–939.
Cornoldi, C., Carretti, B., Drusi, S., & Tencati, C. (2015). Improving problem solving in primary school students: The effect of a training programme focusing on metacognition and working memory. The British Journal of Educational Psychology, 85 (3), 424–439.
Cropley, A. (2006). In praise of convergent thinking. Creativity Research Journal, 18 (3), 391–404.
Csapó, B., & Funke, J. (2017). The nature of problem solving. Using research to inspire 21st century learning . OECD Publishing.
Danner, D., Hagemann, D., Holt, D. V., Hager, M., Schankin, A., Wüstenberg, S., & Funke, J. (2011). Measuring performance in dynamic decision making. Journal of Individual Differences, 32 (4), 225–233.
Fischer, A., Greiff, S., & Funke, J. (2011). The process of solving complex problems. The Journal of Problem Solving, 4 (1). https://doi.org/10.7771/1932-6246.1118
Flavell, J. H. (1979). Metacognition and cognitive monitoring. The American Psychologist, 34 (10), 906–911.
Forthmann, B., Beaty, R. E., & Johnson, D. R. (2022). Semantic spaces are not created equal—How should we weigh them in the Sequel? European Journal of Psychological Assessment: Official Organ of the European Association of Psychological Assessment . https://doi.org/10.1027/1015-5759/a000723
Funke, J. (2001). Dynamic systems as tools for analysing human judgement. Thinking and Reasoning, 7 (1), 69–89.
Funke, J. (2019). Problem solving. In R. J. Sternberg & J. Funke (Eds.), The psychology of human thought: An introduction (pp. 155–176). Heidelberg University.
Gobert, J. D., Sao Pedro, M., Raziuddin, J., & Baker, R. S. (2013). From log files to assessment metrics: Measuring students’ science inquiry skills using educational data mining. Journal of the Learning Sciences, 22 (4), 521–563.
Goldstein, S., Princiotta, D., & Naglieri, J. A. (2015). Handbook of intelligence: Evolutionary theory, historical perspective, and current concepts . Springer.
Gottschling, J., Krieger, F., & Greiff, S. (2022). The fight against infectious diseases: The essential role of higher-order thinking and problem-solving. Journal of Intelligence, 10 (1). https://doi.org/10.1016/j.intell.2011.11.003
Greiff, S., Wüstenberg, S., & Avvisati, F. (2015). Computer-generated log-file analyses as a window into students’ minds? A showcase study based on the PISA 2012 assessment of problem solving. Computers and Education, 91 , 92–105.
Greiff, S., Wüstenberg, S., Csapó, B., Demetriou, A., Hautamäki, J., Graesser, A. C., & Martin, R. (2014). Domain-general problem solving skills and education in the 21st century. Educational Research Review, 13 , 74–83.
Greiff, S., Wüstenberg, S., & Funke, J. (2012). Dynamic problem solving: A new assessment perspective. Applied Psychological Measurement, 36 (3), 189–213.
Greiff, S., Wüstenberg, S., Molnár, G., Fischer, A., Funke, J., & Csapó, B. (2013). Complex problem solving in educational contexts-something beyond g : Concept, assessment, measurement invariance, and construct validity. Journal of Educational Psychology, 105 (2), 364–379.
Guilford, J. P. (1950). Creativity. American Psychologist, 5 , 444–454.
Horn, J. L., & Cattell, R. B. (1966). Refinement and test of the theory of fluid and crystallized general intelligences. Journal of Educational Psychology, 57 (5), 253.
Lotz, C., Scherer, R., Greiff, S., & Sparfeldt, J. R. (2017, October). Intelligence in action—Effective strategic behaviors while solving complex problems. Intelligence, 64 , 98–112.
Lubart, T. I. (1994). Creativity . In R. J. Sternberg (Ed.), Thinking and problem solving (pp. 290–332). Academic Press.
Mayer, R. E., & Wittrock, M. C. (2006). Problem solving. In P. A. Alexander & P. H. Winne (Eds.), Handbook of educational psychology (2nd ed., pp. 287–299). Routledge.
McGrew, K. S. (2009). CHC theory and the human cognitive abilities project: Standing on the shoulders of the giants of psychometric intelligence research. Intelligence, 37 (1), 1–10.
Mihalca, L., Mengelkamp, C., & Schnotz, W. (2017). Accuracy of metacognitive judgments as a moderator of learner control effectiveness in problem-solving tasks. Metacognition and Learning, 12 , 357–379.
Neisser, U., Boodoo, G., Bouchard, T. J., Jr., Boykin, A. W., Brody, N., Ceci, S. J., Halpern, D. F., Loehlin, J. C., Perloff, R., Sternberg, R. J., & Urbina, S. (1996). Intelligence: Knowns and unknowns. The American Psychologist, 51 (2), 77–101.
Nicolay, B., Krieger, F., Stadler, M., Gobert, J., & Greiff, S. (2021). Lost in transition—Learning analytics on the transfer from knowledge acquisition to knowledge application in complex problem solving. Computers in Human Behavior, 115 . https://doi.org/10.1016/j.chb.2020.106594
OECD (2013). PISA 2012 assessment and analytical framework . OECD Publishing.
Book Google Scholar
OECD (2014). PISA 2012 Results: Creative Problem Solving: Students’ Skills in Tackling Real-Life Problems (Volume V). OECD publishing.
Osman, M. (2010). Controlling uncertainty: A review of human behavior in complex dynamic environments. Psychological Bulletin, 136 (1), 65–86.
Plucker, J. A., Beghetto, R. A., & Dow, G. T. (2004). Why isn’t creativity more important to educational psychologists? Potentials, pitfalls, and future directions in creativity research. Educational Psychologist, 39 , 83–96.
Popper, K. (1999). All life is problem solving . Routledge.
