- Data Structures
- Linked List
- Binary Tree
- Binary Search Tree
- Segment Tree
- Disjoint Set Union
- Fenwick Tree
- Red-Black Tree
- Advanced Data Structures

Hungarian Algorithm for Assignment Problem | Set 2 (Implementation)
Given a 2D array , arr of size N*N where arr[i][j] denotes the cost to complete the j th job by the i th worker. Any worker can be assigned to perform any job. The task is to assign the jobs such that exactly one worker can perform exactly one job in such a way that the total cost of the assignment is minimized.
Input: arr[][] = {{3, 5}, {10, 1}} Output: 4 Explanation: The optimal assignment is to assign job 1 to the 1st worker, job 2 to the 2nd worker. Hence, the optimal cost is 3 + 1 = 4. Input: arr[][] = {{2500, 4000, 3500}, {4000, 6000, 3500}, {2000, 4000, 2500}} Output: 4 Explanation: The optimal assignment is to assign job 2 to the 1st worker, job 3 to the 2nd worker and job 1 to the 3rd worker. Hence, the optimal cost is 4000 + 3500 + 2000 = 9500.
Different approaches to solve this problem are discussed in this article .
Approach: The idea is to use the Hungarian Algorithm to solve this problem. The algorithm is as follows:
- For each row of the matrix, find the smallest element and subtract it from every element in its row.
- Repeat the step 1 for all columns.
- Cover all zeros in the matrix using the minimum number of horizontal and vertical lines.
- Test for Optimality : If the minimum number of covering lines is N , an optimal assignment is possible. Else if lines are lesser than N , an optimal assignment is not found and must proceed to step 5.
- Determine the smallest entry not covered by any line. Subtract this entry from each uncovered row, and then add it to each covered column. Return to step 3.
Consider an example to understand the approach:
Let the 2D array be: 2500 4000 3500 4000 6000 3500 2000 4000 2500 Step 1: Subtract minimum of every row. 2500, 3500 and 2000 are subtracted from rows 1, 2 and 3 respectively. 0 1500 1000 500 2500 0 0 2000 500 Step 2: Subtract minimum of every column. 0, 1500 and 0 are subtracted from columns 1, 2 and 3 respectively. 0 0 1000 500 1000 0 0 500 500 Step 3: Cover all zeroes with minimum number of horizontal and vertical lines. Step 4: Since we need 3 lines to cover all zeroes, the optimal assignment is found. 2500 4000 3500 4000 6000 3500 2000 4000 2500 So the optimal cost is 4000 + 3500 + 2000 = 9500
For implementing the above algorithm, the idea is to use the max_cost_assignment() function defined in the dlib library . This function is an implementation of the Hungarian algorithm (also known as the Kuhn-Munkres algorithm) which runs in O(N 3 ) time. It solves the optimal assignment problem.
Below is the implementation of the above approach:
Time Complexity: O(N 3 ) Auxiliary Space: O(N 2 )
Please Login to comment...
Similar reads.
- Mathematical
Improve your Coding Skills with Practice
What kind of Experience do you want to share?
linear_sum_assignment #
Solve the linear sum assignment problem.
The cost matrix of the bipartite graph.
Calculates a maximum weight matching if true.
An array of row indices and one of corresponding column indices giving the optimal assignment. The cost of the assignment can be computed as cost_matrix[row_ind, col_ind].sum() . The row indices will be sorted; in the case of a square cost matrix they will be equal to numpy.arange(cost_matrix.shape[0]) .
for sparse inputs
The linear sum assignment problem [1] is also known as minimum weight matching in bipartite graphs. A problem instance is described by a matrix C, where each C[i,j] is the cost of matching vertex i of the first partite set (a ‘worker’) and vertex j of the second set (a ‘job’). The goal is to find a complete assignment of workers to jobs of minimal cost.
Formally, let X be a boolean matrix where \(X[i,j] = 1\) iff row i is assigned to column j. Then the optimal assignment has cost
where, in the case where the matrix X is square, each row is assigned to exactly one column, and each column to exactly one row.
This function can also solve a generalization of the classic assignment problem where the cost matrix is rectangular. If it has more rows than columns, then not every row needs to be assigned to a column, and vice versa.
This implementation is a modified Jonker-Volgenant algorithm with no initialization, described in ref. [2] .
Added in version 0.17.0.
https://en.wikipedia.org/wiki/Assignment_problem
DF Crouse. On implementing 2D rectangular assignment algorithms. IEEE Transactions on Aerospace and Electronic Systems , 52(4):1679-1696, August 2016, DOI:10.1109/TAES.2016.140952

Hungarian Algorithm Introduction & Python Implementation
How to use hungarian method to resolve the linear assignment problem..
By Eason on 2021-08-02
In this article, I will introduce how to use Hungarian Method to resolve the linear assignment problem and provide my personal Python code solution.
So… What is the linear assignment problem?
The linear assignment problem represents the need to maximize the available resources (or minimize the expenditure) with limited resources. For instance, below is a 2D matrix, where each row represents a different supplier, and each column represents the cost of employing them to produce a particular product. Each supplier can only specialize in the production of one of these products. In other words, only one element can be selected for each column and row in the matrix, and the sum of the selected elements must be minimized (minimized cost expense).
The cost of producing different goods by different producers:
Indeed, this is a simple example. By trying out the possible combinations, we can see that the smallest sum is 13, so supplier A supplies Bubble Tea , supplier B supplies milk tea, and supplier C supplies Fruit Tea . However, such attempts do not follow a clear rule and become inefficient when applied to large tasks. Therefore, the next section will introduce step by step the Hungarian algorithm, which can be applied to the linear assignment problem.
Hungarian Algorithm & Python Code Step by Step
In this section, we will show how to use the Hungarian algorithm to solve linear assignment problems and find the minimum combinations in the matrix. Of course, the Hungarian algorithm can also be used to find the maximum combination.
Step 0. Prepare Operations
First, an N by N matrix is generated to be used for the Hungarian algorithm (Here, we use a 5 by 5 square matrix as an example).
The above code randomly generates a 5x5 cost matrix of integers between 0 and 10.
If we want to find the maximum sum, we could do the opposite. The matrix to be solved is regarded as the profit matrix, and the maximum value in the matrix is set as the common price of all goods. The cost matrix is obtained by subtracting the profit matrix from the maximum value. Finally, the cost matrix is substituted into the Hungarian algorithm to obtain the minimized combination and then remapped back to the profit matrix to obtain the maximized sum value and composition result.
The above code randomly generates a 5x5 profit matrix of integers between 0 and 10 and generate a corresponding cost matrix
By following the steps above, you can randomly generate either the cost matrix or the profit matrix. Next, we will move into the introduction of the Hungarian algorithm, and for the sake of illustration, the following sections will be illustrated using the cost matrix shown below. We will use the Hungarian algorithm to solve the linear assignment problem of the cost matrix and find the corresponding minimum sum.
Example cost matrix:
Step 1. Every column and every row subtract its internal minimum
First, every column and every row must subtract its internal minimum. After subtracting the minimum, the cost matrix will look like this.
Cost matrix after step 1:
And the current code is like this:
Step 2.1. Min_zero_row Function Implementation
At first, we need to find the row with the fewest zero elements. So, we can convert the previous matrix to the boolean matrix(0 → True, Others → False).
Transform matrix to boolean matrix:
Corresponding Boolean matrix:
Therefore, we can use the “min_zero_row” function to find the corresponding row.
The row which contains the least 0:

Third, mark any 0 elements on the corresponding row and clean up its row and column (converts elements on the Boolean matrix to False). The coordinates of the element are stored in mark_zero.
