- En español – ExME
- Em português – EME

Systematic reviews vs meta-analysis: what’s the difference?
Posted on 24th July 2023 by Verónica Tanco Tellechea
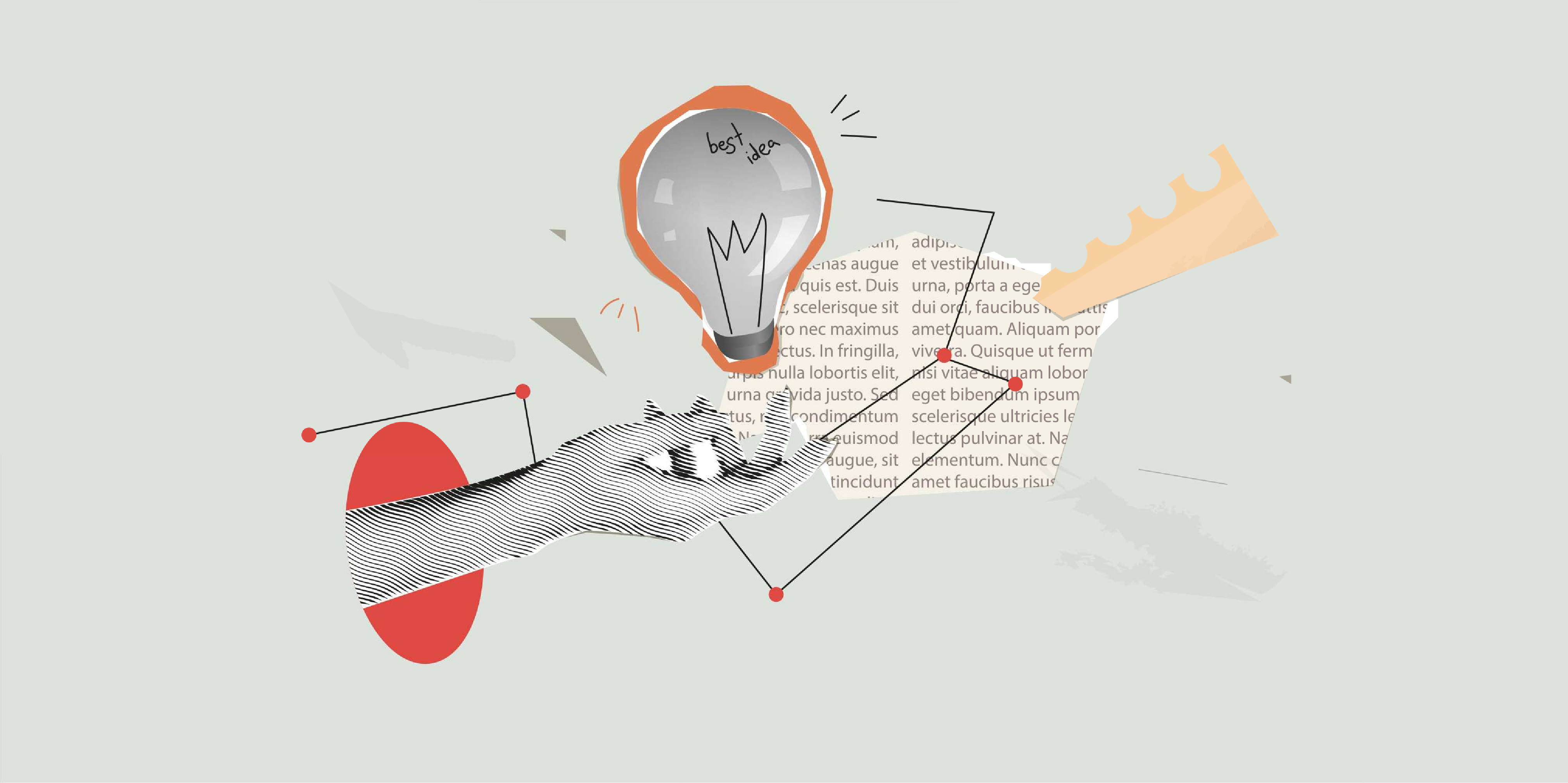
You may hear the terms ‘systematic review’ and ‘meta-analysis being used interchangeably’. Although they are related, they are distinctly different. Learn more in this blog for beginners.
What is a systematic review?
According to Cochrane (1), a systematic review attempts to identify, appraise and synthesize all the empirical evidence to answer a specific research question. Thus, a systematic review is where you might find the most relevant, adequate, and current information regarding a specific topic. In the levels of evidence pyramid , systematic reviews are only surpassed by meta-analyses.
To conduct a systematic review, you will need, among other things:
- A specific research question, usually in the form of a PICO question.
- Pre-specified eligibility criteria, to decide which articles will be included or discarded from the review.
- To follow a systematic method that will minimize bias.
You can find protocols that will guide you from both Cochrane and the Equator Network , among other places, and if you are a beginner to the topic then have a read of an overview about systematic reviews.
What is a meta-analysis?
A meta-analysis is a quantitative, epidemiological study design used to systematically assess the results of previous research (2) . Usually, they are based on randomized controlled trials, though not always. This means that a meta-analysis is a mathematical tool that allows researchers to mathematically combine outcomes from multiple studies.
When can a meta-analysis be implemented?
There is always the possibility of conducting a meta-analysis, yet, for it to throw the best possible results it should be performed when the studies included in the systematic review are of good quality, similar designs, and have similar outcome measures.
Why are meta-analyses important?
Outcomes from a meta-analysis may provide more precise information regarding the estimate of the effect of what is being studied because it merges outcomes from multiple studies. In a meta-analysis, data from various trials are combined and generate an average result (1), which is portrayed in a forest plot diagram. Moreover, meta-analysis also include a funnel plot diagram to visually detect publication bias.
Conclusions
A systematic review is an article that synthesizes available evidence on a certain topic utilizing a specific research question, pre-specified eligibility criteria for including articles, and a systematic method for its production. Whereas a meta-analysis is a quantitative, epidemiological study design used to assess the results of articles included in a systematic-review.
DEFINITION | Synthesis of empirical evidence regarding a specific research question | Statistical tool used with quantitative outcomes of various studies regarding a specific topic |
RESULTS | Synthesizes relevant and current information regarding a specific research question (qualitative). | Merges multiple outcomes from different researches and provides an average result (quantitative). |
Remember: All meta-analyses involve a systematic review, but not all systematic reviews involve a meta-analysis.
If you would like some further reading on this topic, we suggest the following:
The systematic review – a S4BE blog article
Meta-analysis: what, why, and how – a S4BE blog article
The difference between a systematic review and a meta-analysis – a blog article via Covidence
Systematic review vs meta-analysis: what’s the difference? A 5-minute video from Research Masterminds:
- About Cochrane reviews [Internet]. Cochranelibrary.com. [cited 2023 Apr 30]. Available from: https://www.cochranelibrary.com/about/about-cochrane-reviews
- Haidich AB. Meta-analysis in medical research. Hippokratia. 2010;14(Suppl 1):29–37.
Verónica Tanco Tellechea
Leave a reply cancel reply.
Your email address will not be published. Required fields are marked *
Save my name, email, and website in this browser for the next time I comment.
Subscribe to our newsletter
You will receive our monthly newsletter and free access to Trip Premium.
Related Articles
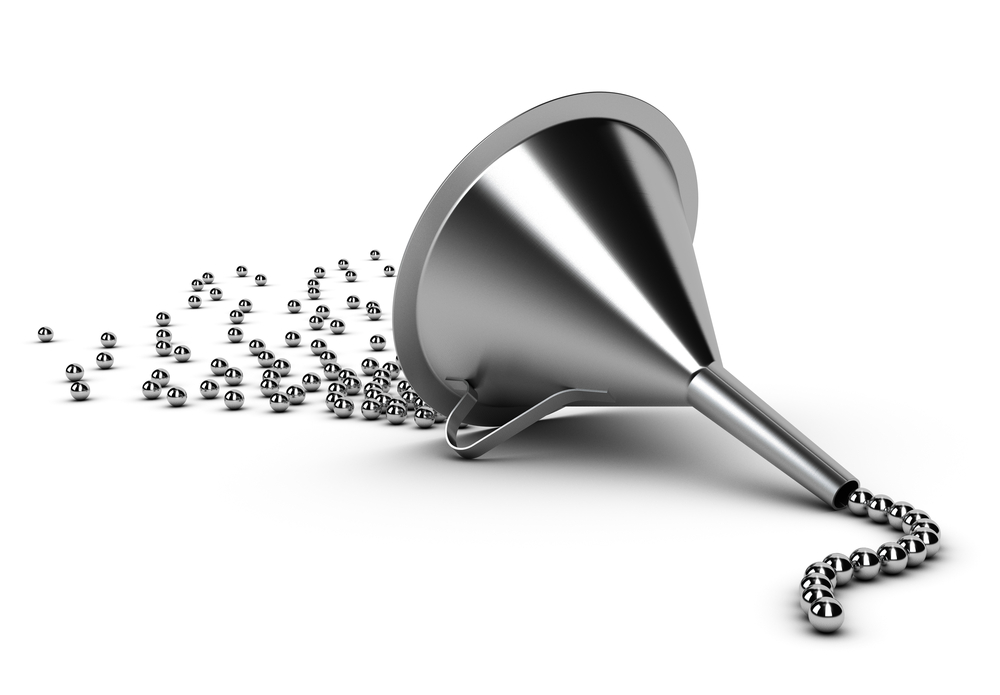
How to read a funnel plot
This blog introduces you to funnel plots, guiding you through how to read them and what may cause them to look asymmetrical.
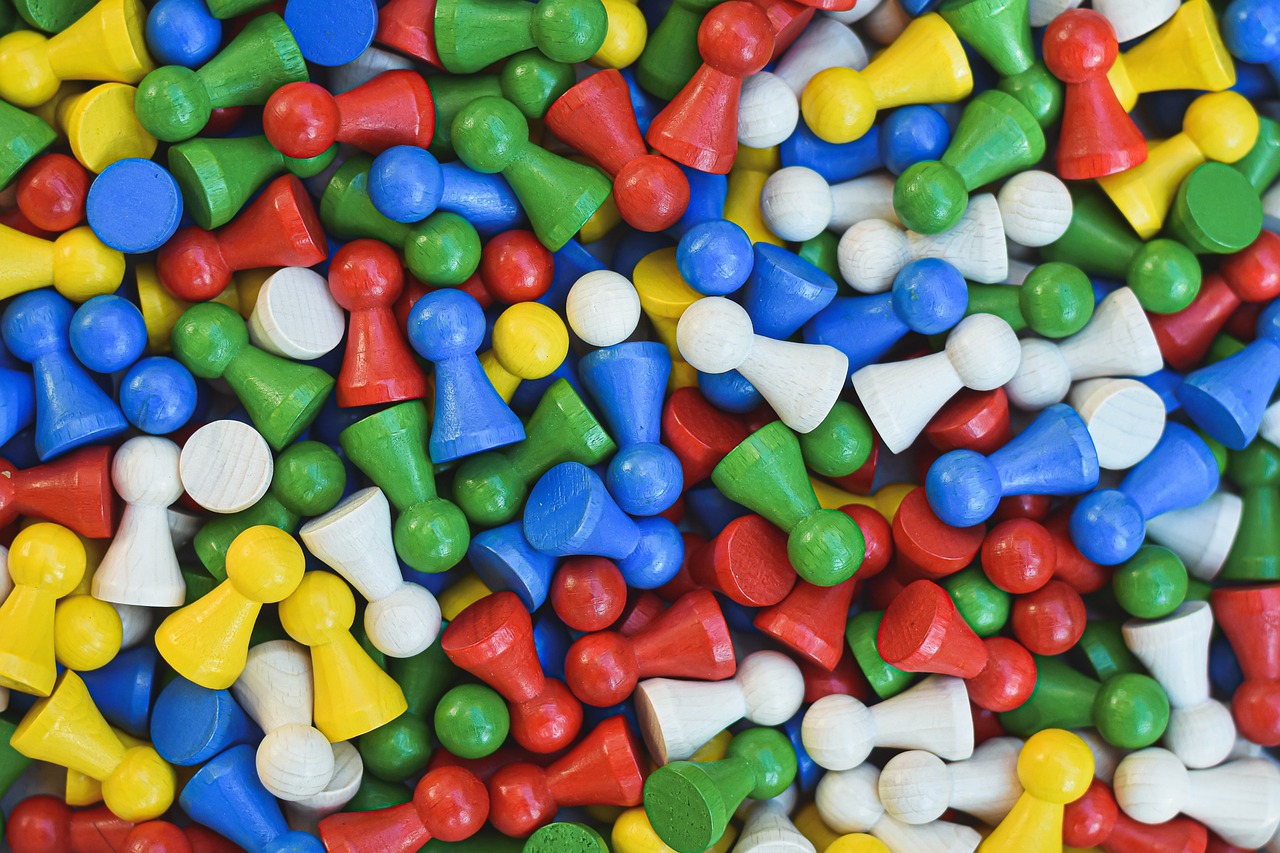
Heterogeneity in meta-analysis
When you bring studies together in a meta-analysis, one of the things you need to consider is the variability in your studies – this is called heterogeneity. This blog presents the three types of heterogeneity, considers the different types of outcome data, and delves a little more into dealing with the variations.
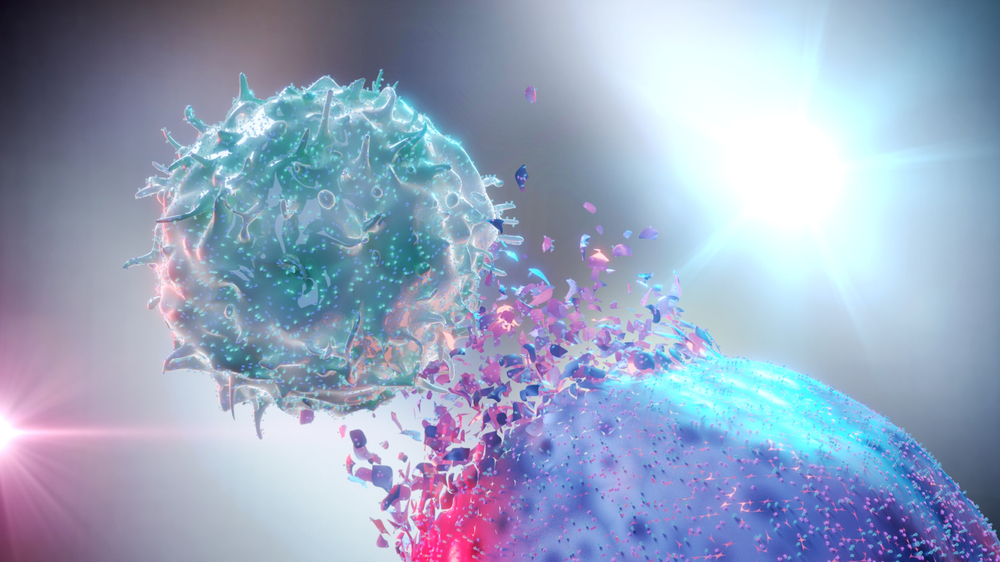
Natural killer cells in glioblastoma therapy
As seen in a previous blog from Davide, modern neuroscience often interfaces with other medical specialities. In this blog, he provides a summary of new evidence about the potential of a therapeutic strategy born at the crossroad between neurology, immunology and oncology.

Introduction to Meta-Analysis: A Guide for the Novice
- Experimental Psychology
- Methodology
- Statistical Analysis
Free Meta-Analysis Software and Macros
MetaXL (Version 2.0)
RevMan (Version 5.3)
Meta-Analysis Macros for SAS, SPSS, and Stata
Opposing theories and disparate findings populate the field of psychology; scientists must interpret the results of any single study in the context of its limitations. Meta-analysis is a robust tool that can help researchers overcome these challenges by assimilating data across studies identified through a literature review. In other words, rather than surveying participants, a meta-analysis surveys studies. The goal is to calculate the direction and/or magnitude of an effect across all relevant studies, both published and unpublished. Despite the utility of this statistical technique, it can intimidate a beginner who has no formal training in the approach. However, any motivated researcher with a statistics background can complete a meta-analysis. This article provides an overview of the main steps of basic meta-analysis.
Meta-analysis has many strengths. First, meta-analysis provides an organized approach for handling a large number of studies. Second, the process is systematic and documented in great detail, which allows readers to evaluate the researchers’ decisions and conclusions. Third, meta-analysis allows researchers to examine an effect within a collection of studies in a more sophisticated manner than a qualitative summary.
However, meta-analysis also involves numerous challenges. First, it consumes a great deal of time and requires a great deal of effort. Second, meta-analysis has been criticized for aggregating studies that are too different (i.e., mixing “apples and oranges”). Third, some scientists argue that the objective coding procedure used in meta-analysis ignores the context of each individual study, such as its methodological rigor. Fourth, when a researcher includes low-quality studies in a meta-analysis, the limitations of these studies impact the mean effect size (i.e., “garbage in, garbage out”). As long as researchers are aware of these issues and consider the potential influence of these limitations on their findings, meta-analysis can serve as a powerful and informative approach to help us draw conclusions from a large literature base.
Identifying the Right Question
Similar to any research study, a meta-analysis begins with a research question. Meta-analysis can be used in any situation where the goal is to summarize quantitative findings from empirical studies. It can be used to examine different types of effects, including prevalence rates (e.g., percentage of rape survivors with depression), growth rates (e.g., changes in depression from pretreatment to posttreatment), group differences (e.g., comparison of treatment and placebo groups on depression), and associations between variables (e.g., correlation between depression and self-esteem). To select the effect metric, researchers should consider the statistical form of the results in the literature. Any given meta-analysis can focus on only one metric at a time. While selecting a research question, researchers should think about the size of the literature base and select a manageable topic. At the same time, they should make sure the number of existing studies is large enough to warrant a meta-analysis.
Determining Eligibility Criteria
After choosing a relevant question, researchers should then identify and explicitly state the types of studies to be included. These criteria ensure that the studies overlap enough in topic and methodology that it makes sense to combine them. The inclusion and exclusion criteria depend on the specific research question and characteristics of the literature. First, researchers can specify relevant participant characteristics, such as age or gender. Second, researchers can identify the key variables that must be included in the study. Third, the language, date range, and types (e.g., peer-reviewed journal articles) of studies should be specified. Fourth, pertinent study characteristics, such as experimental design, can be defined. Eligibility criteria should be clearly documented and relevant to the research question. Specifying the eligibility criteria prior to conducting the literature search allows the researcher to perform a more targeted search and reduces the number of irrelevant studies. Eligibility criteria can also be revised later, because the researcher may become aware of unforeseen issues during the literature search stage.
Conducting a Literature Search and Review
The next step is to identify, retrieve, and review published and unpublished studies. The goal is to be exhaustive; however, being too broad can result in an overwhelming number of studies to review.
Online databases, such as PsycINFO and PubMed, compile millions of searchable records, including peer-reviewed journals, books, and dissertations. In addition, through these electronic databases, researchers can access the full text of many of the records. It is important that researchers carefully choose search terms and databases, because these decisions impact the breadth of the review. Researchers who aren’t familiar with the research topic should consult with an expert.
Additional ways to identify studies include searching conference proceedings, examining reference lists of relevant studies, and directly contacting researchers. After the literature search is completed, researchers must evaluate each study for inclusion using the eligibility criteria. At least a subset of the studies should be reviewed by two individuals (i.e., double coded) to serve as a reliability check. It is vital that researchers keep meticulous records of this process; for publication, a flow diagram is typically required to depict the search and results. Researchers should allow adequate time, because this step can be quite time consuming.
Calculating Effect Size
Next, researchers calculate an effect size for each eligible study. The effect size is the key component of a meta-analysis because it encodes the results in a numeric value that can then be aggregated. Examples of commonly used effect size metrics include Cohen’s d (i.e., group differences) and Pearson’s r (i.e., association between two variables). The effect size metric is based on the statistical form of the results in the literature and the research question. Because studies that include more participants provide more accurate estimates of an effect than those that include fewer participants, it is important to also calculate the precision of the effect size (e.g., standard error).
Meta-analysis software guides researchers through the calculation process by requesting the necessary information for the specified effect size metric. I have identified some potentially useful resources and programs below. Although meta-analysis software makes effect size calculations simple, it is good practice for researchers to understand what computations are being used.
The effect size and precision of each individual study are aggregated into a summary statistic, which can be done with meta-analysis software. Researchers should confirm that the effect sizes are independent of each other (i.e., no overlap in participants). Additionally, researchers must select either a fixed effects model (i.e., assumes all studies share one true effect size) or a random effects model (i.e., assumes the true effect size varies among studies). The random effects model is typically preferred when the studies have been conducted using different methodologies. Depending on the software, additional specifications or adjustments may be possible.
During analysis, the effect sizes of the included studies are weighted by their precision (e.g., inverse of the sampling error variance) and the mean is calculated. The mean effect size represents the direction and/or magnitude of the effect summarized across all eligible studies. This statistic is typically accompanied by an estimate of its precision (e.g., confidence interval) and p -value representing statistical significance. Forest plots are a common way of displaying meta-analysis results.
Depending on the situation, follow-up analyses may be advised. Researchers can quantify heterogeneity (e.g., Q, t 2 , I 2 ), which is a measure of the variation among the effect sizes of included studies. Moderator variables, such as the quality of the studies or age of participants, may be included to examine sources of heterogeneity. Because published studies may be biased towards significant effects, it is important to evaluate the impact of publication bias (e.g., funnel plot, Rosenthal’s Fail-safe N ). Sensitivity analysis can indicate how the results of the meta-analysis would change if one study were excluded from the analysis.
If properly conducted and clearly documented, meta-analyses often make significant contributions to a specific field of study and therefore stand a good chance of being published in a top-tier journal. The biggest obstacle for most researchers who attempt meta-analysis for the first time is the amount of work and organization required for proper execution, rather than their level of statistical knowledge.
Recommended Resources
Borenstein, M., Hedges, L. V., Higgins, J. P., & Rothstein, H. R. (2009). Introduction to meta-analysis . Hoboken, NJ: Wiley.
Cooper, H., Hedges, L., & Valentine, J. (2009). The handbook of research synthesis and meta-analysis (2nd ed.). New York, NY: Russell Sage Foundation.
Lipsey, M. W., & Wilson, D. B. (2001). Practical meta-analysis . Thousand Oaks, California: Sage Publications.
Rothstein, H. R., Sutton, A. J., & Borenstein, M. (2005). Publication bias in meta-analysis: Prevention, assessment, and adjustments . Hoboken, NJ: Wiley.
It is nice to see the software we developed (MetaXL) being mentioned. However, the reason we developed the software and made publicly available for free is that we disagree with an important statement in the review. This statement is “researchers must select either a fixed effects model (i.e., assumes all studies share one true effect size) or a random effects model (i.e., assumes the true effect size varies among studies)”. We developed MetaXL because we think that the random effects model is seriously flawed and should be abandoned. We implemented in MetaXL two additional models, the Inverse Variance heterogeneity model and the Quality Effects model, both meant to be used in case of heterogeneity. More details are in the User Guide, available from the Epigear website.
Thank you very much! The article really helped me to start understanding what meta-analysis is about
thank you for sharing this article; it is very helpful.But I am still confused about how to remove quickly duplicates papers without wasting time if we more than 10 000 papers?
Not being one to blow my own horn all the time, but I would like to suggest that you may want to take a look at a web based application I wrote that conducts a Hunter-Schmidt type meta-analysis. The Meta-Analysis is very easy to use and corrects for sampling and error variance due to reliability. It also exports the results in excel format. You can also export the dataset effect sizes (r, d, and z), sample sizes and reliability information in excel as well.
http://www.lyonsmorris.com/lyons/MaCalc/index.cfm
APS regularly opens certain online articles for discussion on our website. Effective February 2021, you must be a logged-in APS member to post comments. By posting a comment, you agree to our Community Guidelines and the display of your profile information, including your name and affiliation. Any opinions, findings, conclusions, or recommendations present in article comments are those of the writers and do not necessarily reflect the views of APS or the article’s author. For more information, please see our Community Guidelines .
Please login with your APS account to comment.
About the Author
Laura C. Wilson is an Assistant Professor in the Psychology Department at the University of Mary Washington. She earned a PhD in Clinical Psychology from Virginia Tech and MA in General/Experimental Psychology from The College of William & Mary. Her main area of expertise is post-trauma functioning, particularly in survivors of sexual violence or mass trauma (e.g., terrorism, mass shootings, combat). She also has interest in predictors of violence and aggression, including psychophysiological and personality factors.

Careers Up Close: Joel Anderson on Gender and Sexual Prejudices, the Freedoms of Academic Research, and the Importance of Collaboration
Joel Anderson, a senior research fellow at both Australian Catholic University and La Trobe University, researches group processes, with a specific interest on prejudice, stigma, and stereotypes.

Experimental Methods Are Not Neutral Tools
Ana Sofia Morais and Ralph Hertwig explain how experimental psychologists have painted too negative a picture of human rationality, and how their pessimism is rooted in a seemingly mundane detail: methodological choices.
APS Fellows Elected to SEP
In addition, an APS Rising Star receives the society’s Early Investigator Award.
Privacy Overview
Cookie | Duration | Description |
---|---|---|
__cf_bm | 30 minutes | This cookie, set by Cloudflare, is used to support Cloudflare Bot Management. |
Cookie | Duration | Description |
---|---|---|
AWSELBCORS | 5 minutes | This cookie is used by Elastic Load Balancing from Amazon Web Services to effectively balance load on the servers. |
Cookie | Duration | Description |
---|---|---|
at-rand | never | AddThis sets this cookie to track page visits, sources of traffic and share counts. |
CONSENT | 2 years | YouTube sets this cookie via embedded youtube-videos and registers anonymous statistical data. |
uvc | 1 year 27 days | Set by addthis.com to determine the usage of addthis.com service. |
_ga | 2 years | The _ga cookie, installed by Google Analytics, calculates visitor, session and campaign data and also keeps track of site usage for the site's analytics report. The cookie stores information anonymously and assigns a randomly generated number to recognize unique visitors. |
_gat_gtag_UA_3507334_1 | 1 minute | Set by Google to distinguish users. |
_gid | 1 day | Installed by Google Analytics, _gid cookie stores information on how visitors use a website, while also creating an analytics report of the website's performance. Some of the data that are collected include the number of visitors, their source, and the pages they visit anonymously. |
Cookie | Duration | Description |
---|---|---|
loc | 1 year 27 days | AddThis sets this geolocation cookie to help understand the location of users who share the information. |
VISITOR_INFO1_LIVE | 5 months 27 days | A cookie set by YouTube to measure bandwidth that determines whether the user gets the new or old player interface. |
YSC | session | YSC cookie is set by Youtube and is used to track the views of embedded videos on Youtube pages. |
yt-remote-connected-devices | never | YouTube sets this cookie to store the video preferences of the user using embedded YouTube video. |
yt-remote-device-id | never | YouTube sets this cookie to store the video preferences of the user using embedded YouTube video. |
yt.innertube::nextId | never | This cookie, set by YouTube, registers a unique ID to store data on what videos from YouTube the user has seen. |
yt.innertube::requests | never | This cookie, set by YouTube, registers a unique ID to store data on what videos from YouTube the user has seen. |
University of Houston Libraries
- Literature Reviews in the Health Sciences
- Review Comparison Chart
- Decision Tools
- Systematic Review
- Meta-Analysis
- Scoping Review
- Mapping Review
- Integrative Review
- Rapid Review
- Realist Review
- Umbrella Review
- Review of Complex Interventions
- Diagnostic Test Accuracy Review
- Narrative Literature Reviews
- Standards and Guidelines
Navigate the links below to jump to a specific section of the page:
When is conducting a Meta-Analysis appropriate?
Methods and guidance, examples of meta-analyses, supplementary resources.
A meta-analysis is defined by Haidlich (2010) as "quantitative, formal, epidemiological study design used to systematically assess previous research studies to derive conclusions about that body of research. Outcomes from a meta-analysis may include a more precise estimate of the effect of treatment or risk factor for disease, or other outcomes , than any individual study contributing to the pooled analysis" (p.29).
According to Grant & Booth (2009) , a meta-analysis is defined as a "technique that statistically combines the results of quantitative studies to provide a more precise effect of the results" (p.94).
Characteristics
- A meta-analysis can only be conducted after the completion of a systematic review , as the meta-analysis statistically summarizes the findings from the studies synthesized in a particular systematic review. A meta-analysis cannot exist with a pre-existing systematic review . Grant & Booth (2009) state that "although many systematic reviews present their results without statistically combining data [in a meta-analysis], a good systematic review is essential to a meta-analysis of the literature" (p.98).
- Conducting a meta-analysis requires all studies that will be statistically summarized to be similar - i.e. that population, intervention, and comparison. Grant & Booth (2009) state that "more importantly, it requires that the same measure or outcome be measured in the same way at the same time intervals" (p.98).
When to Use It: According to the Cochrane Handbook , "an important step in a systematic review is the thoughtful consideration of whether it is appropriate to combine the numerical results of all, or perhaps some, of the studies. Such a meta-analysis yields an overall statistic (together with its confidence interval) that summarizes the effectiveness of an experimental intervention compared with a comparator intervention" (section 10.2).
Conducting meta-analyses can have the following benefits, according to Deeks et al. (2021, section 10.2) :
- To improve precision. Many studies are too small to provide convincing evidence about intervention effects in isolation. Estimation is usually improved when it is based on more information.
- To answer questions not posed by the individual studies. Primary studies often involve a specific type of participant and explicitly defined interventions. A selection of studies in which these characteristics differ can allow investigation of the consistency of effect across a wider range of populations and interventions. It may also, if relevant, allow reasons for differences in effect estimates to be investigated.
- To settle controversies arising from apparently conflicting studies or to generate new hypotheses. Statistical synthesis of findings allows the degree of conflict to be formally assessed, and reasons for different results to be explored and quantified.
The following resource provides further support on conducting a meta-analysis.
Methods & Guidance
- Cochrane Handbook for Systematic Reviews of Interventions. Chapter 10: Analysing data and undertaking meta-analyses
A comprehensive overview on meta-analyses within the Cochrane Handbook.
Reporting Guideline
- PRISMA 2020 checklist
PRISMA (2020) is a 27-item checklist that replaces the PRISMA (2009) statement , which ensures proper and transparent reporting for each element in a systematic review and meta-analysis. "It is an expanded checklist that details reporting recommendations for each item, the PRISMA 2020 abstract checklist, and the revised flow diagrams for original and updated reviews."
- Marioni, R. E., Suderman, M., Chen, B. H., Horvath, S., Bandinelli, S., Morris, T., Beck, S., Ferrucci, L., Pedersen, N. L., Relton, C. L., Deary, I. J., & Hägg, S. (2019). Tracking the epigenetic clock across the human life course: a meta-analysis of longitudinal cohort data . The journals of gerontology: Series A, Biological sciences and medical sciences , 74 (1), 57–61. doi: 10.1093/gerona/gly060
Deeks, J.J., Higgins, J.P.T., & Altman, D.G. (Eds.). (2021). Chapter 10: Analysing data and undertaking meta-analyses . In Higgins, J.P.T., Thomas J., Chandler, J., Cumpston, M., Li, T., Page, M.J., & Welch, V.A. (Eds.), Cochrane Handbook for Systematic Reviews of Interventions version 6.2. Cochrane. Available from www.training.cochrane.org/handbook
Grant, M. J., & Booth, A. (2009). A typology of reviews: an analysis of 14 review types and associated methodologies . Health information and libraries journal , 26 (2), 91–108. doi: 10.1111/j.1471-1842.2009.00848.x
Haidich A. B. (2010). Meta-analysis in medical research . Hippokratia , 14 (Suppl 1), 29–37.
Seidler, A.L., Hunter, K.E., Cheyne, S., Ghersi, D., Berlin, J.A., & Askie, L. (2019). A guide to prospective meta-analysis . BMJ , 367 , l5342. doi: 10.1136/bmj.l5342
- << Previous: Systematic Review
- Next: Scoping Review >>
Limitations of a Meta-Analysis
The following challenges of conducting meta-analyses in systematic reviews are derived from Grant & Booth (2009) , Haidlich (2010) , and Deeks et al. (2021) .
- Can be challenging to ensure that studies used in a meta-analysis are similar enough, which is a crucial component
- Meta-analyses can perhaps be misleading due to biases such as those concerning specific study designs, reporting, and biases within studies
Medical Librarian

- Last Updated: Sep 5, 2023 11:14 AM
- URL: https://guides.lib.uh.edu/reviews
Main Navigation Menu
Systematic reviews.
- Getting Started with Systematic Reviews
What is a Systematic Review and Meta-Analysis
Differences between systematic and literature reviews.
- Finding and Evaluating Existing Systematic Reviews
- Steps in a Systematic Review
- Step 1: Developing a Question
- Step 2: Selecting Databases
- Step 3: Grey Literature
- Step 4: Registering a Systematic Review Protocol
- Step 5: Translate Search Strategies
- Step 6: Citation Management Tools
- Step 7: Article Screening
- Other Resources
- Interlibrary Loan (ILL)
A systematic review collects and analyzes all evidence that answers a specific research question. In a systematic review, a question needs to be clearly defined and have inclusion and exclusion criteria. In general, specific and systematic methods selected are intended to minimize bias. This is followed by an extensive search of the literature and a critical analysis of the search results. The reason why a systematic review is conducted is to provide a current evidence-based answer to a specific question that in turn helps to inform decision making. Check out the Centers for Disease Control and Prevention and Cochrane Reviews links to learn more about Systematic Reviews.
A systematic review can be combined with a meta-analysis. A meta-analysis is the use of statistical methods to summarize the results of a systematic review. Not every systematic review contains a meta-analysis. A meta-analysis may not be appropriate if the designs of the studies are too different, if there are concerns about the quality of studies, if the outcomes measured are not sufficiently similar for the result across the studies to be meaningful.
Centers for Disease Control and Prevention. (n.d.). Systematic Reviews . Retrieved from https://www.cdc.gov/library/researchguides/sytemsaticreviews.html
Cochrane Library. (n.d.). About Cochrane Reviews . Retrieved from https://www.cochranelibrary.com/about/about-cochrane-reviews

Source: Kysh, Lynn (2013): Difference between a systematic review and a literature review. [figshare]. Available at: https://figshare.com/articles/Difference_between_a_systematic_review_and_a_literature_review/766364
- << Previous: Getting Started with Systematic Reviews
- Next: Finding and Evaluating Existing Systematic Reviews >>
- Last Updated: May 7, 2024 1:42 PM
- URL: https://guides.library.ucmo.edu/systematicreviews
Have a language expert improve your writing
Run a free plagiarism check in 10 minutes, generate accurate citations for free.
- Knowledge Base
Methodology
- Systematic Review | Definition, Example, & Guide
Systematic Review | Definition, Example & Guide
Published on June 15, 2022 by Shaun Turney . Revised on November 20, 2023.
A systematic review is a type of review that uses repeatable methods to find, select, and synthesize all available evidence. It answers a clearly formulated research question and explicitly states the methods used to arrive at the answer.
They answered the question “What is the effectiveness of probiotics in reducing eczema symptoms and improving quality of life in patients with eczema?”
In this context, a probiotic is a health product that contains live microorganisms and is taken by mouth. Eczema is a common skin condition that causes red, itchy skin.
Table of contents
What is a systematic review, systematic review vs. meta-analysis, systematic review vs. literature review, systematic review vs. scoping review, when to conduct a systematic review, pros and cons of systematic reviews, step-by-step example of a systematic review, other interesting articles, frequently asked questions about systematic reviews.
A review is an overview of the research that’s already been completed on a topic.
What makes a systematic review different from other types of reviews is that the research methods are designed to reduce bias . The methods are repeatable, and the approach is formal and systematic:
- Formulate a research question
- Develop a protocol
- Search for all relevant studies
- Apply the selection criteria
- Extract the data
- Synthesize the data
- Write and publish a report
Although multiple sets of guidelines exist, the Cochrane Handbook for Systematic Reviews is among the most widely used. It provides detailed guidelines on how to complete each step of the systematic review process.
Systematic reviews are most commonly used in medical and public health research, but they can also be found in other disciplines.
Systematic reviews typically answer their research question by synthesizing all available evidence and evaluating the quality of the evidence. Synthesizing means bringing together different information to tell a single, cohesive story. The synthesis can be narrative ( qualitative ), quantitative , or both.
Prevent plagiarism. Run a free check.
Systematic reviews often quantitatively synthesize the evidence using a meta-analysis . A meta-analysis is a statistical analysis, not a type of review.
A meta-analysis is a technique to synthesize results from multiple studies. It’s a statistical analysis that combines the results of two or more studies, usually to estimate an effect size .
A literature review is a type of review that uses a less systematic and formal approach than a systematic review. Typically, an expert in a topic will qualitatively summarize and evaluate previous work, without using a formal, explicit method.
Although literature reviews are often less time-consuming and can be insightful or helpful, they have a higher risk of bias and are less transparent than systematic reviews.
Similar to a systematic review, a scoping review is a type of review that tries to minimize bias by using transparent and repeatable methods.
However, a scoping review isn’t a type of systematic review. The most important difference is the goal: rather than answering a specific question, a scoping review explores a topic. The researcher tries to identify the main concepts, theories, and evidence, as well as gaps in the current research.
Sometimes scoping reviews are an exploratory preparation step for a systematic review, and sometimes they are a standalone project.
A systematic review is a good choice of review if you want to answer a question about the effectiveness of an intervention , such as a medical treatment.
To conduct a systematic review, you’ll need the following:
- A precise question , usually about the effectiveness of an intervention. The question needs to be about a topic that’s previously been studied by multiple researchers. If there’s no previous research, there’s nothing to review.
- If you’re doing a systematic review on your own (e.g., for a research paper or thesis ), you should take appropriate measures to ensure the validity and reliability of your research.
- Access to databases and journal archives. Often, your educational institution provides you with access.
- Time. A professional systematic review is a time-consuming process: it will take the lead author about six months of full-time work. If you’re a student, you should narrow the scope of your systematic review and stick to a tight schedule.
- Bibliographic, word-processing, spreadsheet, and statistical software . For example, you could use EndNote, Microsoft Word, Excel, and SPSS.
A systematic review has many pros .
- They minimize research bias by considering all available evidence and evaluating each study for bias.
- Their methods are transparent , so they can be scrutinized by others.
- They’re thorough : they summarize all available evidence.
- They can be replicated and updated by others.
Systematic reviews also have a few cons .
- They’re time-consuming .
- They’re narrow in scope : they only answer the precise research question.
The 7 steps for conducting a systematic review are explained with an example.
Step 1: Formulate a research question
Formulating the research question is probably the most important step of a systematic review. A clear research question will:
- Allow you to more effectively communicate your research to other researchers and practitioners
- Guide your decisions as you plan and conduct your systematic review
A good research question for a systematic review has four components, which you can remember with the acronym PICO :
- Population(s) or problem(s)
- Intervention(s)
- Comparison(s)
You can rearrange these four components to write your research question:
- What is the effectiveness of I versus C for O in P ?
Sometimes, you may want to include a fifth component, the type of study design . In this case, the acronym is PICOT .
- Type of study design(s)
- The population of patients with eczema
- The intervention of probiotics
- In comparison to no treatment, placebo , or non-probiotic treatment
- The outcome of changes in participant-, parent-, and doctor-rated symptoms of eczema and quality of life
- Randomized control trials, a type of study design
Their research question was:
- What is the effectiveness of probiotics versus no treatment, a placebo, or a non-probiotic treatment for reducing eczema symptoms and improving quality of life in patients with eczema?
Step 2: Develop a protocol
A protocol is a document that contains your research plan for the systematic review. This is an important step because having a plan allows you to work more efficiently and reduces bias.
Your protocol should include the following components:
- Background information : Provide the context of the research question, including why it’s important.
- Research objective (s) : Rephrase your research question as an objective.
- Selection criteria: State how you’ll decide which studies to include or exclude from your review.
- Search strategy: Discuss your plan for finding studies.
- Analysis: Explain what information you’ll collect from the studies and how you’ll synthesize the data.
If you’re a professional seeking to publish your review, it’s a good idea to bring together an advisory committee . This is a group of about six people who have experience in the topic you’re researching. They can help you make decisions about your protocol.
It’s highly recommended to register your protocol. Registering your protocol means submitting it to a database such as PROSPERO or ClinicalTrials.gov .
Step 3: Search for all relevant studies
Searching for relevant studies is the most time-consuming step of a systematic review.
To reduce bias, it’s important to search for relevant studies very thoroughly. Your strategy will depend on your field and your research question, but sources generally fall into these four categories:
- Databases: Search multiple databases of peer-reviewed literature, such as PubMed or Scopus . Think carefully about how to phrase your search terms and include multiple synonyms of each word. Use Boolean operators if relevant.
- Handsearching: In addition to searching the primary sources using databases, you’ll also need to search manually. One strategy is to scan relevant journals or conference proceedings. Another strategy is to scan the reference lists of relevant studies.
- Gray literature: Gray literature includes documents produced by governments, universities, and other institutions that aren’t published by traditional publishers. Graduate student theses are an important type of gray literature, which you can search using the Networked Digital Library of Theses and Dissertations (NDLTD) . In medicine, clinical trial registries are another important type of gray literature.
- Experts: Contact experts in the field to ask if they have unpublished studies that should be included in your review.
At this stage of your review, you won’t read the articles yet. Simply save any potentially relevant citations using bibliographic software, such as Scribbr’s APA or MLA Generator .
- Databases: EMBASE, PsycINFO, AMED, LILACS, and ISI Web of Science
- Handsearch: Conference proceedings and reference lists of articles
- Gray literature: The Cochrane Library, the metaRegister of Controlled Trials, and the Ongoing Skin Trials Register
- Experts: Authors of unpublished registered trials, pharmaceutical companies, and manufacturers of probiotics
Step 4: Apply the selection criteria
Applying the selection criteria is a three-person job. Two of you will independently read the studies and decide which to include in your review based on the selection criteria you established in your protocol . The third person’s job is to break any ties.
To increase inter-rater reliability , ensure that everyone thoroughly understands the selection criteria before you begin.
If you’re writing a systematic review as a student for an assignment, you might not have a team. In this case, you’ll have to apply the selection criteria on your own; you can mention this as a limitation in your paper’s discussion.
You should apply the selection criteria in two phases:
- Based on the titles and abstracts : Decide whether each article potentially meets the selection criteria based on the information provided in the abstracts.
- Based on the full texts: Download the articles that weren’t excluded during the first phase. If an article isn’t available online or through your library, you may need to contact the authors to ask for a copy. Read the articles and decide which articles meet the selection criteria.
It’s very important to keep a meticulous record of why you included or excluded each article. When the selection process is complete, you can summarize what you did using a PRISMA flow diagram .
Next, Boyle and colleagues found the full texts for each of the remaining studies. Boyle and Tang read through the articles to decide if any more studies needed to be excluded based on the selection criteria.
When Boyle and Tang disagreed about whether a study should be excluded, they discussed it with Varigos until the three researchers came to an agreement.
Step 5: Extract the data
Extracting the data means collecting information from the selected studies in a systematic way. There are two types of information you need to collect from each study:
- Information about the study’s methods and results . The exact information will depend on your research question, but it might include the year, study design , sample size, context, research findings , and conclusions. If any data are missing, you’ll need to contact the study’s authors.
- Your judgment of the quality of the evidence, including risk of bias .
You should collect this information using forms. You can find sample forms in The Registry of Methods and Tools for Evidence-Informed Decision Making and the Grading of Recommendations, Assessment, Development and Evaluations Working Group .
Extracting the data is also a three-person job. Two people should do this step independently, and the third person will resolve any disagreements.
They also collected data about possible sources of bias, such as how the study participants were randomized into the control and treatment groups.
Step 6: Synthesize the data
Synthesizing the data means bringing together the information you collected into a single, cohesive story. There are two main approaches to synthesizing the data:
- Narrative ( qualitative ): Summarize the information in words. You’ll need to discuss the studies and assess their overall quality.
- Quantitative : Use statistical methods to summarize and compare data from different studies. The most common quantitative approach is a meta-analysis , which allows you to combine results from multiple studies into a summary result.
Generally, you should use both approaches together whenever possible. If you don’t have enough data, or the data from different studies aren’t comparable, then you can take just a narrative approach. However, you should justify why a quantitative approach wasn’t possible.
Boyle and colleagues also divided the studies into subgroups, such as studies about babies, children, and adults, and analyzed the effect sizes within each group.
Step 7: Write and publish a report
The purpose of writing a systematic review article is to share the answer to your research question and explain how you arrived at this answer.
Your article should include the following sections:
- Abstract : A summary of the review
- Introduction : Including the rationale and objectives
- Methods : Including the selection criteria, search method, data extraction method, and synthesis method
- Results : Including results of the search and selection process, study characteristics, risk of bias in the studies, and synthesis results
- Discussion : Including interpretation of the results and limitations of the review
- Conclusion : The answer to your research question and implications for practice, policy, or research
To verify that your report includes everything it needs, you can use the PRISMA checklist .
Once your report is written, you can publish it in a systematic review database, such as the Cochrane Database of Systematic Reviews , and/or in a peer-reviewed journal.
In their report, Boyle and colleagues concluded that probiotics cannot be recommended for reducing eczema symptoms or improving quality of life in patients with eczema. Note Generative AI tools like ChatGPT can be useful at various stages of the writing and research process and can help you to write your systematic review. However, we strongly advise against trying to pass AI-generated text off as your own work.
If you want to know more about statistics , methodology , or research bias , make sure to check out some of our other articles with explanations and examples.
- Student’s t -distribution
- Normal distribution
- Null and Alternative Hypotheses
- Chi square tests
- Confidence interval
- Quartiles & Quantiles
- Cluster sampling
- Stratified sampling
- Data cleansing
- Reproducibility vs Replicability
- Peer review
- Prospective cohort study
Research bias
- Implicit bias
- Cognitive bias
- Placebo effect
- Hawthorne effect
- Hindsight bias
- Affect heuristic
- Social desirability bias
A literature review is a survey of scholarly sources (such as books, journal articles, and theses) related to a specific topic or research question .
It is often written as part of a thesis, dissertation , or research paper , in order to situate your work in relation to existing knowledge.
A literature review is a survey of credible sources on a topic, often used in dissertations , theses, and research papers . Literature reviews give an overview of knowledge on a subject, helping you identify relevant theories and methods, as well as gaps in existing research. Literature reviews are set up similarly to other academic texts , with an introduction , a main body, and a conclusion .
An annotated bibliography is a list of source references that has a short description (called an annotation ) for each of the sources. It is often assigned as part of the research process for a paper .
A systematic review is secondary research because it uses existing research. You don’t collect new data yourself.
Cite this Scribbr article
If you want to cite this source, you can copy and paste the citation or click the “Cite this Scribbr article” button to automatically add the citation to our free Citation Generator.
Turney, S. (2023, November 20). Systematic Review | Definition, Example & Guide. Scribbr. Retrieved June 18, 2024, from https://www.scribbr.com/methodology/systematic-review/
Is this article helpful?
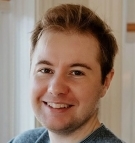
Shaun Turney
Other students also liked, how to write a literature review | guide, examples, & templates, how to write a research proposal | examples & templates, what is critical thinking | definition & examples, get unlimited documents corrected.
✔ Free APA citation check included ✔ Unlimited document corrections ✔ Specialized in correcting academic texts
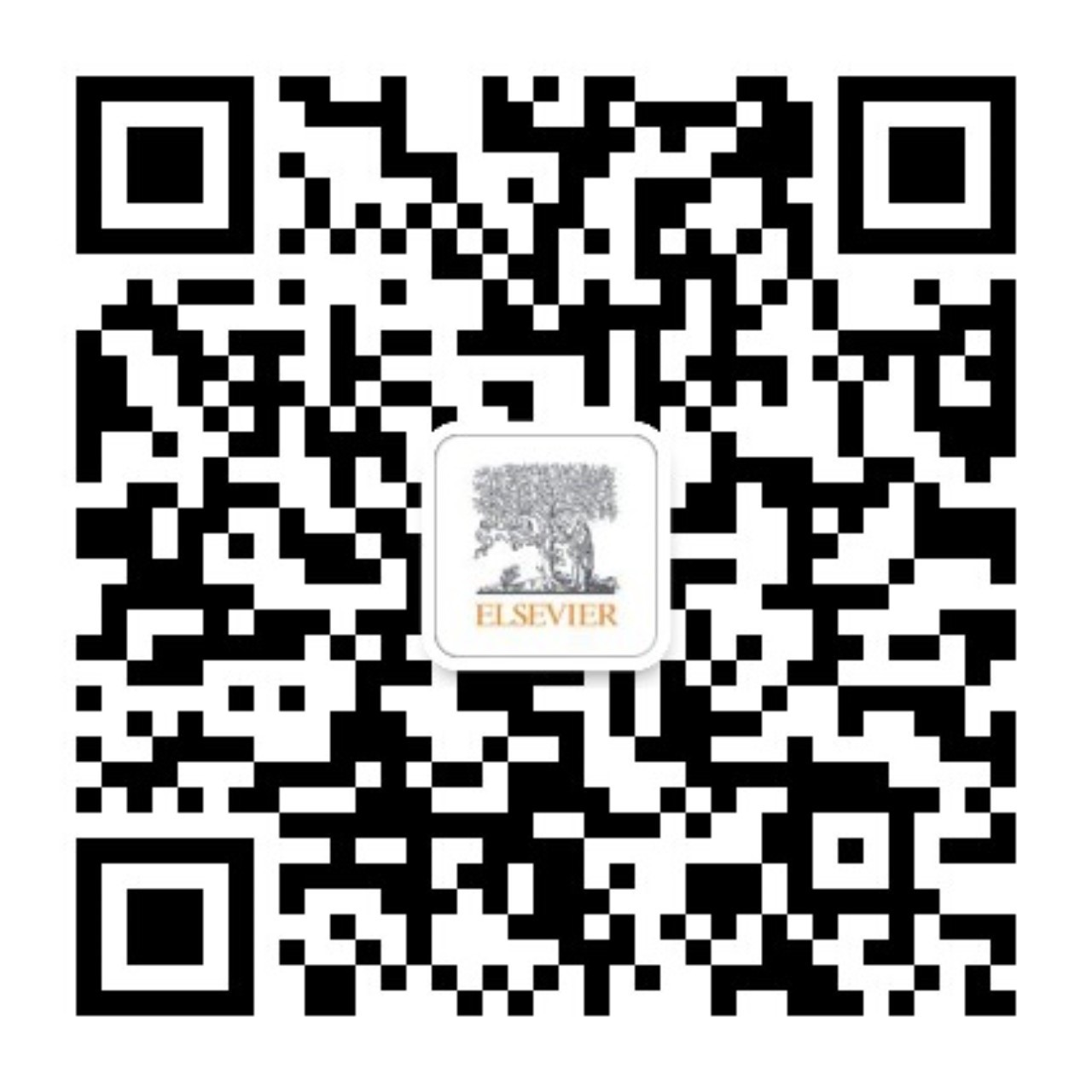
- Manuscript Review
Systematic Review VS Meta-Analysis
- 3 minute read
- 60.8K views
Table of Contents
How you organize your research is incredibly important; whether you’re preparing a report, research review, thesis or an article to be published. What methodology you choose can make or break your work getting out into the world, so let’s take a look at two main types: systematic review and meta-analysis.
Let’s start with what they have in common – essentially, they are both based on high-quality filtered evidence related to a specific research topic. They’re both highly regarded as generally resulting in reliable findings, though there are differences, which we’ll discuss below. Additionally, they both support conclusions based on expert reviews, case-controlled studies, data analysis, etc., versus mere opinions and musings.
What is a Systematic Review?
A systematic review is a form of research done collecting, appraising and synthesizing evidence to answer a particular question, in a very transparent and systematic way. Data (or evidence) used in systematic reviews have their origin in scholarly literature – published or unpublished. So, findings are typically very reliable. In addition, they are normally collated and appraised by an independent panel of experts in the field. Unlike traditional reviews, systematic reviews are very comprehensive and don’t rely on a single author’s point of view, thus avoiding bias.
Systematic reviews are especially important in the medical field, where health practitioners need to be constantly up-to-date with new, high-quality information to lead their daily decisions. Since systematic reviews, by definition, collect information from previous research, the pitfalls of new primary studies is avoided. They often, in fact, identify lack of evidence or knowledge limitations, and consequently recommend further study, if needed.
Why are systematic reviews important?
- They combine and synthesize various studies and their findings.
- Systematic reviews appraise the validity of the results and findings of the collected studies in an impartial way.
- They define clear objectives and reproducible methodologies.
What is a Meta-analysis?
This form of research relies on combining statistical results from two or more existing studies. When multiple studies are addressing the same problem or question, it’s to be expected that there will be some potential for error. Most studies account for this within their results. A meta-analysis can help iron out any inconsistencies in data, as long as the studies are similar.
For instance, if your research is about the influence of the Mediterranean diet on diabetic people, between the ages of 30 and 45, but you only find a study about the Mediterranean diet in healthy people and another about the Mediterranean diet in diabetic teenagers. In this case, undertaking a meta-analysis would probably be a poor choice. You can either pursue the idea of comparing such different material, at the risk of findings that don’t really answer the review question. Or, you can decide to explore a different research method (perhaps more qualitative).
Why is meta-analysis important?
- They help improve precision about evidence since many studies are too small to provide convincing data.
- Meta-analyses can settle divergences between conflicting studies. By formally assessing the conflicting study results, it is possible to eventually reach new hypotheses and explore the reasons for controversy.
- They can also answer questions with a broader influence than individual studies. For example, the effect of a disease on several populations across the world, by comparing other modest research studies completed in specific countries or continents.