Roth, B., Romeyke, S., Spinath, F. M., Domnick, F., Schäfer, S., & Becker, N. (2015). Intelligence and school grades: A meta-analysis. Intelligence, 53 , 118–137.
Schmidt, F. L., & Hunter, J. (2004). General mental ability in the world of work: Occupational attainment and job performance. Journal of Personality and Social Psychology, 86 (1), 162–173. https://doi.org/10.1037/0022-3514.86.1.162
Schneider, W. J., & McGrew, K. S. (2018). The Cattell–Horn–Carroll theory of cognitive abilities. In D. P. Flanagan & E. M. McDonough (Eds.), Contemporary intellectual assessment: Theories, tests, and issues (pp. 73–163). The Guilford Press.
Schweizer, F., Wüstenberg, S., & Greiff, S. (2013). Validity of the MicroDYN approach: Complex problem solving predicts school grades beyond working memory capacity. Learning and Individual Differences, 24 , 42–52.
Spearman, C. (1904). ‘General Intelligence’, objectively determined and measured. The American Journal of Psychology, 15 (2), 201–292.
Spearman, C. (1927). The abilities of man: Their nature and measurement . Macmillan and Co..
Stadler, M., Becker, N., Gödker, M., Leutner, D., & Greiff, S. (2015). Complex problem solving and intelligence: A meta-analysis. Intelligence, 53 , 92–101.
Stanovich, K. E. (2009). What intelligence tests miss . Yale University Press.
Sternberg, R. J. (2001). Why schools should teach for wisdom: The balance theory of wisdom in educational settings. Educational Psychologist, 36 (4), 227–245.
Sternberg, R. J. (2003). Wisdom, intelligence, and creativity synthesized . Cambridge University Press.
Sternberg, R. J. (2021). Adaptive intelligence: How to survive and thrive in a world of uncertainty . Cambridge University Press.
Sternberg, R. J. (2022). Meta-intelligence: Understanding, control, and coordination of higher cognitive processes. In R. M. Holm-Hadulla, J. Funke, & M. Wink (Eds.), Intelligence—Theories and applications (pp. 339–349). Springer International Publishing.
Chapter Google Scholar
Sternberg, R. J., Glaveanu, V., Karami, S., Kaufman, J. C., Phillipson, S. N., & Preiss, D. D. (2021). Meta-intelligence: Understanding, control, and interactivity between creative, analytical, practical, and wisdom-based approaches in problem solving. Journal of Intelligence, 9 (2). https://doi.org/10.3390/jintelligence9020019
Sternberg, R. J., & Glück, J. (2022). The psychology of wisdom: An introduction . Cambridge University Press.
Sternberg, R. J., & Lubart, T. I. (1995). Defying the crowd: Cultivating creativity in a culture of conformity . Free Press.
Stylianou, D. A. (2002). On the interaction of visualization and analysis: The negotiation of a visual representation in expert problem solving. The Journal of Mathematical Behavior, 21 (3), 303–317.
Thurstone, L. L. (1938). Primary mental abilities. Psychometric monograph no. 1 . University of Chicago Press.
Thurstone, L. L., & Thurstone, T. G. (1941). Factorial studies of intelligence . University of Chicago Press.
Veenman, M. V. J., Van Hout-Wolters, B. H. A. M., & Afflerbach, P. (2006). Metacognition and learning: conceptual and methodological considerations. Metacognition and Learning. https://doi.org/10.1007/s11409-006-6893-0
Winne, P. H., & Baker, R. S. (2013). The potentials of educational data mining for researching metacognition, motivation and self-regulated learning. Journal of Educational Data Mining, 5 (1), 1–8.
Wu, H., & Molnár, G. (2021). Logfile analyses of successful and unsuccessful strategy use in complex problem-solving: A cross-national comparison study. European Journal of Psychology of Education . https://doi.org/10.1007/s10212-020-00516-y
Wüstenberg, S., Greiff, S., & Funke, J. (2012). Complex problem solving—More than reasoning? Intelligence, 40 (1), 1–14.
Zohar, A., & Barzilai, S. (2013). A review of research on metacognition in science education: Current and future directions. Studies in Science Education. https://doi.org/10.1080/03057267.2013.847261
Download references
Author information
Authors and affiliations.
TU Dortmund University, Dortmund, Germany
Florian Krieger
University of Luxembourg, Esch-sur-Alzette, Luxembourg
Samuel Greiff
You can also search for this author in PubMed Google Scholar
Corresponding author
Correspondence to Samuel Greiff .
Editor information
Editors and affiliations.
College of Human Ecology, Cornell University, Ithaca, NY, USA
Robert J. Sternberg
Neag School of Education, University of Connecticut, Storrs, CT, USA
James C. Kaufman
Educational Psychology Faculty, Mississippi State University, Starkville, MS, USA
Sareh Karami
Rights and permissions
Reprints and permissions
Copyright information
© 2023 The Author(s), under exclusive license to Springer Nature Switzerland AG
About this chapter
Krieger, F., Greiff, S. (2023). Intelligence, Creativity, and Wisdom: A Case for Complex Problem Solving?. In: Sternberg, R.J., Kaufman, J.C., Karami, S. (eds) Intelligence, Creativity, and Wisdom. Palgrave Macmillan, Cham. https://doi.org/10.1007/978-3-031-26772-7_10

Download citation
DOI : https://doi.org/10.1007/978-3-031-26772-7_10
Published : 28 June 2023
Publisher Name : Palgrave Macmillan, Cham
Print ISBN : 978-3-031-26771-0
Online ISBN : 978-3-031-26772-7
eBook Packages : Behavioral Science and Psychology Behavioral Science and Psychology (R0)
Share this chapter
Anyone you share the following link with will be able to read this content:
Sorry, a shareable link is not currently available for this article.