Hence, the boolean matrix will look like this:
The boolean matrix after the first process. The fourth row has been changed to all False.
The process is repeated several times until the elements in the boolean matrix are all False. The below picture shows the order in which they are marked.
The possible answer composition:

Step 2.2. Mark_matrix Function Implementation
After getting Zero_mat from the step 2–1, we can check it and mark the matrix according to certain rules. The whole rule can be broken down into several steps:
- Mark rows that do not contain marked 0 elements and store row indexes in the non_marked_row
- Search non_marked_row element, and find out if there are any unmarked 0 elements in the corresponding column
- Store the column indexes in the marked_cols
- Compare the column indexes stored in marked_zero and marked_cols
- If a matching column index exists, the corresponding row_index is saved to non_marked_rows
- Next, the row indexes that are not in non_marked_row are stored in marked_rows
Finally, the whole mark_matrx function is finished and then returns marked_zero , marked_rows , marked_cols. At this point, we will be able to decide the result based on the return information.
If we use the example cost matrix, the corresponding marked_zero , marked_rows, and marked_cols are as follows:
- marked_zero : [(3, 2), (0, 4), (1, 1), (2, 0), (4, 3)]
- marked_rows : [0, 1, 2, 3, 4]
- marked_cols : []
Step 3. Identify the Result
At this step, if the sum of the lengths of marked_rows and marked_cols is equal to the length of the cost matrix, it means that the solution of the linear assignment problem has been found successfully, and marked_zero stores the solution coordinates. Fortunately, in the example matrix, we find the answer on the first try. Therefore, we can skip to step 5 and calculate the solution.
However, everything is hardly plain sailing. Most of the time, we will not find the solution on the first try, such as the following matrix:
After Step 1 & 2 , the corresponding matrix, marked_rows, and marked_cols are as follows:
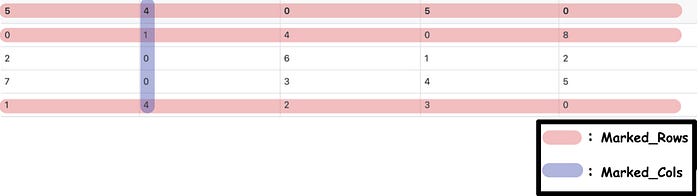
The sum of the lengths of Marked_Rows and Marked_Cols is 4 (less than 5).
Apparently, the sum of the lengths is less than the length of the matrix. At this time, we need to go into Step 4 to adjust the matrix.
Step 4. Adjust Matrix
In Step 4, we're going to put the matrix after Step 1 into the Adjust_Matrix function . Taking the latter matrix in Step 3 as an example, the matrix to be modified in Adjust_Matrix is:
The whole function can be separated into three steps:
- Find the minimum value for an element that is not in marked_rows and not in marked_cols . Hence, we can find the minimum value is 1.
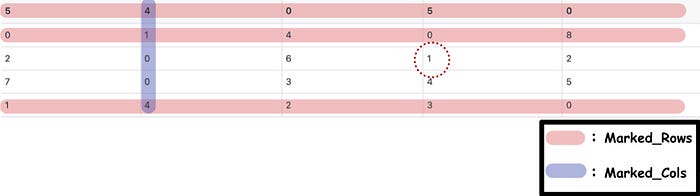
- Subtract the elements which not in marked_rows nor marked_cols from the minimum values obtained in the previous step.
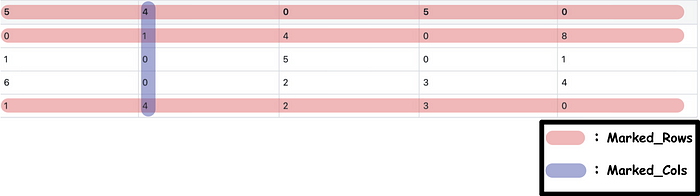
- Add the element in marked_rows , which is also in marked_cols , to the minimum value obtained by Step 4–1.

Return the adjusted matrix and repeat Step 2 and Step 3 until the conditions satisfy the requirement of entering Step 5.
Step 5. Calculate the Answer
Using the element composition stored in marked_zero , the minimum and maximum values of the linear assignment problem can be calculated.

The minimum composition of the assigned matrix and the minimum sum is 18.

The maximum composition of the assigned matrix and the maximum sum is 43.
The code of the Answer_Calculator function is as follows:
The complete code is as follows:
Hungarian algorithm - Wikipedia
Continue Learning
How to upload files to sharepoint using python.
Upload files to MS SharePoint using Office365 Python SDK
The Mysteries of Floating-Point Numbers
Exploring the Fascinating World of Computational Precision
How To Track Phone Number Location With Python
No hacking — in 8 lines of code
How to Update Discord bot status with Discord.py
Personalizing your bot's displayed activity for your discord server with Python
Run Python Code in React with Pyodide
How to Run Python Code in the Browser with Web Assembly, React, and Pyodide
How to Implement a Maximum Likelihood Estimation Code for Any Distribution
A guide for writing MLE code for any distribution under 5 minutes in case SciPy fails.
munkres 1.1.4
pip install munkres Copy PIP instructions
Released: Sep 15, 2020
Munkres (Hungarian) algorithm for the Assignment Problem
Verified details
Maintainers.
Unverified details
Project links.
View statistics for this project via Libraries.io , or by using our public dataset on Google BigQuery
License: Apache Software License (Apache Software License)
Author: Brian Clapper
Classifiers
- Science/Research
- OSI Approved :: Apache Software License
- OS Independent
- Scientific/Engineering :: Mathematics
- Software Development :: Libraries :: Python Modules
Project description
Introduction.
The Munkres module provides an implementation of the Munkres algorithm (also called the Hungarian algorithm or the Kuhn-Munkres algorithm), useful for solving the Assignment Problem.
For complete usage documentation, see: https://software.clapper.org/munkres/
Project details
Release history release notifications | rss feed.
Sep 15, 2020
Feb 15, 2019
Feb 12, 2019
Jun 27, 2017
May 23, 2017
Jan 2, 2017
Jun 22, 2016
Dec 3, 2014
Nov 3, 2013
Mar 29, 2010
Aug 2, 2009
Jun 30, 2008
Download files
Download the file for your platform. If you're not sure which to choose, learn more about installing packages .
Source Distribution
Uploaded Sep 15, 2020 Source
Built Distribution
Uploaded Sep 15, 2020 Python 2 Python 3
Hashes for munkres-1.1.4.tar.gz
Algorithm | Hash digest | |
---|---|---|
SHA256 | ||
MD5 | ||
BLAKE2b-256 |
Hashes for munkres-1.1.4-py2.py3-none-any.whl
Algorithm | Hash digest | |
---|---|---|
SHA256 | ||
MD5 | ||
BLAKE2b-256 |
- português (Brasil)
Supported by
Stack Exchange Network
Stack Exchange network consists of 183 Q&A communities including Stack Overflow , the largest, most trusted online community for developers to learn, share their knowledge, and build their careers.
Q&A for work
Connect and share knowledge within a single location that is structured and easy to search.
Difference between solving Assignment Problem using the Hungarian Method vs. LP
When trying to solve for assignments given a cost matrix, what is the difference between
using Scipy's linear_sum_assignment function (which I think uses the Hungarian method)
describing the LP problem using a objective function with many boolean variables, add in the appropriate constraints and send it to a solver, such as through scipy.optimize.linprog ?
Is the later method slower than Hungarian method's O(N^3) but allows for more constraints to be added?