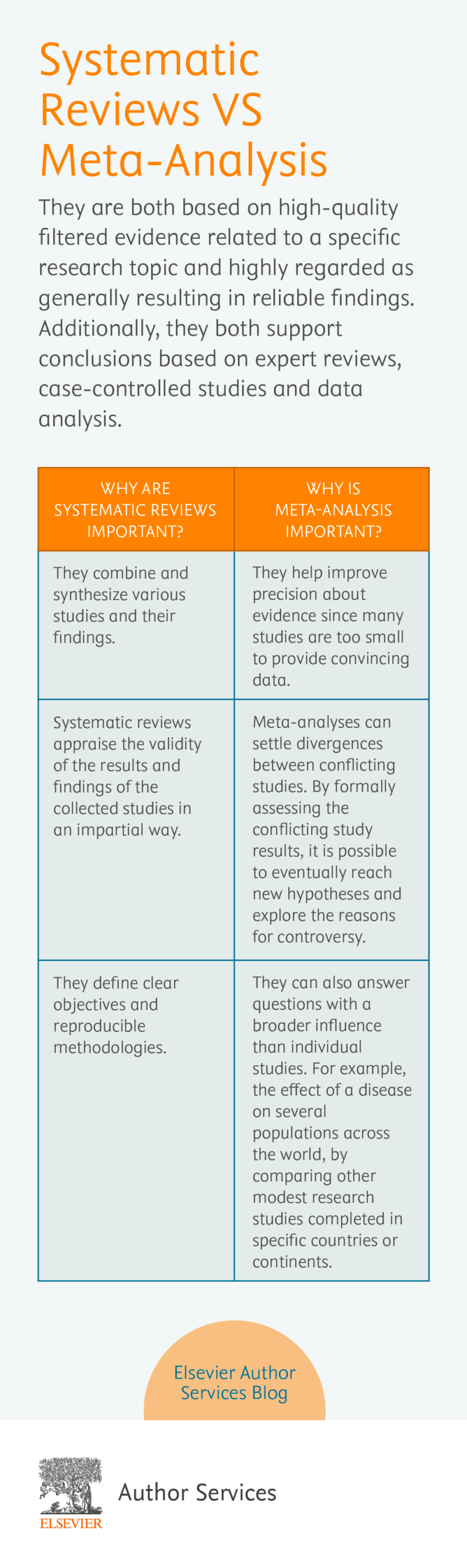
Undertaking research approaches, like systematic reviews and/or meta-analysis, involve great responsibility. They provide reliable information that has a real impact on society. Elsevier offers a number of services that aim to help researchers achieve excellence in written text, suggesting the necessary amendments to fit them into a targeted format. A perfectly written text, whether translated or edited from a manuscript, is the key to being respected within the scientific community, leading to more and more important positions like, let’s say…being part of an expert panel leading a systematic review or a widely acknowledged meta-analysis.
Check why it’s important to manage research data .
Language Editing Services by Elsevier Author Services:
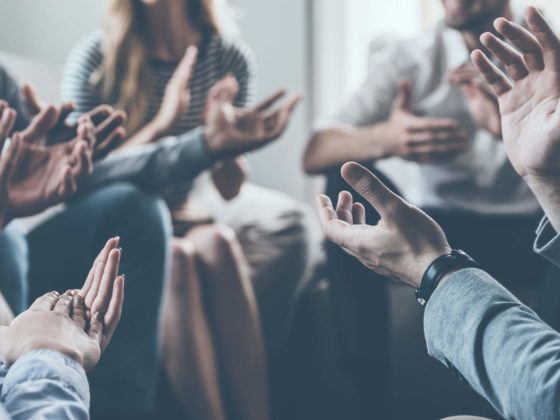
- Publication Recognition
What is a Good H-index?
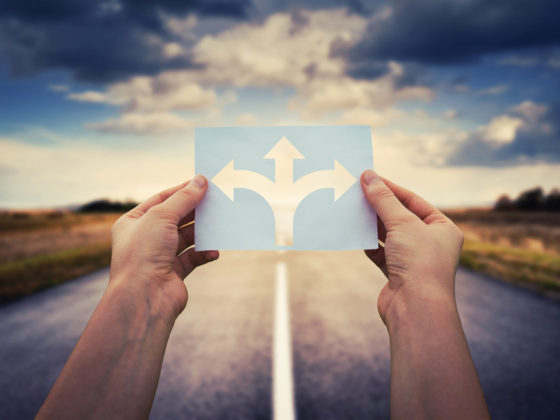
- Research Process
What is a Research Gap
You may also like.
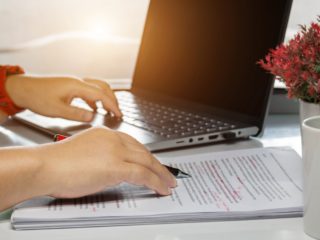
Essential for High-Quality Paper Editing: Three Tips to Efficient Spellchecks
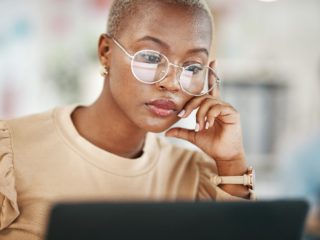
If You’re a Researcher, Remember These Before You Are Submitting Your Manuscript to Journals!
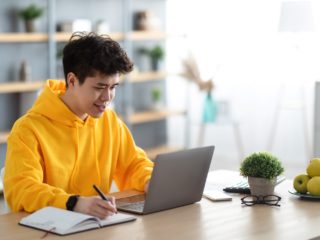
Navigating “Chinglish” Errors in Academic English Writing
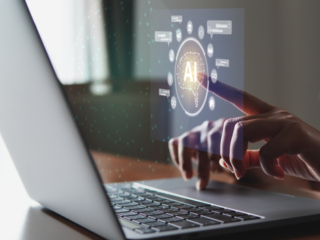
Is The Use of AI in Manuscript Editing Feasible? Here’s Three Tips to Steer Clear of Potential Issues
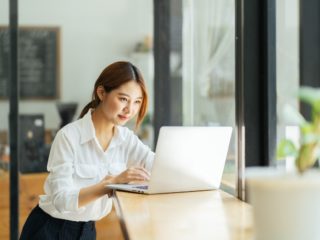
A profound editing experience with English-speaking experts: Elsevier Language Services to learn more!
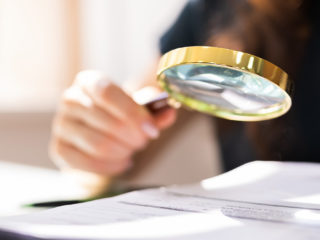
Research Fraud: Falsification and Fabrication in Research Data
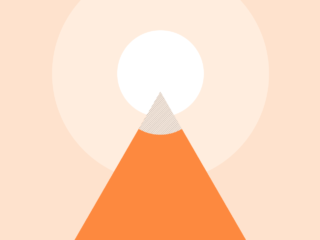
Professor Anselmo Paiva: Using Computer Vision to Tackle Medical Issues with a Little Help from Elsevier Author Services
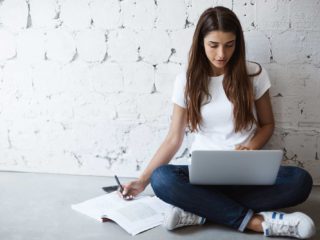
What is the main purpose of proofreading a paper?
Input your search keywords and press Enter.
What Is the Difference Between a Systematic Review and a Meta-analysis?
- First Online: 02 February 2019
Cite this chapter
- Shakib Akhter 8 , 9 ,
- Thierry Pauyo 10 &
- Moin Khan 8 , 9
4636 Accesses
18 Citations
Distinguishing between a systematic review and meta-analysis is essential to understand the role each plays in presenting and analysing data and estimates of treatment effects. Often, novice researchers mistakenly use these terms synonymously. A thorough understanding of the similarities and differences between these two research methodologies is needed to appropriately evaluate the quality of conclusions emerging from such studies. The systematic review allows the researcher to synthesize and critically appraise a number of studies in a specific context to provide evidence-based conclusions. Comparatively, atop the hierarchical chain of evidence lies the meta-analysis, in which a systematic review is performed and then statistical methods are employed to quantitatively pool the results of a selected number of studies in a specific context. This design is a robust method of combined analysis and is therefore deemed the highest level of evidence when pooling high-quality randomized controlled trials. Understanding and appreciating the methodological differences in these two designs are elemental in planning, implementing, and evaluating high-quality research.
This is a preview of subscription content, log in via an institution to check access.
Access this chapter
- Available as PDF
- Read on any device
- Instant download
- Own it forever
- Available as EPUB and PDF
- Durable hardcover edition
- Dispatched in 3 to 5 business days
- Free shipping worldwide - see info
Tax calculation will be finalised at checkout
Purchases are for personal use only
Institutional subscriptions
Similar content being viewed by others
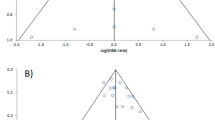
A guide to appropriately planning and conducting meta-analyses—Part 1: indications, assumptions and understanding risk of bias
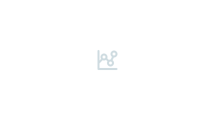
A guide to appropriately planning and conducting meta-analyses: part 2—effect size estimation, heterogeneity and analytic approaches
Quality Assessment in Meta-analysis
Aslam S, Emmanuel P. Formulating a researchable question: a critical step for facilitating good clinical research. Indian J Sex Transm Dis AIDS. 2010;31(1):47.
Article Google Scholar
Brighton B, Bhandari M, Tornetta P, Felson DT. Hierarchy of evidence: from case reports to randomized controlled trials. Clin Orthop Relat Res. 2003;413:19–24.
Burns PB, Rohrich RJ, Chung KC. The levels of evidence and their role in evidence-based medicine. Plast Reconstr Surg. 2011;128(1):305–10.
Article CAS Google Scholar
Egger M, Davey-Smith G, Altman D. Systematic reviews in health care: meta-analysis in context. Somerset: Wiley; 2013.
Google Scholar
GRADE Working Group. Grading quality of evidence and strength of recommendations. BMJ. 2004;328(7454):1490.
Green S, Higgins JP. Preparing a cochrane review. In: Cochrane handbook for systematic reviews of interventions; 2012. p. 11–30.
Guyatt GH, Sackett DL, Sinclair JC, Hayward R, Cook DJ, Cook RJ. Users’ guides to the medical literature. IX. A method for grading health care recommendations. Evidence-Based Medicine Working Group. JAMA. 1995;274(22):1800–4.
Higgins JPT, Thompson SG, Deeks JJ, Altman DG. Measuring inconsistency in meta-analyses. BMJ. 2003;327(7414):557–60.
Hopewell S, Mcdonald S, Clarke M, Egger M. Grey literature in meta-analyses of randomized trials of health care interventions. Cochrane Database Syst Rev. 2007;(2):MR000010.
Jinha AE. Article 50 million: an estimate of the number of scholarly articles in existence. Learned Publ. 2010;23(3):258–63.
Kagoma YK, Crowther MA, Douketis J, Bhandari M, Eikelboom J, Lim W. Use of antifibrinolytic therapy to reduce transfusion in patients undergoing orthopedic surgery: a systematic review of randomized trials. Thromb Res. 2009;123(5):687–96.
Khan KS, Kunz R, Kleijnen J, Antes G. Five steps to conducting a systematic review. J R Soc Med. 2003;96(3):118–21.
Khan KS, Kunz R, Kleijnen J, Antes G. Systematic reviews to support evidence-based medicine: how to review and apply findings of healthcare research. London: Royal Society of Medicine Press; 2003.
Khan M, Evaniew N, Bedi A, Ayeni OR, Bhandari M. Arthroscopic surgery for degenerative tears of the meniscus: a systematic review and meta-analysis. Can Med Assoc J. 2014;186(14):1057–64.
Lau J, Ioannidis JP, Schmid CH. Quantitative synthesis in systematic reviews. Ann Intern Med. 1997;127(9):820.
Liberati A, Al tman DG, Tetzlaff J, Murlow C, Gøtzsche PC, Clarke M, et al. The PRISMA statement for reporting systematic reviews and meta-analyses of studies that evaluate health care interventions: explanation and elaboration. Ann Intern Med. 2009;151(4):W65–94.
Matthew EF, Eleni EP, George AM, Georgios P. Comparison of PubMed, Scopus, Web of Science, and Google Scholar: strengths and weaknesses. Fed Am Soc Exp Biol. 2015;20 Sep 2007.
Mchugh ML. Interrater reliability: the kappa statistic. Biochem Med. 2012;22(3):276–82.
Moher D, Jadad AR, Nichol G, Penman M, Tugwell P, Walsh S. Assessing the quality of randomized controlled trials: an annotated bibliography of scales and checklists. Control Clin Trials. 1995;16:62–73.
Pae C-U. Why systematic review rather than narrative review? Psychiatry Investig. 2015;12(3):417.
Russell RM. Issues and challenges in conducting systematic reviews to support development of nutrient reference values: workshop summary. Rockville: U.S. Dept. of Health and Human Services, Agency for Healthcare Research and Quality; 2009.
Santos JRA. Cronbach’s alpha: a tool for assessing the reliability of scales. J Ext. 1999;37:2.
Slim K, Nini E, Forestier D, Kwiatkowski F, Panis Y, Chipponi J. Methodological index for non-randomized studies (MINORS): development and validation of a new instrument. ANZ J Surg. 2003;73(9):712–6.
Torgerson C. Systematic reviews. London: Continuum; 2003.
Uman LS. Systematic reviews and meta-analyses. J Can Acad Child Adolesc Psychiatry. 2011;20(1):57–9.
PubMed PubMed Central Google Scholar
Verhagen AP, Vet HCD, Bie RAD, Boers M, Brandt PAVD. The art of quality assessment of RCTs included in systematic reviews. J Clin Epidemiol. 2001;54(7):651–4.
Weil RJ. The future of surgical research. PLoS Med. 2004;1(1):e13.
Wright RW, Brand RA, Dunn W, Spindler KP. How to write a systematic review. Clin Orthop Relat Res. 2007;455:23–9.
Zeng X, Zhang Y, Kwong JS, Zhang C, Li S, Sun F, et al. The methodological quality assessment tools for preclinical and clinical studies, systematic review and meta-analysis, and clinical practice guideline: a systematic review. J Evid Based Med. 2015;8(1):2–10.
Download references
Author information
Authors and affiliations.
Department of Orthopaedic Surgery, McMaster University, Hamilton, ON, Canada
Shakib Akhter & Moin Khan
Department of Health, Evidence, and Impact, McMaster University, Hamilton, ON, Canada
Department of Orthopaedic Surgery, McGill University, Montreal, QC, Canada
Thierry Pauyo
You can also search for this author in PubMed Google Scholar
Corresponding author
Correspondence to Shakib Akhter .
Editor information
Editors and affiliations.
UPMC Rooney Sports Complex, University of Pittsburgh, Pittsburgh, PA, USA
Volker Musahl
Department of Orthopaedics, Sahlgrenska Academy, Gothenburg University, Sahlgrenska University Hospital, Gothenburg, Sweden
Jón Karlsson
Department of Orthopaedic Surgery and Traumatology, Kantonsspital Baselland (Bruderholz, Laufen und Liestal), Bruderholz, Switzerland
Michael T. Hirschmann
McMaster University, Hamilton, ON, Canada
Olufemi R. Ayeni
Hospital for Special Surgery, New York, NY, USA
Robert G. Marx
Department of Orthopaedic Surgery, NorthShore University HealthSystem, Evanston, IL, USA
Jason L. Koh
Institute for Medical Science in Sports, Osaka Health Science University, Osaka, Japan
Norimasa Nakamura
Rights and permissions
Reprints and permissions
Copyright information
© 2019 ISAKOS
About this chapter
Akhter, S., Pauyo, T., Khan, M. (2019). What Is the Difference Between a Systematic Review and a Meta-analysis?. In: Musahl, V., et al. Basic Methods Handbook for Clinical Orthopaedic Research. Springer, Berlin, Heidelberg. https://doi.org/10.1007/978-3-662-58254-1_37
Download citation
DOI : https://doi.org/10.1007/978-3-662-58254-1_37
Published : 02 February 2019
Publisher Name : Springer, Berlin, Heidelberg
Print ISBN : 978-3-662-58253-4
Online ISBN : 978-3-662-58254-1
eBook Packages : Medicine Medicine (R0)
Share this chapter
Anyone you share the following link with will be able to read this content:
Sorry, a shareable link is not currently available for this article.
Provided by the Springer Nature SharedIt content-sharing initiative
- Publish with us
Policies and ethics
- Find a journal
- Track your research
A Guide to Conducting Reviews: Meta-Analysis
- Literature Review
- Systematic Review
- Meta-Analysis
- Scoping Review
- Rapid Review
- Umbrella Review
- How and Where to Search?
- Courses and Webinars
- Helpful Tools
- Service Charter
- Online Consultation Form
- Publications Co-authored by AUB University Librarians
- Subject librarians
Definition : A specialized subset of systematic reviews, meta-analysis is a statistical technique for combining the findings from disparate quantitative studies and using the pooled data to come to new statistical conclusions. Not all systematic reviews include meta-analysis, but all meta-analyses are found in systematic reviews.
Aim : To synthesize evidence across studies to detect effects, estimate their magnitudes, and analyze the factors influencing those effects.
Key characteristics:
- Uses statistical methods to objectively evaluate, synthesize, and summarize results.
- Systematic reviews and meta-analyses are undertaken by a research team rather than individual researchers to facilitate expedited review of studies and reduce researcher bias.
Strengths : Combines individual studies to determine overall evidence-based strength. Conclusions produced by meta-analysis are statistically stronger than the analysis of any single study, due to increased numbers of subjects, greater diversity among subjects, or accumulated effects and results.
Drawbacks/Limitations : Combining data from disparate studies produces misleading or unreliable results. For a meta-analysis to be valid, all included studies must be sufficiently similar.
Source : TARG Bristol. (2017, November 13). A three minute primer on meta-analysis [Video]. YouTube. https://www.youtube.com/watch?v=i675gZNe3MY
Further Reading: e-Books
- << Previous: Systematic Review
- Next: Scoping Review >>
- Last Updated: May 13, 2024 8:36 AM
- URL: https://aub.edu.lb.libguides.com/conductingreviews
Warning: The NCBI web site requires JavaScript to function. more...

An official website of the United States government
The .gov means it's official. Federal government websites often end in .gov or .mil. Before sharing sensitive information, make sure you're on a federal government site.
The site is secure. The https:// ensures that you are connecting to the official website and that any information you provide is encrypted and transmitted securely.
- Publications
- Account settings
- Browse Titles
NCBI Bookshelf. A service of the National Library of Medicine, National Institutes of Health.
InformedHealth.org [Internet]. Cologne, Germany: Institute for Quality and Efficiency in Health Care (IQWiG); 2006-.

InformedHealth.org [Internet].
In brief: what are systematic reviews and meta-analyses.
Last Update: September 8, 2016 ; Next update: 2024.
Individual studies are often not big and powerful enough to provide reliable answers on their own. Or several studies on the effects of a treatment might come to different conclusions. In order to find reliable answers to research questions, you therefore have to look at all of the studies and analyze their results together.
Systematic reviews summarize the results of all the studies on a medical treatment and assess the quality of the studies. The analysis is done following a specific, methodologically sound process. In a way, it’s a “study of studies.” Good systematic reviews can provide a reliable overview of the current knowledge in a certain area.
They are normally done by teams of authors working together. The authors are usually specialists with backgrounds in medicine, epidemiology, medical statistics and research.
- How are systematic reviews performed?
Systematic reviews can only provide reliable answers if the studies they are based on are searched for and selected very carefully. The individual steps needed before they can be published are usually quite complex.
- Research question: First of all, the researchers have to decide exactly what question they want to find the answer to. Which treatment should be looked at in which group of people, and what should it be compared with? What should be measured? This set of key questions is also referred to as the PICO framework. PICO stands for P opulation (patient group), I ntervention (the treatment or diagnostic test under investigation), C ontrol (comparison group) and O utcome (variable to be measured). The research question also determines which criteria to use when selecting studies to include in the review – for instance, only certain types of studies .
- Research : Once they know what they are looking for, the researchers have to search as thoroughly and comprehensively as possible for all the studies that might help answer the question. This can easily add up to as many as several hundred studies. Searches for studies are usually done in international databases. Most study results are published online and in English. The relevant information is filtered out using sophisticated methods. The researchers often try to find any unpublished data by contacting and asking other scientists, looking through lists of sources used in other publications, and sometimes even by looking at conference transcripts. One big problem is that some studies are never published. Compared to studies in which treatments are found to have positive outcomes, studies that don’t find any benefits are often published later or never published at all. As a result, the studies that are found and included in reviews might make a treatment seem better than it really is. This kind of systematic bias is also known as “publication bias.”
- Selection: The suitability of every study that is found has to be checked using very specific pre-defined criteria. Studies that do not fulfill the criteria are not included in the review. The suitability of a study is usually assessed by at least two researchers who go through all the studies separately and then compare and discuss their conclusions. This is done in order to try to avoid including unsuitable studies in the review.
- Assessment: The studies that fulfill all the inclusion criteria are carefully assessed . The analysis should provide a comprehensive overview of what is known, and what isn’t known, about the topic in question.
- Peer review: The researchers provide a detailed report of the steps they took, their research methods and what they found. A draft version is critically assessed and commented on by experts. This is called "peer reviewing."
- Publication: If the systematic review “passes” the peer review, it can be published in scientific journals and relevant databases. One important source of systematic reviews is the “Cochrane Library” database. It is run by the Cochrane Collaboration – an international network of researchers who have specialized in producing systematic reviews.
- Keeping the information up-to-date: In order to stay up-to-date, systematic reviews must be updated regularly.
- What is a meta-analysis?
Sometimes the results of all of the studies found and included in a systematic review can be summarized and expressed as an overall result. This is known as a meta-analysis. The overall outcome of the studies is often more conclusive than the results of individual studies.
But it only makes sense to do a meta-analysis if the results of the individual studies are fairly similar (homogeneous). If there are big differences between the results, there are likely to be important differences between the studies. These should be looked at more closely. It is then sometimes possible to split the participants into smaller subgroups and summarize the results separately for each subgroup.
- Bucher H.C. Kritische Bewertung von Studien zu diagnostischen Tests. In: Kunz R, Ollenschläger G, Raspe H, Jonitz G, Donner-Banzhoff N (eds.): Lehrbuch evidenzbasierte Medizin in Klinik und Praxis. Cologne: Deutscher Ärzte-Verlag; 2007.
- Cochrane Germany. Systematische Übersichtsarbeiten der Cochrane Library.
- Greenhalgh T. Einführung in die Evidence-based Medicine: kritische Beurteilung klinischer Studien als Basis einer rationalen Medizin. Bern: Huber; 2003.
- Institute for Quality and Efficiency in Health Care (IQWiG, Germany). Glossar . [ PubMed : 23101074 ]
- Ziegler, A, Lange S, Bender R. Systematische Übersichten und Meta-Analysen. Dtsch Med Wochenschr 2007; 132: e48-e52. [ PubMed : 17530598 ]
IQWiG health information is written with the aim of helping people understand the advantages and disadvantages of the main treatment options and health care services.
Because IQWiG is a German institute, some of the information provided here is specific to the German health care system. The suitability of any of the described options in an individual case can be determined by talking to a doctor. informedhealth.org can provide support for talks with doctors and other medical professionals, but cannot replace them. We do not offer individual consultations.
Our information is based on the results of good-quality studies. It is written by a team of health care professionals, scientists and editors, and reviewed by external experts. You can find a detailed description of how our health information is produced and updated in our methods.
- Cite this Page InformedHealth.org [Internet]. Cologne, Germany: Institute for Quality and Efficiency in Health Care (IQWiG); 2006-. In brief: What are systematic reviews and meta-analyses? [Updated 2016 Sep 8].
- Disable Glossary Links
In this Page
Informed health links, related information.
- PubMed Links to PubMed
Recent Activity
- In brief: What are systematic reviews and meta-analyses? - InformedHealth.org In brief: What are systematic reviews and meta-analyses? - InformedHealth.org
Your browsing activity is empty.
Activity recording is turned off.
Turn recording back on
Connect with NLM
National Library of Medicine 8600 Rockville Pike Bethesda, MD 20894
Web Policies FOIA HHS Vulnerability Disclosure
Help Accessibility Careers
Thank you for visiting nature.com. You are using a browser version with limited support for CSS. To obtain the best experience, we recommend you use a more up to date browser (or turn off compatibility mode in Internet Explorer). In the meantime, to ensure continued support, we are displaying the site without styles and JavaScript.
- View all journals
- Explore content
- About the journal
- Publish with us
- Sign up for alerts
- Open access
- Published: 10 June 2024
A meta-analysis of the effects of design thinking on student learning
- Qing Yu ORCID: orcid.org/0000-0003-1889-1481 1 ,
- Kun Yu 1 &
- Rongri Lin 1
Humanities and Social Sciences Communications volume 11 , Article number: 742 ( 2024 ) Cite this article
346 Accesses
1 Altmetric
Metrics details
- Science, technology and society
Design thinking (DT) is becoming an innovative and popular teaching method. Recently, DT has been used as an unconventional method to develop skills of problem-solving, creativity, and innovation. However, its effects on student learning are unclear . This research aimed to examine the DT’s effects on student learning. The meta-analytic result based on 25 articles showed that DT positively affected student learning ( r = 0.436, p < 0.001). Moreover, the learning outcome, treatment duration, grade level, DT model, and region had moderating effects. Additionally, moderator analysis suggested that DT instruction was more effective: (1) when class size is <=30; (2) on multidiscipline; (3) with long-term duration (>=3 months); (4) for secondary school and university students; (5) on student learning engagement, motivation, problem-solving skills, and academic achievement; (6) with the model of Observe, Synthesize, Ideate, and Prototype, and Empathize, Define, Ideate, Prototype, Test; (7) when team size is <=7; (8) on African and Asian students.
Similar content being viewed by others
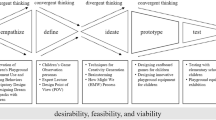
Using design thinking for interdisciplinary curriculum design and teaching: a case study in higher education
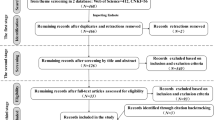
The effectiveness of collaborative problem solving in promoting students’ critical thinking: A meta-analysis based on empirical literature
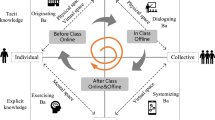
Blended knowledge sharing model in design professional
Introduction.
Design thinking (DT) is attracting more and more attention and interest worldwide (Aris et al., 2022 ). DT was introduced by Rowe ( 1987 ) and was first applied in education in 2005 (Çeviker-Çınar et al., 2017 ). Today, DT has been widely applied in nearly all stages of education (Pande and Bharathi, 2020 ), from formal to informal educational contexts (Aris et al., 2022 ). DT is a process, a method (Rowe, 1987 ), or a “philosophy” (Çeviker-Çınar et al., 2017 ). In education, DT is a teaching method and a learning orientation that enables learners to generate creative ideas and impactful change and actively explore problem solutions (Beckman and Barry, 2007 ; Lor, 2017 ; Retna, 2016 ). DT can help solve many fundamental educational issues (Koh et al., 2015 ). However, previous studies did not reach consensus about DT’s effects on student learning. Moreover, eliciting DT is not always easy because of its complexity and open-endedness (Becker and Mentzer, 2015 ). Therefore, this study carried out a meta-analysis to examine the relationship between DT and student learning.
Conceptual framework
Design thinking.
DT has various definitions. The most widely used definition in education is proposed by Razzouk and Shute ( 2012 ): “an analytic and creative process that engages a person in opportunities to experiment, create and prototype models, gather feedback, and redesign.” DT is a promising, practical method that can be applied to education (Brown, 2008 ; Rusmann and Ejsing-Duun, 2022 ). It is often integrated into the teaching process as an instructional method. DT consists of a set of logically organized stages or processes, each pointing to cultivating students’ key competencies. When students are engaged in DT instruction, they need to follow DT’s steps to move forward with their projects, thereby increasing their ability to perform better. DT also points to addressing problems in real situations (Xu et al., 2024 ), which could increase their interest, motivation, and engagement (Grau and Rockett, 2022 ; Lin et al., 2020a ). In sum, DT has become a dynamic, nonlinear, and spiraling process that can facilitate deep learning (Liu and Li, 2023 ) and eventually result in better student performance (Howard et al., 2021 ).
DT emphasizes learner-centeredness (Glen et al., 2014 ), which can help teachers and students cope with 21st century challenges and complex real-world problems (Gleason and Jaramillo Cherrez, 2021 ; Xu et al., 2024 ; Yande, 2023 ). For teachers , DT provides a framework for solving complex and emerging problems (Henriksen et al., 2020a ); DT also provides good solution strategies and guidance for teachers to design innovative instruction and improve instruction. For students , DT can improve students’ class participation and learning intention, create favorable atmospheres and enjoyment, enhance interaction between each other and creative confidence, deepen their discussion on projects, and eventually improve teachers’ instruction (Balakrishnan, 2022 ; Tu et al., 2018 ). Moreover, DT can also nurture the competencies necessary for students, such as communication, collaboration, teamwork, problem-solving skills, creativity, empathy, critical thinking, and metacognition (Abolhasani et al., 2021 ; Balakrishnan, 2022 ; Guaman-Quintanilla et al., 2023 ; Retna, 2016 ; Rusmann and Ejsing-Duun, 2022 ). In general, the value of DT in education is to help students grow, empower teachers’ development, and promote teaching change.
DT has gradually become the new normal, with students readily embracing the DT process and appreciating its merits (Retna, 2016 ). Meanwhile, a variety of DT models are proposed for use in different domains. Simon ( 1969 ) proposed the first DT model, which entails a one-way linear process of three steps: analysis, synthesis, and evaluation. The most widely applied model in education is that of the Stanford model (Liu et al., 2024a ), which has five stages: empathize, define, ideate, prototype, and test (EDIPT) (Plattner, 2009 ), especially in school and university educational settings. IDEO ( 2013 ) defined five stages of DT for educators: discovery, interpretation, ideation, experimentation, and evolution. To apply DT in K-12 (Liu and Li, 2023 ), Carroll et al. ( 2010 ) extend the EDIPT model to six stages, e.g., understand, observe, point of view, ideate, prototype, and test. Brown’s DT model has been widely used, with three stages: inspiration, ideation, and implementation (Brown, 2008 ). The Design Council’s DT model assists designers or non-designers in solving some of the most complex social, economic, and environmental problems. It has four stages: discover, define, develop, and implement (Design Council, 2015 ). The DT model selected should aim to meet both students’ needs and instructional goals (Brannon, 2022 ). It should be noted that the processes contained in different DT models may vary and therefore produce different results.
DT’s effects and research gaps
Recently, there have been gradually increasing explorations to investigate the impacts of DT on students’ learning performance in education. However, there is no consensus on the effectiveness of DT. The results can be classified into three types: (a) DT can promote students’ learning significantly (Albay and Eisma, 2021 ; Bawaneh and Alnamshan, 2023 ; Chang and Tsai, 2021 ; Dawbin et al., 2021 ; Hsiao et al., 2017 ; Kuo et al., 2022 ; Ladachart et al., 2022 ; Lin et al., 2020a ; Liu and Ko, 2021 ; Nazim and Mohammad, 2022 ; Padagas, 2021 ; Pratomo and Wardani, 2021 ; Simeon et al., 2022 ; Tsai, 2015 ; Ziadat and Sakarneh, 2021 ); (b) DT does not significantly enhance student learning (Khongprakob and Petsangsri, 2022 ; Kuo et al., 2022 ; Lin et al., 2020b ; Yalçın and Erden, 2021 ); (c) there are negative correlations between DT and learning outcomes (Chou and Shih, 2022 ; Lake et al., 2021 ).
It can be seen that DT’s effectiveness is still questionable . DT is an emerging topic that needs in-depth investigation (Baker III and Moukhliss, 2020 ). Some research gaps need to be addressed urgently. First , it lacks specific guidance and references on DT instruction. In-service teachers are unfamiliar with DT (Bressler and Annetta, 2022 ; Liu et al., 2024a ), which may reduce DT’s effects. Students may also undergo confusion and frustration when participating in DT courses (Glen et al., 2015 ; Razali et al., 2022 ). Therefore, it is crucial to explore where the DT approach may be more appropriate for the classroom setting (Lor, 2017 ). For instance, what is the most effective class size, team size, duration, or DT model? Second , DT’s effects are questioned (Rao et al., 2022 ). Namely, a systematic assessment of DT’s effectiveness is limited (Liedtka, 2015 ). There is no meta-analysis to deliver robust evidence on the effectiveness of DT in education. To summarize, with DT’s widespread introduction into education, performing a meta-analysis to reveal DT’s overall effects on student performance and possible influencing moderators is necessary and valuable.
Research purpose
Considering that there is no quantitative, comprehensive evidence on DT’s effects in education, we tried to solve the following questions:
RQ1 . What are the research characteristics of the included empirical studies of DT on student learning (e.g., publication year, research design, class size, grade level, duration, subject, team size, DT model, and region/countries)?
RQ2 . What is the overall effect of DT on student learning?
RQ3 . What are the DT’s effects on student learning under the potential moderators (e.g., learning outcome, class size, grade level, duration, subject, team size, DT model, and region)?
Compared to a mere literature review, meta-analysis can provide precise quantitative effects (Grant and Booth, 2009 ). Meta-analysis can integrate various empirical research results to calculate the overall effect value (Lipsey and Wilson, 2001 ). This research was conducted based on the process proposed by Field and Gillett ( 2010 ).
Literature searching
We mainly retrieved the documents from the Web of Science (Core Collection), Scopus, and Google Scholar. Some topic words, i.e., (“Design Thinking”) AND (“Learning Performance” OR “Learning Outcomes” OR “Academic Achievement” OR “Academic Performance”), were combined to search for the target documents. The search span was confined from January 2005 to June 2023. 1204 articles were retrieved preliminarily through the search, and 1059 articles were obtained after removing duplicated literature.
Selecting criteria and process
We selected literature based on the below criteria:
(1) It must report the relationship between DT and student learning performance;
(2) It must be empirical studies (experimental, quasi-experimental, or correlational research);
(3) The research participants should receive intervention through DT teaching;
(4) It should provide necessary data for calculating effect sizes in targeted papers (e.g., sample size, mean, standard deviation, the value of t or p );
(5) It should be peer-reviewed and published in English .
After the initial of screening of titles and abstracts and the removal of duplicates, 296 articles were selected. Whole-text articles were initially assessed for eligibility, and 84 articles that met the inclusion criteria remained. Finally, after the articles were read in full, 25 peer-reviewed studies were obtained. The literature searching and selection were conducted strictly according to the standard processes (Moher et al., 2009 ) (Fig. 1 ).
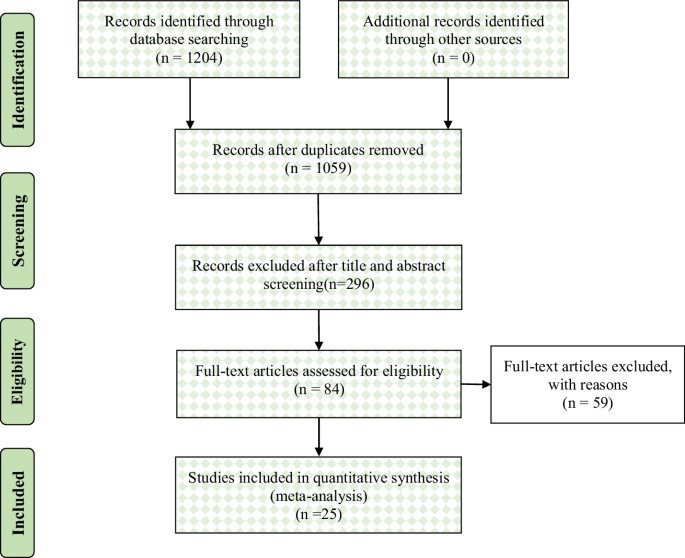
Flow diagram.
Literature Quality and Bias Assessment
One database cannot include all the published literature, so searching multiple authoritative databases can control the literature search bias (Stang, 2010 ). Higgins et al. ( 2019 ) recommend searching at least two databases. So, we selected three databases to reduce the search literature search bias (Kelley and Kelley, 2019 ).
The included criteria’s inaccuracy will result in literature selection bias (Sterne et al., 2016 ). We strictly drew up the selection criteria to reduce this bias, e.g., study purpose and design, intervention of DT, and published language (Liu et al., 2024b ).
We assessed the literature quality based on the criteria of Downs and Black ( 1998 ), which have 27 questions and five categories. We found that all selected studies got majority points in more than four of the above categories (range 18 to 21), so they were high-quality (Carter et al., 2017 ).
Coding potential moderators
Moderators are possible factors that influence DT’s effects. The eight moderators were divided into the background and method.
Background moderators
Learning outcome: DT’s learning outcomes are less examined. Examining DT’s effectiveness on different learning outcomes is necessary (Razzouk and Shute, 2012 ). It was coded into academic achievement, self-efficacy, learning motivation, problem-solving ability, creative thinking, and learning engagement.
Treatment duration: The DT process could take a long time to explore (Carroll et al., 2010 ), and it may moderate DT’s effect on learning. It was divided into <1, 1–3, and >3 months.
Class size: It is an important index of teaching effects (Retna, 2016 ). So, it may moderate DT’s effect on student learning. It was divided into 1–30, 31–50, 51–100, and >100.
Grade level: There should be a clear distinction regarding how DT is applied to different learning stages (Lor, 2017 ). It was divided into kindergarten, primary, junior high, high school, and university.
Subject: DT was not always useful across all subjects (Retna, 2016 ), and van de Grift and Kroeze ( 2016 ) found that it could enhance interdisciplinary education. Namely, the subject may moderate DT’s effects. It was divided into STEM, No-STEM, and multidiscipline.
Region: It refers to the area where the study was performed. The education system’s cultural context must also be considered when applying DT (Retna, 2016 ). So, the region is also considered a potential moderator. It was divided into Asia, America, Austria, Europe, and Africa.
Method moderators
DT model: It refers to DT’s specific processes or stages. The implementation of DT relies on specific models, and different models contain different operations. Therefore, the role of DT models should be considered. We coded the DT model into 9 types:
3IE =Inspiration, Ideation, Implementation, and Evaluation;
UOPIPT =Understand, Observe, Perspective, Imagination, Prototype, and Test;
EDIPT =Empathize, Define, Ideate, Prototype, and Test;
EDEIPT =Empathize, Define, Elaborate, Ideate, Prototype, and Test;
OSIP =Observation, Synthesis, Ideation, and Prototype;
PAS =Preparation, Assimilation, Strategic control;
2UPPI =User focus (User as an information source and User as a codeveloper), Problem framing, Prototype, and Iteration;
CTC =Copy, Tinker, and Create;
LAUNCH =Look, listen and learn, Ask, Understand, Navigate ideas, Create, and Highlight and fix.
Team size: This variable refers to the number of team members. DT pedagogy emphasizes the use of student teams (Beckman and Barry, 2007 ), and team size is one of the causes of conflicts around teamwork (Aflatoony et al., 2018 ). So, the team size may moderate DT’s effect. It was divided into 1–4, 5–7, and >=8.
Data analysis
CMA 3.0 was used to analyze the effect sizes and moderators’ effects. In order to overcome the differences in different studies, the Pearson correlation coefficient r was selected as the effect size (Borenstein et al., 2005 ). Since the paper sample sizes varied widely, the authors employed the Fisher Z -transformation based on the weighted study sample sizes to calculate the ultimate r and 95% confidence intervals (Lei et al., 2020 ).
Publication Bias
We used the funnel plot, classic fail-safe N , and trim-and-fill method to examine the publication bias. If there is no publication bias in the data, the scatter of the funnel would be spread symmetrically. First , the funnel plot showed that the samples in this study were not evenly distributed (Fig. 2 ). Second , fail-safe Nfs quantifies the threshold at which publication bias becomes an issue. CMA can calculate the threshold ( Nfs ). Next, the fail-safe Nfs indicated that Nfs = 9179 was far larger than 220 (5* K + 10, K = 42). Last , the trim-and-fill method can create plots of potentially missing studies to search for symmetry between the literature (Duval and Tweedie, 2000 ). This method found just five missing values on the right of the funnel plot (Fig. 3 ). In sum, it can be concluded that the data included were free from publication bias.
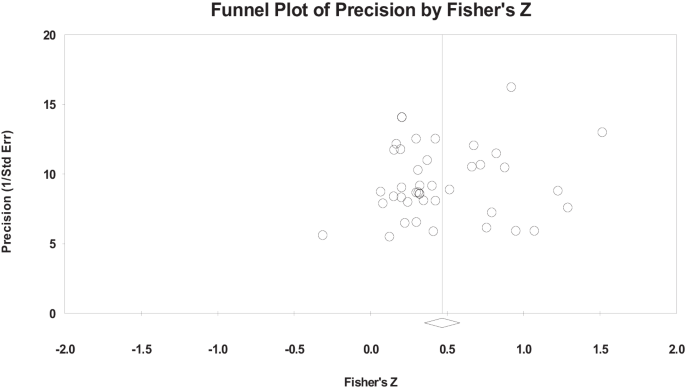
Funnel plot.
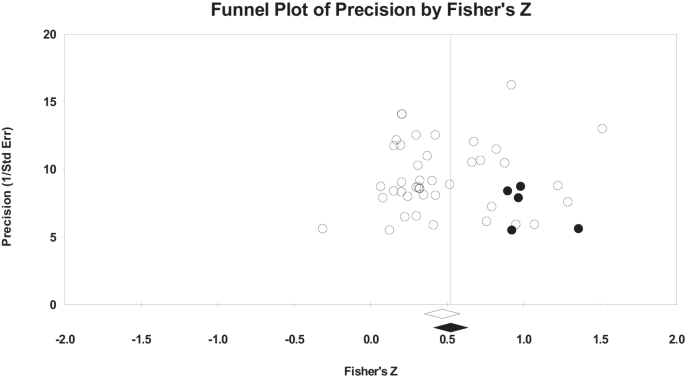
Funnel plot after trill-and-fill.
Actually, literature selection may cause publication bias. To minimize this bias, we strictly developed the selection criteria, e.g., study purpose and design, intervention of DT, necessary data, and peer-review. Especially, we limited the language of publication to English. This may exclude some potential literature published in other languages; it is one limitation of the current research and could be addressed in the future.
Homogeneity test and sensitivity analysis
The values of Q and I 2 can be used to determine whether heterogeneity exists. The result was Q = 554.908 ( p < 0.001) (Table 1 ), which was significant. Moreover, I 2 = 92.611% > 75%, according to Higgins et al. ( 2003 ), meant the heterogeneity was high. Thus, the random-effects model should be selected (Borenstein et al., 2009 ; Wilson et al., 2020 ). Moreover, moderating analyses were also necessary to be analyzed.
To confirm the robustness of this research, we used the one-study-removal method to examine the sensitivity. The result suggested that each overall effect size fell within a reasonable range (from 0.418 to 0.467). Thus, this study is robust.
General characteristics of the included 25 studies
To answer RQ1, reveal the current state of empirical research on DT, and provide complementary evidence for subsequent meta-analyses, a descriptive analysis of the included literature was conducted. The literature included was published between 2015 and 2023, e.g., 1 in 2015 (4.00%), 1 in 2017 (4.00%), 3 in 2020 (12.00%), 8 in 2021 (32.00%), 6 in 2022 (24.00%), and 6 in 2023 (24.00%). The result indicated a growing interest in empirical research on the use of DT for teaching and learning in education. In terms of study design, only 2 were correlational studies (Lin et al., 2020a ; Roth et al., 2020 ), while the other 23 were experimental studies (including pre-experiment, quasi-experiment, and true-experiment). Descriptive results are as follows:
(1) Grade level: kindergarten ( N = 1, 4.00%), primary school ( N = 3, 12.00%), junior high school ( N = 2, 8.00%), high school ( N = 9, 36.00%), and university ( N = 10, 40.00%).
(2) Class size: 0–30 ( N = 9, 36.00%), 31–50 ( N = 10, 40.00%), and >=51( N = 6, 24.00%).
(3) Duration: 0–1 month ( N = 8, 32.00%), 1–3 months ( N = 7, 28.00%), and =>3 months ( N = 10, 40.00%).
(4) Subject: STEM ( N = 16, 64.00%), No-STEM ( N = 6, 24.00%), and multidiscipline ( N = 3, 12.00%).
(5) DT model: EDIPT ( N = 14, 56.00%), 3IE ( N = 1, 4.00%), UOPIPT ( N = 1, 4.00%), LAUNCH ( N = 1, 4.00%), OSIP ( N = 1, 4.00%), PAS ( N = 1, 4.00%), PPI2U ( N = 1, 4.00%), EDEIPT ( N = 1, 4.00%), CTC ( N = 1, 4.00%), and Unknown ( N = 3, 12.00%) (Fig. 4 ).
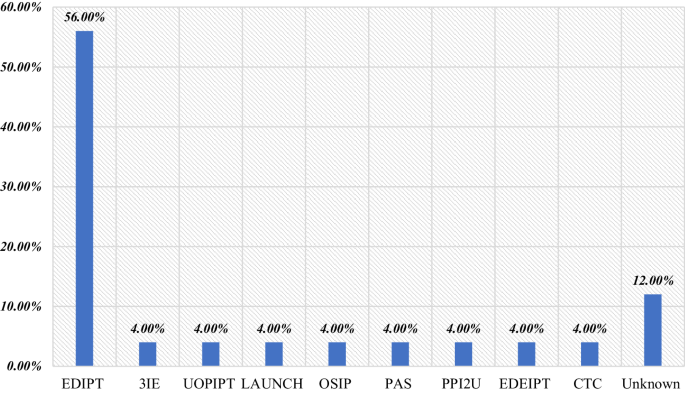
(7) Team size : 0–4 ( N = 7, 53.85%) and 5–7 ( N = 6, 46.15%).
(8) Region : Asia ( N = 21, 84.00%), America ( N = 1, 4.00%), Australia ( N = 1, 4.00%), Europe ( N = 1, 4.00%), and Africa ( N = 1, 4.00%) (Fig. 5 ).
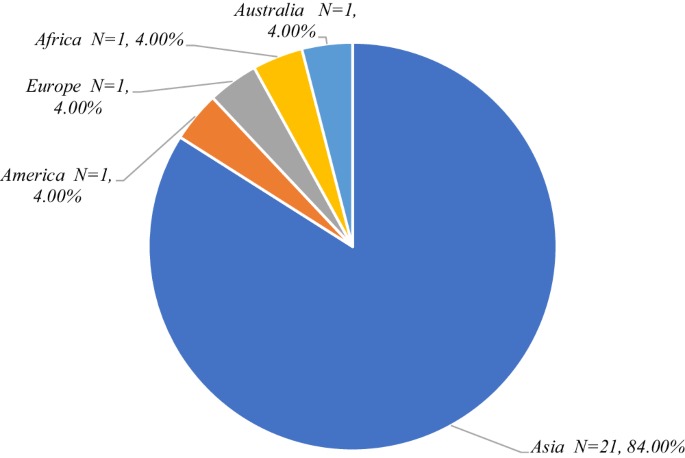
(9) Countries : China ( N = 12, 48.00%), Thailand ( N = 2, 8.00%), Australia ( N = 1, 4.00%), Austria ( N = 1, 4.00%), Philippines ( N = 2, 8.00%), Saudi Arabia ( N = 2, 8.00%), Nigeria ( N = 1, 4.00%), America ( N = 1, 4.00%), Indonesia ( N = 1, 4.00%), Jordan ( N = 1, 4.00%), and Turkey ( N = 1, 4.00%).
In general, the results revealed that most research used EDIPT ( N = 14) as a DT model and focused primarily on the learning of STEM subjects ( N = 16, 64.00%) by high school ( N = 9, 36.00%) and university students ( N = 10, 40.00%) in Asia ( N = 21, 84.00%).
Overall effect size
When r = 0.1, there is a small effect size; r = 0.3 is a medium effect size; and r = 0.5 is a large effect size (Cohen, 2013 ). The overall effect size of DT was upper-medium ( r = 0.436, 95% CI [0.342, 0.525], p < 0.001) (Table 1 ). Moreover, each study’s effect sizes were also provided (Fig. 6 ). The red diamond represents the overall effect size and its CI in the forest plot. Favours A meant the result was in favor of regular instruction, while Favours B meant the result was in support of DT instruction.
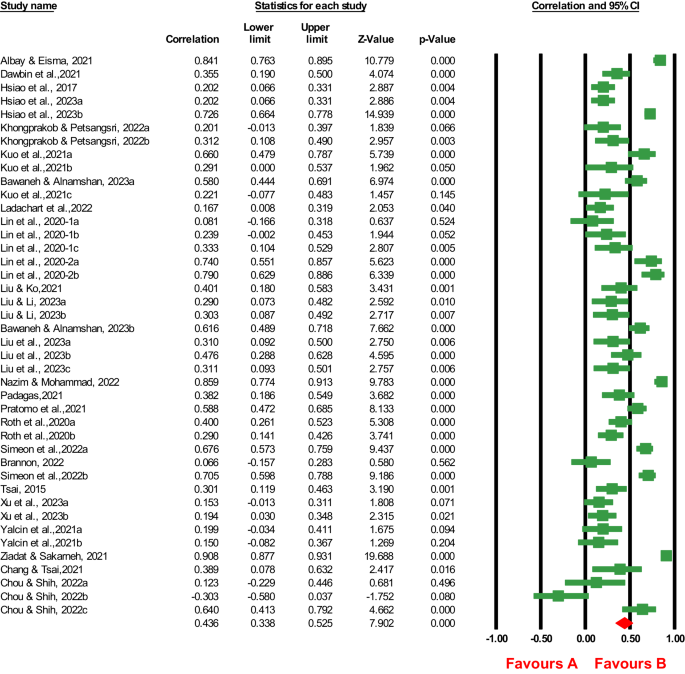
Forest plot.
Moderator analysis
Learning outcome.
The order of effect sizes from large to small was learning engagement ( r = 0.740), learning motivation ( r = 0.608), academic achievement ( r = 0.450), problem-solving ability ( r = 0.447), creative thinking ( r = 0.329), and self-efficacy ( r = 0.230) (Table 2 ). The between-groups effect ( p < 0.01) indicated that the learning outcome had a moderating effect.
The order of effect sizes from large to small was <=30 ( r = 0.609), 31–50 ( r = 0.422), and >=51 ( r = 0.389) (Table 2 ). The result of between-group effects was Q = 0.856 ( p > 0.05), indicating that the class size had no moderating effect.
Treatment duration
The result showed that the effect size of >=3 months ( r = 0.535) was the largest, the next was <=1 month ( r = 0.456), and 1–3 months ( r = 0.245) was the smallest (Table 2 ). The between-groups effect ( p < 0.001) indicated that the treatment had a moderating effect.
Grade level
The order of effect sizes from large to small was high school ( r = 0.538), university ( r = 0.463), junior high school ( r = 0.443, p > 0.05), primary school ( r = 0.222), and kindergarten ( r = 0.174) (Table 2 ). The between-groups effect ( p < 0.01) indicated that the grade level had a moderating effect.
The order of effect sizes from large to small was multidiscipline ( r = 0.604), No-STEM ( r = 0.470), and STEM ( r = 0.393) (Table 3 ). The between-groups effect indicated that the subject had no moderating effect.
The order of effect sizes from large to small was OSIP ( r = 0.766), EDIPT ( r = 0.522), 2UPPI ( r = 0.346), PAS ( r = 0.301), UOPIPT ( r = 0.297), 3IE ( r = 0.222), CTC ( r = 0.191, p > 0.05), EDEIPT ( r = 0.174), and LAUNCH ( r = 0.066, p > 0.05) (Table 3 ). The Q test of the between-groups effect was significant ( p < 0.001), indicating that the DT model had a moderating effect.
The order of effect sizes from large to small was 0–4 ( r = 0.477) and 5–7 ( r = 0.441) (Table 3 ). The between-groups effect ( p > 0.05) indicated that the team size had no moderating effect.
The order of effect sizes from large to small was Africa ( r = 0.690), Asia ( r = 0.435), Australia ( r = 0.355), Europe ( r = 0.346), and America ( r = 0.066, p > 0.05) (Table 3 ). The between-groups effect ( Q = 50.576, p < 0.001) indicated that the region had a moderating effect.
Discussions and implications
This meta-analysis investigates DT’s effect on student learning with 42 validated effect sizes from 25 independent empirical articles. This research reveals that DT has an upper-medium effect on student learning. DT is the gaping link between the theoretical discoveries of social transformation pedagogy and the practical application of the skills needed for the future (Scheer et al., 2012 ). The DT process entails a set of logical stages that point to students’ key competencies. DT instruction can increase students’ involvement, establish a positive learning climate, and promote interaction and communication between teachers and students (Tu et al., 2018 ). Moreover, DT relies on teamwork and hands-on activities, which are beneficial for student learning (Holstermann et al., 2010 ; Oje, 2021 ; Sung et al., 2017 ; Swanson et al., 2019 ). Certainly, connecting DT with courses’ content may be a challenge (Hennessey and Mueller, 2020 ). Overall, if educators organize DT instruction appropriately, it will be effective in improving student learning.