Provided by the Springer Nature SharedIt content-sharing initiative
- Publish with us
Policies and ethics
- Find a journal
- Track your research
Thank you for visiting nature.com. You are using a browser version with limited support for CSS. To obtain the best experience, we recommend you use a more up to date browser (or turn off compatibility mode in Internet Explorer). In the meantime, to ensure continued support, we are displaying the site without styles and JavaScript.
- View all journals
- Explore content
- About the journal
- Publish with us
- Sign up for alerts
- Perspective
- Published: 25 January 2022
Intelligent problem-solving as integrated hierarchical reinforcement learning
- Manfred Eppe ORCID: orcid.org/0000-0002-5473-3221 1 nAff4 ,
- Christian Gumbsch ORCID: orcid.org/0000-0003-2741-6551 2 , 3 ,
- Matthias Kerzel 1 ,
- Phuong D. H. Nguyen 1 ,
- Martin V. Butz ORCID: orcid.org/0000-0002-8120-8537 2 &
- Stefan Wermter 1
Nature Machine Intelligence volume 4 , pages 11–20 ( 2022 ) Cite this article
5524 Accesses
34 Citations
7 Altmetric
Metrics details
- Cognitive control
- Computational models
- Computer science
- Learning algorithms
- Problem solving
According to cognitive psychology and related disciplines, the development of complex problem-solving behaviour in biological agents depends on hierarchical cognitive mechanisms. Hierarchical reinforcement learning is a promising computational approach that may eventually yield comparable problem-solving behaviour in artificial agents and robots. However, so far, the problem-solving abilities of many human and non-human animals are clearly superior to those of artificial systems. Here we propose steps to integrate biologically inspired hierarchical mechanisms to enable advanced problem-solving skills in artificial agents. We first review the literature in cognitive psychology to highlight the importance of compositional abstraction and predictive processing. Then we relate the gained insights with contemporary hierarchical reinforcement learning methods. Interestingly, our results suggest that all identified cognitive mechanisms have been implemented individually in isolated computational architectures, raising the question of why there exists no single unifying architecture that integrates them. As our final contribution, we address this question by providing an integrative perspective on the computational challenges to develop such a unifying architecture. We expect our results to guide the development of more sophisticated cognitively inspired hierarchical machine learning architectures.
This is a preview of subscription content, access via your institution
Access options
Access Nature and 54 other Nature Portfolio journals
Get Nature+, our best-value online-access subscription
24,99 € / 30 days
cancel any time
Subscribe to this journal
Receive 12 digital issues and online access to articles
111,21 € per year
only 9,27 € per issue
Buy this article
- Purchase on SpringerLink
- Instant access to full article PDF
Prices may be subject to local taxes which are calculated during checkout

Similar content being viewed by others
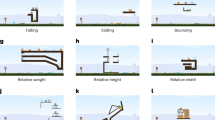
Phy-Q as a measure for physical reasoning intelligence
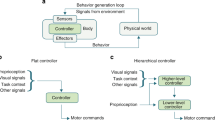
Hierarchical motor control in mammals and machines
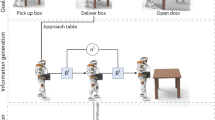
Hierarchical generative modelling for autonomous robots
Gruber, R. et al. New Caledonian crows use mental representations to solve metatool problems. Curr. Biol. 29 , 686–692 (2019).
Article Google Scholar
Butz, M. V. & Kutter, E. F. How the Mind Comes into Being (Oxford Univ. Press, 2017).
Perkins, D. N. & Salomon, G. in International Encyclopedia of Education (eds. Husen T. & Postelwhite T. N.) 6452–6457 (Pergamon Press, 1992).
Botvinick, M. M., Niv, Y. & Barto, A. C. Hierarchically organized behavior and its neural foundations: a reinforcement learning perspective. Cognition 113 , 262–280 (2009).
Tomov, M. S., Yagati, S., Kumar, A., Yang, W. & Gershman, S. J. Discovery of hierarchical representations for efficient planning. PLoS Comput. Biol. 16 , e1007594 (2020).
Arulkumaran, K., Deisenroth, M. P., Brundage, M. & Bharath, A. A. Deep reinforcement learning: a brief survey. IEEE Signal Process. Mag. 34 , 26–38 (2017).
Li, Y. Deep reinforcement learning: an overview. Preprint at https://arxiv.org/abs/1701.07274 (2018).
Sutton, R. S. & Barto, A. G. Reinforcement Learning : An Introduction 2nd edn (MIT Press, 2018).
Neftci, E. O. & Averbeck, B. B. Reinforcement learning in artificial and biological systems. Nat. Mach. Intell. 1 , 133–143 (2019).
Eppe, M., Nguyen, P. D. H. & Wermter, S. From semantics to execution: integrating action planning with reinforcement learning for robotic causal problem-solving. Front. Robot. AI 6 , 123 (2019).
Oh, J., Singh, S., Lee, H. & Kohli, P. Zero-shot task generalization with multi-task deep reinforcement learning. In Proc. 34th International Conference on Machine Learning ( ICML ) (eds. Precup, D. & Teh, Y. W.) 2661–2670 (PMLR, 2017).
Sohn, S., Oh, J. & Lee, H. Hierarchical reinforcement learning for zero-shot generalization with subtask dependencies. In Proc. 32nd International Conference on Neural Information Processing Systems ( NeurIPS ) (eds Bengio S. et al.) Vol. 31, 7156–7166 (ACM, 2018).
Hegarty, M. Mechanical reasoning by mental simulation. Trends Cogn. Sci. 8 , 280–285 (2004).
Klauer, K. J. Teaching for analogical transfer as a means of improving problem-solving, thinking and learning. Instruct. Sci. 18 , 179–192 (1989).
Duncker, K. & Lees, L. S. On problem-solving. Psychol. Monographs 58, No.5 (whole No. 270), 85–101 https://doi.org/10.1037/h0093599 (1945).
Dayan, P. Goal-directed control and its antipodes. Neural Netw. 22 , 213–219 (2009).
