Google Scholar Metrics
Google Scholar Metrics provide an easy way for authors to quickly gauge the visibility and influence of recent articles in scholarly publications. Scholar Metrics summarize recent citations to many publications, to help authors as they consider where to publish their new research.
To get started, you can browse the top 100 publications in several languages , ordered by their five-year h-index and h-median metrics. To see which articles in a publication were cited the most and who cited them, click on its h-index number to view the articles as well as the citations underlying the metrics.
You can also explore publications in research areas of your interest. To browse publications in a broad area of research, select one of the areas in the left column. For example: Engineering & Computer Science or Health & Medical Sciences .
To explore specific research areas, select one of the broad areas, click on the "Subcategories" link and then select one of the options. For example: Databases & Information Systems or Development Economics.
Browsing by research area is, as yet, available only for English publications. You can, of course, search for specific publications in all languages by words in their titles.
Scholar Metrics are currently based on our index as it was in July 2024 .
Available Metrics
The h-index of a publication is the largest number h such that at least h articles in that publication were cited at least h times each. For example, a publication with five articles cited by, respectively, 17, 9, 6, 3, and 2, has the h-index of 3.
The h-core of a publication is a set of top cited h articles from the publication. These are the articles that the h-index is based on. For example, the publication above has the h-core with three articles, those cited by 17, 9, and 6.
The h-median of a publication is the median of the citation counts in its h-core. For example, the h-median of the publication above is 9. The h-median is a measure of the distribution of citations to the articles in the h-core.
Finally, the h5-index , h5-core , and h5-median of a publication are, respectively, the h-index, h-core, and h-median of only those of its articles that were published in the last five complete calendar years.
We display the h5-index and the h5-median for each included publication. We also display an entire h5-core of its articles, along with their citation counts, so that you can see which articles contribute to the h5-index. And there's more! Click on the citation count for any article in the h5-core to see who cited it.
Coverage of Publications
Scholar Metrics currently cover articles published between 2019 and 2023 , both inclusive. The metrics are based on citations from all articles that were indexed in Google Scholar in July 2024 . This also includes citations from articles that are not themselves covered by Scholar Metrics.
Since Google Scholar indexes articles from a large number of websites, we can't always tell in which journal a particular article has been published. To avoid misidentification of publications, we have included only the following items:
- journal articles from websites that follow our inclusion guidelines ;
- selected conference articles in Engineering and Computer Science.
Furthermore, we have specifically excluded the following items:
- court opinions, patents, books, and dissertations;
- publications with fewer than 100 articles published between 2019 and 2023;
- publications that received no citations to articles published between 2019 and 2023.
Overall, Scholar Metrics cover a substantial fraction of scholarly articles published in the last five years. However, they don't currently cover a large number of articles from smaller publications.
Inclusion and Corrections
If you can't find the journal you're looking for, try searching by its abbreviated title or alternate title. There're sometimes several ways to refer to the same publication. (Fun fact: we've seen 959 ways to refer to PNAS.)
If you're wondering why your journal is not included, or why it has fewer citations than it surely deserves, that is often a matter of configuring your website for indexing in Google Scholar. Please refer to the inclusion manual . Also, keep in mind that Scholar Metrics only include publications with at least a hundred articles in the last five years.
- Privacy & Terms


Journal impact factors
Announcing the latest impact factors.
The journal impact factor (JIF), as calculated by Clarivate Analytics, is a measure of the average number of times articles from a two-year time frame have been cited in a given year, according to citations captured in the Web of Science database.
The 2023 JIF (released in 2024), for example, was calculated as follows:
A = the number of times articles which published in 2021–2022 were cited in indexed journals during 2023
B = the total number of research and review articles from the journal published in 2021–2022
2023 JIF = A/B
This listing includes only journals that have a 2023 JIF. For a full list of journals published by APA, please visit the Journals homepage to browse our portfolio by title or subject.
Journal Title | 2023 JIF | 2023 5-Year JIF | Journal Citation Reports Subject Category |
---|---|---|---|
2.3 | 3.8 | ||
12.3 | 16.2 | ||
2.4 | 2.9 | ||
1.6 | 2.0 | ||
1.3 | 2.4 | ||
1.1 | 1.5 | ||
2.9 | 4.2 | ||
1.2 | -- | ||
4.7 | 7.8 | ||
0.9 | -- | ||
1.9 | 1.8 | ||
3.2 | 3.8 | ||
1.0 | -- | ||
3.1 | 5.0 | ||
0.8 | 1.6 | ||
3.4 | 4.5 | ||
1.1 | -- | ||
2.4 | 2.6 | ||
1.2 | 1.9 | ||
1.8 | 2.4 | ||
3.1 | 4.9 | ||
1.1 | 0.8 | ||
2.1 | 4.4 | ||
9.4 | 11.8 | ||
2.8 | 4.7 | ||
1.1 | 1.6 | ||
4.5 | 6.3 | ||
3.8 | 5.5 | ||
2.5 | 3.1 | ||
5.6 | 6.7 | ||
1.2 | 1.4 | ||
2.7 | 3.0 | ||
3.7 | 4.7 | ||
2.1 | 2.6 | ||
2.2 | 2.9 | ||
2.3 | 3.3 | ||
2.1 | 2.6 | ||
0.6 | 0.9 | ||
1.6 | 1.3 | ||
5.9 | 11.7 | ||
6.4 | 9.2 | ||
3.1 | 7.8 | ||
1.8 | 2.6 | ||
1.0 | 1.7 | ||
2.4 | 3.2 | ||
3.0 | -- | ||
2.6 | 3.1 | ||
0.9 | 1.4 | ||
3.0 | 3.5 | ||
1.5 | 2.0 | ||
1.8 | 2.3 | ||
1.0 | 1.2 | ||
3.3 | 4.9 | ||
17.3 | 30.3 | ||
7.6 | 11.5 | ||
5.1 | 8.0 | ||
1.9 | 2.9 | ||
2.7 | 5.6 | ||
3.7 | 3.8 | ||
3.2 | 3.7 | ||
2.7 | 4.9 | ||
1.4 | -- | ||
2.7 | 3.0 | ||
2.1 | 3.0 | ||
2.2 | 2.9 | ||
3.6 | -- | ||
2.4 | 4.1 | ||
2.3 | 2.4 | ||
0.6 | -- | ||
2.6 | 4.6 | ||
11.7 | -- | ||
1.9 | 3.2 | ||
3.9 | 3.2 | ||
1.4 | 1.7 | ||
2.7 | -- | ||
1.8 | 2.2 | ||
1.9 | -- | ||
2.3 | -- |
Following the release of the 2023 Journal Citation Reports (JCR) from Clarivate Analytics, APA Publishing is pleased to report that Journal Impact Factors (JIFs) have been assigned to 89% (79) of our titles. Among our ranked journals, 24% are in the top 10 of their categories and 46% are in their category’s top quartile.
Notably, 15 APA-published journals indexed in Clarivate’s Emerging Sources Citation Index received their first-ever category rankings this year, including:
- Qualitative Psychology (11.7 JIF; ranked 7 of 218 in Multidisciplinary Psychology; published on behalf of The Society for Qualitative Inquiry in Psychology, a section of APA Division 5 )
- Couple and Family Psychology: Research and Practice (1.9 JIF; ranked 24 of 66 in Family Studies; published on behalf of APA Division 43: Society for Couple and Family Psychology )
- Stigma and Health (2.7 JIF; ranked 27 of 76 in Social Psychology and 136 of 403 in Public, Environmental, & Occupational Health)
- Translational Issues in Psychological Science (1.9 JIF; ranked 47 of 92 in Psychology and 92 of 218 in Multidisciplinary Psychology)
- Traumatology (2.3 JIF; ranked 70 of 180 in Clinical Psychology, 73 of 218 in Multidisciplinary Psychology, and 131 of 276 in Psychiatry; published on behalf of the Green Cross Academy of Traumatology )
Twenty APA-published titles saw an increase in their JIF, and 31 journals rose in rank within their category. Twenty-six journals rose in quartile within their category. Some examples include the following:
- Journal of Neuroscience, Psychology, and Economics saw a 129% increase in its JIF, rising from 0.7 to 1.6 and jumping in rank from 135th to 109th in the Multidisciplinary Psychology category (and from 344th to 273rd in the Economics category)
- History of Psychology saw a 120% increase in its JIF, rising from 0.5 to 1.1 and jumping in rank from 140th to 132nd in the Multidisciplinary Psychology category (and from 27th to 10th in the History of Social Sciences category).
- Asian American Journal of Psychology , published on behalf of the Asian American Psychological Association, saw a 60% increase in its JIF, rising from 1.5 to 2.4 and jumping in rank from 104th to 65th in the Multidisciplinary Psychology category (and from 13th to 6th in the Ethnic Studies category).
Other portfolio highlights:
- APA publishes 4 journals in the top 10 of the large and diverse Psychology, Multidisciplinary category: Psychological Bulletin (ranking 3rd out of 218 journals, 17.3 JIF), American Psychologist (ranking 6th, 12.3 JIF), Qualitative Psychology (ranking 7th, 11.7 JIF; published on behalf of The Society for Qualitative Inquiry in Psychology, a Section of APA Division 5), and Psychological Methods (ranking 10th, 7.6 JIF).
- Five more APA journals are ranked in the top quartile of the Psychology, Multidisciplinary category: Psychological Review (ranking 19th, 5.1 JIF), Psychology of Sexual Orientation and Gender Diversity (ranking 27th, 3.6 JIF; published on behalf of APA Division 44: Society for the Psychology of Sexual Orientation and Gender Diversity ), Psychology of Addictive Behaviors (ranking 39th, 3.2 JIF; published on behalf of APA Division 50: Society of Addiction Psychology ), Motivation Science (ranking 44th, 3.0 JIF, published on behalf of Society for the Science of Motivation ), and Canadian Psychology / Psychologie canadienne (ranking 46th, 2.9 JIF; published on behalf of the Canadian Psychological Association ).
- Psychological Bulletin remains the journal with the highest JIF in APA’s portfolio (17.3 JIF). In addition to ranking 3rd out of the 218 journals in the Psychology, Multidisciplinary category, it also ranks 3rd out of the 92 journals in the Psychology category.
- Two APA journals rank in the top 20 of the Psychology, Applied category: Journal of Applied Psychology (ranking 3rd, 9.4 JIF) and Journal of Occupational Health Psychology (ranking 12th, 5.9 JIF). Journal of Applied Psychology earned the most all-time citations (51,849) in this 113-journal category.
- Psychoanalytic Psychology (1.0 JIF), published on behalf of APA Division 39: Division of Psychoanalysis , ranks 4th of the 13 titles in the Psychology, Psychoanalysis category, marking over a decade in the category’s top quartile.
- Four APA titles rank in the top 25 of the Psychology, Experimental category: Journal of Experimental Psychology: General (ranking 9th of 99, 3.7 JIF), Emotion (ranking 12th, 3.4 JIF), Journal of Applied Research in Memory and Cognition (ranked 23rd, 2.8 JIF; published on behalf of Society for Applied Research in Memory and Cognition), and Psychology of Aesthetics, Creativity, and the Arts (ranking 25th, 2.7 JIF; published on behalf of APA Division 10: Society for the Psychology of Aesthetics, Creativity, and the Arts ).
- Journal of Consulting and Clinical Psychology ranks 3rd for all-time citations (20,413) in the 180-journal Psychology, Clinical category.
Six APA titles rank in the top quartile of that category, including Clinical Psychology: Science and Practice (ranking 13th, 4.7 JIF; published on behalf of APA Division 12: Society of Clinical Psychology ), Journal of Consulting and Clinical Psychology (ranking 17th, 4.5 JIF), and Health Psychology (ranking 36th, 3.1 JIF, published on behalf of APA Division 38: Society for Health Psychology ).
Four APA titles rank in the top 25 of the Psychology, Educational category: Journal of Educational Psychology (ranking 3rd out of 74, 5.6 JIF), Journal of Counseling Psychology (ranking 11th, 3.8 JIF), School Psychology (ranking 5th, 3.9 JIF, the journal’s highest rank and JIF to date; published on behalf of APA Division 16: School Psychology ), and Journal of Diversity in Higher Education (ranking 24th, 2.5 JIF; published on behalf of the National Association of Diversity Officers in Higher Education ).
Journal of Educational Psychology received a total of 20,586 citations, the second highest in the category.
- Journal of Personality and Social Psychology (6.4 JIF) again earned the most all-time citations (79,644) in the competitive Psychology, Social category and ranked 4th of 76 journals, marking over a decade as one of the top 5 journals in this category.
Showcasing the breadth and depth of our program, journals published by APA are also highly ranked in 23 categories related to psychology found in the Arts and Humanities Citation Index, the Social Sciences Citation Index, and the Science Citation Index Expanded. Some examples include the following:
- Humanities, Multidisciplinary : Psychology of Aesthetics, Creativity, and the Arts (2.7 JIF), published on behalf of APA Division 10: Society for the Psychology of Aesthetics, Creativity, and the Arts, is ranked number 2 out of the 146 journals in the Humanities, Multidisciplinary category.
- Ethnic Studies : Cultural Diversity & Ethnic Minority Psychology (3.2 JIF), published on behalf of APA Division 45: Society for the Psychological Study of Culture, Ethnicity, and Race , retained its number 1 ranking out of the 39 journals in the growing Ethnic Studies category. We are proud to see this journal as the established go-to source for research on critical societal issues.
- Religion : Psychology of Religion and Spirituality (2.2 JIF), published on behalf of APA Division 36: Society for the Psychology of Religion and Spirituality , is ranked 4th out of the 339 journals in Religion , a category in Clarivate’s Arts and Humanities Citation Index.
- Social Work : American Journal of Orthopsychiatry (2.3 JIF), published on behalf of the Global Alliance for Behavioral Health and Social Justice , ranks 8th out of the 91 titles in the expanding Social Work category.
- Rehabilitation : Two APA journals indexed in the Rehabilitation category remain in the category’s top two quartiles: Rehabilitation Psychology (ranking 56th out of 73, 1.9 JIF) and Psychiatric Rehabilitation Journal (ranking 66th, 1.8 JIF).
- Communication : Psychology of Popular Media (2.1 JIF) is ranked 59th out of the 227 journals in the Communication category.
- Criminology & Penology : Psychological Violence (2.4 JIF) remains in the top quartile of the Criminology & Penology category, ranking 14th out of 113.
- Law: The two APA journals listed in the Law category remain in the category’s top 10% by JIF. Psychology, Public Policy, and the Law (2.3 JIF) is ranked 24 of 421 journals. Law and Human Behavior (2.4 JIF), published on behalf of APA Division 41: American Psychology-Law Society, is ranked 20th of 421 in the Law category, putting it in the top 5% of Law journals.
- Management : Journal of Applied Psychology (9.4 JIF) remains in the top quartile of the competitive Management category, ranking 14th out of 401 journals. The journal also has the highest total citations in the category (51,849).
APA Publishing is grateful to our editors, authors, reviewers, and publishing partners for their dedication to scholarship. Thank you for publishing with APA Journals.
- APA publishing resources
- Reviewer Resource Center
- Editor resource center
- Why publish with APA Journals™
- Equity, diversity, and inclusion in APA Journals™
APA Publishing Insider
APA Publishing Insider is a free monthly newsletter with tips on APA Style, open science initiatives, active calls for papers, research summaries, and more.
Visit the APA Style website for style and grammar guidelines, free instructional aids, reference examples, the APA Style blog, APA Style products, and more.
Contact Journals
Unfortunately we don't fully support your browser. If you have the option to, please upgrade to a newer version or use Mozilla Firefox , Microsoft Edge , Google Chrome , or Safari 14 or newer. If you are unable to, and need support, please send us your feedback .
We'd appreciate your feedback. Tell us what you think! opens in new tab/window
Measuring a journal’s impact
Journal-level metrics.
Metrics have become a fact of life in many - if not all - fields of research and scholarship. In an age of information abundance (often termed ‘information overload’), having a shorthand for the signals for where in the ocean of published literature to focus our limited attention has become increasingly important.
Research metrics are sometimes controversial, especially when in popular usage they become proxies for multidimensional concepts such as research quality or impact. Each metric may offer a different emphasis based on its underlying data source, method of calculation, or context of use. For this reason, Elsevier promotes the responsible use of research metrics encapsulated in two “golden rules”. Those are: always use both qualitative and quantitative input for decisions (i.e. expert opinion alongside metrics), and always use more than one research metric as the quantitative input. This second rule acknowledges that performance cannot be expressed by any single metric, as well as the fact that all metrics have specific strengths and weaknesses. Therefore, using multiple complementary metrics can help to provide a more complete picture and reflect different aspects of research productivity and impact in the final assessment.
On this page we introduce some of the most popular citation-based metrics employed at the journal level. Where available, they are featured in the “Journal Insights” section on Elsevier journal homepages ( for example opens in new tab/window ), which links through to an even richer set of indicators on the Journal Insights homepage ( for example opens in new tab/window ).
CiteScore metrics
CiteScore metrics are a suite of indicators calculated from data in Scopus, the world’s leading abstract and citation database of peer-reviewed literature.
Calculating the CiteScore is based on the number of citations to documents (articles, reviews, conference papers, book chapters, and data papers) by a journal over four years, divided by the number of the same document types indexed in Scopus and published in those same four years. For more details, see this FAQ opens in new tab/window .
CiteScore is calculated for the current year on a monthly basis until it is fixed as a permanent value in May the following year, permitting a real-time view on how the metric builds as citations accrue. Once fixed, the other CiteScore metrics are also computed and contextualise this score with rankings and other indicators to allow comparison.
CiteScore metrics are:
Current: A monthly CiteScore Tracker keeps you up-to-date about latest progression towards the next annual value, which makes next CiteScore more predictable.
Comprehensive: Based on Scopus, the leading scientific citation database.
Clear: Values are transparent and reproducible to individual articles in Scopus.
The scores and underlying data for nearly 26,000 active journals, book series and conference proceedings are freely available at www.scopus.com/sources opens in new tab/window or via a widget (available on each source page on Scopus.com) or the Scopus API.
SCImago Journal Rank (SJR)
SCImago Journal Rank (SJR) is based on the concept of a transfer of prestige between journals via their citation links. Drawing on a similar approach to the Google PageRank algorithm - which assumes that important websites are linked to from other important websites - SJR weights each incoming citation to a journal by the SJR of the citing journal, with a citation from a high-SJR source counting for more than a citation from a low-SJR source. Like CiteScore, SJR accounts for journal size by averaging across recent publications and is calculated annually. SJR is also powered by Scopus data and is freely available alongside CiteScore at www.scopus.com/sources opens in new tab/window .
Source Normalized Impact per Paper (SNIP)
Source Normalized Impact per Paper (SNIP) is a sophisticated metric that intrinsically accounts for field-specific differences in citation practices. It does so by comparing each journal’s citations per publication with the citation potential of its field, defined as the set of publications citing that journal. SNIP therefore measures contextual citation impact and enables direct comparison of journals in different subject fields, since the value of a single citation is greater for journals in fields where citations are less likely, and vice versa. SNIP is calculated annually from Scopus data and is freely available alongside CiteScore and SJR at www.scopus.com/sources opens in new tab/window .
Journal Impact Factor (JIF)
Journal Impact Factor (JIF) is calculated by Clarivate Analytics as the average of the sum of the citations received in a given year to a journal’s previous two years of publications (linked to the journal, but not necessarily to specific publications) divided by the sum of “citable” publications in the previous two years. Owing to the way in which citations are counted in the numerator and the subjectivity of what constitutes a “citable item” in the denominator, JIF as received sustained criticism for many years for its lack of transparency and reproducibility and the potential for manipulation of the metric. Available for only 11,785 journals (Science Citation Index Expanded plus Social Sciences Citation Index, per December 2019), JIF is based on an extract of Clarivate’s Web of Science database, and includes citations that could not be linked to specific articles in the journal, so-called unlinked citations.
Although originally conceived as an author-level metric, the h-index (and some of its numerous variants) have come to be applied to higher-order aggregations of research publications, including journals. A composite of productivity and citation impact, h-index is defined as the greatest number of publications h for which the count of lifetime citations is greater than or equal to h . Being bound at the upper limit only by total productivity, h-index favours older and more productive authors and journals. As h-index can only ever rise, it is also insensitive to recent changes in performance. Finally, the ease of increasing h-index does not scale linearly: an author with an h-index of 2 needs only publish a 3rd paper and have all three of them cited at least 3 times to rise to an h-index of 3; an author with an h-index of 44 must publish a 45th paper and have it and all the other attain 45 citations each before progressing to an h-index of 45. h-index is therefore of limited usefulness to distinguish between authors, since most have single-digit h-indexes.
University Library
Introduction to Impact Factor and Other Research Metrics
- Types of Metrics
Impact Factor
- Identifying Journals
- More Resources
More Information
- About Journal Impact Factor Visit this article published by Thomson Reuters on journal impact factor to learn more about the bibliometric and how it measures importance.
- Annual Reviews Rankings in JCR Visit this page to see how Annual Review Journals currently rank in Journal Citation Reports.
- SCImago Journal and Country Rank The SCImago Journal & Country Rank is a publicly available portal that includes the journals and country scientific indicators developed from the information contained in the Scopus database.
Ask a Librarian

Impact factor, or Journal Impact Factor, is a measure of the frequency with which the "average article" published in a given scholarly journal has been cited in a particular year or period and is often used to measure or describe the importance of a particular journal to its field. Impact factor was originally developed by Eugene Garfield, the founder of Institute of Scientific Information, which is now a part of Clarivate Analytics. Journal Impact Factor can be found in the Journal Citation Reports or the JCR, as it's commonly known. Over the years various organizations have been created similar journal-level metrics, such as SCImago Journal & Country Rank .
This page describes how to find impact factor in Journal Citation Reports .
Journal Citation Reports
Clarivate Analytics (formerly Institute for Scientific Information (ISI)) ranks, evaluates, and compares journals within subject categories and publishes the results in Journal Citation Reports . Journal Citation Reports provides ranking for journals in science, technology, and the social sciences. For every journal, the database collects and/or calculates information such as:
- citation/article counts
- impact factor
- immediacy index
- cited half-life
- citing half-life
- source data listing
- citing journal listing
- cited journal listing
- publisher information
- subject categories
Find Journal Citation Reports (JCR)
Follow the instructions below to find the Journal Citation Reports using the Library's resources.
- Begin at the Library homepage .
- Click on the orange tab that says "Find Materials," then scroll down until you see a laptop icon with the words "Databases by Subject and A-Z"; click on the icon.
- Type journal citations reports in the search box on the left side of the screen and then click on the magnifying glass to search that title.
- Your result will say "Journal Citation Reports"; click on it. It might ask you to provide your Net ID and password if you are off campus.
Find the Impact Factor
- Once in the database you either search by journal title (if you know which journal you want to see) or browse by category, which will let you view journals by JIF by discipline.
- On the left side you can choose search criteria, like impact factor range, year, and if the journal is open access.
- It is important to choose the right edition based on your subject area, as you won't be able to see specific journals if you choose the wrong one. Once you have finished selecting what to search, click Submit.
- You can't access impact factors from last year because the calculations only happen every two years (i.e. if the current year is 2021 the farthest you can go back is 2020). Most people choose the most current year they can access.
- Journals limited by the subject area, publisher, or geographic region.
- View all journals in order to browse.
- Search for a specific journal if you already know its title
Once you find a journal, the JCR gives you information about the journal, including the journal's abbreviations, how often it is published each year, the publisher, and the ISSN.
Controversy
Many people have questioned the legitimacy of impact factor. Here are a few reasons why:
- Impact factor focuses purely on the numbers. There is no consideration of qualitative elements that have become important in today's world.
- Impact factor fails to incorporate more recent ways of sharing and using research, including Twitter mentions and posts, citation management downloads, and news and community information.
- Because impact factor is based on citations in only indexed journals , it fails to incorporate statistics from journals that might not be indexed and other sources like conference papers (which are important in the social sciences).
- Basic or summary information is usually cited the most in academia. That means that journals that publish articles with basic information are more likely to have higher impact factors. Journals that publish obscure or innovative information might not have as high of an impact factor.
- Some argue that impact factor is encouraging scholars to stick with mainstream topics and research.
- Scholars don't always have to cite something for it to be influential. Sometimes researchers just read something and it influences them, regardless of if they cite it in a future paper or piece of research.
- The journals in the JCR are mostly published in English. This means that many international sources aren't included in the conversation.
- It has been argued that journals have the ability to skew impact factor for their own journal. Before publishing an author, they will ask the author to cite more articles within their journal so that their impact factor goes up. This is NOT a common occurrence but instead something we should be aware of.
- << Previous: Types of Metrics
- Next: Identifying Journals >>
- Last Updated: Feb 28, 2024 12:49 PM
- URL: https://guides.library.illinois.edu/impact
Journal and Article Metrics Guide
Download our PDF guide to understand more about journal and article metrics.
Understand journal and article metrics before you submit
When deciding where to submit your manuscript, consider a broad range of journal and article metrics to decide which journal will help you achieve your goals of getting the best reach, recognition and impact for your research.
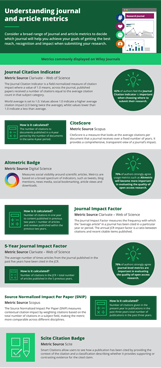
Journal Metrics
Journal metrics are useful for evaluating the collective impact of the journal’s total output, not the impact of an author’s individual contribution. Most of the indices that supply journal-level metrics, are selective and employ a rigorous screening process that includes an assessment of the journal’s potential citation impact alongside quality control measures, such as the journal’s publication ethics standards, research integrity practices, peer review model, and other criteria.
Article Metrics
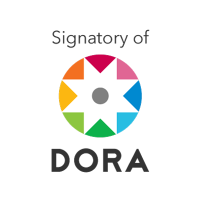
Metrics Most Commonly Displayed on Wiley Journals*
Metric Name | Metric Source | Metric Description |
---|---|---|
Journal Impact Factor |
| The Journal Impact Factor is defined as all citations to the journal in the current JCR year to items published in the previous two years, divided by the total number of scholarly items (these comprise articles, reviews, and proceedings papers) published in the journal in the previous two years. . |
5-Year Journal Impact Factor |
| The 5-year journal Impact Factor is the average number of times articles from the journal published in the past five years have been cited in the JCR year. It is calculated by dividing the number of citations in the JCR year by the total number of articles published in the five previous years. . |
Journal Citation Indicator |
| Designed to complement the Journal Impact Factor, the Journal Citation Indicator is field-normalized so it can be easily interpreted and compared across different disciplines. . |
Altmetric Badge | Digital Science | Altmetrics go beyond more traditional citation metrics to measure social visibility around scientific articles. These metrics are based on a broad spectrum of indicators, such as tweets, blog mentions, news media, social bookmarking, article views, and downloads. |
CiteScore |
| The CiteScore is calculated by dividing the number of citations to documents published in a 4-year period by the number of documents in same 4-year period. . |
SNIP |
| The Source Normalized Impact per Paper (SNIP) measures average citations in Year X to papers published in the previous 3 years. Citations are weighted by the citation potential of the journal’s subject category, thereby making the metric more comparable across different disciplines. |
Scite Citation Badge | scite | Smart Citations allow users to see how a publication has been cited by providing the context of the citation and a classification describing whether it provides supporting or contrasting evidence for the cited claim. . |
Submission to First Decision | Journal data | The time in days between timestamps, or dates if no timestamp is available, between the received date (the latest submission date recorded against the first version of the paper, i.e. where multiple revisions have been submitted, the original paper) and the date that an editor makes any type of decision, including desk rejections, rejection without receiving external peer review and withdrawals, on that first submitted version of a paper. This is calculated and displayed as a median. |
Submission to Acceptance | Journal data | The time in days between timestamps, or dates if no timestamp is available, between the received date (the latest submission date recorded against the paper) and the date that an editor makes a final decision of 'accept' on the latest submitted version of a paper (i.e. where multiple revisions have been submitted, the latest paper). This is calculated and displayed as a median. |
Acceptance Rates | Journal data | The percentage of papers accepted during a given period as a proportion of all papers arriving at a final decision in that period. This is calculated according to the final decision date rather than the year in which the paper was submitted. Note: the Acceptance Rate metric includes rejections with no review. Acceptance Rates can be impacted by the journal’s scope, peer review model (sound science vs. selective), commissioning strategy (invited reviews, special issues), eligible document types, study design requirements, and many other factors. Please visit the individual journal homepage for more information and context. In many cases, rejected articles are eligible for transfer to a journal that is in scope. |
Full Text Views | Journal data | The number of unique usage events taking place in the given year on Wiley Online Library, including all forms of full text access (HTML/PDF/Full Text Author Manuscript). Events relating to abstract views / access denied notifications are not included. All usage linked to crawlers is removed. |
*Not every journal will have these metrics available. Clarivate and Scopus metrics require a journal to be indexed which is a selective process. If a journal has not applied for indexing, or has not been accepted for indexing, then it may not display all the available metrics. Indices contain a variety of metrics used to measure journal performance and impact. Visit the Index website to learn more about the available metrics. For more information about Wiley’s Open Research initiatives and opportunities to showcase and gain recognition for your individual contributions, visit the Open Research page .
ScienceDirect Support Center
To post social content, you must have a display name. The page will refresh upon submission. Any pending input will be lost.
Where do I find the Impact Factor of a journal?
The Impact Factor is a measure of scientific influence of scholarly journals. It measures the average number of citations received in a particular year by papers published in the journal during the two preceding years and is produced by a publisher called Thomson Reuters. The Impact Factor can be found on the Journal home page of journals that have an Impact Factor.
Please note: Not all journals have an Impact Factor.
Follow these steps to find the Impact Factor of a journal:
- Search for a journal using the ‘Journal/book title’ field on the ScienceDirect homepage or browse journal titles by selecting ' Journals & Books ' in the top right corner.
- Click the journal title to navigate to the journal’s home page.
- The Impact Factor and Journal CiteScore are mentioned in the header on the right side of the page.
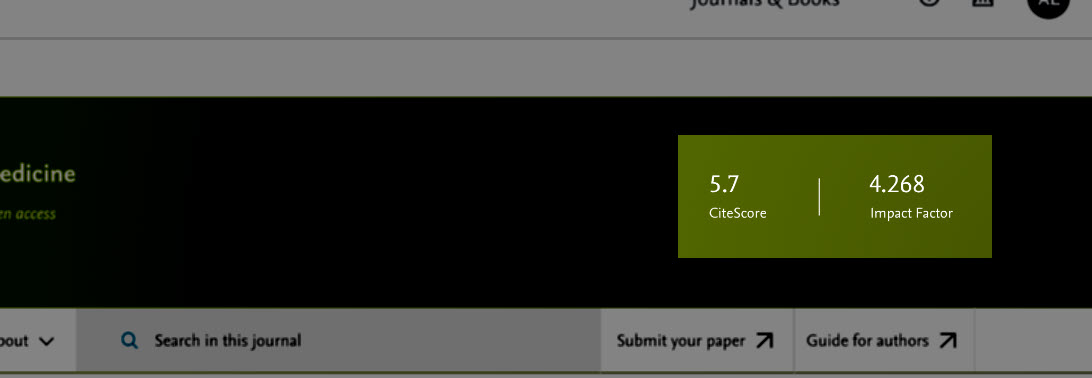
Was this answer helpful?
Thank you for your feedback, it will help us serve you better. If you require assistance, please scroll down and use one of the contact options to get in touch.
Help us to help you:
Thank you for your feedback!
- Why was this answer not helpful?
- It was hard to understand / follow.
- It did not answer my question.
- The solution did not work.
- There was a mistake in the answer.
- Feel free to leave any comments below: Please enter your feedback to submit this form
Related Articles:
- What are Article Metrics?
- Where can I find the DOI?
- What can I do on a journal home page?
- Discover content from other publishers on ScienceDirect
- What are the technical requirements for ScienceDirect?
For further assistance:

An official website of the United States government
The .gov means it’s official. Federal government websites often end in .gov or .mil. Before sharing sensitive information, make sure you’re on a federal government site.
The site is secure. The https:// ensures that you are connecting to the official website and that any information you provide is encrypted and transmitted securely.
- Publications
- Account settings
Preview improvements coming to the PMC website in October 2024. Learn More or Try it out now .
- Advanced Search
- Journal List
- J Gen Intern Med
- v.37(7); 2022 May