It has a moderating effect. Specifically, DT can promote learners’ creative thinking, learning engagement, motivation, problem-solving ability, self-efficacy, and academic achievement. Notably, the effects of learning motivation, engagement, and academic achievement are large. The DT process entails a set of logical stages that point to students’ key competencies. DT is a dynamic, nonlinear, and spiraling process that can facilitate deep learning (Liu and Li, 2023 ), interest, motivation, creativity, and engagement, and eventually improve student learning (Howard et al., 2021 ; Rao et al., 2022 ). However, there are significant differences in the impacts of DT on student learning outcomes. DT models consist of a set of stages, and some models are complex and challenging. So, its effect on self-efficacy is smaller than other types of learning outcomes. In sum, DT still has great potential to enhance various learning outcomes.
It has no moderating effect. Specifically, <= 30 ( r = 0.609) has a large effect, >= 51 ( r = 0.389) and 31–50 ( r = 0.422) have upper-medium effects. The result suggests that the smaller the class size, the better DT’s effects. DT is a guided, student-oriented process where learners need close supervision, guidance, and feedback (Retna, 2016 ). When the class size is large (>= 51), it is hard for teachers to provide prompt guidance and feedback. Moreover, large class sizes challenge teachers’ effective classroom management and interactions (Blatchford et al., 2009 ). Of course, >= 51 is broad. So, DT’s effects on larger class sizes (e.g., 51–80, etc.) need more exploration. Based on the result, we recommend that educators keep the class size below 51 students. Moreover, if conditions permit, more teachers could be involved in one class (e.g., two teachers) (Retna, 2016 ).
It has a moderating effect. Specifically, the effect of >= 3 months ( r = 0.535) is large, <= 1 month ( r = 0.456) has an upper-medium effect, and 1–3 months ( r = 0.245) has an upper-small effect. Generally, the effect of 1–3 months is best (Yu et al., 2023 ), but our result is the smallest. The novelty effect may result in a larger effect at <=1 month than at 1–3 months. The decrease in the 1–3 months’ effect may be due to the novelty effect wearing off as students slowly familiarize themselves with DT and face learning challenges. Guaman-Quintanilla et al. ( 2023 ) noted that it is challenging to experience the entire process of DT within a limited time. Namely, time constraints are a challenge for students and educators (McLaughlin et al., 2023 ; Retna, 2016 ; Razali et al., 2022 ). Longer durations are needed for educators to conduct DT instruction to make students engage in DT (Razali et al., 2022 ). Actually, DT is a long-term journey to develop students’ abilities and skills, so enough time should be allocated. In short, though DT is effective for these durations, <=1 month or >= 3 months are more effective. More future research could examine the 1–3 months’ effect on DT.
It has a moderating effect. Specifically, high school ( r = 0.538) has the best effect; university ( r = 0.463) has an upper-medium effect; primary school ( r = 0.222) and kindergarten ( r = 0.174) have small effects; and junior high school ( r = 0.443, p > 0.05) has an insignificant effect. DT has been used in all stages of education, and DT is also effective. In this research, DT shows greater potential for high school and university students than for primary school and kindergarten students. DT is a task- and activity-oriented learning process that relies on team communication and collaboration, DT studies at different stages might yield different results due to cognitive-developmental differences (Mentzer et al., 2015 ). Given the complexity of DT, more DT instruction could be applied to university and secondary school students. Moreover, for researchers, more studies should be carried out at diverse grade levels, especially in kindergarten ( k = 2) and junior high school ( k = 4).
It has no moderating effect, but the effect of multidiscipline is better than that of STEM and No-STEM. This suggests that DT can foster multidisciplinary learning, consistent with previous studies (Chang and Tsai, 2021 ; de Figueiredo, 2021 ; van de Grift and Kroeze, 2016 ). DT has typical interdisciplinary features (Lugmayr et al., 2014 ) and can promote new solutions, innovation, and collaboration opportunities for complex problems in multidisciplinary areas (Cook and Bush, 2018 ; Gleason and Jaramillo Cherrez, 2021 ). At the same time, DT can be integrated into the subjects of STEM or No-STEM to promote learning and teaching (Hsiao et al., 2023 ). DT is taught as a concept rather than affiliated with a specific discipline (Lor, 2017 ). We recommend integrating DT into existing courses rather than adding additional add-on activities (Sandars and Goh, 2020 ), especially for multidisciplinary learning (Hsiao et al., 2023 ). Different disciplines or subjects have their own suitable design processes (Sung and Kelley, 2019 ), the result provides a broad subject division for reference. Future research could explore DT’s effects on more detailed subjects. Besides, most of DT was applied to STEM subjects ( k = 32), fewer to No-STEM and multidiscipline. So, DT’s effects on both latter subjects should be viewed cautiously and pay more research attention.
It has a moderating effect, indicating that different DT models could generate heterogeneity. Specially, OSIP ( r = 0.766) and EDIPT ( r = 0.522) have large effects; PPI2U ( r = 0.346) and PAS ( r = 0.301) have lower-medium effects; UOPIPT ( r = 0.297), 3IE ( r = 0.222), EDEIPT ( r = 0.174) have small effects; and CTC ( r = 0.191, p > 0.05) and LAUNCH ( r = 0.066, p > 0.05) have no significant effects. Before DT can be effectively implemented to solve complicated problems, it is essential to have a solid grasp and comprehension of the different stages of the DT process (Dam and Teo, 2019 ). Different DT models involve different steps or stages, which may affect the processes of cognition and learning. For instance, EDIPT is easier for middle school students (Sarooghi et al.m 2019 ). Based on the result of this meta-analysis, we recommend that educators adopt the models of EDIPT and OSIP in DT instruction. Importantly, educators should not rely too heavily on the pre-determined procedural DT processes, which may hinder the creative potential of DT (Wells, 2013 ). Educators can rationalize the DT model based on their actual situations (Li and Zhan, 2022 ). It is also necessary to mention that, with the exception of EDIPT, the numbers of effect sizes included in other DT models are small, so their results should be treated cautiously and more explorations are needed.
It has no moderating effect. Team sizes of 0–4 ( r = 0.477) and 5–7 ( r = 0.441) have upper-medium effects. Teamwork and team collaboration are great challenges for many students. DT could enhance students’ teamwork (Guaman-Quintanilla et al., 2022 ). Success in DT requires teamwork, and larger teams can enrich the diversity of perspectives and increase the likelihood of solutions (Sung et al., 2017 ). Moreover, the composition of teams is also important (Apedoe et al., 2012 ). Generally speaking, heterogeneous ability groups may be appropriate in DT (Lou et al., 1996 ), i.e., both low-ability and high-ability students, and both male and female students (Yu and Yu, 2023 ). From the result of this research, 2–7 members in one group are beneficial. A larger number of teams may limit the teachers’ ability to guide and facilitate each team’s, and individual students’ learning (Apedoe et al., 2012 ). We recommend having <=7 members in one group. Specifically, when the class size is large, 5–7 is better; otherwise, 2–4 will be better. However, the result shows a broad team size for reference only. So, future research could explore which specific composition of teams (from 2 to 7 or above) in DT instruction is better.
It has a moderating effect. Specifically, Africa ( r = 0.690) has a large effect, Asia ( r = 0.435), and Australia ( r = 0.355), and Europe ( r = 0.346) have upper-medium effects, while America has an insignificant effect. This may be due to differences in cultural and educational systems in different regions. Different from individualistic cultures (e.g., America, Australia, Austria), most Asian countries are collectivist (e.g., China, Thailand, Indonesia, etc.), and students in these countries tend to value team goals more than individual goals (De Mooij and Hofstede, 2010 ). So, DT has an upper-medium effect on Asian students. Since the study distribution between different regions was highly uneven, this result should be treated judiciously. For instance, except for Asia, other regions’ studies are small, e.g., Australia ( N = 1), Europe ( N = 1), Africa ( N = 1), and America ( N = 1), so these regions need more attention. In general, DT positively impacts student learning in diverse regions, and DT is recommended to enhance Asian students’ learning.
Implications for future practice and work
This meta-analysis makes an evidence-based analysis of DT’s effects on student learning, and we provide some meaningful suggestions for future practice and research. These are also major contributions to the existing literature.
First , though DT’s effects on different types of learning outcomes are significantly different, it is still an effective teaching method to improve student learning. Educators can apply DT to enhance student academic performance, creative thinking, learning engagement, motivation, and problem-solving ability. Due to the limited amount of learning engagement and self-efficacy, their effects should be treated cautiously.
Second , a smaller size means a larger DT’s effect. Educators should keep the class size <51. Future research could focus more on exploring DT’s effects on larger class sizes (e.g., 51–80, etc.).
Third , treatment duration is a critical factor. <= 1 month or >=3 months are more suggested. Particularly, DT’s effect is smallest when the duration is 1–3 months, and this needs more future research.
Fourth , grade level is a key factor. DT could be applied to university and high school students. DT’s effect on junior high school is insignificant . Researchers could carry out more studies at kindergarten ( k = 2) and junior high school ( k = 4).
Fifth , DT can be used in the subjects of STEM, No-STEM, or multidiscipline. Meanwhile,future research could explore more on No-STEM, multidiscipline, and more detailed subjects.
Sixth , the DT model is also a critical factor that should be considered. Based on the results of this study, we recommend that educators adopt the models of EDIPT. Importantly, except for EDIPT, other models’ effects need more exploration.
Seventh , in terms of team size, it is suggested to have <= 7 members in one group. Specifically, when the class size is large, 5–7 is better; otherwise, 2–4 will be preferred. However, the result shows a wide range. Future research could explore which specific composition of teams (from 2 to 7 or above) is better for DT instruction.
Eighth , regional analysis suggests that DT is most used in Asia and is most suggested to support Asian student learning. However, the number of effect sizes in other regions is very small. Thus, their results should be viewed with caution, and future researchers can take more steps to test DT’s effects in America, Africa, Australia, and Europe.
Conclusions, limitations and future research
Conclusions.
This meta-analytic evidence reveals DT’s effects in education based on 25 empirical studies. We find that DT has an upper-medium positive effect on students’ learning. Specifically, DT can lead to higher learners’ creative thinking, learning engagement, motivation, problem-solving ability, self-efficacy, and academic achievement. In comparison, DT has better effects on student learning motivation, engagement, and academic achievement. Furthermore, the learning outcome, grade level, treatment duration, DT model, and region moderate DT’s effects on student learning. Namely, these moderators will affect DT’s effectiveness.
DT is on-trend worldwide (Aris et al., 2022 ), and it has profoundly changed many educators’ thinking about how to instruct to support learning (Hubbard and Datnow, 2020 ). Teachers are vital in DT instruction; they should be facilitators and navigators, not lecturers (Henriksen et al., 2020b ; Retna, 2016 ; Rusmann and Ejsing-Duun, 2022 ). In sum, DT can potentially promote learning at different grade levels, but the effectiveness of DT in education depends upon the goals (Panke, 2019 ). It is critical to make teachers see the value of DT in classrooms (Carroll et al. 2010 ) and conduct DT instruction with guidance and rules. This paper provides evidence-based findings for educators and researchers.
Limitations, research gaps, and future directions
There are several limitations that should be solved for future work. First , the literature is distributed unevenly by region, grade level, and DT model, so more future studies could be taken at kindergarten ( k = 2), junior high school ( k = 4), America ( k = 1), Australia ( k = 1), Africa ( k = 2), Europe ( k = 2), learning engagement ( k = 1), self-efficacy ( k = 3), and DT model except EDIPT. Second , the literature included in this meta-analysis was published in English . Future work could include other language studies. Third , the heterogeneity is considerable, and some potential moderators may be overlooked. Future work could explore more factors that influence DT’s effectiveness, e.g., learning environments. Fourth , the included literature is not large; future research could focus on experimental design to explore DT’s effects on student learning. Last , a meta-analysis may not display the whole status and findings of DT in education. Future researchers could conduct a systematic literature review to compensate for the neglected aspects of the current research.
Data availability
All data is provided in the forest plot and references. The details are provided at https://doi.org/10.7910/DVN/EHGCGS .
References (*included in this meta-analysis)
Abolhasani Z, Dehghani M, Javadipour M, Salehi K, Mohammadhasani N (2021) An analysis of the role of design thinking in promoting the 21st-century skills: a systematic review. Technol Educ J 16(1):81–98. https://doi.org/10.22061/tej.2021.7206.2508
Aflatoony L, Wakkary R, Neustaedter C (2018) Becoming a design thinker: assessing the learning process of students in a secondary level design thinking course. Int J Art Design Educ 37(3):438–453. https://doi.org/10.1111/jade.12139
*Albay EM, Eisma DV (2021) Performance task assessment supported by the design thinking process: Results from a true experimental research. Soc Sci Human Open 3(1):100116. https://doi.org/10.1016/j.ssaho.2021.100116
Apedoe XS, Ellefson MR, Schunn CD (2012) Learning together while designing: does group size make a difference? J Sci Edu Technol 21(1):83–94. https://doi.org/10.1007/s10956-011-9284-5
Aris NM, Ibrahim NH, Abd Halim ND, Ali S, Rusli NH, Suratin MNM, Hassan FC (2022) Evaluating the academic trends on design thinking research: A bibliometric analysis from 2000 to 2021. J Positive School Psychol 6(4):1022–1038
Google Scholar
Baker III FW, Moukhliss S (2020) Concretising design thinking: a content analysis of systematic and extended literature reviews on design thinking and human‐centred design. Rev Educ 8(1):305–333. https://doi.org/10.1002/rev3.3186
Balakrishnan B (2022) Exploring the impact of design thinking tool among design undergraduates: a study on creative skills and motivation to think creatively. Int J Technol Design Educ 32(3):1799–1812. https://doi.org/10.1007/s10798-021-09652-y
*Bawaneh AK, Alnamshan MM (2023) Design Thinking in Science Education: Enhancing Undergraduate Students’ Motivation and Achievement in Learning Biology. Int J Inf Educ Technol 13(4):621–633. https://doi.org/10.18178/ijiet.2023.13.4.1846
Beckman SL, Barry M (2007) Innovation as a learning process: embedding design thinking. California Manag Rev 50(1):25–56. https://doi.org/10.2307/41166415
Becker K, Mentzer N (2015) Engineering design thinking: high school students’ performance and knowledge. In 2015 International Conference on Interactive Collaborative Learning, IEEE, (pp 5–12), Firenze, Italy
Blatchford P, Russell A, Brown P (2009) Teaching in large and small classes. In: LJ Saha, & AG Dworkin (eds.), International Handbook of Research on Teachers and Teaching. Springer, Boston, MA
Borenstein M, Hedges L, Higgins J, Rothstein H (2005) Comprehensive meta-analysis (version 3.3) (p. 104). Englewood, NJ: Biostat
Borenstein M, Hedges LV, Higgins JP, Rothstein HR (2009) Introduction to meta-analysis. John Wiley & Sons
Brown T (2008) Design thinking. Harvard Business Review 86(6):84–92
PubMed Google Scholar
*Brannon ME (2022) Exploring the impact of design thinking on creativity in preservice teachers. Doctoral dissertation, Kent State University
Bressler DM, Annetta LA (2022) Using game design to increase teachers’ familiarity with design thinking. Int J Technol Design Educ 32(2):1023–1035. https://doi.org/10.1007/s10798-020-09628-4
Carroll M, Goldman S, Britos L, Koh J, Royalty A, Hornstein M (2010) Destination, imagination and the fires within: design thinking in a middle school classroom. Int J Art Design Educ 29(1):37–53. https://doi.org/10.1111/j.1476-8070.2010.01632.x
Carter G, Milner A, McGill K, Pirkis J, Kapur N, Spittal MJ (2017) Predicting suicidal behaviours using clinical instruments: systematic review and meta-analysis of positive predictive values for risk scales. Br J Psychiatry 210(6):387–395. https://doi.org/10.1192/bjp.bp.116.182717
Article PubMed Google Scholar
Çeviker-Çınar G, Mura G, Demirbağ-Kaplan M (2017) Design thinking: a new road map in business education. Design J 20(S1):977–987. https://doi.org/10.1080/14606925.2017.1353042
Article Google Scholar
*Chang YS, Tsai MC (2021) Effects of design thinking on artificial intelligence learning and creativity. Educ Stud 1–18. https://doi.org/10.1080/03055698.2021.1999213
*Chou PN, Shih RC (2022) Engineering design thinking in LEGO robot projects: an experimental study. In International Conference on Innovative Technologies and Learning. Springer, (p 324–333)
Cohen J (2013) S tatistical power analysis for the behavioral sciences. Routledge
Cook KL, Bush SB (2018) Design thinking in integrated STEAM learning: surveying the landscape and exploring exemplars in elementary grades. School Sci Mathe 118 (3-4):93–103. https://doi.org/10.1111/ssm.12268
Dam RF, Teo YS (2019) 5 stages in the design thinking process. https://www.interaction-design.org/literature/article/5-stages-in-the-design-thinking-process
*Dawbin B, Sherwen M, Dean S, Donnelly S, Cant R(2021) Building empathy through a design thinking project: A case study with middle secondary schoolboys. Issues Educ Res 31(2):440–457
De Mooij M, Hofstede G (2010) The Hofstede model: applications to global branding and advertising strategy and research. Int J Advertis 29(1):85–110. https://doi.org/10.2501/S026504870920104X
de Figueiredo MD (2021) Design is cool, but… A critical appraisal of design thinking in management education Int J Manag Educ 19(1):100429. https://doi.org/10.1016/j.ijme.2020.100429
Design Council (2015) What is the framework for innovation? Design Council’s evolved Double Diamond. https://www.designcouncil.org.uk/news-opinion/what-framework-innovation-design-councils-evolved-double-diamond
Downs SH, Black N (1998) The feasibility of creating a checklist for the assessment of the methodological quality both of randomised and non-randomised studies of health care interventions. J Epidemiol Commun Health 52(6):377–384. https://doi.org/10.1136/jech.52.6.377
Article CAS Google Scholar
Duval S, Tweedie R (2000) Trim and fill: a simple funnel-plot-based method of testing and adjusting for publication bias in meta-analysis. Biometrics 56(2):455–463. https://doi.org/10.1111/j.0006-341x.2000.00455.x
Article CAS PubMed Google Scholar
Field AP, Gillett R (2010) How to do a meta‐analysis. Br J Math Stat Psychol 63(3):665–694. https://doi.org/10.1348/000711010X502733
Article MathSciNet PubMed Google Scholar
Gleason B, Jaramillo Cherrez N (2024) Design thinking approach to global collaboration and empowered learning: virtual exchange as innovation in a teacher education course. TechTrends 65(3):348–358. https://doi.org/10.1007/s11528-020-00573-6
Glen R, Suciu C, Baughn C (2014) The need for design thinking in business schools. Acad Manag Learn Educ 13(4):653–667. https://doi.org/10.5465/amle.2012.0308
Glen R, Suciu C, Baughn CC, Anson R (2015) Teaching design thinking in business schools. Int J Manag Educ 13(2):182–192. https://doi.org/10.1016/j.ijme.2015.05.001
Grant MJ, Booth A (2009) A typology of reviews: an analysis of 14 review types and associated methodologies. Health Inform Lib J 26(2):91–108. https://doi.org/10.1111/j.1471-1842.2009.00848.x
Grau SL, Rockett T (2022) Creating student-centred experiences: using design thinking to create student engagement. J Entrep 31(2_suppl):S135–S159. https://doi.org/10.1177/09713557221107443
Guaman-Quintanilla S, Everaert P, Chiluiza K, Valcke M (2022) Fostering teamwork through design thinking: evidence from a multi-actor perspective. Educ Sci 12(4):279. https://doi.org/10.3390/educsci12040279
Guaman-Quintanilla S, Everaert P, Chiluiza K, Valcke M (2023) Impact of design thinking in higher education: a multi-actor perspective on problem solving and creativity. Int J Technol Design Educ 33(1):217–240. https://doi.org/10.1007/s10798-021-09724-z
Henriksen D, Jordan M, Foulger TS, Zuiker S, Mishra P (2020a) Essential tensions in facilitating design thinking: collective reflections. J Formative Design Learn 4(1):5–16. https://doi.org/10.1007/s41686-020-00045-3
Henriksen D, Gretter S, Richardson C (2020b) Design thinking and the practicing teacher: addressing problems of practice in teacher education. Teach Educ 31(2):209–229. https://doi.org/10.1080/10476210.2018.1531841
Hennessey E, Mueller J (2020) Teaching and learning design thinking (DT). Can J Educ/Revue Canadienne de l'éducation 43(2):498–521
Higgins JP, Thompson SG, Deeks JJ, Altman DG (2003) Measuring inconsistency in meta-analyses. BMJ 327(7414):557–560. https://doi.org/10.1136/bmj.327.7414.557
Article PubMed PubMed Central Google Scholar
Higgins JP, Thomas J, Chandler J, Cumpston M, Li T, Page MJ, Welch VA (2019) Cochrane handbook for systematic reviews of interventions, 2nd edn. John Wiley & Sons
Holstermann N, Grube D, Bögeholz S (2010) Hands-on activities and their influence on students’ interest. Res Sci Educ 40(5):743–757. https://doi.org/10.1007/s11165-009-9142-0
Howard JL, Bureau JS, Guay F, Chong JX, Ryan RM (2021) Student motivation and associated outcomes: a meta-analysis from self-determination theory. Perspect Psychol Sci 16(6):1300–1323. https://doi.org/10.1177/1745691620966789
*Hsiao HS, Yu KC, Chang YS, Chien YH, Lin KY, Lin CY, … Lin YW (2017) The study on integrating the design thinking model and STEM activity unit for senior high school living technology course. In 2017 7th World Engineering Education Forum. IEEE, (pp 383–390), Kuala Lumpur, Malaysia
*Hsiao HS, Chang YC, Lin KY, Chen JC, Lin CY, Chung GH, Chen JH (2023) Applying the design thinking model to hands-on mechatronics STEM activities for senior high school students to improve the learning performance and learning behavior. Int J Technol Design Educ 33(a):1389–1408
Hubbard L, Datnow A (2020)Design thinking, leadership, and the grammar of schooling: Implications for educational change. Am J Educ 126(4):499–518. https://doi.org/10.1086/709510
IDEO (2013) Design thinking for educators. https://www.ideo.com/post/design-thinking-for-educators
Kelley GA, Kelley KS (2019) Systematic reviews and meta-analysis in rheumatology: a gentle introduction for clinicians. Clin Rheumatol 38(8):2029–2038. https://doi.org/10.1007/s10067-019-04590-6
*Khongprakob N, Petsangsri S (2022) Promoting Undergraduate Creativity and Positive Learning Outcomes through a Design Thinking and Visual Thinking Teaching Model. J Positive Psychol Wellbeing 6(1):3809–3821
Koh JHL, Chai CS, Wong B, Hong HY (2015) Design thinking for education: conceptions and applications in teaching and learning. Springer, Singapore
*Kuo HC, Yang YTC, Chen JS, Hou TW, Ho MT(2022) The impact of design thinking PBL robot course on college students’ learning motivation and creative thinking. IEEE Transac Educ 65(22):1–8. https://doi.org/10.1109/TE.2021.3098295
*Ladachart L, Cholsin J, Kwanpet S, Teerapanpong R, Dessi A, Phuangsuwan L, Phothong W(2022) Ninth-grade students’ perceptions on the design-thinking mindset in the context of reverse engineering. Int J Technol Design Educ 32(5):2445–2465. https://doi.org/10.1007/s10798-021-09701-6
Lake D, Flannery K, Kearns M (2021) A Cross-Disciplines and Cross-Sector Mixed-Methods Examination of Design Thinking Practices and Outcome. Innov Higher Edu 46(3):337–356. https://doi.org/10.1007/s10755-020-09539-1
Lei H, Chiu MM, Li F, Wang X, Geng YJ (2020) Computational thinking and academic achievement: A meta-analysis among students. Children and Youth Services Review 118:105439. https://doi.org/10.1016/j.childyouth.2020.105439
Liedtka J (2015) Perspective: linking design thinking with innovation outcomes through cognitive bias reduction. J Prod Innov Manag 32(6):925–938. https://doi.org/10.1111/jpim.12163
*Lin PY, Hong HY, Chai CS(2020a) Fostering college students’ design thinking in a knowledge-building environment. Educ Technol Res Dev 68(3):949–974. https://doi.org/10.1007/s11423-019-09712-0
*Lin L, Shadiev R, Hwang WY, Shen S(2020b) From knowledge and skills to digital works: An application of design thinking in the information technology course. Thinking Skills Creativ 36:100646. https://doi.org/10.1016/j.tsc.2020.100646
Lipsey MW, Wilson DB (2001) Practical meta-analysis. Sage, Thousand Oaks, CA, USA
*Liu GC, Ko CH (2021) Effects of social media and design thinking on corporate identity design course in Taiwan. E-Learn Digital Media 18(3):251–268. https://doi.org/10.1177/2042753020950879
Li T, Zhan Z (2022) A systematic review on design thinking Integrated Learning in K-12 education. Appl Sci 12(16):8077. https://doi.org/10.3390/app12168077
*Liu S, Li C (2023) Promoting design thinking and creativity by making: a quasi-experiment in the information technology course. Thinking Skills Creativ 49:101335. https://doi.org/10.1016/j.tsc.2023.101335
*Liu X, Gu J, Xu J (2024a) The impact of the design thinking model on pre-service teachers’ creativity self-efficacy, inventive problem-solving skills, and technology-related motivation. Int J Technol Design Educ 34(1):167–190. https://doi.org/10.1007/s10798-023-09809-x
Liu S, Zhao X, Meng X, Ji W, Liu L, Li W, Tao Y, Peng Y, Yang Q (2024b) Research on the application of extended reality in the construction and management of landscape engineering. Electronics 13(5):897. https://doi.org/10.3390/electronics13050897
Lor R (2017) Design thinking in education: a critical review of literature. In International Academic Conference on Social Sciences and Management / Asian Conference on Education and Psychology. Bangkok, Thailand, (p 37–68)
Lou Y, Abrami PC, Spence JC, Poulsen C, Chambers B, d’Apollonia S (1996) Within-class grouping: a meta-analysis. Rev Educ Res 66(4):423–458. https://doi.org/10.3102/00346543066004423
Lugmayr A, Stockleben B, Zou Y, Anzenhofer S, Jalonen M (2014) Applying “design thinking” in the context of media management education. Multimedia Tools Appl 71:119–157. https://doi.org/10.1007/s11042-013-1361-8
McLaughlin JE, Lake D, Chen E, Guo W, Knock M, Knotek S (2023) Faculty experiences and motivations in design thinking teaching and learning. Front Educ 8:1172814. https://doi.org/10.3389/feduc.2023.1172814
Mentzer N, Becker K, Sutton M (2015) Engineering design thinking: high school students’ performance and knowledge. J Eng Educ 104(4):417–432. https://doi.org/10.1002/jee.20105
Moher D, Liberati A, Tetzlaff J, Altman DG, Group PRISMA (2009) Preferred reporting items for systematic reviews and meta-analyses: the PRISMA statement. Ann Internal Med 151(4):264–269. https://doi.org/10.7326/0003-4819-151-4-200908180-00135
*Nazim M, Mohammad T (2022) Implications of design thinking in an EFL classroom: writing in context. Theory Pract Language Stud 12(12):2723–2730. https://doi.org/10.17507/tpls.1212.31
Oje O (2021) The effects of hands-on learning on stem student motivation: a meta-analysis. Master thesis, Washington State University. https://doi.org/10.7273/000000061
*Padagas RC(2021) Design Thinking in a Professional Nursing Course–Its Effectiveness and Unearthed Lessons Revista Românească pentru Educaţie Multidimensională 13(2):132–146
Panke S (2019) Design thinking in education: perspectives, opportunities and challenges Open Educ Stud 1(1):281–306. https://doi.org/10.1515/edu-2019-0022
Pande M, Bharathi SV (2020) Theoretical foundations of design thinking–A constructivism learning approach to design thinking. Thinking Skills Creativ 36:100637. https://doi.org/10.1016/j.tsc.2020.100637
Plattner H (2009) An introduction to design thinking: Process guide. Stanford Institute of Design
*Pratomo LC, Wardani DK (2021) The effectiveness of design thinking in improving student creativity skills and entrepreneurial alertness. Int J Instruct 14(4):695–712. https://doi.org/10.29333/iji.2021.14440a
Rao H, Puranam P, Singh J (2022) Does design thinking training increase creativity? Results from a field experiment with middle-school students. Innovation 24(2):315–332. https://doi.org/10.1080/14479338.2021.1897468
Razali NH, Ali NNN, Safiyuddin SK, Khalid F (2022) Design thinking approaches in education and their challenges: a systematic literature review. Creative Educ 13(7):2289–2299. https://doi.org/10.4236/ce.2022.137145
Razzouk R, Shute V (2012) What is design thinking and why is it important? Rev Educ Res 82(3):330–348. https://doi.org/10.3102/0034654312457429
Retna KS (2016) Thinking about “design thinking”: a study of teacher experiences. Asia Pac J Educ 36(S1):5–19. https://doi.org/10.1080/02188791.2015.1005049
*Roth K, Globocnik D, Rau C, Neyer AK(2020) Living up to the expectations: the effect of design thinking on project success Creativ Innov Manag 29(4):667–684. https://doi.org/10.1111/caim.12408
Rowe P (1987) Design thinking. The MIT Press, Cambridge, MA, USA
Rusmann A, Ejsing-Duun S (2022) When design thinking goes to school: a literature review of design competences for the K-12 level. Int J Technol Design Educ 32(4):2063–2091. https://doi.org/10.1007/s10798-021-09692-4
Sarooghi H, Sunny S, Hornsby J, Fernhaber S (2019) Design thinking and entrepreneurship education: Where are we, and what are the possibilities? J Small Bus Manag 57(S1):78–93. https://doi.org/10.1111/jsbm.12541
Sandars J, Goh PS (2020) Design thinking in medical education: the key features and practical application. J Med Educ Curricular Dev 7:1–5. https://doi.org/10.1177/2382120520926518
Scheer A, Noweski C, Meinel C (2012) Transforming constructivist learning into action: Design thinking in education. Design Technol Edu: Int J 17(3):8–19
Simon HA (1969) The sciences of the artificial. The MIT Press, Cambridge, MA, USA
*Simeon MI, Samsudin MA, Yakob N(2022) Effect of design thinking approach on students’ achievement in some selected physics concepts in the context of STEM learning Int J Technol Design Educ 32(1):185–212. https://doi.org/10.1007/s10798-020-09601-1
Stang A (2010) Critical evaluation of the Newcastle-Ottawa scale for the assessment of the quality of nonrandomized studies in meta-analyses. Eur J Epidemiol 25(9):603–605. https://doi.org/10.1007/s10654-010-9491-z
Sterne JA, Hernán MA, Reeves BC, Savović J, Berkman ND, Viswanathan M, Higgins JP (2016) ROBINS-I: a tool for assessing risk of bias in non-randomised studies of interventions. BMJ 355:i4919. https://doi.org/10.1136/bmj.i4919
Sung YT, Yang JM, Lee HY (2017) The effects of mobile-computer-supported collaborative learning: meta-analysis and critical synthesis. Rev Educ Res 87(4):768–805. https://doi.org/10.3102/0034654317704307
Sung E, Kelley TR (2019) Identifying design process patterns: a sequential analysis study of design thinking. Int J Technol Design Educ 29(2):283–302. https://doi.org/10.1007/s10798-018-9448-1
Swanson E, McCulley LV, Osman DJ, Scammacca Lewis N, Solis M (2019) The effect of team-based learning on content knowledge: a meta-analysis. Active Learn Higher Educ 20(1):39–50. https://doi.org/10.1177/1469787417731201
*Tsai CW (2015) Investigating the effects of web-mediated design thinking and co-regulated learning on developing students’ computing skills in a blended course. Univ Access Inform Soc 14(2):295–305. https://doi.org/10.1007/s10209-015-0401-8
Tu JC, Liu LX, Wu KY (2018) Study on the learning effectiveness of Stanford design thinking in integrated design education. Sustainability 10(8):2649. https://doi.org/10.3390/su10082649
van de Grift TC, Kroeze R (2016) Design thinking as a tool for interdisciplinary education in health care. Acad Med 91(9):1234–1238. https://doi.org/10.1097/ACM.0000000000001195
Wells A (2013) The importance of design thinking for technological literacy: a phenomenological perspective. Int J Technol Design Educ 23(3):623–636. https://doi.org/10.1007/s10798-012-9207-7
Article MathSciNet Google Scholar
Wilson ML, Ritzhaupt AD, Cheng L (2020) The impact of teacher education courses for technology integration on pre-service teacher knowledge: A meta-analysis study. Comput Educ 156:103941. https://doi.org/10.1016/j.compedu.2020.103941
*Xu W, Chen JC, Lou YF, Chen H (2024) Impacts of maker education-design thinking integration on knowledge, creative tendencies, and perceptions of the engineering profession. Int J Technol Design Educ 34(1):75–107. https://doi.org/10.1007/s10798-023-09810-4
Yande A (2023) Enhancing Student Learning Outcomes using Design Thinking Strategies. Honor thesis, University of Texas at Austin
*Yalçın V, Erden Ş(2021) The effect of STEM activities prepared according to the design thinking model on preschool children’s creativity and problem-solving skills. Thinking Skills Creativ 41:100864. https://doi.org/10.1016/j.tsc.2021.100864
Yu Q, Yu K (2023) Knowledge Sharing Behavior of Team Members in Blended Team-Based Learning: Moderating of Team Learning Ability. Asia-Pac Educ Res 1–13. https://doi.org/10.1007/s40299-023-00795-1
Yu Q, Yu K, Li B, Wang Q (2023) Effectiveness of blended learning on students’ learning performance: a meta-analysis. J Res Technol Educ 1–22. https://doi.org/10.1080/15391523.2023.2264984
*Ziadat AH, Sakarneh MA (2021) Online design thinking problems for enhancing motivation of gifted students. Int J Learn Teach Educ Res 20(8):91–107. https://doi.org/10.26803/ijlter.20.8.6
Download references
Acknowledgements
We are very grateful to the editor and reviewers for their constructive comments and hard work. We would also like to express our gratitude to Springer Nature.
Author information
Authors and affiliations.
Fudan University, Shanghai, China
Qing Yu, Kun Yu & Rongri Lin
You can also search for this author in PubMed Google Scholar
Contributions
Qing Yu and Kun Yu: conceptualization, data curation and analysis, investigation, methodology, validation, and writing-review & editing. Qing Yu: writing-original draft, project administration, and resources. Rongri Lin: investigation, validation, resources, and writing-review & editing.
Corresponding authors
Correspondence to Qing Yu , Kun Yu or Rongri Lin .
Ethics declarations
Competing interests.
The authors declare no competing interests.
Ethical approval
This article does not contain any studies with human participants performed by any of the authors.
Informed consent
Additional information.
Publisher’s note Springer Nature remains neutral with regard to jurisdictional claims in published maps and institutional affiliations.
Rights and permissions
Open Access This article is licensed under a Creative Commons Attribution 4.0 International License, which permits use, sharing, adaptation, distribution and reproduction in any medium or format, as long as you give appropriate credit to the original author(s) and the source, provide a link to the Creative Commons licence, and indicate if changes were made. The images or other third party material in this article are included in the article’s Creative Commons licence, unless indicated otherwise in a credit line to the material. If material is not included in the article’s Creative Commons licence and your intended use is not permitted by statutory regulation or exceeds the permitted use, you will need to obtain permission directly from the copyright holder. To view a copy of this licence, visit http://creativecommons.org/licenses/by/4.0/ .
Reprints and permissions
About this article
Cite this article.
Yu, Q., Yu, K. & Lin, R. A meta-analysis of the effects of design thinking on student learning. Humanit Soc Sci Commun 11 , 742 (2024). https://doi.org/10.1057/s41599-024-03237-5
Download citation
Received : 26 January 2024
Accepted : 23 May 2024
Published : 10 June 2024
DOI : https://doi.org/10.1057/s41599-024-03237-5
Share this article
Anyone you share the following link with will be able to read this content:
Sorry, a shareable link is not currently available for this article.
Provided by the Springer Nature SharedIt content-sharing initiative
Quick links
- Explore articles by subject
- Guide to authors
- Editorial policies

- Introduction
- Conclusions
- Article Information
The black boxes represent point estimates, and the areas of the boxes are proportional to the weight of the studies. The weights displayed are based on bayesian analysis with vague priors. The whiskers represent CIs. Width of the diamonds represents the trials’ pooled estimate CI, and the middle point represents the point estimates.
ªCredible intervals are presented for bayesian analysis.
The black boxes represent point estimates, and the whiskers represent the pooled estimate CrIs from bayesian analysis. CrI indicates credible interval; ICU, intensive care unit.
ªCIs are presented for frequentist analysis.
A, The cumulative posterior distribution of the estimated RR, with the y-axis corresponding to the probability the RR is less than or equal to the value on the x-axis. The blue-gray area indicates a beneficial intervention (ie, RR lower than 1). The dashed vertical line indicates the median. B, The full posterior distribution of the estimated RR, with the dashed vertical line indicating the median value and the area highlighted in tan indicating the percentile-based 95% credible interval. The orange area is related to an RR greater than 1 (ie, the intervention is associated with higher mortality vs standard care). The dotted line at an RR of 1 indicates no treatment effect. The figure demonstrates that the probability that prolonged infusions of β-lactam antibiotics is associated with a reduced risk of all-cause 90-day mortality (to any extent) compared with intermittent infusions is more than 99%.
eAppendix 1. Study Protocol
eAppendix 2. Electronic Search Strategy
eAppendix 3. Semi-Informative Priors for Heterogeneity Parameter Details
eFigure 1. Preferred Reporting Items for Systematic Reviews and Meta-Analyses (PRISMA) Flowchart of Search Strategy and Included Studies
eTable 1. Excluded Reports and Reasons for Exclusion
eTable 2. Microbiological Characteristics and Beta-Lactam Antibiotic Dosing Regimen Details of Included Randomized Controlled Trials
eTable 3. Definition of Primary and Secondary Outcomes in Studies
eTable 4. Unpublished Outcome Data Obtained From Study Authors
eFigure 2. Risk of Bias Assessments
eTable 5. Additional Outcome Statistics for the Primary Bayesian Model, Sensitivity Analyses, and Secondary Outcomes
eFigure 3. Funnel Plots
eFigure 4. All-Cause 90-Day Mortality by Study Beta-Lactam Antibiotic i.e. Piperacillin/Tazobactam Versus Meropenem
eFigure 5. All-Cause 90-Day Mortality by Culture-Positive Infection Versus Culture-Negative Infection
eFigure 6. All-Cause 90-Day Mortality by Gram-Negative Infection Versus Gram-Positive Infection
eFigure 7. All-Cause 90-Day Mortality by Receipt of Kidney Replacement Therapy Versus No Kidney Replacement Therapy
eFigure 8. All-Cause 90-Day Mortality by Lung Infection Versus Other Infections
eFigure 9. All-Cause 90-Day Mortality by Sepsis Versus Septic Shock
eFigure 10. All-Cause 90-Day Mortality by Sex i.e. Male Versus Female Participants
eFigure 11. Forest Plot for ICU Mortality for the Comparison Between Prolonged Infusions of Beta-Lactam Antibiotics Versus Standard Intermittent Infusions
eFigure 12. Forest Plot for Clinical Cure for the Comparison Between Prolonged Infusions of Beta-Lactam Antibiotics Versus Standard Intermittent Infusions
eFigure 13. Forest Plot for Microbiological Cure for the Comparison Between Prolonged Infusions of Beta-Lactam Antibiotics Versus Standard Intermittent Infusions
eFigure 14. Forest Plot for Adverse Events for the Comparison Between Prolonged Infusions of Beta-Lactam Antibiotics Versus Standard Intermittent Infusions
eFigure 15. Forest Plot for ICU Length of Stay for the Comparison Between Prolonged Infusions of Beta-Lactam Antibiotics Versus Standard Intermittent Infusions
Data Sharing Statement
- Selective Decontamination of the Digestive Tract and Hospital Mortality in Critically Ill Patients JAMA Original Investigation November 15, 2022 This randomized clinical trial assesses the effect of selective decontamination of the digestive tract compared with standard care on in-hospital mortality among critically ill patients receiving mechanical ventilation. The SuDDICU Investigators for the Australian and New Zealand Intensive Care Society Clinical Trials Group; Catherine Boschert; Emma Broadfield; Timothy Chimunda; Jason Fletcher; Cameron Knott; Sanjay Porwal; Julie Smith; Deepak Bhonagiri; Monique Leijten; Sandhya Narayan; David Sanchez; Peta Saunders; Carli Sherriff; Jonathan Barrett; Gabrielle Hanlon; Sarah Jelly-Butterworth; Julie O'Donnell; Judith Watson; Shailesh Bihari; Julia Brown; Sharon Comerford; Russell Laver; JoAnne McIntyre; Tapaswi Shrestha; Jin Xia; Samantha Bates; Gerard Fennessy; Craig French; Sathyajith Kootayi; Fiona Marshall; Rebecca McEldrew; Forbes McGain; Rebecca Morgan; John Mulder; Anna Tippett; Miriam Towns; Ellie Barker; Shelley Donovan; Katrina Ellis; Atul Gaur; Hannah Gibbons; Rebecca Gregory; Eloise Hair; Mary Keehan; Jess Naumoff; Elisha Turner; Gail Brinkerhoff; Dustin Bush; Federica Cazzola; Ken Havill; Paul Healey; Amber Poulter; Krishna Sunkara; Anders Aneman; Rachel Choit; Kelsey Dobell-Brown; Kairui Guo; Jillian Lee; Lien Lombardo; Zachariah Manalil; Jennene Miller; Jordan Rogers; Antony Stewart; Jana Yanga; Rebecca Gresham; Julie Lowrey; Kristy Masters; Christina Whitehead; Beverly Zaratan; Matthew Grigg; Meg Harward; Cassie Jones; Josephine Mackay; Jason Meyer; Emma Saylor; Ellen Venz; James Walsham; Krista Wetzig; Nerissa Brown; Marianne Chapman; Kathleen Glasby; Samuel Gluck; Tejaswini Murthy; Stephanie O'Connor; Eamon Raith; Justine Rivett; Joannies Yap; Angela Ashelford; Frances Bass; Emily Fitzgerald; Oliver Flower; Bernard Hudson; Pierre Janin; Elizabeth Limbrey; Sharon Mar; Anne O'Connor; Melissa Owen; Naomi Pallas; Julia Pilowsky; Veronica Roach; Elizabeth Ruse; Wade Stedman; Miyuki Tokumitsu; Elizabeth Yarad; Deborah Inskip; Theresa Jacques; Adeline Kintono; Catherine Milner; Rebecca Sidoli; Catherine Kurenda; Sandra Peake; Patricia Williams; Jeremy Cohen; Amanda Davie; Amy Owens; Roslyn Purcell; Balasubramanian Venkatesh; Cartan Costello; Alan Davey-Quinn; Michael Davies; Ahmed Elgendy; Wenli Geng; Veerendra Jagarlamudi; Matthew Mac Partlin; Mahadev Patil; Adam Purdon; Martin Sterba; Andrea Marshall; Anthony Delaney; Marwa Abdel-All; Hayley Clark; Natalie Espinosa; Benjamin Finfer; Miranda Hardie; Dijlah Moungatonga; Conrad Nangla; Fiona Osbourne; Tina Schneider; Prakriti Shrestha; Elizabeth Wilson; Isabella Schoeler; Manuela Armenis; Dominic Byrne; Amrutha Nagarajaiah; Prakesh Velappan; Parisa Glass; Kate Myburgh; Philippa Smith; Martina Bachmaier; Daryll Knowles; Michael Tattersall; John A. Myburgh, MD, PhD; Ian M. Seppelt, MD; Fiona Goodman, BN; Laurent Billot, MSc; Maryam Correa, PhD; Joshua S. Davis, MD, PhD; Anthony C. Gordon, MD; Naomi E. Hammond, PhD; Jon Iredell, MD, PhD; Qiang Li, MBiostat; Sharon Micallef, BN; Jennene Miller, BN; Jayanthi Mysore, MS; Colman Taylor, PhD; Paul J. Young, MD, PhD; Brian H. Cuthbertson, MD; Simon R. Finfer, MD
- Continuous vs Intermittent β-Lactam Antibiotic Infusions in Critically Ill Patients With Sepsis JAMA Original Investigation June 12, 2024 This clinical trial compares the efficacy of continuous vs intermittent infusion of a β-lactam antibiotic (piperacillin-tazobactam or meropenem) in decreasing all-cause mortality at 90 days in critically ill patients with sepsis. Joel M. Dulhunty, MD, PhD; Stephen J. Brett, MD; Jan J. De Waele, MD, PhD; Dorrilyn Rajbhandari, PGDip(Clinical Nursing); Laurent Billot, MRes; Menino O. Cotta, PhD; Joshua S. Davis, MD, PhD; Simon Finfer, MD; Naomi E. Hammond, RN, PhD; Serena Knowles, RN, PhD; Xiaoqiu Liu, PhD; Shay McGuinness, MD; Jayanthi Mysore, MS; David L. Paterson, MD, PhD; Sandra Peake, MD, PhD; Andrew Rhodes, MD, MD(Res); Jason A. Roberts, BPharm, PhD; Claire Roger, MD, PhD; Charudatt Shirwadkar, MD; Therese Starr, RN; Colman Taylor, PhD; John A. Myburgh, MD, PhD; Jeffrey Lipman, MD, DMed(Res); BLING III Study Investigators; Rinaldo Bellomo; Glenn Eastwood; Leah Peck; Helen Young; Andrew Cheng; Luke Lau; Magdalena Luciuk; Margaret Muteitha; Manoj Saxena; Catherine Boschert; Timothy Chimunda; Cameron Knott; Julie Smith; Kiran Nand; Treena Sara; Peter Chan; Graeme Duke; John Dyett; Kym Gellie; Stephanie Hunter; Melissa Kaufman; Owen Roodenburg; Joseph Veto; Julia Affleck; Mahesh Ramanan; Megan Ratcliffe; Alexis Tabah; Timothy Warhurst; Maimoonbe Gough; Maree Houbert; Julie Pitman; Mandy Tallott; James Winearls; Shelley Donovan; Katrina Ellis; Atul Gaur; Mary Keehan; Amy Kelty; Elisha Turner; Cynthia Bierl; Gail Brinkerhoff; Dustin Bush; Amber-Louise Poulter; Krishna Sunkara; Melanie Cooper; Lynette Morrison; Sunil Sane; Joanne Sutton; Hayden White; Vishwanath Biradar; Milind Sanap; Natalie Soar; Megan Davis; Meg Harward; Cassie Jones; Josie Mackay; Niki McGuinness; Jason Meye; Emma Saylor; Adam Suliman; Alex Tabah; Ellen Venz; James Walsham; Krista Wetzig; Catherine Kurenda; Patricia Williams; Stuart Baker; Maree Duroux; Kylie Jacobs; Hamish Pollock; Sam Shone; Jenie Butler; Cheryl Fourie; Marianne Kirrane; Melissa Lassig-Smith; Daisy Lindsay; Amelia Livermore; Bree-Yana McConnochie; Lynn Murray; Georgina Peacock; Janine Stuart; Sidharth Agarwal; Margaret Phillips; Kirsty Smyth; David Cooper; Rick McAllister; Yasmine Ali Abdelhamid; Deborah Barge; Kathleen Byrne; Adam Deane; Sarah Doherty; Simon Iles; Brie Jelbart; Thomas Rechnitzer; Angela Ashelford; Frances Bass; Celia Bradford; Anthony Delaney; Jonathan Gatward; Roger Harris; Pierre Janin; Richard Lee; Elizabeth Limbrey; Sharon Mar; Naomi Pallas; Julia Pilowsky; Melanie Ross; Wade Stedman; Miyuki Tokumitsu; Elizabeth Yarad; Maryam Alborzi; Heidi Buhr; Jennifer Coles; David Gattas; Deborah Inskip; Asako Ito; Jennene Miller; Claire Reynolds; Sam Rudham; Alison Bone; Claire Cattigan; Tania Elderkin; Tania Salerno; Jeremy Cohen; Amanda Davie; Amy Owens; Roslyn Purcell; Balasubramanian Venkatesh; David Bowen; Peter Clark; Mohammad Hamidi; Jenyfer Joy; Jing Kong; Vineet Nayyar; Kaatje Cruysberghs; Philippe Jorens; Petra Vertongen; Marc Diltoer; Marie Claire Van Malderen; Anne Joosten; Maxime Van Cutsem; Caroline Abbenhuijs; Dominique Durand; Nancy Matumikina; Fabio Taccone; Stephanie Bracke; Lesley De Coster; Luc De Crop; Anouska De Smeytere; Jolien Van Hecke; Daisy Vermeiren; Jan Heerman; Steffi Ryckaert; Annelies Van Damme ; Nicolas De Schryver; Anne Thirifays; Els Fannes; Niels Van Regenmortel; Corentine Alauzet; Thibaut Belveyre; Jessica Breistroff; Aurélie Gutehrle; Emmanuel Novy; Maximilien Saint Gilles; Marie Bonnefoy; Audrey Clavier; Pierre Ducq; Antoine Frouin; Sébastien Moschietto; Marion Touzet; Claire Dahyot-Fizelier; Angéline Jamet; Angela Kostencovska; Quentin Saint Genis; Sami Alaya; Ali Mofredj; Caroline Boutin; Loubna Elotmani; Martin Mahul; Laurent Muller; Nicolas Perin; Sanihah Che Omar; Suki Ismet; Noor Iellieya Maisarah Jamaludin; Md Fadhil Mat Amin; Mohd Zulfakar Mazlan; Husna Mohd Nor; Nabila Mohd Sukri; Nurul Amira Nizar; Mahamarowi Omar; Era Nabilah Roslan; Zeti Norfidiyati Salmuna; Tuan Zulfadli Syazwan Tuan Mohd Adnan; W Mohd Nazarudin W Hassan; Halimatun Arwa Yaakub; Mohd Shahnaz Hasan; Marhainie Mohamad; Mohamad Irfan Othman Jailani; Helmi Sulaiman; Farisha Ali; Magdalena Butler; Keri-Anne Cowdrey; Eileen Gilder; Jane Hallion; Immanuel Hennessy; Philippa Neal; Karina O’Connor; Rachael Parke; Samantha Ryan; Melissa Woollett (now Robertson); Yan Chen; Colin McArthur; Rachael McConnochie; Caroline O’Connor; Catherine Simmonds; Brandon Burke; Tara Doyle; Jan Mehrtens; Stacey Morgan; Anna Morris; Kym Van Der Heyden; Dinu Girijadevi; Vivian Lai; Rima Song; Tony Williams; Amelia Butler; Caitriona Fiske; Annette Forrest; Erin Furborough; Robert Martynoga; Renesh Nair; Sarah Rogers; Livia Schischka; Kara Trask; April Aguilar; Colin Barnes; Ben Barry; Niña Beehre; Kirsha Delaney; Dick Dinsdale; Kim Grayson; Deb Hendry; Anna Hunt; April Jane; Harriet Judd; Charlotte Latimer-Bell; Cassie Lawrence; Eden Lesona; Rosie Martin; Agnes Mckay Vucago; Alex Millington; James Moore; Leanlove Navarra; Shaanti Olatunji; Alex Psirides; Yve Robertson; Hannah Smellie; Rhoze Sol Cruz; Raulle Sol Cuz; Shawn Sturland; Kate Tietjens; Bob Ure; Lawrence Walker; Jason Wright; Chelsea Young; Sara Andertun; Martin Annborn; Maria Nelderup ; Lisa Boström; Susann Schrey; Marina Larsson; Fredrik Sjövall ; Maria Alvarez Corral; Anthony Ashton; Nicholas J Cortes ; Denise Griffin; Nycola Muchenje; McDonald Mupudzi; Dawn Trodd; Leonie Benham; Zena Bradshaw; Gemma Brown; Janette Brown; Jason Cupitt; Lisa Elawamy; Alan Fahey; James Glen; Shamina Hankinson; Gareth Hardy; Jazmine McCooey; Sam Remnant; Ella Riedel; Emma Stoddard; Bhagawathi Suryanarayanan; Lauren Thornborough; Emma Ward; Scott Warden; Conor Wilkinson; Jeremy Bewley; Anna Chillingworth; Georgia Efford; Zoe Garland; Lisa Grimmer; Rebekah Johnson; Katie Sweet; Denise Webster; Kim Kim Wright; Mandy Austin; Vallish Bhardwaj ; Stacey Cotterell ; Fiona McNeela; Victoria Mead ; Lauren Perkins; Jayachandran Radhakrishnan ; Sue Smolen ; Christopher Westall; David Antcliffe; Ziortza Fernandez; Eleanor Jepson; Roceld Rojo; Anita Tamang Gurung; Maie Templeton; Delores Beach; Melanie Colegrove; Maria Faulkner; Laura McKay; Jos Wickett; Laurence Wilson; Zakaulla Belagodu; Ryan Coe; Katy Collins; Charlotte Kamundi; Naomi Oakley; Carmel Stuart; Nikitas Nikitas; Ana Sanchez-Rodriguez; Kayleigh Spencer; Liana Stapleton; Bethan Stowe; Colin Wells; Sarah Doyle ; Stephanie Dukes ; Sarah Horton ; James Rees ; Mark Shortland ; Ruth Thomas ; Sophie Webber ; Trish Williams ; Lauren Butler; Leigh Dunn; Arti Gulati; Jonathan Shelton; Yvonne Bland; Lianne Chapman; Rozzie Dolman; George Evetts; Danni Greener; Istvan Kajtor; Stephen Lewis; Cat Lumby; Helen Rayner; Susanne Cathcart; Pamela MacTavish; Kathryn Puxty; Christine Aitken; Elizabeth Boyd ; Julie Buckley ; Charlene Hamilton; Ilkin Kasmani ; Thomas Keast; Phil McCall; Ruth McLaren; Ben Shelley ; Sonia Sousa Arias; Jenny Wong; Susan Anderson; Eleanor Andrews ; Janine Birch; Emma Collins; Mark Forrest; Surpreet Grewal; Kate Hammerton; Andrew Holland; Robin Jootun; Ryan O’Leary; Kyaw Pyae Soan; Carl Stevenson; Samantha Summers; Laura Troth; Louise Foster; Andrew Gratrix; Matthew Hines; Victoria Martinson; Vanessa Rivers; Elizabeth Stones; Sarah Bartholomew; Stephanie Bell; Kate Turner; Karen Winter; Uwe Franke; Evie Headlam; Keith Hugill; Jessica Jones; Abigail List; Joanne Morley; Tracy Ruddick; Amy Thompson; Nikkita Adams ; Emma Clarey ; Maeve Cockrell ; Ele Corcoran; Maria Depante; Clare Finney; Aparna George ; Philip Hopkins; Nauman Hussain; Reena Mehta; Kevin O'Reilly; Mandeep Phull ; John Smith; Mandy Gill; Jill Kirk; Pulak Paul; Sarah Shelton; Hanan Abdullise; Samantha Bacciarelli; Julia Balcioglu; Isabel Bradley; Sabrina Carta; Rebecca Crowe; Marion Gillespie; Margaret Grout; Rosaleen Herdman-Grant; Olumayowa Ogunwemimo; Nicole Richard; Kathryn Sollesta; Andrew Swain; Joan Willis; Miriam Davey; Rebecca Seaman; James Wood; Iram Ahmed; Rebecca Collins; Tessa Glazebrook; Paul Hayden; Thyra Khyere Diaborr; Robert Musalagani; Linda Ofori; Vonganyi Ogbeide; Sandra Sowah; Rebecca Squires; Louise Mew; James Pocock; Richard Stewart; Sara Beth Sutherland; Pauline Austin ; Louise Cabrelli; Matthew Casey; Susan Chapman ; Hayley McKie; Jessica Reynolds ; Tracy Smith; Gail Waddell ; Jack Wilkinson; Bryan Yates; Ali Alayan ; Andrew Ashcroft; Sarah Buckley ; Elizabeth Denis; Lucy Gurr; Amy Major; Alex Metcalfe ; Alice Poole ; Alistair Rose ; Brendan Sloan ; Sharwend Supermanian ; Nicola Thompson; Katie Wilson ; Julie Camsooksai ; Yasmin De’Ath; Sarah Jenkins; Emma Langridge; Rebecca Miln; Sarah Patch; Magda Pomichowska; Henrik Reschreiter; Suzanne Roffe ; Sarah Savage; Harriet Noble; Helen Claridge; Aimi Collins; Zoe Daly; Dovy Domingo; James McNicholas; Angie Nown; Steve Rose; Mansoor Bangash; Colin Bergin; Ronald Carrera; Amy Clark; Lauren Cooper; Natalie Dooley; Karen Ellis; Emma Fellows; Stephanie Goundry; Samantha Harkett; Saffron King; Tracy Mason; Aoife Neal; Alex Newton-Cox; Dhruv Parekh; Jaimin Patel ; Christopher Sheridan; Hazel Smith; Elaine Spruce; Sam Bosompem; Parveen Dugh; Mariha Khalid; Lace Paulyn Rosaroso; Mandeep K Phull ; Tatiana Pogreban; Saima Ashraf; Marc Chikhani; Louise Conner; Martin Dent; Daniel Harvey; Treesa Joseph; Megan Meredith; Lucy Morris; Cecilia Peters; Lucy Ryan; Julia Sampson; Anju Thomas; Catherine Walton; Shauna Bartley; Parminder Bhuie; Sabi Gurung Rai; Nicola Jacques; Andrew Walden; Madhu Balasubramaniam; Hugo Buckley; Scott Latham; Emily Bevan; Arthur Goldsmith; Irina Grecu; Jane Martin; Simon Williams; Caroline Wrey Brown; Amaia Arrieta; Maria Fernandez; Alex Fowler; Ryan Haines; Mareena Joseph; Tim Martin; Filipa Santos; Fatima Seidu; Ruzena Uddin; Parjam Zolfaghari; Claudio Addari; Sekina Bakare; Lauren Barraclough; Thomas Bemand; Ethel Black; Matt Clayton; Arnold Dela Rosa; Luke Edwards; Hugh Furness; Richard Gordon-Williams; Nicole Greenshields; Natalie Hester; Francesca Holden; Ryan Howle; Emma Hunt; Aatif Husain; Sultan Iqbal; Venkateswaran Jambunathan; Shaman Jhanji; Ahalya Kadirvelarasan; Harriet Kemp; Suzannah Lant; Sarah Loftus; Sam Maese; Laura Matthews; Olivia Morley; Kshiteeja Naik; Isabel Norris; Nicola Ocean; Luke Parker; Amee Patel; Shivali Patel; Ravishankar Raobaikady; Martin Shao Foong Chong; William Sherwood; Sam Smith; Prakhar Srilastava; Sharjeel Tahir; Shamil Tanna; Kate Tatham; Benjamin Thomas; Pete Thomas; Shree Voralia; Kritchai Vutipongsatorn; Zoszka Webb; Tanith Westerman; Alasdair Wills; Hafsana Ali; Michele Bossy; Naomi Boyer; Letitia Bullman; Dorota Burda; Paula Carveli; Sandra Chmielewska; Daniel Cottam; Nichalus Dawson; Jeanny Del Socorro; Lui Forni; James Hilton; Sherin John; Justin Kirk-Bayley; Donna May Sanga; Kathryn Mcintosh; Jame McKinley; Rugaia Montaser; Tahir Nasser; Razan Nour; Elizabeth Poter; Stephanie Rouse; Armorel Salberg; Joe Tooley; Emma Tyson; Jerik Verula; Eva Wilson; Waldtraud Wutte; Emma Boxall ; Kathryn Cawley; Joy Dearden; Reece Doonan; John MacDonald; Danielle McLaughlan; Alice Baker; Clare Bolger; Rachel Burnish; Michael Carter; Rebecca Cusack; Susan Jackson; Karen Salmon; Lijun Ding; Sarah Farnell Ward; Helen Farrah; Geraldine Gray; Pamela Kabungo; Susannah Leaver; Romina Pepermans Saluzzio; Christine Sicat; Maria Thanasi; Vince Ventura; Nikki Yun; Dorota Banach; Anthony Gordon; Amal Mohammed; Ehsan Ahmadnia; Mike Barker; Aneta Bociek; Lynda Cameron; Fabiola D’Amato; Fraser Hanks; Sarah Khorshid; Rosario Lim; Shelley Lorah; Kyma Morera; Marlies Ostermann; Gill Radcliffe; Chris Remmington; Natasha Thaladi; Duncan Wyncoll; Judith Abrams; Oliver Dare; Geraldine Hambrook; Katarina Manso; Elizabeth McKerrow; Pradeep Shanmugasundaram; Sarah Cornell; Alistair Roy; Lindsey Woods; Hayley Dolan; Thomas Billyward; Pamela Bremmer; Carl Hawkins; Sophie Jackson; Geraldine Ward; Michele Clark; Vijay Jagannathan; Sarah Purvis; Pam Race; Thomas Robb; Simon Sinclair; Jenny Brooks; Jade Cole; Michelle Davies; Rhys Davies; Helen Hill; Matt Morgan; Craig Spencer; Emma Thomas; Angharad Williams; Chiara Ellis; Elvira Hoxha; Valerie Page; Xiaobei Zhao; Nick Ivin; Rachel Johnston; Louise Ma; Anezka Pratley; Aine Ruttledge; Kathryn Simpson; Greg Barton; Anthony Cochrane; Clare Harrop; Chelcie Jewitt; Charlotte Preston; Karen Shuker
- Resolving the Dilemma on Continuous vs Intermittent β-Lactam Antibiotics in Sepsis JAMA Editorial June 12, 2024 W. Joost Wiersinga, MD, PhD, MBA; Michiel A. van Agtmael, MD, PhD
See More About
Select your interests.