Dolan, R. J. & Dayan, P. Goals and habits in the brain. Neuron 80 , 312–325 (2013).
O’Doherty, J. P., Cockburn, J. & Pauli, W. M. Learning, reward, and decision making. Annu. Rev. Psychol. 68 , 73–100 (2017).
Tolman, E. C. & Honzik, C. H. Introduction and removal of reward, and maze performance in rats. Univ. California Publ. Psychol. 4 , 257–275 (1930).
Google Scholar
Butz, M. V. & Hoffmann, J. Anticipations control behavior: animal behavior in an anticipatory learning classifier system. Adaptive Behav. 10 , 75–96 (2002).
Miller, G. A., Galanter, E. & Pribram, K. H. Plans and the Structure of Behavior (Holt, Rinehart & Winston, 1960).
Botvinick, M. & Weinstein, A. Model-based hierarchical reinforcement learning and human action control. Philos. Trans. R. Soc. B Biol. Sci. 369 , 20130480 (2014).
Wiener, J. M. & Mallot, H. A. ’Fine-to-coarse’ route planning and navigation in regionalized environments. Spatial Cogn. Comput. 3 , 331–358 (2003).
Stock, A. & Stock, C. A short history of ideo-motor action. Psychol. Res. 68 , 176–188 (2004).
Hommel, B., Müsseler, J., Aschersleben, G. & Prinz, W. The theory of event coding (TEC): a framework for perception and action planning. Behav. Brain Sci. 24 , 849–878 (2001).
Hoffmann, J. in Anticipatory Behavior in Adaptive Learning Systems : Foundations , Theories and Systems (eds Butz, M. V. et al.) 44–65 (Springer, 2003).
Kunde, W., Elsner, K. & Kiesel, A. No anticipation-no action: the role of anticipation in action and perception. Cogn. Process. 8 , 71–78 (2007).
Barsalou, L. W. Grounded cognition. Annu. Rev. Psychol. 59 , 617–645 (2008).
Butz, M. V. Toward a unified sub-symbolic computational theory of cognition. Front. Psychol. 7 , 925 (2016).
Pulvermüller, F. Brain embodiment of syntax and grammar: discrete combinatorial mechanisms spelt out in neuronal circuits. Brain Lang. 112 , 167–179 (2010).
Sutton, R. S., Precup, D. & Singh, S. Between MDPs and semi-MDPs: a framework for temporal abstraction in reinforcement learning. Artif. Intell. 112 , 181–211 (1999).
Article MathSciNet MATH Google Scholar
Flash, T. & Hochner, B. Motor primitives in vertebrates and invertebrates. Curr. Opin. Neurobiol. 15 , 660–666 (2005).
Schaal, S. in Adaptive Motion of Animals and Machines (eds. Kimura, H. et al.) 261–280 (Springer, 2006).
Feldman, J., Dodge, E. & Bryant, J. in The Oxford Handbook of Linguistic Analysis (eds Heine, B. & Narrog, H.) 111–138 (Oxford Univ. Press, 2009).
Fodor, J. A. Language, thought and compositionality. Mind Lang. 16 , 1–15 (2001).
Frankland, S. M. & Greene, J. D. Concepts and compositionality: in search of the brain’s language of thought. Annu. Rev. Psychol. 71 , 273–303 (2020).
Hummel, J. E. Getting symbols out of a neural architecture. Connection Sci. 23 , 109–118 (2011).
Haynes, J. D., Wisniewski, D., Gorgen, K., Momennejad, I. & Reverberi, C. FMRI decoding of intentions: compositionality, hierarchy and prospective memory. In Proc. 3rd International Winter Conference on Brain-Computer Interface ( BCI ), 1-3 (IEEE, 2015).
Gärdenfors, P. The Geometry of Meaning : Semantics Based on Conceptual Spaces (MIT Press, 2014).
Book MATH Google Scholar
Lakoff, G. & Johnson, M. Philosophy in the Flesh (Basic Books, 1999).
Eppe, M. et al. A computational framework for concept blending. Artif. Intell. 256 , 105–129 (2018).
Turner, M. The Origin of Ideas (Oxford Univ. Press, 2014).
Deci, E. L. & Ryan, R. M. Self-determination theory and the facilitation of intrinsic motivation. Am. Psychol. 55 , 68–78 (2000).
Friston, K. et al. Active inference and epistemic value. Cogn. Neurosci. 6 , 187–214 (2015).
Berlyne, D. E. Curiosity and exploration. Science 153 , 25–33 (1966).
Loewenstein, G. The psychology of curiosity: a review and reinterpretation. Psychol. Bull. 116 , 75–98 (1994).
Oudeyer, P.-Y., Kaplan, F. & Hafner, V. V. Intrinsic motivation systems for autonomous mental development. In IEEE Transactions on Evolutionary Computation (eds. Coello, C. A. C. et al.) Vol. 11, 265–286 (IEEE, 2007).
Pisula, W. Play and exploration in animals—a comparative analysis. Polish Psychol. Bull. 39 , 104–107 (2008).
Jeannerod, M. Mental imagery in the motor context. Neuropsychologia 33 , 1419–1432 (1995).
Kahnemann, D. & Tversky, A. in Judgement under Uncertainty : Heuristics and Biases (eds Kahneman, D. et al.) Ch. 14, 201–208 (Cambridge Univ. Press, 1982).
Wells, G. L. & Gavanski, I. Mental simulation of causality. J. Personal. Social Psychol. 56 , 161–169 (1989).
Taylor, S. E., Pham, L. B., Rivkin, I. D. & Armor, D. A. Harnessing the imagination: mental simulation, self-regulation and coping. Am. Psychol. 53 , 429–439 (1998).
Kaplan, F. & Oudeyer, P.-Y. in Embodied Artificial Intelligence , Lecture Notes in Computer Science Vol. 3139 (eds Iida, F. et al.) 259–270 (Springer, 2004).