Measures of Impact for Journals, Articles, and Authors
Elizabeth m suelzer.
1 Medical College of Wisconsin, Milwaukee, WI USA
Jeffrey L. Jackson
2 Zablocki VA Medical Center, Milwaukee, WI USA
Journals and authors hope the work they do is important and influential. Over time, a number of measures have been developed to measure author and journal impact. These impact factor instruments are expanding and can be difficult to understand. The varying measures provide different perspectives and have varying strengths and weaknesses. A complete picture of impact for individual researchers and journals requires using multiple measures and does not fully capture all aspects of influence. There are only a few players in the scholarly publishing world that collect data on article citations: Clarivate Analytics, Elsevier, and Google Scholar (Table (Table1). 1 ). Measures of influence for authors and journals based on article citations use one of these sources and may vary slightly because of differing journal coverage.
Citation Databases
Organization | Product | Years | Platform | Details |
---|---|---|---|---|
Elsevier | Scopus | 1970–present | SCImago | Contains citation information from over 39,000 journals; continually adding older content; covers 240 academic disciplines; requires subscription |
Clarivate | Web of Science | 1900–present | Journal Citation Report | Contains citation information from over 21,100 journals; covers over 250 academic disciplines; requires subscription |
Google Scholar | Not provided | Google Scholar | Freely accessible product of Google; collects citation and reference information using web crawlers that roam through websites containing scholarly information. |
Individual Authors
Researchers make contributions to their fields in many ways: through education, advocacy, mentorship, collaboration, reviewing grants and articles, editorial activities, and leadership. For better or worse, their impact is usually based on the number of research articles they publish and how often those articles are cited. Some activities, such as writing editorials for leading journals, book chapters, or other clinical texts; testifying before Congress; or helping to shape government or health system policy, can be highly influential, but not credited in these measures of influence.
A common problem authors have in determining their impact is duplicate names, either from being inconsistent in the name they use (e.g., Jackson JL vs Jackson J) or name changes. There are several ways to establish a persistent and unique digital identifier. Researchers should take advantage of all.
ORCID ( www.orcid.org )
Many funders require an ORCID identifier as part of grant submission. ORCID is free, and all authors can sign up to create a unique identifier. ORCID does not track measures of impact, but cooperates with other sites that do by maintaining a list of publications that authors can review for completeness and accuracy.
ResearcherID ( www.researcherid.com )
This site provides a unique identifier and pulls information from Web of Science (Clarivate) to generate an h -index. It has a dashboard that generates a Web of Science author impact plot, provides authors a year-by-year report on impact, and generates a “citation” map that shows the location of citations. ResearcherID is also used by Publons, another Clarivate product, that tracks peer review and editorial activity. Access requires a subscription.
Scopus and Web of Science
Scopus and Web of Science are independent sites that create unique identifiers for authors based on proprietary software. Identifiers are automatically assigned and may result in the creation of more than one identifier, particularly if authors have had multiple affiliations, have a common name, have changed names, or have been inconsistent in their name. Authors can review the identifiers assigned and merge different listings. Access to these databases requires a subscription.
In addition, authors can create a Google Scholar account, which will also track and assess author impact. Google Scholar is free. Authors should regularly review their account to make sure their article list is accurate.
Measures of Impact for Authors
There are a number of different measures of individual author impact; each has strengths and weaknesses (Table (Table2). 2 ). All are limited in that they do not account for author effort and order. Most can be skewed by self-citation and favor those who have been publishing longer. 2
Author Measures of Influence
Measure | How calculated | Strengths | Weaknesses |
---|---|---|---|
-index | Number of articles ( ) that have been cited times | Easy to calculate Combines quality/quantity | Skewed by self-citation Does not account for author order or effort Biased against early-career authors |
-index | Sum citations of top articles and take the square root and round | Easy to calculate Combines quality/quantity | Skewed by self-citation Does not account for author order or effort Biased against early-career authors Highly influenced by high-impact articles |
i-10-index | Number of articles that have been cited at least 10 times | Easy to calculate Combines quality/quantity | Favors productivity over quality Does not account for author order Biased against early-career authors Ten citations are an arbitrary cut-point |
iCite | Field and time adjusted and benchmarked against median for NIH–funded publications | Provides a benchmark Not biased against early-career authors | Difficult to calculate Highly influenced by high-impact articles |
Altmetrics | Weighted measure based on 15 sources | Accumulates quickly Provides measure of societal/cultural interest Provides a different perspective on article/author influence than citations | May not predict importance Not predictive of citations Evolving measures Reflects “popular” topics Can be gamed by using “popular” terms in title Uncertain how to use measures |
PlumX analytics | Provides metrics in 5 categories: citations, usage, captures, mentions and social media. |
H-Index , developed by Jorge E. Hirsch in 2005, is defined as the number of published papers that have been cited at least h times. 3 An h -index of 40 means th.e author has 40 articles cited at least 40 times. This simple metric is widely used for evaluating an authors’ impact. Citation databases like Web of Science, Scopus (Elsevier), and Google Scholar provide h -index information in their author profiles, though the reported h -index may vary due to citation coverage. The h -index favors authors that publish a continuous stream of papers with persistent, above-average impact. It measures the cumulative impact of an author’s work and combines quantity and quality. However, it does not account for the author effort and order, is biased against early-career researchers with fewer publications, and can be skewed by self-citation.
G-Index , created in 2006 by Leo Egghe, is defined as the largest number such that the top “ g ” articles received together at least g 2 citations. 4 This metric favors highly cited articles; a single highly cited article will increase the g -index considerably, while only increasing the h -index by 1.
i-10-Index , calculated by Google Scholar, is a straightforward metric that shows the number of publications with at least 10 citations.
Measures of Impact for Individual Articles
This is an NIH dashboard of bibliometrics for articles. iCite has three modules: Influence, Translation, and Open Citations. Influence is based on a relative citation ratio (RCR), comparing article citations to the median for NIH–funded publications, the value of which is set at 1.0. Among NIH–funded studies, the 90 th percentile for RCR is 3.81. Among all studies, the 90 th percentile is 2.24. Individual paper influence is reported and can be used to select manuscripts that best represent one’s work. Translation provides a measure of translation from bench to bedside by breaking down whether most of the author’s publications are molecular/cellular, animal, or human. Citations provide a count of the total citations and give citation statistics (mean, median, SE, maximum) as well as a list of the citing articles for each paper.
Alternative measures of influence
There are measures of influence of individual articles that are not based on citations. They provide a snapshot of article impact in a number of alternate venues, such as public policy documents, news articles, blogs, and social media.
Altmetric tracks more than 15 different sources, including public policy documents, news articles, blog posts, mentions in syllabi, reference managers, and social networks, such as Twitter and Facebook. The results are weighted; some sources, such as news articles, get greater weight. For example, in 2020, the weights of the various sources were news stories: 8, blogs: 5, Q&A forums: 2.5, Twitter: 1, Google: 1, and Facebook: 0.25. Altmetrics can be displayed as a “badge,” a symbol with a number in the middle of a circle with the strands colored to reflect the elements that went into the score. Researchers can sign up to create an altmetric badge for their articles ( www.altmetric.com ). To create a badge, the article must have a DOI number. Altmetrics for any specific article reflects popular interest in the topic rather than scientific importance. At JGIM, article altmetrics do not correlate with citations. Altmetrics can accumulate quickly; many metrics, such as Twitter and Facebook mentions, tend to occur within days of publication, while citations can take years. Altmetrics can be applied to scholarly products other than research publications, such as curricula and software. However, altmetrics can be gamed; “popular” topics tend to get more play than others. It is still unclear how to use altmetrics; most rank and tenure committees do not include these measures in promotion deliberations.
PlumX Analytics
PlumX gathers metrics into 5 categories: citations, usage, captures, mentions, and social media. Citations include traditional citations as well as ones that may have societal impact, such as policy documents. Usage measures views, downloads, and measures of how often the article is read. Captures indicate that a reader is planning on coming back to the article; it can indicate future citations. Mentions refer to news articles, blog posts, and other public mentions of the paper. PlumX Social Media refers to tweets and Facebook likes and shares, among several sources. It provides a picture of how much public attention articles are getting. PlumX analytics suffer from the same issues as altmetrics and citations. PlumX analytics are embedded in several platforms, including Mendeley, Science Direct, and Scopus and on many open-access journal platforms.
Measures of Impact for Journals
Historically, there were many reasons why certain journals rose to the top: highly respected editors, a long publishing history, and a track record of influential work policy makers and clinicians cared about. In 1975, Thompson Reuters debuted SCI Journal Citation Reports , ranking journals based on article citations. 5 Subsequently, this has been the primary basis for journal prestige.
Journal evaluation metrics that use citation data favor some disciplines over others. Disciplines vary widely in the amount of research output, the number of citations that are normally included in papers, and the tendency of a discipline to cite recent articles. 6 For example, Acta Poetica focuses on literary criticism. Its impact factor would be a poor measure of the journal’s influence. In addition, one needs to consider where the evaluation tool is collecting their data. Databases like Web of Science and Scopus may have stronger coverage of some disciplines, impacting the citation metrics that are generated. 6
Some resources assign journals to subject categories, making it possible to compare journals within their discipline. A good analogy is points scored in sporting events. Seven points in American football is a poor offensive outing, while 7 points in European football is a juggernaut. Comparing journals within the same discipline provides better information about the journal’s relative importance.
Journal Citation Reports
Journal Impact Factor (JIF). This is published annually by Clarivate and uses citation data from Web of Science. It has been the “gold standard” for measuring journal impact since its creation. 7 Journal editors nervously await release of their impact factor every summer. The JIF is calculated by dividing the total number of citations in the previous 2 years by the number of “source” articles published the following year. JGIM had 2810 citations in 2020 for articles published in 2018 and 2019; 548 of these articles were categorized as source material. Dividing 2810/548 yields our 2020 impact factor of 5.128. Not everything journals publish is considered source material. Clarivate does not provide guidance to journals on how they decide what types of material to count. In general, letters and editorials are not included. JGIM falls in the Medicine, General & Internal and the Health Care Sciences & Services categories, ranking 27 th and 11 th , respectively, in each. Seeking high JIF has led some journals to reduce the number of articles they publish, increase the amount of non-source papers, and focus on work they believe will be highly cited. The JIF is also susceptible to journal self-citation.
Journal Citation Indicator (JCI) is a normalized metric that debuted in 2021; a score of 1.0 means that journal articles were cited on average the same as other journals in that category. 8 JGIM has a JCI of 1.48 (Table (Table3), 3 ), meaning we have a 48% more citation impact than other journals in our category. Based on the JCI, JGIM ranks 23 rd in Medicine, General & Internal and 15 th in Health Care Sciences & Services.
Journal Measures of Impact
Measure | JGIM score | How calculated | Strengths | Weaknesses |
---|---|---|---|---|
Impact factor | 5.13 | Number of citations in a given year to articles published in the previous 2 years, divided by the number of source articles | Easy to calculate Combines quality/quantity | Can be gamed by journals Not a measure of quality Not all citation types are counted Skewed by journal self-citation Clarivate is vague about criteria for articles to be counted as source articles Not all journals have an impact factor Favors journals that publish systematic reviews |
Citation indicator | 1.48 | Normalizes the impact factor compared to other journals in that category | Gives a context for a specific journal Combines quality/quantity | |
5-year impact factor | 6.07 | Average number of citations over 5 years, divided by the number of source articles | Easy to calculate Combines quality/quantity Provides a measure of how long article influence is sustained | |
Immediacy index | 1.86 | Number of citations occurring in the same year of publication | Easy to calculate Combines quality/quantity Provides information on how quickly research is incorporated | |
Eigenfactor | 0.029 | Number of journal article citations over 5 years, factoring in the impact factor of the citing journal | Freely available Takes into account quality of journal citing article Covers 5 years Excludes journal self-citations | Assigns journals to 1 category. Difficult to interpret. Similar to raw citation counts. 5 years may be too long Favors disciplines with high-impact journals |
Normalized eigenfactor score | 6.07 | Normalizes the eigenfactor score so that the mean is 1.0 | Normalized Same as eigenfactor | |
Influence score | 2.58 | Calculated by multiplying the eigenfactor by 0.01, dividing by the number of articles in the journal, normalized a mean of 1.0 | Provides measure of influence Normalized Same as eigenfactor | |
CiteScore | 4.9 | Calculated by dividing the number of citations to documents (articles, reviews, conference papers, book chapters, and data papers) over 4 years by the number of articles published by the journal during the index year | Longer time allows time for citations to occur Sources are transparent Updated monthly | |
Scimago journal rank | 1.75 | Citations of articles in 1 year to articles over 3 years, weighted by the prestige of the citing journals | Updated annually | Favors fields with high-impact journals Susceptible to self-citation |
Source normalized impact per paper | 1.47 | Measures actual citations relative to citations expected for the field | Normalized | Favors journals that publish more review articles Not as reliable for journals that publish fewer articles Sensitive to outliers |
Scimago -index | 180 | Number of cited articles at least times in past 5 years | Easy to calculate. Combines volume/quality | Includes self-citations Favors established researchers |
H-5 index | 65 | Number of cited articles at least times in past 5 years | Easy to calculate |
* Source articles: articles that are counted in the denominator
5-Year Impact Factor is the average number of times articles published in the previous 5 years were cited in the indexed year. It gives information on the sustained influence of journal publications. JGIM’s 2020 score was 6.070, meaning that articles published in 2014–2019 were cited an average of 6 times in 2020.
Immediacy Index is the number of citations that occur in the year of publication. Journals with high immediacy index scores are rapidly cited. JGIM has a score of 1.861. This measure has been criticized for penalizing articles published later in the year.
Eigenfactor Score , a metric created in 2007 by Carl Bergstrom and Jevin West of the University of Washington, is based on the number of times articles from a journal over the past 5 years have been cited in the indexed year and gives citations in highly cited journals more weight than lesser cited ones. Self-citations by the journal are excluded. JGIM’s 2020 eigenfactor score was 0.02895. This measure suffers from being difficult to understand.
The Normalized Eigenfactor Score provides a normalized metric of the Eigenfactor Score, setting a score of 1 as the average for all journals. Like the Eigenfactor Score, citations that come from highly cited journals carry more weight than citations from less cited journals and journal self-citations are excluded. JGIM’s score is 6.07, meaning that JGIM was sixfold more influential than the average journal in the Web of Science database.
Article Influence . This measure is calculated by dividing the Eigenfactor Score by the number of a journal’s articles over the first 5 years after publication. It is calculated by multiplying the Eigenfactor Score by 0.01 and dividing by the number of articles in the journal, then normalized as a fraction of all articles in all publications, such that the mean is 1.0. JGIM’s most recent influence score is 2.579. This indicates that JGIM is more than twice as influential as the average journal.
CiteScore is calculated by dividing the number of citations from documents (articles, reviews, conference papers, book chapters, and data papers) over the previous 4 years by the number of articles indexed in Scopus published by the journal during those years. JGIM’s CiteScore is 4.6. Cite scores are calculated on a monthly basis. Among 122 internal medicine journals, JGIM is ranked 40 th by the CiteScore.
SCImago Journal Rank (SJR) also uses Scopus data and weights citations according to the prestige of the citing journal, taking into account the thematic closeness of the citing and cited journals. 9 It is calculated based on citations in 1 year to articles published in the previous 3 years. JGIM’s SJR is 1.746, which puts us 13 th on the list of “internal medicine” journals.
SCImago H-Index calculates the number of journal articles ( h ) that have been cited at least h times. It is the same calculation used to evaluate authors; SCImago calculates the journal h -index using Scopus citation data. JGIM has an h -index of 180, meaning that 180 of our articles have been cited more than 180 times. The h -index measures the productivity and impact of journal publications.
Source Normalized Impact per Paper (SNIP) compares each journal’s citations per article with the citations expected in its field. It allows a comparison of the journal’s impact across fields, because it adjusts for the likelihood of journal articles in that field being cited. JGIM’s SNIP is 1.471 which ranks us as 23 rd among 112 internal medicine journals.
Google Scholar
H5-index. Google Scholar calculates an H5-index for journals, which is the number of articles in the last 5 years with at least h citations. Google Scholar classifies JGIM as a primary care health journal. JGIM has an H5-index of 65, making it the top-ranked journal in this category. Google Scholar does not make available the citation sources; consequently, it is difficult to tell how complete the data is.
Journal Altmetrics
Like individual articles, altmetrics can be generated for journals. They have the same advantages and disadvantages as individual article altmetrics. In 2020, JGIM had 2.5 million downloads, 61 k linkouts, and 33 k social media mentions. Journal editors may have a poor understanding of altmetrics and struggle to know what to do with the data. Altimetrics reflect popular interest. For example, in 2020, the COVID pandemic captured public interest; articles focused on aspects of the pandemic received considerable public attention. For JGIM, the top altimetric article examined the impact of masking on preventing the spread of COVID and had an altmetric score of 4829.
JGIM is interested in these measures to ensure that we (like our authors) are having an impact. However, we are not obsessed on these measures and will continue to put forward what feels most important and relevant for academic general internists.
Declarations
The authors had no conflicts of interest with this article.
Publisher’s Note
Springer Nature remains neutral with regard to jurisdictional claims in published maps and institutional affiliations.
University of Illinois Chicago
University library, search uic library collections.
Find items in UIC Library collections, including books, articles, databases and more.
Advanced Search
Search UIC Library Website
Find items on the UIC Library website, including research guides, help articles, events and website pages.
- Search Collections
- Search Website
Measuring Your Impact: Impact Factor, Citation Analysis, and other Metrics: Citation Analysis
- Measuring Your Impact
Citation Analysis
Find your h-index.
- Other Metrics/ Altmetrics
- Journal Impact Factor (IF)
- Selecting Publication Venues
About Citation Analysis
What is Citation Analysis?
The process whereby the impact or "quality" of an article is assessed by counting the number of times other authors mention it in their work.
Citation analysis invovles counting the number of times an article is cited by other works to measure the impact of a publicaton or author. The caviat however, there is no single citation analysis tools that collects all publications and their cited references. For a thorough analysis of the impact of an author or a publication, one needs to look in multiple databases to find all possible cited references. A number of resources are available at UIC that identify cited works including: Web of Science, Scopus, Google Scholar, and other databases with limited citation data.
Citation Analysis - Why use it?
To find out how much impact a particular article or author has had, by showing which other authors cited the work within their own papers. The H-Index is one specific method utilizing citation analysis to determine an individuals impact.
Web of Science
Web of Science provides citation counts for articles indexed within it. It i ndexes over 10,000 journals in the arts, humanities, sciences, and social sciences.
- Enter the name of the author in the top search box (e.g. Smith JT).
- Select Author from the drop-down menu on the right.
- To ensure accuracy for popular names, enter Univ Illinois in the middle search box, then select “Address” from the field drop down menu on the right. (You might have to add the second search box by clicking "add another field" before you enter the address)
- Click on Search
- a list of publications by that author name will appear. To the right of each citation, the number of times the article has been cited will appear. Click the number next to "times cited" to view the articles that have cited your article
Scopus provide citation counts for articles indexed within it (limited to article written in 1996 and after). It indexes o ver 15,000 journals from over 4,000 international publishers across the disciplines.
- Once in Scopus, click on the Author search tab.
- Enter the name of the author in the search box. If you are using initials for the first and/or middle name, be sure to enter periods after the initials (e.g. Smith J.T.).
- To ensure accuracy if it is a popular name, you may enter University of Illinois in the affiliation field.
- If more than one profile appears, click on your profile (or the profile of the person you are examining).
- Once you click on the author's profile, a list of the publications will appear and to the right of each ctation, the number of times the article has been cited will appear.
- Click the number to view the articles that have cited your article
Dimensions (UIC does not subscribe but parts are free to use)
- Indexes over 28000 journals
- Does not display h-index in Dimensions but can calculate or if faculty, look in MyActivities
- Includes Altmetrics score
- Google Scholar
Google Scholar provides citation counts for articles found within Google Scholar. Depending on the discipline and cited article, it may find more cited references than Web of Science or Scopus because overall, Google Scholar is indexing more journals and more publication types than other databases. Google Scholar is not specific about what is included in its tool but information is available on how Google obtains its content . Limiting searches to only publications by a specific author name is complicated in Google Scholar. Using Google Scholar Citations and creating your own profile will make it easy for you to create a list of publications included in Google Scholar. Using your Google Scholar Citations account, you can see the citation counts for your publications and have GS calculate your h-index. (You can also search Google Scholar by author name and the title of an article to retrieve citation information for a specific article.)
- Using your google (gmail) account, create a profile of all your articles captured in Google Scholar. Follow the prompt on the scrren to set up your profile. Once complete, this will show all the times the articles have been cited by other documents in Google Scholar and your h-index will be provided. Its your choice whether you make your profile public or private but if you make it public, you can link to it from your own webpages.
Try Harzing's Publish or Perish Tool in order to more selectively examine published works by a specific author.
Databases containing limited citation counts:
- PubMed Central
- Science Direct
- SciFinder Scholar
About the H-index
The h-index is an index to quantify an individual’s scientific research output ( J.E. Hirsch ) The h-index is an index that attempts to measure both the scientific productivity and the apparent scientific impact of a scientist. The index is based on the set of the researcher's most cited papers and the number of citations that they have received in other people's publications ( Wikipedia ) A scientist has index h if h of [his/her] Np papers have at least h citations each, and the other (Np − h) papers have at most h citations each.
Find your h-index at:
Below are instructions for obtaining your h-index from Web of Science, Scopus, and Google Scholar.
Web of Science provides citation counts for articles indexed within it. It indexes over 12,000 journals in the arts, humanities, sciences, and social sciences. To find an author's h-index in WOS:
- To ensure accuracy for popular names, add an additional search box and enter "Univ Illinois" and then select “Address” from the field drop down menu on the right.
- Click on Citation Report on the right hand corner of the results page. The H-index is on the right of the screen.
- If more than one profile appears, click on your profile (or the profile of the person you are examining). Under the Research section, you will see the h-index listed.
- If you have worked at more than one place, your name may appear twice with 2 separate h-index ratings. Select the check box next to each relevent profile, and click show documents.
Google Scholar
- Using your google (gmail) account, create a profile of all your articles captured in Google Scholar. Follow the prompt on the screen to set up your profile. Once complete, this will show all the times the articles have been cited by other documents in Google Scholar and your h-index will be provided. Its your choice whether you make your profile public or private but if you make it public, you can link to it from your own webpages.
- See Albert Einstein's
- Harzing’s Publish or Perish (POP)
- Publish or Perish Searches Google Scholar. After searching by your name, deselect from the list of articles retrieved those that you did not author. Your h-index will appear at the top of the tool. Note:This tool must be downloaded to use
- << Previous: Measuring Your Impact
- Next: Find Your H-Index >>
- Last Updated: Jun 14, 2024 1:10 PM
- URL: https://researchguides.uic.edu/if

- Harvard Library
- Research Guides
- HKS Library & Research Services
Measuring Your Scholarly Impact
Author & article impact, journal rankings & evaluation, visualization software, statements on responsible research metrics.
Article & Author Impact
- Web of Science (Harvard Login) A multidisciplinary database, with searchable author abstracts, covering the journal literature of the sciences, social sciences and arts. Create personal account to generate citation reports by author and create citation maps for articles.
- Google Scholar Citations Track citations to your publications. Determine which authors are citing to your publications. Graph your citations over time. Note: You must register for a Google account using an academic email account. Authors of scholarly articles should claim their Google Scholar page to verify that your publications listings are accurate and complete. You can also create an author profile by following the instructions on the Google Scholar Citation page
- Publish or Perish A free author and journal impact metrics software program developed by Anne Wil Harzing that retrieves and analyzes citations to articles and books. The software uses Google Scholar to obtain the raw citations. The tool can be used to locate most cited articles and books by searching in general citations field.
- ORCID Register for an ORCID number. An ORCID is a unique id number that distinguishes you from every other researcher. This id is essential if you have a common last and first name as it distinguishes you from other scholars. Read more about ORCID at the Library's ORCID page. You can also use Harvard ORCID Connect to allow Harvard to access your ORCID more easily. This means internal scholarly and administrative systems can pull in your public ORCID data, saving you time, maintaining the accuracy and consistency of your data, and creating meaningful connections between systems. The id can be integrated into the research workflow such as manuscript and grant submissions and it supports automated linkages between you and your professional activities.
Journal Metrics
Journal metrics are used to identify key journals in a research field. This identification may be most useful to authors who are considering which journals to submit manuscripts to for future publication.
The Impact Factor may be the most familiar metric in academics. Eugene Garfield of Thomson Scientific first introduced this idea in the 1950s. Impact Factor calculations are now available through Thomson’s Journal Citation Reports (JCR) and the Elsevier product, Scopus.
Despite their merits, journal metrics can be misused for evaluating individual authors. Altmetrics is an alternative for measuring scholarly impact. Altmetrics measures the use of social media tools such as bookmarks, links, blog postings, and tweets to gauge the importance of scholarly output by authors. Using altmetrics as a measure of scholarly impact is controversial as social media tweets and mentions can be gamed by authors.
Journal Evaluation Resources
- Journal Citation Reports (Harvard Login) Database for journal evaluation, using citation data drawn from over 8,400 scholarly and technical journals worldwide in the sciences and social sciences. Coverage is both multidisciplinary and international, and incorporates journals from over 3,000 publishers in 60 nations.
- Google Scholar Metrics Defaults to top 100 publications in English, ordered by their five-year h-index and h-median metrics. Use the search box to search for individual journal titles. Compare the publications that are of interest to you. Explore publications by subject area by going to the left column, selecting your language, and picking a general search category. You can refine your results further by clicking on the subcategory link under each general subject category. more... less... For additional information about google inclusion and exclusion policies for metrics, go to https://scholar.google.com/intl/en/scholar/metrics.html#overview
- SJR (Scimago Journal & Country Rank) A free ranking tool for journals. Data from Elsevier product, Scopus. You can limit results by country and geographic region.
- SciRev Provides information on journal response times and review duration based on feedback from individuals. Heavy focus on science journals but includes some social science and business/economics journals.
Conducting Literature Reviews
Scholars will often publish journal articles that evaluate the top ranked journals in their discipline. Conduct a search in a large interdisciplinary database such as Proquest Social Sciences Premium Collection or Ebsco's Academic Search Premier using keywords such as "top journals" or "highly ranked journals" and the field. For example, you could search in Academic Search Premier using the terms "top ranked journals" and "economics". You can also select a narrower subject specific database such as EconLit . Alternatively search across a number of different databases for full-text articles using the Google Scholar search option.
Defining Altmetrics
The term "altmetrics" (alternative metrics) is used to describe approaches to measure the impact of scholarship by using new social media tools such as bookmarks, links, blog postings, inclusion in citation management tools, mentions and tweets to measure the importance of scholarly output.
Proponents of altmetrics believe that using altmetrics will help measure the impact of an article in a more comperhensive and objective way than was done with more traditional scholarly impact measures such as journal impact factor. However, there are limits to this approach and caution should be used to not rely on any one particular measure in evaluting the importance of scholarship.
- Altmetrics, A Manifesto Web site devoted to altmetrics. Started by group of librarians and researchers who are active in promoting altmetrics as an alternative to more traditional forms of tracking article impact.
- Impact Story ImpactStory aggregates altmetrics measures from articles, datasets, blog posts, and more.
- Altmetric (Free Tools) Private company that sells access to altmetrics products. Individual researchers can download a free bookmarklet to check altmetrics on individual articles.
- Open Syllabus Project Creators of site scraped college Web sites and have put together the metadata for over 1 million syllabi. New metric based on this project, the "Teaching Score" (TS), is a numerical indicator of the frequency with which a particular work is taught.
- Leiden Madtrics Leiden Madtrics is the official blog of the Centre for Science and Technology Studies (CWTS) at Leiden University. The blog looks specifically at the processes of evaluating research, developing research policy, and the myriad ways academic research makes an impact in society
Free Software for Visualizing Citations
- CitNetExplorer A product of CWTS of Leiden University, this tool allows citation networks to be imported directly from the Web of Science database and used to visualize and analyze citation networks of scientific publications.
- Gephi An open-source software for visualizing and analysing large networks graphs. Gephi uses a 3D render engine to display graphs in real-time and speed up the exploration. You can use it to explore, analyse, spatialise, filter, cluterize, manipulate and export all types of graphs.
- Sci2 Network visualization tool that allows for easy data import from standard comma-separated lists and generates network analytics as well as visualizations.
- VOSviewer Created by Leiden University's CWTS, a software tool for constructing and visualizing bibliometric networks. Offers text mining functionality that can be used to construct and visualize networks of important terms extracted from a body of scientific literature.
- Local Citation Network Construct and visualizes citation networks to identify the most influential papers in a given topic or field.
- Citation Gecko Start from a small set of 'seed papers' that define an area you are interested. Gecko will search the citation network for connected papers allowing you to quickly identify important papers that you may have missed.You can connect to your Zotero libraries.
- Leiden Manifesto for Research Metrics
- San Francisco Declaration on Research Assessment
- Last Updated: Aug 1, 2023 2:18 PM
- URL: https://guides.library.harvard.edu/hks/scholarlyimpact
Harvard University Digital Accessibility Policy
What is a Good Impact Factor for a Journal?
- Research Process
A good journal impact factor (IF) is often the main consideration for researchers when they’re looking for a place to publish their work. Many researchers assume that a high impact factor indicates a more prestigious journal.
Updated on February 22, 2024

A good journal impact factor (IF) is often the main consideration for researchers when they’re looking for a place to publish their work. Many researchers assume that a high impact factor indicates a more prestigious journal. And that means more recognition for the manuscript author(s).
So, by that logic, the higher the impact factor, the better the journal, right?
Well, it’s not that simple.
In principle, a higher IF is better than a lower IF, but there are many conditions, variations, and other issues to consider.
There’s no single determinant of what makes a good journal impact factor. It depends on the field of research, and what you mean by “good.” What is “good” for a breakthrough immunology study may not apply as “good” for an incremental regional economics study.
Using impact factors in the academic world to rank journals remains controversial. The San Francisco Declaration on Research Assessment (DORA) , for example, tried to tackle the issue of over-reliance on journal IFs when evaluating published research.
Yet researchers continue to associate a good IF with better quality research. So, until DORA or others develop a better solution, we’re stuck with the IF, simplistic as it may be.
Read on to increase your understanding of impact factors and learn what’s a good one for your research.
First, what’s an impact factor?

A journal impact factor is a metric that assesses the citation rate of articles published in a particular journal over a specific time – that’s usually 2 years (see below).
For example, an IF of 3 means that published articles have been cited on average 3 times during the previous 2 years.
How impact factors are calculated
The IF for a particular year is calculated as the ratio of the total times the journal’s articles were cited in the previous 2 years to the total citable items it published in those 2 years.

For example, in 2018, Nature had an IF of 43.070. That's a good journal impact factor. This is calculated as follows:

(Adapted from https://clarivate.libguides.com/jcr )
(Source: 2018 Journal Citation Reports)
Clarivate Analytics annually computes IFs for journals indexed in Web of Science. These scores are then collectively published in the Journal Citation Reports (JCR) database.
Clarivate publishes two different JCR databases every year. The Science Citation Index (SCI) is for the STEM (science, technology, engineering, and mathematics) disciplines, and the Social Science Citation Index (SSCI) is for, you guessed it, the social sciences. These are the only acceptable and reputable sources for impact factors out there: If your journal is using another index, then beware – it could be predatory .
Types of impact factors and metrics
In addition to the 2-year impact factor, Clarivate offers metrics for short-, medium-, and long-term analysis of a JCR journal’s performance. These metrics include:
- Immediacy index – Average number of times an article is cited during the same year it’s published.
- Citing and cited half-life – Median age of citations produced and received by a journal, respectively, during the current JCR year.
- 5-year impact factor – Average number of times articles published in a journal during the past 5 years have been cited in the current JCR year.
- Eigenfactor Score (ES) – Similar to the 5-year impact factor; differences are that (ES) eliminates self-citations and considers the importance of citations received by a journal.
- Article influence score – Derived from the ES; measures the average influence of each article published in a journal.
Journal ranking within a specific subject category can also be indicated by quartiles. Many universities around the world prefer the use of these metrics rather than raw IF for selecting journals. Four quartiles rank journals from highest to lowest based on the impact factor: Q1, Q2, Q3, and Q4.
Q1 comprises the most (statistically) prestigious journals within the subject category; i.e., the top 25% of the journals on the list. Q2 journals fall in the 25%–50% group, Q3 journals in the 50%–75% group, and finally, Q4 in the 75%–100% group.
Numbers = status, and many authors, or their institutions, insist on publication in a Q1 or at least a Q2 journal.
An alternative ranking system, and one that is free to access, Scimago Journal & Country Rank , also uses quartiles. Be sure not to confuse the two.
OK, back to the main question: What’s a good impact factor?
As mentioned, separate JCR databases are published for STEM and social sciences.
Discrepancies in fields
The main reason is that there are wide discrepancies in impact factor scores across different research fields. Some of the likely causes for these discrepancies are:
- Differences in citation behavior in different research fields; e.g., review articles tend to attract more citations than research articles, and the tendency to cite books in the social sciences.
- Differences in types of research; e.g., interdisciplinary and basic research attract more citations than intradisciplinary and applied research.
- Differences in field coverage by JCR; e.g., more in-depth coverage of STEM fields compared with humanities and the social sciences.
To put things into perspective, data prepared by SCI Journal for the 2018/2019 journal impact factor rankings are shown in this table.

Source: scijournal.org
The table illustrates where a journal subject area ranks in the four classes: top 80%, top 60%, top 40%, and top 20%. Outliers were removed for the sake of cleaner data.
STEM impact factors
The data demonstrate how journals for subject areas show a range of impact factors.
Large fields, such as the life sciences, generally have higher IFs. They get cited more, so that makes sense. For example, it's natural that a study on a vaccine breakthrough will lead to more citations than a study on community development.
This obviously skews the use of impact factors when assessing researchers across a university. We can’t all keep up with the biologists!
For example, the top-ranked journal by Clarivate in 2019 was CA: A Cancer Journal for Clinicians, which had a remarkably high IF of 292.278. The New England Journal of Medicine , which has long been a prestigious journal, came in second with a high IF of 74.699. Those are amazingly good journal impact factors.
Conversely, reputable journals in smaller fields, such as mathematics, tend to have lower impact factors than the natural sciences.
For example, the 2019 JCR impact factors for respectable mathematical journals such as Inventiones Mathematicae and Duke Mathematical Journal were 2.986 and 2.194, respectively.
Social sciences impact factors
As for the social sciences, you could simply argue they’re “less popular” than the natural sciences. So, their reputable journals also tend to have lower impact factors.
For example, well-regarded journals such as the American Journal of Sociology and the British Journal of Sociology had JCR 2019 impact factors of 3.232 and 2.908, respectively. Those are good impact factors for the social sciences but would look rather low for STEM unless it was a regional or niche topic.
Therefore, what may be seen as an excellent impact factor in mathematics and the social sciences may be viewed as way below average in the life sciences.
That’s not to say the social sciences are less important. It just means they’re comparably researched and cited less.
Niche and specialized impact factors
The comparison doesn’t end there. Another aspect that shouldn’t be overlooked is the research subfield. Journals in, say, a physics subfield such as astronomy have a different impact factor than journals in fluid dynamics.
For example, two well-known astrophysics journals, MNRAS and Astrophysical Journal , had JCR 2019 IFs of 5.356 and 5.745, respectively. Meanwhile, two respectable journals in fluid dynamics, Journal of Fluid Mechanics and Physics of Fluids had JCR 2019 IFs of 3.354 and 3.514, respectively.
They may not be in The New England Journal of Medicine territory, but all are reputable journals.

So, all things considered, what is a good journal impact factor?
You have to look at the bigger picture here because there’s a lot more to consider than the single numeric representation.
If you’re in a field/subfield with high-impact-factor journals, it’s only logical that the cutoff for a good IF will also be high. And, of course, it’ll be lower for a field/subfield with lower impact factor journals.
Impact factor statistics should, thus, be interpreted relatively and with caution, because the scores represented are not absolute.
A good impact factor is either, in short, what your institution or you say it is. Otherwise, it’s one that's sufficient to connote prestige while still being a good forum for your research to be read and cited.
Let’s look at a few of the other factors, apart from IF, to help you choose your target journal.
Pros and cons of using impact factor to judge a journal’s quality
The impact factor was used initially to rank journals, which will then help you decide on which one to which to submit your research. Some of the pros of an IF include:
- Easily accessible
- Gives a general picture of a journal’s prestige and reputation
- Is pretty good for comparison within a field if not across fields
- Appeals to people and institutions that like rankings and numbers
Despite its popularity, the impact factor is clearly a flawed metric, and its use to judge if a journal is good is criticized. That’s what we’ve seen with DORA, among plenty of others.
In addition to the previously mentioned shortcoming of not being able to use the impact factor for comparing journals across fields, other cons include:
- Ambiguous description of what “citable items” are
- Lack of consideration of highly cited papers resulting in skewed citation distributions
- Encouragement of self-citations by journals
The criticism is nothing new (see Kurmis, 2003 , among others). But we’ve got to live with it until there’s something better.
On a personal note, we’re rather tired of seeing the great stress of publishing in a Q1 journal that’s placed on researchers. Especially those from certain economies that pressure their researchers to publish when they’d be better off fostering good, reflective, valuable research.
So here are some other factors with impact, even if they’re not impact factors.

Things other than impact factor to consider when choosing a journal
A good impact factor may be a requirement by your institution. But it shouldn’t be the only aspect you consider when choosing where to publish your manuscript.
Aims and scope
Another key factor is whether the work to be published fits within the aims and scope of the journal.
You can determine this by analyzing the journal’s subjects covered, types of articles published, and peer-review process. Some very targeted journals would welcome your research with open arms.
Target audience
An additional factor to consider is the target audience. Who is likely to read and cite the article? Where do these researchers publish? This can facilitate the shortlisting of some journals.
Other factors, apart from IF, for choosing your target journal
Other tips for choosing a journal include:
- Find journals that publish research that’s similar to yours, especially if it’s quite specialized
- Read as many published articles as you can in your target journal
- Go through your list of references to see which journals have the most citations
- Find out where fellow researchers and colleagues publish
Well-known publishers like Springer and Elsevier also list factors for choosing a journal.
Scimago as an alternative
Scimago Journal Rank (SJR) , as mentioned above, is a useful portal that scores and ranks journals, which are indexed in Elsevier’s Scopus database, based on citation data.
The SJR indicator (PDF) not only measures the citations received from a journal but also the importance or prestige of the journal where these citations come from. It can be used to view journal rankings by subject category and compare journals within the same field.
And here’s a great scholarly article with useful references that provide information on how to identify and avoid submitting to predatory journals.
Conclusion on what’s a good impact factor, especially for you and your research
What makes a good impact factor boils down to the field of research and the host of arbiters of “good.” Highly reputable journals may have low impact factors not because they lack credibility, but because they’re in specialized/niche fields with low citations.
The interpretation of what is a “good” journal impact factor varies. Possibly due to the ambitious nature of some researchers or the ignorance of others.
An IF can indeed serve as a starting point during decision-making, but, if possible, and if you don’t have to meet some arbitrary target, more emphasis should be paid to publishing high-quality research.
The prevailing mindset should be that a journal stands to benefit more from the good-quality research it publishes rather than the other way around.