Customize your JAMA Network experience by selecting one or more topics from the list below.
- Academic Medicine
- Acid Base, Electrolytes, Fluids
- Allergy and Clinical Immunology
- American Indian or Alaska Natives
- Anesthesiology
- Anticoagulation
- Art and Images in Psychiatry
- Artificial Intelligence
- Assisted Reproduction
- Bleeding and Transfusion
- Caring for the Critically Ill Patient
- Challenges in Clinical Electrocardiography
- Climate and Health
- Climate Change
- Clinical Challenge
- Clinical Decision Support
- Clinical Implications of Basic Neuroscience
- Clinical Pharmacy and Pharmacology
- Complementary and Alternative Medicine
- Consensus Statements
- Coronavirus (COVID-19)
- Critical Care Medicine
- Cultural Competency
- Dental Medicine
- Dermatology
- Diabetes and Endocrinology
- Diagnostic Test Interpretation
- Drug Development
- Electronic Health Records
- Emergency Medicine
- End of Life, Hospice, Palliative Care
- Environmental Health
- Equity, Diversity, and Inclusion
- Facial Plastic Surgery
- Gastroenterology and Hepatology
- Genetics and Genomics
- Genomics and Precision Health
- Global Health
- Guide to Statistics and Methods
- Hair Disorders
- Health Care Delivery Models
- Health Care Economics, Insurance, Payment
- Health Care Quality
- Health Care Reform
- Health Care Safety
- Health Care Workforce
- Health Disparities
- Health Inequities
- Health Policy
- Health Systems Science
- History of Medicine
- Hypertension
- Images in Neurology
- Implementation Science
- Infectious Diseases
- Innovations in Health Care Delivery
- JAMA Infographic
- Law and Medicine
- Leading Change
- Less is More
- LGBTQIA Medicine
- Lifestyle Behaviors
- Medical Coding
- Medical Devices and Equipment
- Medical Education
- Medical Education and Training
- Medical Journals and Publishing
- Mobile Health and Telemedicine
- Narrative Medicine
- Neuroscience and Psychiatry
- Notable Notes
- Nutrition, Obesity, Exercise
- Obstetrics and Gynecology
- Occupational Health
- Ophthalmology
- Orthopedics
- Otolaryngology
- Pain Medicine
- Palliative Care
- Pathology and Laboratory Medicine
- Patient Care
- Patient Information
- Performance Improvement
- Performance Measures
- Perioperative Care and Consultation
- Pharmacoeconomics
- Pharmacoepidemiology
- Pharmacogenetics
- Pharmacy and Clinical Pharmacology
- Physical Medicine and Rehabilitation
- Physical Therapy
- Physician Leadership
- Population Health
- Primary Care
- Professional Well-being
- Professionalism
- Psychiatry and Behavioral Health
- Public Health
- Pulmonary Medicine
- Regulatory Agencies
- Reproductive Health
- Research, Methods, Statistics
- Resuscitation
- Rheumatology
- Risk Management
- Scientific Discovery and the Future of Medicine
- Shared Decision Making and Communication
- Sleep Medicine
- Sports Medicine
- Stem Cell Transplantation
- Substance Use and Addiction Medicine
- Surgical Innovation
- Surgical Pearls
- Teachable Moment
- Technology and Finance
- The Art of JAMA
- The Arts and Medicine
- The Rational Clinical Examination
- Tobacco and e-Cigarettes
- Translational Medicine
- Trauma and Injury
- Treatment Adherence
- Ultrasonography
- Users' Guide to the Medical Literature
- Vaccination
- Venous Thromboembolism
- Veterans Health
- Women's Health
- Workflow and Process
- Wound Care, Infection, Healing
Others Also Liked
- Download PDF
- X Facebook More LinkedIn
Abdul-Aziz MH , Hammond NE , Brett SJ, et al. Prolonged vs Intermittent Infusions of β-Lactam Antibiotics in Adults With Sepsis or Septic Shock : A Systematic Review and Meta-Analysis . JAMA. Published online June 12, 2024. doi:10.1001/jama.2024.9803
Manage citations:
© 2024
- Permissions
Prolonged vs Intermittent Infusions of β-Lactam Antibiotics in Adults With Sepsis or Septic Shock : A Systematic Review and Meta-Analysis
- 1 University of Queensland Centre for Clinical Research (UQCCR), Faculty of Medicine, The University of Queensland, Brisbane, Queensland, Australia
- 2 Critical Care Program, The George Institute for Global Health and University of New South Wales, Sydney, New South Wales, Australia
- 3 Malcolm Fisher Department of Intensive Care, Royal North Shore Hospital, Sydney, New South Wales, Australia
- 4 Department of Surgery and Cancer, Imperial College, London, United Kingdom
- 5 Department of Intensive Care Medicine, Ghent University Hospital, Ghent, Belgium
- 6 Statistics Division, The George Institute for Global Health and University of New South Wales, Sydney, New South Wales, Australia
- 7 Department of Business Economics, Health and Social Care, University of Applied Sciences and Arts of Southern Switzerland, Manno, Switzerland
- 8 Department of Clinical Research, University of Bern, Bern, Switzerland
- 9 Department of Intensive Care Medicine, Royal Brisbane and Women’s Hospital, Brisbane, Queensland, Australia
- 10 Redcliffe Hospital, Redcliffe, Queensland, Australia
- 11 Faculty of Medicine, The University of Queensland, Brisbane, Queensland, Australia
- 12 Department of Intensive Care Medicine, Westmead Hospital, Sydney, New South Wales, Australia
- 13 UQ Library, The University of Queensland, Brisbane, Queensland, Australia
- 14 Department of Anesthesiology, Faculty of Medicine, University Malaya, Kuala Lumpur, Malaysia
- 15 Division of Critical Care, University of Witwatersrand, Chris Hani Baragwanath Academic Hospital, Johannesburg, South Africa
- 16 Jamieson Trauma Institute, Royal Brisbane and Women’s Hospital, Brisbane, Queensland, Australia
- 17 Division of Anesthesiology Critical Care Emergency and Pain Medicine, Nîmes University Hospital, University of Montpellier, Nîmes, France
- 18 School of Population Health, University of New South Wales, Sydney, New South Wales, Australia
- 19 Department of Anesthesia and Intensive Care, IRCCS San Raffaele Scientific Institute, Milan, Italy
- 20 Vita-Salute San Raffaele University, Milan, Italy
- 21 Department of Intensive Care, St George Hospital, Kogarah, New South Wales, Australia
- 22 Service d’anesthésie-réanimation et médicine péri-opératoire Brabois adulte, CHRU de Nancy, Nancy, France
- 23 Université de Lorraine, SIMPA, Nancy, France
- 24 Département d’anesthésie et réanimation, douleur et médecine d’urgence, CHU Carémeau, Nîmes, France
- 25 UR UM 103IMAGINE, Faculté de Médecine, Montpellier Université, Nîmes, France
- 26 Intensive and Perioperative Care, Skåne University Hospital, Malmö, Sweden
- 27 Department of Clinical Sciences, Lund University, Lund, Sweden
- 28 Department of Diagnostic and Experimental Medicine, University of Bologna, Bologna, Italy
- 29 Herston Infectious Diseases Institute (HeIDI), Metro North Health, Brisbane, Queensland, Australia
- Editorial Resolving the Dilemma on Continuous vs Intermittent β-Lactam Antibiotics in Sepsis W. Joost Wiersinga, MD, PhD, MBA; Michiel A. van Agtmael, MD, PhD JAMA
- Original Investigation Selective Decontamination of the Digestive Tract and Hospital Mortality in Critically Ill Patients The SuDDICU Investigators for the Australian and New Zealand Intensive Care Society Clinical Trials Group; Catherine Boschert; Emma Broadfield; Timothy Chimunda; Jason Fletcher; Cameron Knott; Sanjay Porwal; Julie Smith; Deepak Bhonagiri; Monique Leijten; Sandhya Narayan; David Sanchez; Peta Saunders; Carli Sherriff; Jonathan Barrett; Gabrielle Hanlon; Sarah Jelly-Butterworth; Julie O'Donnell; Judith Watson; Shailesh Bihari; Julia Brown; Sharon Comerford; Russell Laver; JoAnne McIntyre; Tapaswi Shrestha; Jin Xia; Samantha Bates; Gerard Fennessy; Craig French; Sathyajith Kootayi; Fiona Marshall; Rebecca McEldrew; Forbes McGain; Rebecca Morgan; John Mulder; Anna Tippett; Miriam Towns; Ellie Barker; Shelley Donovan; Katrina Ellis; Atul Gaur; Hannah Gibbons; Rebecca Gregory; Eloise Hair; Mary Keehan; Jess Naumoff; Elisha Turner; Gail Brinkerhoff; Dustin Bush; Federica Cazzola; Ken Havill; Paul Healey; Amber Poulter; Krishna Sunkara; Anders Aneman; Rachel Choit; Kelsey Dobell-Brown; Kairui Guo; Jillian Lee; Lien Lombardo; Zachariah Manalil; Jennene Miller; Jordan Rogers; Antony Stewart; Jana Yanga; Rebecca Gresham; Julie Lowrey; Kristy Masters; Christina Whitehead; Beverly Zaratan; Matthew Grigg; Meg Harward; Cassie Jones; Josephine Mackay; Jason Meyer; Emma Saylor; Ellen Venz; James Walsham; Krista Wetzig; Nerissa Brown; Marianne Chapman; Kathleen Glasby; Samuel Gluck; Tejaswini Murthy; Stephanie O'Connor; Eamon Raith; Justine Rivett; Joannies Yap; Angela Ashelford; Frances Bass; Emily Fitzgerald; Oliver Flower; Bernard Hudson; Pierre Janin; Elizabeth Limbrey; Sharon Mar; Anne O'Connor; Melissa Owen; Naomi Pallas; Julia Pilowsky; Veronica Roach; Elizabeth Ruse; Wade Stedman; Miyuki Tokumitsu; Elizabeth Yarad; Deborah Inskip; Theresa Jacques; Adeline Kintono; Catherine Milner; Rebecca Sidoli; Catherine Kurenda; Sandra Peake; Patricia Williams; Jeremy Cohen; Amanda Davie; Amy Owens; Roslyn Purcell; Balasubramanian Venkatesh; Cartan Costello; Alan Davey-Quinn; Michael Davies; Ahmed Elgendy; Wenli Geng; Veerendra Jagarlamudi; Matthew Mac Partlin; Mahadev Patil; Adam Purdon; Martin Sterba; Andrea Marshall; Anthony Delaney; Marwa Abdel-All; Hayley Clark; Natalie Espinosa; Benjamin Finfer; Miranda Hardie; Dijlah Moungatonga; Conrad Nangla; Fiona Osbourne; Tina Schneider; Prakriti Shrestha; Elizabeth Wilson; Isabella Schoeler; Manuela Armenis; Dominic Byrne; Amrutha Nagarajaiah; Prakesh Velappan; Parisa Glass; Kate Myburgh; Philippa Smith; Martina Bachmaier; Daryll Knowles; Michael Tattersall; John A. Myburgh, MD, PhD; Ian M. Seppelt, MD; Fiona Goodman, BN; Laurent Billot, MSc; Maryam Correa, PhD; Joshua S. Davis, MD, PhD; Anthony C. Gordon, MD; Naomi E. Hammond, PhD; Jon Iredell, MD, PhD; Qiang Li, MBiostat; Sharon Micallef, BN; Jennene Miller, BN; Jayanthi Mysore, MS; Colman Taylor, PhD; Paul J. Young, MD, PhD; Brian H. Cuthbertson, MD; Simon R. Finfer, MD JAMA
- Original Investigation Continuous vs Intermittent β-Lactam Antibiotic Infusions in Critically Ill Patients With Sepsis Joel M. Dulhunty, MD, PhD; Stephen J. Brett, MD; Jan J. De Waele, MD, PhD; Dorrilyn Rajbhandari, PGDip(Clinical Nursing); Laurent Billot, MRes; Menino O. Cotta, PhD; Joshua S. Davis, MD, PhD; Simon Finfer, MD; Naomi E. Hammond, RN, PhD; Serena Knowles, RN, PhD; Xiaoqiu Liu, PhD; Shay McGuinness, MD; Jayanthi Mysore, MS; David L. Paterson, MD, PhD; Sandra Peake, MD, PhD; Andrew Rhodes, MD, MD(Res); Jason A. Roberts, BPharm, PhD; Claire Roger, MD, PhD; Charudatt Shirwadkar, MD; Therese Starr, RN; Colman Taylor, PhD; John A. Myburgh, MD, PhD; Jeffrey Lipman, MD, DMed(Res); BLING III Study Investigators; Rinaldo Bellomo; Glenn Eastwood; Leah Peck; Helen Young; Andrew Cheng; Luke Lau; Magdalena Luciuk; Margaret Muteitha; Manoj Saxena; Catherine Boschert; Timothy Chimunda; Cameron Knott; Julie Smith; Kiran Nand; Treena Sara; Peter Chan; Graeme Duke; John Dyett; Kym Gellie; Stephanie Hunter; Melissa Kaufman; Owen Roodenburg; Joseph Veto; Julia Affleck; Mahesh Ramanan; Megan Ratcliffe; Alexis Tabah; Timothy Warhurst; Maimoonbe Gough; Maree Houbert; Julie Pitman; Mandy Tallott; James Winearls; Shelley Donovan; Katrina Ellis; Atul Gaur; Mary Keehan; Amy Kelty; Elisha Turner; Cynthia Bierl; Gail Brinkerhoff; Dustin Bush; Amber-Louise Poulter; Krishna Sunkara; Melanie Cooper; Lynette Morrison; Sunil Sane; Joanne Sutton; Hayden White; Vishwanath Biradar; Milind Sanap; Natalie Soar; Megan Davis; Meg Harward; Cassie Jones; Josie Mackay; Niki McGuinness; Jason Meye; Emma Saylor; Adam Suliman; Alex Tabah; Ellen Venz; James Walsham; Krista Wetzig; Catherine Kurenda; Patricia Williams; Stuart Baker; Maree Duroux; Kylie Jacobs; Hamish Pollock; Sam Shone; Jenie Butler; Cheryl Fourie; Marianne Kirrane; Melissa Lassig-Smith; Daisy Lindsay; Amelia Livermore; Bree-Yana McConnochie; Lynn Murray; Georgina Peacock; Janine Stuart; Sidharth Agarwal; Margaret Phillips; Kirsty Smyth; David Cooper; Rick McAllister; Yasmine Ali Abdelhamid; Deborah Barge; Kathleen Byrne; Adam Deane; Sarah Doherty; Simon Iles; Brie Jelbart; Thomas Rechnitzer; Angela Ashelford; Frances Bass; Celia Bradford; Anthony Delaney; Jonathan Gatward; Roger Harris; Pierre Janin; Richard Lee; Elizabeth Limbrey; Sharon Mar; Naomi Pallas; Julia Pilowsky; Melanie Ross; Wade Stedman; Miyuki Tokumitsu; Elizabeth Yarad; Maryam Alborzi; Heidi Buhr; Jennifer Coles; David Gattas; Deborah Inskip; Asako Ito; Jennene Miller; Claire Reynolds; Sam Rudham; Alison Bone; Claire Cattigan; Tania Elderkin; Tania Salerno; Jeremy Cohen; Amanda Davie; Amy Owens; Roslyn Purcell; Balasubramanian Venkatesh; David Bowen; Peter Clark; Mohammad Hamidi; Jenyfer Joy; Jing Kong; Vineet Nayyar; Kaatje Cruysberghs; Philippe Jorens; Petra Vertongen; Marc Diltoer; Marie Claire Van Malderen; Anne Joosten; Maxime Van Cutsem; Caroline Abbenhuijs; Dominique Durand; Nancy Matumikina; Fabio Taccone; Stephanie Bracke; Lesley De Coster; Luc De Crop; Anouska De Smeytere; Jolien Van Hecke; Daisy Vermeiren; Jan Heerman; Steffi Ryckaert; Annelies Van Damme ; Nicolas De Schryver; Anne Thirifays; Els Fannes; Niels Van Regenmortel; Corentine Alauzet; Thibaut Belveyre; Jessica Breistroff; Aurélie Gutehrle; Emmanuel Novy; Maximilien Saint Gilles; Marie Bonnefoy; Audrey Clavier; Pierre Ducq; Antoine Frouin; Sébastien Moschietto; Marion Touzet; Claire Dahyot-Fizelier; Angéline Jamet; Angela Kostencovska; Quentin Saint Genis; Sami Alaya; Ali Mofredj; Caroline Boutin; Loubna Elotmani; Martin Mahul; Laurent Muller; Nicolas Perin; Sanihah Che Omar; Suki Ismet; Noor Iellieya Maisarah Jamaludin; Md Fadhil Mat Amin; Mohd Zulfakar Mazlan; Husna Mohd Nor; Nabila Mohd Sukri; Nurul Amira Nizar; Mahamarowi Omar; Era Nabilah Roslan; Zeti Norfidiyati Salmuna; Tuan Zulfadli Syazwan Tuan Mohd Adnan; W Mohd Nazarudin W Hassan; Halimatun Arwa Yaakub; Mohd Shahnaz Hasan; Marhainie Mohamad; Mohamad Irfan Othman Jailani; Helmi Sulaiman; Farisha Ali; Magdalena Butler; Keri-Anne Cowdrey; Eileen Gilder; Jane Hallion; Immanuel Hennessy; Philippa Neal; Karina O’Connor; Rachael Parke; Samantha Ryan; Melissa Woollett (now Robertson); Yan Chen; Colin McArthur; Rachael McConnochie; Caroline O’Connor; Catherine Simmonds; Brandon Burke; Tara Doyle; Jan Mehrtens; Stacey Morgan; Anna Morris; Kym Van Der Heyden; Dinu Girijadevi; Vivian Lai; Rima Song; Tony Williams; Amelia Butler; Caitriona Fiske; Annette Forrest; Erin Furborough; Robert Martynoga; Renesh Nair; Sarah Rogers; Livia Schischka; Kara Trask; April Aguilar; Colin Barnes; Ben Barry; Niña Beehre; Kirsha Delaney; Dick Dinsdale; Kim Grayson; Deb Hendry; Anna Hunt; April Jane; Harriet Judd; Charlotte Latimer-Bell; Cassie Lawrence; Eden Lesona; Rosie Martin; Agnes Mckay Vucago; Alex Millington; James Moore; Leanlove Navarra; Shaanti Olatunji; Alex Psirides; Yve Robertson; Hannah Smellie; Rhoze Sol Cruz; Raulle Sol Cuz; Shawn Sturland; Kate Tietjens; Bob Ure; Lawrence Walker; Jason Wright; Chelsea Young; Sara Andertun; Martin Annborn; Maria Nelderup ; Lisa Boström; Susann Schrey; Marina Larsson; Fredrik Sjövall ; Maria Alvarez Corral; Anthony Ashton; Nicholas J Cortes ; Denise Griffin; Nycola Muchenje; McDonald Mupudzi; Dawn Trodd; Leonie Benham; Zena Bradshaw; Gemma Brown; Janette Brown; Jason Cupitt; Lisa Elawamy; Alan Fahey; James Glen; Shamina Hankinson; Gareth Hardy; Jazmine McCooey; Sam Remnant; Ella Riedel; Emma Stoddard; Bhagawathi Suryanarayanan; Lauren Thornborough; Emma Ward; Scott Warden; Conor Wilkinson; Jeremy Bewley; Anna Chillingworth; Georgia Efford; Zoe Garland; Lisa Grimmer; Rebekah Johnson; Katie Sweet; Denise Webster; Kim Kim Wright; Mandy Austin; Vallish Bhardwaj ; Stacey Cotterell ; Fiona McNeela; Victoria Mead ; Lauren Perkins; Jayachandran Radhakrishnan ; Sue Smolen ; Christopher Westall; David Antcliffe; Ziortza Fernandez; Eleanor Jepson; Roceld Rojo; Anita Tamang Gurung; Maie Templeton; Delores Beach; Melanie Colegrove; Maria Faulkner; Laura McKay; Jos Wickett; Laurence Wilson; Zakaulla Belagodu; Ryan Coe; Katy Collins; Charlotte Kamundi; Naomi Oakley; Carmel Stuart; Nikitas Nikitas; Ana Sanchez-Rodriguez; Kayleigh Spencer; Liana Stapleton; Bethan Stowe; Colin Wells; Sarah Doyle ; Stephanie Dukes ; Sarah Horton ; James Rees ; Mark Shortland ; Ruth Thomas ; Sophie Webber ; Trish Williams ; Lauren Butler; Leigh Dunn; Arti Gulati; Jonathan Shelton; Yvonne Bland; Lianne Chapman; Rozzie Dolman; George Evetts; Danni Greener; Istvan Kajtor; Stephen Lewis; Cat Lumby; Helen Rayner; Susanne Cathcart; Pamela MacTavish; Kathryn Puxty; Christine Aitken; Elizabeth Boyd ; Julie Buckley ; Charlene Hamilton; Ilkin Kasmani ; Thomas Keast; Phil McCall; Ruth McLaren; Ben Shelley ; Sonia Sousa Arias; Jenny Wong; Susan Anderson; Eleanor Andrews ; Janine Birch; Emma Collins; Mark Forrest; Surpreet Grewal; Kate Hammerton; Andrew Holland; Robin Jootun; Ryan O’Leary; Kyaw Pyae Soan; Carl Stevenson; Samantha Summers; Laura Troth; Louise Foster; Andrew Gratrix; Matthew Hines; Victoria Martinson; Vanessa Rivers; Elizabeth Stones; Sarah Bartholomew; Stephanie Bell; Kate Turner; Karen Winter; Uwe Franke; Evie Headlam; Keith Hugill; Jessica Jones; Abigail List; Joanne Morley; Tracy Ruddick; Amy Thompson; Nikkita Adams ; Emma Clarey ; Maeve Cockrell ; Ele Corcoran; Maria Depante; Clare Finney; Aparna George ; Philip Hopkins; Nauman Hussain; Reena Mehta; Kevin O'Reilly; Mandeep Phull ; John Smith; Mandy Gill; Jill Kirk; Pulak Paul; Sarah Shelton; Hanan Abdullise; Samantha Bacciarelli; Julia Balcioglu; Isabel Bradley; Sabrina Carta; Rebecca Crowe; Marion Gillespie; Margaret Grout; Rosaleen Herdman-Grant; Olumayowa Ogunwemimo; Nicole Richard; Kathryn Sollesta; Andrew Swain; Joan Willis; Miriam Davey; Rebecca Seaman; James Wood; Iram Ahmed; Rebecca Collins; Tessa Glazebrook; Paul Hayden; Thyra Khyere Diaborr; Robert Musalagani; Linda Ofori; Vonganyi Ogbeide; Sandra Sowah; Rebecca Squires; Louise Mew; James Pocock; Richard Stewart; Sara Beth Sutherland; Pauline Austin ; Louise Cabrelli; Matthew Casey; Susan Chapman ; Hayley McKie; Jessica Reynolds ; Tracy Smith; Gail Waddell ; Jack Wilkinson; Bryan Yates; Ali Alayan ; Andrew Ashcroft; Sarah Buckley ; Elizabeth Denis; Lucy Gurr; Amy Major; Alex Metcalfe ; Alice Poole ; Alistair Rose ; Brendan Sloan ; Sharwend Supermanian ; Nicola Thompson; Katie Wilson ; Julie Camsooksai ; Yasmin De’Ath; Sarah Jenkins; Emma Langridge; Rebecca Miln; Sarah Patch; Magda Pomichowska; Henrik Reschreiter; Suzanne Roffe ; Sarah Savage; Harriet Noble; Helen Claridge; Aimi Collins; Zoe Daly; Dovy Domingo; James McNicholas; Angie Nown; Steve Rose; Mansoor Bangash; Colin Bergin; Ronald Carrera; Amy Clark; Lauren Cooper; Natalie Dooley; Karen Ellis; Emma Fellows; Stephanie Goundry; Samantha Harkett; Saffron King; Tracy Mason; Aoife Neal; Alex Newton-Cox; Dhruv Parekh; Jaimin Patel ; Christopher Sheridan; Hazel Smith; Elaine Spruce; Sam Bosompem; Parveen Dugh; Mariha Khalid; Lace Paulyn Rosaroso; Mandeep K Phull ; Tatiana Pogreban; Saima Ashraf; Marc Chikhani; Louise Conner; Martin Dent; Daniel Harvey; Treesa Joseph; Megan Meredith; Lucy Morris; Cecilia Peters; Lucy Ryan; Julia Sampson; Anju Thomas; Catherine Walton; Shauna Bartley; Parminder Bhuie; Sabi Gurung Rai; Nicola Jacques; Andrew Walden; Madhu Balasubramaniam; Hugo Buckley; Scott Latham; Emily Bevan; Arthur Goldsmith; Irina Grecu; Jane Martin; Simon Williams; Caroline Wrey Brown; Amaia Arrieta; Maria Fernandez; Alex Fowler; Ryan Haines; Mareena Joseph; Tim Martin; Filipa Santos; Fatima Seidu; Ruzena Uddin; Parjam Zolfaghari; Claudio Addari; Sekina Bakare; Lauren Barraclough; Thomas Bemand; Ethel Black; Matt Clayton; Arnold Dela Rosa; Luke Edwards; Hugh Furness; Richard Gordon-Williams; Nicole Greenshields; Natalie Hester; Francesca Holden; Ryan Howle; Emma Hunt; Aatif Husain; Sultan Iqbal; Venkateswaran Jambunathan; Shaman Jhanji; Ahalya Kadirvelarasan; Harriet Kemp; Suzannah Lant; Sarah Loftus; Sam Maese; Laura Matthews; Olivia Morley; Kshiteeja Naik; Isabel Norris; Nicola Ocean; Luke Parker; Amee Patel; Shivali Patel; Ravishankar Raobaikady; Martin Shao Foong Chong; William Sherwood; Sam Smith; Prakhar Srilastava; Sharjeel Tahir; Shamil Tanna; Kate Tatham; Benjamin Thomas; Pete Thomas; Shree Voralia; Kritchai Vutipongsatorn; Zoszka Webb; Tanith Westerman; Alasdair Wills; Hafsana Ali; Michele Bossy; Naomi Boyer; Letitia Bullman; Dorota Burda; Paula Carveli; Sandra Chmielewska; Daniel Cottam; Nichalus Dawson; Jeanny Del Socorro; Lui Forni; James Hilton; Sherin John; Justin Kirk-Bayley; Donna May Sanga; Kathryn Mcintosh; Jame McKinley; Rugaia Montaser; Tahir Nasser; Razan Nour; Elizabeth Poter; Stephanie Rouse; Armorel Salberg; Joe Tooley; Emma Tyson; Jerik Verula; Eva Wilson; Waldtraud Wutte; Emma Boxall ; Kathryn Cawley; Joy Dearden; Reece Doonan; John MacDonald; Danielle McLaughlan; Alice Baker; Clare Bolger; Rachel Burnish; Michael Carter; Rebecca Cusack; Susan Jackson; Karen Salmon; Lijun Ding; Sarah Farnell Ward; Helen Farrah; Geraldine Gray; Pamela Kabungo; Susannah Leaver; Romina Pepermans Saluzzio; Christine Sicat; Maria Thanasi; Vince Ventura; Nikki Yun; Dorota Banach; Anthony Gordon; Amal Mohammed; Ehsan Ahmadnia; Mike Barker; Aneta Bociek; Lynda Cameron; Fabiola D’Amato; Fraser Hanks; Sarah Khorshid; Rosario Lim; Shelley Lorah; Kyma Morera; Marlies Ostermann; Gill Radcliffe; Chris Remmington; Natasha Thaladi; Duncan Wyncoll; Judith Abrams; Oliver Dare; Geraldine Hambrook; Katarina Manso; Elizabeth McKerrow; Pradeep Shanmugasundaram; Sarah Cornell; Alistair Roy; Lindsey Woods; Hayley Dolan; Thomas Billyward; Pamela Bremmer; Carl Hawkins; Sophie Jackson; Geraldine Ward; Michele Clark; Vijay Jagannathan; Sarah Purvis; Pam Race; Thomas Robb; Simon Sinclair; Jenny Brooks; Jade Cole; Michelle Davies; Rhys Davies; Helen Hill; Matt Morgan; Craig Spencer; Emma Thomas; Angharad Williams; Chiara Ellis; Elvira Hoxha; Valerie Page; Xiaobei Zhao; Nick Ivin; Rachel Johnston; Louise Ma; Anezka Pratley; Aine Ruttledge; Kathryn Simpson; Greg Barton; Anthony Cochrane; Clare Harrop; Chelcie Jewitt; Charlotte Preston; Karen Shuker JAMA
Question Does the administration of β-lactam antibiotics by prolonged infusion reduce 90-day mortality compared with intermittent infusion in adult patients with sepsis or septic shock?
Findings This systematic review and bayesian meta-analysis of 18 randomized trials that included 9108 critically ill adults with sepsis or septic shock reported a 99.1% posterior probability that prolonged infusions were associated with lower 90-day mortality compared with intermittent infusions (risk ratio, 0.86).
Meaning Prolonged infusions of β-lactam antibiotics are associated with a reduced risk of death in critically ill adult patients with sepsis or septic shock compared with intermittent infusions.
Importance There is uncertainty about whether prolonged infusions of β-lactam antibiotics improve clinically important outcomes in critically ill adults with sepsis or septic shock.
Objective To determine whether prolonged β-lactam antibiotic infusions are associated with a reduced risk of death in critically ill adults with sepsis or septic shock compared with intermittent infusions.
Data Sources The primary search was conducted with MEDLINE (via PubMed), CINAHL, Embase, Cochrane Central Register of Controlled Trials (CENTRAL), and ClinicalTrials.gov from inception to May 2, 2024.
Study Selection Randomized clinical trials comparing prolonged (continuous or extended) and intermittent infusions of β-lactam antibiotics in critically ill adults with sepsis or septic shock.
Data Extraction and Synthesis Data extraction and risk of bias were assessed independently by 2 reviewers. Certainty of evidence was evaluated with the Grading of Recommendations Assessment, Development and Evaluation approach. A bayesian framework was used as the primary analysis approach and a frequentist framework as the secondary approach.
Main Outcomes and Measures The primary outcome was all-cause 90-day mortality. Secondary outcomes included intensive care unit (ICU) mortality and clinical cure.
Results From 18 eligible randomized clinical trials that included 9108 critically ill adults with sepsis or septic shock (median age, 54 years; IQR, 48-57; 5961 men [65%]), 17 trials (9014 participants) contributed data to the primary outcome. The pooled estimated risk ratio for all-cause 90-day mortality for prolonged infusions of β-lactam antibiotics compared with intermittent infusions was 0.86 (95% credible interval, 0.72-0.98; I 2 = 21.5%; high certainty), with a 99.1% posterior probability that prolonged infusions were associated with lower 90-day mortality. Prolonged infusion of β-lactam antibiotics was associated with a reduced risk of intensive care unit mortality (risk ratio, 0.84; 95% credible interval, 0.70-0.97; high certainty) and an increase in clinical cure (risk ratio, 1.16; 95% credible interval, 1.07-1.31; moderate certainty).
Conclusions and Relevance Among adults in the intensive care unit who had sepsis or septic shock, the use of prolonged β-lactam antibiotic infusions was associated with a reduced risk of 90-day mortality compared with intermittent infusions. The current evidence presents a high degree of certainty for clinicians to consider prolonged infusions as a standard of care in the management of sepsis and septic shock.
Trial Registration PROSPERO Identifier: CRD42023399434
Critically ill adults who develop sepsis and septic shock face high morbidity and mortality. Early and appropriate antibiotic administration is central to the treatment of such patients. There is uncertainty about effective antibiotic dosing, specifically the duration of infusion in this patient population, due to physiologic perturbations and supportive treatments that may alter antibiotic pharmacokinetics. 1 - 3 Pathogens causing an infection during an intensive care unit (ICU) admission may have reduced antibiotic susceptibility.
β-Lactam antibiotics are widely used as first-line antibiotics for the treatment of sepsis and septic shock. These agents display time-dependent bactericidal activity that is optimal when the free drug concentration remains above the minimum inhibitory concentration of the infecting pathogen for at least 40% to 70% of the dosing interval. 4 There is a biological rationale that prolonged infusions of β-lactam antibiotics may be more effective compared with conventional intermittent dosing. 5 , 6 This rationale is supported by pharmacokinetic-pharmacodynamic studies, which demonstrate that prolonged infusions achieve β-lactam antibiotic exposures associated with maximal bacterial-killing more consistently than intermittent infusions. 7 Whether the effects of prolonged β-lactam antibiotic infusion compared with intermittent infusion result in improved patient-centered outcomes remains uncertain. 8 - 13
Two recently published multinational randomized clinical trials, the Continuous Infusion vs Intermittent Administration of Meropenem in Critically Ill Patients (MERCY) 14 and the Beta-Lactam Infusion Group (BLING) III 15 trials, have added substantially to the body of evidence. To provide an updated summary of current evidence, this systematic review and bayesian meta-analysis was conducted to assess whether administration of β-lactam antibiotics by prolonged infusion was associated with reduced 90-day all-cause mortality and other relevant outcomes compared with intermittent infusion.
A systematic review of randomized clinical trials was performed according to a prespecified published protocol (eAppendix 1 in Supplement 1 ). 16 The review was reported in accordance with the Preferred Reporting Items for Systematic Reviews and Meta-analyses ( PRISMA ) statement 17 and was registered at the International Prospective Register of Systematic Reviews ( CRD42023399434 ).
Randomized clinical trials that recruited critically ill adult participants with sepsis or septic shock and compared the administration of prolonged infusions with intermittent infusions of 1 or more β-lactam antibiotics were included. Conventional and current definitions of sepsis and septic shock at participant recruitment were accepted. 18 - 21 Prolonged infusion was defined as either an extended infusion (intravenous β-lactam antibiotic administration for 2 hours or longer during a dosing interval) or a continuous infusion (constant intravenous β-lactam antibiotic administration that could be administered as a sequential 6-, 8-, 12-, or 24-hour infusion). Intermittent infusion was defined as intravenous β-lactam antibiotic administration for fewer than 2 hours during a dosing interval.
A systematic search of MEDLINE (via PubMed), CINAHL, Embase, Cochrane Central Register of Controlled Trials (CENTRAL), and ClinicalTrials.gov from inception to May 2, 2024, was conducted. The search was performed with no restrictions on language, publication date, or publication status. The search terms were created by a research librarian (L.E.) in collaboration with content area experts in antibiotic pharmacokinetics-pharmacodynamics, critical care, and infectious diseases. The search strategy included a combination of key words and Medical Subject Headings terms to identify randomized clinical trials that included “critically ill patients” or “intensive care unit,” “sepsis” or “septic shock,” “beta-lactam” or “carbapenem” or “cephalosporin” or “monobactam” or “penicillin,” and “continuous infusion” or “extended infusion” or “prolonged infusion” or “intermittent infusion.”
Manual searches of reference lists of included studies and other systematic reviews were undertaken to identify additional studies. eAppendix 2 in Supplement 1 provides additional details of the electronic search strategy.
Using the Covidence systematic review software (Veritas Health Innovation), a minimum of 2 reviewers (H.E., E.N., or I.Z.) independently screened all identified references for inclusion based on the study title and abstract. A minimum of 2 reviewers (H.E., E.N., or I.Z.) independently assessed the full text for inclusion of potentially eligible studies, with disagreements resolved by consensus or, if necessary, consultation with a third reviewer (M.H.A.-A., N.E.H., A.D., or J.A.R.).
Two reviewers (H.E. and I.Z.) independently extracted data from each included study by using a standardized data collection form. Discrepancies were resolved by consensus or, if necessary, by consultation with a third reviewer (M.H.A.-A.). Available data were extracted as outlined in the protocol (eAppendix 1 in Supplement 1 ), 16 including characteristics of the included studies, study design, demographic and clinical details of the study population, details of the intervention and comparison group (study antibiotic, study antibiotic dosing regimen, and concomitant antibiotics), and study outcomes. Attempts were made to contact corresponding authors of included studies to obtain essential aggregate-level data. There was no imputation for missing data. Access to aggregate-level data of 2 trials 15 , 22 before their publication was obtained from the respective corresponding authors.
Using the Cochrane Risk of Bias Tool for randomized trials version 2, 2 reviewers (H.E. and I.Z.) with no affiliation with the included trials independently assessed the risk of bias for each trial. The risk of bias was assessed for all outcomes of interest. Any discrepancies were resolved by consensus or, if necessary, consultation with a third reviewer (M.H.A.-A., N.E.H., or A.D.).
The primary outcome was all-cause 90-day mortality. For studies in which 90-day mortality was not reported, the closest time to day 90 (before or after) was used.
Data were also collected for the following secondary outcomes: ICU mortality, ICU length of stay (as reported in the original study), clinical cure (as defined in the original study), microbiologic cure (as defined in the original study), and adverse events (as defined in the original study).
There were 7 prespecified subgroups for the primary outcome: (1) administration of meropenem vs piperacillin-tazobactam; (2) culture-positive infection vs culture-negative infection; (3) gram-negative infection vs gram-positive infection; (4) receipt of kidney replacement therapy vs no kidney replacement therapy; (5) lung infection vs other infections; (6) sepsis vs septic shock; and (7) male vs female participants. The prespecified hypotheses for these comparisons are detailed in the protocol (eAppendix 1 in Supplement 1 ). 16 When results suggested possible subgroup effects, the Instrument to Assess the Credibility of Effect Modification Analyses ( ICEMAN ) 23 guidelines were used to assess their credibility.
A bayesian framework was used as the primary statistical approach, and a frequentist framework was used as the secondary approach. A random-effects model was used in the analyses and pooled estimates of effect sizes as risk ratios (RRs) for binary outcomes, and mean differences for continuous outcomes were presented. Continuous variables presented in formats not readily amenable to pooling were converted to mean and SD with the method described by Wan et al. 24 Along with the pooled estimates of effect sizes, 95% credible intervals (CrIs) for the bayesian meta-analysis and 95% CIs for the frequentist model were presented.
For the bayesian approach, primary analysis using vague priors (log of the RR assumed to have a normal distribution with a mean of 0 and an SD of 2) and sensitivity analyses examining treatment effects using weakly informative priors of effect and heterogeneity parameters were conducted. 25 The full description of priors is presented in the protocol (eAppendix 1 and eAppendix 3 in Supplement 1 ). 16 For the frequentist approach, a random-effects model using Hartung-Knapp-Sidik-Jonkman 26 and DerSimonian-Laird estimates of the between-study variance was used. A random-effects model was chosen a priori for all analyses because of anticipated between-study variation in trial design and implementation of the interventions.
Quantitative heterogeneity was assessed with the posterior estimates of the heterogeneity parameter (τ) with its 95% CrI. The proportion of variation across studies owing to heterogeneity rather than chance was assessed with the I 2 statistic. Subgroup heterogeneity was assessed by including an interaction term in the bayesian analysis to obtain an estimate and 95% CrI for the ratio of RRs (RRRs) from the posterior distribution of the interaction estimate. The presence of small-study effects was assessed by visual assessment of the contour-enhanced funnel plots and formal Egger regression test. 27 , 28
All statistical analyses were performed with R version 4.3.1 (R Foundation for Statistical Computing). The bayesian analysis was performed with the bayesmeta package for bayesian analysis, 29 and the metafor package was used for the frequentist analysis. 30
The Grading of Recommendations Assessment, Development and Evaluation (GRADE) approach was used to evaluate the overall certainty of evidence that prolonged infusions of β-lactam antibiotics compared with intermittent infusions improve each outcome measure to any degree. 31 , 32
The results of the search and reasons for study exclusion are detailed in eFigure 1 and eTable 1 in Supplement 1 . From 2494 records, 18 eligible randomized clinical trials including 9108 critically ill adult participants with sepsis or septic shock were included (5961 men [65%] and 3147 women [35%]). 14 , 15 , 22 , 33 - 47 Table 1 presents the characteristics of included trials. Details on microbiologic characteristics, β-lactam antibiotic dosing regimens, and outcome definitions of included trials are summarized in eTables 2 and 3 in Supplement 1 . Apart from 1 trial that is not yet published, 22 all other trials were published in peer-reviewed journals. Aggregate-level data from the unpublished trial, 22 as well as additional unpublished aggregate-level data from 10 trials, 14 , 15 , 35 - 37 , 39 - 43 were obtained directly from study authors (eTable 4 in Supplement 1 ).
The 18 included trials had a median of 59 trial participants (IQR, 28-139 participants). The median age of participants in the included trials was 54 years (IQR, 48-57 years). The median Acute Physiology and Chronic Health Evaluation II score was 20 (IQR, 18-22), and the median Sequential [Sepsis-related] Organ Failure Assessment score was 8 (IQR, 6-11). Of the included randomized clinical trials, 17 trials compared continuous infusions of β-lactam antibiotics with intermittent infusions, 14 , 15 , 22 , 33 - 44 , 46 , 47 and 1 trial compared extended infusion with intermittent infusion. 45 Meropenem was studied in 11 trials, 14 , 15 , 22 , 36 , 38 - 41 , 43 , 44 , 46 piperacillin-tazobactam in 8 trials, 15 , 22 , 34 , 37 , 39 , 40 , 42 , 43 cefepime in 3 trials, 33 , 43 , 47 ticarcillin-clavulanate in 2 trials, 39 , 40 and amoxicillin-clavulanate, 22 ampicillin-sulbactam, 45 ceftriaxone, 35 and imipenem-cilastatin 22 in 1 trial each. In 13 trials, an equivalent total daily dose of β-lactam antibiotics was used in both the prolonged and intermittent infusion groups. 14 , 15 , 33 , 35 , 36 , 39 - 42 , 44 - 47 The median duration of randomly assigned β-lactam antibiotic treatment was 7 days (IQR, 6-10 days) and 9 days (IQR, 6-11 days) in the prolonged and intermittent infusion groups, respectively.
Risks of bias assessments are presented in eFigure 2 in Supplement 1 . Four trials were adjudicated as having low risk of bias in all domains for all outcomes of interest. 14 , 15 , 39 , 40 The overall risk of bias was adjudicated as low for 10 of 17 trials contributing all-cause 90-day mortality data. 14 , 15 , 35 , 36 , 38 - 43
There were 17 randomized clinical trials (9014 participants) that contributed data to the primary outcome. The times of follow-up differed across the included trials: 7 trials (n = 249) reported mortality at ICU discharge, 34 - 36 , 41 , 42 , 44 , 46 3 trials (n = 436) reported mortality at hospital discharge, 38 , 39 , 45 4 trials (n = 8117) reported mortality at day 90, 14 , 15 , 22 , 40 1 trial (n = 140) reported mortality at day 30, 43 and 2 trials (n = 72) did not provide the definition of the mortality end point, 33 , 47 as shown in eTable 3 in Supplement 1 .
Using a bayesian random-effects model with vague priors, the pooled estimated RR for all-cause 90-day mortality for prolonged infusions of β-lactam antibiotics compared with intermittent infusions was 0.86 (95% CrI, 0.72-0.98; τ = 0.11; I 2 = 21.5%), with a 99.1% posterior probability that prolonged infusions were associated with lower 90-day mortality ( Figure 1 , Figure 2 , and Figure 3 ; eTable 5 in Supplement 1 ). The certainty in the evidence was adjudicated as high, as presented in Table 2 . The primary outcome results were similar in the sensitivity analyses using semi-informative priors and the specified frequentist methods ( Figures 1 and 2 ; eTable 5 in Supplement 1 ). There was no evidence of small-study effects by visual assessment of the contour-enhanced funnel plots or by Egger regression test (eFigure 3A in Supplement 1 ).
The primary outcome of all-cause 90-day mortality was evaluated in 7 prespecified subgroups ( Figure 2 ). As presented in eTable 5 and eFigures 4 through 10 in Supplement 1 , there was no evidence that the pooled estimate for prolonged infusions of β-lactam antibiotics compared with intermittent infusions for all-cause 90-day mortality was different by meropenem vs piperacillin-tazobactam (RRR, 1.00; 95% CrI, 0.75-1.29); culture-positive vs culture-negative infection (RRR, 1.13; 95% CrI, 0.91-1.72); gram-negative vs gram-positive infection (RRR, 1.13; 95% CrI, 0.85-1.79); kidney replacement therapy vs no kidney replacement therapy (RRR, 1.08; 95% CrI, 0.82-1.53); lung infection vs other infections (RRR, 0.90; 95% CrI, 0.64-1.15); sepsis vs septic shock (RRR, 0.97; 95% CrI, 0.75-1.23); and male vs female participants (RRR, 0.91; 95% CrI, 0.71-1.12).
The secondary outcomes are presented in Table 2 and Figure 2 (eTable 5 and eFigures 11-15 in Supplement 1 ). Assessment of small-study effects is presented in eFigure 3B-F in Supplement 1 . Compared with the use of intermittent infusions, use of prolonged infusions of β-lactam antibiotics was associated with a reduced risk of ICU mortality (RR, 0.84; 95% CrI, 0.70-0.97; high certainty) (eFigure 11 in Supplement 1 ) and an increase in clinical cure (RR, 1.16; 95% CrI, 1.07-1.31; moderate certainty) (eFigure 12 in Supplement 1 ). There were no detectable differences in microbiologic cure (RR, 1.18; 95% CrI, 0.96-1.48; very low certainty) (eFigure 13 in Supplement 1 ), adverse events (RR, 0.89; 95% CrI, 0.51-1.57; very low certainty) (eFigure 14 in Supplement 1 ), and duration of ICU length of stay (mean difference, −0.42; 95% CrI, −1.09 to 0.26; low certainty) (eFigure 15 in Supplement 1 ).