Schmidhuber, J. Formal theory of creativity, fun, and intrinsic motivation. IEEE Trans. Auton. Mental Dev. 2 , 230–247 (2010).
Friston, K., Mattout, J. & Kilner, J. Action understanding and active inference. Biol. Cybern. 104 , 137–160 (2011).
Oudeyer, P.-Y. Computational theories of curiosity-driven learning. In The New Science of Curiosity (ed. Goren Gordon), 43-72 (Nova Science Publishers, 2018); https://arxiv.org/abs/1802.10546
Colombo, M. & Wright, C. First principles in the life sciences: the free-energy principle, organicism and mechanism. Synthese 198 , 3463–3488 (2021).
Article MathSciNet Google Scholar
Huang, Y. & Rao, R. P. Predictive coding. WIREs Cogn. Sci. 2 , 580–593 (2011).
Friston, K. The free-energy principle: a unified brain theory? Nat. Rev. Neurosci. 11 , 127–138 (2010).
Knill, D. C. & Pouget, A. The Bayesian brain: the role of uncertainty in neural coding and computation. Trends Neurosci. 27 , 712–719 (2004).
Clark, A. Whatever next? Predictive brains, situated agents, and the future of cognitive science. Behav. Brain Sci. 36 , 181–204 (2013).
Clark, A. Surfing Uncertainty : Prediction , Action and the Embodied Mind (Oxford Univ. Press, 2016).
Zacks, J. M., Speer, N. K., Swallow, K. M., Braver, T. S. & Reyonolds, J. R. Event perception: a mind/brain perspective. Psychol. Bull. 133 , 273–293 (2007).
Eysenbach, B., Ibarz, J., Gupta, A. & Levine, S. Diversity is all you need: learning skills without a reward function. In International Conference on Learning Representations (ICLR, 2019).
Frans, K., Ho, J., Chen, X., Abbeel, P. & Schulman, J. Meta learning shared hierarchies. In Proc. International Conference on Learning Representations https://openreview.net/pdf?id=SyX0IeWAW (ICLR, 2018).
Heess, N. et al. Learning and transfer of modulated locomotor controllers. Preprint at https://arxiv.org/abs/1610.05182 (2016).
Jiang, Y., Gu, S., Murphy, K. & Finn, C. Language as an abstraction for hierarchical deep reinforcement learning. In Neural Information Processing Systems ( NeurIPS ) (eds. Wallach, H. et al.) 9414–9426 (ACM, 2019).
Li, A. C., Florensa, C., Clavera, I. & Abbeel, P. Sub-policy adaptation for hierarchical reinforcement learning. In Proc. International Conference on Learning Representations https://openreview.net/forum?id=ByeWogStDS (ICLR, 2020).
Qureshi, A. H. et al. Composing task-agnostic policies with deep reinforcement learning. In Proc. International Conference on Learning Representations https://openreview.net/forum?id=H1ezFREtwH (ICLR, 2020).
Sharma, A., Gu, S., Levine, S., Kumar, V. & Hausman, K. Dynamics-aware unsupervised discovery of skills. In Proc. International Conference on Learning Representations https://openreview.net/forum?id=HJgLZR4KvH (ICLR, 2020).
Tessler, C., Givony, S., Zahavy, T., Mankowitz, D. J. & Mannor, S. A deep hierarchical approach to lifelong learning in minecraft. In Proc. 31st AAAI Conference on Artificial Intelligence 1553–1561 (AAAI, 2017).
Vezhnevets, A. et al. Strategic attentive writer for learning macro-actions. In Neural Information Processing Systems ( NIPS ) (eds. Lee, D. et al.) 3494–3502 (NIPS, 2016).
Devin, C., Gupta, A., Darrell, T., Abbeel, P. & Levine, S. Learning modular neural network policies for multi-task and multi-robot transfer. In Proc. International Conference on Robotics and Automation ( ICRA ) (eds. Okamura, A. et al.) 2169–2176 (IEEE, 2017).
Hejna, D. J., Abbeel, P. & Pinto, L. Hierarchically decoupled morphological transfer. In Proc. International Conference on Machine Learning ( ICML ) (eds. Daumé III, H. & Singh, A.) 11409–11420 (PMLR, 2020).
Hamrick, J. B. et al. On the role of planning in model-based deep reinforcement learning. In Proc. International Conference on Learning Representations https://openreview.net/pdf?id=IrM64DGB21 (ICLR, 2021).
Sutton, R. S. Integrated architectures for learning, planning, and reacting based on approximating dynamic programming. In Proc. 7th International Conference on Machine Learning ( ICML ) (eds. Porter, B. W. & Mooney, R. J.) 216–224 (Morgan Kaufmann, 1990).
Nau, D. et al. SHOP2: an HTN planning system. J. Artif. Intell. Res. 20 , 379–404 (2003).
Article MATH Google Scholar
Lyu, D., Yang, F., Liu, B. & Gustafson, S. SDRL: interpretable and data-efficient deep reinforcement learning leveraging symbolic planning. In Proc. AAAI Conference on Artificial Intelligence Vol. 33, 2970–2977 (AAAI, 2019).
Ma, A., Ouimet, M. & Cortés, J. Hierarchical reinforcement learning via dynamic subspace search for multi-agent planning. Auton. Robot. 44 , 485–503 (2020).
Bacon, P.-L., Harb, J. & Precup, D. The option-critic architecture. In Proc. 31st AAAI Conference on Artificial Intelligence 1726–1734 (AAAI, 2017).
Dietterich, T. G. State abstraction in MAXQ hierarchical reinforcement learning. In Advances in Neural Information Processing Systems ( NIPS ) (eds. Solla, S. et al.) Vol. 12, 994–1000 (NIPS, 1999).