The AJE Team
See our "Privacy Policy"
Find the right journal for your work and with your desired impact factor
AJE will compile a report of three Journal Recommendations specifically for your scientific manuscript. This way, you can get pursue the impact factor you want and/or pursue which journal is most suitable or is likely to publish your work faster.
Evaluating Information Sources
- Evaluate Your Sources
- Publication Types and Bias
- Reading Scholarly Articles
Journal Impact Factors
Links summary.
- Author Impact / Citations
- Author H-index
- Author h-index Options
- Author Citation Reports in Web of Science
- What are metrics?
- Cited Articles = Confusing Statistics
- Predatory Publishing
According to Journal Citation Reports (JCR) , an impact factor is a ratio focusing on original research.
Impact factor = # of citations to all items published in that journal in the past two years (divided by) # of articles and reviews published over those past two years referencing those citations
For example, if a journal has an impact factor of 2.5, this means in the indexed year each article published was cited on average 2.5 times in the previous two years in that journal.
Impact factor is used for journals only.
JCR only includes 12,000 journals and conference proceedings from over 3,300 publishers.
- InCitesTM: Journal Citation Reports® This Web of Science hosted database is a citation-based research evaluation tool for journal performance metrics with the goal of offering a systematic and objective means to evaluate the journals based on citation data.
- Tips for Using JCR Tips for using the Web of Science InCitesTM Journal Citation Reports
Metrics on the Web
- Eigenfactor® Project
- Google Scholar - Metrics
- InCitesTM: Journal Citation Reports®
- PlumX Metrics
Research Guides
- Impact Metrics and Scholarly Attribution (UCLA)
- Research Impact Metrics (UM)
- Spreading the Word: Publishing Your Research & Extending Your Impact (USC)
- << Previous: Reading Scholarly Articles
- Next: Predatory Publishing >>
- Last Updated: Mar 8, 2024 1:17 PM
- URL: https://libguides.usc.edu/evaluate
- All subject areas
- Agricultural and Biological Sciences
- Arts and Humanities
- Biochemistry, Genetics and Molecular Biology
- Business, Management and Accounting
- Chemical Engineering
- Computer Science
- Decision Sciences
- Earth and Planetary Sciences
- Economics, Econometrics and Finance
- Engineering
- Environmental Science
- Health Professions
- Immunology and Microbiology
- Materials Science
- Mathematics
- Multidisciplinary
- Neuroscience
- Pharmacology, Toxicology and Pharmaceutics
- Physics and Astronomy
- Social Sciences
- All subject categories
- Acoustics and Ultrasonics
- Advanced and Specialized Nursing
- Aerospace Engineering
- Agricultural and Biological Sciences (miscellaneous)
- Agronomy and Crop Science
- Algebra and Number Theory
- Analytical Chemistry
- Anesthesiology and Pain Medicine
- Animal Science and Zoology
- Anthropology
- Applied Mathematics
- Applied Microbiology and Biotechnology
- Applied Psychology
- Aquatic Science
- Archeology (arts and humanities)
- Architecture
- Artificial Intelligence
- Arts and Humanities (miscellaneous)
- Assessment and Diagnosis
- Astronomy and Astrophysics
- Atmospheric Science
- Atomic and Molecular Physics, and Optics
- Automotive Engineering
- Behavioral Neuroscience
- Biochemistry
- Biochemistry, Genetics and Molecular Biology (miscellaneous)
- Biochemistry (medical)
- Bioengineering
- Biological Psychiatry
- Biomaterials
- Biomedical Engineering
- Biotechnology
- Building and Construction
- Business and International Management
- Business, Management and Accounting (miscellaneous)
- Cancer Research
- Cardiology and Cardiovascular Medicine
- Care Planning
- Cell Biology
- Cellular and Molecular Neuroscience
- Ceramics and Composites
- Chemical Engineering (miscellaneous)
- Chemical Health and Safety
- Chemistry (miscellaneous)
- Chiropractics
- Civil and Structural Engineering
- Clinical Biochemistry
- Clinical Psychology
- Cognitive Neuroscience
- Colloid and Surface Chemistry
- Communication
- Community and Home Care
- Complementary and Alternative Medicine
- Complementary and Manual Therapy
- Computational Mathematics
- Computational Mechanics
- Computational Theory and Mathematics
- Computer Graphics and Computer-Aided Design
- Computer Networks and Communications
- Computer Science Applications
- Computer Science (miscellaneous)
- Computer Vision and Pattern Recognition
- Computers in Earth Sciences
- Condensed Matter Physics
- Conservation
- Control and Optimization
- Control and Systems Engineering
- Critical Care and Intensive Care Medicine
- Critical Care Nursing
- Cultural Studies
- Decision Sciences (miscellaneous)
- Dental Assisting
- Dental Hygiene
- Dentistry (miscellaneous)
- Dermatology
- Development
- Developmental and Educational Psychology
- Developmental Biology
- Developmental Neuroscience
- Discrete Mathematics and Combinatorics
- Drug Discovery
- Drug Guides
- Earth and Planetary Sciences (miscellaneous)
- Earth-Surface Processes
- Ecological Modeling
- Ecology, Evolution, Behavior and Systematics
- Economic Geology
- Economics and Econometrics
- Economics, Econometrics and Finance (miscellaneous)
- Electrical and Electronic Engineering
- Electrochemistry
- Electronic, Optical and Magnetic Materials
- Emergency Medical Services
- Emergency Medicine
- Emergency Nursing
- Endocrine and Autonomic Systems
- Endocrinology
- Endocrinology, Diabetes and Metabolism
- Energy Engineering and Power Technology
- Energy (miscellaneous)
- Engineering (miscellaneous)
- Environmental Chemistry
- Environmental Engineering
- Environmental Science (miscellaneous)
- Epidemiology
- Experimental and Cognitive Psychology
- Family Practice
- Filtration and Separation
- Fluid Flow and Transfer Processes
- Food Animals
- Food Science
- Fuel Technology
- Fundamentals and Skills
- Gastroenterology
- Gender Studies
- Genetics (clinical)
- Geochemistry and Petrology
- Geography, Planning and Development
- Geometry and Topology
- Geotechnical Engineering and Engineering Geology
- Geriatrics and Gerontology
- Gerontology
- Global and Planetary Change
- Hardware and Architecture
- Health Informatics
- Health Information Management
- Health Policy
- Health Professions (miscellaneous)
- Health (social science)
- Health, Toxicology and Mutagenesis
- History and Philosophy of Science
- Horticulture
- Human Factors and Ergonomics
- Human-Computer Interaction
- Immunology and Allergy
- Immunology and Microbiology (miscellaneous)
- Industrial and Manufacturing Engineering
- Industrial Relations
- Infectious Diseases
- Information Systems
- Information Systems and Management
- Inorganic Chemistry
- Insect Science
- Instrumentation
- Internal Medicine
- Issues, Ethics and Legal Aspects
- Leadership and Management
- Library and Information Sciences
- Life-span and Life-course Studies
- Linguistics and Language
- Literature and Literary Theory
- LPN and LVN
- Management Information Systems
- Management, Monitoring, Policy and Law
- Management of Technology and Innovation
- Management Science and Operations Research
- Materials Chemistry
- Materials Science (miscellaneous)
- Maternity and Midwifery
- Mathematical Physics
- Mathematics (miscellaneous)
- Mechanical Engineering
- Mechanics of Materials
- Media Technology
- Medical and Surgical Nursing
- Medical Assisting and Transcription
- Medical Laboratory Technology
- Medical Terminology
- Medicine (miscellaneous)
- Metals and Alloys
- Microbiology
- Microbiology (medical)
- Modeling and Simulation
- Molecular Biology
- Molecular Medicine
- Nanoscience and Nanotechnology
- Nature and Landscape Conservation
- Neurology (clinical)
- Neuropsychology and Physiological Psychology
- Neuroscience (miscellaneous)
- Nuclear and High Energy Physics
- Nuclear Energy and Engineering
- Numerical Analysis
- Nurse Assisting
- Nursing (miscellaneous)
- Nutrition and Dietetics
- Obstetrics and Gynecology
- Occupational Therapy
- Ocean Engineering
- Oceanography
- Oncology (nursing)
- Ophthalmology
- Oral Surgery
- Organic Chemistry
- Organizational Behavior and Human Resource Management
- Orthodontics
- Orthopedics and Sports Medicine
- Otorhinolaryngology
- Paleontology
- Parasitology
- Pathology and Forensic Medicine
- Pathophysiology
- Pediatrics, Perinatology and Child Health
- Periodontics
- Pharmaceutical Science
- Pharmacology
- Pharmacology (medical)
- Pharmacology (nursing)
- Pharmacology, Toxicology and Pharmaceutics (miscellaneous)
- Physical and Theoretical Chemistry
- Physical Therapy, Sports Therapy and Rehabilitation
- Physics and Astronomy (miscellaneous)
- Physiology (medical)
- Plant Science
- Political Science and International Relations
- Polymers and Plastics
- Process Chemistry and Technology
- Psychiatry and Mental Health
- Psychology (miscellaneous)
- Public Administration
- Public Health, Environmental and Occupational Health
- Pulmonary and Respiratory Medicine
- Radiological and Ultrasound Technology
- Radiology, Nuclear Medicine and Imaging
- Rehabilitation
- Religious Studies
- Renewable Energy, Sustainability and the Environment
- Reproductive Medicine
- Research and Theory
- Respiratory Care
- Review and Exam Preparation
- Reviews and References (medical)
- Rheumatology
- Safety Research
- Safety, Risk, Reliability and Quality
- Sensory Systems
- Signal Processing
- Small Animals
- Social Psychology
- Social Sciences (miscellaneous)
- Social Work
- Sociology and Political Science
- Soil Science
- Space and Planetary Science
- Spectroscopy
- Speech and Hearing
- Sports Science
- Statistical and Nonlinear Physics
- Statistics and Probability
- Statistics, Probability and Uncertainty
- Strategy and Management
- Stratigraphy
- Structural Biology
- Surfaces and Interfaces
- Surfaces, Coatings and Films
- Theoretical Computer Science
- Tourism, Leisure and Hospitality Management
- Transplantation
- Transportation
- Urban Studies
- Veterinary (miscellaneous)
- Visual Arts and Performing Arts
- Waste Management and Disposal
- Water Science and Technology
- All regions / countries
- Asiatic Region
- Eastern Europe
- Latin America
- Middle East
- Northern America
- Pacific Region
- Western Europe
- ARAB COUNTRIES
- IBEROAMERICA
- NORDIC COUNTRIES
- Afghanistan
- Bosnia and Herzegovina
- Brunei Darussalam
- Czech Republic
- Dominican Republic
- Netherlands
- New Caledonia
- New Zealand
- Papua New Guinea
- Philippines
- Puerto Rico
- Russian Federation
- Saudi Arabia
- South Africa
- South Korea
- Switzerland
- Syrian Arab Republic
- Trinidad and Tobago
- United Arab Emirates
- United Kingdom
- United States
- Vatican City State
- Book Series
- Conferences and Proceedings
- Trade Journals

- Citable Docs. (3years)
- Total Cites (3years)

Title | Type | --> | |||||||||||
---|---|---|---|---|---|---|---|---|---|---|---|---|---|
1 | journal | 106.094 Q1 | 211 | 49 | 124 | 4844 | 35427 | 89 | 381.89 | 98.86 | 43.95 | ||
2 | journal | 37.044 Q1 | 39 | 3 | 13 | 897 | 955 | 13 | 100.11 | 299.00 | 27.78 | ||
3 | journal | 35.910 Q1 | 508 | 123 | 336 | 11462 | 13599 | 153 | 34.50 | 93.19 | 29.41 | ||
4 | journal | 30.448 Q1 | 306 | 47 | 136 | 3645 | 2240 | 136 | 11.14 | 77.55 | 26.67 | ||
5 | journal | 26.837 Q1 | 505 | 105 | 304 | 10805 | 10951 | 163 | 31.23 | 102.90 | 44.33 | ||
6 | journal | 24.342 Q1 | 892 | 439 | 1496 | 32820 | 53447 | 1207 | 31.30 | 74.76 | 40.19 | ||
7 | journal | 22.399 Q1 | 391 | 239 | 731 | 8584 | 13091 | 153 | 19.72 | 35.92 | 34.15 | ||
8 | journal | 22.344 Q1 | 359 | 95 | 353 | 6242 | 4811 | 351 | 10.33 | 65.71 | 23.89 | ||
9 | journal | 21.836 Q1 | 184 | 117 | 335 | 8842 | 13775 | 196 | 31.17 | 75.57 | 26.86 | ||
10 | journal | 21.048 Q1 | 217 | 127 | 400 | 9888 | 10807 | 183 | 28.36 | 77.86 | 38.85 | ||
11 | journal | 20.544 Q1 | 1184 | 1388 | 4522 | 14603 | 107246 | 1824 | 21.69 | 10.52 | 38.26 | ||
12 | journal | 19.139 Q1 | 352 | 83 | 227 | 5043 | 1938 | 221 | 7.00 | 60.76 | 16.91 | ||
13 | journal | 19.045 Q1 | 630 | 595 | 1363 | 16478 | 36243 | 729 | 27.23 | 27.69 | 43.99 | ||
14 | journal | 18.663 Q1 | 71 | 0 | 19 | 0 | 963 | 19 | 0.00 | 0.00 | 0.00 | ||
15 | journal | 18.587 Q1 | 23 | 11 | 16 | 0 | 802 | 16 | 47.57 | 0.00 | 81.69 | ||
16 | journal | 18.530 Q1 | 215 | 83 | 261 | 4493 | 2531 | 258 | 7.04 | 54.13 | 17.80 | ||
17 | journal | 18.509 Q1 | 1391 | 3770 | 8037 | 74917 | 160102 | 3840 | 19.40 | 19.87 | 38.12 | ||
18 | journal | 18.117 Q1 | 511 | 485 | 1066 | 13393 | 17008 | 461 | 13.24 | 27.61 | 35.19 | ||
19 | journal | 17.828 Q1 | 833 | 271 | 851 | 115878 | 50519 | 819 | 49.76 | 427.59 | 30.50 | ||
20 | journal | 17.701 Q1 | 223 | 75 | 273 | 3371 | 1946 | 268 | 6.24 | 44.95 | 13.84 | ||
21 | journal | 17.654 Q1 | 234 | 108 | 410 | 6448 | 4495 | 409 | 8.04 | 59.70 | 16.43 | ||
22 | journal | 17.507 Q1 | 398 | 178 | 590 | 11546 | 12604 | 360 | 19.83 | 64.87 | 41.91 | ||
23 | journal | 17.497 Q1 | 229 | 229 | 609 | 6629 | 16808 | 379 | 26.18 | 28.95 | 29.53 | ||
24 | journal | 17.300 Q1 | 639 | 336 | 654 | 13672 | 13100 | 504 | 19.88 | 40.69 | 37.01 | ||
25 | journal | 16.061 Q1 | 388 | 36 | 103 | 14097 | 4303 | 99 | 42.66 | 391.58 | 14.94 | ||
26 | journal | 16.009 Q1 | 467 | 169 | 540 | 11148 | 13815 | 304 | 23.17 | 65.96 | 36.44 | ||
27 | journal | 15.966 Q1 | 264 | 102 | 252 | 19168 | 11266 | 244 | 38.64 | 187.92 | 24.30 | ||
28 | journal | 15.827 Q1 | 140 | 106 | 297 | 4359 | 4041 | 62 | 12.99 | 41.12 | 41.35 | ||
29 | journal | 15.620 Q1 | 328 | 23 | 84 | 4178 | 2696 | 83 | 27.02 | 181.65 | 40.68 | ||
30 | journal | 14.943 Q1 | 115 | 16 | 42 | 403 | 896 | 41 | 24.10 | 25.19 | 77.78 | ||
31 | journal | 14.796 Q1 | 388 | 400 | 978 | 11477 | 15900 | 588 | 17.52 | 28.69 | 33.83 | ||
32 | journal | 14.780 Q1 | 123 | 0 | 13 | 0 | 374 | 11 | 12.56 | 0.00 | 0.00 | ||
33 | journal | 14.707 Q1 | 32 | 46 | 35 | 4815 | 2160 | 34 | 61.71 | 104.67 | 36.44 | ||
34 | journal | 14.618 Q1 | 160 | 70 | 247 | 587 | 5353 | 230 | 21.11 | 8.39 | 58.79 | ||
35 | journal | 14.605 Q1 | 109 | 23 | 72 | 5797 | 1938 | 70 | 14.90 | 252.04 | 45.57 | ||
36 | journal | 14.577 Q1 | 419 | 262 | 637 | 10044 | 17562 | 466 | 27.42 | 38.34 | 28.93 | ||
37 | journal | 14.293 Q1 | 421 | 123 | 346 | 10202 | 6211 | 207 | 17.40 | 82.94 | 32.86 | ||
38 | journal | 14.231 Q1 | 558 | 306 | 834 | 9499 | 20730 | 593 | 24.08 | 31.04 | 24.85 | ||
39 | journal | 14.175 Q1 | 210 | 28 | 92 | 3163 | 1260 | 86 | 10.59 | 112.96 | 42.59 | ||
40 | journal | 13.942 Q1 | 294 | 144 | 670 | 5180 | 12698 | 362 | 18.81 | 35.97 | 39.02 | ||
41 | book series | 13.670 Q1 | 210 | 14 | 42 | 3772 | 1271 | 39 | 23.96 | 269.43 | 26.09 | ||
42 | journal | 13.655 Q1 | 311 | 89 | 563 | 4857 | 6315 | 559 | 11.14 | 54.57 | 23.11 | ||
43 | journal | 13.609 Q1 | 165 | 93 | 250 | 5332 | 1699 | 250 | 6.02 | 57.33 | 15.88 | ||
44 | journal | 13.578 Q1 | 455 | 233 | 688 | 15608 | 13409 | 550 | 16.89 | 66.99 | 40.35 | ||
45 | journal | 13.315 Q1 | 136 | 180 | 471 | 6682 | 12109 | 368 | 24.27 | 37.12 | 26.28 | ||
46 | journal | 13.080 Q1 | 260 | 243 | 827 | 1865 | 14374 | 679 | 16.58 | 7.67 | 62.53 | ||
47 | journal | 12.511 Q1 | 635 | 252 | 983 | 61439 | 44032 | 979 | 38.71 | 243.81 | 32.40 | ||
48 | journal | 12.324 Q1 | 81 | 55 | 137 | 2838 | 862 | 137 | 6.20 | 51.60 | 17.36 | ||
49 | journal | 12.294 Q1 | 46 | 62 | 154 | 8174 | 4174 | 86 | 27.10 | 131.84 | 31.14 | ||
50 | journal | 12.288 Q1 | 44 | 60 | 79 | 4858 | 3323 | 78 | 42.06 | 80.97 | 33.06 |
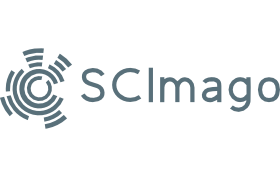
Follow us on @ScimagoJR Scimago Lab , Copyright 2007-2024. Data Source: Scopus®

Cookie settings
Cookie Policy
Legal Notice
Privacy Policy

SAE Earns New Impact Factors from Clarivate Analytics’ Institute of Scientific Information
Posted: August 27, 2024
In June 2024, the Institute of Scientific Information (ISI) from Clarivate Analytics released a list of 2023 impact factors.
SAE International has earned Journal Impact Factors (JIFs) for nine journals.
Understanding Impact Factors
Clarivate determines journal impact factors using a ratio that measures how often an article is cited in a year against the total number of citable content piece published in the previous two-year period.
The ISI uses a quality-driven process to determine which journals receive impact factors. Subject matter experts evaluate content and measure the citations of each journal found within the Web of Science platform. Authors and librarians around the world look to ISI impact factors when deciding which journals to purchase and where to publish them.
Due to a crowded market of publications, ranking on the scale is difficult to achieve, and any journal that earns an impact factor is seen as a valuable contributor to the field.
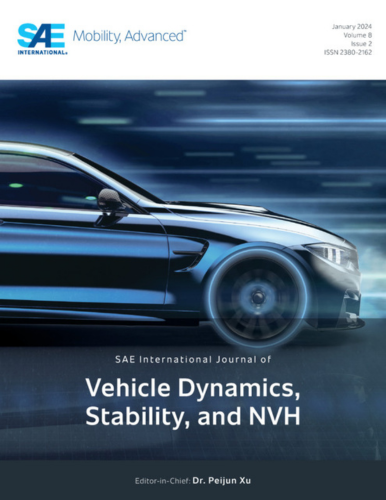
- SAE International Journal of Vehicle Dynamics, Stability, and NVH: 2.8
- SAE International Journal of Engines: 1.1
- SAE International Journal of Fuels and Lubricants: 1.0
- SAE International Journal of Electrified Vehicles: 0.7
- SAE International Journal of Transportation Safety: 0.7
- SAE International Journal of Materials and Manufacturing: 0.6
- SAE International Journal of Commercial Vehicles: 0.6
- SAE International Journal of Passenger Vehicle Systems: 0.5
- SAE International Journal of Aerospace: 0.3
- Passenger Cars: Mechanical Systems (formerly Passenger Vehicle Systems): 1.3
Why It Matters
With fraudulent behavior on the rise, JIFs serve as indicators of reliability and trustworthiness. These numerical scores validate SAE's dedication to its journals, authors, and editorial boards by gauging the rate at which journals are cited in scholarly content.
Clarivate Analytics has identified over 21,000 academic journals from 250 scientific research fields. Its annual Journal Citation Report examines thousands of publications for JIF consideration and marks Clarivate as one of the most reliable sources for identifying cutting-edge data.
The SAE Approach
The SAE International Scholarly Journals Program ensures quality through a rigorous peer-review process.
Kim Martin, SAE’s Editorial Director, Journals, Technical Papers, and SAE MobilityRxiv®, recognizes the need for a reliable, credible outlet to publish research that’s beneficial to both the author and the reader.
“One of the big things for publishing with SAE is that we have a name in this business, and there is authority behind the SAE brand. We're working with many different universities and organizations across industry. Publishing with us can provide authors that boost in credibility,” she previously shared in an SAE Blog.
Keep up to date with all of SAE’s Scholarly Journals . For publishing opportunities, visit us online.
Thank you for visiting nature.com. You are using a browser version with limited support for CSS. To obtain the best experience, we recommend you use a more up to date browser (or turn off compatibility mode in Internet Explorer). In the meantime, to ensure continued support, we are displaying the site without styles and JavaScript.
- View all journals
- Explore content
- About the journal
- Publish with us
- Sign up for alerts
- Open access
- Published: 22 August 2024
Child maltreatment as a transdiagnostic risk factor for the externalizing dimension: a Mendelian randomization study
- Julian Konzok ORCID: orcid.org/0000-0002-4232-4105 1 ,
- Mathias Gorski ORCID: orcid.org/0000-0002-9103-5860 2 ,
- Thomas W. Winkler ORCID: orcid.org/0000-0003-0292-5421 2 ,
- Sebastian E. Baumeister 3 ,
- Varun Warrier ORCID: orcid.org/0000-0003-4532-8571 4 ,
- Michael F. Leitzmann 1 &
- Hansjörg Baurecht 1
Molecular Psychiatry ( 2024 ) Cite this article
222 Accesses
3 Altmetric
Metrics details
- Psychiatric disorders
Observational studies suggest that child maltreatment increases the risk of externalizing spectrum disorders such as attention deficit hyperactivity disorder (ADHD), conduct disorder (CD), antisocial personality disorder (ASPD), and substance use disorder (SUD). Yet, only few of such associations have been investigated by approaches that provide strong evidence for causation, such as Mendelian Randomization (MR). Establishing causal inference is essential given the growing recognition of gene-environment correlations, which can confound observational research in the context of childhood maltreatment. Evaluating causality between child maltreatment and the externalizing phenotypes, we used genome-wide association study (GWAS) summary data for child maltreatment (143,473 participants), ADHD (20,183 cases; 35,191 controls), CD (451 cases; 256,859 controls), ASPD (381 cases; 252,877 controls), alcohol use disorder (AUD; 13,422 cases; 244,533 controls), opioid use disorder (OUD; 775 cases; 255,921 controls), and cannabinoid use disorder (CUD; 14,080 cases; 343,726 controls). We also generated a latent variable ‘common externalizing factor’ (EXT) using genomic structural equation modeling. Genetically predicted childhood maltreatment was consistently associated with ADHD (odds ratio [ OR ], 10.09; 95%-CI, 4.76–21.40; P = 1.63 × 10 −09 ), AUD ( OR , 3.72; 95%-CI, 1.85–7.52; P = 2.42 × 10 −04 ), and the EXT ( OR , 2.64; 95%-CI, 1.52–4.60; P = 5.80 × 10 −04 ) across the different analyses and pleiotropy-robust methods. A subsequent GWAS on childhood maltreatment and the externalizing dimension from Externalizing Consortium (EXT-CON) confirmed these results. Two of the top five genes with the strongest associations in EXT GWAS, CADM2 and SEMA6D, are also ranked among the top 10 in the EXT-CON. The present results confirm the existence of a common externalizing factor and an increasing vulnerability caused by child maltreatment, with crucial implications for prevention. However, the partly diverging results also indicate that specific influences impact individual phenotypes separately.
Similar content being viewed by others
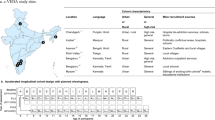
The Consortium on Vulnerability to Externalizing Disorders and Addictions (c-VEDA): an accelerated longitudinal cohort of children and adolescents in India
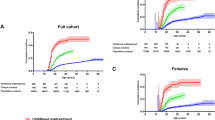
Re-examining the link between childhood maltreatment and substance use disorder: a prospective, genetically informative study
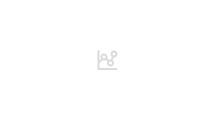
Gene – maltreatment interplay in adult ADHD symptoms: main role of a gene–environment correlation effect in a Brazilian population longitudinal study
Introduction.
Several lines of research indicate high comorbidity among externalizing psychopathologies and significant heritability of a common externalizing factor [ 1 , 2 ]. This common externalizing factor encompasses disinhibition, impulsivity, antisocial-aggressive behavior as well as substance (ab)use [ 1 ]. Clinically, the externalizing spectrum comprises attention-deficit/hyperactivity disorder (ADHD), conduct disorder (CD), antisocial personality disorder (ASPD), and substance use disorders (SUD) [ 3 ]. Multiple studies have demonstrated a shared genetic basis for these disorders [ 4 , 5 ].
One of the main candidates influencing the etiology of psychiatric disorders is childhood maltreatment. Childhood maltreatment encompasses emotional, sexual, physical abuse, and emotional and physical neglect [ 6 ]. A wide range of observational studies, including case-control designs, showed that childhood maltreatment increases risks for ADHD [ 7 ], ASPD [ 8 ] and SUD [ 9 ]. Twin and family studies demonstrated that childhood maltreatment has a heritability of 6 to 62% [ 10 , 11 ], depending on the subtype. These findings appear surprising, given that childhood maltreatment is an environmental, and thus potentially modifiable, determinant. However, investigations have shown that such heritability is derived from gene-environment correlations (rGE) with passive (i.e., family environment influenced by shared genetic factors of parents and infants), evocative/ reactive (i.e., parental style partly caused by hereditary characteristics of the offspring), and active (i.e., selection of specific contexts influenced by heritable traits) subtypes [ 12 ] (see Fig. 1 ). This raises the question of whether the relationship between childhood maltreatment and externalizing disorders is causal or is mainly driven by rGE. In the second case, risk for psychiatric diseases will only slightly be modified by childhood maltreatment, with crucial implications for prevention and treatment. Besides experimental and quasi-experimental designs controlling for genetic confounding, Mendelian Randomization (MR) [ 13 ] can be used to assess causal effects of environmental risk factors on mental health outcomes under certain key assumptions, even in the presence of rGE [ 14 ]. However, in the presence of rGE, particular attention should be paid to methods (e.g., Causal analysis using summary effect estimates, CAUSE) [ 15 ] reducing bias (e.g., correlated pleiotropy) arising from genetic correlation [ 14 , 16 ]. Correlated pleiotropy occurs when genetic variants influence both exposure and outcome through a heritable shared factor (see Fig. 1 ), which can bias MR analysis.
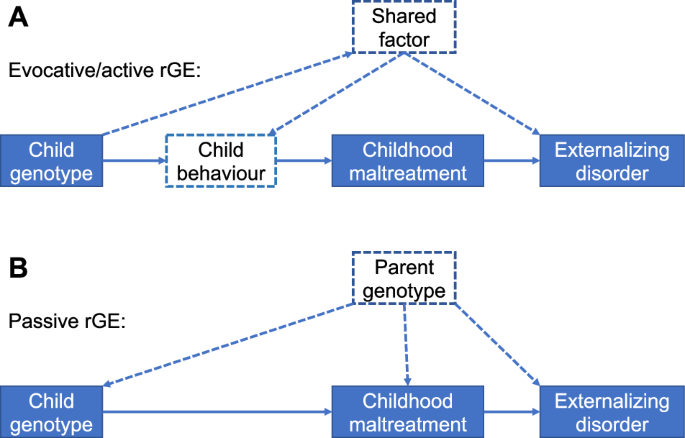
In evocative/active rGE ( A ), connection between child genotypes and exposure is conditioned on child behavior potentially resulting in a pathway to the outcome independent of the exposure. In passive rGE ( B ), causal estimation could be confounded by parent genotype. Dashed arrows symbolize potential confounding or pleiotropic pathways, solid arrows represent causal pathways.
To the best of our knowledge, only one two-sample MR study investigated the effect of childhood maltreatment on ADHD indicating an increasing risk [ 12 ]. Evidence from MR studies for other externalizing disorders (e.g., ASPD, SUD) is sparse. Furthermore, observational studies suggest an effect of externalizing problems on the risk of childhood maltreatment [ 7 ], which so far has only been investigated in ADHD patients using an MR approach. Additionally, no investigation has examined the externalizing factor reflecting common variation across externalizing disorders and a shared genetic basis. Evidence for childhood maltreatment as a transdiagnostic risk factor would have crucial implications for prevention strategies and programs (e.g., target individuals).
The current study aimed at investigating the causal relationship between childhood maltreatment and the risk of externalizing disorders accounting for rGE. In addition, we were interested in examining whether such a relationship also exists for a common externalizing factor reflecting comorbidity and continuity of externalizing disorders over the lifespan.
Materials and methods
Single-nucleotide polymorphisms (SNPs) were used as instrumental variables (IVs) to estimate the effect of the exposure on the outcomes unbiased from any unobserved confounding under the condition of valid IVs. IVs are valid if the following three assumptions are fulfilled. (i) The genetic variant is associated with the exposure (relevance assumption), (ii) the variant-outcome association is independent of a potential confounder (exchangeability assumption) and (iii) the genetic variant influences the outcome exclusively through the exposure, independent of any horizontal pleiotropy i.e., independent of any confounder or direct effect (exclusion restriction). Furthermore, several complementary analyses were conducted to evaluate potential biasing effects of correlated and uncorrelated horizontal pleiotropy as well as reverse causation [ 13 , 17 ].
Selection of instrumental variables for childhood maltreatment
Linkage-disequilibrium-(LD)-independent SNPs associated with childhood maltreatment were selected from a GWAS in 143,473 participants [ 12 ] (exclusively from UK Biobank to avoid overlap with the outcome GWAS) (Supplementary Table S1 ) at a genome-wide level of significance ( P value < 5 × 10 −8 ). Within this clumping algorithm, we excluded SNPs that exhibited strand ambiguity and had a minor allele frequency of less than 0.01. We applied a threshold of r 2 at 0.001 and employed a window size of 10 Mb. Then, we calculated the F -statistic and the proportion of the variance explained by childhood maltreatment by summarizing values from all SNPs. In the UK Biobank, participants completed the five-item Childhood Trauma Screener [ 18 ], which is a retrospective assessment. This screener includes one question for each of the five trauma subtypes (emotional, sexual, and physical abuse, and emotional and physical neglect), with responses ranging from 0 (never true) to 4 (very often true), resulting in total scores ranging from 0 to 20. The continuously coded total score was included in the GWAS for childhood maltreatment. In the original study, the authors identified 16 significant SNPs associated with 9 genomic risk loci [ 12 ] for the UK Biobank only data set.
Genome-wide association study summary statistics for the six externalizing disorders
To maintain consistency in the used phenotype definitions, we focused on GWAS for externalizing disorders clinically diagnosed by ICD or DSM, resulting in partly diminished numbers of cases due to limited data availability. For ADHD, we used data from a meta-analysis of samples of the Psychiatric Genomics Consortium (PGC) and the iPSYCH project totaling 20,183 cases and 35,191 controls [ 19 ]. For CD and ASPD, GWAS summary statistics stemmed from the FinnGen Consortium with 451 cases and 256,859 controls and 381 cases and 252,877 controls, respectively [ 20 ]. The GWAS on AUD and OUD were also conducted by the FinnGen Consortium with 13,422 cases and 244,533 controls and 775 cases and 255,921 controls, respectively [ 20 ]. Summary data statistics for cannabinoid use disorder (CUD) were derived from the PGC Substance Use Disorders working group, iPSYCH, and deCODE, with 14,080 cases and 343,726 controls [ 21 ] (Supplementary Table S1 , S2 ). Manhattan and quantil-quantil (Q-Q) plots of the used GWAS summary data are depicted in Supplementary Fig. S3 , S4 .
Statistical analyses
Shared genetic basis of the externalizing phenotypes: the externalizing factor (ext).
Using GenomicSEM, a common factor model and a commonfactorGWAS function were performed with a diagonally weighted least squares (DWLS) estimation, integrating the GWAS of the six externalizing phenotypes to a common factor GWAS. We assessed the model fit using the comparative fit index (CFI), standardized root mean square residual (SRMR), and the standardized loading of the common externalizing factor on the specific phenotypes. CFI scores of ≥0.90 indicate adequate fit, while values of ≥0.95 imply a good model fit [ 22 ]. SRMR values below 0.10 suggest an adequate model fit, values less than 0.05 point to a good fit [ 23 ]. SNPs with a significant heterogeneity test ( P < 0.05) were excluded from the common factor GWAS, and the effective sample size estimation was conducted with a minor allele frequency between 0.4 and 0.1. In the following MR analyses, this more holistic phenotype was termed ‘externalizing factor’ (EXT). To determine the individual importance of each externalizing disorder in shaping the overall EXT, we stepwise excluded each externalizing disorder and correlated these models in a leave-one-out-analysis. Additionally, heterogeneity analysis was applied using Q SNP statistic to test whether each SNP-externalizing-disorder association is conditioned on the common EXT. A significant Q SNP heterogeneity statistic indicated a pathway from the genetic variant to the externalizing disorder, independent of the common EXT.
To identify independent significant SNPs and corresponding genomic risk loci associated with the EXT, we used Functional Mapping and Annotation (FUMA) [ 24 ]. Within Multi-marker Analysis of GenoMic Annotation (MAGMA) gene-based association analysis, genome-wide significant SNPs were initially mapped to 19,176 protein-coding genes, and the SNPs within each gene were collectively tested for their association with EXT. Significance threshold for this analysis was Bonferroni corrected and defined at 2.61 × 10 −6 .
Primary analysis
Power analyses were performed following Brion et al. [ 25 ] (for detailed information see Supplementary Material 1 ). In MR analysis, Wald ratios (i.e. ratio of coefficients method) were calculated by dividing the logistic regression coefficient of the SNP-outcome associations by the regression coefficient of the SNP-exposure associations for each genetic variant selected from exposure GWAS. The delta method was used for standard error calculation. The ratio estimates (presumed to be linear on the log odds ratio scale) were subsequently combined using a multiplicative random effects model in an inverse variance weighted estimate (IVW) over all SNPs. The odds ratio is obtained by using the ratio estimates as exponent to the basis e [ 13 , 17 ]. We used a false-discovery rate (FDR) corrected threshold of .05 ( q -value) to account for multiple testing.
Sensitivity analysis: test for directional pleiotropy and pleiotropy-robust methods
For outlier diagnostics indicating invalid instruments (violation of the exclusion restriction assumption), the Q and I² statistic were used to test globally for heterogeneity. Additionally, leave-one out analysis was conducted to check whether the overall estimate was driven by a specific SNP. Furthermore, the MR Egger intercept test was conducted to evaluate potential influences of directional pleiotropic effects (i.e., the average pleiotropic effect deviates from zero and is shifted in one direction). Weighted median, radial regression MR, and MR pleiotropy residual sum and outlier (MR PRESSO) [ 26 ] were conducted as pleiotropy-robust methods.
Causal analysis using summary effect estimates (CAUSE) and test for reverse causation
Facing the low effective sample size in some summary statistics, we employed Causal Analysis Using Summary Effect estimates (CAUSE) that uses all genetic variants for causal estimation, thereby increasing statistical power [ 15 ]. CAUSE aims to distinguish between a causal effect of the exposure on the outcome (i.e., correlation of between all SNP-exposure and SNP-outcome estimates of all genetic variants associated with the exposure) from correlated pleiotropy induced by a shared (unknown) factor (i.e., correlation only in a subset of variants). Causal inference was obtained by a Bayesian approach comparing the two nested models, the causal model allowing a nonzero causal effect and the sharing model with causal effect fixed at zero [ 15 ].
Reverse causation analysis, i.e. exposure and outcome were swapped, was also carried out by CAUSE due to the low number of genetic instruments and effective sample size in some outcome GWAS.
Replication analysis
As replication analysis, we used SNPs from a second childhood maltreatment GWAS of 15,651 individuals of European descent from the Avon Longitudinal Study of Parents and Children (ALSPAC) [ 27 ], Adolescent Brain Cognitive Development Study (ABCD) [ 28 ], and Generation R [ 29 ] recording childhood maltreatment prospectively using multiple questionnaires at multiple instances (majority parent report, several self-report) [ 12 ]. Since this GWAS for childhood maltreatment is also of limited statistical power, we again employed the CAUSE approach which increases power by incorporating all genetic variants.
In addition, we rerun the primary analysis replacing the estimated EXT by the externalizing factor obtained from a GWAS conducted by the Externalizing Consortium (EXT-CON) excluding 23andme [ 5 , 30 ] with 579 genome-wide significant SNPs. These SEM-GWAS employed a broader definition of externalizing traits for inclusion and did not limit their analysis solely to ICD-coded disorders.
All analyses were performed using the packages MRInstruments (0.3.2), MendelianRandomization (0.6.0), TwoSampleMR (0.5.6), MRPRESSO (1.0) and cause (1.2.0) in R, version 4.2.2 (2022/10/31). We report the methods and results following the STROBE-MR (Strengthening the Reporting of Observational studies in Epidemiology – Mendelian randomization) statement [ 31 ].
The common factor model exhibited a CFI of 1 and a SRMR of 0.097, indicating a good model fit (CFI > 0.95, SRMR < 0.10). All indicators showed standardized loadings on the EXT over 0.60, with strong loadings for AUD (0.84, SE = 0.05, p = 2.31 × 10 −54 ) and CUD (0.82, SE = 0.06, p = 2.57 × 10 −40 ), moderate loadings for ADHD (0.63, SE = 0.05, p = 1.29 × 10 −31 ), CD (0.74, SE = 0.06, p = 2.37 × 10 −13 ), ASPD (0.77, SE = 0.09, p = 2.31 × 10 −54 ), and OUD (0.75, SE = 0.11, p = 1.15 × 10 −12 ) (see Fig. 2 ). The Supplementary Fig. S1 depicts the genetic correlation matrix of the indicator GWAS. The EXT explained 39.2% of the variance of ADHD, 54.6% of CD, 58.6% of ASPD, 69.7% of AUD, 57.0% of OUD, and 66.9% of CUD. The chi-squared-test yielded a non-significant result (χ 2 ( df = 9) = 6.91, P = 0.65), indicating a better fit for the common model to the observed GWAS data. This also confirmed the existence of a shared genetic basis of the six externalizing phenotypes. Q SNP analysis identified four SNPs displaying remarkable heterogeneity ( P < 5 × 10 −08 ), but only one overlapped with the genome-wide significant variants associated with the EXT, indicating no pleiotropic effect among individual externalizing disorders, independent of the common EXT. After excluding SNPs with a significant heterogeneity test ( P < 0.05), the common GWAS comprised 6,004,696 SNPs associated with the EXT. Each individual externalizing disorders notably contributed to the EXT, as evidenced by comparable correlation between the different leave-one-out models ( r g : 0.90–0.99, SE: 0.01–0.17). The common factor GWAS exhibited 45 independent genome-wide significant genetic variants with 39 genomic risk loci. Figure 3 illustrates the top 10 genes with the strongest associations (see also Supplementary Table S3 ). The top five genes include forkhead box P2 (FOXP2), cell adhesion molecule 2 (CADM2), glutamate ionotropic receptor delta type subunit 2 (GRID2), bassoon presynaptic cytomatrix protein (BSN), and semaphoring 6D (SEMA6D). These genes were previously associated among others with externalizing disorders and other psychiatric [ 32 , 33 ] as well as addiction related traits [ 34 , 35 ].