In this systematic review and meta-analysis, β-lactam antibiotic administration by prolonged infusion was associated with a reduced risk of mortality at 90 days for critically ill adult participants with sepsis or septic shock compared with intermittent infusion. The bayesian analysis found a 14-percentage-point relative reduction in the risk of mortality at 90 days with prolonged β-lactam antibiotic infusions compared with intermittent infusions. The number needed to treat for prolonged β-lactam antibiotic infusions to prevent 1 death was 26 patients. The use of prolonged infusions of β-lactam antibiotics was associated with a reduced risk of mortality at ICU discharge. In addition, the use of prolonged infusions was associated with an increased probability of clinical cure. Compared with that of intermittent infusions, the effect of prolonged infusions on microbiologic cure, adverse events, and the duration of ICU length of stay was uncertain.
The observation of reduced risk of mortality in the present analysis is consistent with findings from previous meta-analyses. 9 - 13 In combination, the current evidence presents a higher degree of certainty for clinicians to consider prolonged β-lactam antibiotic infusions as a standard of care in the management of sepsis and septic shock.
The present review has several strengths. Authors of previous meta-analyses 9 - 13 have acknowledged limitations in the quality of the included trials. By incorporating data from 6 recently published trials, 14 , 15 , 22 , 45 - 47 this review provides the most up-to-date evidence, to our knowledge, on the treatment effect of prolonged infusions of β-lactam antibiotics compared with intermittent infusions for critically ill adult patients with sepsis or septic shock. This review included trials that recruited critically ill adult participants with sepsis or septic shock to mitigate population heterogeneity reported in previous meta-analyses. 48 The addition of these trials has increased the sample size of the present analysis, providing greater confidence and precision in estimating the effects of prolonged infusions of β-lactam antibiotics on clinically important outcomes. Ten trials assessed as having a low risk of bias contributed 95% of the data to this analysis. The inclusion of trials that have recruited patients from geographically diverse regions (18 countries across 5 continents) enhances the generalizability of findings to a broader range of treatment settings. The use of both bayesian and frequentist analyses ensures a comprehensive assessment and robust interpretation of the treatment effect under study.
Potential challenges associated with prolonged infusion administration, including drug instability and incompatibility with other intravenous medications, the need for a dedicated intravenous portal, and the potential effect on clinical workload, require some considerations before broad implementation. Future studies should determine the optimal duration of infusion when β-lactam antibiotics are administered as prolonged infusions. Because no credible subgroup was identified in this analysis, studies to further identify specific subsets of patients with sepsis or septic shock who are most likely to benefit from prolonged β-lactam antibiotic infusions are warranted. Including specific health economic analyses in future trials may provide additional insights for routine use of prolonged β-lactam antibiotic infusions, and this recommendation can then be considered for inclusion in future sepsis treatment guidelines and treatment bundles.
This study has several limitations. First, the trials included used various definitions for sepsis and septic shock. To allow for this variation, we accepted all conventional and contemporary definitions for sepsis and septic shock used at the original trials. Second, although the present analysis combined both extended and continuous infusions as prolonged infusions, only 1 trial compared extended infusions with intermittent infusions. Third, variable definitions of clinical cure were used across studies and the determination of cure can be subjective. Fourth, the association between prolonged infusions of β-lactam antibiotics and microbiologic cure, adverse events, and the duration of ICU length of stay remains very uncertain because the quality of evidence concerning these outcomes was very low.
Among adults in the ICU with sepsis or septic shock, the use of prolonged β-lactam antibiotic infusions was associated with a reduced risk of 90-day mortality compared with intermittent infusions. The current evidence presents a high degree of certainty for clinicians to consider prolonged infusions as a standard of care in the management of sepsis and septic shock.
Accepted for Publication: May 7, 2024.
Published Online: June 12, 2024. doi:10.1001/jama.2024.9803
Corresponding Author: Jason A. Roberts, BPharm, PhD, UQ Centre for Clinical Research, Bldg 71/918 RBWH Herston, Brisbane QLD 4029, Australia ( [email protected] ).
Author Contributions: Drs Abdul-Aziz and Devaux had full access to all of the data in the study and take responsibility for the integrity of the data and the accuracy of the data analysis. Drs Abdul-Aziz, Hammond, Delaney, and Roberts contributed equally.
Concept and design: Abdul-Aziz, Hammond, Cotta, De Waele, Di Tanna, Dulhunty, Lipman, Myburgh, Delaney, Roberts.
Acquisition, analysis, or interpretation of data: Abdul-Aziz, Hammond, Brett, De Waele, Devaux, Di Tanna, Dulhunty, Elkady, Eriksson, Hasan, Khan, Liu, Monti, Novy, Omar, Rajbhandari, Roger, Sjövall, Zaghi, Zangrillo, Delaney, Roberts.
Drafting of the manuscript: Abdul-Aziz, Hammond, Di Tanna, Dulhunty, Eriksson, Delaney, Roberts.
Critical review of the manuscript for important intellectual content: Abdul-Aziz, Hammond, Brett, Cotta, De Waele, Devaux, Di Tanna, Dulhunty, Elkady, Hasan, Khan, Lipman, Liu, Monti, Myburgh, Novy, Omar, Rajbhandari, Roger, Sjövall, Zaghi, Zangrillo, Delaney, Roberts.
Statistical analysis: Devaux, Di Tanna, Liu.
Administrative, technical, or material support: Abdul-Aziz, Hammond, Brett, Cotta, Dulhunty, Hasan, Lipman, Monti, Myburgh, Rajbhandari, Roger, Sjövall, Zangrillo, Delaney, Roberts.
Supervision: Abdul-Aziz, Hammond, De Waele, Di Tanna, Delaney, Roberts.
Creation of search terms: Abdul-Aziz.
Risk of bias assessment: Abdul-Aziz, Elkady, Zaghi.
Study selection and data collection: Abdul-Aziz, Elkady.
Conflict of Interest Disclosures: Dr Abdul-Aziz reported being a writing committee member of the BLISS trial, which is included in this meta-analysis. Dr Hammond reported being a writing committee member of the BLING III trial, which is included in this meta-analysis. Dr Brett reported a consultancy payment to his university from GSK for work on an analysis of sotrovimab in COVID-19 infection outside the submitted work; and reported being a writing committee member of the BLING III trial. Dr De Waele reported receiving honoraria from Pfizer, Menarini, and MSD paid to his institution; and consultancy fees from Viatris paid to his institution outside the submitted work. Dr Dulhunty reported being a writing committee member of the BLING I, BLING II, and BLING III trials, which are included in this meta-analysis. Dr Khan reported receiving grants from the Critical Care Society of Southern Africa outside the submitted work. Dr Liu reported being a writing committee member of the BLING III trial. Dr Monti reported receiving honoraria and consultancy fees from AOP Health, InfectoFos, and Pfizer outside the submitted work; and being a writing committee member of the MERCY trial, which is included in this meta-analysis. Dr Myburgh reported being a writing committee member of the BLING I, BLING II, and BLING III trials. Dr Omar reported receiving honoraria from Jafron Biomedical Co for lectures given on the removal of toxins using hemoadsorption therapy in poisoned patients outside the submitted work. Ms Rajbhandari reported being a writing committee member of the BLING III trial. Dr Roger reported receiving honoraria and consultancy fees from Shionogi, bioMérieux, Advanz Pharma, MSD, AOP Orphan, Viatris, Pfizer, and Fresenius outside the submitted work. Dr Zangrillo reported being a writing committee member of the MERCY trial. Dr Roberts reported receiving consulting fees from Qpex Biopharma, Gilead, Advanz Pharma, Pfizer, Sandoz, MSD, Cipla, and bioMérieux; receiving grants from Qpex Biopharma, the British Society for Antimicrobial Chemotherapy, Pfizer, and bioMérieux outside the submitted work; and being a writing committee member of the BLING I, BLING II, and BLING III trials. Dr Roberts reported being a writing committee member of the BLING I, BLING II, and BLING III trials and of the BLISS trial. No other disclosures were reported.
Funding/Support: The George Institute for Global Health and the Centre of Research Excellence–Personalising Antimicrobial Dosing to Reduce Resistance (CRE RESPOND; Australian National Health and Medical Research Council Centres of Research Excellence, APP2007007), The University of Queensland, provided in-kind support for this work. Dr Hammond was supported by an Emerging Leadership grant (APP1196320) from the National Health and Medical Research Council of Australia. Dr De Waele was supported by a Senior Clinical Investigator Fellowship from the Research Foundation–Flanders (FWO 7881020N). Dr Sjövall was supported by a grant from the Swedish Research Council (2019-05908). Drs Myburgh and Roberts were supported by leadership Investigator Grant fellowships (APP1173079 [Dr Myburgh]; APP2009736 and APP2007007 [Dr Roberts]) from the National Health and Medical Research Council of Australia. Dr Roberts was supported by an Advancing Queensland clinical research fellowship and a Centre of Research Excellence fellowship.
Role of the Funder/Sponsor: Other than the specified roles of the coauthors, The George Institute for Global Health and The University of Queensland had no role in the design and conduct of the study; collection, management, analysis, and interpretation of the data; preparation, review, or approval of the manuscript; and decision to submit the manuscript for publication.
Meeting Presentation: Presented at the Critical Care Reviews (CCR) meeting; June 12, 2024; Belfast, UK.
Data Sharing Statement: See Supplement 2 .
- Register for email alerts with links to free full-text articles
- Access PDFs of free articles
- Manage your interests
- Save searches and receive search alerts
Loading metrics
Open Access
Peer-reviewed
Research Article
Clinical outcomes of chikungunya: A systematic literature review and meta-analysis
Roles Data curation, Formal analysis, Methodology, Software, Visualization, Writing – original draft
* E-mail: [email protected]
Affiliation Asc Academics B.V., Groningen, Netherlands

Roles Conceptualization, Writing – original draft
Affiliations Valneva Austria GmbH, Vienna, Austria, Department of Health Sciences, University Medical Center Groningen, Groningen, Netherlands
Roles Conceptualization, Data curation, Methodology, Project administration, Writing – review & editing
Affiliations Asc Academics B.V., Groningen, Netherlands, Department of Health Sciences, University Medical Center Groningen, Groningen, Netherlands
Roles Investigation, Project administration
Roles Data curation, Investigation
Roles Conceptualization, Supervision
Affiliation Valneva Austria GmbH, Vienna, Austria
Roles Supervision
Affiliations Department of Health Sciences, University Medical Center Groningen, Groningen, Netherlands, Department of Economics, Econometrics & Finance, University of Groningen, Faculty of Economics & Business, Groningen, The Netherlands, Center of Excellence for Pharmaceutical Care Innovation, Universitas Padjadjaran, Bandung, Indonesia, Division of Pharmacology and Therapy, Faculty of Medicine Universitas Airlangga, Surabaya, Indonesia
Affiliations Asc Academics B.V., Groningen, Netherlands, Department of Health Sciences, University Medical Center Groningen, Groningen, Netherlands, Department of Health Technology and Services Research, University of Twente, Enschede, The Netherlands
- Kris Rama,
- Adrianne M. de Roo,
- Timon Louwsma,
- Hinko S. Hofstra,
- Gabriel S. Gurgel do Amaral,
- Gerard T. Vondeling,
- Maarten J. Postma,
- Roel D. Freriks
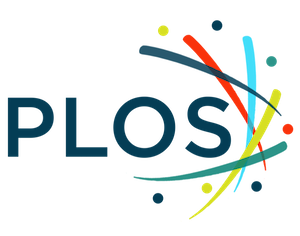
- Published: June 7, 2024
- https://doi.org/10.1371/journal.pntd.0012254
- Peer Review
- Reader Comments
This is an uncorrected proof.
Chikungunya is a viral disease caused by a mosquito-borne alphavirus. The acute phase of the disease includes symptoms such as fever and arthralgia and lasts 7–10 days. However, debilitating symptoms can persist for months or years. Despite the substantial impact of this disease, a comprehensive assessment of its clinical picture is currently lacking.
We conducted a systematic literature review on the clinical manifestations of chikungunya, their prevalence and duration, and related hospitalization. Embase and MEDLINE were searched with no time restrictions. Subsequently, meta-analyses were conducted to quantify pooled estimates on clinical outcomes, the symptomatic rate, the mortality rate, and the hospitalization rate. The pooling of effects was conducted using the inverse-variance weighting methods and generalized linear mixed effects models, with measures of heterogeneity reported.
The systematic literature review identified 316 articles. Out of the 28 outcomes of interest, we were able to conduct 11 meta-analyses. The most prevalent symptoms during the acute phase included arthralgia in 90% of cases (95% CI: 83–94%), and fever in 88% of cases (95% CI: 85–90%). Upon employing broader inclusion criteria, the overall symptomatic rate was 75% (95% CI: 63–84%), the chronicity rate was 44% (95% CI: 31–57%), and the mortality rate was 0.3% (95% CI: 0.1–0.7%). The heterogeneity between subpopulations was more than 92% for most outcomes. We were not able to estimate all predefined outcomes, highlighting the existing data gap.
Chikungunya is an emerging public health concern. Consequently, a thorough understanding of the clinical burden of this disease is necessary. Our study highlighted the substantial clinical burden of chikungunya in the acute phase and a potentially long-lasting chronic phase. Understanding this enables health authorities and healthcare professionals to effectively recognize and address the associated symptoms and raise awareness in society.
Author summary
Chikungunya disease is an emerging public health concern. The disease is transmitted by mosquitoes and characterized by arthralgia and fever in the acute phase, lasting 7–10 days. Additionally, some individuals experience chronic symptoms such as arthralgia and tiredness that can last from months to years. Chikungunya is mainly present in the Americas and Asian countries, but the mosquitoes transmitting the disease are spreading to other regions due to climate change, amongst others. This increased disease threat highlights the importance of understanding chikungunya symptoms. However, there are currently no precise estimates on the prevalence of chikungunya symptoms. Therefore, we analysed the available literature on the clinical manifestations of chikungunya. We found that 75% of infected people develop symptoms, primarily characterized by arthralgia in 90% and fever in 88% of cases. Chronic symptoms affect 44% of symptomatic people, and 0.3% of patients with chikungunya die. Unfortunately, we were not able to estimate all predefined outcomes of interest because we did not find enough studies publishing on some of these, demonstrating that there is still much unknown around the clinical manifestations of chikungunya. However, the results can help healthcare workers early identifying chikungunya and raise awareness of this debilitating disease.
Citation: Rama K, de Roo AM, Louwsma T, Hofstra HS, S. Gurgel do Amaral G, Vondeling GT, et al. (2024) Clinical outcomes of chikungunya: A systematic literature review and meta-analysis. PLoS Negl Trop Dis 18(6): e0012254. https://doi.org/10.1371/journal.pntd.0012254
Editor: Richard A. Bowen, Colorado State University, UNITED STATES
Received: February 26, 2024; Accepted: May 28, 2024; Published: June 7, 2024
Copyright: © 2024 Rama et al. This is an open access article distributed under the terms of the Creative Commons Attribution License , which permits unrestricted use, distribution, and reproduction in any medium, provided the original author and source are credited.
Data Availability: All relevant data are within the manuscript and its Supporting information files.
Funding: This paper was funded by Valneva Austria GmbH. AMR and GTV are Valneva employees. The funder had no role in study design, data collection and analysis, decision to publish, or preparation of the manuscript.
Competing interests: I have read the journal’s policy and the authors of this manuscript have the following competing interests: KR, TL, HSH, and GSG are employees of Asc Academics. Asc Academics has received consultancy fees for this project from Valneva Austria GmbH. AMR and GTV are Valneva employees and own stock options of Valneva. MJP reports grants and honoraria from various pharmaceutical companies, including those developing, producing, and marketing vaccines. He holds stocks in Health-Ecore (Zeist, Netherlands) and PAG BV (Groningen, Netherlands), and advises ASC Academics (Groningen, Netherlands). These competing interest will not alter adherence to PLOS policies on sharing data and materials.
Introduction
Chikungunya is a viral disease caused by a mosquito-borne alphavirus, the chikungunya virus (CHIKV) [ 1 ]. The infection is characterized by an acute phase with symptoms including fever, arthralgia, and myalgia. While most infected individuals fully recover after the acute phase of the disease, between 30–40% of patients develop persistent symptoms, such as chronic arthritis, fatigue, stiffness, depression, and sleep and neurological disorders, which can last from months to several years [ 2 , 3 ]. Long-term effects lead to significant limitations in daily activities and reduce the patients’ overall quality of life [ 4 – 6 ]. Nevertheless, despite the negative impact of the disease on the quality of life, the awareness and societal understanding of chikungunya remain limited, even among the afflicted populations and healthcare workers [ 7 ]. Chikungunya has been identified as a public health threat based on several records of CHIKV outbreaks worldwide, with a risk of exacerbation in the future due to the global spread of CHIKV [ 8 ]. The distribution of the CHIKV vectors ( Aedes aegypti and Aedes albopictus ) is one of the main factors contributing to the disease’s dissemination. This expansion is attributed to globalization and climate change, allowing the Aedes mosquitos to survive in areas previously considered unsuitable [ 9 , 10 ]. Prevention against the disease consists predominantly of mosquito population control [ 11 ]. Recently, the FDA approved the first chikungunya vaccine, presenting a new tool to fight the disease and potentially alleviate the associated economic and health burdens [ 12 ]. Despite the increasing interest in CHIKV and the recent announcement of a vaccine, uncertainties persist regarding the clinical burden of chikungunya. Although multiple studies have explored one or more health outcomes associated with chikungunya [ 3 , 13 , 14 ], to the best of our knowledge, no extensive meta-analysis was performed to quantify pooled estimates on the clinical presentation of chikungunya. To address this gap, we conducted a comprehensive systematic literature review (SLR) on the clinical manifestations of chikungunya, and proceeded with a robust yet flexible meta-analysis. This approach allowed us to provide estimates on a broad spectrum of endpoints on the health outcomes of chikungunya. We paid particular attention to the symptomatic, mortality, and chronicity rates for a comprehensive understanding of the disease in both acute and chronic phases. Our study aims to contribute valuable insights into the overall clinical outcomes of chikungunya. This, in turn, can inform public health intervention strategies and enhance global surveillance by enabling earlier detection of outbreaks.
Literature search and study selection
The SLR adhered to the Preferred Reporting Items for Systematic Reviews and Meta-Analyses 2020 (PRISMA 2020) guidelines, with searches conducted on MEDLINE In-Process via PubMed.com, and Embase via Embase.com without time limits. Grey literature searches were performed for the years 2019–2023 to capture data that may not have yet been included in the databases. The search string included terms related to chikungunya and study design. Eligibility criteria were developed using a Population, Intervention, Comparator, Outcomes, Study (PICOS) framework. The inclusion criteria focused on the clinical manifestations of chikungunya, their prevalence and duration, and related hospitalization, and excluded in vitro/preclinical studies, reviews, and non-English articles. Specifics can be found in S1 Text .
Screening and data extraction
All retrieved articles were deduplicated and titles and abstracts were screened against the PICOS criteria using Rayyan. From the selected articles, full texts were examined for eligibility, followed by detailed data extraction organized by study design, patient characteristics, and outcomes of interest. The whole screening process was conducted by two independent reviewers (GG, HH), resolving conflicts through consensus. An exhaustive feasibility assessment ensured the inclusion of studies with explicit criteria and comparable reporting methods, reducing heterogeneity and potential outlier influence. The risk of bias was determined using a modified Downs and Black checklist [ 15 ] and NIH quality assessment tool for observational studies [ 16 ], see S2 Text . Discrepancies were resolved by consensus. No protocol for this systematic review and meta-analysis was registered.
Population and data analysis
The meta-analysis was performed using the meta package of the R statistical software to create a pooled estimate of the most important clinical outcomes of chikungunya. The outcomes of interest were the overall symptomatic, chronicity, and mortality rates, the underreporting factor, the duration of the acute and chronic phase, the hospitalization and outpatient rate (acute and chronic), the mortality rate per region, and the rate and duration of the following symptoms: arthralgia, arthritis, fatigue, fever, headache, joint swelling, myalgia, nausea, rash, and vomiting. The distinction between arthralgia and arthritis was made based on the definition used in the original study.
Both fixed-effects and random-effects models with logit transformation were estimated, where a random-effects model was chosen in case of high heterogeneity. Fixed-effects meta-analyses employed inverse-variance weighting, while random-effects accounted for between-study heterogeneity and are better suited to account for the larger variations in outcomes reported. Heterogeneity was assessed using Cochran’s Q , I 2 , H 2 statistics, and τ 2 estimation. Outlier analyses employed the leave-one-out method, Baujat plots, and statistical distance measures. All results were visually represented using forest plots, providing a clear and concise graphical representation of the individual study findings and the overall meta-analysis result.
Our study utilized subpopulations—subsets of the original populations defined by particular demographic and clinical features. These features correspond to the data reported in the studies we analyzed and the segmentation into subpopulations was based on the inclusion or exclusion criteria set forth in the original research papers. This approach allowed us to perform a more granular analysis. The clinical outcomes of interest were analyzed for a target population to ensure comparability among included studies, which excluded children under 15, individuals with comorbidities or concurrent infections, and pregnant women. Additionally, we excluded unconfirmed CHIKV cases and studies involving chronic patients reporting on the acute phase due to recall bias. Lastly, retrospective studies focusing on mortality were excluded as they exhibited evidence of selection bias. Meta-analyses were performed when an endpoint was reported at least five times for a given subpopulation.
A preliminary search indicated that data on chronicity, mortality, and symptomatic cases was predominantly reported for a more general population, including individuals under the age of 15 and chronic patients. Therefore, we decided to apply less strict criteria on the studies reporting these outcomes, allowing us to estimate these endpoints. Additionally, to detail the development of chronic symptoms, we estimated the chronic rate at various points from disease onset by dividing studies reporting on chronicity rates following a CHIKV infection into subgroups based on time intervals (three, six, and 12 months). The inclusion criteria for each subgroup were to fall within the time windows created by consecutive intervals (e.g., 90–180 days for three months). We excluded studies extending beyond 24 months to avoid a selection bias, as these already focused on patients with pre-existing chronic conditions.
For the mortality rates, we separated the groups that reported outcomes for high-risk populations from those dealing with the general population with lower risk. This stratification allowed us to account for potential confounding variables. Older age and comorbidities have been identified to increase the risk for mortality [ 2 , 17 ]. Therefore, we classify as high-risk of mortality the groups with a minimum age over 65 (or median above 70 when missing), and previous conditions that induced prior intensive care exceeding 24 hours.
To estimate the overall symptomatic rate, we included studies that explicitly reported symptomatic rates based on one or more of the symptoms commonly associated with the disease. Symptomatic patients were often an implicit inclusion criteria, or a precondition for laboratory testing, making most of the studies reporting on the symptomatic rate unusable. We excluded the studies that had a 100% symptomatic rate to prevent selection bias, as including those would lead to a skewed perspective due to symptoms being part of their inclusion criteria.
Literature search
The SLR was conducted on 4 July 2023 and yielded 16,308 hits. After removing 6,285 duplicates, 10,023 studies were screened by titles and abstracts. From these, a total of 316 articles were deemed suitable for inclusion. The process of the SLR is detailed in Fig 1 , which illustrates the PRISMA diagram of the included studies. The complete PRISMA checklist is provided in S3 Text . The quality assessment of included studies can be found in S1 Table .
- PPT PowerPoint slide
- PNG larger image
- TIFF original image
https://doi.org/10.1371/journal.pntd.0012254.g001
Study characteristics
The categorization of study designs in the included articles was made with careful consideration, taking into account the diversity in how these studies defined their methodologies. The judgment used in categorizing these studies was guided by the definitions provided within the paper itself. When a study described its design in a way that matched more than one predefined category, the predominant one was chosen. This approach aimed to respect the original terminology used by the study authors while also creating a coherent framework for analysis.
Of the 316 articles included, 231 studies were observational, 11 were experimental, and for 74 studies this was not reported. Of the observational studies, 106 were cross-sectional, 35 were cohort, 29 were longitudinal, 25 were retrospective, 23 were prospective, and 13 were case-control or case-series studies. Of the experimental studies, there were 6 trials from phase I to III with double and single-blind designs. Goals ranged from assessing treatments like chloroquine and vaccines’ effectiveness to exploring seroprevalence and chronic CHIKV effects. Two trials investigated new mRNA treatment mechanisms. The focus was solely on CHIKV, not on coinfections.
The study location varied: Southern Asia was the most represented with 78 articles, followed by South America, with 67. There were 41 articles from The Caribbean region, 41 from Eastern Africa, 28 from South-Eastern Asia, 14 from Central America. Eight, six, five, four, and three articles were from Western Europe, Northern America, Southern Europe, Middle Africa, and Western Africa, respectively. Two or one articles were from Eastern Asia, Micronesia, Northern Europe, or Southern Africa. A total of 193 studies reported mean or median age. Data on co-infection with Zika and/or dengue were reported in 11 studies. An overview of the study characteristics, including details on the experimental studies, can be found in S2 Table .
The most commonly reported symptom was fever, reported in 57.9% of the studies (N = 183), followed by rash in 54.1% (N = 171), headache in 51.3% (N = 162), and arthralgia in 47.8% (N = 151). Most studies reported high rates (70% to 100%) of fever. Among the 151 studies reporting arthralgia rates, the symptom prevalence ranged from 1% to 100%, as studies presented heterogeneous settings, including, for example, recovered patients, patients in the acute phase, or chronic patients. Duration of symptoms was reported in 22 studies. Taking all symptoms into account, the mean duration of symptoms ranged from two days (fever) to 342 days (arthralgia). It is important to note that the studies presented heterogeneous groups of patients when reporting on the duration of symptoms, which could explain the wide range reported in literature. The hospitalization rate was reported by 53 studies. The hospitalization rate varied between 0%, reported by five different studies [ 18 – 22 ], and 93% in a study by Reller and colleagues [ 23 ].
The development of chronic disease after CHIKV infection was reported in 68 studies. Most studies defined chronic CHIKV infection as the presence of symptoms three months after the infection. Arthralgia was reported as a chronic symptom in 67 studies, joint swelling was reported in 11 studies, myalgia was reported in eight studies, stiffness, especially in the morning, was reported in six studies, and arthritis was reported in four studies. The percentage of patients developing chronic disease ranged from 16% in a study conducted during an outbreak in Chennai, India [ 24 ] to 100% in two other studies [ 25 , 26 ]. Fifty of the included studies reported data on mortality, of which 22 reported no deaths in the study population. The highest reported mortality rate was 36.67%, or 36,670 per 100,000 population, reported by Gupta and colleagues. This study population consisted of chikungunya patients who had been admitted to the intensive care [ 27 ].
Meta-analyses feasibility and results
From the 316 articles retrieved from the SLR, we extracted 756 distinct subpopulations. Each subpopulation corresponds to a group defined by a unique set of inclusion and exclusion criteria as per the definitions provided in each original study. Out of the 756 subpopulations, 335 were used for the analysis of the general population, while 52 where used for the target population. From the 28 selected clinical outcomes, we were able to conduct 11 meta-analyses for the target population, see Fig 2 . The number of studies and subpopulations available for each endpoint is shown in Table 1 . The forest plots from the individual meta-analyses can be found in S1 Fig , and the outlier analysis for each endpoint with the Baujat plot is presented in S2 Fig . No studies or subpopulations were excluded based on outlier analyses. Below, we present the 11 estimates from the meta-analyses on the target population, followed by the results of the analysis on mortality, chronicity, and overall symptomatic rates in the general population.
https://doi.org/10.1371/journal.pntd.0012254.g002
Presented are the number of studies and number of subpopulations reporting on the specific outcomes, the pooled estimates, confidence intervals and I 2 of the estimated endpoints. CI: confidence interval. I 2 : I-squared statistic of heterogeneity.
https://doi.org/10.1371/journal.pntd.0012254.t001
Chikungunya symptoms estimates in the target population.
The prevalence of arthralgia in symptomatic adults with confirmed chikungunya was estimated at 89.7%, while arthritis was less frequent at 17.6%. Fatigue was observed in 56% of patients, fever in 87.8%, and headache affected 49.5% of the population. Joint swelling was reported in 50% of patients, myalgia in 62.9%, nausea in 34.7%, rash in 44.3%, and vomiting in 17.1%. The hospitalization rate during the acute phase of chikungunya was reported by nine subpopulations and estimated at 17%. All results are presented in Table 1 , showing the pooled effect estimate for each symptom, reflecting the average rate of occurrence in the studied populations within specified confidence intervals. Each symptom analysis showed substantial heterogeneity between subpopulations, indicated by high I 2 statistics.
Chronicity, mortality, and overall symptomatic rate.
The meta-analysis for chronicity rate showed declining rates over time: 43.89% at three months, 34.39% at six months, and 31.87% at twelve months, see Fig 3 . Notably, persistent high heterogeneity was observed across subgroups ( I 2 between 96–97%). Mortality rates were estimated at 0.32% (320 per 100,000 population), for normal-risk populations and 15.34% (15,340 per 100,000 population) for high-risk populations, see Fig 4 . The latter displayed higher heterogeneity ( I 2 = 97%) compared to the normal risk ( I 2 = 87%). The meta-analysis estimates that 74.9% of the general population with CHIKV infection were symptomatic, with a 95% confidence interval from 63% to 84%, see Fig 5 . A total of eight studies with corresponding eight subgroups were included in this analysis. I 2 statistics showed a heterogeneity of 91%. Results of the outlier and influential cases analysis can be found in S2 Fig .
https://doi.org/10.1371/journal.pntd.0012254.g003
https://doi.org/10.1371/journal.pntd.0012254.g004
https://doi.org/10.1371/journal.pntd.0012254.g005
Chikungunya poses an emerging global health threat; however, uncertainties around the health burden of this infectious disease persist. This SLR and meta-analyses aim to consolidate existing research on the clinical manifestations of chikungunya. The objective of this study was to provide accurate estimates on the symptomatology of this disease, with a specific focus on the chronicity, mortality, and overall symptomatic rates. Overall, our findings emphasize the substantial disease burden associated with a CHIKV infection.
Arthralgia, fever, and myalgia were the most prevalent symptoms, affecting 89.7%, 87.8%, and 62.9% of symptomatic adults, respectively. These symptoms are also described in previous literature as most common for chikungunya [ 17 , 28 ]. It’s important to note that these symptoms were often implicitly used when initially detecting suspected cases. Although we removed all explicit inclusion criteria, these estimates are likely affected by selection bias. The hospitalization rate of 17% underscores the challenges for healthcare systems during outbreaks. The disease burden related to these symptoms makes chikungunya a significant burden for local healthcare systems, highly influencing the quality of life of infected individuals [ 6 ].
The number of studies that provided data on mortality, chronicity, and overall symptomatic rate was limited for the target adult population. Thus, we decided to use less restrictive population criteria for these specific outcomes. Within this broader general population, we found a 0.32% (320 per 100,000 population) mortality rate in the low-risk group. This is slightly higher than the common reported case-fatality rate of 0.1% (100 per 100,000 population), although reports on mortality rated may vary [ 2 , 6 ]. To our knowledge, no previous meta-analysis on mortality rates has been performed. Therefore, we argue that 0.32% (320 per 100,000 population) is a realistic estimate for the general population. While this percentage is still relatively low compared to other arboviruses [ 29 ], mortality rates can be drastically higher in high-risk groups. Our meta-analysis revealed a mortality rate of 15.34% (15,340 per 100,000 population) in elderly and individuals with previous emergency department or intensive care admissions.
In defining the high-risk group for mortality, we included hospitalized patients who are typically older. As a result, the average age within this group was higher and advanced age is a recognized risk factor for increased mortality from CHIKV infection [ 30 ]. The task of separating the effects of comorbid conditions from the direct impact of CHIKV on mortality rates is complex. These factors often interact with each other, complicating the attribution of cause of death to CHIKV alone—particularly when our analysis could not conclusively establish the causes listed on death certificates. Furthermore, we recognize the possibility of publication bias in existing research on severe CHIKV cases. There may be an overrepresentation of studies focusing on severe outcomes and elevated mortality rates among individuals with underlying health complications or atypical presentations of CHIKV. Such a bias could lead to an overestimation of the mortality risk associated with the virus. Nonetheless, our SLR showed mortality rates up to 36.67% (36,670 per 100,000 population) in specific populations, demonstrating that despite its low rates in the general population, the impact of mortality should not be overlooked [ 27 ].
The chronic phase of chikungunya can be debilitating and long-lasting, leading to a significant health burden for individuals affected. Results from our meta-analysis showed a chronicity rate of 43.89% at three months, 34.39% at six months, and 31.87% at 12 months post-infection, indicating the lasting health burden. A meta-analysis conducted by Paixao and colleagues on the chronicity rate of chikungunya showed similar outcomes, with an overall no-recovery rate of 43% after three months [ 3 ]. One notable difference, possibly due to variations in methodologies, is that Paixao and colleagues reported slightly lower rates over time. Both studies indicate a stabilization over time, but more research is needed to comprehensively map the progression of the chronic phase. In conclusion, long-term chronic illness majorly impacts the quality of life of chikungunya patients [ 4 , 6 ], making these results alarming, especially in light of the potential growing spread of the disease [ 9 , 10 ].
The significant disease burden related to chikungunya was further underlined by an overall symptomatic rate of 74.9% in the general population. The symptomatic rate of chikungunya was estimated between 72% and 97% by the CDC Yellow Book, showing that our estimate could be on the low end [ 17 ]. A reason for this could be the various definitions of symptomatic manifestations across studies, which posed a challenge in deriving a precise estimate for this outcome. Additionally, estimates in the literature are mainly based on patients showing healthcare-seeking behaviour, leaving out asymptomatic patients. Therefore, these estimates are likely to be overestimated. Because we created our estimate based on the total general population, we expect them to provide a better reflection of reality.
Two studies identified in the SLR were designed to investigate treatment options for Chikungunya and therefore included control groups. However, we excluded control populations without confirmed CHIKV from our analysis because our focus was on populations with confirmed CHIKV. In instances where multiple treatment options were assessed among confirmed CHIKV populations, these groups were included in the analysis as we aimed to understand the symptomatology of the disease at presentation in its acute phase. It should be noted that the inclusion of these populations did not significantly influence the outcomes of our study since the primary interest was in the manifestation of symptoms rather than treatment efficacy.
Although we obtained estimates for 11 of the 28 predefined endpoints, estimation for several endpoints proved infeasible due to their infrequent reporting as identified in the SLR. We did not obtain estimates for the underreporting factor, the length of the acute and the chronic phase, the duration of the different symptoms, and the frequencies of hospitalization and outpatient care. Even considering the subpopulation analysis method used, we could not estimate more endpoints. The limited number of studies reflects the uncertainty and novelty associated with chikungunya and the need for more research in this field.
In cases where meta-analyses were feasible for the endpoints, we encountered challenges due to poor data quality or absent data. This is attributable to two main reasons: firstly, the reporting of several endpoints varied inconsistently across studies, preventing their combination in a meta-analysis; and secondly, some studies that reported the desired endpoint did not meet the inclusion criteria, resulting in sparse data that hindered meaningful analysis. As a result, significant knowledge gaps persist regarding various aspects of chikungunya. Further research is necessary to fill these gaps and enhance our understanding of this disease. Additionally, consistent and strict reporting criteria on the clinical picture of chikungunya are needed to help create more comprehensive estimates. Enhanced quality and quantity of data could facilitate the possibility to study potential differences in symptomatology for the different CHIKV subtypes. Furthermore, it could enable investigations into the pathogenicity of CHIKV over the years by comparing data from previous outbreaks.
A strength of our study is the use of subpopulation analyses. We discovered that extracting subpopulations from individual studies allows more endpoints to be estimated, offering comparable populations that limit heterogeneity. The use of subgroups could be useful for future research and mitigate some of the data discrepancies detected in the SLR.
The main limitation of our study is the significant presence of heterogeneity indicated by an average I 2 statistic of 92%. This reflects substantial differences in the inclusion criteria among the studies, a tendency inherent in the disease area of CHIKV as shown by other meta-analyses reporting similar, or even higher, levels of heterogeneity [ 3 ]. There are several reasons for this high heterogeneity. First, data collection on chikungunya is conducted mostly during the outbreaks which limits the possibility of establishing strict scientific protocols as researchers must adapt to the dynamic nature of the event. Secondly, a standardized methodology for reporting endpoints is lacking, making it challenging to compare studies in a meta-analysis. Thirdly, we noticed that including older individuals affected our results, by showing lower symptomatic rates but higher mortality and hospitalization rates. Future studies might exclude this demographic for more precise age-related outcomes. Additionally, other, less known, symptoms might have influenced the disease estimates. An example of this is depressive symptoms related to chikungunya. A study included in our analysis has potentially skewed our meta-analysis results with inflated estimates for fatigue, headache, and rash because they investigated depressive symptoms during the CHIKV infection [ 31 ]. This highlights how undisclosed factors that increase the population’s vulnerability to chikungunya symptoms can potentially impact the research. Another limitation is the potential for confounding factors contributing to symptom prevalence, which we were unable control for in our study. There’s an implicit assumption that the symptoms described have a causal association with Chikungunya; however, some symptoms such as myalgia and fatigue are commonly prevalent in the population and may not be causally related to CHIKV infection. The difficulty in establishing a direct causal relationship between these symptoms and CHIKV should be taken into consideration when interpreting the results. We acknowledge that this could affect the precision of the associations drawn in our analysis and suggest that future research should aim to discern the specific attributable risk of CHIKV for these symptoms. Lastly, outbreaks often occur in locations with limited surveillance systems, leading to lacking or less accurate data from these areas. The high heterogeneity shows the need for additional research in the fields, as well as standardized methodologies in studying chikungunya. Additionally, it emphasizes the importance of meta-analyses like these to come to accurate estimates.
Chikungunya is recognized as a global public health threat, and the disease is expected to spread further due to globalization and climate change. At the same time, vector control and surveillance systems remain limited. Consequently, a thorough understanding of the clinical burden of chikungunya is important to inform public health intervention strategies and improve global surveillance. Our study showed that chikungunya poses a significant health burden, with 74.9% of infected individuals experiencing symptomatic disease. Chronic symptoms are found in 43.4% of patients and can be debilitating and long-lasting. We were not able to create pooled estimates on all endpoints, highlighting the still existing data gap in literature here. Nevertheless, the outcomes determined add to the growing body of evidence underlining the debilitating consequences of chikungunya. With the growing threat of chikungunya, health authorities and healthcare professionals must be prepared to adequately diagnose patients affected by the disease and consider public health interventions to limit its burden. Our findings contribute to the comprehension of chikungunya’s clinical outcomes, essential for improving global surveillance and detecting potential outbreaks.
Supporting information
S1 text. literature search and study selection..
Containing the search strategy and PICOs of the studies included in the SLR.
https://doi.org/10.1371/journal.pntd.0012254.s001
S2 Text. Quality assessment tools.
Modified Downs & Black checklist and the NIH quality assessment tool.
https://doi.org/10.1371/journal.pntd.0012254.s002

S3 Text. PRISMA 2020 checklist.
https://doi.org/10.1371/journal.pntd.0012254.s003
S1 Table. Quality assessment of included studies.
https://doi.org/10.1371/journal.pntd.0012254.s004
S2 Table. Summary of study characteristics.
https://doi.org/10.1371/journal.pntd.0012254.s005
S1 Fig. Forest plots of the clinical outcomes of chikungunya.
https://doi.org/10.1371/journal.pntd.0012254.s006
S2 Fig. Influential case and outlier analysis with Baujat plots.
https://doi.org/10.1371/journal.pntd.0012254.s007
Acknowledgments
We would like to thank the internal teams of Asc Academics who helped during the data extraction phase of the SLR, as well as Roma Kwiatkiewicz from Asc Academics for providing medical writing support.
- View Article
- PubMed/NCBI
- Google Scholar
- 2. European Centre for Disease Prevention and Control [Internet]. Factsheet about chikungunya. [cited 2024 February 20]. Available from: https://www.ecdc.europa.eu/en/chikungunya/facts/factsheet .
- 8. Zavala-Colon M, Gonzalez-Sanchez JA. 2. In: Engohang-Ndong J, editor. History and Geographic Distribution of Chikungunya Virus. Rijeka: IntechOpen; 2022. p. 15–24. Available from: https://doi.org/10.5772/intechopen.98662 .
- 12. Food and Drug Administration [Internet]. FDA Approves First Vaccine to Prevent Disease Caused by Chikungunya Virus. [cited 2024 February 20]. Available from: https://www.fda.gov/news-events/press-announcements/fda-approves-first-vaccine-prevent-disease-caused-chikungunya-virus .
- 16. National Heart, Lung, and Blood Institute [Internet]. Study Quality Assessment Tools. [cited 2024 February 20]. Available from: https://www.nhlbi.nih.gov/health-topics/study-quality-assessment-tools .
- 17. Centers for Disease Control and Prevention [Internet]. CDC Yellow Book 2024. [cited 2024 February 20]. Available from: https://wwwnc.cdc.gov/travel/yellowbook/2024/infections-diseases/chikungunya .
- Open access
- Published: 18 June 2024
Effects of exercise training on cardiac output in subjects with heart failure with preserved ejection fraction (HFpEF) — a protocol for a systematic review and meta-analysis
- Raphael Schoch 1 na1 ,
- Benedikt Gasser 1 na1 ,
- Philippe Beck 1 ,
- Christian Appenzeller-Herzog 2 &
- Arno Schmidt-Trucksäss ORCID: orcid.org/0000-0002-4662-3911 1 , 3
Systematic Reviews volume 13 , Article number: 159 ( 2024 ) Cite this article
Metrics details
Patients with heart failure with preserved ejection fraction (HFpEF) commonly experience exercise intolerance, resulting in reduced cardiorespiratory fitness. This is characterised by a decreased maximal oxygen uptake (V̇O 2peak ), which is determined by the product of cardiac output (CO) and arteriovenous oxygen difference (a-vDO 2 ). While exercise training has been shown to improve V̇O 2peak in HFpEF patients, the effects on CO remain unclear. The aim of this study is to systematically review and analyse the current evidence on the effects of supervised exercise training on CO in patients with HFpEF.
We will systematically search for literature describing the effects of supervised exercise training on CO in patients with HFpEF. All eligible studies published before 30 June 2023 in the following electronic databases will be included: MEDLINE (Ovid), Embase (Ovid), SPORTDiscus (EBSCOhost), and CENTRAL (Cochrane Library). Effect sizes will be extracted for CO before and after a supervised exercise training intervention at rest and maximal exercise. Mass of heterogeneity ( I 2 ) will be calculated, and either fixed-effect models or random-effect models will be used for meta-analysis. To detect a potential publication bias, funnel plot analyses will be performed.
While several studies have reported a positive effect of supervised exercise training on cardiorespiratory fitness, attempts to assess the underlying determinants of V̇O 2peak , CO, and a-vDO 2 are much scarcer, especially in patients with HFpEF. From a physiological perspective, measuring CO before and after supervised exercise training seems to be a reasonable way to accurately operationalise a potential improvement in cardiac function.
Systematic review registration
PROSPERO CRD42022361485.
Peer Review reports
Strengths and limitations of this study
To the best of our knowledge, this is the first protocol planning to calculate the pooled effect estimate of supervised exercise training on CO in patients with HFpEF.
By focusing on the specific physiological parameter CO, the systematic review will provide valuable insights into the impact of exercise training on cardiovascular function.
A possible limitation will be the inclusion of studies written in English, French, and German only.
A second possible limitation will be the possible heterogeneity in exercise training interventions. Variations in exercise duration, intensity, and frequency may have an impact on comparability and generalisability of the results.
Introduction
Heart failure with preserved ejection fraction (HFpEF) is estimated to be prevalent in approximately 50% or more of individuals diagnosed with heart failure (HF) [ 1 , 2 ]. It is defined as left ventricular ejection fraction > 50%, elevated levels of brain natriuretic peptides, objective evidence of structural heart disease or diastolic dysfunction [ 3 ], and associated with increased mortality, and health care expenditures [ 4 ]. A key characteristic of HFpEF is reduced cardiorespiratory fitness, defined as the maximal oxygen uptake (V̇O 2peak ) [ 4 ]. V̇O 2peak is the product of two main determinants: cardiac output (CO) and arteriovenous oxygen difference (a-vDO 2 ). CO is the product of the heart rate (HR), the number of heart beats per minute, and the stroke volume (SV; the volume of blood pumped from the ventricle per beat). CO and a-vDO 2 (and consequently V̇O 2peak ) are limited [ 5 ]. Nevertheless, in supervised exercise training studies, only the two markers V̇O 2peak and peak power output are usually reported [ 6 ], but neither CO nor a-vDO 2 .
While several studies have investigated the physiological mechanism underlying the reduced V̇O 2peak in patients with HF with reduced ejection fraction (HFrEF) [ 7 , 8 , 9 ], considerably, less is known about the mechanism in patients with HFpEF [ 10 ]. Although several studies have demonstrated that exercise training improves V̇O 2peak in patients with HFpEF [ 11 , 12 , 13 , 14 , 15 ], less is known about the effect of exercise training on CO in patients with HFpEF. Abudiab et al. showed that reduced cardiorespiratory fitness is associated with an insufficient CO in relation to metabolic demand [ 16 ]. In contrast, Haykowsky et al. reported that increased a-vDO 2 is primarily contributing to improved V̇O 2peak in patients with HFpEF, while SV and CO were not significantly altered after 16 weeks of endurance training [ 17 ]. Similar to these results, Fu et al. demonstrated that improvement in V̇O2peak with high-intensity interval training was associated with an improved a-vDO2. However, maximal HR, SV index, and cardiac index remained unchanged after training [ 18 ].
On a general note, the improvement in exercise capacity is mainly operationalised with V̇O 2peak , which does not provide clear indications of training effects on direct cardiac function like CO. As there is still a lack of understanding concerning adaption processes in patients with HFpEF, a thorough examination of the effect of supervised exercise training on CO at rest and maximal exercise is crucial and will assist the development of valuable therapeutic recommendations [ 19 ].
Furthermore, existing reviews have focused on parameters such as the ratio of peak early to late mitral inflow velocities (E/A ratio), the ratio of early diastolic mitral inflow to annular velocities (E/E’ ratio), or changes in left ventricular ejection fraction during resting conditions. These reviews and meta-analyses contributed significantly to the understanding of HFpEF pathophysiology [ 20 , 21 , 22 , 23 , 24 , 25 , 26 ].
However, our review aims to specifically examine the impact of exercise training on cardiac output at rest and during exercise in patients with HFpEF. By exploring the dynamic changes in cardiac output during exercise, our review seeks to elucidate novel insights into the pathophysiology of HFpEF. We believe that this approach will provide a comprehensive understanding of the physiological response to exercise in HFpEF, thereby advancing the current knowledge base in this field.
Why it is important to do this review
Given the limited success of medical treatments in improving prognosis for patients with HFpEF [ 27 , 28 , 29 ] and the current therapeutic approaches primarily focused on relieving HF symptoms [ 19 ], it is crucial to better understand the effects of exercise training on V̇O 2peak and the underlying determinants CO and a-vDO 2 .
Therefore, to enhance the design of future studies and pinpoint therapeutic targets, a more comprehensive understanding of the basic mechanisms contributing to V̇O 2 limitation in HFpEF is crucial [ 16 ].
This review is also important because it is still not clear which exercise intensity is best to improve the cardiorespiratory fitness in patients with HFpEF. Wisløff et al. (2007) demonstrated that high-intensity interval training (HIIT) was superior to moderate continuous training (MCT) in terms of improving cardiorespiratory fitness in heart failure patients [ 30 ]. Later, smaller studies were able to confirm these findings in patients with HFpEF [ 11 , 12 ], but the recently published OptimEx study refuted previous findings and did not find superiority of HIIT compared to MCT in patients with HFpEF [ 31 ]. In order to determine which training protocol or exercise training is superior to another, an improvement in V̇O 2peak has often been considered as the crucial factor. It would, however, be beneficial to also obtain more information regarding the improvement of CO, as it is a critical determinant of exercise performance, and there is no consensus yet about the superior training protocol [ 11 , 12 , 31 ].
Furthermore, to the best of our knowledge, there is currently no systematic review and meta-analysis focusing on the effects of exercise training on CO in HFpEF. Therefore, reviewing and meta-analysing the literature to identify studies measuring CO in patients with HFpEF are a first step to determine whether exercise can improve CO, potentially assisting in the development of valid therapeutic recommendations for patients with HFpEF.
Aim and review question
This study aims at systematically reviewing and meta-analysing the literature on effects of exercise training on CO in patients with HFpEF. As hypothesis with potential falsification, it shall be stated that an exercise training intervention as compared to standard of care has no effect on CO.
Material and methods
This systematic review protocol is reported according to the guidelines of the Preferred Reporting Items for Systematic Reviews and Meta-Analyses Protocols (PRISMA-P) [ 32 ]. The protocol was submitted for registration in International Prospective Register of Systematic Reviews (PROSPERO) on 20 September 2022 and registered on 5 October 2022 (registration number CRD42022361485). The research question was formulated according to PICOS (Population, Intervention, Comparison, Outcome, Study type) framework (Table 1 ) with the aim of capturing the current evidence regarding the impact of exercise compared to standard care on the difference in CO from pre-to post-intervention.
Patient and public involvement
Patients or the public were not involved in the design, conduct, reporting, or dissemination plans of this research.
Study eligibility criteria
Given the variability and challenge associated with the diagnosis of HFpEF [ 33 ], we have defined HFpEF by the following signs and symptoms: left ventricular ejection fraction > 50%, elevated levels of brain natriuretic peptides (BNP > 35 pg·ml−1 or NTproBNP > 125 pg·ml−1), objective evidence of structural heart disease, or diastolic dysfunction usually provided by echocardiography [ 3 ], without acute or severe coronary, valvular, or pulmonary disease that could mimic HF symptoms.
It is acknowledged that there might be diversity within the study populations concerning the definition of HFpEF. Therefore, we intend to provide a detailed description and discussion of the different definitions of HFpEF in the manuscript.
Inclusion criteria
All studies investigating the effect of a supervised exercise training on CO in patients with HFpEF.
All clinical studies, which were published until the date of the last search and have the design of a randomised controlled trial (with standard care applied to a control group)
Studies measuring CO or SV at rest before and after a supervised exercise training
All forms of supervised exercise training that can be considered having more than a single training session.
Exclusion criteria
Studies reporting estimated CO
Studies published in languages other than English, German, and French
Study designs other than randomised controlled trial or non-original articles (for example editorials, letters, reviews), meta-analyses, case reports, and conference abstracts
Information sources and search strategy
Search strategies were developed in collaboration with an information specialist (C. A.-H.) using the Peer Review of Electronic Search Strategies framework [ 34 ]. We will search MEDLINE (Ovid), Embase (Ovid), SPORTDiscus (EBSCOhost), and CENTRAL (Cochrane Library) for randomised controlled trials using database-specific subjects headings and text-word synonyms around the concepts HFpEF, exercise training, and CO. Nonhuman studies and conference abstracts will be excluded. The detailed search strings can be found in online supplemental document.
To complement the results of database searching, the bibliographic references and citing articles of all included articles will be collected from citationchaser, Scopus, and the Web of Science, deduplicated and screened for eligibility (backward and forward citation searching).
Study records: data management, selection process, and data collection
Studies were imported into EndNote (version 21), to remove duplicates, and then entered into Rayyan [ 35 ] for the screening process. The titles and abstracts of retrieved records will be reviewed independently by two authors (P. B. and R. S.). Articles will be categorised as ‘include’,’exclude’, or ‘uncertain’ based on the prespecified eligibility criteria. For articles categorized as ‘include’ or ‘uncertain’, the full text will be retrieved and independently reviewed for eligibility by two authors (P. B. and R. S.). Any discrepancies during title/abstract or full-text screening will be resolved through discussion between the two screening authors. If no resolution can be found, a third party (B.) will make the final judgement. Data will be extracted from the full texts and entered into a standardised Excel form. One author will extract the data (B. G.), and a second author will independently check the extractions (R. S.). Any discrepancies will be resolved through discussion, involving a third party (A. S. T.) if necessary. Corresponding authors will be contacted twice via email in case of any missing or unclear data. Publications will be excluded from meta-analysis if there is no response or if the required data cannot be provided. The data to be extracted are shown in Table 2 . If there are more than two assessment time points (beyond pre-post), the initial assessment and the one closest to the end of the intervention will be considered.
Outcome and prioritisation
The primary outcome evaluated in this study is the difference in CO levels at rest and under peak power from before to after a supervised exercise training in subjects with HFpEF.
Risks-of-bias (quality) assessment
The risk of bias at study level will be assessed using the RoB 2 tool by two authors independently (P. B. and R. S.) [ 36 ]. Discrepancies will be resolved through discussion (or with a third party, if necessary, AST).
Data synthesis
Standard mean differences (SMD) at the study level will be calculated as the difference in mean change of CO before versus after exercise training or standard care for the control group. SMD will be interpreted as small d = 0.2, moderate d = 0.5, or large d ≥ 0.8. Furthermore, to detect the effect of exercise training, we will compare and meta-analyse the pre- versus post-differences in CO in the exercise training group and the control group by calculating a pooled SMD. Cochran’s Q statistic will be calculated, providing a measure of the variance between the SMD (with p < 0.05 indicating evidence for heterogeneity), while I 2 provides a measure of the amount of variance between studies in terms of heterogeneity (with I 2 > 50% indicating substantial heterogeneity) [ 37 , 38 ]. Thereby, heterogeneity variance shall be estimated using restricted maximum likelihood method (REML). If study heterogeneity is substantial ( I 2 > 50%), random-effect models shall be used for meta-analysis of SMD (otherwise fixed-effect models). Statistical analyses will be conducted using Review Manager software (version 5.3, Copenhagen: The Nordic Cochrane Center, The Cochrane Collaboration 2014) and R (version 4.1.1) [ 39 ].
Additional analysis
No additional analyses will be conducted.
Meta-bias(es)
If 10 or more studies will be found, funnel plots according to Egger will be calculated to detect a potential publication bias [ 40 ].