- linear-programming
- combinatorial-optimization
- assignment-problem
- $\begingroup$ The main difference between a mathematical model and a heuristic algorithm to solve a specific problem is more likely to prove optimality rather feasibility. Now, one can decide which one to be selected in order to satisfy needs. $\endgroup$ – A.Omidi Commented Mar 20, 2022 at 7:54
- 4 $\begingroup$ @A.Omidi the Hungarian method is an exact algorithm $\endgroup$ – fontanf Commented Mar 20, 2022 at 8:26
- $\begingroup$ @fontanf, you are right. What I said was to compare the exact and heuristic methods and it is not specific to Hungarian alg. Thanks for your hint. $\endgroup$ – A.Omidi Commented Mar 20, 2022 at 10:32
The main differences probably are that there is a somewhat large overhead you have to pay when solving the AP as a linear program: You have to build an LP model and ship it to a solver. In addition, an LP solver is a generalist. It solves all LP problems and focus in development is to be fast on average on all LPs and also to be fast-ish in the pathological cases.
When using the Hungarian method, you do not build a model, you just pass the cost matrix to a tailored algorithm. You will then use an algorithm developed for that specific problem to solve it. Hence, it will most likely solve it faster since it is a specialist.
So if you want to solve an AP you should probably use the tailored algorithm. If you plan on extending your model to handle other more general constraints as well, you might need the LP after all.
Edit: From a simple test in Python, my assumption is confirmed in this specific setup (which is to the advantage of the Hungarian method, I believe). The set up is as follows:
- A size is chosen in $n\in \{5,10,\dots,500\}$
- A cost matrix is generated. Each coefficient $c_{ij}$ is generated as a uniformly distributed integer in the range $[250,999]$ .
- The instance is solved using both linear_sum_assignment and as a linear program. The solution time is measured as wall clock time and only the time spent by linear_sum_assignment and the solve function is timed (not building the LP and not not generating the instance)
For each size, I have generated and solved ten instances, and I report the average time only.
And then there is of course the "but". I am not a ninja in Python and I have used pyomo for modelling the LPs. I believe that pyomo is known to be slow-ish whenbuilding models, hence I have only timed the solver.solve(model) part of the code - not building the model. There is however possibly a hugh overhead cost coming from pyomo translating the model to "gurobian" (I use gurobi as solver).

- 1 $\begingroup$ Do you have some benchmark results to support this claim? Intuitively, I would have thought that the Hungarian method would be much slower in practice $\endgroup$ – fontanf Commented Mar 20, 2022 at 7:39
- $\begingroup$ @fontanf I only have anecdotal proof from past experiments. Maybe an LP solver can work faster for repeated solves, where you exploit that the model is already build and basis info is available. But I honestly don't know. $\endgroup$ – Sune Commented Mar 20, 2022 at 9:37
- 1 $\begingroup$ It might be the case that the Hungarian method is faster for small problems (due to the overhead Sune mentioned for setting up an LP model) while simplex (or dual simplex, or maybe barrier) might be faster for large models because the setup cost is "amortized" better. (I'm just speculating here.) $\endgroup$ – prubin ♦ Commented Mar 20, 2022 at 15:30
- 2 $\begingroup$ The Hungarian algorithm is, of course, O(n^3) for fully dense assignment problems. I don't know if there is a simplex bound explicitly for assignments. Simplex is exponential in the worst case and linear in variables plus constraints (n^2 + 2n here) in practice. But assignments are highly degenerate (n positive basics out of 2n rows). Dual simplex may fare better than primal. Hungarian is all integer for integer costs, whereas a standard simplex code won't be unless it knows to detect that in preprocessing. That may lead to some overhead for linear algebra. Ha, an idea for a class project! $\endgroup$ – mjsaltzman Commented Mar 20, 2022 at 17:04
- 1 $\begingroup$ Just for the sake of completeness, here 's the same with gurobipy instead of Pyomo. On my machine, all LPs (n = 500) are solved in less than a second compared to roughly 4 seconds with Pyomo. $\endgroup$ – joni Commented Mar 21, 2022 at 16:21
Your Answer
Sign up or log in, post as a guest.
Required, but never shown
By clicking “Post Your Answer”, you agree to our terms of service and acknowledge you have read our privacy policy .
Not the answer you're looking for? Browse other questions tagged linear-programming combinatorial-optimization assignment-problem or ask your own question .
- Featured on Meta
- Upcoming sign-up experiments related to tags
Hot Network Questions
- How are "pursed" and "rounded" synonymous?
- Why is completeness (as in Gödel completeness theorem) a desirable feature?
- A chess engine in Java: generating white pawn moves
- Co-authors with little contribution
- Is it better to show fake sympathy to maintain a good atmosphere?
- How to produce this table: Probability datatable with multirow
- How to model an optimization problem with mutual exclusivity of two variables, without introducing integer variables?
- Drawing waves using tikz in latex
- Diagnosing tripped breaker on the dishwasher circuit?
- How many different kinds of fairy units?
- What is the relationship between gravitation, centripetal and centrifugal force on the Earth?
- How to join two PCBs with a very small separation?
- What does this symbol do? Box with 1
- What does "vultures on someone's shoulder" mean?
- Why can't I conserve mass instead of moles and apply ratio in this problem?
- Should mail addresses for logins be stored hashed to minimize impact of data loss?
- How can I take apart a bookshelf?
- How to bid a very strong hand with values in only 2 suits?
- A 90s (maybe) made-for-TV movie (maybe) about a group of trainees on a spaceship. There is some kind of emergency and all experienced officers die
- How to use IX as a return stack?
- Can a planet have a warm, tropical climate both at the poles and at the equator?
- Have there been any scholarly attempts and/or consensus as regards the missing lines of "The Ruin"?
- Power of multiplication of powers
- Why only Balmer series of hydrogen spectrum is visible?
- Implementation of the Hungarian algorithm
- Connection to the Successive Shortest Path Algorithm
- Task examples
- Practice Problems
Hungarian algorithm for solving the assignment problem ¶
Statement of the assignment problem ¶.
There are several standard formulations of the assignment problem (all of which are essentially equivalent). Here are some of them:
There are $n$ jobs and $n$ workers. Each worker specifies the amount of money they expect for a particular job. Each worker can be assigned to only one job. The objective is to assign jobs to workers in a way that minimizes the total cost.
Given an $n \times n$ matrix $A$ , the task is to select one number from each row such that exactly one number is chosen from each column, and the sum of the selected numbers is minimized.
Given an $n \times n$ matrix $A$ , the task is to find a permutation $p$ of length $n$ such that the value $\sum A[i]\left[p[i]\right]$ is minimized.
Consider a complete bipartite graph with $n$ vertices per part, where each edge is assigned a weight. The objective is to find a perfect matching with the minimum total weight.
It is important to note that all the above scenarios are " square " problems, meaning both dimensions are always equal to $n$ . In practice, similar " rectangular " formulations are often encountered, where $n$ is not equal to $m$ , and the task is to select $\min(n,m)$ elements. However, it can be observed that a "rectangular" problem can always be transformed into a "square" problem by adding rows or columns with zero or infinite values, respectively.
We also note that by analogy with the search for a minimum solution, one can also pose the problem of finding a maximum solution. However, these two problems are equivalent to each other: it is enough to multiply all the weights by $-1$ .

Hungarian algorithm ¶
Historical reference ¶.
The algorithm was developed and published by Harold Kuhn in 1955. Kuhn himself gave it the name "Hungarian" because it was based on the earlier work by Hungarian mathematicians Dénes Kőnig and Jenő Egerváry. In 1957, James Munkres showed that this algorithm runs in (strictly) polynomial time, independently from the cost. Therefore, in literature, this algorithm is known not only as the "Hungarian", but also as the "Kuhn-Mankres algorithm" or "Mankres algorithm". However, it was recently discovered in 2006 that the same algorithm was invented a century before Kuhn by the German mathematician Carl Gustav Jacobi . His work, About the research of the order of a system of arbitrary ordinary differential equations , which was published posthumously in 1890, contained, among other findings, a polynomial algorithm for solving the assignment problem. Unfortunately, since the publication was in Latin, it went unnoticed among mathematicians.