The rectangles symbolize the indicators, the latent common factor is presented as circle. Single headed arrows indicate the direction of the regression effect with the standardized loadings. Double headed arrows reflect standardized residuals.
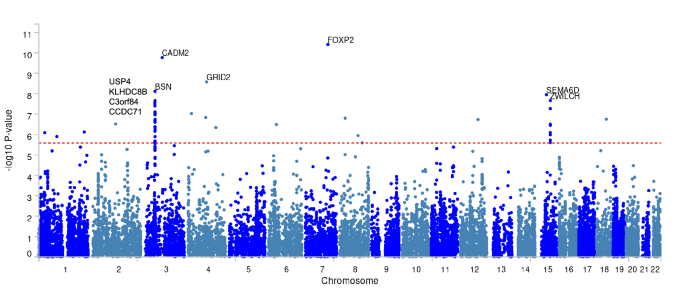
The 10 significant genes with the strongest association are labeled. The red dashed line indicates Bonferroni corrected significance threshold at 2.61 × 10 −6 .
Power analysis
The analysis had a power of ≥90% to detect a minimum OR of 2.00 for ADHD, 5.00 for CD, >5.00 for ASPD, 1.80 for AUD, 4.00 for OUD, and 1.80 for CUD (Supplementary Table S4 ).
The 6 selected genetic instruments explained 0.2% of the variability of the exposure, with a minimum F -statistic of 29.85 (Supplementary Table S5 ). The Standard IVW MR analysis showed significant effects corrected for multiple testing of childhood maltreatment on ADHD, AUD and the EXT. The effects of childhood maltreatment on CD, ASPD, OUD and CUD did not reach statistical significance (see Fig. 4 and Supplementary Table S6 ).
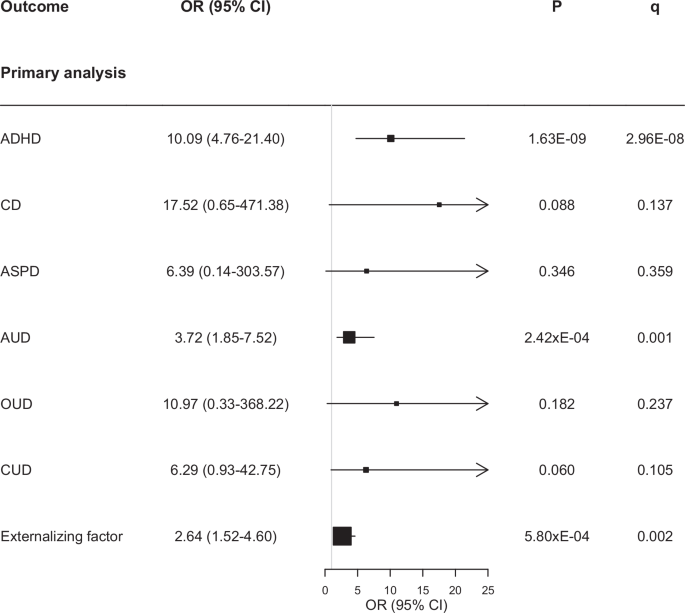
CI confidence interval, OR odds ratio, P = p value, q = adjusted p values using a FDR approach.
For CUD and the EXT, we observed heterogeneity between the Wald ratios of the IVW estimates suggesting pleiotropy. However, the MR Egger intercept test indicated no directional pleiotropy for both outcomes (Supplementary Table S7 ). Visual inspection of funnel plots (Supplementary Fig. S2 ) supported these findings and showed no strong deviation from symmetrical distributions, indicating balanced rather than directional pleiotropy and does not distort causal estimation. The applied random effects IVW model accounts for additional heterogeneity. Consistent with this, pleiotropy-robust methods (weighted median, radial regression MR, and MR PRESSO) showed similar results to the random effects IVW for all phenotypes (Supplementary Table S6 ). Additionally, the stepwise leave-one-out analysis did not reveal any genetic variant as a leverage point with high influence (see Supplementary Table S8 ).
The CAUSE approach confirmed the significant causal associations of childhood maltreatment with ADHD ( OR = 1.90, 95% credible interval ( CredIn ): 1.23–2.91, P = 0.004), ASPD ( OR = 9.12, 95% CredIn : 1.07–79.04; P = 0.045), and the EXT ( OR = 1.34, 95% CredIn : 1.14–1.67; P = 1.43 × 10 −07 ). However, there was no significant difference between the causal and shared model for CD ( OR = 3.94, 95% CredIn : 0.56–25.79; P = 0.153), AUD ( OR = 1.35, 95% CredIn : 0.84–2.14; P = 0.201), OUD ( OR = 2.41, 95% CredIn : 0.51–11.14; P = 0.260), and CUD ( OR = 2.36, 95% CredIn : 0.87–1.42; P = 0.179) suggesting no causal association of child maltreatment on those traits.
Reverse causation analyses suggested only a significant causal influence of ADHD ( OR = 1.01, 95% CredIn : 1.00–1.02, P = 8.85 × 10 −05 ) on childhood maltreatment with estimates close to one, but not for CD ( OR = 1.01, 95% CredIn : 0.99–1.04, p = 0.514), ASPD ( OR = 1.01, 95% CredIn : 0.99–1.03, P = 0.327), AUD ( OR = 1.02, 95% CredIn: 0.99–1.04, P = 0.050), OUD ( OR = 1.00, 95% CredIn: 0.98–1.02, P = 1.00), CUD ( OR = 1.00, 95% CredIn: 0.98–1.02, P = 1.00), and the EXT ( OR = 1.01, 95% CredIn: 0.99–1.04, P = 0.514).
Analysis using an independent childhood maltreatment GWAS replicates only the finding of a causal effect of childhood maltreatment on the EXT ( OR = 1.31, 95% CredIn : 1.04–1.64, P = 0.021), but not on ADHD and AUD. The null association with all other externalizing disorders was confirmed (Supplementary Table S9 ).
In a second replication we replaced the summary statistics of the calculated EXT by the EXT-CON [ 5 , 30 ]. Again, genetically predicted childhood maltreatment is causally associated with the EXT-CON (1.69, 95% CI : 1.30–2.21, p = 8.83 × 10 −05 ).
The current study investigated the causal association between childhood maltreatment and the risk for externalizing disorders such as ADHD, CD, ASPD, and substance use disorders such as AUD, OUD and CUD. Genetically predicted childhood maltreatment strongly increased the risk for ADHD, and AUD in later life, aligning with previous observational studies of ADHD [ 7 ] and alcohol use disorder patients [ 36 ]. In contrast to observational methods, MR methods have the advantage of effectively accounting for effects of unobserved confounding factors. This point is important to emphasize, as there are other potential (confounding) factors (e.g., socioeconomic status, rGE) that contribute to both childhood maltreatment and mental disorders. Furthermore, the causal effect of childhood maltreatment on externalizing disorders is supported by animal studies that infer causality through experiments that are ethically unacceptable in humans. These studies demonstrated that early childhood stress influences alcohol and drug consumption and other behavioral differences in monkeys and rodents [ 37 , 38 ].
We found no causal effect of childhood maltreatment on the risk for development of CD, ASPD, OUD, and CUD. It is important to note that GWAS for CD, ASPD and OUD exhibited a rather small proportion of cases compared to controls, which led to limited power to detect differences. Thus, we performed the CAUSE approach using all genetic variants for causal estimation, thereby increasing statistical power. Using CAUSE, childhood maltreatment is besides ADHD and AUD also causally related with ASPD. However, this causal relation was not identified by IVW analysis, which may be due to low power. Further research using GWAS with a larger effective sample size is needed for clarification.
The main methodological challenge in the presence of rGE is pleiotropy, which can lead to an inaccurate causal estimation. MR PRESSO and MR egger rely on the InSIDE assumption (i.e., pleiotropic effect has to be independent of the instrument strength). Both methods can deal with horizontal pleiotropy (i.e., independent genetic effects on exposure and outcome), but not correlated pleiotropy (i.e., variants influence exposure and outcome through shared genetic factor) induced by rGE [ 26 , 39 ]. Median based estimations are robust to all forms of pleiotropy, albeit to a lesser extent. In contrast, the CAUSE approach distinguishes the causal effect of uncorrelated and correlated pleiotropy induced by rGE [ 15 ]. Across the different pleiotropy-robust methods, including CAUSE, childhood maltreatment was consistently associated with risk for the EXT, confirming a causal effect despite rGE. While CAUSE models a single unobserved factor to account for shared and correlated effects, other approaches directly incorporate family and sibling data to control for biasing family effects [ 40 ]. Since we did not have access to parental genotypic data, we were unable to perform within-family MR. Future studies should aim to replicate our findings using within-family designs to further validate the results.
Our analyses also indicated reverse causation between childhood maltreatment and ADHD. This is consistent with a previous MR using partially overlapping data sources [ 12 ]. Not surprisingly, externalizing behavior and temperament are associated with inadequate parental response, which, along with certain other factors (e.g., low parental self control, socioeconomic status), may promote maladaptive parent-child interactions and childhood maltreatment. To date, only a few observational and MR studies have shown this finding [ 7 , 12 ].
Our study demonstrated for the first time that childhood maltreatment leads to a significant susceptibility to the common EXT. This result was also confirmed with the common factor GWAS from the Externalizing Consortium (EXT-CON) [ 5 , 30 ]. Of note, two of the top five genes associated with EXT in our study (CADM2, SEMA6D) also ranked among the top 10 in the EXT-CON GWAS. In contrast to the EXT-CON model, we incorporated also antisocial traits into our EXT model, a well-established facet of the externalizing dimension in various research lines [ 1 , 2 ]. MR analyses of both independent datasets revealed a robust causal relationship, however with divergent odds ratios, possibly attributed to the limited number of genetic instruments due to small sample size. Previous research supports the notion of a highly heritable externalizing factor ( h 2 : 81–84%) [ 1 , 2 ] underlying externalizing phenotypes. Our structural equation modeling revealed a substantial unexplained variance in specific phenotypes, indicating additional factors (i.e., what distinguishes ADHD from ASPD). This is in line with the hierarchical model of the externalizing spectrum [ 1 , 2 ], positing the existence of both general and specific etiological factors. Furthermore, the divergent effect estimates for different disorders in our study also suggest the existence of additional specific factors contributing to the manifestation of individual disorders.
Moreover, when regarding childhood maltreatment as a comprehensive risk factor, it raises the possibility that it might also play a role in increasing susceptibility within the internalizing dimension, such as depression and anxiety. We plan to investigate this aspect in an upcoming study, where we will assess the impact on both the externalizing and internalizing dimensions.
Our study has several limitations. Firstly, the genetic variants we selected explained only a small fraction of the overall variance in childhood maltreatment. Consequently, the GWAS for childhood maltreatment revealed a relatively low number of genome-wide significant SNPs as instruments for MR analyses. Nonetheless, our chosen instruments demonstrated a minimum F-statistic of 29.85, which indicates no evidence of weak instrument bias, reinforcing the reliability of our selected instruments. Furthermore, the complementary CAUSE approach utilizes all available genetic variants, enhancing statistical power. Secondly, as previously mentioned, the statistical power of specific analyses, particularly those related to CD, ASPD, and OUD, was constrained by the relatively small number of cases falling below 1000. However, the incorporation of the complementary CAUSE approach allowed us to generate better powered causal estimates, thereby reducing the false-positive rate. Thirdly, despite exclusively including GWAS on ICD-coded outcomes, it’s possible that variation in measurement methods existed across the different cohorts.
The current study demonstrated that childhood maltreatment ranks among the etiological influences of the common externalizing factor, besides the existence of factors contributing to the specific phenotypes separately. This has crucial implications for prevention strategies. First, it underlines the importance of primary and secondary prevention services, as childhood maltreatment has now been established as a vulnerability factor for numerous psychiatric conditions. Second, our findings support the use of a comprehensive understanding of externalizing disorders in the development of tertiary prevention services for childhood maltreatment, regardless of the onset of externalizing disorders. For instance, interventions could focus on negative emotionality, low fearfulness and effortful control [ 41 ] or target the biological changes (e.g., altered cortisol reactivity), also associated with early stages of the externalizing spectrum [ 42 ].
Data availability
GWAS summary statistics for attention deficit hyperactivity disorder and cannabinoid use disorder from the Psychiatric Genomics Consortium are available at https://pgc.unc.edu/for-researchers/download-results/ , for conduct disorder, antisocial personality disorder, alcohol use disorder, opioid use disorder from the FinnGen Consortium at https://www.finngen.fi/en/access_results/ . The R code is available from the corresponding author on request.
Krueger RF, Hicks BM, Patrick CJ, Carlson SR, Iacono WG, McGue M. Etiologic connections among substance dependence, antisocial behavior, and personality: modeling the externalizing spectrum. J Abnorm Psychol. 2002;111:411–24.
Article PubMed Google Scholar
Young DS, Kramer LD, Maffei JG, Dusek RJ, Backenson PB, Mores CN, et al. Molecular epidemiology of eastern equine encephalitis virus, New York. Emerg Infect Dis. 2008;14:454–60.
Article CAS PubMed PubMed Central Google Scholar
Krueger RF, Tackett JL. The externalizing spectrum of personality and psychopathology: an Empirical and quantitive alternative to discrete disorder approaches. In: Beauchaine TP, Hinshaw SP, editors. The Oxford handbook of externalizing spectrum disorders. Oxford: Oxford University Press; 2015. p. 79–89.
Google Scholar
Hicks BM, Krueger RF, Iacono WG, McGue M, Patrick CJ. Family transmission and heritability of externalizing disorders: a twin-family study. Arch Gen Psychiatry. 2004;61:922–8.
Karlsson Linnér R, Mallard TT, Barr PB, Sanchez-Roige S, Madole JW, Driver MN, et al. Multivariate analysis of 1.5 million people identifies genetic associations with traits related to self-regulation and addiction. Nat Neurosci. 2021;24:1367–76.
Cicchetti D, Barnett D. Toward the development of a scientific nosology of child maltreatment. In: Cicchetti D, Grove WM, editors. Thinking clearly about psychology: Essays in honor of Paul E Meehl. 2. Minnesota, USA: University of Minnesota Press; 1991. p. 346–77.
Ouyang L, Fang X, Mercy J, Perou R, Grosse SD. Attention-deficit/hyperactivity disorder symptoms and child maltreatment: a population-based study. J Pediatr. 2008;153:851–6.
Luntz BK, Widom CS. Antisocial personality disorder in abused and neglected children grown up. Am J Psychiatry. 1994;151:670–4.
Article CAS PubMed Google Scholar
Harrison PA, Fulkerson JA, Beebe TJ. Multiple substance use among adolescent physical and sexual abuse victims. Child Abuse Negl. 1997;21:529–39.
Pittner K, Bakermans-Kranenburg MJ, Alink LR, Buisman RS, van den Berg LJ, Block LHC-d, et al. Estimating the heritability of experiencing child maltreatment in an extended family design. Child Maltreatment. 2020;25:289–99.
Schulz-Heik RJ, Rhee SH, Silvern L, Lessem JM, Haberstick BC, Hopfer C, et al. Investigation of genetically mediated child effects on maltreatment. Behav Genet. 2009;39:265–76.
Article PubMed Central Google Scholar
Warrier V, Kwong AS, Luo M, Dalvie S, Croft J, Sallis HM, et al. Gene-environment correlations and causal effects of childhood maltreatment on physical and mental health: a genetically informed approach. Lancet Psychiatry. 2021;8:373–86.
Article PubMed PubMed Central Google Scholar
Burgess S, Foley CN, Zuber V. Inferring causal relationships between risk factors and outcomes using genetic variation. Handbook of Statistical Genomics: Two Volume Set. UK: John Wiley & Sons Ltd; 2019. p. 651–20.
Chapter Google Scholar
Jaffee SR, Price TS. Genotype–environment correlations: implications for determining the relationship between environmental exposures and psychiatric illness. Psychiatry. 2008;7:496–9.
Morrison J, Knoblauch N, Marcus JH, Stephens M, He X. Mendelian randomization accounting for correlated and uncorrelated pleiotropic effects using genome-wide summary statistics. Nat Genet. 2020;52:740–7.
Avinun R. The E is in the G: gene–environment–trait correlations and findings from genome-wide association studies. Perspect Psychol Sci. 2020;15:81–9.
Hemani G, Bowden J, Davey Smith G. Evaluating the potential role of pleiotropy in Mendelian randomization studies. Hum Mol Genet. 2018;27:195–208.
Article Google Scholar
Glaesmer H, Schulz A, Häuser W, Freyberger HJ, Brähler E, Grabe H-J. Der childhood trauma screener (CTS)–Entwicklung und Validierung von Schwellenwerten zur Klassifikation. Psychiatr Prax. 2013;40:220–6.
Demontis D, Walters RK, Martin J, Mattheisen M, Als TD, Agerbo E, et al. Discovery of the first genome-wide significant risk loci for attention deficit/hyperactivity disorder. Nat Genet. 2019;51:63–75.
Kurki, M.I., Karjalainen, J., Palta, P. et al. FinnGen provides genetic insights from a well-phenotyped isolated population. Nature 2023;613:508–18.
Johnson EC, Demontis D, Thorgeirsson TE, Walters RK, Polimanti R, Hatoum AS, et al. A large-scale genome-wide association study meta-analysis of cannabis use disorder. Lancet Psychiatry. 2020;7:1032–45.
Hu LT, Bentler PM. Cutoff criteria for fit indexes in covariance structure analysis: conventional criteria versus new alternatives. Structural Equation Modeling: a Multidisciplinary Journal. 1999;6:1–55.
Bentler PM, Hu LT. Structural Equation Modeling: Concepts, Issues, and Applications. California, USA: SAGE Publications; 1995.
de Leeuw CA, Mooij JM, Heskes T, Posthuma D. MAGMA: generalized gene-set analysis of GWAS data. PLoS Comput Biol. 2015;11:e1004219.
Brion M-JA, Shakhbazov K, Visscher PM. Calculating statistical power in Mendelian randomization studies. Int J Epidemiol. 2013;42:1497–501.
Verbanck M, Chen C-Y, Neale B, Do R. Detection of widespread horizontal pleiotropy in causal relationships inferred from Mendelian randomization between complex traits and diseases. Nat Genet. 2018;50:693–8.
Boyd A, Golding J, Macleod J, Lawlor DA, Fraser A, Henderson J, et al. Cohort profile: the ‘children of the 90s’—the index offspring of the Avon Longitudinal Study of Parents and Children. Int J Epidemiol. 2013;42:111–27.
Casey BJ, Cannonier T, Conley MI, Cohen AO, Barch DM, Heitzeg MM, et al. The adolescent brain cognitive development (ABCD) study: imaging acquisition across 21 sites. Dev Cogn Neurosci. 2018;32:43–54.
Kooijman MN, Kruithof CJ, van Duijn CM, Duijts L, Franco OH, van IJzendoorn MH, et al. The Generation R Study: design and cohort update 2017. Eur J Epidemiol. 2016;31:1243–64.
Williams CM, Poore H, Tanksley PT, Kweon H, Courchesne-Krak NS, Londono-Correa D, et al. Guidelines for evaluating the comparability of down-sampled GWAS summary statistics. bioRxiv. 2023:2023.03. 21.533641.
Smith GD, Davies NM, Dimou N, Egger M, Gallo V, Golub R, et al. STROBE-MR: guidelines for strengthening the reporting of Mendelian randomization studies. PeerJ Prepr. 7:e27857v1. [Preprint]. 2019 [cited 2023 Dec 17]. Available from: https://peerj.com/preprints/27857/ . https://doi.org/10.7287/peerj.preprints.27857v1 .
Soler Artigas M, Sánchez-Mora C, Rovira P, Richarte V, Garcia-Martínez I, Pagerols M, et al. Attention-deficit/hyperactivity disorder and lifetime cannabis use: genetic overlap and causality. Mol Psychiatry. 2020;25:2493–503.
Strawbridge RJ, Ward J, Cullen B, Tunbridge EM, Hartz S, Bierut L, et al. Genome-wide analysis of self-reported risk-taking behaviour and cross-disorder genetic correlations in the UK Biobank cohort. Transl Psychiatry. 2018;8:39.
Levey DF, Galimberti M, Deak JD, Wendt FR, Bhattacharya A, Koller D, et al. Multi-ancestry genome-wide association study of cannabis use disorder yields insight into disease biology and public health implications. Nat Genet. 2023;55:2094–103.
Xu K, Li B, McGinnis KA, Vickers-Smith R, Dao C, Sun N, et al. Genome-wide association study of smoking trajectory and meta-analysis of smoking status in 842,000 individuals. Nat Commun. 2020;11:5302.
Widom CS, Hiller-Sturmhöfel S. Alcohol abuse as a risk factor for and consequence of child abuse. Alcohol Res Health. 2001;25:52.
CAS PubMed PubMed Central Google Scholar
Bassey RB, Gondré-Lewis MC. Combined early life stressors: prenatal nicotine and maternal deprivation interact to influence affective and drug seeking behavioral phenotypes in rats. Behav Brain Res. 2019;359:814–22.
Moffett M, Vicentic A, Kozel M, Plotsky P, Francis D, Kuhar M. Maternal separation alters drug intake patterns in adulthood in rats. Biochem Pharmacol. 2007;73:321–30.
Bowden J, Davey Smith G, Haycock PC, Burgess S. Consistent estimation in Mendelian randomization with some invalid instruments using a weighted median estimator. Genet Epidemiol. 2016;40:304–14.
Brumpton B, Sanderson E, Heilbron K, Hartwig FP, Harrison S, Vie GÅ, et al. Avoiding dynastic, assortative mating, and population stratification biases in Mendelian randomization through within-family analyses. Nat Commun. 2020;11:1–13.
Krieger FV, Stringaris A Temperament and vulnerability to externalzing behaviour. In: Beauchaine TP, Hinshaw SP, editors. The Oxford handbook of externalizing spectrum disorders . USA: Oxford University Press, 2015.
Konzok J, Henze GI, Peter H, Giglberger M, Bärtl C, Massau C, et al. Externalizing behavior in healthy young adults is associated with lower cortisol responses to acute stress and altered neural activation in the dorsal striatum. Psychophysiology. 2021;58:e13936.
Download references
Acknowledgements
We assert that all procedures contributing to this work comply with the ethical standards of the relevant national and institutional committees on human experimentation and with the Helsinki Declaration of 1975, as revised in 2008. All procedures involving human participants were approved by the committee in the original studies. Written informed consent was obtained from all participants in the original studies. Additionally, we want to acknowledge the participants and investigators of the FinnGen study.
Open Access funding enabled and organized by Projekt DEAL.
Author information
Authors and affiliations.
Department of Epidemiology and Preventive Medicine, University of Regensburg, Regensburg, Germany
Julian Konzok, Michael F. Leitzmann & Hansjörg Baurecht
Department of Genetic Epidemiology, University of Regensburg, Regensburg, Germany
Mathias Gorski & Thomas W. Winkler
Institute of Health Services Research in Dentistry, University of Münster, Münster, Germany
Sebastian E. Baumeister
Department of Psychiatry, University of Cambridge, Cambridge, UK
Varun Warrier
You can also search for this author in PubMed Google Scholar
Contributions
JK and HB designed the study. JK, HB, TWW, MG, VW and SEB performed the analysis. JK drafted the manuscript. SEB, TWW, MG, VW, MFL and HB provided editorial revisions and suggestions.
Corresponding author
Correspondence to Julian Konzok .
Ethics declarations
Competing interests.
The authors declare no competing interests.
Additional information
Publisher’s note Springer Nature remains neutral with regard to jurisdictional claims in published maps and institutional affiliations.
Supplementary information
41380_2024_2700_moesm1_esm.docx.
Supplements: Child maltreatment as a transdiagnostic risk factor for the externalizing dimension: A Mendelian Randomization study
Rights and permissions
Open Access This article is licensed under a Creative Commons Attribution 4.0 International License, which permits use, sharing, adaptation, distribution and reproduction in any medium or format, as long as you give appropriate credit to the original author(s) and the source, provide a link to the Creative Commons licence, and indicate if changes were made. The images or other third party material in this article are included in the article’s Creative Commons licence, unless indicated otherwise in a credit line to the material. If material is not included in the article’s Creative Commons licence and your intended use is not permitted by statutory regulation or exceeds the permitted use, you will need to obtain permission directly from the copyright holder. To view a copy of this licence, visit http://creativecommons.org/licenses/by/4.0/ .
Reprints and permissions
About this article
Cite this article.
Konzok, J., Gorski, M., Winkler, T.W. et al. Child maltreatment as a transdiagnostic risk factor for the externalizing dimension: a Mendelian randomization study. Mol Psychiatry (2024). https://doi.org/10.1038/s41380-024-02700-8
Download citation
Received : 17 December 2023
Revised : 14 August 2024
Accepted : 15 August 2024
Published : 22 August 2024
DOI : https://doi.org/10.1038/s41380-024-02700-8
Share this article
Anyone you share the following link with will be able to read this content:
Sorry, a shareable link is not currently available for this article.
Provided by the Springer Nature SharedIt content-sharing initiative
Quick links
- Explore articles by subject
- Guide to authors
- Editorial policies