Certainty of cumulative evidence
The certainty of evidence will be evaluated with the Grading of Recommendations Assessment, Development and Evaluation system, which is a tool classifying evidence into one of four categories ranging from very low to high [ 41 ].
Ethics and dissemination
The present work is a systematic review and meta-analysis protocol. No human participants will be involved; therefore, no ethics approval is required. It is planned to communicate the study results in a peer-reviewed journal and as a conference presentation.
The aim of this systematic review and meta-analysis is to investigate the effects of supervised exercise training on CO in patients with HFpEF.
This research is of utmost importance because current exercise training interventions primarily focus on improving V̇O 2peak , while the impact on CO, a critical determinant of exercise performance, remains largely unknown, and CO is crucial for understanding exercise intolerance in patients with HFpEF.
The systematic review and meta-analysis aims to address this knowledge gap and determine whether supervised exercise training has an effect on CO in patients with HFpEF in order to achieve a potential improvement of the therapeutic recommendations in the future.
Availability of data and materials
All data will be included in the final published systematic review and meta-analysis article.
Abbreviations
Arteriovenous oxygen difference
Body mass index
Brain natriuretic peptides
Cardiac output
Cardiopulmonary exercise testing
Heart failure
Heart failure with preserved ejection fraction
Heart failure with reduced ejection fraction
High-intensity interval training
Moderate continuous training
N-terminal pro-B-type natriuretic peptide
Preferred Reporting Items for Systematic Reviews and Meta-Analyses Protocols
International Prospective Register of Systematic Reviews
Randomised controlled trial
Restricted maximum likelihood
Standard mean difference
Stroke volume
Maximal oxygen uptake
Maeder MT, Kaye DM. Heart failure with normal left ventricular ejection fraction. J Am Coll Cardiol. 2009;53(11):905–18.
Article PubMed Google Scholar
Owan TE, Hodge DO, Herges RM, Jacobsen SJ, Roger VL, Redfield MM. Trends in prevalence and outcome of heart failure with preserved ejection fraction. N Engl J Med. 2006;355(3):251–9.
Article CAS PubMed Google Scholar
McDonagh TA, Metra M, Adamo M, Gardner RS, Baumbach A, Böhm M, et al. 2021 ESC guidelines for the diagnosis and treatment of acute and chronic heart failure. Eur Heart J. 2021;42(36):3599–726.
Haykowsky MJ, Kitzman DW. Exercise physiology in heart failure and preserved ejection fraction. Heart Fail Clin. 2014;10(3):445–52.
Article PubMed PubMed Central Google Scholar
Fu Q, Levine BD. Exercise and the autonomic nervous system. Handb Clin Neurol. 2013;117:147–60.
Gasser BA, Boesing M, Schoch R, Brighenti-Zogg S, Kröpfl JM, Thesenvitz E, et al. High-intensity interval training for heart failure patients with preserved ejection fraction (HIT-HF)-rational and design of a prospective, randomized, controlled trial. Front Physiol. 2021;12: 734111.
Esposito F, Mathieu-Costello O, Shabetai R, Wagner PD, Richardson RS. Limited maximal exercise capacity in patients with chronic heart failure: partitioning the contributors. J Am Coll Cardiol. 2010;55(18):1945–54.
Sullivan MJ, Knight JD, Higginbotham MB, Cobb FR. Relation between central and peripheral hemodynamics during exercise in patients with chronic heart failure. Muscle blood flow is reduced with maintenance of arterial perfusion pressure. Circulation. 1989;80(4):769–81.
Wilson JR, Mancini DM, Dunkman WB. Exertional fatigue due to skeletal muscle dysfunction in patients with heart failure. Circulation. 1993;87(2):470–5.
Haykowsky MJ, Brubaker PH, John JM, Stewart KP, Morgan TM, Kitzman DW. Determinants of exercise intolerance in elderly heart failure patients with preserved ejection fraction. J Am Coll Cardiol. 2011;58(3):265–74.
Angadi SS, Mookadam F, Lee CD, Tucker WJ, Haykowsky MJ, Gaesser GA. High-intensity interval training vs. moderate-intensity continuous exercise training in heart failure with preserved ejection fraction: a pilot study. J Appl Physiol (1985). 2015;119(6):753–8.
da Donelli Silveira A, de Beust Lima J, da Silva Piardi D, Dos Santos Macedo D, Zanini M, Nery R, et al. High-intensity interval training is effective and superior to moderate continuous training in patients with heart failure with preserved ejection fraction: a randomized clinical trial. Eur J Prev Cardiol. 2020;27(16):1733–43.
Article Google Scholar
Edelmann F, Gelbrich G, Düngen HD, Fröhling S, Wachter R, Stahrenberg R, et al. Exercise training improves exercise capacity and diastolic function in patients with heart failure with preserved ejection fraction: results of the Ex-DHF (Exercise training in Diastolic Heart Failure) pilot study. J Am Coll Cardiol. 2011;58(17):1780–91.
Kitzman DW, Brubaker PH, Morgan TM, Stewart KP, Little WC. Exercise training in older patients with heart failure and preserved ejection fraction: a randomized, controlled, single-blind trial. Circ Heart Fail. 2010;3(6):659–67.
Smart NA, Haluska B, Jeffriess L, Leung D. Exercise training in heart failure with preserved systolic function: a randomized controlled trial of the effects on cardiac function and functional capacity. Congestive heart failure (Greenwich, Conn). 2012;18(6):295–301.
Abudiab MM, Redfield MM, Melenovsky V, Olson TP, Kass DA, Johnson BD, Borlaug BA. Cardiac output response to exercise in relation to metabolic demand in heart failure with preserved ejection fraction. Eur J Heart Fail. 2013;15(7):776–85.
Haykowsky MJ, Brubaker PH, Stewart KP, Morgan TM, Eggebeen J, Kitzman DW. Effect of endurance training on the determinants of peak exercise oxygen consumption in elderly patients with stable compensated heart failure and preserved ejection fraction. J Am Coll Cardiol. 2012;60(2):120–8.
Fu TC, Yang NI, Wang CH, Cherng WJ, Chou SL, Pan TL, Wang JS. Aerobic interval training elicits different hemodynamic adaptations between heart failure patients with preserved and reduced ejection fraction. Am J Phys Med Rehabil. 2016;95(1):15–27.
Ponikowski P, Voors AA, Anker SD, Bueno H, Cleland JGF, Coats AJS, et al. 2016 ESC guidelines for the diagnosis and treatment of acute and chronic heart failure: the task force for the diagnosis and treatment of acute and chronic heart failure of the European Society of Cardiology (ESC)developed with the special contribution of the Heart Failure Association (HFA) of the ESC. Eur Heart J. 2016;37(27):2129–200.
Lin B, Siskin M, Wang B, Natarajan S. Does exercise training improve exercise tolerance, quality of life, and echocardiographic parameters in patients with heart failure with preserved ejection fraction? A systematic review and meta-analysis of randomized controlled trials. Heart Fail Rev. 2023;28(4):795–806.
Edwards JJ, O’Driscoll JM. Exercise training in heart failure with preserved and reduced ejection fraction: a systematic review and meta-analysis. Sports Medicine - Open. 2022;8(1):76.
Zhuang C, Luo X, Wang Q, Wang W, Sun R, Zhang X, Yu J. The effect of exercise training and physiotherapy on diastolic function, exercise capacity and quality of life in patients with heart failure with preserved ejection fraction: a systematic review and meta-analysis. Kardiol Pol. 2021;79(10):1107–15.
Fukuta H, Goto T, Wakami K, Kamiya T, Ohte N. Effects of exercise training on cardiac function, exercise capacity, and quality of life in heart failure with preserved ejection fraction: a meta-analysis of randomized controlled trials. Heart Fail Rev. 2019;24(4):535–47.
Chan E, Giallauria F, Vigorito C, Smart NA. Exercise training in heart failure patients with preserved ejection fraction: a systematic review and meta-analysis. Monaldi Arch Chest Dis. 2016;86(1–2):759.
PubMed Google Scholar
Dieberg G, Ismail H, Giallauria F, Smart NA. Clinical outcomes and cardiovascular responses to exercise training in heart failure patients with preserved ejection fraction: a systematic review and meta-analysis. J Appl Physiol (1985). 2015;119(6):726–33.
Pandey A, Parashar A, Kumbhani D, Agarwal S, Garg J, Kitzman D, et al. Exercise training in patients with heart failure and preserved ejection fraction: meta-analysis of randomized control trials. Circ Heart Fail. 2015;8(1):33–40.
Burkhoff D. Mortality in heart failure with preserved ejection fraction: an unacceptably high rate. Eur Heart J. 2012;33(14):1718–20.
Pitt B, Pfeffer MA, Assmann SF, Boineau R, Anand IS, Claggett B, et al. Spironolactone for heart failure with preserved ejection fraction. N Engl J Med. 2014;370(15):1383–92.
Solomon SD, Zile M, Pieske B, Voors A, Shah A, Kraigher-Krainer E, et al. The angiotensin receptor neprilysin inhibitor LCZ696 in heart failure with preserved ejection fraction: a phase 2 double-blind randomised controlled trial. Lancet (London, England). 2012;380(9851):1387–95.
Wisløff U, Støylen A, Loennechen JP, Bruvold M, Rognmo Ø, Haram PM, et al. Superior cardiovascular effect of aerobic interval training versus moderate continuous training in heart failure patients: a randomized study. Circulation. 2007;115(24):3086–94.
Mueller S, Winzer EB, Duvinage A, Gevaert AB, Edelmann F, Haller B, et al. Effect of high-intensity interval training, moderate continuous training, or guideline-based physical activity advice on peak oxygen consumption in patients with heart failure with preserved ejection fraction: a randomized clinical trial. JAMA. 2021;325(6):542–51.
Moher D, Shamseer L, Clarke M, Ghersi D, Liberati A, Petticrew M, et al. Preferred Reporting Items for Systematic Review and Meta-Analysis Protocols (PRISMA-P) 2015 statement. Syst Rev. 2015;4(1):1.
Pieske B, Tschöpe C, de Boer RA, Fraser AG, Anker SD, Donal E, et al. How to diagnose heart failure with preserved ejection fraction: the HFA–PEFF diagnostic algorithm: a consensus recommendation from the Heart Failure Association (HFA) of the European Society of Cardiology (ESC). Eur Heart J. 2019;40(40):3297–317.
McGowan J, Sampson M, Salzwedel DM, Cogo E, Foerster V, Lefebvre C. PRESS Peer Review of Electronic Search Strategies: 2015 guideline statement. J Clin Epidemiol. 2016;75:40–6.
Ouzzani M, Hammady H, Fedorowicz Z, Elmagarmid A. Rayyan—a web and mobile app for systematic reviews. Syst Rev. 2016;5(1):210.
BMJ Sterne JAC, Savović J, Page MJ, Elbers RG, Blencowe NS, Boutron I, et al. RoB 2: a revised tool for assessing risk of bias in randomised trials. BMJ. 2019;366:l4898.
Higgins JP, Thompson SG, Deeks JJ, Altman DG. Measuring inconsistency in meta-analyses. BMJ (Clinical research ed). 2003;327(7414):557–60.
Langan D, Higgins JPT, Simmonds M. Comparative performance of heterogeneity variance estimators in meta-analysis: a review of simulation studies. Research synthesis methods. 2017;8(2):181–98.
R Development Core Team. R: a language and environment for statistical computing. Vienna: R Foundation for Statistical Computing; 2021.
Google Scholar
Egger M, Davey Smith G, Schneider M, Minder C. Bias in meta-analysis detected by a simple, graphical test. BMJ (Clinical research ed). 1997;315(7109):629–34.
Guyatt GH, Oxman AD, Vist GE, Kunz R, Falck-Ytter Y, Alonso-Coello P, Schünemann HJ. GRADE: an emerging consensus on rating quality of evidence and strength of recommendations. BMJ (Clinical research ed). 2008;336(7650):924–6.
Download references
Acknowledgements
Not applicable.
Open access funding provided by University of Basel This study was supported by the Swiss National Science Foundation (Grant number: 185217) and the Department of Sport, Exercise and Health of the University of Basel, Division of Sports and Exercise Medicine, Switzerland.
Author information
Raphael Schoch and Benedikt Gasser are equal first authors.
Authors and Affiliations
Department of Sport, Exercise and Health, Division of Sport and Exercise Medicine, University of Basel, Grosse Allee 6, 4052, Basel, Switzerland
Raphael Schoch, Benedikt Gasser, Philippe Beck & Arno Schmidt-Trucksäss
University Medical Library, University of Basel, 4051, Basel, Switzerland
Christian Appenzeller-Herzog
Department of Clinical Research, University of Basel, 4031, Basel, Switzerland
Arno Schmidt-Trucksäss
You can also search for this author in PubMed Google Scholar
Contributions
BG and RS conceptualised the study. CA-H and RS designed the search string. BG and RS wrote the protocol. AST, CA-H, and PB critically revised it. CA-H was responsible for the search strategy. CA-H and BG designed the data synthesis strategy. BG registered the protocol in PROSPERO. PB and RS will conduct the systematic review and BG and RS the meta-analysis. The final manuscript will be written by BG and RS. All authors read and approved the final protocol.
Corresponding author
Correspondence to Arno Schmidt-Trucksäss .
Ethics declarations
Ethics approval and consent to participate, consent for publication, competing interests.
The authors declare that they have no competing interests.
Additional information
Publisher's note.
Springer Nature remains neutral with regard to jurisdictional claims in published maps and institutional affiliations.
Rights and permissions
Open Access This article is licensed under a Creative Commons Attribution 4.0 International License, which permits use, sharing, adaptation, distribution and reproduction in any medium or format, as long as you give appropriate credit to the original author(s) and the source, provide a link to the Creative Commons licence, and indicate if changes were made. The images or other third party material in this article are included in the article's Creative Commons licence, unless indicated otherwise in a credit line to the material. If material is not included in the article's Creative Commons licence and your intended use is not permitted by statutory regulation or exceeds the permitted use, you will need to obtain permission directly from the copyright holder. To view a copy of this licence, visit http://creativecommons.org/licenses/by/4.0/ . The Creative Commons Public Domain Dedication waiver ( http://creativecommons.org/publicdomain/zero/1.0/ ) applies to the data made available in this article, unless otherwise stated in a credit line to the data.
Reprints and permissions
About this article
Cite this article.
Schoch, R., Gasser, B., Beck, P. et al. Effects of exercise training on cardiac output in subjects with heart failure with preserved ejection fraction (HFpEF) — a protocol for a systematic review and meta-analysis. Syst Rev 13 , 159 (2024). https://doi.org/10.1186/s13643-024-02529-w
Download citation
Received : 15 August 2023
Accepted : 11 April 2024
Published : 18 June 2024
DOI : https://doi.org/10.1186/s13643-024-02529-w
Share this article
Anyone you share the following link with will be able to read this content:
Sorry, a shareable link is not currently available for this article.
Provided by the Springer Nature SharedIt content-sharing initiative
- Heart failure with preserved ejection fraction (HFpEF)
- Cardiac output (CO)
- Systematic review
- Meta-analysis
Systematic Reviews
ISSN: 2046-4053
- Submission enquiries: Access here and click Contact Us
- General enquiries: [email protected]
- Open access
- Published: 07 June 2024
The association between empathy and burnout in medical students: a systematic review and meta-analysis
- P. Cairns 1 ,
- A. E. Isham 2 &
- R. Zachariae 1 , 3
BMC Medical Education volume 24 , Article number: 640 ( 2024 ) Cite this article
309 Accesses
Metrics details
Burnout levels in medical students are higher than in other student groups. Empathy is an increasingly desired outcome of medical schools. Empathy is negatively associated with burnout in physicians. Our objective was to quantitatively review the available literature on associations between empathy and burnout in medical students, and to explore associations between specific empathy aspects (cognitive and affective) and burnout sub-dimensions (emotional exhaustion, depersonalization and personal accomplishment).
A comprehensive search of the literature published up until January 2024 was undertaken in the PubMed, EMBASE, CINAHL, The Cochrane Library, and PsycINFO databases. Two independent reviewers screened 498 records and quality-rated and extracted data from eligible studies. The effect size correlations (ESr) were pooled using a random-effects model and between-study variation explored with meta-regression. The review was preregistered with PROSPERO (#CRD42023467670) and reported following the PRISMA guidelines.
Twenty-one studies including a total of 27,129 medical students published between 2010 and 2023 were included. Overall, empathy and burnout were negatively and statistically significantly associated (ESr: -0.15, 95%CI [-0.21; -0.10], p < .001). When analyzing sub-dimensions, cognitive empathy was negatively associated with emotional exhaustion (ESr: -0.10, 95%CI [-0.17; -0.03], p = .006) and depersonalization (ESr: -0.15, 95%CI [-0.24; 0.05], p = .003), and positively associated with personal accomplishment (ESr: 0.21, 95%CI [0.12; 0.30], p < .001). Affective empathy was not statistically significantly associated with emotional exhaustion, depersonalization or personal accomplishment. Supplementary Bayesian analysis indicated the strongest evidence for the positive association between cognitive empathy and personal accomplishment. Response rate and gender moderated the relationship so that higher response rates and more male respondents strengthen the negative association between empathy and burnout.
Greater empathy, in particular cognitive empathy, is associated with lower burnout levels in medical students. This appears to be primarily driven by cognitive empathy's positive association with personal accomplishment.
Protocol registration
#CRD42023467670
Peer Review reports
The purpose of this systematic review and meta-analysis was to examine the relationship between empathy and burnout in medical students. Burnout, defined as a state of emotional exhaustion, depersonalization, and a reduced sense of personal accomplishment [ 1 ], has become a pervasive issue within the medical field. One systematic review and meta-analysis of 4,664 international medical residents reported an overall burnout prevalence rate of 35.7% [ 2 ]. In US physicians, reports indicate a prevalence of burnout of 37.9%, compared to 27.8% in the general population [ 3 ]. Research indicates that burnout prevalence rates in medical students range from 7 to 75.2%, depending on the country in which the study was carried out, the instruments used and the cutoff-criteria for burnout symptomatology [ 4 ], with an overall suggested prevalence rate of 37.23% [ 5 ]. One US study found that 49.6% of medical students may experience burnout, compared to 35.7% of U.S. college graduates aged 22 to 32 [ 6 ]. Prospective studies suggest that burnout may increase from the first year of medical school to the final year [ 7 , 8 ].
Burnout among medical students has been found to be associated with poorer academic performance, increased rates of substance abuse, and impaired mental health, which could impact future physicians' ability to provide high quality, compassionate patient care [ 9 ]. Individual studies suggest that burnout may also be negatively associated with medical student empathy [ 10 ] .
Although multiple definitions of empathy have been suggested [ 11 ], it is generally considered to have three dimensions: cognitive empathy in which physicians use their cognitive abilities to take the perspective of their patient, an affective component in which physicians feel the emotions they believe their patient is experiencing, and a behavioral component in which the physicians communicate their understanding [ 12 ]. A consensus has grown around the definition of therapeutic empathy in recent years, defined as ' a physician's ability to understand the patient, communicate that understanding and act upon it in a therapeutic way. ' [ 13 ]. This definition prioritizes the cognitive understanding of the patient over the affective feeling of their emotions [ 14 ].
Medical students are increasingly expected to use and develop empathy as part of their medical education, as shown in curriculums specifically highlighting communication skills and empathy [ 15 , 16 ]. This is important given that empathetic healthcare consultations are associated with increased physician-patient trust [ 17 ], improved psychological and physical patient outcomes [ 18 , 19 ], and an increase in patient satisfaction by lowering patient anxiety and distress [ 19 , 20 ].
Therapeutic empathy may have benefits for physicians as well as patients. One systematic review of healthcare workers found negative associations between empathy and subdimensions of burnout: emotional exhaustion, depersonalization and reduced personal accomplishment [ 21 ]. Specifically, the authors found a negative association between perspective-taking (cognitive empathy) and depersonalization, and a positive association between perspective-taking and personal accomplishment. Similarly, they found a negative association between empathic concern (affective empathy) and depersonalization and a positive association between empathic concern and personal accomplishment. Emotional exhaustion was not related to either perspective-taking or empathic concern. Potential moderators were not explored in this systematic review, but a number of individual studies have suggested that gender [ 22 ] and age [ 23 ], amongst other variables, are also associated with empathy and burnout with the possibility of acting as moderators. Empathic concern (affective empathy) is higher in women compared to men, and perspective-taking (cognitive empathy) is higher in women and younger people compared to men and older people [ 23 ].
Despite having similarly high burnout levels to physicians, no systematic review and meta-analysis has explored the possible association between empathy and burnout in medical students. Given the high levels of burnout in medical students and an increasing focus on empathic skills in medical education, the aim of this systematic review and meta-analysis was to explore the possible association between empathy and burnout in medical students. This knowledge could be important for developing preventive strategies to avoid burnout, maintaining the mental health of medical students, promoting medical career sustainability and ensuring quality of care for present and future patients.
Purpose of the study
This systematic review and meta-analysis aimed to provide a quantitative synthesis of the existing literature on the relationship between empathy and burnout in medical students. We addressed the following research questions:
What is the overall association between empathy and burnout in medical students?
Are specific dimensions of empathy (cognitive, emotional, behavioral) differentially related to specific burnout dimensions (emotional exhaustion, depersonalization, reduced personal accomplishment)?
What moderating factors may influence the relationship between empathy and burnout in medical students (e.g. gender, age, study level, region)?
The protocol for the present study was preregistered in the International Prospective Register of Systematic Reviews (PROSPERO) (#CRD42023467670) [ 24 ]. The present study deviates from the protocol in the following ways: a) due to an existing systematic review on healthcare workers, physicians were no longer included as a population of interest, and b) due to a lack of studies, compassion fatigue was not included as a focus of this review. The review was conducted in accordance with the guidelines for Meta-Analysis Of Observational Studies in Epidemiology (MOOSE) [ 25 ] and is reported in line with the Preferred Reporting Items for Systematic Reviews and Meta-Analyses (PRISMA) guidelines [ 26 ].
Search strategy and selection criteria
A comprehensive literature search was conducted on September 22, 2023 as per protocol, with an updated search conducted on January 10, 2024. The updated search only included medical students because physicians were no longer a population of interest. No publication date restrictions were applied. The electronic databases searched were: PubMed, EMBASE, CINAHL, The Cochrane Library, and PsycINFO. Where possible, relevant MeSH (Medical Subject Headings) terms or MeSH term equivalents were included in each database search. The specific search terms were: medical student* AND empathy AND burnout.
The study inclusion criteria were guided by the PICO (Population, Intervention/Exposure, Comparator, Outcome) approach [ 27 ]. Population: Medical students; Exposure: Medical school; Comparator: N/A; Outcome: Empathy and burnout assessed with a standardized, validated measurement scale. The study exclusion criteria were Population: Non-medical students; Exposure: Non-medical school; Comparator: N/A; Outcome: Non-empathy and burnout measures. Empathy and burnout assessed with non-standardized, unvalidated measurement scales.
We included correlational studies, including cross-sectional and longitudinal, prospective survey-based studies. Furthermore, only English-language papers published in peer-reviewed journals were considered eligible. We chose not to include non-English papers, as this might introduce biases related to language, publication bias, methodological heterogeneity, and challenges in access and quality assessment. While inclusivity is important, the potential for bias introduced by non-English papers outweighs the benefits of attempting a more comprehensive review. We excluded randomized controlled trials (RCTs), qualitative studies, case studies, open trials, uncontrolled trials, reviews and study protocols. The reason for this was that they were not designed to collect correlational data and test correlational hypotheses, or that they do not provide quantitative data. Additionally, including data from trials, e.g., baseline or control group data, may provide less generalizable data due to often highly selected study samples and that data are likely to be influenced by the experimental setup of such trials. Grey literature, for example, conference abstracts, trial registrations, dissertations and studies with N<10 was also not considered eligible.
The literature search and data extraction were conducted using the Covidence systematic review software [ 28 ]. In the first round of screening, PC and AEI independently screened the title and abstract of all identified references and excluded ineligible studies. In the second round of screening, the full text of the remaining studies were evaluated independently by PC and AEI and reasons for exclusion were registered. After each screening, the two authors discussed discrepancies, and reached a negotiated decision. Uncertainties and disagreements were discussed with the last author (RZ).
Quality assessment
A methodological quality assessment was undertaken independently by two authors (PC and AEI) for all included studies, using the National Institutes of Health Quality Assessment Tool for Observational Cohort and Cross-Sectional Studies [ 29 ]. The quality terms included whether the studies had a clearly defined research question and study population, whether the participation rate of eligible participants was at least 50%, whether the subjects were recruited from the same or similar populations, whether inclusion and exclusion criteria were used for all participants, whether a sample size justification such as a power description was provided, and if key confounding variables were measured and adjusted for statistically, among other questions.
Data extraction
Data extraction was performed independently by two authors (PC and AEI) and included authors, publication year, empathy aspect (cognitive, affective or behavioral), burnout dimension (emotional exhaustion, depersonalization or personal accomplishment), correlation statistic (Pearson's r , Spearman's ρ , or standardized β values), sample size, effect direction, whether the correlation was adjusted for other covariates (yes or no), number of covariates, study design (cross-sectional or longitudinal), response rate (as percentage), whether the relationship between empathy and burnout in medical students was the primary focus of the study (yes or no), sampling method (convenience, random), sample mean age, gender of participants (percent women), country, region (e.g., North America, Middle East, Europe, Asia), study level (early, late, or mixed), empathy scale, empathy subscale, burnout scale and burnout subscale. A meta-analysis was conducted when a minimum of three studies assessing an association between an empathy and a burnout dimension were available.
Categorization of empathy and burnout data
Empathy in the context of medical education is a multidimensional construct that encompasses cognitive, emotional, and behavioral components [ 12 ]. Cognitive empathy refers to the ability to understand the thoughts and perspectives of others, emotional empathy involves feeling and sharing the emotions of others, and behavioral empathy entails demonstrating empathetic behaviors, such as active listening and providing emotional support [ 30 ]. In the caring professions, 11 empathy measurement tools are available [ 31 ]. In order to make a meta-analysis possible and reduce the complexity of the findings, all empathy questionnaires were categorized as measuring either cognitive, affective or behavioral empathy. The study characteristics table (Table 1 ) provides the measurement tool used in each study and the empathy aspect categorized by the authors.
Burnout among medical students is usually conceptualized within the framework of the Maslach Burnout Inventory (MBI), which identifies three key dimensions: emotional exhaustion, depersonalization, and reduced personal accomplishment [ 1 ]. Emotional exhaustion refers to feelings of fatigue and emotional depletion, depersonalization involves cynicism and detachment from patients, and reduced personal accomplishment reflects a diminished sense of personal achievement and competence [ 1 ]. However, at least four other measurement tools for occupational burnout exist [ 51 ] with various dimensions, broadly aligning with the three dimensions of the MBI. All burnout questionnaires were categorized as measuring either emotional exhaustion, depersonalization or personal accomplishment. The study characteristics table (Table 1 ) provides the measurement tool used in each study and the burnout dimensions it was categorized as covering.
The three dimensions of burnout have different directions. For emotional exhaustion and depersonalization, greater scores signify greater burnout. For personal accomplishment, higher scores indicate less burnout. Therefore, when examining the association between global empathy and global burnout, correlations between empathy and personal accomplishment were reverse scored. When calculating the associations between the specific aspects of empathy and the various dimensions of burnout, personal accomplishment was not reverse scored.
Meta-analytic strategy
The effect size correlation (ESr) was used as the standardized effect size for the association between empathy and burnout. If correlations were not reported directly, ESr was converted from other data, for example, differences between means and standard deviations, regression coefficients, numbers or rates of study participants in relevant groups, χ 2 , F , or t statistics using various formulas. The calculations were conducted independently by two authors (PC and AEI) and checked by a third author (RZ) in case of disagreement. In case of missing data from the published report, the data was requested from the authors.
Effect sizes were calculated for both the unadjusted bivariate associations and the associations found in multivariate analyses adjusted for other covariates. Correlations between global empathy and global burnout were operationalized as the averaged correlations across the various individual dimensions for each study. The ESr was also used as a standardized effect size for the association between the different aspects of empathy (cognitive, affective and behavioural) and the dimensions of burnout (emotional exhaustion, depersonalization and personal accomplishment). The pooled effect size (ESr) and its 95% confidence interval was calculated using a random effects model. Heterogeneity was explored by calculating the I 2 statistic. The I 2 statistic is an estimate of the variance in a pooled ES that is accounted for by heterogeneity, i.e., true differences between effect sizes rather than sampling error [ 52 ]. We also calculated Tau ( T ), which represents the standard deviation of the true effect sizes, and the 95% prediction interval. The prediction interval takes both the random error and the systematic variance into consideration and quantifies the distribution of the ESs, indicating the range that 95% of results of future studies (from the same family of studies) are expected to fall within [ 53 ].
Publication bias, i.e., the tendency for statistically significant findings in the hypothesized direction to be more likely to be published, is a widespread problem in meta-analyses [ 54 ]. Although publication bias may be less likely in correlational than effect studies, we explored the possibility of using funnel plots and Egger's tests [ 55 ], but only when K > 10 (K = number of studies) [ 54 ]. If the results were suggestive of possible publication bias, we planned a sensitivity analysis adjusting the effect sizes using the Duval and Tweedie trim-and-fill method [ 56 ].
Possible sources of heterogeneity were explored with moderator analyses. When data were available for 10 independent samples or more, the possible influence of both continuous moderators (i.e., mean sample age, percent of women in the sample, response rate) and dichotomous moderators (i.e., student study level (late vs. early) and study quality (good or fair vs. poor)) were analyzed with meta-regression (computational model: maximum likelihood). For dichotomous moderators, the variable had to show sufficient variability, i.e. K > 3 in the smallest category. The R 2 equivalent was calculated for moderators reaching statistical significance.
All analyses were conducted using Comprehensive Meta-Analysis v4 [ 57 ] and various formulas in Microsoft Excel.
Supplementary Bayesian analyses
To aid the interpretation of the results, a Bayesian Model-Averaged meta-analysis [ 58 ] was conducted. The procedure examines the results of four models: a) Fixed-effect null hypothesis (fH 0 ), b) fixed-effect alternative hypothesis (fH 1 ), c) random-effects null hypothesis (rH 0 ), and d) random effects alternative hypothesis (rH 1 ). Bayesian Model-Averaged analysis thus avoids selecting either a fixed- or random-effects model and addresses two questions considering the observed data: What is the plausibility that the overall effect is non-zero and the ES are heterogeneous? An uninformed prior probability was chosen, i.e., 25%, of each of the four models, and 2000 iterations were used. With regard to parameter distributions, previously recommended defaults were chosen [ 58 ]. Thus, a zero-centered Cauchy prior with a scale of 0.707 for the ES was used. For the between-study variation, an empirically informed prior distribution on non-zero between-study deviation estimates based on standardized mean difference ESs from 705 meta-analyses published in Psychological Bulletin between 1990 and 2013 was used [ 59 ]. This distribution has been approximated by an Inverse-Gamma (1, 0.15) prior on the standard deviation (Tau) [ 58 ]. For each analysis, we calculated the Bayes Factor (BF) [ 60 ], which represents the posterior probability of the alternative hypothesis (H 1 ) relative to the probability of the null hypothesis. Based on the BF, the strength of the evidence was then categorized as "weak", “moderate”, “strong”, “very strong”, and “decisive” [ 61 ]. The Bayesian analyses were conducted with the computer software JASP (Version 0.17.1) [ 62 ].
Search results
A total of 498 articles were identified through digital database searches. After removal of duplicates, a total of 311 records were eligible for title and abstract screening. A total of 265 records were excluded after screening, leaving 46 articles eligible for full text screening. 25 articles were then excluded primarily due to “not responding to request for additional data” (68%), leaving 21 papers that were included in the systematic review. Ninety percent agreement was achieved by reviewers during the abstract review process and 95% agreement during full-text screening. All disagreements were resolved through negotiation. This negotiation involved the two reviewers PC and AEI providing the reason for their decision to include or exclude the relevant study. These reasons were then double-checked by both PC and AEI reviewing the individual study collaboratively. This led to agreement on whether to include or exclude the study in question. The study selection process is visualized in Fig. 1 . Authors of 25 studies were contacted and asked to provide additional data. Seven authors replied and provided the requested data.
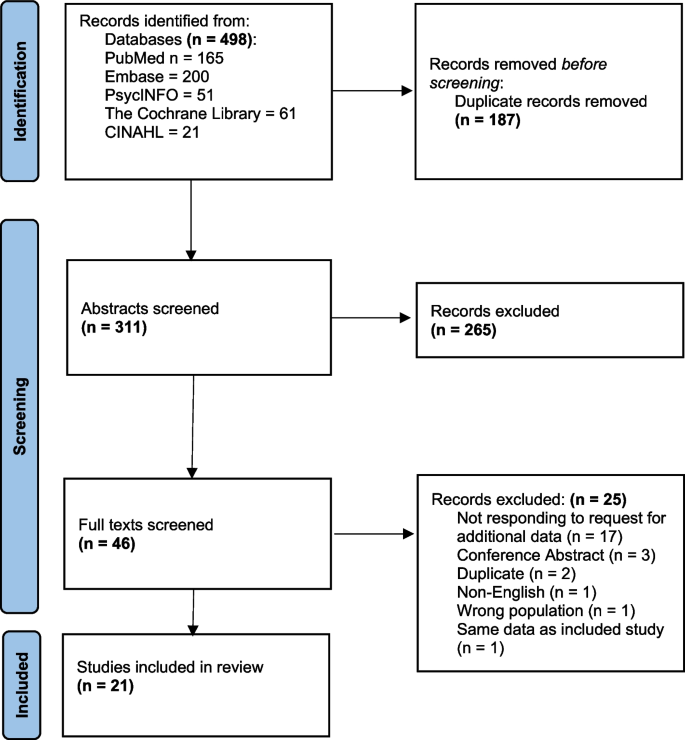
Preferred Reporting Items for Systematic Reviews and Meta-Analyses diagram
Study characteristics
The participant characteristics, the empathy and burnout dimensions examined, the measurement tools used, and other characteristics of the included studies are summarized in Table 1 . The identified studies reported on 21 independent samples including a total of 27,129 medical students, with sample sizes ranging from 76 [ 47 ] to 14,126 [ 41 ]. The included articles were published between 2010 and 2023. The studies were broadly geographically distributed, with 7 studies conducted in North America, 4 in Asia, 4 in Europe, 3 in South America, 2 in Oceania, and 1 in the Middle East. Mean sample ages ranged from 19.9 [ 50 ] to 27.7 [ 41 ] years, with an overall weighted mean sample age of 25.2 years. The majority (90.4%) of the studies ( K = 19) used a cross-sectional design, and 9.6% ( K = 2) used a longitudinal design. As the majority of studies employed cross-sectional surveys across multiple study years, it was not possible to construct a continuous study year variable. We, therefore, categorized the study year as either early (years 1-3), late (years 4-6+), or mixed (1-6+).
Quality rating
See supplementary table S1 for an overview of the quality ratings of each study. Two studies were assessed to be of good quality (>9 criteria met), and 19 studies to be of fair quality (5 to 9 criteria met). Studies received high ratings when they presented a clear definition of the research question and study population, when there was a sufficient timeframe between longitudinal measurements, and when a sample size justification, i.e., statistical power calculation, was reported.
Overall association between empathy and burnout
The most commonly used scale to assess empathy was the Jefferson Scale of Empathy - Student version (K = 8), followed by the Interpersonal Reactivity Index (K = 7). The most commonly used scale to assess burnout was the Maslach Burnout Inventory – Student version (K = 5) and the Oldenburg Burnout Inventory (K = 5), followed by the Maslach Burnout Inventory – General Survey (K = 4) and the Maslach Burnout Inventory – Human Services Scale (K = 4). As mentioned above, empathy was characterized as having cognitive, affective, and behavioral components. Due to a lack of studies (K = 1), the behavioral aspect of empathy was not explored in this meta-analysis.
As shown in Table 2 and Fig. 2 , global empathy was negatively associated with global burnout in medical students, with the pooled correlation corresponding to a small effect size [ 63 ]. The supplementary Bayesian analysis indicated that, based on the available evidence, the alternative hypothesis, i.e., that the association between global empathy and global burnout is non-zero, was approximately 35 times more likely than the null hypothesis, corresponding to “very strong evidence” [ 61 ].
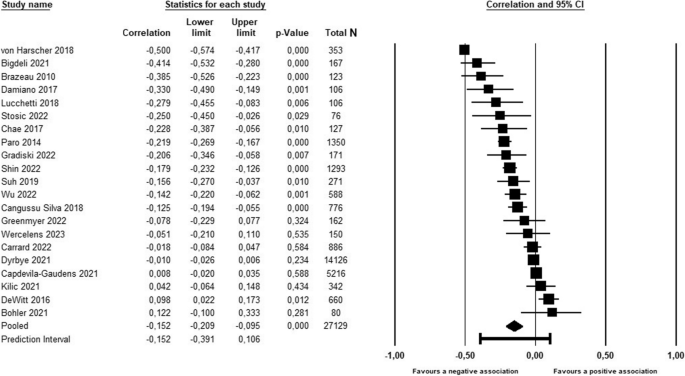
Forest plot of the association between empathy global and burnout global
As seen in Table 2 , the associations between global empathy and the three subcomponents of burnout all reached statistical significance. Again, the correlations were of small magnitude (ESr -0.19 to 0.20) and in the expected directions, with negative associations between global empathy and emotional exhaustion and depersonalization, and a positive association between global empathy and personal accomplishment. While there was “very strong evidence” for depersonalization and personal accomplishment, the results of the Bayesian analysis favored the null hypothesis for emotional exhaustion, albeit only with moderate level of evidence.
Associations between sub-dimensions of empathy and burnout
As shown in Table 2 , the associations between cognitive empathy and the three sub-dimensions of burnout all reached statistical significance. Again, the correlations were of small magnitude (ESr -0.10 to 0.21) and in the expected directions, with negative associations between cognitive empathy and emotional exhaustion and depersonalization, and a positive association between cognitive empathy and personal accomplishment. While there was “decisive evidence” for personal accomplishment and “weak evidence” for depersonalization, the results of the Bayesian analysis favored the null hypothesis for emotional exhaustion, albeit only with weak level of evidence.
As seen in Table 2 , the associations between affective empathy and the three sub-dimensions of burnout did not reach statistical significance. The results of the Bayesian analysis favored the null hypothesis with “strong evidence” for emotional exhaustion, “moderate evidence” for depersonalization, and “weak evidence” for personal accomplishment.
Publication bias
When examining the overall results, i.e., the association between global empathy and global burnout, we found no clear indications of possible publication bias. When inspecting the funnel plot (See supplementary materials, Figure S1), it did not appear particularly skewed, and neither Egger’s regression test ( p = 0.311) nor the rank correlation tests for Funnel plot asymmetry ( p = 0.740) reached statistical significance.
Heterogeneity
As shown in Table 2 , the results were characterized by considerable heterogeneity, with I 2 values ranging from 91.9% to 95.9%. This suggests that very high proportions of the variation in the correlations between empathy and burnout are explained by systematic, i.e., non-random, between-study differences. Based on the variation of the true values, the prediction intervals, i.e., the range of values that the results of 95% of future similar studies are expected to fall within, were wide for most association estimates.
Moderating variables
As seen in Table 3 , when exploring the potential sources of heterogeneity, the percentage of women in the sample and the response rate were the most consistent statistically significant moderators of the associations between empathy and burnout, explaining between 25% and 73% of the variation. The positive slopes found for the percentage of women and the associations between global empathy and global burnout and depersonalization, and the negative slope found for the association between global empathy and personal accomplishment, indicates that stronger negative associations between empathy and burnout were found in samples with fewer women, i.e., more men. The slopes found for response rates indicated that stronger associations in the expected direction between global empathy and two of the burnout dimensions were found in studies with higher response rates. The results for the remaining moderators, i.e., sample mean age, student study level (early vs. late), and study quality (good or fair vs. poor) did either not reach statistical significance or could not be analyzed due to insufficient data.
The purpose of this systematic review and meta-analysis was to examine the relationship between empathy and burnout in medical students. We found a negative, statistically significant association between empathy and burnout in medical students, with a small effect size. This relationship appeared to be primarily driven by cognitive empathy, which was negatively associated with emotional exhaustion and depersonalization and positively associated with personal accomplishment. Affective empathy was not statistically significant with any of the burnout sub-dimensions. Gender moderated the relationship between empathy and burnout such that the negative relationship between empathy and burnout was stronger in samples with more men. Finally, the response rates of included studies also moderated the relationship between empathy and burnout such that stronger, negative association between empathy and depersonalization and a stronger, positive association between empathy and personal accomplishment were found in studies with higher response rates. Sample mean age, student study level, and study quality either did not reach statistical significance or could not be analyzed due to insufficient data.
These results have some shared findings with that of a systematic review and meta-analysis examining empathy and burnout in healthcare workers, specifically doctors and nurses [ 21 ]. In this population, shared findings with our results included a negative association between perspective taking (cognitive empathy) and depersonalization, and a positive association with personal accomplishment. Furthermore, they also found no association between empathic concern (affective empathy) and emotional exhaustion. Some results from this population differed to ours. They did not find a significant association between perspective taking (cognitive empathy) and emotional exhaustion, which we did albeit with a Bayes Factor below 1 indicating weak support for the null hypothesis (no association between cognitive empathy and emotional exhaustion). Furthermore, empathic concern (affective empathy) was significantly negatively associated with depersonalization and significantly positively associated with personal accomplishment in their sample, whereas our sample showed no association between affective empathy and any burnout subscale.
The lack of associations or small effect size correlations between emotional exhaustion and cognitive and affective empathy which both we and the review including doctors and nurses [ 21 ] present, suggest that emotional exhaustion may not be influenced so much by empathy-related factors, but perhaps things such as high workloads and lack of sleep [ 64 ]. Possible explanations for differences between our findings could be that there are differences between how medical students’ and healthcare workers’ affective empathy and burnout interact, or that our use of multiple affective empathy scales compared to their use of the IRI only impacted the results.
Given that empathy involves other-orientated processes, including considering the other person’s perspective and feeling the emotions that the other may be experiencing, the negative association between empathy and depersonalization is less surprising.
Given that cognitive empathy involves taking the other person’s perspective, one could have expected that the main driver of the negative association between cognitive empathy and burnout was a negative association with depersonalization (viewing people as objects rather than human beings). Although this negative association was statistically significant, the main driver of cognitive empathy’s negative association with burnout was clearly a positive association with personal accomplishment. One explanation for this could be that by using cognitive empathy, medical students may be able to give the patients they encounter more personalized care plans that suit the patient’s life situation and values, as well as creating rapport and a sense of trust [ 65 ]. Medical students may perceive this as clinical competence, especially if they pass communication-based assessments such as OSCEs [ 66 ] as a result of this, and feel a sense of personal accomplishment. This greater sense of personal accomplishment may contribute to feeling less burnt out.
The finding that gender moderated the relationship between empathy and burnout such that the negative relationship between empathy and burnout was stronger in samples with more men, is a novel finding. Evidence suggests that both burnout and empathy levels are higher in women, yet the authors of the present study did not note any ceiling effect in women’s empathy or burnout scores in the eligible studies, or greater variability in men’s empathy or burnout scores which could explain gender’s moderating effect. One possible explanation is that women’s empathy is more robust so that that they can maintain higher empathy even whilst experiencing higher burnout, but further research is warranted.
The non-significant findings of this meta-analysis are also of interest. Affective empathy was not statistically significantly related to any of the burnout sub-dimensions. The lack of association between affective empathy and emotional exhaustion provided the strongest support for the null hypothesis of any association analyzed in this meta-analysis, as shown by a Bayes Factor approaching zero. These results do not support the idea that higher affective empathy is associated with higher emotional exhaustion or lower emotional exhaustion. Affective empathy was primarily measured using the Empathic Concern component of the Interpersonal Reactivity Index, which assesses an individual’s ‘ feeling for ’ another individual [ 67 ]. Other questionnaires assessing affective empathy included the Toronto Empathy Questionnaire, and subscales of the Empathy Quotient, the Questionnaire of Cognitive and Affective Empathy, and the Basic Empathy Questionnaire. These questionnaires generally assess an individual’s ‘ feeling with ’ another individual [ 31 ]. The results related to affective empathy did not appear to depend on the questionnaire used or the conceptualization of affective empathy as ‘ feeling for’ or ‘ feeling with ’ another. However, the affective empathy questionnaires used in the included studies do not have the highest reliability and validity [ 31 ] and these associations had the highest heterogeneity scores. This provides some doubts in interpreting these results, and further research is warranted.
Clinical implications
Although the results of this review do not provide evidence of direct causal links between empathy and burnout, they do indicate that in situations where medical student empathy is high, burnout is highly likely to be low, and vice versa. Given that medical student burnout is associated with poorer academic performance, increased rates of substance abuse, and impaired mental health [ 9 ], and higher medical student empathy is associated with higher personal accomplishment and clinical competence [ 68 ], medical educators are advised to create learning environments which foster empathy and reduce burnout. By creating preventative strategies to avoid burnout, maintaining the mental health of medical students, incorporating empathy-enhancing curriculums and promoting medical career sustainability, they can ensure quality of care for present and future patients.
Recommendations for future research
The vast majority of studies present in the literature and available for analysis used self-reported empathy measures. Although these scales are helpful ways to measure internal empathic attitudes among medical students, patient-rated empathy scales can provide useful information on patients’ ability to detect these attitudes. Future studies could examine the associations between patient-rated empathy and burnout in medical students. It is also well-known that self-efficacy, i.e., the confidence in one’s ability to exert a certain behavior, is a reliable predictor of the actual behavior [ 69 ], and future studies could explore the possible associations between medical student empathy and their self-efficacy in exhibiting patient-centered behaviors in the interaction with patients [ 70 ].
The behavioral component of empathy was not analyzed due to a lack of studies measuring it (K = 1) but could provide useful information on the expression of medical student internal empathic attitudes. Future studies could examine the associations between behavioral empathy and burnout in medical students. It was also not possible to examine study year as a moderating variable as studies did not report results from single year groups. Given that empathy may decline as medical students progress through medical school and burnout may increase [ 7 , 8 ], it may of use to observe the correlation between these two variables year-by-year.
Study limitations
Some study limitations should be noted. First, the high I 2 values suggest that a large proportion of the variation in results stems from underlying systematic differences between the available studies, rather than random error. While we identified two possible sources of the between-study variation, i.e., gender and response rates, the remaining included moderators either failed to explain a significant proportion of the variation or the data were not sufficient to conduct an analysis. Second, the included studies had assessed empathy and burnout with a range of different scales, diminishing across-study comparability and increasing between-study variability. Despite the fact that the most valid, standardized empathy measurement scales were used, the validity and reliability of these scales are still the topic of debate [ 31 ]. Thirdly, the majority of the studies included in this meta-analysis were cross-sectional in their study design and used bivariate correlations. Finally, burnout can be conceptualized in different ways, and it is possible that our merging of different concepts into one of the three MBI sub-dimensions: emotional exhaustion, depersonalization and personal accomplishment, may have reduced their explanatory power. For example, we combined the OBLI’s ‘disengagement’ sub-dimension with the MBI’s ‘depersonalization’. However, when rerunning the analysis separately for disengagement and depersonalization, it did not affect the results.
Due to only one study measuring behavioral empathy, we were not able to analyze its association with burnout. Further studies which categorize behaviors such as active listening and addressing patient emotions as behavioral empathy and analyze the association between these behaviors and burnout are required.
Our study is the first systematic review and meta-analysis to examine the association between empathy and burnout in medical students. Our results confirm an overall negative relationship between empathy and burnout in medical students. Furthermore, cognitive empathy appears to be negatively associated with the burnout sub-dimensions of emotional exhaustion and depersonalization and most robustly positively associated with personal accomplishment. Affective empathy was not consistently associated with any of the burnout sub-dimensions. Future research should examine which modifiable parts of the medical learning environment could be altered to lower burnout and foster empathy.
Availability of data and materials
Data is provided within the manuscript or supplementary information files. For extraction sheets, please contact the author Patrick Cairns [email protected]
Abbreviations
Effects size correlations
The International Prospective Register of Systematic Reviews
The guidelines for Meta-Analysis Of Observational Studies in Epidemiology
Preferred Reporting Items for Systematic Reviews and Meta-Analyses
Medical Subject Headings
Population, Intervention/Exposure, Comparator, Outcome
Maslach Burnout Inventory
Bayes Factor
Objective, structured, clinical examination
Maslach C, Schaufeli WB, Leiter MP. Job burnout. Ann Rev Psychol. 2001;52(1):397–422.
Article Google Scholar
Rodrigues H, Cobucci R, Oliveira A, Cabral JV, Medeiros L, Gurgel K, Souza T, Gonçalves AK. Burnout syndrome among medical residents: A systematic review and meta-analysis. PloS One. 2018;13(11):e0206840.
Shanafelt TD, Boone S, Tan L, Dyrbye LN, Sotile W, Satele D, West CP, Sloan J, Oreskovich MR. Burnout and satisfaction with work-life balance among US physicians relative to the general US population. Arch Intern Med. 2012;172(18):1377–85.
Erschens R, Keifenheim KE, Herrmann-Werner A, Loda T, Schwille-Kiuntke J, Bugaj TJ, Nikendei C, Huhn D, Zipfel S, Junne F. Professional burnout among medical students: systematic literature review and meta-analysis. Med Teach. 2019;41(2):172–83.
Almutairi H, Alsubaiei A, Abduljawad S, Alshatti A, Fekih-Romdhane F, Husni M, Jahrami H. Prevalence of burnout in medical students: A systematic review and meta-analysis. Int J Soc Psychiatry. 2022;68(6):1157–70.
Dyrbye LN, West CP, Satele D, Boone S, Tan L, Sloan J, Shanafelt TD. Burnout among US medical students, residents, and early career physicians relative to the general US population. Acad Med. 2014;89(3):443–51.
Ranasinghe PD, Owusu JT, Bertram A, Michtalik H, Yeh HC, Cofrancesco J Jr, Levine D, Miller ER III, Marinopoulos S. Depressive symptoms and burnout among medical students: a prospective study. J Gen Intern Med. 2022;37(1):64–9.
Ofei-Dodoo S, Moser SE, Kellerman R, Wipperman J, Paolo A. Burnout and other types of emotional distress among medical students. Med Sci Educ. 2019;29:1061–9.
IsHak W, Nikravesh R, Lederer S, Perry R, Ogunyemi D, Bernstein C. Burnout in medical students: a systematic review. Clin Teach. 2013;10(4):242–5.
Paro HB, Silveira PS, Perotta B, Gannam S, Enns SC, Giaxa RR, Bonito RF, Martins MA, Tempski PZ. Empathy among medical students: is there a relation with quality of life and burnout? PloS One. 2014;9(4):e94133.
Cuff BM, Brown SJ, Taylor L, Howat DJ. Empathy: a review of the concept. Emot Rev. 2016;8(2):144–53.
Nembhard IM, David G, Ezzeddine I, Betts D, Radin J. A systematic review of research on empathy in health care. Health Serv Res. 2023;58(2):250–63.
Mercer SW, Reynolds WJ. Empathy and quality of care. Br J Gen Pract. 2002;52(Suppl):S9-12.
Google Scholar
Howick J, Bizzari V, Dambha-Miller H. Therapeutic empathy: what it is and what it isn’t. J Royal Soc Med. 2018;111(7):233–6.
Venktaramana V, Loh EK, Wong CJ, Yeo JW, Teo AY, Chiam CS, Foo DJ, Teo F, Liang J, Raveendran V, Chng LC. A systematic scoping review of communication skills training in medical schools between 2000 and 2020. Med Teach. 2022;44(9):997–1006.
Winter R, Ward A, Norman RI, Howick J. A survey of clinical empathy training at UK medical schools. BMC Med Educ. 2023;23(1):1–3.
Kim SS, Kaplowitz S, Johnston MV. The effects of physician empathy on patient satisfaction and compliance. Eval Health Prof. 2004;27(3):237–51.
Howick J, Moscrop A, Mebius A, Fanshawe TR, Lewith G, Bishop FL, Mistiaen P, Roberts NW, Dieninytė E, Hu XY, Aveyard P. Effects of empathic and positive communication in healthcare consultations: a systematic review and meta-analysis. J Royal Soc Med. 2018;111(7):240–52.
Mistiaen P, Van Osch M, Van Vliet L, Howick J, Bishop FL, Di Blasi Z, Bensing J, Van Dulmen S. The effect of patient–practitioner communication on pain: a systematic review. Eur J Pain. 2016;20(5):675–88.
Derksen F, Bensing J, Lagro-Janssen A. Effectiveness of empathy in general practice: a systematic review. Br J Gen Pract. 2013;63(606):e76-84.
Delgado N, Delgado J, Betancort M, Bonache H, Harris LT. What is the link between different components of empathy and burnout in healthcare professionals? A systematic review and meta-analysis. Psychol Res Behav Manag. 2023;31:447–63.
Suh WW, Cho SH, Yoo JY, Kim HS, Song HR, Kim WJ, Lee SM, Hong M. Relationship between psychological correlates and empathy in medical students: a cross-sectional study. Psychiatry Investig. 2019;16(10):766.
Sommerlad A, Huntley J, Livingston G, Rankin KP, Fancourt D. Empathy and its associations with age and sociodemographic characteristics in a large UK population sample. PloS One. 2021;16(9):e0257557.
Booth A, Clarke M, Dooley G, Ghersi D, Moher D, Petticrew M, Stewart L. The nuts and bolts of PROSPERO: an international prospective register of systematic reviews. Syst Rev. 2012;1(1):1–9.