Kulkarni, T. D., Narasimhan, K. R., Saeedi, A. & Tenenbaum, J. B. Hierarchical deep reinforcement learning: integrating temporal abstraction and intrinsic motivation. In Neural Information Processing Systems ( NIPS ) (eds. Lee, D. et al.) 3675–3683 (NIPS, 2016).
Shankar, T., Pinto, L., Tulsiani, S. & Gupta, A. Discovering motor programs by recomposing demonstrations. In Proc. International Conference on Learning Representations https://openreview.net/attachment?id=rkgHY0NYwr&name=original_pdf (ICLR, 2020).
Vezhnevets, A. S., Wu, Y. T., Eckstein, M., Leblond, R. & Leibo, J. Z. Options as responses: grounding behavioural hierarchies in multi-agent reinforcement learning. In Proc. International Conference on Machine Learning ( ICML ) (eds. Daumé III, H. & Singh, A.) 9733–9742 (PMLR, 2020).
Ghazanfari, B., Afghah, F. & Taylor, M. E. Sequential association rule mining for autonomously extracting hierarchical task structures in reinforcement learning. IEEE Access 8 , 11782–11799 (2020).
Levy, A., Konidaris, G., Platt, R. & Saenko, K. Learning multi-level hierarchies with hindsight. In Proc. International Conference on Learning Representations https://openreview.net/pdf?id=ryzECoAcY7 (ICLR, 2019).
Nachum, O., Gu, S., Lee, H. & Levine, S. Data-efficient hierarchical reinforcement learning. In Proc. 32nd International Conference on Neural Information Processing Systems (NIPS) (eds. Bengio, S. et al.) 3307–3317 (NIPS, 2018).
Rafati, J. & Noelle, D. C. Learning representations in model-free hierarchical reinforcement learning. In Proc. 33rd AAAI Conference on Artificial Intelligence 10009–10010 (AAAI, 2019).
Röder, F., Eppe, M., Nguyen, P. D. H. & Wermter, S. Curious hierarchical actor-critic reinforcement learning. In Proc. International Conference on Artificial Neural Networks ( ICANN ) (eds. Farkaš, I. et al.) 408–419 (Springer, 2020).
Zhang, T., Guo, S., Tan, T., Hu, X. & Chen, F. Generating adjacency-constrained subgoals in hierarchical reinforcement learning. In Neural Information Processing Systems ( NIPS ) (eds. Larochelle, H. et al.) 21579-21590 (NIPS, 2020).
Lample, G. & Chaplot, D. S. Playing FPS games with deep reinforcement learning. In Proc. 31st AAAI Conference on Artificial Intelligence 2140–2146 (AAAI, 2017).
Vezhnevets, A. S. et al. FeUdal networks for hierarchical reinforcement learning. In Proc. 34th International Conference on Machine Learning ( ICML ) (eds. Precup, D. & Teh, Y. W.) Vol. 70, 3540–3549 (PMLR, 2017).
Wulfmeier, M. et al. Compositional Transfer in Hierarchical Reinforcement Learning. In Robotics: Science and System XVI (RSS) (eds. Toussaint M. et al.) (Robotics: Science and Systems Foundation, 2020); https://arxiv.org/abs/1906.11228
Yang, Z., Merrick, K., Jin, L. & Abbass, H. A. Hierarchical deep reinforcement learning for continuous action control. IEEE Trans. Neural Netw. Learn. Syst. 29 , 5174–5184 (2018).
Toussaint, M., Allen, K. R., Smith, K. A. & Tenenbaum, J. B. Differentiable physics and stable modes for tool-use and manipulation planning. In Proc. Robotics : Science and Systems XIV ( RSS ) (eds. Kress-Gazit, H. et al.) https://ipvs.informatik.uni-stuttgart.de/mlr/papers/18-toussaint-RSS.pdf (Robotics: Science and Systems Foundation, 2018).
Akrour, R., Veiga, F., Peters, J. & Neumann, G. Regularizing reinforcement learning with state abstraction. In Proc. IEEE / RSJ International Conference on Intelligent Robots and Systems ( IROS ) 534–539 (IEEE, 2018).
Schaul, T. & Ring, M. Better generalization with forecasts. In Proc. 23rd International Joint Conference on Artificial Intelligence ( IJCAI ) (ed. Rossi, F.) 1656–1662 (AAAI, 2013).
Colas, C., Akakzia, A., Oudeyer, P.-Y., Chetouani, M. & Sigaud, O. Language-conditioned goal generation: a new approach to language grounding for RL. Preprint at https://arxiv.org/abs/2006.07043 (2020).
Blaes, S., Pogancic, M. V., Zhu, J. J. & Martius, G. Control what you can: intrinsically motivated task-planning agent. Neural Inf. Process. Syst. 32 , 12541–12552 (2019).
Haarnoja, T., Hartikainen, K., Abbeel, P. & Levine, S. Latent space policies for hierarchical reinforcement learning. In Proc. International Conference on Machine Learning ( ICML ) (eds. Dy, J. & Krause, A.) Vol. 4, 2965–2975 (PMLR, 2018).
Rasmussen, D., Voelker, A. & Eliasmith, C. A neural model of hierarchical reinforcement learning. PLoS ONE 12 , e0180234 (2017).
Riedmiller, M. et al. Learning by playing—solving sparse reward tasks from scratch. In Proc. International Conference on Machine Learning ( ICML ) (eds. Dy, J. & Krause, A.) Vol. 10, 6910–6919 (PMLR, 2018).
Yang, F., Lyu, D., Liu, B. & Gustafson, S. PEORL: integrating symbolic planning and hierarchical reinforcement learning for robust decision-making. In Proc. 27th International Joint Conference on Artificial Intelligence ( IJCAI ) (ed. Lang, J.) 4860–4866 (IJCAI, 2018).
Machado, M. C., Bellemare, M. G. & Bowling, M. A Laplacian framework for option discovery in reinforcement learning. In Proc. International Conference on Machine Learning (ICML) (eds. Precup, D. & Teh, Y. W.) Vol. 5, 3567–3582 (PMLR, 2017).