Information
- Author Services
Initiatives
You are accessing a machine-readable page. In order to be human-readable, please install an RSS reader.
All articles published by MDPI are made immediately available worldwide under an open access license. No special permission is required to reuse all or part of the article published by MDPI, including figures and tables. For articles published under an open access Creative Common CC BY license, any part of the article may be reused without permission provided that the original article is clearly cited. For more information, please refer to https://www.mdpi.com/openaccess .
Feature papers represent the most advanced research with significant potential for high impact in the field. A Feature Paper should be a substantial original Article that involves several techniques or approaches, provides an outlook for future research directions and describes possible research applications.
Feature papers are submitted upon individual invitation or recommendation by the scientific editors and must receive positive feedback from the reviewers.
Editor’s Choice articles are based on recommendations by the scientific editors of MDPI journals from around the world. Editors select a small number of articles recently published in the journal that they believe will be particularly interesting to readers, or important in the respective research area. The aim is to provide a snapshot of some of the most exciting work published in the various research areas of the journal.
Original Submission Date Received: .
- Active Journals
- Find a Journal
- Proceedings Series
- For Authors
- For Reviewers
- For Editors
- For Librarians
- For Publishers
- For Societies
- For Conference Organizers
- Open Access Policy
- Institutional Open Access Program
- Special Issues Guidelines
- Editorial Process
- Research and Publication Ethics
- Article Processing Charges
- Testimonials
- Preprints.org
- SciProfiles
- Encyclopedia
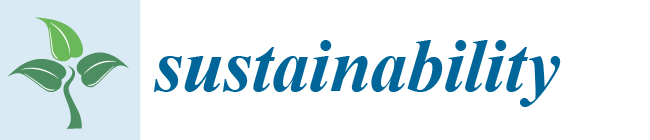
Article Menu
- Subscribe SciFeed
- Recommended Articles
- Google Scholar
- on Google Scholar
- Table of Contents
Find support for a specific problem in the support section of our website.
Please let us know what you think of our products and services.
Visit our dedicated information section to learn more about MDPI.
JSmol Viewer
Research on energy-saving efficiency and influencing factors of green and low-carbon enterprises based on three-stage dea and tobit models.
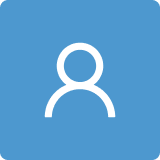
1. Introduction
2. research design, 2.1. three-stage dea model construction, 2.1.1. phase 1: analyzing the efficiency of traditional dea models, 2.1.2. phase 2: sfa regression modeling, 2.1.3. phase 3: adjusted dea efficiency analysis, 2.2. tobit regression model, 2.3. indicator selection, 2.3.1. input and output variables, 2.3.2. environmental variables, 2.3.3. influencing factors, 3. empirical analysis, 3.1. data sources, 3.2. measurement of energy-saving efficiency of green and low-carbon enterprises, 3.2.1. analysis of traditional dea results in the first stage, 3.2.2. second-stage sfa regression results, 3.2.3. phase-iii adjusted dea efficiency analysis, 3.2.4. analysis of influencing factors, 4. conclusions and recommendations, author contributions, institutional review board statement, informed consent statement, data availability statement, conflicts of interest.
- China Academy of Engineering Science and Technology Development Strategy. 2021 Report on the Development of Strategic Emerging Industries in China ; Science Press: Beijing, China, 2020; pp. 141–142. [ Google Scholar ]
- Li, D.B.; Yuan, X.; Wang, Y. Efficiency analysis of science and technology-based enterprises in the new three boards of Sichuan province based on DEA model. Natl. Circ. Econ. 2023 , 22 , 136–139. [ Google Scholar ] [ CrossRef ]
- Wu, D.R.; Xu, Y.J. Research on the efficiency of rural e-commerce level and regional economic development in Jiangxi Province—Based on DEA model. Shanxi Agric. Econ. 2023 , 21 , 50–53. [ Google Scholar ] [ CrossRef ]
- Zhang, J.J.; Huang, B.R.; Su, L.Y. Research on energy saving and emission reduction potential of traditional industries in Jiangxi Province based on DEA model. Pract. Underst. Math. 2018 , 48 , 9–19. [ Google Scholar ]
- Sun, Y.C.; Rao, Z.H.; Tang, M.C. Evaluation of Energy Saving and Emission Reduction Efficiency in Guangdong Province Based on Undesired Output. J. Harbin Inst. 2020 , 41 , 31–33. [ Google Scholar ]
- Wang, H.Y.; Lian, J.L. Evaluation of operational efficiency of new energy wind power manufacturing enterprises based on three-stage DEA. Mod. Ind. Econ. Inf. Technol. 2023 , 13 , 238–241. [ Google Scholar ] [ CrossRef ]
- Zhang, J.G.; Yang, H.J. Evaluation of provincial energy saving and emission reduction efficiency based on DDF-DEA three-stage model. China Popul.-Resour. Environ. 2018 , 28 , 24–31. [ Google Scholar ]
- Zhu, Z.H.; Li, J.J. Evaluation and trend analysis of regional energy conservation and emission reduction efficiency in Fujian Province—Based on three-stage SBM-DEA model. J. Fujian Bus. Coll. 2020 , 5 , 1–8+81. [ Google Scholar ] [ CrossRef ]
- Zhang, H.; Mao, J.H.; Wang, T.Y. The main influencing factors of technological innovation efficiency improvement in China’s intelligent manufacturing enterprises—An analysis based on the three-stage DEA-Tobit model. Res. Sci. Technol. Manag. 2023 , 43 , 95–101. [ Google Scholar ]
- Yang, Q.S.; Yang, T.A.; Shen, Z.G. Evaluation of Innovation Efficiency and Analysis of Influencing Factors in National High-Tech Zones-Based on Three-Stage DEA-Tobit Model. Innov. Technol. 2023 , 23 , 13–27. [ Google Scholar ] [ CrossRef ]
- Ma, W.B.; Zhu, H. Research on the Measurement of Innovation Efficiency and Influencing Factors of Green and Low-Carbon Enterprises-Based on Three-Stage DEA and Tobit Model. Soft Sci. 2023 , 1–12. [ Google Scholar ]
- Li, J.N. Research on Green Innovation Efficiency and Its Influencing Factors under the Perspective of Science and Technology Finance ; Gannan Normal University: Ganzhou, China, 2023. [ Google Scholar ]
- Tian, J.L.; Xiang, S.; Rong, W. Exploring the Measurement of Regional Forestry Eco-Efficiency and Influencing Factors in China Based on the Super-Efficient DEA-Tobit Two Stage Model. Forests 2023 , 14 , 300. [ Google Scholar ] [ CrossRef ]
- Han, B.; Feng, X.W.; Su, Y. Research on innovation efficiency measurement and influencing factors of Chinese new energy vehicle listed companies—Based on three-stage DEA and Tobit panel model. Sci. Technol. Prog. Countermeas. 2023 , 40 , 110–120. [ Google Scholar ]
- Lu, F.; Han, H.; He, B. Evaluation on the development of urban low-carbon passenger transportation structure in Tianjin. Res. Transp. Bus. Manag. 2024 , 55 , 101142. [ Google Scholar ] [ CrossRef ]
- Fried, H.O.; Lovell, C.K.; Schmidt, S.S. Accounting for Environmental Effects and Statistical Noise in Data Envelopment Analysis. J. Product. Anal. 2002 , 17 , 157–174. [ Google Scholar ] [ CrossRef ]
- Qin, J.J. Energy saving and carbon reduction paths in industrial enterprises. Resour. Conserv. Environ. Prot. 2023 , 12 , 1–4. [ Google Scholar ] [ CrossRef ]
- Wen, Z.L.; Zou, W. Analysis of energy-saving diagnostic problems and countermeasures for green low-carbon transformation in small and medium-sized enterprises in Jiangxi Province. Energy Res. Manag. 2023 , 4 , 10–15. [ Google Scholar ] [ CrossRef ]
- Lin, R.J. Research on Energy Measurement Management and Energy Saving and Consumption Reduction of Enterprises under the Goal of “Double Carbon”. Energy Energy Conserv. 2023 , 12 , 72–74. [ Google Scholar ] [ CrossRef ]
- Dong, Y. Research on the Impact of Energy-Saving and Environmental Protection Industrial Policy on the Investment Efficiency of Green Enterprises ; Hebei University: Tianjin, China, 2023. [ Google Scholar ] [ CrossRef ]
- Liu, S.; Kuang, J.; Ding, D.; Madsen, D.Ø.; Shi, X.; Fan, X. Low-carbon governance, fiscal decentralization, and enterprise green development: Evidence from China. PLoS ONE 2024 , 19 , e0296490. [ Google Scholar ] [ CrossRef ]
- Xiao, R.Q.; Chen, Z.W.; Qian, L. Research on innovation efficiency of China’s high-tech manufacturing industry under the perspective of heterogeneous technology. Manag. Sci. 2018 , 31 , 48–68. [ Google Scholar ]
- Dai, K.Z. The impact of technology market development on export technology complexity and its role mechanism. China Ind. Econ. 2018 , 117–135. [ Google Scholar ]
- Vandevenne, N.; Riel, V.J.; Poels, G. Green Enterprise Architecture (GREAN)—Leveraging EA for Environmentally Sustainable Digital Transformation. Sustainability 2023 , 15 , 14342. [ Google Scholar ] [ CrossRef ]
- Xia, L. The impact of government subsidies on corporate R&D investment--an empirical analysis based on strategic emerging industries. Friends Account. 2020 , 24 , 132–137. [ Google Scholar ]
- Su, Y.; Lin, Y.N. Research on the impact of government subsidies on R&D investment of new energy enterprises. Sci. Manag. Res. 2021 , 39 , 102–110. [ Google Scholar ] [ CrossRef ]
Click here to enlarge figure
Variable Type | Variant | Specific Definitions | Unit | Data Sources |
---|---|---|---|---|
Input variable | Financial investment in energy efficiency | Enterprises’ investment funds for energy-saving renovation in the t years | billions | China Research Data Service Platform (CNRDS), Corporate Annual Reports |
Electricity consumption | Total electricity consumption of the enterprise in the t years | billion kilowatt-hours (kWh) | ||
Equipment usage | Main production equipment used by the enterprise in the t years | item on a program | ||
Output variable | Average carbon emissions per unit of GDP | Carbon emissions per unit of GDP produced by an enterprise in the t years | million per tonne | |
Average energy consumption per unit of GDP | Ratio of total energy consumption of the enterprise in the t years to total GDP in that year | million per tonne of standard coal | ||
Operating revenue | Total business income in the t years | billions |
Norm | Financial Investment in Energy Efficiency | Electricity Consumption | Equipment Usage | |||
---|---|---|---|---|---|---|
Regression Coefficient | t-Statistic | Regression Coefficient | t-Statistic | Regression Coefficient | t-Statistic | |
Average carbon emissions per unit of GDP | 0.698 ** | 2.115 | 0.703 ** | 2.059 | 0.705 ** | 2.256 |
Average energy consumption per unit of GDP | 0.568 ** | 1.992 | 0.742 ** | 2.145 | 0.652 ** | 2.189 |
Operating revenue | 0.615 ** | 2.024 | 0.721 ** | 2.139 | 0.689 ** | 2.146 |
Year | All Enterprises | Southern Enterprises | Northern Enterprises | ||||||
---|---|---|---|---|---|---|---|---|---|
TE | PTE | SE | TE | PTE | SE | TE | PTE | SE | |
2018 | 0.186 | 0.256 | 0.728 | 0.268 | 0.368 | 0.730 | 0.162 | 0.222 | 0.731 |
2019 | 0.139 | 0.309 | 0.450 | 0.165 | 0.264 | 0.626 | 0.126 | 0.344 | 0.367 |
2020 | 0.177 | 0.311 | 0.571 | 0.215 | 0.315 | 0.685 | 0.164 | 0.323 | 0.508 |
2021 | 0.291 | 0.386 | 0.754 | 0.327 | 0.394 | 0.832 | 0.276 | 0.391 | 0.707 |
2022 | 0.272 | 0.382 | 0.713 | 0.275 | 0.340 | 0.810 | 0.243 | 0.374 | 0.651 |
Average value | 0.213 | 0.328 | 0.643 | 0.250 | 0.336 | 0.736 | 0.194 | 0.330 | 0.592 |
ESCRI | EPI | RRI | |||||||
---|---|---|---|---|---|---|---|---|---|
Average TE | Average PTE | Average SE | Average TE | Average PTE | Average SE | Average TE | Average PTE | Average SE | |
South | 0.205 | 0.328 | 0.625 | 0.251 | 0.354 | 0.710 | 0.204 | 0.339 | 0.602 |
North | 0.187 | 0.308 | 0.609 | 0.231 | 0.332 | 0.698 | 0.184 | 0.314 | 0.588 |
Environment Variable | Energy Efficiency Finance Slack Variables | Electricity Consumption Slack Variables | Equipment Usage Slack Variables | |||
---|---|---|---|---|---|---|
Regression Coefficient | t-Statistic | Regression Coefficient | t-Statistic | Regression Coefficient | t-Statistic | |
Constant term | 15,896.257 *** | 5.252 | 8285.215 *** | 3.685 | 94,324.215 *** | 5.842 |
Environmental regulation | −11,243.252 *** | −4.683 | −486.371 *** | −2.669 | −6204.130 *** | −3.144 |
Market competitiveness | −7472.563 *** | −2.251 | −230.054 *** | −2.758 | −4358.861 *** | −3.038 |
Quality of labour | −16,243.452 *** | −4.092 | −704.025 *** | −3.017 | −8123.159 *** | −3.485 |
Level of economic development | −12,520.156 *** | −3.482 | −203.245 *** | −2.825 | −2537.825 *** | −2.984 |
σ | 6,543,314,102 | 10,132,376 | 1,426,301,429 | |||
γ | 0.999 | 0.999 | 0.999 | |||
Log value | −1932.113 | −1019.158 | −1779.234 | |||
LR | 154.647 *** | 2.988 | 142.531 *** | 3.024 | 159.435 *** | 3.154 |
Year | All Enterprises | Southern Enterprises | Northern Enterprises | ||||||
---|---|---|---|---|---|---|---|---|---|
TE | PTE | SE | TE | PTE | SE | TE | PTE | SE | |
2018 | 0.102 | 0.424 | 0.241 | 0.296 | 0.438 | 0.678 | 0.132 | 0.438 | 0.302 |
2019 | 0.083 | 0.447 | 0.187 | 0.141 | 0.436 | 0.324 | 0.051 | 0.471 | 0.109 |
2020 | 0.101 | 0.434 | 0.232 | 0.173 | 0.457 | 0.379 | 0.059 | 0.446 | 0.134 |
2021 | 0.137 | 0.513 | 0.269 | 0.232 | 0.526 | 0.442 | 0.101 | 0.560 | 0.181 |
2022 | 0.141 | 0.442 | 0.320 | 0.218 | 0.445 | 0.490 | 0.055 | 0.461 | 0.120 |
Average value | 0.113 | 0.452 | 0.249 | 0.212 | 0.460 | 0.462 | 0.081 | 0.475 | 0.169 |
ESCRI | EPI | RRI | |||||||
---|---|---|---|---|---|---|---|---|---|
Average TE | Average PTE | Average SE | Average TE | Average PTE | Average SE | Average TE | Average PTE | Average SE | |
South | 0.318 | 0.328 | 0.726 | 0.231 | 0.295 | 0.650 | 0.201 | 0.298 | 0.579 |
North | 0.326 | 0.357 | 0.703 | 0.224 | 0.287 | 0.611 | 0.221 | 0.304 | 0.596 |
All Enterprises | Southern Enterprises | Northern Enterprises | |||||||
---|---|---|---|---|---|---|---|---|---|
Regression Coefficient | Sta Error | t-Statistic | Regression Coefficient | Sta Error | t-Statistic | Regression Coefficient | Sta Error | t-Statistic | |
Scale | −0.126 * | 0.068 | −1.853 | 0.154 * | 0.082 | 1.878 | −0.218 * | 0.117 | −1.863 |
Quality | 0.032 ** | 0.014 | 2.286 | 0.151 ** | 0.075 | 2.012 | 0.068 * | 0.035 | 1.943 |
Gov | 0.015 * | 0.009 | 1.667 | 0.014 * | 0.008 | 1.775 | 0.241 ** | 0.112 | 2.152 |
Ind | −0.009 | 0.047 | −0.191 | −0.006 | 0.057 | −0.105 | −0.014 | 0.062 | −0.226 |
Auto | 0.356 ** | 0.136 | 2.618 | 1.012 ** | 0.598 | 1.692 | 0.987 * | 0.532 | 1.855 |
Lev | −0.106 | 0.146 | −0.728 | −0.252 | 0.225 | 1.117 | −0.214 | 0.208 | −1.028 |
The South | The North | |||||
---|---|---|---|---|---|---|
ESCRI | EPI | RRI | ESCRI | EPI | RRI | |
Scale | 0.215 * | 0.412 * | 0.128 * | −0.224 * | −0.419 * | −0.135 * |
Quality | 0.152 | 0.143 * | 0.115 | 0.128 | 0.168 * | 0.153 |
Gov | 0.425 * | −0.258 * | −0.322 | 0.578 * | 0.425 | 0.326 |
Ind | −0.087 * | 0.105 | −0.112 * | −0.098 * | 0.125 | −0.187 * |
Auto | 1.154 ** | 1.257 * | 1.012 * | 1.028 ** | 1.033 * | 0.998 * |
Lev | −0.382 | −0.395 | −0.585 | −0.446 | −0.582 | −0.348 |
The statements, opinions and data contained in all publications are solely those of the individual author(s) and contributor(s) and not of MDPI and/or the editor(s). MDPI and/or the editor(s) disclaim responsibility for any injury to people or property resulting from any ideas, methods, instructions or products referred to in the content. |

Share and Cite
Xu, F.; Shao, T.; Hu, R.; Zhang, M. Research on Energy-Saving Efficiency and Influencing Factors of Green and Low-Carbon Enterprises Based on Three-Stage DEA and Tobit Models. Sustainability 2024 , 16 , 7373. https://doi.org/10.3390/su16177373
Xu F, Shao T, Hu R, Zhang M. Research on Energy-Saving Efficiency and Influencing Factors of Green and Low-Carbon Enterprises Based on Three-Stage DEA and Tobit Models. Sustainability . 2024; 16(17):7373. https://doi.org/10.3390/su16177373
Xu, Fenfang, Teng Shao, Ruili Hu, and Minbo Zhang. 2024. "Research on Energy-Saving Efficiency and Influencing Factors of Green and Low-Carbon Enterprises Based on Three-Stage DEA and Tobit Models" Sustainability 16, no. 17: 7373. https://doi.org/10.3390/su16177373
Article Metrics
Article access statistics, further information, mdpi initiatives, follow mdpi.
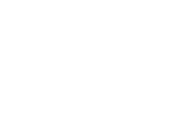
Subscribe to receive issue release notifications and newsletters from MDPI journals
- Open access
- Published: 19 August 2024
The impact of study habits and personal factors on the academic achievement performances of medical students
- Mohammed A. Aljaffer 1 ,
- Ahmad H. Almadani 1 ,
- Abdullah S. AlDughaither 2 ,
- Ali A. Basfar 2 ,
- Saad M. AlGhadir 2 ,
- Yahya A. AlGhamdi 2 ,
- Bassam N. AlHubaysh 2 ,
- Osamah A. AlMayouf 2 ,
- Saleh A. AlGhamdi 3 ,
- Tauseef Ahmad 4 &
- Hamza M. Abdulghani 5
BMC Medical Education volume 24 , Article number: 888 ( 2024 ) Cite this article
510 Accesses
2 Altmetric
Metrics details
Academic achievement is essential for all students seeking a successful career. Studying habits and routines is crucial in achieving such an ultimate goal.
This study investigates the association between study habits, personal factors, and academic achievement, aiming to identify factors that distinguish academically successful medical students.
A cross-sectional study was conducted at the College of Medicine, King Saud University, Riyadh, Saudi Arabia. The participants consisted of 1st through 5th-year medical students, with a sample size of 336. The research team collected study data using an electronic questionnaire containing three sections: socio-demographic data, personal characteristics, and study habits.
The study results indicated a statistically significant association between self-fulfillment as a motivation toward studying and academic achievement ( p = 0.04). The results also showed a statistically significant correlation between recalling recently memorized information and academic achievement ( p = 0.05). Furthermore, a statistically significant association between preferring the information to be presented in a graphical form rather than a written one and academic achievement was also found ( p = 0.03). Students who were satisfied with their academic performance had 1.6 times greater chances of having a high-grade point average (OR = 1.6, p = 0.08).
The results of this study support the available literature, indicating a correlation between study habits and high academic performance. Further multicenter studies are warranted to differentiate between high-achieving students and their peers using qualitative, semi-structured interviews. Educating the students about healthy study habits and enhancing their learning skills would also be of value.
Peer Review reports
Introduction
Academic performance is a common indicator used to measure student achievement [ 1 , 2 ]. It is a compound process influenced by many factors, among which is study habits [ 2 , 3 ]. Study habit is defined as different individual behavior in relation to studying, and is a combination of study methods and skills [ 2 , 3 , 4 ]. Put differently, study habits involve various techniques that would increase motivation and transform the study process into an effective one, thus enhancing learning [ 5 ]. Students’ perspectives and approaches toward studying were found to be the key factors in predicting their academic success [ 6 , 7 ]. However, these learning processes vary from one student to another due to variations in the students’ cognitive processing [ 8 ].
The study habits of students are the regular practices and habits they exhibit during the learning process [ 9 , 10 ]. Over time, several study habits have been developed, such as time management, setting appropriate goals, choosing a comfortable study environment, taking notes effectively, choosing main ideas, and being organized [ 11 ]. Global research shows that study habits impact academic performance and are the most important predictor of it [ 12 ]. It is difficult for medical students to organize and learn a lot of information, and they need to employ study skills to succeed [ 1 , 2 , 5 , 13 ].
Different lifestyle and social factors could affect students’ academic performance. For instance, Jafari et al. found that native students had better study habits compared to dormitory students [ 1 ]. This discrepancy between native and dormitory students was also indicated by Jouhari et al. who illustrated that dormitory students scored lower in attitude, test strategies, choosing main ideas, and concentration [ 10 ]. Regarding sleeping habits, Curcio G et al. found that students with a regular and adequate sleeping pattern had higher Grade Point Average (GPA) scores [ 14 ]. Lifestyle factors, such as watching television and listening to music, were shown to be unremarkable in affecting students’ grades [ 15 , 16 ]. Social media applications, including WhatsApp, Facebook, and Twitter, distract students during learning [ 16 , 17 ].
Motivation was found to be a major factor in students’ academic success. Bonsaksen et al. found that students who chose “to seek meaning” when studying were associated with high GPA scores [ 18 ]. In addition, low scores on “fear of failure” and high scores on “achieving” correlated with a higher GPA [ 8 , 18 ].
Resource-wise, Alzahrani et al. found that 82.7% of students relied on textbooks assigned by the department, while 46.6% mainly relied on the department’s lecture slides [ 19 ]. The study also indicated that 78.8% perceived that the scientific contents of the lectures were adequate [ 19 ]. Another study found that most students relied on the lecture slides (> 83%) along with their notes, followed by educational videos (76.1%), and reference textbooks (46.1%) [ 20 ]. Striking evidence in that study, as well as in another study, indicated that most students tended to avoid textbooks and opted for lecture slides, especially when preparing for exams [ 20 , 21 ].
Several researchers studied the association between different factors and academic performance; however, more is needed to know about this association in the process of education among medical students [ 15 , 20 , 22 ], with some limitations to the conducted studies. Such limitations include the study sample and using self-reported questionnaires, which may generate inaccurate results. Moreover, in Saudi Arabia in particular, the literature concerning the topic remains limited. Since many students are unsatisfied with their performance and seek improvement [ 10 ], the present study was designed and conducted.
Unlike other studies in the region, this study aims to investigate the relationship between study habits and personal factors and measure their influence on academic achievement. The results of this study could raise awareness regarding the effect of study habits and personal factors on students’ performance and would also guide them toward achieving academic success. The study also seeks to identify the factors that distinguish academically successful students from their peers.
Study design, setting, and participants
This observational cross-sectional study, which took place between June and December 2022, was conducted among students attending the College of Medicine at King Saud University (KSU), Riyadh, Saudi Arabia. Its targeted population included all male and female medical students (first to fifth years) attending KSU during the academic year 2021/2022. Whereas, students at other colleges and universities, those who failed to complete the questionnaire, interns (the students who already graduated), and those who were enrolled in the university’s preparatory year, were all excluded from the current study. The sample size was calculated based on a study conducted in 2015 by Lana Al Shawwa [ 15 ]. Using the sample size formula for a single proportion (0.79), the required sample size was 255 using a confidence interval of 95% and a margin of error of 5%. After adding a 20% margin to accommodate non-responses and incomplete responses, the calculated sample size required for this study was 306. However, our research team collected a total of 336 participants for this study to ensure complete representation.
Study instrument
The research team developed and used an electronic questionnaire. The rationale is that no standardized questionnaire measuring the study objectives was found in the literature. However, the questionnaire was tested on a pilot of 15 students to test its clarity and address any possible misconceptions and ambiguity. The study questionnaire was distributed randomly to this cohort, who were asked to fill out the questionnaire. The students reported a complete understanding of the questionnaire’s contents, so the same questionnaire was used without any modifications. The questionnaire, written in English, consisted of three parts. The first part included eleven questions about the socio-demographic status of the participants. The second part contained twenty-one questions examining personal factors such as sleep and caffeine consumption. The last part included twenty-one questions regarding students’ study habits. The questionnaire was constructed based on an ordinal Likert scale which had: strongly agree, agree, neutral, disagree, and strongly disagree as possible answers. The questionnaire was sent to participants through email and social media applications like Twitter and WhatsApp to increase the study response. An informed consent that clearly states the study’s purpose was taken from all participants at the beginning of the questionnaire. In addition, all participants were assured that the collected data would be anonymous and confidential. Each participant was represented by a code for the sole purpose of analyzing the data. Furthermore, no incentives or rewards were given to the participants for their participation.
Study variables
Socio-demographic information (such as age, gender, and academic year), and personal factors (such as motivation, sleeping status, caffeine consumption, and self-management) were the independent variables. Study habits such as attendance, individual versus group study, memorization techniques, revision, learning style, and strategies were also independent variables.
Academic achievement refers to a student’s success in gaining knowledge and understanding in various subjects, as well as the ability to apply that knowledge effectively [ 23 ]. It is a measure of the student’s progress throughout the educational journey, encompassing both academic achievements and personal growth [ 3 , 24 ]. Academic achievement is judged based on the student’s GPA or performance score. In this study, students’ GPA scores, awareness, and satisfaction regarding their academic performance were the dependent variables.
We divided the study sample into two groups based on the GPA. We considered students with high GPAs to be exposed (i.e. exposed to the study habits we are investigating), and students with low GPAs to be the control group. The purpose of this study was to determine why an exposed group of students gets high grades and what study factors they adopt. Based on this exposure (high achieving students), we concluded what methods they used to achieve higher grades. Those in the first group had a GPA greater or equal to 4.5 (out of 5), while those in the second group had a GPA less than 4.5. The students’ data were kept confidential and never used for any other purpose.
Data analysis
The data collected were analyzed by using IBM SPSS Statistical software for Windows version 24.0. Descriptive statistics such as frequency and percentage were used to describe the socio-demographic data in a tabular form. Furthermore, data for categorical variables, including different study habits, motivation factors, memorizing and revising factors, and lifestyle factors, were tabulated and analyzed using the odds ratio test. Finally, we calculated the odds ratio statistic and a p-value of 0.05 to report the statistical significance of our results.
Ethical approval and consent to Participate
Before conducting the study, the research team obtained the Ethics Committee Approval from the Institutional Review Board of the College of Medicine, KSU, Riyadh, Saudi Arabia (project No. E-22-7044). Participants’ agreement/consent to participate was guaranteed by choosing “agree” after reading the consent form at the beginning of the questionnaire. Participation was voluntary, and consent was obtained from all participants. The research team carried out all methods following relevant guidelines and regulations.
The total 336 medical students participated in the study. All participants completed the study questionnaire, and there were no missing or incomplete data, with all of them being able to participate. As shown in Table 1 9.3% of participants were between 18 and 20, 44.9% were between the ages of 21 and 22, and 35.8% were 23–28 years old. In the current study, 62.5% of the participants were males and 37.5% were females. The proportion of first-year students was 21.4%, 20.8% of second-year students, 20.8% of third-year students, 18.2% of fourth-year students, and 18.8% of fifth-year students, according to academic year levels. Regarding GPA scores, 36.9% scored 4.75-5 and 32.4% scored 4.5–4.74. 23.8% achieved 4-4.49, 6.5% achieved 3-3.99, and only 0.4% achieved 2.99 or less. Participants lived with their families in 94.6% of cases, with friends in 1.2% of cases, and alone in 4.2% of cases. For smoking habits, 86.3% did not smoke, 11% reported using vapes, 2.1% used cigarettes, and 0.6% used Shisha. 91.4% of the participants did not report any chronic illnesses; however, 8.6% did. In addition, 83% had no mental illness, 8.9% had anxiety, 6% had depression, and 2.1% reported other mental illnesses.
Table 2 shows motivational factors associated with academic performance. There was a clear difference in motivation factors between students with high and low achievement in the current study. Students with high GPAs were 1.67 times more motivated toward their careers (OR = 1.67, p = 0.09) than those with low GPAs. Furthermore, significant differences were found between those students who had self-fulfillment or ambitions in life they had ~ 2 times higher (OR = 1.93, p = 0.04) GPA scores than low GPA students. Exam results did not motivate exposed or high GPA students (46%) or control students with low GPA students (41%), but the current study showed test results had little impact on low achiever students (OR = 1.03, p = 0.88). Furthermore, 72.6% of high achievers were satisfied with their academic performance, while only 41% of low achiever students were satisfied. Therefore, students who were satisfied with their academic performance had 1.6 times greater chances of a higher GPA (OR = 1.6, p = 0.08). Students who get support and help from those around them are more likely to get high GPAs (OR = 1.1, p = 0.73) than those who do not receive any support. When students reported feeling a sense of family responsibility, the odds (odds ratio) of their receiving higher grades were 1.15 times higher (OR = 1.15, p = 0.6) compared to those who did not feel a sense of family responsibility. The p-value, which indicates the level of statistical significance, was 0.6.
Table 3 shows the study habits of higher achiever students and low achiever students. Most of the high-achieving students (79.0%) attended most of the lectures and had 1.6 times higher chances of getting higher grades (OR = 1.6, p = 0.2) than those who did not attend regular lectures. The current study found that studying alone had no significant impact on academic achievement in either group. However, those students who had studied alone had lower GPAs (OR = 1.07, p = 0.81). The current study findings reported 29.8% of students walk or stand while studying rather than sit, and they had 1.57 times higher GPA chances compared to students with lower GPAs (OR = 0.73, p = 0.27). High achievers (54.0%) preferred studying early in the morning, and these students had higher chances of achieving good GPAs (OR = 1.3, p = 0.28) than low achiever groups of students. The number of students with high achievement (39.5%) went through the lecture before the lesson was taught. These students had 1.08 times higher chances of achieving than low achiever groups of students. Furthermore, students who made a weekly study schedule had 1.3 times higher chances of being good academic achievers than those who did not (OR = 1.3, p = 0.37). Additionally, high-achieving students paid closer attention to the lecturer (1.2 times higher). In addition, students with high GPAs spent more time studying when exam dates approached (OR = 1.3, p = 0.58).
Table 4 demonstrates the relationship between memorizing and revising with high and low GPA students. It was found that high achiever students (58.9%) studied lectures daily and had 1.4 times higher chances of achieving high grades (OR = 1.4, p = 0.16) than the other group. It was found that most of the high achievers (62.1%) skim the lecture beforehand before memorizing it, which led to 1.8 times higher chances of getting good grades in this exam (OR = 1.8, p = 0.06). One regular activity reported by high GPA students (82.3%) was recalling what had just been memorized. For this recalling technique, we found a significant difference between low-achieving students (OR = 0.8, p = 0.63) and high-achieving students (OR = 1.83, p = 0.05). A high achiever student writes notes before speaking out for the memorizing method, which gives 1.2 times greater chances of getting high grades (OR = 1.2, p = 0.55) than a student who does not write notes. A major difference in the current study was that high GPA achievers (70.2%) revise lectures more frequently than low GPA achievers (57.1%). They had 1.5 times more chances of getting high grades if they practiced and revised this method (OR = 1.5, p = 0.13).
Table 5 illustrates the relationship between negative lifestyle factors and students’ academic performance. The current study found that students are less likely to get high exam grades when they smoke. Students who smoke cigarettes and those who vape are 1.14 and 1.07 times respectively more likely to have a decrease in GPA than those who do not smoke. Those students with chronic illnesses had 1.22 times higher chances of a downgrade in the exam (OR = 1.22, p = 0.49). Additionally, students with high GPAs had higher mental pressures (Anxiety = 1.2, Depression = 1.18, and other mental pressures = 1.57) than those with low GPAs.
Learning is a multifaceted process that evolves throughout our lifetimes. The leading indicator that sets students apart is their academic achievement. Hence, it is crucial to investigate the factors that influence it. The present study examined the relationship between different study habits, personal characteristics, and academic achievement among medical students. In medical education, and more so in Saudi Arabia, there needs to be more understanding regarding such vital aspects.
Regarding motivational factors, the present study found some differences between high and low achievers. Students with high GPA scores were more motivated toward their future careers (OR = 1.67, p = 0.09). The study also indicated that students who had ambitions and sought self-fulfillment were more likely to have high GPA scores, which were statistically significant (OR = 1.93, p = 0.04). This was consistent with Bin Abdulrahman et al. [ 20 ], who indicated that the highest motivation was self-fulfillment and satisfying family dreams, followed by a high educational level, aspirations to join a high-quality residency program, and high income. Their study also found that few students were motivated by the desire to be regarded as unique students. We hypothesize that this probably goes back to human nature, where a highly rewarding incentive becomes the driving force of our work. Hence, schools should utilize this finding in exploring ways to enhance students’ motivation toward learning.
The present study did not find a significant effect of previous exam results on academic performance (OR = 1.03, p = 0.88). However, some studies reported that more than half of the high-achieving students admitted that high scores acquired on previous assessments are an important motivational factor [ 15 , 25 , 26 ]. We hypothesize that as students score higher marks, they become pleased and feel confident with their study approach. This finding shows how positive measurable results influence the students’ mentality.
The present study also explored the social environment surrounding medical students. The results indicated that those who were supported by their friends or family were slightly more likely to score higher GPAs (OR = 1.1, p = 0.73); however, the results did not reach a statistical significance. We hypothesize that a supportive and understanding environment would push the students to be patient and look for a brighter future. Our study results were consistent with previous published studies, which showed an association [ 3 , 27 , 28 , 29 , 30 ]. We hypothesize that students who spend most of their time with their families had less time to study, which made their study time more valuable. The findings of this study will hopefully raise awareness concerning the precious time that students have each day.
The association of different study habits among medical students with high and low GPAs was also studied in our study. It was noted that the high-achieving students try to attend their lectures compared to the lower achievers. This was in line with the previous published studies, which showed that significant differences were observed between the two groups regarding the attendance of lectures, tutorials, practical sessions, and clinical teachings [ 31 , 32 ]. The present study found that most students prefer to study alone, regardless of their level of academic achievement (82.1%). This finding is consistent with the study by Khalid A Bin Abdulrahman et al., which also showed that most students, regardless of their GPA, favored studying alone [ 20 ].
The present study findings suggest that a small number of students (29.8%) prefer to walk or stand while studying rather than sit, with most being high achievers (OR = 1.57, P = 0.15). A study reported that 40.3% of students with high GPAs seemed to favor a certain posture or body position, such as sitting or lying on the floor [ 15 ]. These contradictory findings might indicate that which position to adopt while studying should come down to personal preference and what feels most comfortable to each student. The present study also found that high achievers are more likely to prefer studying early in the morning (OR = 1.3, P = 0.28). The authors did not find similar studies investigating this same association in the literature. However, mornings might allow for more focused studying with fewer distractions, which has been shown to be associated with higher achievement in medical students [ 3 , 15 , 33 ].
Our study also found that 39.5% of the academically successful students reviewed pre-work or went through the material before they were taught it (OR = 1.08, p = 0.75), and 25% were neutral. Similar findings were reported in other studies, showing that academically successful students prepared themselves by doing their pre-work, watching videos, and revising slides [ 3 , 9 , 34 ]. Our study showed that 75% of high-achieving students tend to listen attentively to the lecturer (OR = 1.2, p = 0.48). Al Shawa et al. found no significant differences between the high achievers and low achievers when talking about attending lectures [ 15 ]. This could be due to the quality of teachers and the environment of the college or university.
Regarding the relationship between memorizing and revising with high and low GPA students, the present study found that students who study lectures daily are more likely to score higher than those who do not (OR = 1.4, p = 0.16). This finding is consistent with other studies [ 3 , 19 , 35 ]. For skimming lectures beforehand, an appreciable agreement was noted by high GPA students (62.1%), while only (42%) of low GPA students agreed to it. Similarly, previous published studies also found that highlighting and reading the content before memorization were both common among high-achieving students [ 15 , 36 ]. Furthermore, the present study has found recalling what has just been memorized to be statistically significantly associated with high GPA students (OR = 1.83, p = 0.05). Interestingly, we could not find any study that investigated this as an important factor, which could be justified by the high specificity of this question. Besides, when it comes to writing down/speaking out what has just been memorized, our study has found no recognizable differences between high-achieving students (75%) and low-achieving students (69%), as both categories had remarkably high percentages of reading and writing while studying.
The present study has found no statistical significance between regularly revising the lectures and high GPA ( p > 0.05), unlike the study conducted by Deborah A. Sleight et al. [ 37 ]. The difference in findings between our study and Deborah A. Sleight et al. might be due to a limitation of our study, namely the similar backgrounds of our participants. Another explanation could be related to curricular differences between the institutions where the two studies were conducted. Moreover, a statistically significant correlation between not preferring the data being presented in a written form instead of a graphical form and high GPA scores have been found in their study ( p < 0.05). However, a study conducted by Deborah A. Sleight et al. indicated that 66% of high achievers used notes prepared by other classmates compared to 84% of low achievers. Moreover, their study showed that only 59% of high achievers used tables and graphs prepared by others compared to 92% of low achievers. About 63% and 61% of the students in their study reported using self-made study aids for revision and memory aids, respectively [ 37 ].
The present study also examined the effects of smoking and chronic and mental illness, but found no statistical significance; the majority of both groups responded by denying these factors’ presence in their life. A similar finding by Al Shawwa et al. showed no statistical significance of smoking and caffeine consumption between low GPA and high GPA students [ 15 ]. We hypothesize that our findings occurred due to the study’s broad approach to examining such factors rather than delving deeper into them.
High-achieving students’ habits and factors contributing to their academic achievement were explored in the present study. High-achieving students were found to be more motivated and socially supported than their peers. Moreover, students who attended lectures, concentrated during lectures, studied early in the morning, prepared their weekly schedule, and studied more when exams approached were more likely to have high GPA scores. Studying techniques, including skimming before memorizing, writing what was memorized, active recall, and consistent revision, were adopted by high-achievers. To gain deeper insight into students’ strategies, it is recommended that qualitative semi-structured interviews be conducted to understand what distinguishes high-achieving students from their peers. Future studies should also explore differences between public and private university students. Additionally, further research is needed to confirm this study’s findings and provide guidance to all students. Future studies should collect a larger sample size from a variety of universities in order to increase generalizability.
Limitations and recommendations
The present study has some limitations. All the study’s findings indicated possible associations rather than causation; hence, the reader should approach the results of this study with caution. We recommend in-depth longitudinal studies to provide more insight into the different study habits and their impact on academic performance. Another limitation is that the research team created a self-reported questionnaire to address the study objectives, which carries a potential risk of bias. Hence, we recommend conducting interviews and having personal encounters with the study’s participants to reduce the risk of bias and better understand how different factors affect their academic achievement. A third limitation is that the research team only used the GPA scores as indicators of academic achievement. We recommend conducting other studies and investigating factors that cannot be solely reflected by the GPA, such as the student’s clinical performance and skills. Lastly, all participants included in the study share one background and live in the same environment. Therefore, the study’s findings do not necessarily apply to students who do not belong to such a geographic area and point in time. We recommend that future studies consider the sociodemographic and socioeconomic variations that exist among the universities in Saudi Arabia.
Availability of data materials
The datasets used and/or analyzed during the current study are available from the corresponding author on reasonable request.
Abbreviations
Grade Point Average
King Saud University
Institutional review board
Statistical package for the social sciences
Jafari H, Aghaei A, Khatony A. Relationship between study habits and academic achievement in students of medical sciences in Kermanshah-Iran. Adv Med Educ Pract. 2019;10:637–43.
Article Google Scholar
Abid N, Aslam S, Alghamdi AA, Kumar T. Relationships among students’ reading habits, study skills, and academic achievement in English at the secondary level. Front Psychol. 2023;14:1020269.
Abdulghani HM, Al-Drees AA, Khalil MS, Ahmad F, Ponnamperuma GG, Amin Z. What factors determine academic achievement in high achieving undergraduate medical students? A qualitative study. Med Teach. 2014;36(Suppl 1):S43–48.
Muntean LM, Nireștean A, Sima-Comaniciu A, Mărușteri M, Zăgan CA, Lukacs E. The relationship between personality, motivation and academic performance at Medical students from Romania. Int J Environ Res Public Health 2022, 19(15).
Reza HM, Alireza HJIJME. Investigating study Habits of Library and Information Sciences Students of Isfahan University and Isfahan University of Medical Sciences. 2014, 14:751–757.
Kurtz SM, Silverman JD. The Calgary-Cambridge Referenced Observation guides: an aid to defining the curriculum and organizing the teaching in communication training programmes. Med Educ. 1996;30(2):83–9.
Pun J, Kong B. An exploratory study of communication training for Chinese medicine practitioners in Hong Kong to integrate patients’ conventional medical history. BMC Complement Med Ther. 2023;23(1):10.
İlçin N, Tomruk M, Yeşilyaprak SS, Karadibak D, Savcı S. The relationship between learning styles and academic performance in TURKISH physiotherapy students. BMC Med Educ. 2018;18(1):291.
McKeirnan KC, Colorafi K, Kim AP, Stewart AS, Remsberg CM, Vu M, Bray BS. Study behaviors Associated with Student pharmacists’ academic success in an active Classroom Pharmacy Curriculum. Am J Pharm Educ. 2020;84(7):ajpe7695.
Jouhari Z, Haghani F, Changiz T. Assessment of medical students’ learning and study strategies in self-regulated learning. J Adv Med Educ Professionalism. 2016;4(2):72–9.
Google Scholar
Proctor BE, Prevatt FF, Adams KSS, Reaser A, Petscher Y. Study skills profiles of normal-achieving and academically-struggling College students. J Coll Student Dev. 2006;47(1):37–51.
Kyauta AMASY, Garba HS. The role of guidance and counseling service on academic performance among students of umar suleiman college of education, Gashua, Yobe State, Nigeria. KIU J Humanit. 2017;2(2):59–66.
Eva KW, Bordage G, Campbell C, Galbraith R, Ginsburg S, Holmboe E, Regehr G. Towards a program of assessment for health professionals: from training into practice. Adv Health Sci Education: Theory Pract. 2016;21(4):897–913.
Curcio G, Ferrara M, De Gennaro L. Sleep loss, learning capacity and academic performance. Sleep Med Rev. 2006;10(5):323–37.
Al Shawwa L, Abulaban AA, Abulaban AA, Merdad A, Baghlaf S, Algethami A, Abu-Shanab J, Balkhoyor A. Factors potentially influencing academic performance among medical students. Adv Med Educ Pract. 2015;6:65–75.
Ibrahim NK, Baharoon BS, Banjar WF, Jar AA, Ashor RM, Aman AA, Al-Ahmadi JR. Mobile Phone Addiction and its relationship to Sleep Quality and Academic Achievement of Medical students at King Abdulaziz University, Jeddah, Saudi Arabia. J Res Health Sci. 2018;18(3):e00420.
Alkhalaf AM, Tekian A, Park YS. The impact of WhatsApp use on academic achievement among Saudi medical students. Med Teach. 2018;40(sup1):S10–4.
Bonsaksen T, Brown T, Lim HB, Fong K. Approaches to studying predict academic performance in undergraduate occupational therapy students: a cross-cultural study. BMC Med Educ. 2017;17(1):76.
Alzahrani HA, Alzahrani OH. Learning strategies of medical students in the surgery department, Jeddah, Saudi Arabia. Adv Med Educ Pract. 2012;3:79–87.
Bin Abdulrahman KA, Khalaf AM, Bin Abbas FB, Alanazi OT. Study habits of highly effective medical students. Adv Med Educ Pract. 2021;12:627–33.
Jameel T, Gazzaz ZJ, Baig M, Tashkandi JM, Alharenth NS, Butt NS, Shafique A, Iftikhar R. Medical students’ preferences towards learning resources and their study habits at King Abdulaziz University, Jeddah, Saudi Arabia. BMC Res Notes. 2019;12(1):30.
Abdulghani HM, Alrowais NA, Bin-Saad NS, Al-Subaie NM, Haji AM, Alhaqwi AI. Sleep disorder among medical students: relationship to their academic performance. Med Teach. 2012;34(Suppl 1):S37–41.
Hwang G-J, Wang S-Y, Lai C-L. Effects of a social regulation-based online learning framework on students’ learning achievements and behaviors in mathematics. Comput Educ. 2021;160:104031.
Gamage KAA, Dehideniya D, Ekanayake SY. The role of personal values in learning approaches and student achievements. Behav Sci (Basel Switzerland) 2021, 11(7).
Linn Z, Tashiro Y, Morio K, Hori H. Peer evaluations of group work in different years of medical school and academic achievement: how are they related? BMC Med Educ. 2022;22(1):102.
Avonts M, Michels NR, Bombeke K, Hens N, Coenen S, Vanderveken OM, De Winter BY. Does peer teaching improve academic results and competencies during medical school? A mixed methods study. BMC Med Educ. 2022;22(1):431.
Topor DR, Keane SP, Shelton TL, Calkins SD. Parent involvement and student academic performance: a multiple mediational analysis. J Prev Interv Community. 2010;38(3):183–97.
Veas A, Castejón JL, Miñano P, Gilar-Corbí R. Relationship between parent involvement and academic achievement through metacognitive strategies: a multiple multilevel mediation analysis. Br J Educ Psychol. 2019;89(2):393–411.
Núñez JC, Regueiro B, Suárez N, Piñeiro I, Rodicio ML, Valle A. Student Perception of teacher and parent involvement in Homework and Student Engagement: the mediating role of motivation. Front Psychol. 2019;10:1384.
Abdulghani AH, Ahmad T, Abdulghani HM. The impact of COVID-19 pandemic on anxiety and depression among physical therapists in Saudi Arabia: a cross-sectional study. BMC Med Educ. 2022;22(1):751.
Park KH, Park JH, Kim S, Rhee JA, Kim JH, Ahn YJ, Han JJ, Suh DJ. Students’ perception of the educational environment of medical schools in Korea: findings from a nationwide survey. Korean J Med Educ. 2015;27(2):117–30.
Ahrberg K, Dresler M, Niedermaier S, Steiger A, Genzel L. The interaction between sleep quality and academic performance. J Psychiatr Res. 2012;46(12):1618–22.
Dikker S, Haegens S, Bevilacqua D, Davidesco I, Wan L, Kaggen L, McClintock J, Chaloner K, Ding M, West T, et al. Morning brain: real-world neural evidence that high school class times matter. Soc Cognit Affect Neurosci. 2020;15(11):1193–202.
Pittenger AL, Dimitropoulos E, Foag J, Bishop D, Panizza S, Bishop JR. Closing the Classroom Theory to practice gap by simulating a Psychiatric Pharmacy Practice Experience. Am J Pharm Educ. 2019;83(10):7276.
Walck-Shannon EM, Rowell SF, Frey RF. To what extent do Study habits relate to performance? CBE Life Sci Educ. 2021;20(1):ar6.
Abdulghani HM, Alanazi K, Alotaibi R, Alsubeeh NA, Ahmad T, Haque S. Prevalence of potential dropout thoughts and their influential factors among Saudi Medical Students. 2023, 13(1):21582440221146966.
Sleight DA, Mavis BE. Study skills and academic performance among second-Year Medical students in Problem-based learning. Med Educ Online. 2006;11(1):4599.
Download references
Acknowledgements
The authors are grateful to the Deanship of Scientific Research, King Saud University, for.
support through the Vice Deanship of Scientific Research Chairs.
Author information
Authors and affiliations.
Department of Psychiatry, College of Medicine, King Saud University, Riyadh, Saudi Arabia
Mohammed A. Aljaffer & Ahmad H. Almadani
College of Medicine, King Saud University, Riyadh, Saudi Arabia
Abdullah S. AlDughaither, Ali A. Basfar, Saad M. AlGhadir, Yahya A. AlGhamdi, Bassam N. AlHubaysh & Osamah A. AlMayouf
Department of Psychiatry, College of Medicine, Imam Mohammad Ibn Saud Islamic University, Riyadh, Saudi Arabia
Saleh A. AlGhamdi
Department of Medical Education, College of Medicine, King Saud University, P.O. Box: 230155, Riyadh, 11321, Saudi Arabia
Tauseef Ahmad
Department of Medical Education and Family Medicine, College of Medicine, King Saud University, Riyadh, Saudi Arabia
Hamza M. Abdulghani
You can also search for this author in PubMed Google Scholar
Contributions
Conception or design: AHA, MAA, and HMA. Acquisition, analysis, or interpretation of data: AAB, SMA, ASA, YAA, BNA, OAA and SAA. Drafting the work or revising: TA, AHA, ASA AAB. Final approval of the manuscript: MAA, HMA., AHA, and TA. The author(s) read and approved the final manuscript.
Corresponding author
Correspondence to Tauseef Ahmad .
Ethics declarations
Conflict of interest.
The Authors declare that they have no conflict of interest.
Consent for publication
Not applicable.
Competing interests
The authors declare no competing interests.
Human ethics and consent to participate declarations
Additional information, publisher’s note.
Springer Nature remains neutral with regard to jurisdictional claims in published maps and institutional affiliations.
Rights and permissions
Open Access This article is licensed under a Creative Commons Attribution-NonCommercial-NoDerivatives 4.0 International License, which permits any non-commercial use, sharing, distribution and reproduction in any medium or format, as long as you give appropriate credit to the original author(s) and the source, provide a link to the Creative Commons licence, and indicate if you modified the licensed material. You do not have permission under this licence to share adapted material derived from this article or parts of it. The images or other third party material in this article are included in the article’s Creative Commons licence, unless indicated otherwise in a credit line to the material. If material is not included in the article’s Creative Commons licence and your intended use is not permitted by statutory regulation or exceeds the permitted use, you will need to obtain permission directly from the copyright holder. To view a copy of this licence, visit http://creativecommons.org/licenses/by-nc-nd/4.0/ .
Reprints and permissions
About this article
Cite this article.
Aljaffer, M.A., Almadani, A.H., AlDughaither, A.S. et al. The impact of study habits and personal factors on the academic achievement performances of medical students. BMC Med Educ 24 , 888 (2024). https://doi.org/10.1186/s12909-024-05889-y
Download citation
Received : 26 September 2023
Accepted : 12 August 2024
Published : 19 August 2024
DOI : https://doi.org/10.1186/s12909-024-05889-y
Share this article
Anyone you share the following link with will be able to read this content:
Sorry, a shareable link is not currently available for this article.
Provided by the Springer Nature SharedIt content-sharing initiative
- Medical students
- Study habits
- Academic achievement
- Saudi Arabia
BMC Medical Education
ISSN: 1472-6920
- General enquiries: [email protected]
This paper is in the following e-collection/theme issue:
Published on 26.8.2024 in Vol 26 (2024)
Sociotechnical Cross-Country Analysis of Contextual Factors That Impact Patients’ Access to Electronic Health Records in 4 European Countries: Framework Evaluation Study
Authors of this article:

Original Paper
- Jonas Moll 1 , PhD ;
- Isabella Scandurra 1 † , PhD ;
- Annika Bärkås 2, 3 , PhD ;
- Charlotte Blease 2, 4 , PhD ;
- Maria Hägglund 2, 3 , PhD ;
- Iiris Hörhammer 5 , DSC ;
- Bridget Kane 2, 6 , PhD ;
- Eli Kristiansen 7 , ME ;
- Peeter Ross 8, 9 , MD, PhD ;
- Rose-Mharie Åhlfeldt 10 , PhD ;
- Gunnar O Klein 1 , MD, PhD
1 Centre for Empirical Research on Information systems, School of Business, Örebro University, Örebro, Sweden
2 Participatory eHealth and Health Data Research Group, Department of Women's and Children's Health, Uppsala University, Uppsala, Sweden
3 Medtech Science & Innovation Centre, Uppsala University Hospital, Uppsala, Sweden
4 Digital Psychiatry, Department of Psychiatry Beth Israel Deaconess Medical Center, Harvard Medical School, Boston, MA, United States
5 Department of Computer Science, Aalto University, Espoo, Finland
6 Karlstad University Business School, Karlstad, Sweden
7 Norwegian Centre for E-health Research, University Hospital of North Norway, Tromsø, Norway
8 E-Medicine Centre, Department of Health Technologies, Tallinn University of Technology, Tallinn, Estonia
9 Research Department, East Tallinn Central Hospital, Tallinn, Estonia
10 School of Informatics, University of Skövde, Skövde, Sweden
Corresponding Author:
Jonas Moll, PhD
Centre for Empirical Research on Information systems
School of Business
Örebro University
Nova building, 4th floor
Fakultetsgatan 1
Örebro, 70182
Phone: 46 0703174383
Email: [email protected]
Background: The NORDeHEALTH project studies patient-accessible electronic health records (PAEHRs) in Estonia, Finland, Norway, and Sweden. Such country comparisons require an analysis of the sociotechnical context of these services. Although sociotechnical analyses of PAEHR services have been carried out in the past, a framework specifically tailored to in-depth cross-country analysis has not been developed.
Objective: This study aims to develop and evaluate a method for a sociotechnical analysis of PAEHRs that advances a framework for sociotechnical analysis of eHealth solutions first presented by Sittig and Singh. This first article in a series presents the development of the method and a cross-country comparison of the contextual factors that enable PAEHR access and use.
Methods: The dimensions of the framework for sociotechnical analysis were thoroughly discussed and extended in a series of workshops with international stakeholders, all being eHealth researchers focusing on PAEHRs. All countries were represented in the working group to make sure that important national perspectives were covered. A spreadsheet with relevant questions related to the studied services and the various dimensions of the sociotechnical framework was constructed and distributed to the 4 participating countries, and the project participants researched various national sources to provide the relevant data for the comparisons in the 10 sociotechnical dimensions.
Results: In total, 3 dimensions were added to the methodology of Sittig and Singh to separate clinical content from features and functions of PAEHRs and demonstrate basic characteristics of the different countries regarding national and regional steering of health care and information and communications technology developments. The final framework contained the following dimensions: metadata; hardware and software computing infrastructure; features and functions; clinical content shared with patients; human-computer interface; people; workflow and communication; the health care organization’s internal policies, procedures, and culture; national rules, regulations, and incentives; system measurement and monitoring; and health care system context. The dimensions added during the study mostly concerned background information needed for cross-country comparisons in particular. Several similarities were identified among the compared countries, especially regarding hardware and software computing infrastructure. All countries had, for example, one national access point, and patients are provided a PAEHR automatically. Most of the differences could be identified in the health care system context dimension. One important difference concerned the governing of information and communications technology development, where different levels (state, region, and municipality) were responsible in different countries.
Conclusions: This is the first large-scale international sociotechnical analysis of services for patients to access their electronic health records; this study compared services in Estonia, Finland, Norway, and Sweden. A methodology for such an analysis was developed and is presented to enable comparison studies in other national contexts to enable future implementations and evaluations of PAEHRs.
Introduction
Patients’ web-based access to their electronic health records (EHRs) is increasingly being implemented internationally [ 1 - 3 ], and a growing body of literature indicates strong benefits to patients, including understanding their care plans better [ 4 ], feeling more in control of their care [ 4 , 5 ], being better informed about their medication [ 6 ], improved communication with and trust in their clinicians [ 5 , 7 ], and improved patient safety [ 8 ].
Patient-accessible EHRs (PAEHRs) are web-based services providing patients with secure access to view and sometimes edit or comment on their EHRs made available by their health care providers [ 9 ]. A PAEHR is directly linked to the EHRs, which are shared patient records entered and maintained by health care service providers and contain historical data about a patient [ 10 ]. Medical and health data and information in the EHR are created and managed by authorized providers in a digital format capable of being shared with other providers across more than one health care organization [ 11 ]. In addition, a PAEHR may include access to the services supporting a person’s access to health care services (eg, e-booking and e-consultation) and to evidence-based tools (eg, patient guidelines, educational materials, and reimbursement information). It is important to distinguish between PAEHRs and personal health records—the latter being a health record that the patients themselves control and maintain to track their own health, for example, on paper; in Microsoft Excel sheets; or, predominantly, in health care apps. Personal health records are external applications that can potentially be linked with EHRs to enable sharing [ 10 ].
In this study, we focused on national services in the 4 countries of Estonia, Finland, Norway, and Sweden and excluded web-based services designed to provide access to the services of only 1 health care provider. Among the studied countries, Estonia was the first to offer citizens web-based access to their EHR through the service Digilugu that was launched nationwide in 2008 [ 12 ]. The Finnish counterpart, Omakanta, was launched nationwide in 2010 with only limited functionality and reached full functionality in 2015 after a step-by-step adoption of functions [ 13 ]. In Sweden, the national PAEHR service Journalen was launched in the Region Uppsala in 2012 and has been accessible to all citizens since 2018 [ 14 ]. In Norway, the national PAEHR service Helsenorge was first launched in 1 of 4 regions in 2015, and as of 2023, citizens in 3 out of 4 regions can use the service to access their PAEHRs [ 15 ].
Despite the reported benefits of PAEHRs and patients’ web-based record access, implementation is often slow and challenging, and the complexity of health care systems and technical infrastructure leads to great diversity in, for example, the information to which patients are given access and when they can access it across regions and health care settings. Similarly, patients’ adoption and use of PAEHRs also varies across contexts. To understand why these differences exist and better adapt the design and implementation of PAEHRs to a specific context, there is a need for a more fine-grained understanding of the social and technical underpinnings of this innovation.
Sociotechnical Systems
As described by Baxter and Sommerville [ 16 ], the problems that arise when designing and implementing complex IT systems are not just technical, engineering problems. These systems are developed and operated by people working in organizations that inevitably have different, often conflicting goals and views on the role and design of the system. The IT system is part of a broader “sociotechnical” system, and to understand success factors and barriers to implementing web-based record access and identify best practices and guidelines, there is a need to approach these eHealth services as complex sociotechnical systems.
Sittig and Singh [ 17 ] have proposed a multidimensional sociotechnical framework in which any health IT innovation, intervention, application, or device implemented within a complex adaptive health care system can be studied. The sociotechnical framework by Sittig and Singh [ 17 ] identifies eight dimensions of sociotechnical systems in health care that need to be considered in both development and evaluation: (1) hardware and software computing infrastructure; (2) clinical content; (3) human-computer interface; (4) people; (5) workflow and communication; (6) internal organizational policies, procedures, and culture; (7) external rules, regulations, and pressures; and (8) system measurement and monitoring [ 17 ]. The framework breaks down components of the technology to enable researchers to identify specific problems with implementation. It also includes monitoring processes and government structures that need to be in place for the system to achieve its goals. The interrelatedness of the components makes the framework pertinent when eHealth technologies and users are at the core of the investigation.
In 2017, Hägglund and Scandurra [ 18 ] began analyzing the Swedish PAEHR system from a sociotechnical perspective using the framework by Sittig and Singh [ 17 ], and their results laid the foundation for continued work within the NORDeHEALTH research project [ 19 ] involving partners from Estonia, Finland, Norway, and Sweden. These countries were deemed appropriate for the development and first test of the cross-country sociotechnical analysis method derived in this study as they have comparable government and health care structures. In addition, they have all reached maturity in the use of their respective PAEHR systems—these countries are all among the early adopters of this specific type of eHealth service for patients. In a 2021 workshop, a first draft of an extended version of the framework by Sittig and Singh [ 17 ] as a sociotechnical template for analyzing PAEHR implementations was introduced and discussed [ 20 ]. However, the original framework by Sittig and Singh [ 17 ] needed to be more specifically adapted to PAEHRs and their contexts to enable useful cross-country comparisons, a process that will be described further in this paper.
The full results of the sociotechnical analysis of the 4 countries involved in the NORDeHEALTH project are being published in an article series; it is intended for each publication to offer results related to specific and related framework dimensions and, thereby, focus on different themes. This paper is intended to offer a detailed description of the overarching research method as well as a sociotechnical analysis of dimensions providing the context for other articles of the series.
Aims and Research Questions
The aims of this study were to (1) develop a detailed collaborative method suitable for cross-country sociotechnical analyses of PAEHRs and (2) use the data collection to compare contextual factors that enable PAEHR access and use.
Although the proposed work process, including the production of an extensive sociotechnical analysis template, is described in the context of comparing PAEHRs in 4 specific countries, it can be adjusted to cover other eHealth services for patients across other nations. The comparison and discussion regarding the national contexts will provide a robust foundation and understanding of other parts of the sociotechnical analysis, such as included features and national and regional incentives for the use and promotion of PAEHRs. The following research questions (RQs) guided the work presented in this paper:
- RQ 1: how can a sociotechnical framework for health IT be adapted and a collaborative method be developed that is suitable for cross-country sociotechnical analyses of PAEHR services?
- RQ 2: how can the method be used to compare the PAEHRs in Estonia, Finland, Norway, and Sweden?
Research Method
This study has 2 main parts inspired by different phases in the design science methodology [ 21 ]. In the first part, a collaborative method, including a data collection template, for sociotechnical cross-country analysis of PAEHRs was derived: (1) problem identification and motivation; that is, based on earlier experiences with the framework by Sittig and Singh [ 17 ] and the fact that the framework is not adapted for comparisons across contexts, the following problem was defined, how can we conduct fruitful comparative analysis among PAEHRs in different regions and health care settings? (2) objectives of a solution; that is, due to the identified need to perform a complete sociotechnical comparison among health care contexts, the main objective of the new artifact (being a method for collecting data, including a refined framework and a data collection instrument) is to enable a comparison that would consider the sociotechnical contexts of PAEHRs; and (3) design and development; that is, a dedicated collaborative method was developed with a focus on adjusting the framework for cross-country comparisons and deriving a data collection template enabling the collection of all relevant data from the specific countries involved.
The second part of our study (in this paper referred to as data collection ) consists of the collection and comparison of the sociotechnical characteristics of the 4 countries participating in the NORDeHEALTH project: Estonia, Finland, Norway, and Sweden. This can be seen as case studies of the more general questions regarding the availability and use of the PAEHR in a country. However, it is also an evaluation of the method as an artifact in the design science paradigm: (1) demonstration—the data collection template derived from the first part of the study was used to conduct a cross-country comparison among 4 Nordic countries—and (2) evaluation—finally, the results from the comparison were analyzed to evaluate whether the approach provides an understanding that explains differences among countries in the adoption of PAEHRs by regions and providers and patients.
In the remaining sections, the different points of the process are elaborated on, with emphasis on the design and development, demonstration, and evaluation steps. The work was led by a core analysis team (JM, IS, GK, and AB) of health informatics researchers.
Development of the Collaborative Method
The development of the data collection instrument based on the sociotechnical framework proposed by Sittig and Singh [ 17 ] started before the NORDeHEALTH project had begun.
In 2017, a first sociotechnical analysis of the Swedish PAEHR service Journalen was conducted with the aim of increasing the understanding of factors that influence the design, implementation, adoption, and use of the service [ 18 ]. However, the analysis did not go into the details of each dimension of the framework but, rather, highlighted some overall challenges. The results of this early analysis were used as input to an international workshop held in 2021, for which a more detailed template specifically adapted to PAEHRs was developed ( Textbox 1 ). In addition to including specific PAEHR-related questions to each dimension, a new dimension, Features and functions , was added. Some other dimensions were renamed. During the workshop, international experts provided feedback on the template that was incorporated into further refinements, leading to the data collection form presented in this study.
Dimension and description
- Hardware and software computing infrastructure: focuses only on the hardware and software required to run the applications.
- Features and functions: important features and functions in the patient-accessible electronic health record (PAEHR) service or in related services. As there is not yet a strict definition of what functionality is included in a PAEHR or not, we included functions that may be considered external to a PAEHR in some contexts.
- Clinical content shared with patients: includes everything on the data-information-knowledge continuum that is stored in the PAEHR service and made accessible to patients.
- Human-computer interface: focuses on the usability of the PAEHR service.
- People: represents the humans involved in all aspects of the implementation and use of the eHealth application and how they experience the use.
- Workflow and communication: focuses on collaboration and communication among different users and assessing how well the eHealth application supports the current clinical workflow.
- The health care organization’s internal policies, procedures, and culture: affects every other dimension in this model as it includes any internal IT policy documents and managerial procedures that may influence the implementation and use of eHealth.
- National rules, regulations, and incentives: focuses on external forces that facilitate or place constraints on the design, development, implementation, use, and evaluation of eHealth in the respective clinical settings.
- System measurement and monitoring: focuses on the need for an effective system measurement and monitoring program to identify the availability of features and functions and how they are used as well as expected outcomes and unintended consequences of the PAEHR service.
The resulting Microsoft Excel template was then used as a basis for developing a complete data collection form within the scope of the NORDeHEALTH project, including questions, response options, and comment sections for all the dimensions in the sociotechnical analysis framework used. The first version of the form was developed by the core analysis team. The sociotechnical dimensions were not changed by the team, but the added “Features and functions” dimension was retained, and the questions used in the Microsoft Excel template were clarified in many cases. The work process followed a weekly workshop format in which different framework dimensions were in focus and that lasted between February 2021 and April 2021. The draft template took form within a shared Google document, enabling collaborators in the participating countries to continuously offer feedback on the ongoing work. This format ensured that the items in the data collection form were relevant in the context of all involved countries. Finally, an analysis dimension called metadata was added during this iterative process, whereby information about the data collection itself—including the name of the system, the name of the researcher responsible for data collection, and information sources—could be noted.
After the first complete draft had been developed, a digital workshop was held in early May 2021 in which the core analysis team from Sweden (n=4) as well as representatives from Estonia (n=1), Finland (n=1), and Norway (n=2) participated. All participants are coauthors of this paper and are health informatics researchers with several years of experience following the implementation and subsequent use of the PAEHR system in their respective countries. The focus of the workshop was to discuss how to interpret the framework dimensions and uppermost to elaborate on and resolve some of the question formulations that had elicited many comments from other project partners in the shared document. After the 1-hour workshop, the template was refined, after which input was again sought from other project partners through email. After the last questions had been resolved, in October 2021, data collection could start based on the finalized template.
Data Collection
Data collection was undertaken in 2 different phases. The first, longer phase started in October 2021 and ended in November 2021. One representative from each country, who also took part in the workshop where the data collection template was discussed, was assigned to be the main responsible data collector from their country, and communication among these representatives occurred continuously during the data collection period. Project participants from each country filled out a copy of the data collection form ( Multimedia Appendix 1 ) for their main PAEHR systems and with the systems shown in Table 1 in focus.
Country | System |
Sweden | 1177.se and Journalen [ ] |
Estonia | Digilugu [ ] |
Finland | Omakanta [ ] |
Norway | Helsenorge [ ] |
Some ambiguities were identified during the data collection, and these were handled through communication among the responsible researchers. When needed, the data collection master file was updated in accordance with agreed-upon solutions while making sure that everyone always used the question formulations in the latest version. After completion, all main responsible researchers from each country shared their filled-out forms in a shared folder for everyone to access.
After a preliminary walk-through of the collected data performed by the core analysis team, a need for further clarification was identified. In some cases, a few questions remained unanswered, and in other cases, the level of detail varied, requiring follow-up questions. When questions that the researchers from each country needed to elaborate on had been compiled, an additional and final data collection took place in December 2021. This shorter phase of data collection took place through email exchange between the leader of the core analysis team (JM) and the researchers responsible for data collection in each country. Multimedia Appendix 2 summarizes the sources of information that were used during the data collection in each country.
As a last step of data collection for this particular paper, a project representative from each involved country was tasked with collecting and summarizing information to enable a broader overview of national contexts than the developed data collection form could provide. In this final stage, information related to government structure, overall health care system, digital care organization, and steering of health information and communications technology (ICT) developments was gathered from each country. Information was gathered from national statistics, agency web pages, and local contacts within each health care system. Data collection for this study ended in November 2022.
When data collection was completed, the core analysis team began the analysis work. The first step was to copy all answers from the completed data collection forms into a shared analysis document. In this document, each dimension had its own sheet to simplify comparisons within each dimension. In each sheet, the questions from the data collection form were added as rows, and each PAEHR system was added as a column to create a matrix where each column included the answers related to a specific PAEHR system. Each answer, possibly in combination with an additional comment, was added to the corresponding cells in the matrix. Some examples are shown in the Results section. The content of the sheets was then compared across columns (countries) for all questions (rows) to identify similarities as well as aspects that are unique to the specific countries involved. In this step, representatives from Estonia, Finland, Norway, and Sweden were once again invited to discuss the identified similarities and differences.
Ethical Considerations
The study presented in this paper is part of the larger NORDeHEALTH project, which received ethics approval from the appropriate national ethical bodies. This particular study did not involve human participants or sensitive personal data and only focused on contextual and technical details of PAEHRs; there were no specific ethical requirements that needed to be addressed.
Final Data Collection Instrument
The final data collection form inspired by the sociotechnical dimensions in the framework developed by Sittig and Singh [ 17 ], including all updates made during the initial data collection round, can be found in Multimedia Appendix 1 . Table 2 describes the overall content and structure of the form. The data collection form is the end result of the method development and is intended to enable sociotechnical comparisons of eHealth services for patients across countries, with a specific aim to compare PAEHR systems. It is important to note here that the form derived for this study is specifically aimed at comparison of PAEHR systems. For other types of eHealth services for patients, such as self-tracking applications, a different form may have to be derived through the process suggested in the Methods section.
Framework dimension | General description | Questions |
Metadata | Information related to the data collection itself, such as the organizations and persons that are responsible for the data collection in the other dimensions, as well as the geographical and organizational context of the providers of PAEHRs in the country | 1.1-1.6 |
Hardware and software computing infrastructure | The hardware and software required to run the applications; this also includes issues regarding information security, access control, and standards used | 2.1-2.8 |
Features and functions | Important features and functions in the PAEHR service or in related services; some functions that may be considered external to a PAEHR in some contexts have been included, such as appointment booking, requesting renewal of prescriptions, and access to logs | 3.1-3.14 |
Clinical content shared with patients | An inventory of which parts of a professional record are shared with the patients; examples are medications, laboratory test results of various kinds, images, and text notes | 4.1-4.21 |
Human-computer interface | The “Human-computer interface” dimension captures information on studies conducted on the usability of the PAEHR service; it includes both the variables that have been measured and the results of measures from different stakeholders’ perspectives | 5.1-5.10 |
People | “People” includes an overview of various characteristics of the population in the country, including language groups, educational levels, and internet use; it also includes user demographics of the PAEHR system | 6.1-6.6 |
Workflow and communication | This dimension captures information focusing on collaboration and communication between health care professionals and patients and the explicit role of the PAEHR if identified and promoted in the country, possibly for distinct patient groups | 7.1-7.11 |
The health care organization’s internal policies, procedures, and culture | An inventory of internal IT policy documents and managerial procedures that may influence the implementation and use of the PAEHR | 8.1-8.6 |
National rules, regulations, and incentives | An inventory of national regulations that may facilitate or place constraints on the design, development, and implementation of the PAEHR | 9.1-9.9 |
System measurement and monitoring | Collection of information on existing system measurement and monitoring programs to identify the use of the PAEHR system as well as individual functions; this also includes unintended consequences of the PAEHR service and other feedback to the national systems | 10.1-10.5 |
Health care system context | The general context information about the health care system in the specific country where data are collected is recorded in this dimension; information about governance structure and EU membership as well as primary care organization and financing of health care is included in this dimension, and it is especially important if one performs comparisons across several countries | 11.1-11.4 |
a The questions refer to the numbering in Multimedia Appendix 1 .
b PAEHR: patient-accessible electronic health record.
c EU: European Union.
During the work process, some of the dimensions by Sittig and Singh [ 17 ] were adjusted, and some dimensions were added in response to identified needs that were not fulfilled by the original framework. The following dimensions were either updated or added ( Table 2 ):
- Metadata: this dimension was added during the work process to enable collection of information related to the data collection process itself. It stores general information that is important for keeping track, especially in large-scale projects.
- Features and functions: we decided to use the existing framework dimension Clinical content (after renaming it—see the following item on this list) to describe the information to which patients or citizens are given access through the PAEHR (eg, laboratory test results, medications, and clinical notes). However, there is also variation in the functions that PAEHRs provide (eg, whether patients can comment or fill out forms). Considering the importance of these types of differences, we determined that a new dimension was warranted. Thus, this dimension ensured that the focus was not only on the clinical information that patients have access to in the PAEHR but also on the other important functions or features that they can use.
- Clinical content shared with patients: in the original framework, this dimension was called “Clinical content.” The dimension was renamed to make it more PAEHR specific.
- The health care organization’s internal policies, procedures, and culture: in the original framework, this dimension was more generally about policies, procedures, and culture. The decision was made to add “Health care organizations” as a specification of this dimension. This made it better suited for PAEHR analysis.
- National rules, regulations, and incentives: in the original framework, this dimension was named “External rules, regulations, and pressures.” The redefined dimension puts the focus more on the national context in relation to rules and regulations, again to make it more suitable for PAEHR analysis.
- Health care system context: this dimension was also added during the work process to understand the basics of the countries involved in the comparison. This understanding is needed when analyzing the data in the other dimensions as the national context, including health care system financing and steering of health ICT system development, has an effect on all the other dimensions. It is especially important to gather general data about the national context when comparing across several countries. This dimension was added to the final data collection form after the information had been collected in each country, and hence, it was added in response to a need that the data collection form used did not fulfill.
Identified Clusters of Dimensions
As the collected material is extensive, the dimensions were clustered to enable more manageable partitions of the data set. Dimensions that had similar focuses were grouped together. Textbox 2 presents the 4 resulting clusters. The Contextual factors enabling PAEHR access and use cluster gathers dimensions focusing on the user base as well as technical and governmental prerequisites for PAEHR access and use. The added Metadata dimension is also included in this cluster as it is a prerequisite for the type of comparative studies that we have conducted, and it also includes contextual information. The Features and content cluster includes dimensions focusing on what is actually offered to patients in the PAEHR, that is, the content (eg, clinical notes, test results, and images) and functions (eg, secure messaging and prescription renewal) that are provided to patients. The Evaluations of human-computer interaction and use cluster includes the dimensions focusing on how one measures and evaluates PAEHR use, as well as the results of such evaluations. Both internal service provider evaluations and external evaluations are included to provide a broad coverage. Finally, the National and local policies, regulations for use, promotion, workflow, and communication cluster includes dimensions focusing on laws and regulations; the focus in this cluster is also more on health care professionals and their relationship to PAEHRs than on the patients.
Contextual factors enabling patient-accessible electronic health record access and use
- Hardware and software computing infrastructure
- Health care system context
Features and content
- Features and functions
- Clinical content shared with patients
Evaluations of human-computer interaction and use
- Human-computer interface
- System measurement and monitoring
National and local policies, regulations for use, promotion, workflow, and communication
- Workflow and communication
- The health care organization’s internal policies, procedures, and culture
- National rules, regulations, and incentives
In the article series about the results of the sociotechnical analysis, each article focuses on different clusters from Textbox 2 . This paper has the Contextual factors enabling PAEHR access and use cluster in focus; hence, the dimensions belonging to this cluster were considered in detail, and the results of the data collection in relation to those dimensions are presented in the following sections.
Results From the Sociotechnical Comparison of the “Contextual Factors Enabling PAEHR Access and Use” Cluster
In this section, the results gathered by means of the derived sociotechnical data collection form, with a focus on contextual factors, are presented.
The metadata dimension, which was not part of the original sociotechnical framework presented by Sittig and Singh [ 17 ], includes basic information related to the data collection process itself and the system in question. Collected information about the PAEHR systems is presented in Table 3 . Differences can be observed regarding the type of provider responsible for sharing EHR services in each country. In Sweden, the responsible provider is a publicly owned company, Inera AB, whereas the responsible providers in the other countries are institutions or ministries. In Sweden, Finland, and Estonia, the PAEHR service is national; however, in Norway, the studied service, Helsenorge, is only used for PAEHRs in 3 of 4 regions. All countries only provide 1 national PAEHR service ( Table 3 ); however, local PAEHRs can exist in parallel with the national PAEHR. In all studied countries, health information from different sources is collected in a single PAEHR.
Sweden | Norway | Finland | Estonia | |
Name of the national PAEHR service | 1177 Journalen | Helsenorge | Omakanta | Digilugu |
Responsible provider | The Swedish eHealth organization Inera AB | The Norwegian Directorate of eHealth and Norwegian Health Network | The Finnish Social Insurance Institution (Kela) | The Estonian Ministry of Social Affairs (and the Health and Welfare Information Systems Centre and the National Institute for Health Development) |
Geographic area | National | 3 of 4 health regions in Norway | National | National |
Number of national PAEHRs that 1 patient can have | 1 | 1 | 1 | 1 |
a The items shown in the table correspond to questions 1.1 to 1.4 in the data collection form ( Multimedia Appendix 1 ). Question 1.6 regarding information sources is answered in Multimedia Appendix 2 .
c A total of 2 different Swedish systems (1177 and Journalen) were analyzed. As 1177 includes Journalen (which presents most of the PAEHR information) as well as some other related features, the results from the 2 systems are merged here. In cases in which, for example, the setup of Journalen differs from that of the rest of 1177, the differences will be highlighted.
People and Demographics
This dimension was more complex than the others covered in this paper as demographic data were not available from the same time intervals and age intervals in the different countries. In addition, some of the countries did not have user group statistics of the PAEHR available. Hence, a complete comparison across all countries cannot be made, and as a consequence, results will only be summarized at an overall level in this section.
Sweden has the highest number of inhabitants (10.2 million) of the countries compared, and Estonia has the smallest (1.3 million). Norway and Finland have 5.4 and 5.5 million inhabitants, respectively. Thus, there are large differences in population size among the countries. In addition, there are large differences regarding the proportion of immigrants in the countries, with the lowest proportion (9%) in Finland and the highest proportion (31% non-Estonians) in Estonia. In Norway and Sweden, the proportion is approximately 20%. Data on internet use among the populations of the 4 countries were available from 2019 in Finland and 2020 in Estonia, Norway, and Sweden. The data showed that a high proportion of the populations were internet users, with the highest number in Norway (98%) and the lowest in Estonia (89%). Data from all countries showed a general trend of increasing internet use. Estonia and Norway lack statistics on the use of PAEHRs in different user groups (such as age, gender, and profession). In Finland, statistics on PAEHR use in different age groups are collected, with the highest number of users being aged 36 to 50 years (94%) and 18 to 35 years (93%). In Sweden, data are collected for the PAEHR service Journalen when it comes to age intervals and gender. The service is used most frequently by individuals aged 20 to 29 years and 30 to 39 years, and slightly more female (53%) than male (47%) individuals use it. Educational levels are comparable across countries, with the vast majority of the population reaching upper secondary education. The highest proportion with at least 3 years of higher education can be found in Sweden (37%), and the lowest proportion can be found in Finland and Estonia (23%). No statistics from any of the countries could be found regarding the proportion of the population that prefers an interpreter in their contacts with health care.
Hardware and Software Computing Infrastructure
This dimension includes several important contextual factors that are presented in Table 4 . Among the compared countries, 2 different ways of storing data are represented. In Norway, Finland, and Sweden, data are stored in local EHRs and then extracted and presented in the PAEHRs at runtime. For Digilugu in Estonia, centralized storage is used. In most of the studied countries, data are provided to the PAEHR from both private and public providers and from both primary and secondary care. The exception is Helsenorge in Norway, where only secondary care provides data to the PAEHR. Although all health care personnel in Norway are obliged to provide data to the EHR, it is decided on a regional level whether these data should be electronically accessible in the PAEHR. There are clear similarities among the compared countries when it comes to access points, enrollment, and authentication—all countries use 1 national access point, and each patient of a connected provider automatically receives a PAEHR. Authentication in all countries is made possible through a national electronic ID. Web browsers as well as mobile-adapted web browsers can be used by patients in all studied countries to access the PAEHR. In Norway and Sweden, there is also the possibility of using apps for iOS or Android. There are also some differences regarding how data are provided to the PAEHR. In the case of 1177, Omakanta, and Helsenorge, data are automatically linked to the source EHRs at runtime when a patient logs in to the system. In Digilugu, on the other hand, data are either automatically uploaded from source EHRs to a central server or manually uploaded from the EHR by a health care professional. Hence, in Estonia but not in the other studied countries, it is possible for a health care professional to add content to the PAEHR specifically.