Stroup DF, Berlin JA, Morton SC, Olkin I, Williamson GD, Rennie D, Thacker SB. Meta-analysis of observational studies in epidemiology: a proposal for reporting. J Am Med Assoc. 2000;283(15):2008–12.
Page MJ, McKenzie JE, Bossuyt PM, Boutron I, Hoffmann TC, Mulrow CD, Shamseer L, Tetzlaff JM, Akl EA, Brennan SE, Chou R. The PRISMA 2020 statement: an updated guideline for reporting systematic reviews. Int J Surg. 2021;1(88):105906.
Sackett DL. Evidence-based medicine. InSeminars in perinatology. 1997 (Vol. 21, No. 1, p. 3–5). Philadelphia: WB Saunders.
Covidence systematic review software, Veritas Health Innovation, Melbourne, Australia. 2024
National Heart, Lung, and Blood Institute. Quality Assessment Tool for Observational Cohort and Cross-Sectional Studies. National Institutes of Health; 2014. https://www.nhlbi.nih.gov/health-topics/study-quality-assessment-tools .
Hojat M, Spandorfer J, Louis DZ, Gonnella JS. Empathic and sympathetic orientations toward patient care: conceptualization, measurement, and psychometrics. Acad Med. 2011;86(8):989–95.
Hong H, Han A. A systematic review on empathy measurement tools for care professionals. Educ Gerontol. 2020;46(2):72–83.
Bigdeli S, Arabshahi SK, Zazoly AZ, Norouzi A, Yazd NA, Hosseini AF. Empathy, quality of life and occupational burnout among medical students. Res Dev Med Educ. 2021;10(1):21.
Bohler TE, Brown RF, Dunn S. Relationship between affective state and empathy in medical and psychology students. Aust Psychol. 2021;56(4):311–23.
Brazeau CM, Schroeder R, Rovi S, Boyd L. Relationships between medical student burnout, empathy, and professionalism climate. Acad Med. 2010;85(10):S33-6.
Cangussu Silva A, Ezequiel OD, Damiano RF, Granero Lucchetti AL, DiLalla LF, Dorsey JK, Lucchetti G. Translation, transcultural adaptation, and validation of the empathy, spirituality, and wellness in medicine scale to the Brazilian Portuguese Language. Teach Learn Med. 2018;30(4):404–14.
Capdevila-Gaudens P, García-Abajo JM, Flores-Funes D, García-Barbero M, García-Estañ J. Depression, anxiety, burnout and empathy among Spanish medical students. PloS One. 2021;16(12):e0260359.
Carrard V, Bourquin C, Berney S, Schlegel K, Gaume J, Bart PA, Preisig M, Schmid Mast M, Berney A. The relationship between medical students’ empathy, mental health, and burnout: a cross-sectional study. Med Teach. 2022;44(12):1392–9.
Chae SJ, Jeong SM, Chung YS. The mediating effect of calling on the relationship between medical school students’ academic burnout and empathy. Korean J Med Educ. 2017;29(3):165.
Damiano RF, DiLalla LF, Lucchetti G, Dorsey JK. Empathy in medical students is moderated by openness to spirituality. Teach Learn Med. 2017;29(2):188–95.
DeWitt D, Canny BJ, Nitzberg M, Choudri J, Porter S. Medical student satisfaction, coping and burnout in direct-entry versus graduate-entry programmes. Med Educ. 2016;50(6):637–45.
Dyrbye LN, Satele D, West CP. Association of characteristics of the learning environment and US medical student burnout, empathy, and career regret. JAMA Netw Open. 2021;4(8):e2119110.
Gradiski IP, Borovecki A, Ćurković M, San-Martín M, Delgado Bolton RC, Vivanco L. Burnout in international medical students: characterization of professionalism and loneliness as predictive factors of burnout. Int J Environ Res Public Health. 2022;19(3):1385.
Greenmyer JR, Montgomery M, Hosford C, Burd M, Miller V, Storandt MH, Lakpa KL, Tiongson C. Guilt and burnout in medical students. Teach Learn Med. 2022;34(1):69–77.
Kilic R, Nasello JA, Melchior V, Triffaux JM. Academic burnout among medical students: respective importance of risk and protective factors. Public Health. 2021;1(198):187–95.
Lucchetti G, Damiano RF, DiLalla LF, Lucchetti AL, Moutinho IL, da Silva Ezequiel O, Kevin Dorsey J. Cross-cultural differences in mental health, quality of life, empathy, and burnout between US and Brazilian medical students. Acad Psychiatry. 2018;42:62–7.
Shin HS, Park H, Lee YM. The relationship between medical students’ empathy and burnout levels by gender and study years. Patient Educ Counsel. 2022;105(2):432–9.
Stosic MD, Blanch-Hartigan D, Aleksanyan T, Duenas J, Ruben MA. Empathy, friend or foe? Untangling the relationship between empathy and Burnout in helping professions. J Soc Psychol. 2022;162(1):89–108.
von Harscher H, Desmarais N, Dollinger R, Grossman S, Aldana S. The impact of empathy on burnout in medical students: new findings. Psychol Health Med. 2018;23(3):295–303.
Wercelens VO, Bueno ML, Bueno JL, Abrahim RP, Ydy JG, Zanetti HR, Montayre J, Maximiano-Barreto MA. Empathy and psychological concerns among medical students in Brazil during the COVID-19 pandemic. Int J Psychiatry Med. 2023;23:00912174231179069.
Wu W, Ma X, Liu Y, Qi Q, Guo Z, Li S, Yu L, Long Q, Chen Y, Teng Z, Li X. Empathy alleviates the learning burnout of medical college students through enhancing resilience. BMC Med Educ. 2022;22(1):1–1.
Shoman Y, Marca SC, Bianchi R, Godderis L, Van der Molen HF, Canu IG. Psychometric properties of burnout measures: a systematic review. Epidemiol Psychiatr Sci. 2021;30:e8.
Higgins JP, Thompson SG, Deeks JJ, Altman DG. Measuring inconsistency in meta-analyses. Br Med J. 2003;327(7414):557–60.
IntHout J, Ioannidis JP, Rovers M, Goeman JJ. Plea for routinely presenting prediction intervals in meta-analysis. BMJ Open. 2016;6(7):e010247.
Sterne JA, Sutton AJ, Ioannidis JP, Terrin N, Jones DR, Lau J, et al. Recommendations for examining and interpreting funnel plot asymmetry in meta-analyses of randomised controlled trials. BMJ. 2011;343:d4002.
Egger M, Davey SG, Schneider M, Minder C. Bias in meta-analysis detected by a simple, graphical test. BMJ. 1997;315(7109):629–34.
Duval S, Tweedie R. Trim and fill: a simple funnel-plot–based method of testing and adjusting for publication bias in meta-analysis. Biometrics. 2000;56(2):455–63.
Borenstein, M., et al., Comprehensive Meta-Analysis Version 4. 2022, Englewood: Biostat.
Gronau QF, van Erp S, Heck DW, Cesario J, Jonas KJ, Wagenmakers E-J. A Bayesian model-averaged meta-analysis of the power pose effect with informed and default priors: the case of felt power. Comprehensive Results Soc Psychol. 2017;2:123–38.
van Erp S, Verhagen AJ, Grasman RPPP, Wagenmakers E-J. Estimates of between-study heterogeneity for 705 meta-analyses reported in Psychological Bulletin from 1990–2013. J Open Psychol Data. 2017;5:4.
Goodman SN. Toward evidence-based medical statistics. 2: The Bayes factor. Ann Intern Med. 1999;130(12):1005–13.
Jeffreys H. The theory of probability. 3rd ed. Oxford, UK: Oxford University Press; 1961.
JASP_Team. JASP (Version 0.17. 1)[Computer Software]. 2023.
Cohen J. Statistical power analysis for the behavioral sciences. Hillsdale: Lawrence Erlbaum Associates; 1988.
Pagnin D, de Queiroz V, Carvalho YT, Dutra AS, Amaral MB, Queiroz TT. The relation between burnout and sleep disorders in medical students. Acad Psychiatry. 2014;38:438–44.
Wu Q, Jin Z, Wang P. The relationship between the physician-patient relationship, physician empathy, and patient trust. J Gen Internal Med. 2022;37(6):1388–93.
Harden RM. What is an OSCE? Med Teach. 1988;10(1):19–22.
Davis MH. Measuring individual differences in empathy: Evidence for a multidimensional approach. J Personal Soc Psychol. 1983;44(1):113.
Casas RS, Xuan Z, Jackson AH, Stanfield LE, Harvey NC, Chen DC. Associations of medical student empathy with clinical competence. Patient Educ Counsel. 2017;100(4):742–7.
Bandura A. Self-efficacy - The exercise of control. New York: Freeman and Company; 1997.
Zachariae R, O’Connor M, Lassesen B, Olesen M, Kjaer LB, Thygesen M, et al. The self-efficacy in patient-centeredness questionnaire - a new measure of medical student and physician confidence in exhibiting patient-centered behaviors. BMC Med Educ. 2015;15:150.
Download references
Patrick Cairns was funded by a PhD Fellowship from Aarhus University School of Business and Social Sciences. No other funding sources were used.
Author information
Authors and affiliations.
Unit for Psycho-Oncology & Health Psychology, Department of Psychology and Behavioural Sciences, Aarhus University, Aarhus, Denmark
P. Cairns & R. Zachariae
Research and Development Department, Division of Mental Health Services, Akershus University Hospital, Sykehusveien 25, 1478, Nordbyhagen, Norway
A. E. Isham
Department of Oncology, Aarhus University Hospital, Aarhus, Denmark
R. Zachariae
You can also search for this author in PubMed Google Scholar
Contributions
All authors contributed to the protocol of this systematic review and meta-analysis. The literature search and data export were performed by PC. Titles and abstracts were screened by PC and AEI. PC and AEI performed full text review, validated by RZ. Data extraction and quality ratings were performed by PC and AEI and validated by RZ. RZ and PC were responsible for the analyses. PC and RZ wrote the first draft manuscript, and all authors critically revised the manuscript and approved the final version.
Corresponding author
Correspondence to P. Cairns .
Ethics declarations
Ethics approval and consent to participate.
Not applicable.
Consent for publication
Competing interests.
The authors declare no competing interests.
Additional information
Publisher’s note.
Springer Nature remains neutral with regard to jurisdictional claims in published maps and institutional affiliations.
Supplementary Information
Supplementary material 1. , supplementary material 2. , rights and permissions.
Open Access This article is licensed under a Creative Commons Attribution 4.0 International License, which permits use, sharing, adaptation, distribution and reproduction in any medium or format, as long as you give appropriate credit to the original author(s) and the source, provide a link to the Creative Commons licence, and indicate if changes were made. The images or other third party material in this article are included in the article's Creative Commons licence, unless indicated otherwise in a credit line to the material. If material is not included in the article's Creative Commons licence and your intended use is not permitted by statutory regulation or exceeds the permitted use, you will need to obtain permission directly from the copyright holder. To view a copy of this licence, visit http://creativecommons.org/licenses/by/4.0/ . The Creative Commons Public Domain Dedication waiver ( http://creativecommons.org/publicdomain/zero/1.0/ ) applies to the data made available in this article, unless otherwise stated in a credit line to the data.
Reprints and permissions
About this article
Cite this article.
Cairns, P., Isham, A.E. & Zachariae, R. The association between empathy and burnout in medical students: a systematic review and meta-analysis. BMC Med Educ 24 , 640 (2024). https://doi.org/10.1186/s12909-024-05625-6
Download citation
Received : 19 January 2024
Accepted : 03 June 2024
Published : 07 June 2024
DOI : https://doi.org/10.1186/s12909-024-05625-6
Share this article
Anyone you share the following link with will be able to read this content:
Sorry, a shareable link is not currently available for this article.
Provided by the Springer Nature SharedIt content-sharing initiative
- Medical students
- Systematic review
- Meta-analysis
BMC Medical Education
ISSN: 1472-6920
- Submission enquiries: [email protected]
- General enquiries: [email protected]
- Research article
- Open access
- Published: 18 June 2024
Early discharge hospital at home as alternative to routine hospital care for older people: a systematic review and meta-analysis
- Lulu Lin 1 na1 ,
- Mengyuan Cheng 2 , 3 na1 ,
- Yawei Guo 1 na1 ,
- Xiaowen Cao 2 na1 ,
- Weiming Tang 2 , 4 na1 ,
- Xin Xu 1 na1 ,
- Weibin Cheng 2 , 5 , 6 na1 &
- Zhongzhi Xu 1 na1
BMC Medicine volume 22 , Article number: 250 ( 2024 ) Cite this article
Metrics details
The global population of adults aged 60 and above surpassed 1 billion in 2020, constituting 13.5% of the global populace. Projections indicate a rise to 2.1 billion by 2050. While Hospital-at-Home (HaH) programs have emerged as a promising alternative to traditional routine hospital care, showing initial benefits in metrics such as lower mortality rates, reduced readmission rates, shorter treatment durations, and improved mental and functional status among older individuals, the robustness and magnitude of these effects relative to conventional hospital settings call for further validation through a comprehensive meta-analysis.
A comprehensive literature search was executed during April–June 2023, across PubMed, MEDLINE, Embase, Web of Science, and Cumulative Index of Nursing and Allied Health Literature (CINAHL) to include both RCT and non-RCT HaH studies. Statistical analyses were conducted using Review Manager (version 5.4), with Forest plots and I 2 statistics employed to detect inter-study heterogeneity. For I 2 > 50%, indicative of substantial heterogeneity among the included studies, we employed the random-effects model to account for the variability. For I 2 ≤ 50%, we used the fixed effects model. Subgroup analyses were conducted in patients with different health conditions, including cancer, acute medical conditions, chronic medical conditions, orthopedic issues, and medically complex conditions.
Fifteen trials were included in this systematic review, including 7 RCTs and 8 non-RCTs. Outcome measures include mortality, readmission rates, treatment duration, functional status (measured by the Barthel index), and mental status (measured by MMSE). Results suggest that early discharge HaH is linked to decreased mortality, albeit supported by low-certainty evidence across 13 studies. It also shortens the length of treatment, corroborated by seven trials. However, its impact on readmission rates and mental status remains inconclusive, supported by nine and two trials respectively. Functional status, gauged by the Barthel index, indicated potential decline with early discharge HaH, according to four trials. Subgroup analyses reveal similar trends.
Conclusions
While early discharge HaH shows promise in specific metrics like mortality and treatment duration, its utility is ambiguous in the contexts of readmission, mental status, and functional status, necessitating cautious interpretation of findings.
Peer Review reports
Hospital-at-Home (HaH) emerges as an innovative healthcare model, redefining the boundaries of hospital care by extending clinical management to the patient’s residence. Leveraging a meticulous blend of early discharge and admission avoidance strategies, HaH is driven by specialized teams that conduct comprehensive health and rehabilitative evaluations, either remotely or through home visits, ensuring the delivery of nuanced and patient-centric care [ 1 ].
The inception of HaH is particularly poignant against the backdrop of a burgeoning aging population, heralding a paradigm that fosters the efficient allocation of healthcare resources while accentuating the centrality of patient welfare [ 2 ]. It has garnered notable attention and application, particularly in the care of older adult patients grappling with a spectrum of conditions such as orthopedic anomalies [ 3 ] and chronic obstructive pulmonary disease (COPD) [ 4 ].
A synthesis of empirical explorations into HaH underscores its potential to recalibrate the cost-effectiveness landscape of healthcare delivery. The model, through its emphasis on early hospital discharge, appears to nurture an ecosystem that not only preserves but potentially enhances the quality of clinical outcomes [ 3 , 5 ]. For example, a randomized controlled trial demonstrated that a short hospital stay followed by a well-managed home care program is as effective as a traditional 10-day hospitalization course. This approach not only reduces hospitalization costs but also fosters closer relationships between patients and their relatives [ 6 ].
However, the trajectory of HaH is not without its intersections of uncertainty and contention. Notable voices in the academic discourse have raised concerns regarding the sufficiency and robustness of evidence elucidating the comparative impacts of care environments on the rehabilitation outcomes of older individuals [ 7 ]. A nuanced examination of existing literature reveals a confluence of findings, where HaH programs, despite their transformative potential, echo with resonances of variability and ambiguity, particularly concerning readmission timelines [ 8 ].
In navigating these complexities, our study embarks on a systematic review and meta-analysis aimed at unraveling the comparative efficacies of early discharge HaH programs. Anchoring our inquiry are important metrics such as mortality rates, readmission frequencies, durations of treatment, and mental and functional statuses. Our exploration is channeled towards discerning the impact of these programs on older adults, aged 60 and above, within the architectural frameworks of traditional inpatient and HaH care paradigms. This endeavor is inspired by a commitment to enriching the empirical foundations that guide the optimization of HaH strategies in alignment with the evolving contours of patient needs and healthcare excellence.
This systematic review and meta-analysis adhered to the Preferred Reporting of Items for Systematic Reviews and Meta-Analyses (PRISMA) guidelines, as detailed in Additional file 1: Table S1. The methodology employed in this review was guided by the Cochrane Handbook for Systematic Reviews of Interventions. This study was registered with PROSPERO (registration number: 321343). The protocol can be accessed at https://www.crd.york.ac.uk/PROSPERO/display_record.php?RecordID=321343 .
Search strategy and eligibility criteria
From April to June 2023, we conducted a rigorous search across multiple electronic databases to locate studies published subsequent to Gonçalves-Bradley DC’s 2017 review [ 9 ]. This encompassed peer-reviewed articles from databases such as PubMed, MEDLINE, Embase, Web of Science, and CINAHL. Additionally, our search was comprehensive, including grey literature such as abstracts and conference proceedings, and a manual review of references from relevant studies and key trial registries like ClinicalTrials.gov.
We used a combination of Medical Subject Headings (MeSH) and keywords relevant to hospital-sponsored healthcare services in the home setting. All studies considered were published in English. The search strategy, detailed in Additional file 1: Text S1, resulted in 5796 entries, shaping the pool from which we selected suitable literature for our review.
Upon the elimination of duplicate entries from the initial database search, two authors independently reviewed titles and abstracts for eligibility. Any discrepancies in the selection process were first resolved through discussion; if a consensus cannot be reached, a third author would arbitrate.
Eligibility criteria
Our review targeted studies that evaluated the efficacy or effectiveness of hospital-sponsored health care services provided in patients’ homes compared to usual in-hospital care. Eligible studies reported or permitted the extraction of raw data for one or more of our primary outcomes. We focused on studies involving older adults (aged ≥ 60 years) or those with a subgroup of individuals aged ≥ 60 for whom results were separately reported. We imposed no disease-specific restrictions to assess the home hospital programs’ efficacy across diverse health care needs. To ensure a thorough analysis, both randomized controlled trials (RCTs) and non-randomized controlled trials (non-RCTs) were included.
Inclusion criteria
Population: Individuals aged 60 years or older receiving health care services at home, who would otherwise require hospital care. Intervention: Health care services provided by physicians or nurses during acute or non-acute phases of illness at the patient’s home. Comparator: Standard inpatient hospital care.
Exclusion criteria
Excluded were studies focusing on outpatient care, residential care settings, or primarily involving patient self-care at home. Programs offering end-of-life care, social services (e.g., assistance with daily living), or transitional “hospital to home” care were also omitted. Review articles, commentaries, and study protocols were excluded due to the absence of outcome data.
Outcome measures
Primary outcomes included mortality rates during the study period or at specific time points, return hospital rates (admissions post-HaH or readmissions after inpatient care), functional ability measured by the Barthel Index, and quality of life assessed via standardized questionnaires like SF-36 or EQ-5D. Secondary outcomes encompassed cognitive function and depression levels.
Study selection
The selection process, documented via a PRISMA flow diagram (Fig. 1 ), began with removing duplicates from database searches. Two authors independently screened titles and abstracts for eligibility, with full-text articles reviewed for those preliminarily selected. Disagreements were resolved through consensus or consultation with a third author if necessary.
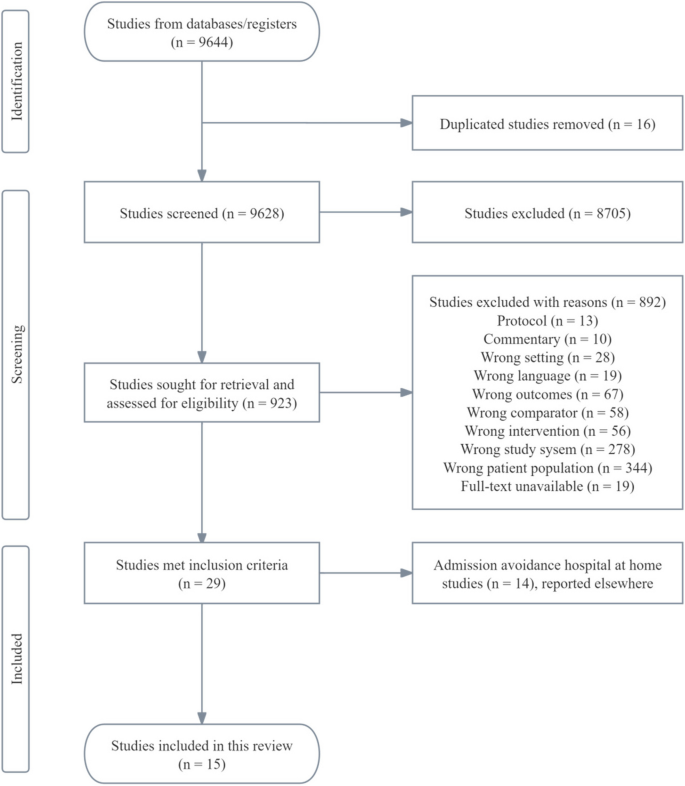
Flow diagram of study selection process
Data extraction
Two independent reviewers are responsible for extracting relevant data from studies that meet the inclusion criteria. Information such as first author, publication year, geographical setting, study design, sample size, duration of follow-up, classification of caregivers, types of health services provided, utilization of telemedicine technologies, control groups, and outcomes measured were systematically documented using an electronic data collection form, accessible in the Additional file 1: Table. S2. In cases of missing or ambiguous data, inquiries were directed to the corresponding authors for clarification.
Quality assessment
In assessing the quality of the included studies, we employed the Version 2 of the Cochrane risk-of-bias tool (RoB2 tool) [ 10 ] for randomized trials and the Risk Of Bias In Non-randomised Studies—of Interventions (ROBINS-I tool) [ 11 ] for non-randomized studies to evaluate potential biases systematically. Our assessment covered biases from the randomization process, deviations from intended interventions, missing outcome data, measurement of outcomes, and the reporting of results. Each domain of bias was judged according to the risk level: low, some concerns, or high. The overall quality of evidence for the outcomes reported in the studies was appraised using the RoB2 for RCTs and the ROBINS-I for non-RCTs and classified into three levels—high, some concerns, and low. Discrepancies in the quality assessment were resolved through discussion or by involving a third author for consensus. We visualized these assessments using Risk of Bias VISualization (robvis) tools [ 12 ] to aid in the clear presentation of our findings. Although certain domains within some studies raised “some concerns,” the collective evaluation of these studies generally indicates a low risk of bias.
Statistical analyses
In conducting a meta-analysis, the conventional practice typically involves segregating RCTs from non-RCTs due to the inherent differences in study design and potential for bias. This separation is rooted in the aim to ensure the integrity and reliability of the analysis by comparing like with like. However, when such a distinction results in an insufficient number of studies within each category, the meta-analysis may face challenges related to statistical power. There is a trade-off between methodological purity and the practical necessity of accruing sufficient data to enable a meaningful analysis. To this end, main analyses were conducted by pooling RCT data and non-RCT data separately. For secondary analyses targeting specific diseases in this study, we have not conducted separate analyses due to an insufficient number of studies within each category, which could compromise the statistical power of the meta-analysis.
For datasets exhibiting homogeneity, a pooled meta-analysis was undertaken. This homogeneity was assessed according to the types of outcomes and their respective measurement time points across studies. Categorical outcomes such as mortality were presented as risk ratios with 95% confidence intervals (CIs), while continuous outcomes were expressed as mean differences (MDs) and 95% CIs. If various measurement techniques were employed, standardized MDs and 95% CIs were used. Statistical analyses were conducted using Review Manager (Version 5.4), with Forest plots and I 2 statistics employed to detect inter-study heterogeneity. For I 2 > 50%, indicative of substantial heterogeneity among the included studies, we employed the random-effects model to account for the variability. For I 2 ≤ 50%, we used the fixed effects model. Substantial heterogeneity was further examined, and subgroup analyses were conducted for randomized controlled trials and specific patient subgroups.
Literature search and studies included
A total of 9628 records were identified through our database search. Of these, 8750 were excluded because they are out of the scope of this analysis. This left 923 records for full-text assessment, from which 892 were excluded for various reasons, including incorrect setting, inappropriate intervention, and unavailability of full text. Consequently, 30 studies met the inclusion criteria. Of these, 15 focused solely on “admission avoidance HaH.” The remaining 15 studies that highlighted “early discharge HaH” were included in this review. Figure 1 demonstrates the selection flow.
Fifteen trials with a cumulative sample size of 4,190 patients were incorporated into this study. The trials involved patients with various health conditions, including cancer [ 13 ], acute medical conditions [ 5 , 14 , 15 , 16 , 17 ], chronic medical conditions [ 4 , 18 , 19 ], orthopedic issues [ 18 , 20 , 21 , 22 , 23 ], and medically complex conditions [ 18 , 24 , 25 ]. Of the 15 studies, 10 were trials related to early discharge HaH [ 4 , 14 , 15 , 19 , 20 , 21 , 22 , 23 , 24 , 25 ]. Meanwhile, five trials studied HaH interventions that incorporated both early discharge and admission avoidance [ 15 , 17 , 18 , 21 , 24 ]. Seven studies employed a randomized controlled trial design, one used a prospective quasi-experiment [ 5 ], one was a prospective, non-randomized real-world cohort comparison [ 13 ], one was a retrospective study [ 22 ], and one was a quasi-experimental longitudinal study [ 21 ]. The characteristics of the included studies are shown in Additional file 1: Table S2. Meta-analysis related to early discharge HaH program in the past 20 years is shown in Additional file 1: Table S3.
Quality of included studies
The risk of bias for RCT and non-RCT studies is shown in Figs. 2 and 3 . Results demonstrate that, although certain domains within some studies raised “some concerns,” the collective evaluation of these studies generally indicates a low risk of bias.
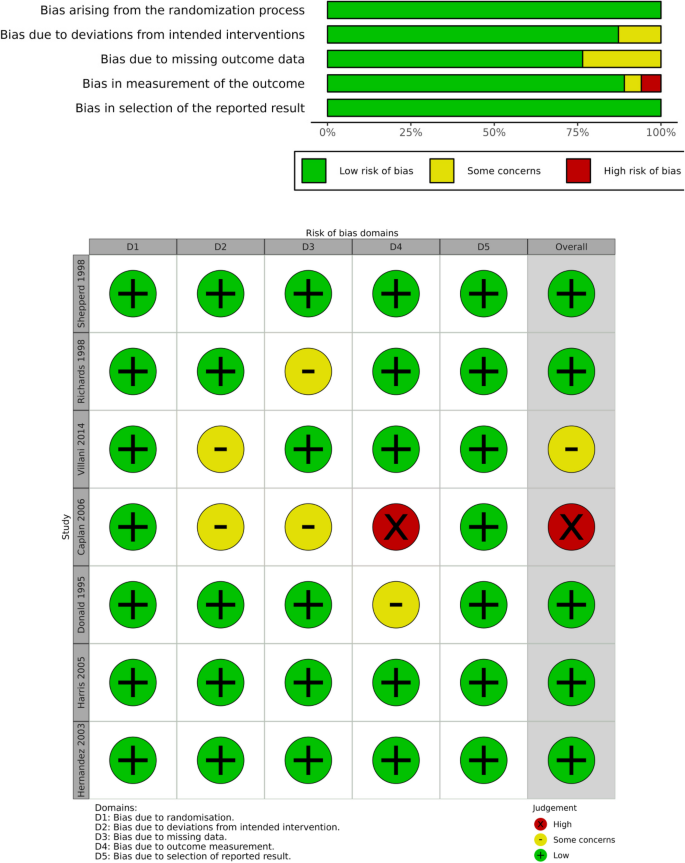
Risk of bias plots for RCT studies
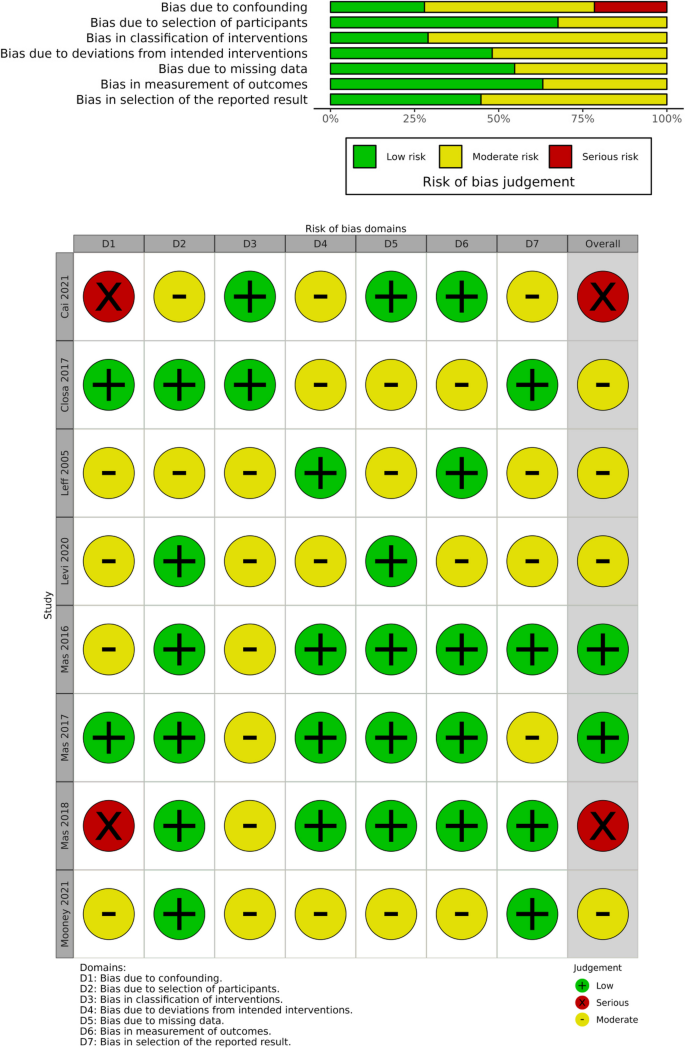
Risk of bias plots for non-RCT studies
Main analysis
The forest plot analysis for mortality outcomes comparing early discharge HaH programs to routine hospital care reveals a nuanced picture: RCTs show no significant difference between HaH and hospital care, with an odds ratio of 1.11 (95% CI: 0.75, 1.65) and minimal heterogeneity ( I 2 = 0%). Non-RCTs, however, indicate a significant mortality reduction in the HaH group (OR = 0.43; 95% CI: 0.26, 0.70), albeit with slightly more heterogeneity ( I 2 = 4%) (Fig. 4 ).
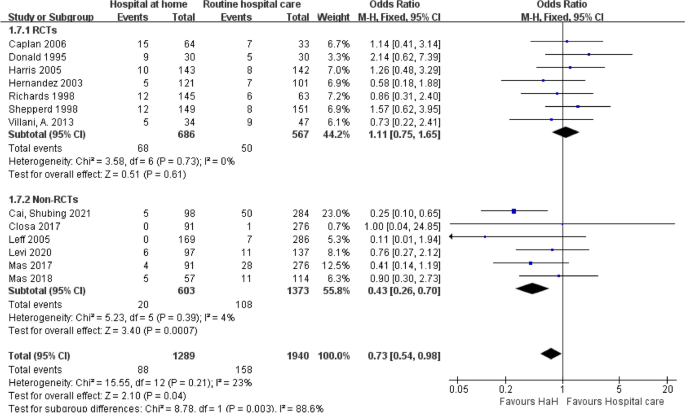
Early discharge hospital at home versus routine hospital care: mortality
Readmission
The forest plot comparing early discharge HaH to routine hospital care shows no significant difference in readmission rates across both RCTs with an odds ratio of 0.97 (95% CI: 0.51, 1.82) and non-RCTs with an OR of 0.75 (95% CI: 0.35, 1.56), and this pattern holds in the combined analysis (OR 0.88; 95% CI: 0.55, 1.40). However, the high heterogeneity observed within both RCTs ( I 2 = 71%) and non-RCTs ( I 2 = 72%) suggests variability in the study outcomes, underscoring the need for cautious interpretation (Fig. 5 ).
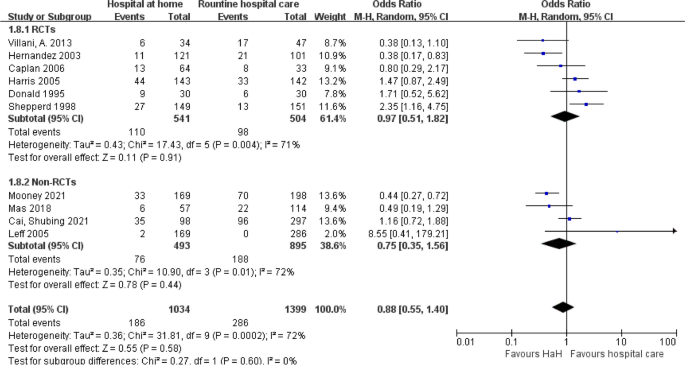
Early discharge hospital at home versus routine hospital care: readmission
Length of treatment
The forest plot for length of treatment shows that in RCTs, the HaH group exhibits no significant difference compared to routine care, with a mean difference of 0.02 (95% CI: − 0.98 to 1.03) and low heterogeneity ( I 2 = 8%). In contrast, non-RCTs demonstrate a significant reduction in treatment duration for HaH with a mean difference of − 1.66 (95% CI: − 3.18 to − 0.14) but with substantial heterogeneity ( I 2 = 90%) (Fig. 6 ).
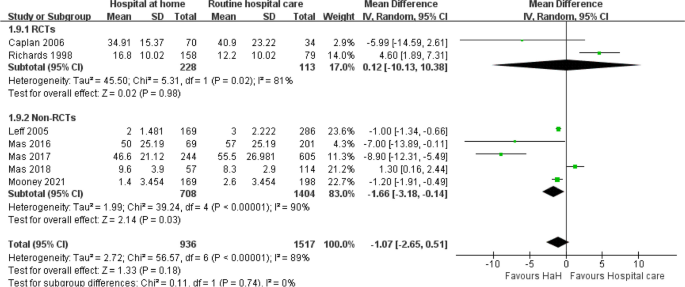
Early discharge hospital at home versus routine hospital care: length of treatment
Functional status — Barthel index at the end up
Figure 7 indicates a notable reduction in treatment length with HaH when compared to standard hospital care, as shown by a mean difference of − 1.82 (95% CI: − 2.55, − 1.09) with negligible heterogeneity ( I 2 = 0%) in non-RCTs. A meta-analysis for RCTs on this outcome was not performed due to the presence of only a single study.
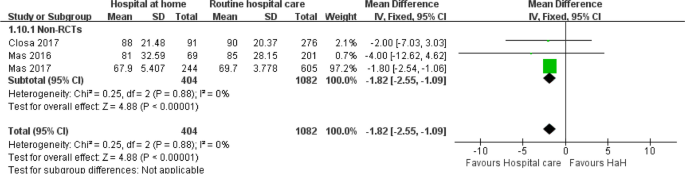
Early discharge hospital at home versus routine hospital care: functional status — Barthel index at the end up
Mental status — MMSE
For the assessment of mental status using MMSE, our analysis considered one randomized controlled trial (RCT) [ 14 ] and one retrospective study [ 22 ]. Due to the presence of only one study in each category, we are not able to pool the results. The findings from both the retrospective study and the RCT indicated no significant difference in MMSE scores between the case group and the control group. Specifically, the retrospective study reported a mean difference of − 1.24 (95% CI: − 3.30 to 0.82, N = 235; P = 0.24), and the RCT showed a mean difference of − 2.59 (95% CI: − 5.45 to 0.27, N = 70; P = 0.08).
Subgroup analyses based on disease classification
Subgroup analyses were conducted in patients with different medical conditions. Patients were classified into five groups: acute medical conditions [ 5 , 14 , 15 , 16 , 17 ], chronic medical conditions [ 4 , 18 , 19 ], orthopedic conditions [ 18 , 20 , 21 , 22 , 23 ], cancer [ 13 ], and medically complex conditions [ 18 , 24 , 25 ].
Figure 8 reported the impact of early discharge HaH programs on mortality across various medical conditions. Cumulatively, the data indicates a significant 25% reduction in mortality risk for HaH participants (RR = 0.75, P = 1.00). Upon dissection by condition, both acute and chronic medical cases, as well as orthopedic and complex medical conditions, showed favorable trends towards HaH programs, even if individual disease categorizations did not always attain statistical significance. Notably, the relatively low heterogeneity among acute and chronic conditions ( I 2 = 20% and I 2 = 0%, respectively) suggests consistent outcomes across these trials. The forest plot further reinforces these findings, with the majority of individual study outcomes leaning towards the early discharge HaH advantage and the aggregate result represented by the diamond, solidly positioned on the “early discharge HaH” side, underscoring the potential benefits of HaH in reducing mortality.
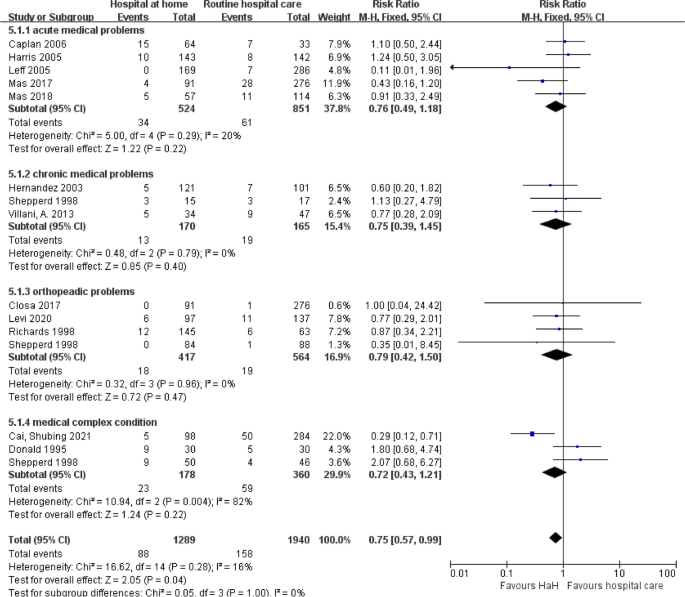
Early discharge hospital at home versus routine hospital care: subgroup analysis based on disease classification — mortality
Figure 9 illuminated the impact of early discharge HaH programs on readmission rates across diverse medical conditions. Overall, the combined data reveals a marginal increase in readmission risk for HaH programs, but the effect is statistically insignificant (RR = 1.04, P = 0.26). When segregating by disease type, acute medical cases depict an almost neutral effect, with a pooled risk ratio near unity (RR = 1.00). Chronic medical problems, while reflecting a slight reduction in readmission risk, also lacked statistical significance. Intriguingly, orthopedic cases displayed a notably higher readmission risk (RR = 3.14), though this observation was based on limited data and requires further substantiation. Medical complex conditions and cancer demonstrated neutral to increased risk, yet again without achieving statistical significance. An overarching observation is the pronounced heterogeneity in some disease categories, such as chronic medical problems ( I 2 = 68%), underscoring the importance of interpreting these results with caution. The forest plot bolsters these conclusions, with a mix of study outcomes on both sides of the neutrality line and the collective result, embodied by the diamond, tending slightly towards the “routine hospital” side. This suggests that while HaH may offer several advantages, it is crucial to consider patient-specific factors and disease categories when assessing its implications for readmission.
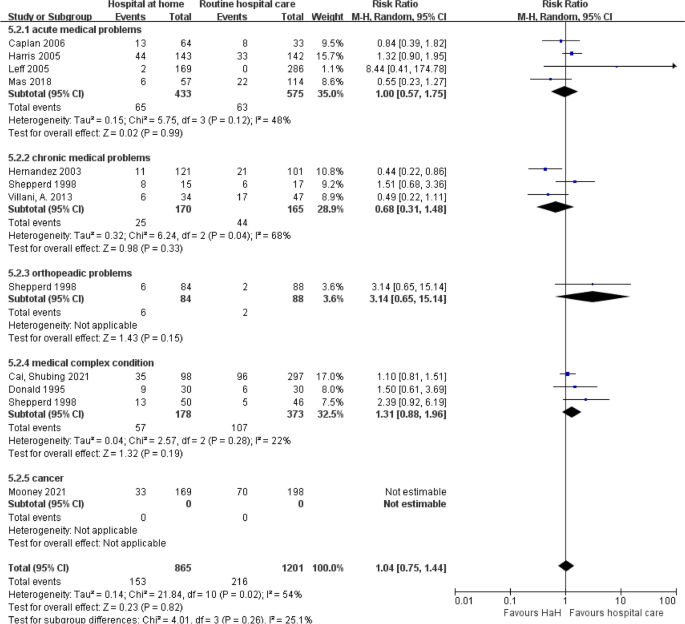
Early discharge hospital at home versus routine hospital care: subgroup analysis based on disease classification — readmission
Figure 10 elucidates the impact of early discharge HaH programs on the duration of treatment, quantified as the standard mean difference across various medical conditions. For acute medical problems, the pooled data indicates a modest reduction in treatment duration for HaH, albeit with a wide confidence interval that borders on the null effect (MDs = − 0.21, P = 0.21). Notably, there is high heterogeneity ( I 2 = 87%), suggesting substantial variability among the included studies for this category. For orthopedic issues, although a reduction in treatment duration is observed for HaH, the confidence interval overlaps with zero, denoting potential insignificance in the effect (MDs = − 0.09). The heterogeneity in this subgroup is also high ( I 2 = 93%), emphasizing the variability of study outcomes. The overall combined effect suggests a negligible reduction in treatment duration with HaH (MDs = − 0.11), and the encompassing forest plot substantiates this with outcomes straddling both sides of the neutrality line. The prevailing observation is that while HaH may potentially reduce the duration of treatment, the extent of this reduction varies considerably across studies and disease categories. Given the high heterogeneity in results, careful interpretation and further research are necessary to delineate the specific scenarios where HaH offers significant time-saving benefits.
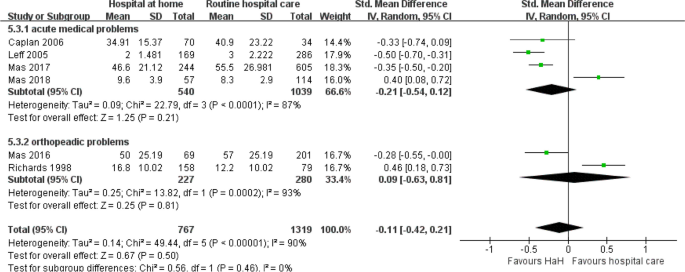
Early discharge hospital at home versus routine hospital care: subgroup analysis based on disease classification — length of treatment
Barthel index at the end up
Figure 11 conducted a subgroup analysis of the Barthel index scores at the endpoint, comparing early discharge HaH to traditional routine hospital care, segmented by acute medical problems and orthopedic conditions. For those with acute medical problems, HaH intervention suggests a significant reduction in Barthel index scores with a standard mean difference of − 0.42 (95% CI: − 0.57 to − 0.27), showcasing its potential efficacy. This result is statistically significant with a p -value of less than 0.00001. However, in orthopedic conditions, the results are less definitive. Although there is a trend towards a decrease in the Barthel index scores within the HaH group (MDs = − 0.11), the confidence interval slightly spans the line of neutrality, indicating potential non-significance. This subgroup also displays moderate heterogeneity ( I 2 = 69%), hinting at diverse outcomes across the incorporated studies. In totality, while HaH seems to influence a reduction in Barthel index scores, the magnitude and robustness of this impact appear to be condition-specific. Collectively, these findings emphasize the potential benefits of HaH, particularly for patients with acute medical problems, but warrant cautious interpretation in the context of orthopedic issues.
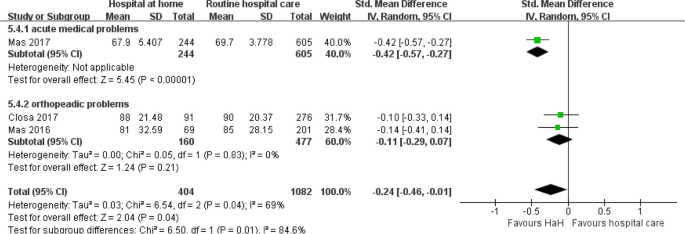
Early discharge hospital at home versus routine hospital care: subgroup analysis based on disease classification — Barthel index at the end up
Fifteen trials were incorporated into this systematic review examining early discharge HaH for older people. Our investigation encompassed a comprehensive exploration of the efficacy of early hospital discharge to home care across several key domains: mortality, readmission rates, duration of treatment, functional status (as quantified by the Barthel index), and cognitive health (as assessed by the MMSE).
Our analysis suggests that early discharge HaH correlates with a decrease in mortality rates, a conclusion drawn from 6 of the non-RCTs. In contrast, the impact of early discharge HaH on readmission rates appears negligible, a conclusion supported by four non-RCTs and five RCTs. The influence of early discharge HaH on cognitive health, based on MMSE scores, is similarly inconsequential, as demonstrated by one RCT and one non-RCT. Moreover, early discharge HaH might be linked to a decline in functional status, as gauged by the Barthel index, based on evidence from one RCT and three non-RCTs. After analyzing the data of the fifteen trials included, regardless of whether there was a statistically significant difference between the early discharge HaH group and the routine hospital care group from the forest map, the diamonds tended to be on the “early discharge HaH” side, emphasizing the role of early discharge HaH in mortality, readmission rate, length of treatment and Barthel index. Our subgroup analysis based on disease classifications—encompassing acute medical problems, chronic conditions, orthopedic issues, cancer, and medically complex conditions—revealed similar trends when compared with the main analysis.
In our analysis, the effectiveness for the same measures—such as mortality, length of treatment, and the Barthel index—between RCTs and non-RCTs is not always identical. This variance likely stems from the inherent differences in the design and execution of these two types of studies. RCTs, with their higher design rigor and controlled environments, minimize selection biases and provide stronger evidence of causality, while non-RCTs, reflecting broader and more diverse populations, may offer findings that are more generalizable but subject to inherent biases and variability. The observed discrepancy of effectiveness for the same measures between RCTs HaH and non-RCTs HaH highlights the importance of considering the real-world scenario in understanding reported intervention effectiveness.
The HaH model is distinguished by their more holistic approach compared to traditional hospital care, particularly for older adults. Its capacity to prevent the development of geriatric syndromes is achieved through the comprehensive method of care that addresses not only the medical but also the physical, emotional, and social needs of patients, thereby enhancing their overall well-being and reducing mortality risks.
Furthermore, the decline in functional status observed in some patients underscores the indispensability of incorporating a multidisciplinary team into the HaH model. Specifically, the integration of physiotherapists and occupational therapists is crucial. These professionals can tailor rehabilitation and daily activity programs to the individual needs of each patient, fostering not only the maintenance but potentially the improvement of functional status. Such an approach ensures that care is not merely reactive but preventive and rehabilitative, addressing both the immediate and long-term health needs of the older adults.
While our meta-analysis indicated that the efficacy of Hospital-at-Home (HaH) programs is equivocal with regard to readmission rates and certain cognitive and functional outcomes, the approach nevertheless offers several distinct advantages that warrant further exploration and development. One of the most salient advantages of HaH programs in the aftermath of the COVID-19 pandemic is the minimization of exposure to nosocomial infections for vulnerable older adults [ 26 , 27 , 28 ]. Traditional hospital settings, by their nature, expose patients to a variety of pathogens, posing an increased risk of acquiring secondary infections. This is especially critical for older adults who are often immunocompromised and thus more susceptible to infections. Implementing HaH programs can thus provide a safer, more controlled environment, reducing the likelihood of cross-infections. The healthcare industry’s experience in the pandemic underscores the urgency for such decentralized healthcare models that can offer quality care while mitigating risks associated with hospital-based treatment [ 29 , 30 , 31 ].
HaH programs could also play a pivotal role in bridging healthcare disparities observed in rural areas and developing countries, which frequently face infrastructural constraints and shortages of healthcare professionals. The burden of chronic diseases such as heart disease, cancer, diabetes, and mental disorders is high in low-income and middle-income countries and is expected to increase with population aging, urbanization, and globalization of risk factors [ 32 , 33 ]. Implementing HaH in these settings may alleviate some of these challenges by providing essential healthcare services directly to the homes of older adults. This is particularly beneficial in geographical locations where distance and limited transportation options make it arduous for individuals to access healthcare facilities. HaH leverages telehealth technologies and portable medical equipment, making it a scalable solution that can extend the reach of quality healthcare to remote and underserved communities [ 34 , 35 ]. While the effectiveness of HaH on specific clinical outcomes may remain under debate, these broader socio-medical benefits highlight the importance of its continued development and implementation. A comprehensive assessment of HaH programs should thus go beyond traditional clinical metrics to include factors like patient safety, accessibility, and equity, especially in the changing landscape of global healthcare [ 33 ].
Meanwhile, in this study, our analysis of functional status is limited to the Barthel index after the end of the trials, while ignoring the trend of changes in the Barthel index before and after the intervention treatment in both groups. Due to the different evaluation indicators used in different trials for the various states of patients before and after hospitalization, the emphasis on measurement indicators also varies. Some trials focus on measuring the index changes before and after admission, while others focus on measuring the Barthel index after discharge. These may reduce the accuracy of our assessment of the improvement in functional status between the early discharge group and the routine hospital care group. Moreover, the overlap of evaluation indicators for cognitive health in different experiments is also relatively low. If different indicators can be standardized, the evaluation in the mental state dimension will be more comprehensive.
In summation, while early discharge HaH presents a viable approach for diminishing mortality and treatment duration, its efficacy remains ambiguous in relation to readmission, as well as cognitive and functional outcomes. Thus, a prudent interpretation of these findings is essential.
Availability of data and materials
The data used to support the findings of this study are included within the article.
Abbreviations
Confidence intervals
Chronic obstructive pulmonary disease
- Early discharge hospital at home
The 5-level EQ-5D version
Hospital at Home
Mean differences
Medical Subject Headings
The Mini-Mental State Examination
Non-randomized controlled trials
Preferred Reporting of Items for Systematic Reviews and Meta-Analyses
International prospective register of systematic reviews
Randomized controlled trials
Version 2 of the Cochrane risk-of-bias tool for randomized trials
Risk Of Bias In Non-randomised Studies—of Interventions
Risk of Bias VISualization
The 36-Item Short Form Health Survey
Shepperd S, Iliffe S, Doll HA, Clarke MJ, Kalra L, Wilson AD, et al. Admission avoidance hospital at home. Cochrane Database Syst Rev. 2016(9):CD007491.
World Health Organization. Decade of healthy ageing: baseline report. 2020.
Google Scholar
Caplan GA, Sulaiman NS, Mangin DA, Aimonino Ricauda N, Wilson AD, Barclay L. A meta-analysis of “hospital in the home.” Med J Aust. 2012;197:512.
Article PubMed Google Scholar
Hernandez C, Casas A, Escarrabill J, Alonso J, Puig-Junoy J, Farrero E, et al. Home hospitalisation of exacerbated chronic obstructive pulmonary disease patients. Eur Respir J. 2003;21:58–67.
Article CAS PubMed Google Scholar
Leff B, Burton L, Mader SL, Naughton B, Burl J, Inouye SK, et al. Hospital at home: feasibility and outcomes of a program to provide hospital-level care at home for acutely Ill older patients background: acutely ill older persons often experience adverse. 2005.
Suwanwela NC, Phanthumchinda K, Limtongkul S, Suvanprakorn P, Thai T, Cross R, et al. Comparison of short (3-day) hospitalization followed by home care treatment and conventional (10-day) hospitalization for acute ischemic stroke. 2002.
Book Google Scholar
Ward D, Drahota A, Gal D, Severs M, Dean TP. Care home versus hospital and own home environments for rehabilitation of older people. Cochrane Database Syst Rev. 2008(4):CD003164.
Qaddoura A, Yazdan-Ashoori P, Kabali C, Thabane L, Haynes RB, Connolly SJ, et al. Efficacy of hospital at home in patients with heart failure: a systematic review and meta-analysis. PLoS One. 2015;10:e0129282.
Article PubMed PubMed Central Google Scholar
Gonçalves-Bradley DC, Iliffe S, Doll HA, Broad J, Gladman J, Langhorne P, et al. Early discharge hospital at home. Cochrane Database Syst Rev. 2017;(6):CD000356.
Sterne JAC, Savović J, Page MJ, Elbers RG, Blencowe NS, Boutron I, et al. RoB 2: a revised tool for assessing risk of bias in randomised trials. BMJ. 2019;366:l4898.
Sterne JA, Hernán MA, Reeves BC, Savović J, Berkman ND, Viswanathan M, et al. ROBINS-I: a tool for assessing risk of bias in non-randomised studies of interventions. BMJ. 2016;355:i4919.
Sterne JA, Hernán MA, Reeves BC, Savović J, Berkman ND, Viswanathan M, et al. ROBINS-I: A tool for assessing risk of bias in non-randomised studies of interventions. Res Synth Methods. 2021;12:55–61.
Mooney K, Titchener K, Haaland B, Coombs LA, O’Neil B, Nelson R, et al. Evaluation of oncology hospital at home: unplanned health care utilization and costs in the huntsman at home real-world trial. J Clin Oncol. 2021;39:2586.