Pathak, D., Agrawal, P., Efros, A. A. & Darrell, T. Curiosity-driven exploration by self-supervised prediction. In Proc. 34th International Conference on Machine Learning ( ICML ) (eds. Precup, D. & Teh, Y. W.) 2778–2787 (PMLR, 2017).
Schillaci, G. et al. Intrinsic motivation and episodic memories for robot exploration of high-dimensional sensory spaces. Adaptive Behav. 29 549–566 (2020).
Colas, C., Fournier, P., Sigaud, O., Chetouani, M. & Oudeyer, P.-Y. CURIOUS: intrinsically motivated modular multi-goal reinforcement learning. In Proc. International Conference on Machine Learning ( ICML ) (eds. Chaudhuri, K. & Salakhutdinov, R.) 1331–1340 (PMLR, 2019).
Hafez, M. B., Weber, C., Kerzel, M. & Wermter, S. Improving robot dual-system motor learning with intrinsically motivated meta-control and latent-space experience imagination. Robot. Auton. Syst. 133 , 103630 (2020).
Yamamoto, K., Onishi, T. & Tsuruoka, Y. Hierarchical reinforcement learning with abductive planning. In Proc. ICML / IJCAI / AAMAS 2018 Workshop on Planning and Learning ( PAL-18 ) (2018).
Wu, B., Gupta, J. K. & Kochenderfer, M. J. Model primitive hierarchical lifelong reinforcement learning . In Proc. International Joint Conference on Autonomous Agents and Multiagent Systems ( AAMAS ) (eds. Agmon, N. et al.) Vol. 1, 34–42 (IFAAMAS, 2019).
Li, Z., Narayan, A. & Leong, T. Y. An efficient approach to model-based hierarchical reinforcement learning. In Proc. 31st AAAI Conference on Artificial Intelligence 3583–3589 (AAAI, 2017).
Hafner, D., Lillicrap, T. & Norouzi, M. Dream to control: learning behaviors by latent imagination. In Proc. International Conference on Learning Representations https://openreview.net/pdf?id=S1lOTC4tDS (ICLR, 2020).
Deisenroth, M. P., Rasmussen, C. E. & Fox, D. Learning to control a low-cost manipulator using data-efficient reinforcement learning. In Robotics : Science and Systems VII ( RSS ) (eds. Durrant-Whyte, H. et al.) 57–64 (Robotics: Science and Systems Foundation, 2011).
Ha, D. & Schmidhuber, J. Recurrent world models facilitate policy evolution. In Proc. 32nd International Conference on Neural Information Processing Systems (NeurIPS) (eds. Bengio, S. et al.) 2455–2467 (NIPS, 2018).
Battaglia, P. W. et al. Relational inductive biases, deep learning and graph networks. Preprint at https://arxiv.org/abs/1806.01261 (2018).
Andrychowicz, M. et al. Hindsight experience replay. In Proc. Neural Information Processing Systems ( NIPS ) (eds. Guyon I. et al.) 5048–5058 (NIPS, 2017); https://papers.nips.cc/paper/7090-hindsight-experience-replay.pdf
Schwartenbeck, P. et al. Computational mechanisms of curiosity and goal-directed exploration. eLife 8 , e41703 (2019).
Haarnoja, T., Zhou, A., Abbeel, P. & Levine, S. Soft actor-critic: off-policy maximum entropy deep reinforcement learning with a stochastic actor. In Proc. International Conference on Machine Learning ( ICML ) (eds. Dy, J. & Krause, A.) 1861–1870 (PMLR, 2018).
Yu, A. J. & Dayan, P. Uncertainty, neuromodulation and attention. Neuron 46 , 681–692 (2005).
Baldwin, D. A. & Kosie, J. E. How does the mind render streaming experience as events? Top. Cogn. Sci. 13 , 79–105 (2021).
Download references
Acknowledgements
We acknowledge funding from the DFG (projects IDEAS, LeCAREbot, TRR169, SPP 2134, RTG 1808 and EXC 2064/1), the Humboldt Foundation and Max Planck Research School IMPRS-IS.
Author information
Manfred Eppe
Present address: Hamburg University of Technology, Hamburg, Germany
Authors and Affiliations
Universität Hamburg, Hamburg, Germany
Manfred Eppe, Matthias Kerzel, Phuong D. H. Nguyen & Stefan Wermter
University of Tübingen, Tübingen, Germany
Christian Gumbsch & Martin V. Butz
Max Planck Institute for Intelligent Systems, Tübingen, Germany
Christian Gumbsch
You can also search for this author in PubMed Google Scholar
Corresponding author
Correspondence to Manfred Eppe .
Ethics declarations
Competing interests.
The authors declare no competing interests.
Additional information
Publisher’s note Springer Nature remains neutral with regard to jurisdictional claims in published maps and institutional affiliations.
Supplementary information
Supplementary information.
Supplementary Boxes 1–6 and Table 1.
Rights and permissions
Reprints and permissions
About this article
Cite this article.
Eppe, M., Gumbsch, C., Kerzel, M. et al. Intelligent problem-solving as integrated hierarchical reinforcement learning. Nat Mach Intell 4 , 11–20 (2022). https://doi.org/10.1038/s42256-021-00433-9
Download citation
Received : 18 December 2020
Accepted : 07 December 2021
Published : 25 January 2022
Issue Date : January 2022
DOI : https://doi.org/10.1038/s42256-021-00433-9
Share this article
Anyone you share the following link with will be able to read this content:
Sorry, a shareable link is not currently available for this article.
Provided by the Springer Nature SharedIt content-sharing initiative
This article is cited by
Efficient stacking and grasping in unstructured environments.