Sweden (Vårdguiden 1177) | Norway (Helsenorge) | Finland (Omakanta) | Estonia (Digilugu) | |
Centralized or distributed data storage? | Data stored in local EHRs | Data stored in local EHRs | Centralized storage | Centralized storage |
Who provides data to the PAEHR ? | Private and public providers and primary and secondary care | Public providers and secondary care | Private and public providers and primary and secondary care | Private and public providers and primary and secondary care |
One national access point per patient portal to the PAEHR? | Yes | Yes | Yes | Yes |
How is enrollment done? | Each patient of a provider automatically receives a PAEHR | Each patient of a provider automatically receives a PAEHR | Each patient of a provider automatically receives a PAEHR | Each patient of a provider automatically receives a PAEHR |
How are users authenticated? | Patients use a national electronic ID of some type | Patients use a national electronic ID of some type | Patients use a national electronic ID of some type | Patients use a national electronic ID of some type |
What technical platform can the patients use for access? | Web browser, mobile-adapted web browser, app for iOS, and app for Android | Web browser, mobile-adapted web browser, app for iOS, and app for Android | Web browser and mobile-adapted web browser | Web browser and mobile-adapted web browser |
How are data provided to the PAEHR? | Automatically linked to source EHRs at runtime | Automatically linked to source EHRs at runtime | Automatically linked to source EHRs at runtime | Automatically uploaded from source EHRs to a central server and manually uploaded from EHRs by a health professional |
Are international standards (eg, FHIR , openEHR, and ISO 13606) used in the interface between local EHRs and the PAEHR? | A national architecture for information services exists that defines information content that is not expressed in any international standard. However, many standards are used to build the integration. International terminologies are used for some aspects, such as for diagnosis and ATC for class of medicinal product. | IHE XDS and IHE plus national information structure standards | National profiles of information content expressed in various HL7 syntaxes—(HL7 version 3: CDA R2 and HL7 FHIR) plus a number of modern web-based service standards for exchange and information security | National profile of old-type HL7 standards, such as HL7 version 3 and HL7 CDA R2 combined with international terminologies such as , ATC, and LOINC |
a The questions correspond to questions 2.1 to 2.8 in the data collection template ( Multimedia Appendix 1 , where all response options can also be found).
b EHR: electronic health record.
c PAEHR: patient-accessible EHR.
d FHIR: Fast Healthcare Interoperability Resources.
e ISO: International Organization for Standardization.
f ICD-10: International Classification of Diseases, 10th Revision.
g ATC: The Anatomical Therapeutic Chemical Classification System.
h IHE: Integrating the Healthcare Enterprise.
i XDS: Cross Enterprise Document Sharing.
j HL7: Health Level Seven.
k CDA R2: Clinical Document Architecture Release 2.
l LOINC: Logical Observation Identifiers Names and Codes.
Health Care System Contexts
During the analysis of the collected data, it became clear that it is necessary to provide basic descriptions of the health care system contexts for readers who are unfamiliar with them. Therefore, a summary of the most essential information for understanding the PAEHR context is provided in Textbox 3 . The full data are provided in Multimedia Appendix 3 .
Governance structure
- A parliamentary republic, which is a member of the European Union (EU)
- There are 3 levels of government: state, regions (19), and municipalities (310)
- A parliamentary republic, which is a member of the EU
- There are 3 levels of government: state, counties (15), and municipalities (79)
- A constitutional monarchy, which is not a member of the EU (but a member of the European Economic Area)
- There are 3 levels of government: state, counties (11), and municipalities (356)
- A constitutional monarchy, which is a member of the EU
- There are 3 levels of government: state, regions (21), and municipalities (296)
General health care system financing
- Municipalities are responsible for providing health care that is financed through local tax.
- Every resident in the country is entitled to health care services from the tax-funded system (funds from the 3 levels of government and a small private sector). Municipal authority hospitals provide specialist care, and privately owned hospitals supplement with, for example, day surgery.
- The health care is organized in 3 levels—primary or family care, specialist care, and nursing care
- Payroll tax covers 78% of all expenditures. The Estonian Health Insurance Fund (EHIF) is the sole provider of universal health coverage. The EHIF covers approximately 95% of the population; the rest is covered by the Ministry of Social Affairs. The ministry also has a main responsibility.
- All health care providers are independent entities; family physicians are local entrepreneurs in private companies.
- The health care is organized in 2 levels—primary care (municipalities) and specialist care (the state). Specialist care is divided into 4 health regions and provides specialist care (mainly hospital based) and ambulance service. The state financing is channeled through the health regions.
- The tax-funded system covers health care for all citizens.
- The health care is organized on 3 levels—the state, regions, and municipalities. The regions with their own elected parliaments decide on regional tax, which provides the major financing for health care. National tax funds also constitute large parts of regions’ and municipalities’ health care budgets. Home care is funded through local municipality taxes.
- The tax-funded system covers everyone, including recent immigrants. It is free for inpatient care, and outpatient care has a low cost. Most of the care is operated by regions, but especially primary care and some specialist services are performed by private companies under contracts with the regions. The private share varies a lot among regions, with the largest private providership in the capital region where also a small private part exists independent of the public system that is financed mainly through optional insurance.
Primary care
- These are municipality-arranged services at municipal health centers.
- They include population health monitoring and the promotion of well-being and health as well as prevention, diagnostic services, and treatment.
- Every EHIF-insured individual (and every Estonian resident) is assigned to a personal general practitioner (GP), who is the first level of contact. The insured individual can choose the GP. Approximately 70% of GPs are in solo practices. Practice lists cover the entire population. There are no copayment fees for primary care services in the EHIF package.
- The GP is the main point of contact for health benefits; they are expected to manage most patient pathways and to refer patients to specialist care or long-term nursing care and rehabilitation.
- Provides >50% of ambulatory care visits.
- Services are arranged by the municipalities based on the GP scheme. All citizens have the right to register with a GP of their choice. There are approximately 5000 GPs in total.
- The GPs are the first line of contact; they coordinate care, are gatekeepers for welfare goods, and manage referrals to specialist care.
- Municipalities also offer, for example, emergency care, home care, and rehabilitation services.
- Services are arranged by the regions. Each citizen is connected to a primary care team (often physicians, nurses, psychologists, and physiotherapists), which is the first line of contact. There are approximately 900 primary care centers, between 10% and 50% private depending on the region. Primary care is never provided by single physicians. Care centers are relatively large, with 2 to 10 physicians and a total staff of 20 to 80 people. Organization differs somewhat among regions.
- Primary care handles many issues directly but may also refer to specialist inpatient or outpatient clinics. They also work with health promotion and preventive care (eg, vaccination) and maternity and child care and are the direct clinical contact of the municipalities’ home health care.
Steering of health information and communications technology (ICT) development
- The national solution (Omakanta) is provided by the Social Insurance Institution (Kela). Private and public providers often also provide local portals with no connection to Omakanta.
- Decisions on regional health ICT systems are often made by municipalities or hospital districts. Health Village (portal implemented by all university hospitals) and Omaolo (municipality collaborative) are national initiatives.
- There are 2 main domains: Central databases, services, and applications: the National Health Information System (central database and services governed by the Ministry of Social Affairs) and the database of the EHIF are the major components. Databases and applications of health care stakeholders: electronic medical records (EMRs) and health information systems (HISs) of care facilities, as well as applications provided and maintained through private companies, are the major components. EMRs and HISs need to comply with central systems and legal regulations on, for example, data sharing.
- The Directorate of eHealth was established in 2016 and is responsible for implementing national policy and steering and coordinating eHealth initiatives with stakeholders. They are generally responsible for the work in national eHealth programs, including the national portal Helsenorge. Since 2020, the Norwegian Health Network has had the responsibility to develop and operate the portal. It is the health regions’ decision whether to use the national offered health portal for patient-accessible electronic health records (PAEHRs) in their region.
- Major decisions are made by the 21 autonomous regions; hence, there is not much national coordination. However, collaborations on common procurement of electronic health record (EHR) systems among regions, as well as among secondary care, primary care, and municipalities in some larger regions, have happened recently (Cambio COSMIC is procured by 17 of 21 regions).
- Inera (subsidiary of the Swedish Association of Local Authorities and Regions) supports regions with ICT development and interoperability issues. Inera manages the 1177 patient portal, where Journalen resides.
- The eHealth authority (under the Ministry of Health and Social Affairs) manages electronic prescriptions of medicinal products. They hold the prescription database and link together all pharmacies.
Aims and Motivation
The study presented in this paper had two main aims: (1) to develop a detailed collaborative method suitable for cross-country sociotechnical analyses of eHealth services for patients’ access to their records and (2) to illustrate the data collection method by comparing results regarding contextual factors enabling PAEHR access and use in 4 European countries. Starting from a sociotechnical analysis framework developed by Sittig and Singh [ 17 ] with an intention to produce a detailed data collection template for cross-country sociotechnical comparisons, a collaboration method was developed and implemented. The resulting data collection instrument was then used in a case study where a cross-country analysis was conducted based on collected data from Estonia, Finland, Norway, and Sweden.
This sociotechnical analysis is valuable because, while innovation in PAEHRs is important, there must be a concomitant, ongoing focus on how these tools are integrated into health systems. Digital innovations can fragment care or risk not being used, and examining the social and technical factors pertaining to PAEHRs in different settings is crucial. This will help us understand potential nonadoption; abandonment; and the effects of using such tools in health care on a wide range of stakeholders, including patients and clinicians.
Method Development
Developing a method that involved collaboration among knowledgeable representatives from all countries involved was a necessity for arriving at the desired result—a data collection instrument that enabled a detailed cross-country sociotechnical system comparison. Earlier research based on more limited sociotechnical comparisons has shown that PAEHR systems differ greatly across countries, mostly due to differences in health care policy, underlying care structures, and health ICT initiatives [ 3 ]. To be able to develop a data collection instrument that both covers important aspects of the investigated countries and systems and is relevant in the respective health care system contexts, it was deemed of high importance to involve experts from each country.
The NORDeHEALTH project participants were not experts on every aspect covered, but they had established connections with relevant health authorities and development companies that could provide necessary information through documents or in-person communication. These contacts were necessary for the success of the study, and hence, the continuous contact with these external national agencies and companies was a vital part of the developed collaboration method. On the basis of the experience with the method development, we argue that these external connections need to be established before doing similar research in the future.
The sociotechnical analysis framework by Sittig and Singh [ 17 ] on which this work was based was general and theoretical in the sense that dimensions but not any specific data collection items were presented. The framework was also aimed toward sociotechnical health systems in general and not PAEHR systems or even eHealth services for patients in particular. Hence, a big part of the method was devoted to developing a data collection instrument that would work in this specific context—a cross-country analysis of PAEHR systems in the 4 involved countries. An inevitable consequence of this is that the data collection instrument developed in this study cannot be used to enable sociotechnical analysis of other kinds of health care systems. This being said, a similar method can be used to develop other data collection instruments that include items for the different framework dimensions that are better suited to other kinds of health care systems.
The framework by Sittig and Singh [ 17 ] has indeed been adapted for sociotechnical analysis of health care technology systems but not for cross-country comparisons. As a consequence, an important part of this work was to amend it for the purpose of comparing health care systems across countries. In total, 2 dimensions were added to the framework for this purpose—metadata and health care system context. It is beneficial to include the Metadata dimension in any kind of sociotechnical analysis, but we argue that it is especially important to include this dimension when comparing data from different countries with their own systems, responsible data collectors, and information sources.
The collection of data for the Health care system context dimension occurred after the data collection that was based on the developed data collection instrument. After data collection, this dimension was added as a last step of the iterative development of the method and the data collection instrument in line with the design science research approach followed in this study. As earlier research has shown that PAEHR systems vary across countries due to contextual factors [ 3 ], this dimension is of high importance to consider in a cross-country comparison of the kind carried out in this study. The national setup of primary and specialist care, as well as general digital care infrastructure and steering of health ICT development, can affect the results of all other framework dimensions. Hence, we argue that this dimension is necessary to include in these kinds of studies.
Country Comparison
The sociotechnical cross-country comparisons regarding contextual factors brought to light several differences and similarities among the involved countries. Hardware and software computing infrastructure was the dimension in which most of the similarities could be found. However, it is noteworthy that the use of standards differs considerably across countries, which could potentially give rise to interoperability issues in case, for example, the Nordic countries were to move toward a joint service. It is also of interest to note that physicians in Estonia manually upload some content to the PAEHR, whereas Sweden and Norway only use the automatic link to EHRs at runtime. In Finland, content is also uploaded to the centralized PAEHR but by technical or administrative staff rather than clinicians. This means that health professionals in Estonia can be said to be active users of the PAEHR services. There are some risks associated with being an active user. Aside from the fact that the workload of health care professionals increases, there is also a risk that the personal opinions of health care professionals may affect their willingness to share information and, consequently, the patients’ potential to access their information. Overall, the level of engagement of health care professionals affects whether patients will be able to access some information. In Norway and in some regions in Sweden, patients can only see notes that are signed by a health care professional. Some regions in these countries also have a default delay in publishing notes to patients regardless of whether they are signed. This makes it possible to hide information from patients for some time, for example, by not signing a note.
When it comes to user demographic information, similarities could also be found, but it was difficult to compare data due to differences among the statistical information that the countries provide and how these data are presented. In these kinds of analyses, it is important to be able to compare user statistics on how different groups use the PAEHR services, and this comparison was not possible as the statistics covered different user groups in Sweden and Finland. Estonia and Norway did not (at the time of data collection) collect statistics on individual PAEHR use. The difference in available national statistics on demographic use clearly shows the need to collect user statistics preferably based on comparable user groups and age intervals. This would aid future comparisons as well as evaluations of PAEHR services within countries.
In the Health care system context dimension, several differences could be found regarding health care system setup and financing. While all involved countries have 3 levels of government (state, regions or counties, and municipalities), there are differences in which levels provide most of the funding and are responsible for different levels of care. While the regions are responsible for most health care services in Sweden, the responsibility is more divided in the other countries. Differences are also found regarding steering of health ICT development. While, in most countries, the national PAEHR solution is managed by government-owned companies or insurance institutions, there are differences in other areas of health ICT.
Strengths and Limitations
To our knowledge, this kind of detailed comparison among PAEHR systems in different countries is novel. While Essén et al [ 3 ] compared PAEHR systems in 10 different countries, including Estonia, Finland, Norway, and Sweden, their comparison focused on the effect of differences in regulations on a few aspects of PAEHRs. Our study expands this research by using a complete sociotechnical framework as a base, enabling more detailed comparisons and a more in-depth analysis of similarities and differences across countries. This kind of comparison is important if we want to understand the effects of contextual factors on the realization of PAEHR systems and will also make it possible for systems to learn from each other. This learning takes place not only when analyzing the results from the data collection but also when carrying out the developed collaboration method.
This study used a specific sociotechnical framework as a base, and even though the framework by Sittig and Singh [ 17 ] is tailored toward health systems, it risks constraining the focus to certain dimensions. There are other sociotechnical frameworks that could have been used instead. This being said, the involved researchers did add and change some dimensions to better fit PAEHR systems in particular and the cross-country comparison. Hence, even though a specific framework was used as an important base, it did not completely constrain the focus.
The number of countries involved—and, hence, the number of compared systems—was fairly limited, especially considering the fact that there are several clear similarities among the Nordic countries when it comes to national contexts. Comparisons involving more diverse countries should be conducted when validating the method.
Future Work
This paper presented the results of a sociotechnical cross-country comparison among PAEHR systems in Estonia, Finland, Norway, and Sweden related to contextual factors. The dimensions that were in focus in this paper cover 4/11 of the total dimensions in the data collection instrument. Thus, there are more in-depth comparisons being made based on the data collected in this study. In future work, more dimensions will be included, and the results will be published in subsequent papers.
In future research, it would also be beneficial to increase the number of countries to be compared. The next step in this direction could be to extend the analysis conducted by Essén et al [ 3 ] by using the framework by Sittig and Singh [ 17 ] and the collaboration method presented in this paper. This would not only include a comparison of PAEHR systems from more countries with larger differences in national contexts, but it would also validate the method in a more complex study. These kinds of more expanded comparisons could also use other frameworks as their base. It is also important to note here that digital forms were not developed in this study as the number of systems to be included in the data collection in the 4 involved countries was fairly limited and, hence, the statistical analysis made possible in many digital tools would not add any value. Instead, the data collection was prepared as a Word template to be filled out digitally. In future research including more countries and systems, it would be well worth introducing digital forms and statistical analyses.
The study presented in this paper only focused on PAEHR systems. Even though these systems are in increasing focus in research nowadays, there are many more types of eHealth services for patients as well as other types of health care systems. In future research, we would like to see this collaboration method used for developing data collection instruments related to other kinds of health care systems, such as apps for self-tracking or systems for video visits.
Future research could also usefully focus in greater depth on particular sociotechnical dimensions of PAEHRs in different countries. For example, regarding human-computer interfaces, investigators might explore the highly specific design features of portals, which may augment psychological dispositions to access health information, or (possibly more importantly) offer feedback to providers.
Conclusions
In this work, we aimed to develop and test a method that would enable cross-country sociotechnical analysis of PAEHRs in 4 countries where PAEHR use has reached maturity: Estonia, Finland, Norway, and Sweden. The main artifact produced during this work was a sociotechnical data collection template that was based on a sociotechnical framework for studying eHealth services derived by Sittig and Singh [ 17 ]. The data collection template not only considers the dimensions in the framework by Sittig and Singh [ 17 ] tailored for single-system analysis but also includes parts deemed necessary for cross-country comparisons of several systems. Close collaboration during the process among researchers from all involved countries ensured relevance in all settings.
The data collection template was tested through data collection in the 4 countries and a sociotechnical analysis. Results indicated several important similarities and differences among the 4 countries, clarifying that the process followed and the data collection template enabled an in-depth cross-country sociotechnical analysis of PAEHR systems. This paper presents the first part of the results from the sociotechnical analysis—similarities and differences among contextual factors—and companion articles will delve deeper into the remaining parts.
Acknowledgments
This work was supported by NordForsk through the funding to the Nordic eHealth for Patients: Benchmarking and Developing for the Future (NORDeHEALTH project 100477); by the Strategic Research Council at the Academy of Finland (grants 352501 and 352503); and by the Swedish Research Council for Health, Working Life, and Welfare through the funding to Beyond Implementation of eHealth (project 2020-01229).
Data Availability
The data sets generated and analyzed during this study are not publicly available due to the fact that additional publications based on this data set are being produced, but are available from the corresponding author on reasonable request.
Conflicts of Interest
CB is the Associate Editor of JMIR Mental Health .
Final data collection instrument.
Information sources.
Descriptions of health care system contexts.
- Cijvat CD, Cornet R, Hägglund M. Factors influencing development and implementation of patients' access to electronic health records-a comparative study of Sweden and the Netherlands. Front Public Health. Jun 8, 2021;9:621210. [ FREE Full text ] [ CrossRef ] [ Medline ]
- Hägglund M, DesRoches C, Petersen C, Scandurra I. Patients' access to health records. BMJ. Oct 02, 2019;367:l5725. [ CrossRef ] [ Medline ]
- Essén A, Scandurra I, Gerrits R, Humphrey G, Johansen MA, Kierkegaard P, et al. Patient access to electronic health records: differences across ten countries. Health Policy Technol. Mar 2018;7(1):44-56. [ CrossRef ]
- Walker J, Leveille S, Bell S, Chimowitz H, Dong Z, Elmore JG, et al. OpenNotes after 7 years: patient experiences with ongoing access to their clinicians' outpatient visit notes. J Med Internet Res. May 06, 2019;21(5):e13876. [ FREE Full text ] [ CrossRef ] [ Medline ]
- Moll J, Rexhepi H, Cajander Å, Grünloh C, Huvila I, Hägglund M, et al. Patients' experiences of accessing their electronic health records: national patient survey in Sweden. J Med Internet Res. Nov 01, 2018;20(11):e278. [ FREE Full text ] [ CrossRef ] [ Medline ]
- DesRoches CM, Bell SK, Dong Z, Elmore J, Fernandez L, Fitzgerald P, et al. Patients managing medications and reading their visit notes: a survey of OpenNotes participants. Ann Intern Med. Jul 02, 2019;171(1):69-71. [ CrossRef ] [ Medline ]
- Bell SK, Folcarelli P, Fossa A, Gerard M, Harper M, Leveille S, et al. Tackling ambulatory safety risks through patient engagement: what 10,000 patients and families say about safety-related knowledge, behaviors, and attitudes after reading visit notes. J Patient Saf. Dec 01, 2021;17(8):e791-e799. [ CrossRef ] [ Medline ]
- Bell SK, Mejilla R, Anselmo M, Darer JD, Elmore JG, Leveille S, et al. When doctors share visit notes with patients: a study of patient and doctor perceptions of documentation errors, safety opportunities and the patient-doctor relationship. BMJ Qual Saf. Apr 2017;26(4):262-270. [ FREE Full text ] [ CrossRef ] [ Medline ]
- Zanaboni P, Kummervold PE, Sørensen T, Johansen MA. Patient use and experience with online access to electronic health records in Norway: results from an online survey. J Med Internet Res. Feb 07, 2020;22(2):e16144. [ FREE Full text ] [ CrossRef ] [ Medline ]
- World Health Organization. Digital health platform handbook: building a digital information infrastructure (infostructure) for health. 2020. URL: https://apps.who.int/iris/handle/10665/337449 [accessed 2023-12-22]
- What is an electronic health record (EHR)? HealthIT.gov. URL: https://www.healthit.gov/faq/what-electronic-health-record-ehr [accessed 2023-12-22]
- Metsallik J, Ross P, Draheim D, Piho G. Ten years of the e-health system in Estonia. In: Proceedings of the 3rd International Workshop on (Meta)Modelling for Healthcare Systems. 2018. Presented at: MMHS 2018; June 13-15, 2018; Bergen, Norway.
- Kujala S, Hörhammer I, Väyrynen A, Holmroos M, Nättiaho-Rönnholm M, Hägglund M, et al. Patients' experiences of web-based access to electronic health records in Finland: cross-sectional survey. J Med Internet Res. Jun 06, 2022;24(6):e37438. [ FREE Full text ] [ CrossRef ] [ Medline ]
- Blease C, Salmi L, Rexhepi H, Hägglund M, DesRoches CM. Patients, clinicians and open notes: information blocking as a case of epistemic injustice. J Med Ethics. May 14, 2021;48(10):785-793. [ FREE Full text ] [ CrossRef ] [ Medline ]
- Om pasientjournal. Helsenorge. URL: https://www.helsenorge.no/pasientjournal/om/ [accessed 2023-09-26]
- Baxter G, Sommerville I. Socio-technical systems: From design methods to systems engineering. Interact Comput. Jan 2011;23(1):4-17. [ FREE Full text ] [ CrossRef ]
- Sittig DF, Singh H. A new sociotechnical model for studying health information technology in complex adaptive healthcare systems. Qual Saf Health Care. Oct 2010;19 Suppl 3(Suppl 3):i68-i74. [ FREE Full text ] [ CrossRef ] [ Medline ]
- Hägglund M, Scandurra I. A socio-technical analysis of patient accessible electronic health records. Stud Health Technol Inform. 2017;244:3-7. [ FREE Full text ] [ Medline ]
- Hägglund M. Nordic countries lead new initiative on patient access to EHRs. BMJ Opinion. May 18, 2021. URL: https://blogs.bmj.com/bmj/2021/05/18/maria-hagglund-nordic-countries-lead-new-initiative-on-patient-access-to-ehrs/ [accessed 2023-12-22]
- Hägglund M, Blease C, Johansen MA, Kujala S, Scandurra I. Exploration of a socio-technical analysis template for patient accessible electronic health records. In: Proceedings of the 2020 Special Topic Conference of the European Federation for Medical Informatics. 2020. Presented at: EFMI STC 2020; November 26-27, 2020; Virtual Event. URL: https://tinyurl.com/349djn9n
- Peffers K, Tuunanen T, Rothenberger MA, Chatterjee S. A design science research methodology for information systems research. J Manage Inf Syst. Dec 08, 2014;24(3):45-77. [ CrossRef ]
- 1177 Vårdguiden. URL: https://www.1177.se/ [accessed 2024-07-02]
- Terviseportaal. URL: https://www.terviseportaal.ee/ [accessed 2024-07-02]
- OmaKanta. Kanta. URL: https://www.kanta.fi/omakanta [accessed 2024-07-02]
- Helsenorge. URL: https://www.helsenorge.no/ [accessed 2024-07-02]
Abbreviations
electronic health record |
information and communications technology |
patient-accessible electronic health record |
research question |
Edited by A Mavragani; submitted 22.12.23; peer-reviewed by A Mahmud, P Pohl; comments to author 29.02.24; revised version received 05.04.24; accepted 08.04.24; published 26.08.24.
©Jonas Moll, Isabella Scandurra, Annika Bärkås, Charlotte Blease, Maria Hägglund, Iiris Hörhammer, Bridget Kane, Eli Kristiansen, Peeter Ross, Rose-Mharie Åhlfeldt, Gunnar O Klein. Originally published in the Journal of Medical Internet Research (https://www.jmir.org), 26.08.2024.
This is an open-access article distributed under the terms of the Creative Commons Attribution License (https://creativecommons.org/licenses/by/4.0/), which permits unrestricted use, distribution, and reproduction in any medium, provided the original work, first published in the Journal of Medical Internet Research (ISSN 1438-8871), is properly cited. The complete bibliographic information, a link to the original publication on https://www.jmir.org/, as well as this copyright and license information must be included.
- Open access
- Published: 24 August 2024
The impact of religious spiritual care training on the spiritual health and care burden of elderly family caregivers during the COVID-19 pandemic: a field trial study
- Afifeh Qorbani 1 ,
- Shahnaz Pouladi 2 ,
- Akram Farhadi 3 &
- Razieh Bagherzadeh 2
BMC Nursing volume 23 , Article number: 584 ( 2024 ) Cite this article
68 Accesses
Metrics details
Family caregiving is associated with many physical and psychological problems for caregivers, but the effect of spiritual support on reducing their issues during a crisis is also the subject of research. The study aims to examine the impact of religious spiritual care training on the spiritual health and care burdens of elderly family caregivers during the COVID-19 pandemic.
The randomized controlled field trial involved 80 Iranian family caregivers in Bushehr City, who were selected by convenience sampling based on the inclusion criteria and divided into experimental (40 people) and control (40 people) groups by simple random sampling in 2021 and 2022. Data collection was conducted using spiritual health and care burden questionnaires using the Porsline software. The virtual intervention included spiritual and religious education. Four virtual sessions were held offline over two weeks. The first session was to get to know the participants and explain the purpose, The second session focused on the burden of care, the third on empowerment, and the fourth on mental health and related issues. In the control group, daily life continued as usual during the study.
Mean changes in existential health (3.40 ± 6.25) and total spiritual health (5.05 ± 11.12) increased in the intervention group and decreased in the control group. There were statistically significant differences between the two groups for existential health (t = 3.78, p = 0.001) and spiritual health (t = 3.13, p = 0.002). Cohen’s d-effect sizes for spiritual health and caregiving burden were 0.415 and 0.366, respectively. There was no statistically significant difference in mean changes in religious health ( p = 0.067) or caregiving burden ( p = 0.638) between the two intervention and control groups.
Given that the religious-spiritual intervention had a positive effect on existential health and no impact on religious health or care burden, it is recommended that comprehensive planning be undertaken to improve the spiritual health of family caregivers to enable them to better cope with critical situations such as a COVID-19 pandemic.
Trial registration
IRCT code number IRCT20150529022466N16 and trial ID number 48,021. (Registration Date2020/06/28)
Peer Review reports
With the global outbreak of COVID-19 on January 12, 2020, and the highly contagious nature of this virus, the World Health Organization issued protocols for limiting community interactions worldwide [ 1 ]. While individuals of all ages are susceptible to COVID-19, The high incidence of infection in older people, the greater severity of the disease, and the increased mortality are significant challenges in implementing appropriate preventive measures and future strategies to protect against this disease in the geriatric population [ 2 , 3 ]. According to the US Centers for Disease Control and Prevention, 31% of COVID-19 cases, 45% of hospitalizations, 53% of intensive care unit admissions, and 80% of COVID-19-related deaths in the United States occur in the elderly [ 4 ].
During the COVID-19 crisis, elderly people required various forms of assistance, including telephone and digital visits, with most of these services provided by family members [ 5 ], Park (2021) reported that long-term caregivers (> 1 year) had more negative somatic physical symptoms (headaches, body aches, and abdominal discomfort), worse mental health, and more significant fatigue than non-caregivers [ 6 ]. Family caregivers can only provide up to 80% of the required care to seniors with Multiple chronic conditions in the community, and they are also responsible for the majority of the costs and shoulder the related burden. This increased reliance on family caregivers has, in turn, heightened their care burden. The burden of care is a significant issue globally, with millions of individuals taking on caregiving responsibilities for their loved ones. The care burden encompasses various dimensions, including time-dependent, evolving, physical, social, and emotional aspects, making it a complex and highly individualized concept [ 7 ]. It often results from a negative imbalance between caregiving responsibilities and other obligations [ 8 ].
In Iran, like in many other countries, this burden can have profound implications on caregivers’ physical, emotional, and financial well-being. By introducing the concept of spiritual health into the discourse, we aim to shed light on a potentially overlooked aspect that could provide additional support and resilience to caregivers. Statistics indicate that caregivers who report a strong sense of spiritual well-being often exhibit lower levels of stress, anxiety, and depression, highlighting the importance of addressing this dimension in caregiving research. The existing literature on caregiver burden focuses mainly on caregiving’s physical and emotional aspects. While these studies provide valuable insights, there is a noticeable gap in understanding the role of spiritual health in mitigating the burden of care. Further exploration is needed to investigate how spiritual well-being can influence the overall caregiving experience and contribute to the well-being of the caregiver and the care recipient. In Iranians’ religious and national culture, the elderly hold a revered position and are highly respected. Reflecting on this cultural perspective, the Prophet of Islam stated, that respecting older people of my community is the same as respecting me [ 9 ]. This cultural context is evident in the fact that 86.4% of elderly individuals in Iran, according to statistics from the welfare organization, live with their children and spouses [ 10 ]. However, when caregiving responsibilities increase, they can overshadow the multiple health dimensions of the older people’s family members, including physical, psychological, social, and spiritual aspects. Coping strategies, such as spiritual-religious approaches, are often employed to manage the challenges [ 11 ].