It is also worth noting that Kuhn's original algorithm had an asymptotic complexity of $\mathcal{O}(n^4)$ , and only later Jack Edmonds and Richard Karp (and independently Tomizawa ) showed how to improve it to an asymptotic complexity of $\mathcal{O}(n^3)$ .
The $\mathcal{O}(n^4)$ algorithm ¶
To avoid ambiguity, we note right away that we are mainly concerned with the assignment problem in a matrix formulation (i.e., given a matrix $A$ , you need to select $n$ cells from it that are in different rows and columns). We index arrays starting with $1$ , i.e., for example, a matrix $A$ has indices $A[1 \dots n][1 \dots n]$ .
We will also assume that all numbers in matrix A are non-negative (if this is not the case, you can always make the matrix non-negative by adding some constant to all numbers).
Let's call a potential two arbitrary arrays of numbers $u[1 \ldots n]$ and $v[1 \ldots n]$ , such that the following condition is satisfied:
(As you can see, $u[i]$ corresponds to the $i$ -th row, and $v[j]$ corresponds to the $j$ -th column of the matrix).
Let's call the value $f$ of the potential the sum of its elements:
On one hand, it is easy to see that the cost of the desired solution $sol$ is not less than the value of any potential.
Lemma. $sol\geq f.$
The desired solution of the problem consists of $n$ cells of the matrix $A$ , so $u[i]+v[j]\leq A[i][j]$ for each of them. Since all the elements in $sol$ are in different rows and columns, summing these inequalities over all the selected $A[i][j]$ , you get $f$ on the left side of the inequality, and $sol$ on the right side.
On the other hand, it turns out that there is always a solution and a potential that turns this inequality into equality . The Hungarian algorithm described below will be a constructive proof of this fact. For now, let's just pay attention to the fact that if any solution has a cost equal to any potential, then this solution is optimal .
Let's fix some potential. Let's call an edge $(i,j)$ rigid if $u[i]+v[j]=A[i][j].$
Recall an alternative formulation of the assignment problem, using a bipartite graph. Denote with $H$ a bipartite graph composed only of rigid edges. The Hungarian algorithm will maintain, for the current potential, the maximum-number-of-edges matching $M$ of the graph $H$ . As soon as $M$ contains $n$ edges, then the solution to the problem will be just $M$ (after all, it will be a solution whose cost coincides with the value of a potential).
Let's proceed directly to the description of the algorithm .
Step 1. At the beginning, the potential is assumed to be zero ( $u[i]=v[i]=0$ for all $i$ ), and the matching $M$ is assumed to be empty.
Step 2. Further, at each step of the algorithm, we try, without changing the potential, to increase the cardinality of the current matching $M$ by one (recall that the matching is searched in the graph of rigid edges $H$ ). To do this, the usual Kuhn Algorithm for finding the maximum matching in bipartite graphs is used. Let us recall the algorithm here. All edges of the matching $M$ are oriented in the direction from the right part to the left one, and all other edges of the graph $H$ are oriented in the opposite direction.
Recall (from the terminology of searching for matchings) that a vertex is called saturated if an edge of the current matching is adjacent to it. A vertex that is not adjacent to any edge of the current matching is called unsaturated. A path of odd length, in which the first edge does not belong to the matching, and for all subsequent edges there is an alternating belonging to the matching (belongs/does not belong) - is called an augmenting path. From all unsaturated vertices in the left part, a depth-first or breadth-first traversal is started. If, as a result of the search, it was possible to reach an unsaturated vertex of the right part, we have found an augmenting path from the left part to the right one. If we include odd edges of the path and remove the even ones in the matching (i.e. include the first edge in the matching, exclude the second, include the third, etc.), then we will increase the matching cardinality by one.
If there was no augmenting path, then the current matching $M$ is maximal in the graph $H$ .
Step 3. If at the current step, it is not possible to increase the cardinality of the current matching, then a recalculation of the potential is performed in such a way that, at the next steps, there will be more opportunities to increase the matching.
Denote by $Z_1$ the set of vertices of the left part that were visited during the last traversal of Kuhn's algorithm, and through $Z_2$ the set of visited vertices of the right part.
Let's calculate the value $\Delta$ :
Lemma. $\Delta > 0.$
Suppose $\Delta=0$ . Then there exists a rigid edge $(i,j)$ with $i\in Z_1$ and $j\notin Z_2$ . It follows that the edge $(i,j)$ must be oriented from the right part to the left one, i.e. $(i,j)$ must be included in the matching $M$ . However, this is impossible, because we could not get to the saturated vertex $i$ except by going along the edge from j to i. So $\Delta > 0$ .
Now let's recalculate the potential in this way:
for all vertices $i\in Z_1$ , do $u[i] \gets u[i]+\Delta$ ,
for all vertices $j\in Z_2$ , do $v[j] \gets v[j]-\Delta$ .
Lemma. The resulting potential is still a correct potential.
We will show that, after recalculation, $u[i]+v[j]\leq A[i][j]$ for all $i,j$ . For all the elements of $A$ with $i\in Z_1$ and $j\in Z_2$ , the sum $u[i]+v[j]$ does not change, so the inequality remains true. For all the elements with $i\notin Z_1$ and $j\in Z_2$ , the sum $u[i]+v[j]$ decreases by $\Delta$ , so the inequality is still true. For the other elements whose $i\in Z_1$ and $j\notin Z_2$ , the sum increases, but the inequality is still preserved, since the value $\Delta$ is, by definition, the maximum increase that does not change the inequality.
Lemma. The old matching $M$ of rigid edges is valid, i.e. all edges of the matching will remain rigid.
For some rigid edge $(i,j)$ to stop being rigid as a result of a change in potential, it is necessary that equality $u[i] + v[j] = A[i][j]$ turns into inequality $u[i] + v[j] < A[i][j]$ . However, this can happen only when $i \notin Z_1$ and $j \in Z_2$ . But $i \notin Z_1$ implies that the edge $(i,j)$ could not be a matching edge.
Lemma. After each recalculation of the potential, the number of vertices reachable by the traversal, i.e. $|Z_1|+|Z_2|$ , strictly increases.
First, note that any vertex that was reachable before recalculation, is still reachable. Indeed, if some vertex is reachable, then there is some path from reachable vertices to it, starting from the unsaturated vertex of the left part; since for edges of the form $(i,j),\ i\in Z_1,\ j\in Z_2$ the sum $u[i]+v[j]$ does not change, this entire path will be preserved after changing the potential. Secondly, we show that after a recalculation, at least one new vertex will be reachable. This follows from the definition of $\Delta$ : the edge $(i,j)$ which $\Delta$ refers to will become rigid, so vertex $j$ will be reachable from vertex $i$ .
Due to the last lemma, no more than $n$ potential recalculations can occur before an augmenting path is found and the matching cardinality of $M$ is increased. Thus, sooner or later, a potential that corresponds to a perfect matching $M^*$ will be found, and $M^*$ will be the answer to the problem. If we talk about the complexity of the algorithm, then it is $\mathcal{O}(n^4)$ : in total there should be at most $n$ increases in matching, before each of which there are no more than $n$ potential recalculations, each of which is performed in time $\mathcal{O}(n^2)$ .
We will not give the implementation for the $\mathcal{O}(n^4)$ algorithm here, since it will turn out to be no shorter than the implementation for the $\mathcal{O}(n^3)$ one, described below.