- Jinbiao Zhu
Journal of Intelligent & Robotic Systems (2024)
Four attributes of intelligence, a thousand questions
- Matthieu Bardal
- Eric Chalmers
Biological Cybernetics (2023)
An Alternative to Cognitivism: Computational Phenomenology for Deep Learning
- Pierre Beckmann
- Guillaume Köstner
- Inês Hipólito
Minds and Machines (2023)
Quick links
- Explore articles by subject
- Guide to authors
- Editorial policies
Sign up for the Nature Briefing: AI and Robotics newsletter — what matters in AI and robotics research, free to your inbox weekly.


IMAGES
VIDEO
COMMENTS
This meta-analysis investigated the relation between intelligence and complex problem solving (CPS). The findings show a substantial mean effect size of M (g) = .433 for the correlation of the two constructs that is highly significant with only little evidence for publication bias. This finding contradicts earlier reviews suggesting a non ...
The purpose of this meta-analysis is to examine the nature and magnitude of the relation between complex problem-solving skills (CPS) and intelligence, a topic that has been widely discussed and that has instigated a vast array of partially contradicting findings in the past. Theoretically, researchers have hypothesized the two constructs to be everything from completely separate to identical ...
1.1. Complex Problem Solving: Definition, Assessment and Relations to Intelligence. According to a widely accepted definition proposed by Buchner (), CPS is "the successful interaction with task environments that are dynamic (i.e., change as a function of users' intervention and/or as a function of time) and in which some, if not all, of the environment's regularities can only be ...
A recent meta-analysis [16], which included 60 studies from 1982 to 2014, showed that the correlations between successful complex problem-solving and intelligence varied from 0.339 to 0.585 ...
This study investigated whether students' knowledge of a certain strategy that was assessed in a paper-and-pencil-based scientific reasoning task as well as their fluid intelligence and learning orientation would be sufficient to explain variance in the application of the VOTAT strategy in solving an interactive complex problem solving (CPS) task.
While research findings support the assumption that (1) affective, (2) (meta-)cognitive, and (3) social processes support complex problem-solving, opportunities of AI for supporting complex ...
The purpose of this meta-analysis is to examine the nature and magnitude of the relation between complex problem-solving skills (CPS) and intelligence, a topic that has been widely discussed and that has instigated a vast array of partially contradicting findings in the past.
Previous research on Complex Problem Solving (CPS) has primarily focused on cognitive factors as outlined below. The current paper discusses the role of motivation during CPS and argues that motivation, emotion, and cognition interact and cannot be studied in an isolated manner. ... Complex problem solving and intelligence: a meta-analysis ...
This paper presents the findings of a scoping literature review focusing on empirical evidence on how artificial intelligence supports human complex problem-solving and the nature of human-AI collaboration in complex problem-solving at the level of (meta-)cognitive and social practices, as well as affective processes. 2. Research on problem-solving
Problem-Solving and Intelligence. The ability to solve complex problems is a defining feature of what most laypeople think of as intelligence. This is also a common theme in how intelligence researchers describe intelligence (Sternberg, 1985a; Sternberg et al., 1981). Over a century ago, the German psychologist Wilhelm Stern (1914), who ...
In this chapter, notions of psychometric intelligence and cognitive psychology were used to analyze individual differences in the ability to execute cognitive processes. Specifically, the performance of good and poor problem solvers through the analysis of the types of errors in the SPM test were studied. Additionally, the relationship between ...
Greiff, Assessing complex problem-solving skills with multiple complex systems, Thinking & Reasoning, с. 1 Greiff, Dynamic problem solving — A new assessment perspective, Applied Psychological Measurement, № 36, с. 189
This meta-analysis investigated the relation between intelligence and complex problem solving (CPS). The findings show a substantial mean effect size of M (g) = .433 for the correlation of the two constructs that is highly significant with only little evidence for publication bias. This finding contradicts earlier reviews suggesting a non ...
Introduction. This chapter covers the roles that intelligence, creativity, and wisdom play during problem solving as an integral competency that is needed to master challenges in the twenty-first century. We suggest that problem solving requires a set of skills that are strongly intertwined with all three concepts: intelligence, creativity, and ...
In this chapter, we discuss the link between intelligence and problem solving in terms of contemporary ideas concerning both. To preview, we argue that the ability to solve problems is not just an aspect or feature of intelligence—it is the essence of intelligence. The chapter is organized into five major sections. In the first section, we consider the question of what a problem is and argue ...
23 Problem-Solving and Intelligence. David Z. Hambrick, Alexander P. Burgoyne, and Erik M. Altmann. The ability to solve complex problems is a defining feature of what most laypeople think of as intelligence. This is also a common theme in how intelligence researchers describe intelligence (Sternberg, 1985a; Sternberg et al., 1981).
Complex problem solving and intelligence: A meta-analysis. Intelligence , 53 , 92-101. 10.1016/j.intell.2015.09.005 First citation in article Crossref , Google Scholar
Rationale: Assessing complex problem-solving skills (CPS) is of great interest to many researchers. However, existing assessments require long testing times making them difficult to include in many studies and experiments. Here, we propose a specific composition of microworlds based on the MicroDYN approach, which allows for valid estimation of CPS in a substantially reduced amount of time (N ...
1.1. Complex Problem Solving: Definition, Assessment and Relations to Intelligence ... A meta-analysis con-ducted byStadler et al.(2015) selected 47 empirical studies (total sample size N = 13,740) ... (total sample size N = 13,740) which focused on the correlation between CPS and intelligence. The results of their analysis confirmed that a ...
According to cognitive psychology and related disciplines, the development of complex problem-solving behaviour in biological agents depends on hierarchical cognitive mechanisms. Hierarchical ...
While the link between metacognitive skills and the creativity of outcomes was established only recently, further exploration is required to understand their interplay in complex problem-solving. This explanatory sequential mixed-methods study investigated the role of metacognition in solving two ill-defined problem-solving tasks: an ...