Caplan GA, Coconis J, Board N, Sayers A, Woods J. Does home treatment affect delirium? A randomised controlled trial of rehabilitation of elderly and care at home or usual treatment (The REACH-OUT trial). Age Ageing. 2006;35:53–60.
Harris R, Ashton T, Broad J, Connolly G, Richmond D. The effectiveness, acceptability and costs of a hospital-at-home service compared with acute hospital care: a randomized controlled trial. 2005.
Mas M, Inzitari M, Sabaté S, Santaeugènia SJ, Miralles R. Hospital-at-home Integrated Care Programme for the management of disabling health crises in older patients: Comparison with bed-based Intermediate Care. Age Ageing. 2017;46:925–31.
Mas M, Santaeugènia SJ, Tarazona-Santabalbina FJ, Gámez S, Inzitari M. Effectiveness of a Hospital-at-Home Integrated Care Program as Alternative Resource for Medical Crises Care in Older Adults With Complex Chronic Conditions. J Am Med Dir Assoc. 2018;19:860–3.
Shepperd S, Harwood D, Jenkinson C, Gray A, Vessey M, Morgan P. Randomised controlled trial comparing hospital at home care with inpatient hospital care. I: three month follow up of health outcomes. Br Med J. 1998;316:1786–91.
Article CAS Google Scholar
Villani A, Malfatto G, Compare A, Rosa FD, Bellardita L, Branzi G, et al. Clinical and psychological telemonitoring and telecare of high risk heart failure patients. J Telemed Telecare. 2014;20:468–75.
Richards SH, Coast J, Gunnell DJ, Peters TJ, Pounsford J, Darlow MA. Randomised controlled trial comparing effectiveness and acceptability of an early discharge, hospital at home scheme with acute hospital care. Br Med J. 1998;316:1796–801.
Closa C, Mas M, Santaeugènia SJ, Inzitari M, Ribera A, Gallofré M. Hospital-at-home Integrated Care Program for Older Patients With Orthopedic Processes: An Efficient Alternative to Usual Hospital-Based Care. J Am Med Dir Assoc. 2017;18:780–4.
Levi Y, Punchik B, Zikrin E, Shacham D, Katz D, Makulin E, et al. Intensive inpatient vs. home-based rehabilitation after hip fracture in the elderly population. Front Med (Lausanne). 2020;7:592693.
Mas MÀ, Closa C, Santaeugènia SJ, Inzitari M, Ribera A, Gallofré M. Hospital-at-home integrated care programme for older patients with orthopaedic conditions: Early community reintegration maximising physical function. Maturitas. 2016;88:65–9.
Cai S, Intrator O, Chan C, Buxbaum L, Haggerty MA, Phibbs CS, et al. Association of Costs and Days at Home with Transfer Hospital in Home. JAMA Netw Open. 2021. https://doi.org/10.1001/jamanetworkopen.2021.14920 .
Donald IP, Baldwin RN, Bannerjee M. Gloucester hospital-at-home: a randomized controlled trial. Age Ageing. 1995;24:434.
Moazzami B, Razavi-Khorasani N, Dooghaie Moghadam A, Farokhi E, Rezaei N. COVID-19 and telemedicine: immediate action required for maintaining healthcare providers well-being. J Clin Virol. 2020;126:104345.
Article CAS PubMed PubMed Central Google Scholar
Ohannessian R, Duong TA, Odone A. Global telemedicine implementation and integration within health systems to fight the COVID-19 pandemic: a call to action. JMIR Public Health Surveill. 2020;6:e18810.
Calton B, Abedini N, Fratkin M. Telemedicine in the Time of Coronavirus. J Pain Symptom Manage. 2020;60:e12–4.
Hollander JE, Carr BG. Virtually perfect? Telemedicine for Covid-19. N Engl J Med. 2020;382:1679.
Nundy S, Patel KK. Hospital-at-home to support COVID-19 surge - time to bring down the walls? JAMA Health Forum. 2020;1:e200504.
Latifi R, Doarn CR. Perspective on COVID-19: finally, telemedicine at center stage. Telemed E Health. 2020;26:1106.
Article Google Scholar
Beaglehole R, Epping-Jordan J, Patel V, Chopra M, Ebrahim S, Kidd M, et al. Series Alma-Ata: Rebirth and revision 3 improving the prevention and management of chronic disease in low-income and middle-income countries: a priority for primary health care. 2008.
Hamine S, Gerth-Guyette E, Faulx D, Green BB, Ginsburg AS. Impact of mHealth chronic disease management on treatment adherence and patient outcomes: A systematic review. J Med Internet Res. 2015;17:e52.
Mueller DB, Tivey D, Croce D. Health-technology assessment: Its role in strengthening health systems in developing countries. Strengthening. 2017. https://doi.org/10.7196/SHS.2017.v2.i1.50 .
Leonard E, de Kock I, Bam W. Barriers and facilitators to implementing evidence-based health innovations in low- and middle-income countries: a systematic literature review. Eval Program Plann. 2020;82:101832.
Download references
Acknowledgements
We would like to thank the reviewers for their helpful comments, which greatly improved this article.
This work was supported in part by the Basic and Applied Research Funding of the Guangzhou Science and Technology Plan (2024A04J4621), in part by the Sun Yat-sen University Basic Start-up Funding (51000–12230014), and in part by the Guangzhou Science and Technology Programme (2024A03J074, and 2023A03J0286).
Author information
Lulu Lin, Mengyuan Cheng, and Yawei Guo contributed equally to this work.
Authors and Affiliations
School of Public Health, Sun Yat-Sen University, Guangzhou, China
Lulu Lin, Yawei Guo, Xin Xu & Zhongzhi Xu
Institute for Healthcare Artificial Intelligence Application, Guangdong Second Provincial General Hospital, Guangzhou, China
Mengyuan Cheng, Xiaowen Cao, Weiming Tang & Weibin Cheng
Bouvé College of Health Sciences, Northeastern University, Boston, MA, USA
Mengyuan Cheng
Institute of Global Health and Infectious Diseases, University of North Carolina at ChapelHill, Chapel Hill, NC, USA
Weiming Tang
School of Data Science, City University of Hong Kong, Hong Kong SAR, China
Weibin Cheng
Faculty of Health Sciences, City University of Macau, Macao SAR, China
You can also search for this author in PubMed Google Scholar
Contributions
LLL, CMY, CWB, and ZZX conceptualized the study. LLL, CMY, and CXW designed the methodology and did the data collection. LLL did the data analysis. TWM, CWB, and ZZX supervised the data collection, analyses, and/or interpretation. GYW and XX verified the data collection and evidence interpretation. ZZX acquired funding. LLL prepared the original draft of the manuscript with the input of TWM, CWB, GYW, and ZZX. All authors edited and reviewed the final manuscript. All authors have read and agreed to the final version of the manuscript and to the decision to submit. All authors had access to all the data. LLL and ZZX have verified the data. All authors read and approved the final manuscript.
Corresponding authors
Correspondence to Weibin Cheng or Zhongzhi Xu .
Ethics declarations
Ethics approval and consent to participate.
Not applicable.
Consent for publication
All of the authors agreed to submit and publish the final manuscript.
Competing interests
The authors declare that they have no competing interests.
Additional information
Publisher’s note.
Springer Nature remains neutral with regard to jurisdictional claims in published maps and institutional affiliations.
Supplementary Information
12916_2024_3463_moesm1_esm.docx.
Additional file 1: Table S1. PRISMA check list. Table S2. Characteristics of included studies. Table S3. Meta analysis on early discharge HaH program in the past 20 years. Text S1. Search Strategy Used In PUBMED.
Rights and permissions
Open Access This article is licensed under a Creative Commons Attribution 4.0 International License, which permits use, sharing, adaptation, distribution and reproduction in any medium or format, as long as you give appropriate credit to the original author(s) and the source, provide a link to the Creative Commons licence, and indicate if changes were made. The images or other third party material in this article are included in the article's Creative Commons licence, unless indicated otherwise in a credit line to the material. If material is not included in the article's Creative Commons licence and your intended use is not permitted by statutory regulation or exceeds the permitted use, you will need to obtain permission directly from the copyright holder. To view a copy of this licence, visit http://creativecommons.org/licenses/by/4.0/ . The Creative Commons Public Domain Dedication waiver ( http://creativecommons.org/publicdomain/zero/1.0/ ) applies to the data made available in this article, unless otherwise stated in a credit line to the data.
Reprints and permissions
About this article
Cite this article.
Lin, L., Cheng, M., Guo, Y. et al. Early discharge hospital at home as alternative to routine hospital care for older people: a systematic review and meta-analysis. BMC Med 22 , 250 (2024). https://doi.org/10.1186/s12916-024-03463-3
Download citation
Received : 03 November 2023
Accepted : 03 June 2024
Published : 18 June 2024
DOI : https://doi.org/10.1186/s12916-024-03463-3
Share this article
Anyone you share the following link with will be able to read this content:
Sorry, a shareable link is not currently available for this article.
Provided by the Springer Nature SharedIt content-sharing initiative
- Home care services
- Older people
- Meta-analysis
BMC Medicine
ISSN: 1741-7015
- Submission enquiries: [email protected]
- General enquiries: [email protected]
- Open access
- Published: 14 June 2024
Effect of Otago exercise on fear of falling in older adults: a systematic review and meta-analysis
- Jianlong Han 1 na1 ,
- Hongwei Wang 1 na1 ,
- Yunxin Ding 1 ,
- Qing Li 1 ,
- Huanqie Zhai 2 &
- Shuling He ORCID: orcid.org/0009-0002-8628-7753 3
BMC Sports Science, Medicine and Rehabilitation volume 16 , Article number: 132 ( 2024 ) Cite this article
61 Accesses
Metrics details
Approximately 40–70% of older adults who have experienced falls develop fear of falling (FOF), with the incidence rate in nursing home residents reaching as high as 79.4%. An increasing number of studies have focused on the effect of the Otago Exercise Programme (OEP) on reducing FOF among older adults, yet comprehensive analysis is lacking due to regional and demographic variations. Therefore, this study integrates the relevant literature to provide evidence supporting interventions aimed at alleviating FOF among older adults.
To evaluate the impact of OEP on FOF in older adults through meta-analysis.
We searched ten databases using computer systems, covering all records up to May 1, 2024. Two researchers independently conducted the literature screening, bias risk assessment, and data extraction. We performed data analysis using RevMan 5.3 and Stata 15.0 software, assessed result stability through sensitivity analysis, and examined publication bias with funnel plots and Egger’s test.
Sixteen RCTs were included. Meta-analysis revealed that the OEP significantly reduced FOF among older adults [SMD = 0.96, 95%CI (0.68, 1.23), P < 0.00001]. Subgroup analysis revealed that interventions lasting more than 16 weeks [SMD = 1.12, 95%CI (0.75, 1.49), P < 0.00001], with a frequency of more than twice a week [SMD = 0.99, 95%CI (0.64, 1.35), P < 0.00001], and for older adults in community and nursing institutions [SMD = 1.03, 95%CI (0.50, 1.57), P = 0.0002] were more effective. A comparison of the 16-week and 24-week interventions revealed that the latter had better outcomes [SMD = 0.87, 95%CI (0.66, 1.08), P = 0.0004].
Current evidence indicates that OEP effectively reduces FOF among older adults. It is recommended that interventions last for more than 24 weeks, occur more than twice a week, and suitable for application among older adults in community settings or elder care institutions.
Peer Review reports
Introduction
Fear of Falling (FOF), refers to the avoidance of daily activities due to the fear of falling, such as cleaning, which can lead to symptoms such as anxiety and palpitations if these activities are undertaken [ 1 ]. FOF is prevalent among older adults, Xiong et al. reported that fear of falling occurs in approximately 49.6% of the population worldwide, with a maximum prevalence of 90.34%, and that the prevalence is high in developing countries and disease groups [ 2 ]. The relationship between FOF and actual falls is closely linked, with each influencing the other in a vicious cycle [ 3 ]. Ho LYW et al. conducted a cross-sectional survey on older stroke patients, and their results showed that severe fear of falling can increase the incidence of falls, restrict daily living activities, and lead to frailty [ 4 ]. Excessive worry about the consequences of falling can reduce social interactions, and trigger negative emotions such as anxiety and depression, severely affecting quality of life [ 5 ]. In a global initiative proposed by Montero-Odasso et al. in 2022 on the prevention and management of falls in older adults, it is recommended to reduce the fear of falling in older adults through exercise [ 6 ]. The Otago Exercise Programme (OEP) was developed by Campbell and others [ 7 ]. OEP through warm-up exercises, strength training, balance training, and walking activities, can be used to develop personalized training plans based on the physical condition of older adults [ 8 ]. Gradual and systematic exercise increases the sensitivity of proprioceptors in older adults thereby improving physical function and reducing FOF [ 9 ]. Currently, relevant meta-analyses have explored the effects of OEP on older adults [ 10 , 11 , 12 ]. Their results indicate that OEP can enhance lower limb strength and balance in older adults. However, there is currently a lack of attention to the psychological issues of falls in older adults, and no studies have deeply explored the impact of OEP on the FOF in older adults. Yu et al.‘s findings suggest that OEP can reduce FOF in older adults [ 11 ], but their work involved only a descriptive analysis of two studies, lacking in-depth investigation. Therefore, to explore in depth the effects of OEP on FOF in older adults, this study integrates and analyzes related literature through meta-analysis to explore the effects of different intervention durations and frequencies, and to provide evidence supporting the application of OEP in the older adult population.
Research design
This study is based on the PICOS framework. This study followed the Preferred Reporting Items for Systematic Reviews and Meta-Analyses (PRISMA2020) guidelines for systematic reviews and meta-analyses. The study was retrospectively registered on the PROSPERO platform (CRD42024529549).
Literature search
We conducted searches in four commonly used Chinese databases: CNKI, Wanfang, VIP, and the China Biology Medicine disc (CBM), as well as five English databases: CINAHL, Embase, Cochrane Library, Web of Science, and PubMed. We retrieved all studies available up to May 1, 2024, using the search terms “otago exercise” “otago” " OEP” " Aged” " Aging” “elderly” “old people” “seniors” “fear of falling” “FOF” and “fear”tailored to the requirements of each database. This was supplemented by manual searches to screen the references of the included literature for selection.
Inclusion and exclusion criteria
Based on the PICOS principle, we established the inclusion criteria. (1) The inclusion criteria were as follows; (i) study subjects: older adults aged 60 years and older; (ii) intervention measures: the control group received conventional exercise training or care, while the intervention group received OEP in addition to the control group’s regimen; (iii) outcome indicators: FOF; (iv) study type: randomized controlled trials (RCTs). The exclusion criteria include: (i) studies combining other interventions; (ii) studies that were duplicate publications or for which the full text was not available.
Screening and data extraction
We imported all literature into EndNote X9 software for screening, which was performed independently by two researchers. The authors excluded duplicates, studies with nonmatching intervention measures, and nonmatching intervention subjects by reading the study titles. By reading the literature abstracts and full texts, they excluded non-RCT literature, studies with nonmatching outcome indicators, and literature for which the full text was not accessible. After completing their selections, the two researchers cross-checked their results. Any disagreements were resolved by a third researcher. Two researchers then extracted the data according to a predesigned data extraction form. The extracted content included the authors’ names, publication year, intervention population, sample size, intervention measures, control measures, duration of a single intervention, intervention period and frequency, and the evaluation tools and results for the outcome indicators. Upon completion, the two researchers cross-checked their work. Any disagreements were discussed and resolved with the involvement of a third researcher.
Risk of literature bias and assessment of publication bias
Two researchers independently assessed the risk of bias in the included studies using the risk of bias assessment tool for randomized controlled trials recommended by the Cochrane Collaboration [ 13 ]. They evaluated seven aspects, each of which were rated as low risk (+), unclear risk (?), or high risk (-). Studies that fully met the criteria were classified as Grade A, those that partially met the criteria as Grade B, and those that did not meet the criteria at all as Grade C. We used funnel plots and Egger’s test to assess whether there was any publication bias in this study. A t value in Egger’s test was greater than 0.05, according to Egger’s test indicated no significant publication bias. We used the GRADE method to rate the quality of evidence for the results [ 13 ], with evidence levels being high, medium, low, and very low.
Statistical methods
We conducted a meta-analysis using Review Manager 5.3 and Stata 17.0 software. Given the wide range of sources of heterogeneity and their impact on the results, we used the standardized mean difference (SMD) and 95% confidence intervals (CI) as the effect sizes for this study. SMD values of 0.2, 0.5, and 0.8 indicate minor, moderate, and large significant effects, respectively [ 12 ]. We assessed the magnitude of heterogeneity using the I² statistic and P-value. For studies with low heterogeneity (I²≤50%, P > 0.1), we performed a meta-analysis using a fixed-effects model. For studies with high heterogeneity (I²≥50%, P < 0.1), we employed a random-effects model. In cases of high heterogeneity, we conducted subgroup analyses to identify sources of heterogeneity and to determine the most suitable frequency and duration of exercise for older adults. We considered P-values < 0.05 to indicate statistical singnificance.
Sensitivity analysis
We performed sensitivity analysis with alternating effect models and sequentially excluded the included studies. If the effect size significantly changes upon switching models or removing a specific study, the results of our study may be unstable.
Literature screening results
A total of 871 studies were identified. Finally, 16 studies were included [ 14 , 15 , 16 , 17 , 18 , 19 , 20 , 21 , 22 , 23 , 24 , 25 , 26 , 27 , 28 , 29 ]. The screening process is shown in Fig. 1 .
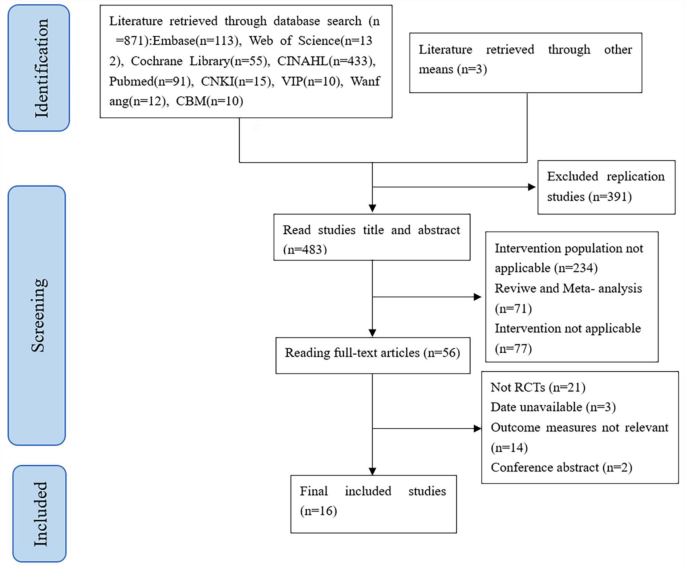
Literature screening flowchart
Literature characteristics and bias risk assessment
Studies from China [ 19 , 20 , 21 , 22 , 23 , 24 , 25 , 26 , 27 , 28 , 29 ], Turkey [ 15 ], Greece [ 17 ], Malaysia [ 18 ], Korea [ 16 ], and Sweden [ 14 ], involved a total of 1290 older adults, 644 of whom were receiving OEP training. The study population mainly consisted of community-dwelling older adults and older stroke patients. Details on the intervention content, frequency, and duration are available in Table 1 . All sixteen studies received a Grade B rating. One study described random allocation without detailing the method of randomization [ 16 ], leading us to assess it as high risk, while seven studies concealed the method of random allocation [ 13 , 14 , 15 , 16 , 20 , 22 , 24 , 26 ], assessed it as low risk. Three studies described the blinding of assessors [ 15 , 20 , 21 ], which we evaluated as low risk. The results of the literature bias risk assessment are presented in Fig. 2 a and b.
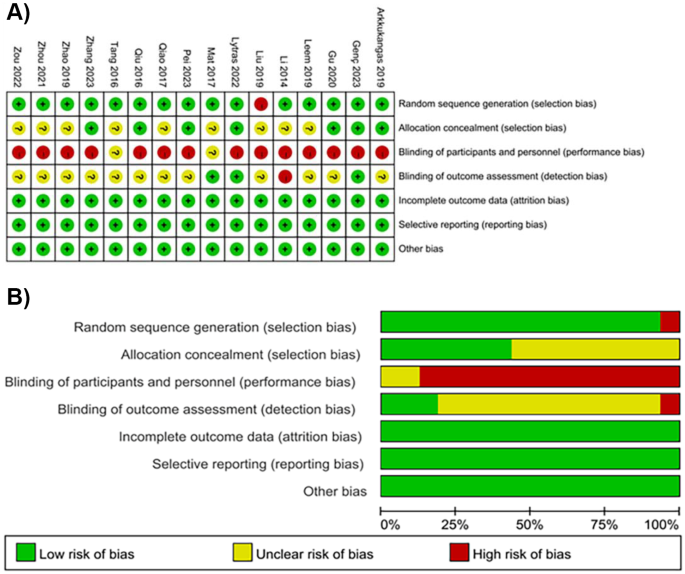
a Risk of bias assessment chart. b Risk of bias assessment chart (expressed as percentages)
Meta-analysis results
The overall meta-analysis results showed heterogeneity among study outcomes (I²=81%, P < 0.00001), leading us to use a random-effects model for aggregation. The results indicated [SMD = 0.96, 95%CI (0.68, 1.23), P < 0.00001], and the difference was statistically significant (Z = 6.78, P < 0.00001), (Fig. 3 ). The level of evidence was medium.
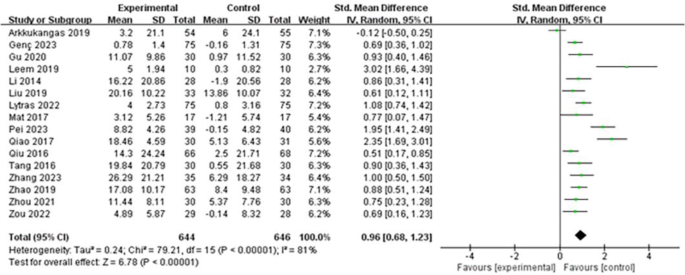
Forest plot of the effect of OEP on fall fear in older adults
Subgroup analysis
Among the 16 included studies, 10 had an intervention duration of ≤ 16 weeks, while 6 had an intervention duration of > 16 weeks. The results indicated that interventions lasting > 16 weeks [SMD = 1.12, 95% CI (0.75, 1.49), P < 0.00001] were more effective than those lasting ≤ 16 weeks [SMD = 0.86, 95% CI (0.49, 1.23), P < 0.00001], (Fig. 4 ). Comparing intervention durations of 16 weeks and 24 weeks, the 24-week intervention [SMD = 0.95, 95% CI (0.75, 1.15), P < 0.00001] was more effective than the 16-week intervention [SMD = 0.61, 95% CI (0.32, 0.90), P < 0.00001], (Fig. 5 ). Subgroup analysis based on intervention frequency revealed that interventions occurring more than twice a week [SMD = 0.99, 95% CI (0.64, 1.35), P < 0.00001] were more effective than those occurring twice or less per week [SMD = 0.97, 95% CI (0.68, 1.25), P < 0.00001], (Fig. 6 ). Subgroup analysis of different populations indicated that the application of OEP was most effective for older adults in communities or nursing homes [SMD = 1.03, 95% CI (0.50, 1.57), P < 0.00001], followed by patients with hemiplegia [SMD = 1.02, 95% CI (0.57, 1.47), P < 0.00001]. The effectiveness was lowest among older adults with joint diseases [SMD = 0.78, 95% CI (0.51, 1.05), P < 0.00001] compared to the previous two groups, (Fig. 7 ).
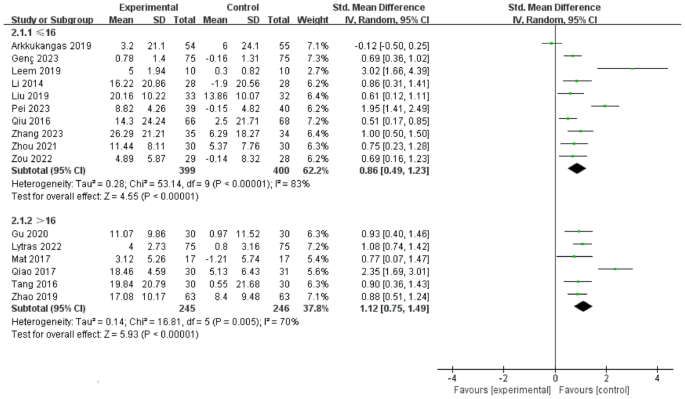
Forest plot of subgroup analysis for ≤ 16 weeks and > 16 weeks
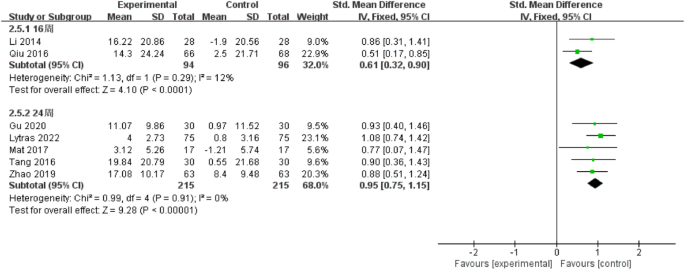
Forest plot of subgroup analysis for 16 weeks and 24 weeks
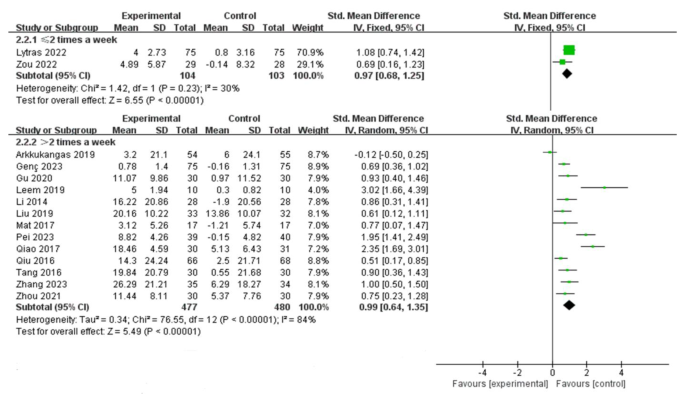
Forest plot of subgroup analysis for intervention frequency
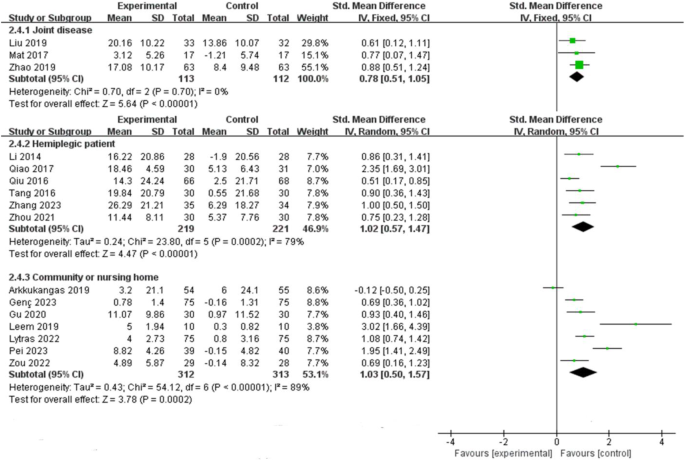
Forest plot of subgroup analysis for intervention populations
Sensitivity analysis and publication bias
We performed a sensitivity analysis using a switching effects model and by individually removing studies. The change in effect size was not significant according to the switching effects model. However, removing the studies by Qiao and Pei et al. [ 16 , 20 ]. led to a more noticeable change in effect size. We created a funnel plot for the included literature to test for publication bias, as shown in Fig. 8 , and conducted Egger’s test. The results, t = 1.66, P = 0.024, suggest the potential for publication bias. To further assess the stability of the results, we applied the trim-and-fill method and performed seven hypothetical studies. The combined results remained statistically significant, indicating stable aggregate outcomes.
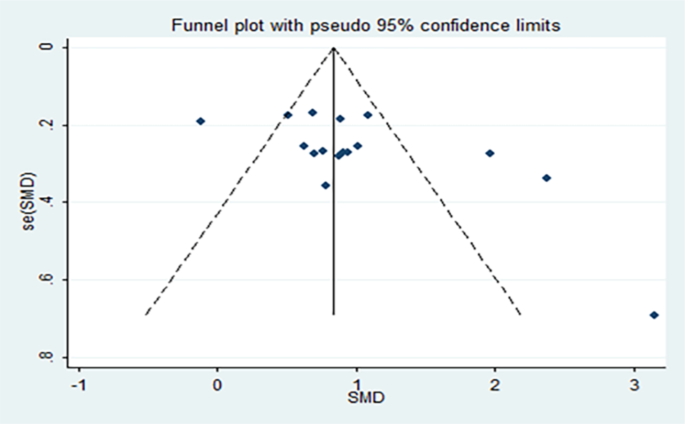
Publication bias test funnel plot
This study included 16 studies. Due to ethical concerns about the intervention experiments, all included studies did not blind participants, leading us to assess them as high risk in the bias risk assessment. However, we noted that in other meta-analyses, if the included studies indicated that the patient had signed an informed consent form, it could be rated as low risk in the assessment of whether the participant was blinded. Three studies implemented blinding for outcome assessors. Conversely, not blinding outcome assessors could lead researchers to be more inclined to report positive expected results, creating bias. All 16 studies clearly defined the inclusion and exclusion criteria, and they provided basic information about the literature, intervention content, outcome indicator assessment tools, and results.
The results of this study indicate that OEP can alleviate psychological FOF among older adults, which is consistent with the findings of Yu [ 11 ] and others. They conducted a descriptive analysis of two studies, and their results showed that the group-based OEP can reduce FOF in older adults. The group-based OEP promotes mutual encouragement among older adults in care facilities, enhancing their enthusiasm and adherence to the training. Research by Leem et al. [ 16 ] included the effects of individual OEP exercises and a combination of OEP with action observation training on FOF in older adults. Their study also compared the effects of the Otago Exercise Program (OEP) combined with action observation training versus OEP alone on fear of falling (FOF) in older adults. The results indicated that the combined training had a superior effect (-5.7 ± 2.11) compared to OEP alone (-5.0 ± 1.94). According to their findings, the difference between the effects of combined training and OEP alone was minimal. Each group in their study included only ten older adults. We believe that combined training extends the exercise duration, and it remains to be seen whether watching videos and prolonged training sessions might reduce exercise adherence in older adults. Further large-sample randomized controlled trials are needed to explore this issue. Currently, OEP alone appears to be more time-efficient and cost-effective, achieving satisfactory results compared to combined training. Future studies should explore the impact of combined versus individual training on compliance among older adults. The overall results showed considerable heterogeneity (I²=81%), which we attributed to clinical heterogeneity. In our study, 3 studies involved older adults with joint diseases, 6 studies involved older adults with hemiplegia, and 7 studies involved older adults in community or nursing institutions. The difference in research subjects might lead to varied intervention outcomes. Therefore we conducted subgroup analyses depending on the intervention population. The results showed that OEP had the greatest effect on older adults in the community and in institutions, followed by hemiplegic patients, but the difference in effects between the two groups was minimal, with an SMD difference of only 0.01, which we considered negligible. The intervention effects in older adults with osteoarthritis are less favorable compared to the other two groups of older adults. The reason for this difference is that in this group of older adults, they are not bothered by diseases and training in a group can increase motivation and compliance with the training. Hemiplegic patients have a strong desire for rehabilitation, hoping to recover as much as possible to their preillness state, leading to high motivation and adherence to exercise. In contrast, older adults with osteoarthritis may be reluctant to train due to pain caused by their condition. The OEP includes resistance exercises, which can cause pain during these activities, resulting in improper training and suboptimal outcomes. Ángeles et al. conducted a quasi-experimental study on 498 community-dwelling individuals aged 65–80 years in accordance with the OEP [ 30 ]. Their results indicated that FOF is a risk factor for falls in older adults, and that OEP can reduce FOF. Differences in outcome measurement tools might also contribute to heterogeneity, suggesting that future studies should use standardized measurement tools. Methodological heterogeneity, differences in intervention duration, and differences in intervention frequency among studies might also lead to varied outcomes. All included studies had a single intervention duration of more than 30 min, corroborated by Chiu et al. [ 31 ], whose results demonstrated that training sessions longer than 30 min are most effective for improving balance in older adults, as recommended in the OEP manual. Additionally, we conducted subgroup analyses based on the intervention period and frequency. The results indicate that interventions lasting longer than 16 weeks, with more than two sessions per week yielded better outcomes, aligning with the OEP manual’s recommendation of at least three sessions per week for 24 weeks. Our study included six interventions lasting more than 16 weeks, two for 16 weeks, and four for 24 weeks. Subgroup analysis revealed that 24-week outcomes surpassed 16-week outcomes; hence, we believe that training should last for at least 24 weeks, as suggested by the OEP manual. The OEP is a progressive training regimen, with a direct relationship between the intervention duration and its effectiveness. Generally, a longer intervention period allows for extended phases at each stage, enabling older adults to adapt well to the current training intensity before moving to the next phase. Shorter intervention periods often result in the study ending before the expected training intensity is reached, or older adults may be subjected to higher intensity training before they have adequately adapted. In the publication bias tests, Begg’s test showed no publication bias, but Egger’s test indicated some bias, suggesting that our results might be unstable due to publication bias. Thus, we used the trim-and-fill method to further verify the stability. The effect size remained significant and greater than zero after including seven hypothetical studies in the trim-and-fill analysis, with no reversal of the results, indicating the stability of our findings.
Our study also has limitations. The included studies did not blind participants, potentially affecting the quality and outcomes of the research and leading to results that might not align with reality. Additionally, due to the subjective nature of outcome measures derived from questionnaires and the lack of blinding among intervention subjects, measurement bias could lead to skewed results. Another limitation is the use of different measurement tools, a potential source of heterogeneity. Sensitivity analyses excluding studies by Qiao and Pei showed significant changes in effect size, identifying a source of heterogeneity. Furthermore, since follow-up durations varied, this study did not explore the long-term effects of OEP on older adults, which future research could investigate.
Current evidence suggests that OEP can alleviate psychological FOF in older adults, with interventions lasting more than 24 weeks, occurring more than twice a week, and has demonstrated high effectiveness when applied among older adults in community settings or elder care institutions. Currently, a variety of assessment tools are used in studies; future research is recommended to employ uniform measurement tools. The psychological issues related to falling in older adults are as important as the falls themselves. Future efforts should focus more on the psychological aspects of falls among older adults, and more high-quality, large-sample studies should be conducted to provide stronger evidence-based support.
Data availability
Data from this study are provided in the manuscript and can also be obtained by contacting the authors.
Legters K. Fear of falling. Phys Ther. 2002;82(3):264–72.
Article PubMed Google Scholar
Xiong W, Wang D, Ren W, Liu X, Wen R, Luo Y. The global prevalence of and risk factors for fear of falling among older adults: a systematic review and meta-analysis. BMC Geriatr. 2024;24(1):321. https://doi.org/10.1186/s12877-024-04882-w . Published 2024 Apr 5.
Article PubMed PubMed Central Google Scholar
Friedman SM, Munoz B, West SK, Rubin GS, Fried LP. Falls and fear of falling: which comes first? A longitudinal prediction model suggests strategies for primary and secondary prevention. J Am Geriatr Soc. 2002;50(8):1329–35. https://doi.org/10.1046/j.1532-5415.2002.50352.x .
Ho LYW, Lai CYY, Lai CKY, Ng SSM. Fatigue predicts level of community integration in people with stroke. Top Stroke Rehabil. 2024;31(5):464–73. https://doi.org/10.1080/10749357.2023.2298536 .
García-Gollarte F, Mora-Concepción A, Pinazo-Hernandis S, et al. Effectiveness of a supervised Group-based Otago Exercise Program on Functional Performance in Frail Institutionalized older adults: a Multicenter Randomized Controlled Trial. J Geriatr Phys Ther. 2023;46(1):15–25. https://doi.org/10.1519/JPT.0000000000000326 .
Campbell AJ, Robertson MC, Gardner MM, Norton RN, Tilyard MW, Buchner DM. Randomised controlled trial of a general practice programme of home based exercise to prevent falls in elderly women. BMJ. 1997;315(7115):1065–9. https://doi.org/10.1136/bmj.315.7115.1065 .
Article CAS PubMed PubMed Central Google Scholar
Montero-Odasso M, van der Velde N, Martin FC et al. World guidelines for falls prevention and management for older adults: a global initiative [published correction appears in Age Ageing. 2023;52(9):] [published correction appears in Age Ageing. 2023;52(10):]. Age Ageing. 2022;51(9):afac205. https://doi.org/10.1093/ageing/afac205 .
Mangione KK, Darreff H, Welsh M, et al. Feasibility of a modified Otago Exercise Program for older adults with cognitive vulnerability. J Appl Gerontol. 2023;42(7):1445–55. https://doi.org/10.1177/07334648231163050 .
Albornos-Muñoz L, Moreno-Casbas MT, Sánchez-Pablo C, et al. Efficacy of the Otago Exercise Programme to reduce falls in community-dwelling adults aged 65–80 years old when delivered as group or individual training. J Adv Nurs. 2018;74(7):1700–11. https://doi.org/10.1111/jan.13583 .
Wu S, Guo Y, Cao Z, et al. Effects of Otago exercise program on physical function in older adults: a systematic review and meta-analysis of randomized controlled trials. Arch Gerontol Geriatr Published Online May. 2024;3. https://doi.org/10.1016/j.archger.2024.105470 .
Peng Y, Yi J, Zhang Y, Sha L, Jin S, Liu Y. The effectiveness of a group-based Otago exercise program on physical function, frailty and health status in older nursing home residents: a systematic review and meta-analysis. Geriatr Nurs. 2023;49:30–43. https://doi.org/10.1016/j.gerinurse.2022.10.014 .
Yi M, Zhang W, Zhang X, Zhou J, Wang Z. The effectiveness of Otago exercise program in older adults with frailty or pre-frailty: a systematic review and meta-analysis. Arch Gerontol Geriatr. 2023;114:105083. https://doi.org/10.1016/j.archger.2023.105083 .
Article CAS PubMed Google Scholar
Higgins JP, Altman DG, Gøtzsche PC, et al. The Cochrane collaboration’s tool for assessing risk of bias in randomised trials. BMJ. 2011;343:d5928. https://doi.org/10.1136/bmj.d5928 . Published 2011 Oct 18.
Arkkukangas M, Söderlund A, Eriksson S, Johansson AC. Fall preventive Exercise with or without Behavior Change Support for Community-Dwelling older adults: a Randomized Controlled Trial with Short-Term follow-up. J Geriatr Phys Ther. 2019;42(1):9–17. https://doi.org/10.1519/JPT.0000000000000129 .
Genç FZ, Bilgili N. The effect of Otago exercises on fear of falling, balance, empowerment and functional mobility in the older people: Randomized controlled trial. Int J Nurs Pract. 2023;29(6):e13194. https://doi.org/10.1111/ijn.13194 .
Leem SH, Kim JH, Lee BH. Effects of Otago exercise combined with action observation training on balance and gait in the old people. J Exerc Rehabil. 2019;15(6):848–54. https://doi.org/10.12965/jer.1938720.360 . Published 2019 Dec 31.
Lytras D, Sykaras E, Iakovidis P, Komisopoulos C, Chasapis G, Mouratidou C. Effects of a modified Otago exercise program delivered through outpatient physical therapy to community-dwelling older adult fallers in Greece during the COVID-19 pandemic: a controlled, randomized, multicenter trial. Eur Geriatr Med. 2022;13(4):893–906. https://doi.org/10.1007/s41999-022-00656-y .
Mat S, Ng CT, Tan PJ, et al. Effect of Modified Otago exercises on postural balance, fear of falling, and fall risk in older fallers with knee osteoarthritis and impaired gait and balance: a secondary analysis. PM R. 2018;10(3):254–62. https://doi.org/10.1016/j.pmrj.2017.08.405 .
Zou Z, Chen Z, Ni Z, Hou Y, Zhang Q. The effect of group-based Otago exercise program on fear of falling and physical function among older adults living in nursing homes: a pilot trial. Geriatr Nurs. 2022;43:288–92. https://doi.org/10.1016/j.gerinurse.2021.12.011 .
Qiao YH, Cao HJ, Lian Y, Ni YC. Follow-up study on Otago exercise program on fear of falling in central hemiplegia patients in communities. West China Med J. 2017;32(1):17–20. https://doi.org/10.7507/1002-0179.201510008 .
Article Google Scholar
Liu H, Ji DH, Chi X, Gu LM, Bai CJ, Zhao QY. Effect of Otago Exercise Programme on balance ability and fear of falling in elderly patients with knee arthroplast. Chin Nurs Manage. 2019;19(1):133–8. https://doi.org/10.3969/j.issn.1672-1756.2019.01.030 .
Zhou Q, Yan DY. The effect of Otago exercise program on elderly patients with fear of falling. Chin Clin Nurs. 2021;13(1):30–1. https://doi.org/10.3969/j.issn.1674-3768.2021.01.008 .
Tang LJ, Yue LC, Liu CX, Wu L, Peng YY. The effect of Otago exercise on fall fear and balance ability of discharged patients with Parkinson’s disease. Chin J Rehabilitation Med. 2016;31(12):1383–5. https://doi.org/10.3969/j.issn.1001-1242.2016.12.020 .
Zhang XB, Wang XL, Yang XZ, Meng YC, Huo LZ, Zhang HX, Liu L, Wang LL. Effect of Otago Exercise based on empowerment theory in Elderly Stroke patients with fear of falling. Med Innov China. 2023;20(6):98–102. https://doi.org/10.3969/j.issn.1674-4985.2023.06.024 .
Li Y, Cheng Y, Zhao LR, Hu YQ. Effect of Otago Exercise Programme on fear of falling in elderly patients with stroke. Chin J Nurs. 2024;49(12):1442–7. https://doi.org/10.3761/j.issn.0254-1769.2014.12.007 .
Pei XY, Lu MQ, Wang FL, Zhang XL, Zhang P, Xing FM. Effect of Otago exercise training on fear of falling in older adults. Chin Nyrsing Res. 2023;37(19):3555–7. https://doi.org/10.12102/j.issn.1009-6493.2023.19.026 .
Zhao QY, Ji DH, Zhang Y. Effect of Otago Exercise Programme on fear of falling in Elderly patients with femoral Neck fractures. J Changchun Univ Chin Med. 2019;35(2):349–52. https://doi.org/10.13463/j.cnki.cczyy.2019.02.044 .
Qiu HZ. Observation on the preventive effect of Otago exercise on the fear of falling in stroke patients. J Chin Physician. 2016;18(5):734–6. https://doi.org/10.3760/cma.j.issn.1008-1372.2016.05.027 .
Gu Y, Shen Y, Yu XP, Zhu Y. Application effect of Otago exercise programme in the elderly with the fear of falling. Chin Nurs Res. 2020;34(7):1253–6. https://doi.org/10.12102/j.issn.1009-6493.2020.07.031 .
Ángeles CM, Laura AM, Consuelo CM, et al. The effect that the Otago Exercise Programme had on fear of falling in community dwellers aged 65–80 and associated factors. Arch Gerontol Geriatr. 2022;99:104620. https://doi.org/10.1016/j.archger.2022.104620 .
Chiu HL, Yeh TT, Lo YT, Liang PJ, Lee SC. The effects of the Otago Exercise Programme on actual and perceived balance in older adults: a meta-analysis. PLoS ONE. 2021;16(8):e0255780. https://doi.org/10.1371/journal.pone . Published 2021 Aug 6.
Download references
Acknowledgements
Not applicable.
No external funding was provided for this study.
Author information
Jianlong Han and Hongwei Wang are co-first authors.
Authors and Affiliations
Jiamusi University, Jiamusi, China
Jianlong Han, Hongwei Wang, Yunxin Ding & Qing Li
The Fourth Affiliated Hospital of Harbin Medical University, Harbin, China
Huanqie Zhai
The First Affiliated Hospital of Jiamusi University, Jiamusi, China
You can also search for this author in PubMed Google Scholar
Contributions
J.L.H. and H.W.W. Write manuscript drawings make picture and tables; Y.X.D., H.Q.Z. and Q.L. Literature screening and data extraction; S.L.H. Manuscript revision and implementation supervision. All authors reviewed the manuscript.
Corresponding author
Correspondence to Shuling He .
Ethics declarations
Ethics approval and consent to participate, consent for publication.
Not applicable. All participants agreed to publish.
Competing interests
The authors declare no competing interests.
Additional information
Publisher’s note.
Springer Nature remains neutral with regard to jurisdictional claims in published maps and institutional affiliations.
Rights and permissions
Open Access This article is licensed under a Creative Commons Attribution 4.0 International License, which permits use, sharing, adaptation, distribution and reproduction in any medium or format, as long as you give appropriate credit to the original author(s) and the source, provide a link to the Creative Commons licence, and indicate if changes were made. The images or other third party material in this article are included in the article’s Creative Commons licence, unless indicated otherwise in a credit line to the material. If material is not included in the article’s Creative Commons licence and your intended use is not permitted by statutory regulation or exceeds the permitted use, you will need to obtain permission directly from the copyright holder. To view a copy of this licence, visit http://creativecommons.org/licenses/by/4.0/ . The Creative Commons Public Domain Dedication waiver ( http://creativecommons.org/publicdomain/zero/1.0/ ) applies to the data made available in this article, unless otherwise stated in a credit line to the data.
Reprints and permissions
About this article
Cite this article.
Han, J., Wang, H., Ding, Y. et al. Effect of Otago exercise on fear of falling in older adults: a systematic review and meta-analysis. BMC Sports Sci Med Rehabil 16 , 132 (2024). https://doi.org/10.1186/s13102-024-00917-2
Download citation
Received : 09 May 2024
Accepted : 31 May 2024
Published : 14 June 2024
DOI : https://doi.org/10.1186/s13102-024-00917-2
Share this article
Anyone you share the following link with will be able to read this content:
Sorry, a shareable link is not currently available for this article.
Provided by the Springer Nature SharedIt content-sharing initiative
- Otago exercise programme
- Older adults
- Fear of falling
- Meta-analysis
BMC Sports Science, Medicine and Rehabilitation
ISSN: 2052-1847
- General enquiries: [email protected]

COMMENTS
A systematic review collects all possible studies related to a given topic and design, and reviews and analyzes their results [ 1 ]. During the systematic review process, the quality of studies is evaluated, and a statistical meta-analysis of the study results is conducted on the basis of their quality. A meta-analysis is a valid, objective ...
Review Manager (RevMan) is a web-based software that manages the entire literature review process and meta-analysis. The meta-analyst uploads all studies to RevMan library, where they can be managed and exanimated for inclusion. Like CMA, RevMan enables authors to conduct overall analysis and moderator analysis. 4.4.6.3 Stata
A systematic review is an article that synthesizes available evidence on a certain topic utilizing a specific research question, pre-specified eligibility criteria for including articles, and a systematic method for its production. Whereas a meta-analysis is a quantitative, epidemiological study design used to assess the results of articles ...
2.1 Step 1: defining the research question. The first step in conducting a meta-analysis, as with any other empirical study, is the definition of the research question. Most importantly, the research question determines the realm of constructs to be considered or the type of interventions whose effects shall be analyzed.
Meta-analysis is a statistical tool that provides pooled estimates of effect from the data extracted from individual studies in the systematic review. The graphical output of meta-analysis is a forest plot which provides information on individual studies and the pooled effect. Systematic reviews of literature can be undertaken for all types of ...
Graphical summary of a meta-analysis of over 1,000 cases of diffuse intrinsic pontine glioma and other pediatric gliomas, in which information about the mutations involved as well as generic outcomes were distilled from the underlying primary literature. Meta-analysis is the statistical combination of the results of multiple studies addressing a similar research question.
First, meta-analysis provides an organized approach for handling a large number of studies. Second, the process is systematic and documented in great detail, which allows readers to evaluate the researchers' decisions and conclusions. Third, meta-analysis allows researchers to examine an effect within a collection of studies in a more ...
A systematic review is a summary of the medical literature that uses explicit and reproducible methods to systematically search, critically appraise, and synthesize on a specific issue. ... The steps undertaken in evaluating the study quality are early definition of study quality and criteria, setting up a good scoring system, developing a ...
Steps of a Systematic Review. Develop a focused research question. Define inclusion/exclusion criteria. Select the outcomes for your review. Find the studies. Abstract the data. Assess quality of the data. Explore data (heterogeneity) Synthesize the data descriptively and inferentially via meta-analysis if appropriate.
A meta-analysis cannot exist with a pre-existing systematic review. Grant & Booth (2009) state that "although many systematic reviews present their results without statistically combining data [in a meta-analysis], a good systematic review is essential to a meta-analysis of the literature" (p.98).
A meta-analysis is the use of statistical methods to summarize the results of a systematic review. Not every systematic review contains a meta-analysis. A meta-analysis may not be appropriate if the designs of the studies are too different, if there are concerns about the quality of studies, if the outcomes measured are not sufficiently similar ...
A meta-analysis is a statistical analysis, not a type of review. A meta-analysis is a technique to synthesize results from multiple studies. It's a statistical analysis that combines the results of two or more studies, usually to estimate an effect size. Systematic review vs. literature review. A literature review is a type of review that ...
A systematic review is a form of research done collecting, appraising and synthesizing evidence to answer a particular question, in a very transparent and systematic way. Data (or evidence) used in systematic reviews have their origin in scholarly literature - published or unpublished. So, findings are typically very reliable.
A meta-analysis (Clinical Vignette 2), much like a systematic review and often an extension of one, also hinges on a systematic and exhaustive search of the literature. A meta-analysis differs from a systematic review in that instead of simply collecting and analysing the data, it employs statistical methods to quantitatively synthesize the ...
Definition: A specialized subset of systematic reviews, meta-analysis is a statistical technique for combining the findings from disparate quantitative studies and using the pooled data to come to new statistical conclusions.Not all systematic reviews include meta-analysis, but all meta-analyses are found in systematic reviews. Aim: To synthesize evidence across studies to detect effects ...
Literature Reviews and Meta Analysis. January 2010. DOI: 10.1007/978--387-09757-2_18. In book: Handbook of Clinical Psychology Competencies. Publisher: Springer New York. Editors: Jay C. Thomas ...
One such way is to conduct a literature review and combine it with a meta-analysis of a relevant topic to provide some evidence of effect. This strategy has been used effectively in articles published in higher-ranked journals (e.g., Carrillat et al., 2018 ; Edeling & Himme, 2018 ; Verlegh & Steenkamp, 1999 ).
A meta-analysis is a type of systematic review that summarises and compares data using statistical techniques. Aim/Definition. A scholarly literature review summarises evidence on a topic using a formal writing style and adopting qualitative data collection methods to select and interpret studies. Can involve some quantitative analysis.
Literature Search. One of the first steps when reviewing a meta-analysis is to determine whether the authors conducted a comprehensive search for clinical trials and other types of studies, some of which may be unpublished, related to the research question. The information sources that were searched should be provided.
Sometimes the results of all of the studies found and included in a systematic review can be summarized and expressed as an overall result. This is known as a meta-analysis. The overall outcome of the studies is often more conclusive than the results of individual studies. But it only makes sense to do a meta-analysis if the results of the ...
Method for systematic literature review and meta-analysis studies: Name and reference of original method: 1). ... Categorize the data on the iterative definition and ready it for further analysis work: Analysis: Data analysis: Quantitative categories, description, and narrative analysis of the organized data:
The document discusses the complex process of conducting a meta-analysis literature review. It explains that a meta-analysis requires meticulously searching literature, extracting and synthesizing data from multiple studies, and using statistical methods to analyze findings and derive conclusions. The challenges include navigating publication bias, heterogeneity between studies, and selecting ...
Compared to a mere literature review, meta-analysis can provide precise quantitative effects (Grant and Booth, 2009).Meta-analysis can integrate various empirical research results to calculate the ...
Findings This systematic review and bayesian meta-analysis of 18 randomized trials that included 9108 critically ill adults with sepsis or septic shock reported a 99.1% posterior probability that prolonged infusions were associated with lower 90-day mortality compared with intermittent infusions (risk ratio, 0.86).
To address this gap, we conducted a comprehensive systematic literature review (SLR) on the clinical manifestations of chikungunya, and proceeded with a robust yet flexible meta-analysis. This approach allowed us to provide estimates on a broad spectrum of endpoints on the health outcomes of chikungunya.
This study aims at systematically reviewing and meta-analysing the literature on effects of exercise training on CO in patients with HFpEF. ... It is acknowledged that there might be diversity within the study populations concerning the definition of HFpEF. ... The aim of this systematic review and meta-analysis is to investigate the effects of ...
The purpose of this systematic review and meta-analysis was to examine the relationship between empathy and burnout in medical students. Burnout, defined as a state of emotional exhaustion, depersonalization, and a reduced sense of personal accomplishment [], has become a pervasive issue within the medical field.One systematic review and meta-analysis of 4,664 international medical residents ...
This systematic review and meta-analysis followed the PRISMA guidelines (checklist in Additional file 1, pp. 2-4). The study protocol was registered at PROSPERO (registration CRD42020177558). ... Given the lack of evidence in the literature (i.e. no existing meta-analysis examined the efficacy of IT vs. untreated control), we performed an ...
This systematic review and meta-analysis adhered to the Preferred Reporting of Items for Systematic Reviews and Meta-Analyses (PRISMA) guidelines, as detailed in Additional file 1: Table S1. The methodology employed in this review was guided by the Cochrane Handbook for Systematic Reviews of Interventions.
Therefore, this study integrates the relevant literature to provide evidence supporting interventions aimed at alleviating FOF among older adults. To evaluate the impact of OEP on FOF in older adults through meta-analysis. We searched ten databases using computer systems, covering all records up to May 1, 2024.