The $\mathcal{O}(n^3)$ algorithm ¶
Now let's learn how to implement the same algorithm in $\mathcal{O}(n^3)$ (for rectangular problems $n \times m$ , $\mathcal{O}(n^2m)$ ).
The key idea is to consider matrix rows one by one , and not all at once. Thus, the algorithm described above will take the following form:
Consider the next row of the matrix $A$ .
While there is no increasing path starting in this row, recalculate the potential.
As soon as an augmenting path is found, propagate the matching along it (thus including the last edge in the matching), and restart from step 1 (to consider the next line).
To achieve the required complexity, it is necessary to implement steps 2-3, which are performed for each row of the matrix, in time $\mathcal{O}(n^2)$ (for rectangular problems in $\mathcal{O}(nm)$ ).
To do this, recall two facts proved above:
With a change in the potential, the vertices that were reachable by Kuhn's traversal will remain reachable.
In total, only $\mathcal{O}(n)$ recalculations of the potential could occur before an augmenting path was found.
From this follow these key ideas that allow us to achieve the required complexity:
To check for the presence of an augmenting path, there is no need to start the Kuhn traversal again after each potential recalculation. Instead, you can make the Kuhn traversal in an iterative form : after each recalculation of the potential, look at the added rigid edges and, if their left ends were reachable, mark their right ends reachable as well and continue the traversal from them.
Developing this idea further, we can present the algorithm as follows: at each step of the loop, the potential is recalculated. Subsequently, a column that has become reachable is identified (which will always exist as new reachable vertices emerge after every potential recalculation). If the column is unsaturated, an augmenting chain is discovered. Conversely, if the column is saturated, the matching row also becomes reachable.
To quickly recalculate the potential (faster than the $\mathcal{O}(n^2)$ naive version), you need to maintain auxiliary minima for each of the columns:
$minv[j]=\min_{i\in Z_1} A[i][j]-u[i]-v[j].$
It's easy to see that the desired value $\Delta$ is expressed in terms of them as follows:
$\Delta=\min_{j\notin Z_2} minv[j].$
Thus, finding $\Delta$ can now be done in $\mathcal{O}(n)$ .
It is necessary to update the array $minv$ when new visited rows appear. This can be done in $\mathcal{O}(n)$ for the added row (which adds up over all rows to $\mathcal{O}(n^2)$ ). It is also necessary to update the array $minv$ when recalculating the potential, which is also done in time $\mathcal{O}(n)$ ( $minv$ changes only for columns that have not yet been reached: namely, it decreases by $\Delta$ ).
Thus, the algorithm takes the following form: in the outer loop, we consider matrix rows one by one. Each row is processed in time $\mathcal{O}(n^2)$ , since only $\mathcal{O}(n)$ potential recalculations could occur (each in time $\mathcal{O}(n)$ ), and the array $minv$ is maintained in time $\mathcal{O}(n^2)$ ; Kuhn's algorithm will work in time $\mathcal{O}(n^2)$ (since it is presented in the form of $\mathcal{O}(n)$ iterations, each of which visits a new column).
The resulting complexity is $\mathcal{O}(n^3)$ or, if the problem is rectangular, $\mathcal{O}(n^2m)$ .
Implementation of the Hungarian algorithm ¶
The implementation below was developed by Andrey Lopatin several years ago. It is distinguished by amazing conciseness: the entire algorithm consists of 30 lines of code .
The implementation finds a solution for the rectangular matrix $A[1\dots n][1\dots m]$ , where $n\leq m$ . The matrix is 1-based for convenience and code brevity: this implementation introduces a dummy zero row and zero column, which allows us to write many cycles in a general form, without additional checks.
Arrays $u[0 \ldots n]$ and $v[0 \ldots m]$ store potential. Initially, they are set to zero, which is consistent with a matrix of zero rows (Note that it is unimportant for this implementation whether or not the matrix $A$ contains negative numbers).
The array $p[0 \ldots m]$ contains a matching: for each column $j = 1 \ldots m$ , it stores the number $p[j]$ of the selected row (or $0$ if nothing has been selected yet). For the convenience of implementation, $p[0]$ is assumed to be equal to the number of the current row.
The array $minv[1 \ldots m]$ contains, for each column $j$ , the auxiliary minima necessary for a quick recalculation of the potential, as described above.
The array $way[1 \ldots m]$ contains information about where these minimums are reached so that we can later reconstruct the augmenting path. Note that, to reconstruct the path, it is sufficient to store only column values, since the row numbers can be taken from the matching (i.e., from the array $p$ ). Thus, $way[j]$ , for each column $j$ , contains the number of the previous column in the path (or $0$ if there is none).
The algorithm itself is an outer loop through the rows of the matrix , inside which the $i$ -th row of the matrix is considered. The first do-while loop runs until a free column $j0$ is found. Each iteration of the loop marks visited a new column with the number $j0$ (calculated at the last iteration; and initially equal to zero - i.e. we start from a dummy column), as well as a new row $i0$ - adjacent to it in the matching (i.e. $p[j0]$ ; and initially when $j0=0$ the $i$ -th row is taken). Due to the appearance of a new visited row $i0$ , you need to recalculate the array $minv$ and $\Delta$ accordingly. If $\Delta$ is updated, then the column $j1$ becomes the minimum that has been reached (note that with such an implementation $\Delta$ could turn out to be equal to zero, which means that the potential cannot be changed at the current step: there is already a new reachable column). After that, the potential and the $minv$ array are recalculated. At the end of the "do-while" loop, we found an augmenting path ending in a column $j0$ that can be "unrolled" using the ancestor array $way$ .
The constant INF is "infinity", i.e. some number, obviously greater than all possible numbers in the input matrix $A$ .
To restore the answer in a more familiar form, i.e. finding for each row $i = 1 \ldots n$ the number $ans[i]$ of the column selected in it, can be done as follows:
The cost of the matching can simply be taken as the potential of the zero column (taken with the opposite sign). Indeed, as you can see from the code, $-v[0]$ contains the sum of all the values of $\Delta$ , i.e. total change in potential. Although several values of $u[i]$ and $v[j]$ could change at once, the total change in the potential is exactly equal to $\Delta$ , since until there is an augmenting path, the number of reachable rows is exactly one more than the number of the reachable columns (only the current row $i$ does not have a "pair" in the form of a visited column):
Connection to the Successive Shortest Path Algorithm ¶
The Hungarian algorithm can be seen as the Successive Shortest Path Algorithm , adapted for the assignment problem. Without going into the details, let's provide an intuition regarding the connection between them.
The Successive Path algorithm uses a modified version of Johnson's algorithm as reweighting technique. This one is divided into four steps:
- Use the Bellman-Ford algorithm, starting from the sink $s$ and, for each node, find the minimum weight $h(v)$ of a path from $s$ to $v$ .
For every step of the main algorithm:
- Reweight the edges of the original graph in this way: $w(u,v) \gets w(u,v)+h(u)-h(v)$ .
- Use Dijkstra 's algorithm to find the shortest-paths subgraph of the original network.
- Update potentials for the next iteration.
Given this description, we can observe that there is a strong analogy between $h(v)$ and potentials: it can be checked that they are equal up to a constant offset. In addition, it can be shown that, after reweighting, the set of all zero-weight edges represents the shortest-path subgraph where the main algorithm tries to increase the flow. This also happens in the Hungarian algorithm: we create a subgraph made of rigid edges (the ones for which the quantity $A[i][j]-u[i]-v[j]$ is zero), and we try to increase the size of the matching.
In step 4, all the $h(v)$ are updated: every time we modify the flow network, we should guarantee that the distances from the source are correct (otherwise, in the next iteration, Dijkstra's algorithm might fail). This sounds like the update performed on the potentials, but in this case, they are not equally incremented.
To deepen the understanding of potentials, refer to this article .
Task examples ¶
Here are a few examples related to the assignment problem, from very trivial to less obvious tasks:
Given a bipartite graph, it is required to find in it the maximum matching with the minimum weight (i.e., first of all, the size of the matching is maximized, and secondly, its cost is minimized). To solve it, we simply build an assignment problem, putting the number "infinity" in place of the missing edges. After that, we solve the problem with the Hungarian algorithm, and remove edges of infinite weight from the answer (they could enter the answer if the problem does not have a solution in the form of a perfect matching).
Given a bipartite graph, it is required to find in it the maximum matching with the maximum weight . The solution is again obvious, all weights must be multiplied by minus one.
The task of detecting moving objects in images : two images were taken, as a result of which two sets of coordinates were obtained. It is required to correlate the objects in the first and second images, i.e. determine for each point of the second image, which point of the first image it corresponded to. In this case, it is required to minimize the sum of distances between the compared points (i.e., we are looking for a solution in which the objects have taken the shortest path in total). To solve, we simply build and solve an assignment problem, where the weights of the edges are the Euclidean distances between points.
The task of detecting moving objects by locators : there are two locators that can't determine the position of an object in space, but only its direction. Both locators (located at different points) received information in the form of $n$ such directions. It is required to determine the position of objects, i.e. determine the expected positions of objects and their corresponding pairs of directions in such a way that the sum of distances from objects to direction rays is minimized. Solution: again, we simply build and solve the assignment problem, where the vertices of the left part are the $n$ directions from the first locator, the vertices of the right part are the $n$ directions from the second locator, and the weights of the edges are the distances between the corresponding rays.
Covering a directed acyclic graph with paths : given a directed acyclic graph, it is required to find the smallest number of paths (if equal, with the smallest total weight) so that each vertex of the graph lies in exactly one path. The solution is to build the corresponding bipartite graph from the given graph and find the maximum matching of the minimum weight in it. See separate article for more details.
Tree coloring book . Given a tree in which each vertex, except for leaves, has exactly $k-1$ children. It is required to choose for each vertex one of the $k$ colors available so that no two adjacent vertices have the same color. In addition, for each vertex and each color, the cost of painting this vertex with this color is known, and it is required to minimize the total cost. To solve this problem, we use dynamic programming. Namely, let's learn how to calculate the value $d[v][c]$ , where $v$ is the vertex number, $c$ is the color number, and the value $d[v][c]$ itself is the minimum cost needed to color all the vertices in the subtree rooted at $v$ , and the vertex $v$ itself with color $c$ . To calculate such a value $d[v][c]$ , it is necessary to distribute the remaining $k-1$ colors among the children of the vertex $v$ , and for this, it is necessary to build and solve the assignment problem (in which the vertices of the left part are colors, the vertices of the right part are children, and the weights of the edges are the corresponding values of $d$ ). Thus, each value $d[v][c]$ is calculated using the solution of the assignment problem, which ultimately gives the asymptotic $\mathcal{O}(nk^4)$ .
If, in the assignment problem, the weights are not on the edges, but on the vertices, and only on the vertices of the same part , then it's not necessary to use the Hungarian algorithm: just sort the vertices by weight and run the usual Kuhn algorithm (for more details, see a separate article ).
Consider the following special case . Let each vertex of the left part be assigned some number $\alpha[i]$ , and each vertex of the right part $\beta[j]$ . Let the weight of any edge $(i,j)$ be equal to $\alpha[i]\cdot \beta[j]$ (the numbers $\alpha[i]$ and $\beta[j]$ are known). Solve the assignment problem. To solve it without the Hungarian algorithm, we first consider the case when both parts have two vertices. In this case, as you can easily see, it is better to connect the vertices in the reverse order: connect the vertex with the smaller $\alpha[i]$ to the vertex with the larger $\beta[j]$ . This rule can be easily generalized to an arbitrary number of vertices: you need to sort the vertices of the first part in increasing order of $\alpha[i]$ values, the second part in decreasing order of $\beta[j]$ values, and connect the vertices in pairs in that order. Thus, we obtain a solution with complexity of $\mathcal{O}(n\log n)$ .
The Problem of Potentials . Given a matrix $A[1 \ldots n][1 \ldots m]$ , it is required to find two arrays $u[1 \ldots n]$ and $v[1 \ldots m]$ such that, for any $i$ and $j$ , $u[i] + v[j] \leq a[i][j]$ and the sum of elements of arrays $u$ and $v$ is maximum. Knowing the Hungarian algorithm, the solution to this problem will not be difficult: the Hungarian algorithm just finds such a potential $u, v$ that satisfies the condition of the problem. On the other hand, without knowledge of the Hungarian algorithm, it seems almost impossible to solve such a problem.
This task is also called the dual problem of the assignment problem: minimizing the total cost of the assignment is equivalent to maximizing the sum of the potentials.
Literature ¶
Ravindra Ahuja, Thomas Magnanti, James Orlin. Network Flows [1993]
Harold Kuhn. The Hungarian Method for the Assignment Problem [1955]
James Munkres. Algorithms for Assignment and Transportation Problems [1957]
Practice Problems ¶
UVA - Crime Wave - The Sequel
UVA - Warehouse
SGU - Beloved Sons
UVA - The Great Wall Game
UVA - Jogging Trails
- Alessandro Minisini (92.97%)
- Oleksandr Kulkov (7.03%)

Index Assignment problem Hungarian algorithm Solve online
The Hungarian algorithm: An example
We consider an example where four jobs (J1, J2, J3, and J4) need to be executed by four workers (W1, W2, W3, and W4), one job per worker. The matrix below shows the cost of assigning a certain worker to a certain job. The objective is to minimize the total cost of the assignment.
82 | 83 | 69 | 92 | |
77 | 37 | 49 | 92 | |
11 | 69 | 5 | 86 | |
8 | 9 | 98 | 23 |
Below we will explain the Hungarian algorithm using this example. Note that a general description of the algorithm can be found here .
Step 1: Subtract row minima
We start with subtracting the row minimum from each row. The smallest element in the first row is, for example, 69. Therefore, we substract 69 from each element in the first row. The resulting matrix is:
13 | 14 | 0 | 23 | (-69) | |
40 | 0 | 12 | 55 | (-37) | |
6 | 64 | 0 | 81 | (-5) | |
0 | 1 | 90 | 15 | (-8) |
Step 2: Subtract column minima
Similarly, we subtract the column minimum from each column, giving the following matrix:
13 | 14 | 0 | 8 | |
40 | 0 | 12 | 40 | |
6 | 64 | 0 | 66 | |
0 | 1 | 90 | 0 | |
(-15) |
Step 3: Cover all zeros with a minimum number of lines
We will now determine the minimum number of lines (horizontal or vertical) that are required to cover all zeros in the matrix. All zeros can be covered using 3 lines:
13 | 14 | 0 | 8 | ||
40 | 0 | 12 | 40 | ||
6 | 64 | 0 | 66 | ||
0 | 1 | 90 | 0 | ||
Step 4: Create additional zeros
First, we find that the smallest uncovered number is 6. We subtract this number from all uncovered elements and add it to all elements that are covered twice. This results in the following matrix:
7 | 8 | 0 | 2 | |
40 | 0 | 18 | 40 | |
0 | 58 | 0 | 60 | |
0 | 1 | 96 | 0 |
Now we return to Step 3.
Again, We determine the minimum number of lines required to cover all zeros in the matrix. Now there are 4 lines required:
7 | 8 | 0 | 2 | ||
40 | 0 | 18 | 40 | ||
0 | 58 | 0 | 60 | ||
0 | 1 | 96 | 0 |
Because the number of lines required (4) equals the size of the matrix ( n =4), an optimal assignment exists among the zeros in the matrix. Therefore, the algorithm stops.
The optimal assignment
The following zeros cover an optimal assignment:
This corresponds to the following optimal assignment in the original cost matrix:
Thus, worker 1 should perform job 3, worker 2 job 2, worker 3 job 1, and worker 4 should perform job 4. The total cost of this optimal assignment is to 69 + 37 + 11 + 23 = 140.
Solve your own problem online
HungarianAlgorithm.com © 2013-2024
- Stack Overflow for Teams Where developers & technologists share private knowledge with coworkers
- Advertising & Talent Reach devs & technologists worldwide about your product, service or employer brand
- OverflowAI GenAI features for Teams
- OverflowAPI Train & fine-tune LLMs
- Labs The future of collective knowledge sharing
- About the company Visit the blog
Collectives™ on Stack Overflow
Find centralized, trusted content and collaborate around the technologies you use most.
Q&A for work
Connect and share knowledge within a single location that is structured and easy to search.
Get early access and see previews of new features.
Pass by reference and slice assignment
In Python, lists are passed by reference to functions. If that is so, what's happening here?
- 3 a[:] = a[:2] is what you should be doing. – Ashwini Chaudhary Commented Jul 26, 2017 at 19:27
- 2 Or del a[2:] would work as well, and be more readable. – Artyer Commented Jul 26, 2017 at 19:29
- f receives a reference to the list, not to the b variable; assigning to a will not affect the b variable. While f is passed a reference, "pass by reference" has a specific meaning that does not apply to Python's parameter passing model. – user2357112 Commented Jul 26, 2017 at 19:34
- 1 nedbatchelder.com/text/names.html is the best reference I've seen for learning about how this part of Python works. – user2357112 Commented Jul 26, 2017 at 19:35
3 Answers 3
In the statement:
you are creating a new local (to f() ) variable which you call using the same name as the input argument a .
That is, what you are doing is equivalent to:
Instead, you should be changing a in place such as:
When you do:
it reassigns a to a new value (The first two items of the list).
All Python arguments are passed by reference. You need to change the object that it is refered to, instead of making a refer to a new object.
Where the first and last assign to slices of the list, changing the list in-place (affecting its value), and the middle one also changes the value of the list, by deleting the rest of the elements.

- array slices are not passed by reference. – David Bandel Commented Dec 24, 2021 at 10:13
- @DavidBandel If you are talking about something like f(a[:2]) , that passes the result of evaluating a[:2] "by reference" to f. On lists, this creates a copy of some part of the list, but that is beside the point (that Python has no "pass-by-value" semantics). For example, f(x := a[:2]); x[0] is a[0] could be False for a list. – Artyer Commented Dec 25, 2021 at 0:52
- How can you even regard something as having been passed by reference if you are never again able to access the thing our "reference" is a pointer to? I'd regard it as a grey area in the value/reference dichotomy. – David Bandel Commented Jan 3, 2022 at 14:22
Indeed the objects are passed by reference but a = a[:2] basically creates a new local variable that points to slice of the list.
To modify the list object in place you can assign it to its slice(slice assignment).
Consider a and b here equivalent to your global b and local a , here assigning a to new object doesn't affect b :
Slice assignment work as expected:
Related: What is the difference between slice assignment that slices the whole list and direct assignment?

Your Answer
Reminder: Answers generated by artificial intelligence tools are not allowed on Stack Overflow. Learn more
Sign up or log in
Post as a guest.
Required, but never shown
By clicking “Post Your Answer”, you agree to our terms of service and acknowledge you have read our privacy policy .
Not the answer you're looking for? Browse other questions tagged python list function reference or ask your own question .
- Featured on Meta
- Upcoming sign-up experiments related to tags
- The return of Staging Ground to Stack Overflow
- Should we burninate the [lib] tag?
- Policy: Generative AI (e.g., ChatGPT) is banned
- What makes a homepage useful for logged-in users
Hot Network Questions
- Huygens' principle and the laws of reflection/refraction
- Visit USA via land border for French citizen
- Why did Geordi have his visor replaced with ocular implants between Generations and First Contact?
- What is the meaning of the angle bracket syntax in `apt depends`?
- Would a spaceport on Ceres make sense?
- Are both vocal cord and vocal chord correct?
- Why only Balmer series of hydrogen spectrum is visible?
- Drawing waves using tikz in latex
- Montreal Airport US arrival to International Departure
- Is it consistent with ZFC that the real line is approachable by sets with no accumulation points?
- What to fill in the document number while applying for Schengen visa "C"?
- Can I tell a MILP solver to prefer solutions with fewer fractions?
- A 90s (maybe) made-for-TV movie (maybe) about a group of trainees on a spaceship. There is some kind of emergency and all experienced officers die
- Algorithm to evaluate "connectedness" of a binary matrix
- Reconstructing Euro results
- Can a planet have a warm, tropical climate both at the poles and at the equator?
- what is the difference between prayer (προσευχῇ) and prayer also translated as supplication (δέησις) in Philippians 4:6?
- What is the relationship between gravitation, centripetal and centrifugal force on the Earth?
- Tombs of Ancients
- Collaborators write their departments for my (undergraduate) affiliation
- Could a transparent frequency-altering material be possible?
- Could space habitats have large transparent roofs?
- What stops a plane from rolling when the ailerons are returned to their neutral position?
- How will the ISS be decommissioned?
Navigation Menu
Search code, repositories, users, issues, pull requests..., provide feedback.
We read every piece of feedback, and take your input very seriously.
Saved searches
Use saved searches to filter your results more quickly.
To see all available qualifiers, see our documentation .
hungarian-algorithm
Here are 23 public repositories matching this topic..., kcg2015 / vehicle-detection-and-tracking.
Computer vision based vehicle detection and tracking using Tensorflow Object Detection API and Kalman-filtering
- Updated May 23, 2020
wmuron / motpy
Library for tracking-by-detection multi object tracking implemented in python
- Updated Apr 24, 2023
mayorx / hungarian-algorithm
(Kuhn-Munkres) numpy implementation, rectangular matrix is supported (|X| <= |Y|). 100x100000 in 0.153 s.
- Updated Dec 8, 2022
sharathadavanne / hungarian-net
Deep-learning-based implementation of the popular Hungarian algorithm that helps solve the assignment problem.
- Updated Aug 31, 2023
tharakarehan / people-counter
A human detection, tracking and counting system built using deep learning based computer vision.
- Updated Dec 21, 2021
Ibrahim5aad / kuhn-munkres-algorithm
A python program to solve assignment problem by the Kuhn–Munkres algorithm (The Hungarian Method).
- Updated Oct 22, 2021
tharakarehan / highway-lane-violation-detector
Desktop app for detecting highway lane violations. Illegal parking, Wrong direction, Illegal over taking can be detected
guoyangqin / approx_bipartite_match
Python implementation of an approximate Euclidean bipartite matching algorithm proposed by a 2004 paper "A Near-Linear Constant-Factor Approximation for Euclidean Bipartite Matching?" by Pankaj Agarwal and Kasturi Varadarajan.
- Updated Jun 11, 2020
yas-sim / openvino-multi-camera-position-estimation-demo
Using multiple cameras to estimate the people's position. Works with OpenVINO toolkit.
- Updated Jul 20, 2021
oleksiygarnik / vehicles-trajectory-detection
A comprehensive solution for detecting and tracking vehicle trajectories using YOLO, Kalman Filter, and the Hungarian Algorithm. Includes a Flask web application for easy video upload and processing.
- Updated May 30, 2024
asujaykk / MultiObjectTracker
An object tracker for realtime multiple objects tracking
- Updated Jan 9, 2023
TmohamedashrafT / Pedestrian-Tracking-Using-Kalman-Filter
Implement the Kalman filter and establish a pipeline for pedestrian detection and tracking using YOLOv5 and the Hungarian algorithm
- Updated Nov 19, 2023
ITU-GammaTeam / GammaSwarm-Demo
The 2022 Gamma Team Integrated Swarm System Demo Repository
- Updated Dec 14, 2023
s-matke / TS-OI
Experimental project solving Travelling Salesman Problem via genetic algorithm, hungarian method, etc. University project for class Operations Research.
- Updated Apr 3, 2023
daniel-jakob / Bottle_Cap_Map
Implementing a Python-based project using computer vision to analyse a wooden beer bottle cap display (shaped like Germany), aiming to correlate bottle caps with their geographic references by minimizing spatial distances between map holes and physical locations.
- Updated Feb 12, 2024
pleiadian53 / biomedical-app
A collection of prototypes for biomedical applications. E.g. optimal protein-drug binding via hungarian algorithm.
- Updated Dec 6, 2020
IsmaelMekene / meteor-realtime-object-tracking
- Updated Nov 24, 2022
Nachiket-Bhide / Masters-Thesis-Research-Work
This repository contains all program files and datasets used in implementation of Masters Thesis Research Work for the topic - "Efficient Clustering via Kernel Principal Component Analysis and Optimal One Dimensional Clustering".
- Updated Mar 16, 2021
Improve this page
Add a description, image, and links to the hungarian-algorithm topic page so that developers can more easily learn about it.
Curate this topic
Add this topic to your repo
To associate your repository with the hungarian-algorithm topic, visit your repo's landing page and select "manage topics."

COMMENTS
Hungarian Algorithm. A Python 3 graph implementation of the Hungarian Algorithm (a.k.a. the Kuhn-Munkres algorithm), an O(n^3) solution for the assignment problem, or maximum/minimum-weighted bipartite matching problem. Usage Install pip3 install hungarian-algorithm Import from hungarian_algorithm import algorithm Inputs
The Hungarian algorithm, aka Munkres assignment algorithm, utilizes the following theorem for polynomial runtime complexity (worst case O(n 3)) and guaranteed optimality: If a number is added to or subtracted from all of the entries of any one row or column of a cost matrix, then an optimal assignment for the resulting cost matrix is also an ...
For implementing the above algorithm, the idea is to use the max_cost_assignment() function defined in the dlib library. This function is an implementation of the Hungarian algorithm (also known as the Kuhn-Munkres algorithm) which runs in O(N 3) time. It solves the optimal assignment problem. Below is the implementation of the above approach:
An array of row indices and one of corresponding column indices giving the optimal assignment. The cost of the assignment can be computed as cost_matrix[row_ind, col_ind].sum(). The row indices will be sorted; in the case of a square cost matrix they will be equal to numpy.arange(cost_matrix.shape[0]). The linear sum assignment problem [1] is ...
Hungarian Algorithm & Python Code Step by Step. In this section, we will show how to use the Hungarian algorithm to solve linear assignment problems and find the minimum combinations in the matrix. Of course, the Hungarian algorithm can also be used to find the maximum combination. Step 0. Prepare Operations.
This is the assignment problem, for which the Hungarian Algorithm offers a solution. Notice: although no one has chosen LB, the algorithm will still assign a player there. In fact, the first step of the algorithm is to create a complete bipartite graph (all possible edges exist), giving new edges weight 0. Define a weighted bipartite graph in ...
Munkres (Hungarian) algorithm for the Assignment Problem. ... (also called the Hungarian algorithm or the Kuhn-Munkres algorithm), useful for solving the Assignment Problem. ... Developed and maintained by the Python community, for the Python community. Donate today! "PyPI", "Python Package Index", ...
This repo contains a crude, but a good shot at, an implementation in Python of the Hungarian Algorithm (or the Munkres Assignment ALgorithm); one of the coolest algorithms I've studied in graph theory (you can't say it isn't). For a full description of the algorithm and its theoretical basis, visit the Wikipedia link.
We'll handle the assignment problem with the Hungarian algorithm (or Kuhn-Munkres algorithm). I'll illustrate two different implementations of this algorithm, both graph theoretic, one easy and fast to implement with O (n4) complexity, and the other one with O (n3) complexity, but harder to implement.
Implementation of the Hungarian (Munkres) Algorithm using Python and NumPy. Usage: hungarian = Hungarian(costMatrix) hungarian.calculate() or hungarian = Hungarian() hungarian.calculate(costMatrix) Handle Profit matrix: hungarian = Hungarian(profitMatrix, isProfitMatrix=True) or costMatrix = Hungarian.makeCostMatrix(profitMatrix) The matrix will be automatically padded if it is not square.
When trying to solve for assignments given a cost matrix, what is the difference between. using Scipy's linear_sum_assignment function (which I think uses the Hungarian method). describing the LP problem using a objective function with many boolean variables, add in the appropriate constraints and send it to a solver, such as through scipy.optimize.linprog?
The Hungarian method is a combinatorial optimization algorithm that solves the assignment problem in polynomial time and which anticipated later primal-dual methods.It was developed and published in 1955 by Harold Kuhn, who gave it the name "Hungarian method" because the algorithm was largely based on the earlier works of two Hungarian mathematicians, Dénes Kőnig and Jenő Egerváry.
Is there an extension of the Hungarian algorithm that caters for the assignment of multiple jobs per worker? In its simplest form, the algorithm assigns a single job to a single worker. My application is a profit maximization problem, with 3 workers and 180 jobs. I'll add constraints as well (minimum of 50 jobs assigned to each worker).
The Hungarian algorithm can be seen as the Successive Shortest Path Algorithm, adapted for the assignment problem. Without going into the details, let's provide an intuition regarding the connection between them. The Successive Path algorithm uses a modified version of Johnson's algorithm as reweighting technique.
STEP 1: Finding the minimum from each row and substracting it from all the other row elements. In the table 1 the minimum elements from each row are highlighted. Table 2 shows the new table after ...
The Hungarian Network (Hnet) is the deep-learning-based implementation of the popular Hungarian algorithm that helps solve the assignment problem. Implementing this algorithm using a DNN allows us to integrate it with other deep-learning tasks that require permutation invariant training (PIT) and train them in a completely differentiable manner without the need of PIT.
The Hungarian algorithm: An example. We consider an example where four jobs (J1, J2, J3, and J4) need to be executed by four workers (W1, W2, W3, and W4), one job per worker. The matrix below shows the cost of assigning a certain worker to a certain job. The objective is to minimize the total cost of the assignment.
Add this topic to your repo. To associate your repository with the hungarian-assignment topic, visit your repo's landing page and select "manage topics." GitHub is where people build software. More than 100 million people use GitHub to discover, fork, and contribute to over 420 million projects.
Hungarian Assignment Python program to solve the assignment problem using Hungarian method. Motivation. During my four year undergraduate course majoring in Computer Science, we had an open elective course called as Operations Research. It employs techniques from other mathematical sciences, such as mathematical modeling, statistical analysis ...
When you do: a = a[:2] it reassigns a to a new value (The first two items of the list).. All Python arguments are passed by reference. You need to change the object that it is refered to, instead of making a refer to a new object.. a[2:] = [] # or del a[2:] # or a[:] = a[:2]
To associate your repository with the hungarian-algorithm topic, visit your repo's landing page and select "manage topics." GitHub is where people build software. More than 100 million people use GitHub to discover, fork, and contribute to over 420 million projects.