There are two dimensions to spiritual health: religious and existential. Religious health refers to how a person understands his or her spiritual well-being when connected to a higher power. Conversely, existential health centers on an individual’s capacity for adaptation to their being, the societal landscape, and the broader environment [ 12 ]. In the past, the significance of spirituality in effectively managing stress was often underestimated; however, recent years have seen increased attention from researchers [ 11 , 13 , 14 ]. It is important to note that the understanding of spirituality is influenced by culture and religion, and its implications may vary for different individuals [ 15 ]. The current research gap lies in the lack of comprehensive studies that assess the intersection of spiritual health and care burden in the Iranian caregiving landscape. While some research exists on the broader topic of spirituality and health, there is a need for targeted investigations that consider the unique cultural and religious factors that shape the Iranian perspective on caregiving. Understanding these nuances can provide valuable insights into how spiritual care practices can be effectively integrated into support systems for caregivers in Iran. To the best of our knowledge, no previous study has investigated the impact of religious-spiritual care training on the spiritual health and care burden of family caregivers of older people during the COVID-19 pandemic. Given the critical role of nurses as caregivers for family and elderly health along with their supportive function [ 16 ], it is essential to identify caregivers at risk during critical situations and address their spiritual needs as part of community-oriented care. The study aimed to examine the impact of religious spiritual care training on the spiritual health and caregiving burden of older family carers during the COVID-19 pandemic. By thoroughly exploring the relationship between spiritual health and the caregiving burden of older family carers, we aim to identify potential strategies and interventions that can improve the well-being of caregivers and the overall quality of care provided to care recipients in Iran.
Study design
This study utilized a randomized controlled field trial design. The choice of a field randomized controlled trial for this study provides a rigorous and systematic approach to evaluating the effectiveness of a spiritual health intervention on care burden among Iranian caregivers. This design ensures internal validity, generalizability, and ethical soundness, thereby strengthening the overall quality of the research findings.
Participants and data collection
Participants were selected from the home care department of the comprehensive rehabilitation service center for the elderly in Mohammadieh, Bushehr City (affiliated with the welfare organization), and four comprehensive urban health centers in Bushehr Port, specifically Kheybar, Quds, Meraj, and Shohada centers. The inclusion criteria encompass caring for elderly individuals who showed a degree of dependence in at least one of their six daily activities, as defined by Katz’s criteria for activities of daily living (ADL). Additionally, caregivers had to possess literacy skills (reading and writing), with at least six months having elapsed since the commencement of their caregiving responsibilities. Furthermore, inclusion criteria require a family relationship between caregivers and elderly individuals in their care, cohabitation with older people, and delivering at least 40 h of care per week. Caregivers had to be at least 18-year-old Shia Muslims. The exclusion criteria dictated that the caregivers be excluded from the study under certain conditions, including the death of either the caregiver or the elderly individual during the study, refusal to continue participation in the study, the presence of neurological and psychiatric diseases, or the use of neuropsychiatric drugs, self-reported drug or alcohol addiction, or prior involvement in a spiritual-religious educational program related to elderly care.
Sample size
Based on the effect sizes observed in the studies by Hosseini et al. (2016) [ 17 ], Mahdavi et al. (2016) [ 18 ], and Moeini et al. (2012) [ 19 ], with a Type I error rate of 0.50 and a power of 80%, and using the G Power 3.1.9.2 software, the required sample size for the two-group test was approximately 80 individuals, with 40 participants in each group. Eligible elderly family caregivers were selected from available candidates and randomly assigned to either the test or control group (Fig. 1 ). Randomization was done using Random Allocation software and by a person who did not know the participants and did not know their characteristics.
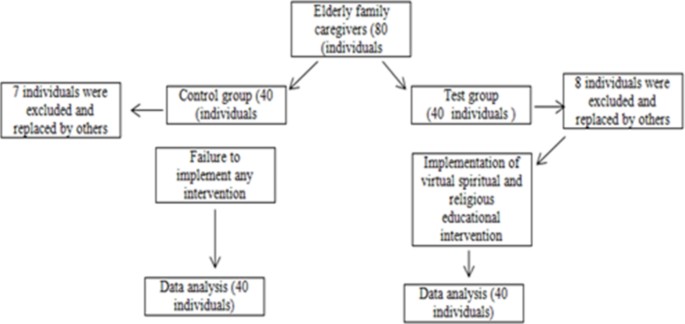
Consort diagram
Instruments
The data collection instruments used in this study consisted of a demographic information form, along with the spiritual health questionnaire developed by Paloutzian and Ellison (1982) and the care burden questionnaire designed by Novak and Guest (1989).
Demographic information form
This form collected information about the caregiver, including age, number of children, family relationship to older people, level of education, occupation, income, and type of housing.
Spiritual health questionnaire (Paloutzian and Ellison, 1982)
The Spiritual Health Questionnaire, developed by Paloutzian and Ellison in 1982, is widely used to assess an individual’s spiritual well-being and beliefs. This questionnaire consists of 20 items that explore different aspects of spirituality, including beliefs, practices, values, and experiences. Participants are asked to respond to statements about spirituality on a six-point Likert scale, with responses to agree strongly or to disagree strongly. This questionnaire includes two subscales: (1) Religious well-being (10 items): This subscale assesses how an individual’s religious beliefs, values, and practices contribute to their overall well-being and sense of purpose. (2) Existential well-being (10 items): This subscale focuses on the individual’s sense of meaning, purpose, and connection to something greater than themselves, regardless of religious affiliation. Each subscale receives a score from 10 to 60. The spiritual health score is the sum of these two subscales and ranges from 20 to 120. In Iran, during the research conducted by Parvizi et al. (2000), the reliability of this questionnaire using Cronbach’s alpha coefficient was 0.82 [ 20 ]. In Hamdami et al.‘s research (2015), Cronbach’s alpha coefficient of the total spiritual health score was 0.79 [ 21 ].
Care burden questionnaire (Novak and Guest, 1989)
The Care Burden Questionnaire, developed by Novak and Guest in 1989, is a widely used instrument for assessing the burden experienced by caregivers who provide care to individuals with chronic illnesses or disabilities. Caregivers are asked to respond to a series of statements concerning caregiving burden on a Likert scale, with response options typically ranging from 1 (Strongly Disagree) to 5 (Strongly Agree). The maximum score that can be attained on this questionnaire is 96, while the minimum score is 0. The questionnaire includes five sub-scales designed to capture a specific aspect of the burden. These include time demands, emotional stress, social isolation, financial strain, and conflicts with other responsibilities. In Iran, in the study of Abbasi et al. (2013), the Cronbach’s alpha coefficient of this questionnaire was 0.90, and its subscales ranged from 0.72 to 0.82 [ 22 ].
Baseline test
Before the intervention, research sessions were initially scheduled to occur in person; however, the coronavirus pandemic rendered it impractical to conduct face-to-face training sessions. As a result, spiritual and religious training was carried out online without impacting b behavioral therapy (CBT) and stress management techniques. It was structured to address the emotional, social, and physical dimensions of caregiver burden while simultaneously fostering coping strategies and self-care practices. The intervention framework was informed by existing research on caregiver interventions, CBT, and stress management. Studies have shown the effectiveness of psychoeducational programs in reducing caregiver burden and enhancing well-being. The incorporation of CBT techniques aimed to help caregivers identify and reframe negative thought patterns, while stress management strategies were included to help caregivers better cope with stressors.
In the test group, the intervention took the form of spiritual care based on the model of Richards and Bergin, which was aligned with Islamic teachings. This model featured six key steps: First, caregivers were guided to pay attention to spiritual-cultural sensitivities. Second, they were trained to establish an open and secure spiritual relationship. Third, potential ethical challenges were addressed. Fourth, caregivers conducted a religious and spiritual assessment of clients. The fifth step involved defining suitable goals for spiritual therapy, and the final step focused on properly implementing spiritual interventions [ 23 ]. The educational sessions covered various dimensions of the care burden, including physical, mental, social, and financial elements, as well as facets of spiritual health, which included the religious dimension (about communication with a transcendent higher power) and the existential dimension (encompassing communication with oneself, creation, and all living beings). These educational sessions were delivered via pre-recorded video presentations developed by a specialist in geriatric nursing and religious education. Participants engaged in four virtual sessions offline, conducted through WhatsApp social messenger, with two sessions held per week. Each session involved the following activities: (1) Following up on the previous session’s topics; (2) providing feedback to participants; (3) summarizing and outlining previous topics to create a connection between the topics discussed; and (4) offering explanations and summaries related to the new session’s topic. One month after the end of the intervention, test and control group participants completed the Mental Health Questionnaire and the Carer’s Burden Questionnaire again. The control group continued with their daily lives as usual throughout the study. Upon its conclusion, the educational materials on spirituality and its various concepts, which had been shared via WhatsApp Messenger, were made available in alignment with the ethical principles that govern such research. The educational content for the sessions was developed by a multidisciplinary team consisting of a nurse psychiatrist, a gerontologist, and a Specialist in Quran and Hadith. The educational content was designed and compiled by the research team to improve practical skills, stress management, self-care, and communication, based on the model of Richards and Bergin and according to the teachings of Islam and the Shia religion. To ensure the accuracy and reliability of the content, the educational materials underwent a rigorous review process involving experts from diverse fields, including caregiving, psychology, and Quranic and Hadith sciences. Feedback from caregivers and pilot testing were also used to refine and validate the content before implementation. A pilot study was conducted to test the intervention’s feasibility, acceptability, and initial effectiveness. The pilot study involved a small group of caregivers who received the intervention, and their feedback was used to refine the program before full implementation. All contributors implementing the intervention received comprehensive training on the educational content and intervention protocols. These trainings were followed daily by viewing the participants’ WhatsApp to receive educational content and listening to audio files, making daily phone calls, and asking them questions over the phone to understand the content and express their questions. The intervention was implemented by a team of trained healthcare professionals, including a social gerontologist, a nursing gerontologist, and a medical-surgical nursing student with a master’s degree. They all had relevant qualifications and expertise in mental health and caregiving support. Potential challenges for implementers could include caregiver resistance, emotional distress, not receiving training materials on time, or difficulty engaging participants. The plan for dealing with such situations included regular monitoring of caregiver progress, open communication, and flexibility in the delivery of sessions. For participants who required more specialized training or support beyond the scope of the intervention, referrals were made through telephone communication with the training session facilitators. Response data from the instruments, such as the Care Burden Questionnaire and other assessment measures, were collected through self-report questionnaires and standardized rating scales administered by trained assessors. Caregivers were asked to respond based on their experiences before and after the intervention. To handle ambiguities in the response data, assessors were trained to clarify any uncertainties or ambiguities in the questions with caregivers. This involved providing clear explanations, and examples, and ensuring that caregivers understood the questions before responding. A specific post-intervention assessment time point was established to standardize the time after the intervention for all participants. This time point was determined based on the intervention duration and the optimal timeframe for assessing the intervention’s impact on caregiver burden based on past studies [ 24 , 25 ]. Caregivers were scheduled for the post-intervention assessment at this standardized time point to ensure consistency across all participants.
Ethical considerations
This study originated from a master’s thesis in internal surgical nursing at the Faculty of Midwifery Nursing, Bushehr University of Medical Sciences, with an ethics code number of IR.BPUMS.REC.1399.042. It is also registered with the Clinical Trial Centre of Iran under IRCT20150529022466N16. The caregivers were furnished with comprehensive information about the study, encompassing its objective, methodology, potential hazards and advantages, confidentiality protocols, and their entitlement to withdraw from the study at any point. Informed consent was obtained from all participants before they participated in the study. Measures were taken to ensure the confidentiality of participants’ personal information and data collected during the study. Participants were assured that their responses would be anonymized, stored securely, and only accessed by authorized research staff.
Data analysis
Due to the peak of the Corona pandemic and the closing of universities in person, the possibility of consulting statistics professors and performing data analysis was delayed for eight months. The data collected during the study were analyzed using SPSS version 19 software. The Shapiro-Wilk test was used to check the distribution of the data. An independent t-test, or Mann-Whitney test, was used to compare quantitative demographic variables between two groups. A Chi-squared or Fisher’s exact test was used to compare qualitative demographic variables between groups. To test the hypotheses above, a paired t-test was employed to ascertain the mean of the primary variables in question, before and after the intervention in each group. An independent t-test was utilized to determine the mean of the variables between groups, and Cohen’s d was calculated as the effect size. Independent t-tests were conducted to compare the mean scores of the changes. The significance level was assumed to be less than 0.05 in all cases.
No statistically significant differences were detected between the groups in terms of demographic variables, suggesting group homogeneity ( p > 0.05) (Tables 1 and 2 ). Regarding spiritual health, within the intervention group, the post-test average score for total spiritual health was significantly higher than the pre-test score ( p = 0.007), in contrast within the control group, the post-test average score was considerably lower than the pre-test score ( p = 0.003). No statistically significant differences were observed between the two groups in terms of mean posttest spiritual health scores (Table 3 ) still, changes in overall spiritual health increased in the intervention group and decreased in the control group, with statistically significant differences between the two groups ( p = 0.002) (Table 4 ). The Cohen’s d effect size for the difference in spiritual health between the intervention and control groups was 0.415, indicating a moderate effect of the intervention (Table 3 ). Within-group analysis showed no statistically significant differences between pre-and post-test scores for total care burden in either group. Furthermore, no statistically significant differences between the two groups were observed in terms of average care burden scores ( p < 0.05) (Table 5 ). Likewise, the average changes in care burden scores between the intervention and control groups showed no statistically significant differences ( p < 0.05) (Table 6 ). The Cohen’s d effect size for the difference in caregiving burden between the intervention and control groups was 0.366, indicating a moderate effect of the intervention on caregiving burden, although not statistically significant (Table 5 ).
This study aimed to evaluate the impact of religious spiritual care training on the spiritual health and care burden experienced by elderly family caregivers in Bushehr during the COVID-19 pandemic. The findings of this study suggest that a religious and spiritual intervention approach can effectively promote existential health and overall spiritual well-being. However, it was observed that this approach did not yield a notable impact on religious health or care burden. The Scores for existential health and overall spiritual health increased in the intervention group after the training, while they decreased in the control group. The mean change in religious health scores between the two groups did not reach statistical significance. These findings are consistent with the study conducted by Sayyadi et al. (2018) [ 26 ], who also observed an increase in spiritual health following a religious psychotherapy intervention. In this study, most family caregivers in the experimental and control groups initially demonstrated moderate to high levels of spiritual health. Similarly, Sayyadi et al. (2018) found higher spiritual health scores in medical and nursing students compared to other student populations. To explain and interpret the consistent findings regarding the positive effects of spiritual health on caregivers in the study by Sayyadi et al. and the current study on the impact of religious spiritual care training on elderly family caregivers, we can consider several factors that may contribute to these findings: (1) Spiritual health is often associated with providing a sense of support, purpose and coping mechanisms during challenging times. Caregivers facing the stress and demands of caregiving may benefit from a solid spiritual foundation to help them navigate their roles and find meaning in their experiences. Studies may have highlighted the role of spiritual health as a resource for caregivers to cope with the emotional and psychological challenges they face. (2) Spiritual health can help to build resilience and foster hope in individuals, including caregivers. By nurturing their spiritual well-being, caregivers may develop a sense of resilience that enables them to cope with adversity and maintain a positive outlook. Studies may have observed the positive impact of spiritual health on caregivers’ resilience and hope, leading to improved well-being and outcomes. (3) Spiritual health is often linked to personal growth and making sense of one’s experiences [ 27 ]. Caregivers possessing a robust spiritual foundation may engage in meaning-making processes, facilitating the discovery of purpose and significance within their caregiving journey. Studies may have underscored the role of spiritual health in promoting personal growth and facilitating meaning-making among caregivers. These factors, alongside the consistent focus on spiritual health across studies, provide a robust framework for understanding the positive impact of spiritual health on caregivers. Recognizing the importance of spiritual well-being within the broader context of caregiver health, and integrating interventions that specifically address spiritual needs, can contribute to improved outcomes and well-being for caregivers. This is supported by the findings of both Sayyadi et al. and the present study. It is important to note that the religious health scores did not increase after the intervention in the current study. The intervention, centered on religious and spiritual care training, had a significant impact on both existential well-being and overall spiritual health. The caregiver survey of palliative care patients will likely target different aspects of spiritual well-being, such as hope and general well-being. In interpreting these results, it is essential to consider the unique components of each intervention and how they may have influenced different aspects of spiritual health. On the other hand, Casalerio et al. (2024) in the study: Promoting Spiritual Coping of Family Caregivers of an Adult Relative with Severe Mental Illness: Development and Test of a Nursing Intervention, reported that the spiritual and religious intervention for caregivers increased their spiritual health dimension and their religious dimension [ 28 ]. These contrasting religious findings with the current study suggest that the effectiveness of religious and spiritual interventions may vary depending on the specific focus and approach of the intervention. Caregivers’ responses to such interventions may be influenced by factors such as the nature of the caring role, the context of the carer’s condition, and individual preferences regarding spirituality and religiosity. Further research and tailored interventions may be needed to address the diverse spiritual needs of caregivers in different care contexts.
Regarding the care burden, the results of the current study demonstrated no statistically significant differences in the average care burden scores within and between the groups. This result contrasts with previous studies by Polat et al. (2024), Xavier et al. (2023) [ 13 , 29 ], Partovirad et al. (2024) [ 11 ], Hekmatpour and colleagues (2018) [ 30 ], Shoghi et al. (2018) [ 31 ], which showed reductions in the care burden following intervention models and the current study care burden result align with previous studies by Khalili et al. (2024) [ 32 ], Salmoirago-Blotcher et al. (2016) [ 33 ], and Karadag Arli (2017) [ 34 ]. One of the reasons why the present study did not show the same effect of spiritual and religious interventions in reducing caregiver burden as similar studies have shown is probably due to the high caregiver burden in the relevant situation. In the present study, caregiver burden had increased due to the conditions of the Corona pandemic, and reducing caregiver burden may require more extended, and more social interventions. One potential explanation for the lack of reduction in care burden scores in the current study is the influence of social interaction theory and attachment theory. These theoretical frameworks emphasize the significance of the dynamic interplay between caregiver and care recipient, particularly highlighting the role of mutual appreciation and non-violent communication in mitigating caregiver burden [ 35 ]. The physical and mental conditions of care recipients, coupled with their inability to engage in appropriate interactions with caregivers during the COVID-19 crisis, may have intensified the care burden. Furthermore, a review of similar studies reveals that most interventions aimed at reducing care burden were conducted over longer periods than our study. These studies typically involved a higher number of sessions, ranging from 8 to 12 (e.g., Mohammadi and Babaei (2018) [ 36 ], Rahgooy et al. (2018) [ 37 ], Sotoudeh et al. (2018) [ 38 ] and Salehinejad et al. (2017) [ 39 ] Consequently, the shorter duration and fewer sessions in our study may have limited the effectiveness of the intervention in reducing the care burden. Additionally, the limitations imposed by social distancing measures may have exacerbated the needs of elderly individuals, leading to an increased caregiver burden. Furthermore, a review of similar studies reveals that most interventions aimed at reducing care burden were conducted over more extended periods than our study. These studies typically involved a higher number of sessions, ranging from 8 to 12 (e.g., Mohammadi and Babaei (2018) [ 30 ], Rahgooy et al. (2018) [ 32 ], Sotoudeh et al. (2018) [ 39 ] and Salehinejad et al. Consequently, the shorter duration and fewer sessions in our study may have limited the effectiveness of the intervention in reducing the care burden.
Limitations
This study had limitations. The limitations imposed by the pandemic, including the need for social distancing, made it impossible to conduct face-to-face training sessions and deprived participants and carers of the opportunity for close, face-to-face communication during the spiritual and religious intervention. This limitation may have affected the participants’ internal beliefs, emotions, and motivations. The restrictions imposed by the pandemic, through the utilization of routine telephone communications and collaboration with pertinent academic staff, exemplify adaptability and ingenuity in maintaining communication with participants. This multi-channel approach may have helped to ensure continued engagement and support for participants throughout the intervention. Despite the challenges posed by the lack of face-to-face communication, the study managed to keep participants engaged through alternative means. The regular phone calls and coordination with the professors may have fostered a sense of connection and support, potentially enhancing participants’ overall experience and engagement with the intervention. The lack of face-to-face interaction during the spiritual and religious intervention may have limited the depth of participants’ engagement and the impact on their internal beliefs and motivations. This limitation could affect the validity of the study findings, as face-to-face communication is often crucial for building trust and rapport in interventions of this nature. The short duration of the intervention and the constraints imposed by the pandemic may have limited the generalizability of the study results. Further research utilizing more extended intervention periods and more diverse participant groups may enhance the generalizability of the findings to a broader population. Utilizing virtual platforms for interactive sessions and group discussions could facilitate the replication of the advantages of face-to-face communication and cultivate a sense of community among participants. Conducting long-term follow-up studies to track the sustained effects of spiritual and religious interventions on caregiver burden could provide valuable insights into the lasting impact and effectiveness of the intervention over time.
Based on the study, the results were mixed. The religious and spiritual intervention was effective in improving existential health and overall spiritual health but did not have a significant impact on religious health and caregiving burden. The training in religious and spiritual care was determined to be effective in enhancing the existential well-being of elderly family caregivers, as evidenced by an increase in their sense of meaning, purpose, and fulfillment in the caregiving role. The intervention demonstrated effectiveness in improving caregivers’ overall spiritual health, suggesting positive outcomes in terms of emotional well-being, connectedness, and resilience. Notwithstanding the favorable outcomes in existential and general spiritual well-being, the intervention did not demonstrate a notable impact on religious well-being and caregiver burden, underscoring domains that may warrant further investigation and the development of alternative intervention strategies. It is crucial to recognize the intricate nature of caregiving dynamics and the various ways in which spirituality and religion can impact the well-being of caregivers. The result of the study indicates that integrating religious and spiritual care training could effectively enhance the existential and holistic spiritual well-being of elderly family caregivers. Practitioners and caregivers can utilize this intervention to foster a greater sense of meaning and spiritual well-being within the caregiving context. In addition, the study highlights the importance of personalized interventions that consider individual differences in spiritual beliefs and coping strategies. In conclusion, while the religious and spiritual intervention showed promising results in improving certain aspects of the spiritual health of elderly family caregivers in Bushehr, further research is needed to address the nuances of religious health and care burden. By carefully considering these key findings and implications, practitioners and researchers can tailor interventions to better support caregivers’ holistic well-being in the face of challenges such as the COVID-19 pandemic.
Data availability
The datasets used and analyzed during the current study are available from the corresponding author on reasonable request.
Na Zhu DZ, Wang W, Li X, Yang B, Song J, Zhao X, Huang B, Shi W, Lu R, Niu P, Zhan F. A novel coronavirus from patients with pneumonia in China, 2019. 2020:727–33. https://www.nejm.org/doi/pdf/ https://doi.org/10.1056/NEJMoa2001017
Dhama K, Patel SK, Kumar R, Rana J, Yatoo MI, Kumar A, et al. Geriatric population during the COVID-19 pandemic: problems, considerations, exigencies, and beyond. Front Public Health. 2020;8:574198. https://doi.org/10.3389/fpubh.2020.574198
Article PubMed PubMed Central Google Scholar
Zhang J, Wang J, Liu H, Wu C. Association of dementia comorbidities with caregivers’ physical, psychological, social, and financial burden. BMC Geriatr. 2023;23(1):60. https://doi.org/10.1186/s12877-023-03774-9
Shahid ZKR, McClafferty B, Kepko D, Ramgobin D, Patel R. COVID-19 and older adults: what we know. J Am Geriatr Soc. 2020;68(5):926–9. https://doi.org/.1111/jgs.16472 . Epub 2020 Apr 20. PMID: 32255507; PMCID: PMC7262251.
Moradi M, Navab E, Sharifi F, Namadi B, Rahimidoost M. The effects of the COVID-19 pandemic on the elderly: a systematic review. J Salmand: Iran J Ageing. 2021;16(1):2–29. https://doi.org/10.3389/fpubh.2020.574198
Article Google Scholar
Park SS. Caregivers’ mental health and somatic symptoms during COVID-19. J Gerontol B Psychol Sci Soc Sci. 2021;76(4). https://doi.org/10.1093/geronb/gbaa121 . PMID: 32738144; PMCID: PMC7454918.
Malmir S, Navipour H, Negarandeh R. Exploring challenges among Iranian family caregivers of seniors with multiple chronic conditions: a qualitative research study. BMC Geriatr. 2022;22(1):270. https://doi.org/10.1186/s12877-022-02881-3
Phillips R, Durkin M, Engward H, Cable G, Iancu M. The impact of caring for family members with mental illnesses on the caregiver: a scoping review. Health Promot Int. 2023;38(3). https://doi.org/10.1093/heapro/daac049 . PMID: 35472137; PMCID: PMC10269136.
jaafari zadeh H, SmS K, rahgozar M. Health care status of elderly people with disabilities in District 13 of Tehran Municipality and their problems. Journal of Urmia Nursing And Midwifery Faculty. 2006;4(2):67–81.
Nafei ARV, Ghafori R, Khalvati M, Eslamian A, Sharifi D, et al. Death anxiety and related factors among older adults in Iran: findings from a National Study. Salmand Iran J Ageing. 2024;19(1):144–57. https://doi.org/10.32598/sija.2023.1106.1
Partovirad M, Rizi S, Amrollah Majdabadi Z, Mohammadi F, Hosseinabadi A, Nikpeyma N. Assessing the relationship between spiritual intelligence and care burden in family caregivers of older adults with chronic diseases. 2024. https://doi.org/10.21203/rs.3.rs-4343106/v1
Davarinia Motlagh Quchan A, Mohammadzadeh F, Mohamadzadeh Tabrizi Z, Bahri N. The relationship between spiritual health and COVID-19 anxiety among nurses: a national online cross-sectional study. Sci Rep. 2024;14(1):16356. https://doi.org/10.1038/s41598-024-67523-7
Article CAS PubMed PubMed Central Google Scholar
Türkben Polat H, Kiyak S. Spiritual well-being and care burden in caregivers of patients with breast cancer in Turkey. J Relig Health. 2023;62(3):1950–63. https://doi.org/10.1007/s10943-022-01695-2 . Epub 2022 Dec 5. PMID: 36469230; PMCID: PMC9734401.
Article PubMed Google Scholar
Francesco Chirico KB, Batra R, Ferrari G, Crescenzo P. Spiritual well-being and burnout syndrome in healthcare: asystematic review. School of Medicine Faculty Publications. 2023;15(3). https://doi.org/10.19204/2023/sprt2
Rezaei H, Niksima SH, Ghanei Gheshlagh R. Burden of care in caregivers of Iranian patients with chronic disorders: a systematic review and meta-analysis. Health Qual Life Outcomes. 2020;18(1):261. https://doi.org/10.1186/s12955-020-01503-z . PMID: 32746921; PMCID: PMC7398060.
Kurtgöz A, Edis EK. Spiritual care from the perspective of family caregivers and nurses in palliative care: a qualitative study. BMC Palliat Care. 2023;22(1):161. https://doi.org/10.1186/s12904-023-01286-2
Hosseini MA, Mohammadzaheri S, Fallahi Khoshkenab, Mohammadi Shahbolaghi M, Reza Soltani F, Sharif Mohseni M. Effect of mindfulness program on caregivers’ strain on Alzheimer’s disease caregivers. Salmand: Iran J Ageing. 2016;11(3):448–55. https://doi.org/10.21859/sija-1103448
Mahdavi B, Fallahi-Khoshknab M, Mohammadi F, Hosseini MA, Haghi M. Effects of spiritual group therapy on caregiver strain in-home caregivers of the elderly with Alzheimer’s disease. Arch Psychiatr Nurs. 2017;31(3):269–73. https://doi.org/10.1016/j.apnu.2016.12.003
Moeini M, Ghasemi TMG, Yousefi H, Abedi HJIjon, Research M. The effect of spiritual care on the spiritual health of patients with cardiac ischemia. 2012;17(3):195. https://pubmed.ncbi.nlm.nih.gov/23833611/
Parvizi S, Allahbakhshian M, Jafarpour allavi M, Haghani H. The relationship between spiritual health and quality of life in multiple sclerosis patients. Zahedan J Res Med Sci. 2000;12(3):29–33. https://civilica.com/doc/963361/
Google Scholar
Hamdami M, GHasemi jovineh R, Zahrakar K, Karimi K. The role of spiritual health and mindfulness in students’ psychological capital. Res Med Educ. 2015;8(2):2736. https://doi.org/10.18869/acadpub.rme.8.2.27
Abbasi A, Ashraf Rezaei N, Asayesh H, Shariati A, Rahmani H, Molaei E, et al. Relationship between caregiving pressure and coping skills of caregivers of patients undergoing hemodialysis. Urmia School Nurs Midwifery. 2011;10(4):533–9.
Pouladi S ss, Bahreyni M, Bagherzadeh R. The effect of spiritual-religious psychotherapy on self-concept and sense of coherence in cancer patients of Bushehr City, 2017–2018. Bushehr University of Medical Sciences.
Modarres MAS. Effect of religion-based spirituality education on happiness of postmenopausal women: an interventional study. Health Spiritual Med Ethics. 2021;8(1):44–54. https://doi.org/10.29252/jhsme.8.1.44
Jahdi F, mA B, Mahani M, Behboudi Moghaddam Z. The effect of prenatal group care on the empowerment of pregnant women. Payesh. 2014;13(2):229–34.
Sayyadi M, Sayyad S, Vahabi A, Vahabi B, Noori B, Amani M. Evaluation of the spiritual health level and its related factors in the students of Sanandaj Universities, 2015. Shenakht J Psychol Psychiatry. 2019;6(1):1–10. https://doi.org/10.22122/cdj.v6i3.332
Koburtay TJD, Aljafari A. Religion, spirituality, and well-being: a systematic literature review and futuristic agenda. Bus Ethics Environ Responsib. 2023;32(1):41–57. https://doi.org/10.1111/beer.12478
Casaleiro TMH, Caldeira S. Promoting spiritual coping of family caregivers of an adult relative with severe mental illness development and test of a nursing intervention. Healthcare. 2024;12(13). https://doi.org/10.3390/healthcare12131247
Xavier FT, Esperandio MRG. Spirituality and caregiver burden of people with intellectual disabilities: an empirical study. Int J Latin Am Religions. 2023;7(1):17–35. https://doi.org/10.1007/s41603-023-00196-8
Hekmatpour DB, EM.Dehkordi LM. The effect of patient care education on the burden of care and the quality of life of caregivers of stroke patients. Multidisciplinary Healthc. 2019;12(2):7–11. https://doi.org/10.2147/JMDH.S232970 . PMID: 30936715; PMCID: PMC6430991.
Shoghi MN, Seyedfatemi., Shahbazi B. M S. The effect of family-center empowerment model(FCEM) on the care burden of the patient of children diagnosed with cancer. 2019;20(6):1757–68. https://doi.org/10.31557/APJCP.2019.20.6.1757
Khalili Z, Habibi E, Kamyari N, Tohidi S, Yousofvand V. Correlation between spiritual health, anxiety, and sleep quality among cancer patients. Int J Afr Nurs Sci. 2024;20:100668. https://doi.org/10.1016/j.ijans.2024.100668
Salmoirago-Blotcher EFG, Leung K, Volturo G, Boudreaux E, Crawford S, et al. An exploration of the role of religion/spirituality in the promotion of physicians’ well-being in emergency medicine. Prev Med Rep. 2016;1(3):189–95. https://doi.org/10.1016/j.pmedr.2016.01.009 . PMID: 27419014; PMCID: PMC4929145.
Karadag Arli SBA, Erisik E. An investigation of the relationship between nurses’ views on spirituality and spiritual care and their level of burnout. J Holist Nurs. 2017;35(3):214–20. https://doi.org/10.1177/0898010116652974 . Epub 2016 May 30. PMID: 27241132.
Jafari M, Alipour F, Raheb G, Mardani M. Perceived stress and burden of care in elderly caregivers: the moderating role of resilience Salmand. Iran J Ageing. 2022;17(1):62–75. https://doi.org/10.32598/sija.2021.2575.2
Mohammadi F, Babaie M. The impact of participation in support groups on spiritual health and care burden of elderly patients with Alzheimer’s family caregivers. Iran J Ageing. 2012;12(19):29–37. https://salmandj.uswr.ac.ir/article-1-374-en.html
Rahgooy AF, Sojoudi M. T. Investigating the effect of the empowerment program on the care burden of mothers with children with phenylketonuria and the level of phenylalanine in the children’s serum. TEHRAN: University of Rehabilitation Sciences and Social Welfare; 2016.
Sotoudeh RPS, Alavi M. The effect of a family-based training program on the care burden of family caregivers of patients undergoing hemodialysis. Iran J Nurse Midwifery Res. 2019;24(2):144. https://doi.org/10.4103/ijnmr.IJNMR_93_18 . PMID: 30820227; PMCID: PMC6390430.
Salehi Nejad S, Azami M, Motamedi F, Bahaadinbeigy K, Sedighi B, Shahesmaili A. The effect of web-based information intervention in caregiving burden in caregivers of patients with dementia. J J Health Biomedical Inf. 2017;4(3):181–91. https://www.sid.ir/fa/VEWSSID/J_pdf/3007513960302.pdf
Download references
Acknowledgements
We would like to express our gratitude to the Student Research Committee, the Persian Gulf Martyrs Hospital’s Clinical Research Development Center, and all the elderly caregivers who participated in this research, as their contributions were invaluable.
Research expenses by the vice president of research and the student research committee of Bushehr University of Medical Sciences, Iran, have been paid.
Author information
Authors and affiliations.
Bushehr University of Medical Sciences, Bushehr, Islamic Republic of Iran
Afifeh Qorbani
Nursing and Midwifery Faculty of Bushehr, University of Medical Sciences, Bushehr, Islamic Republic of Iran
Shahnaz Pouladi & Razieh Bagherzadeh
Persian Gulf Tropical Medicine Research Center, Persian Gulf Biomedical Sciences Research Institute, Bushehr University of Medical Sciences, Bushehr, Islamic Republic of Iran
Akram Farhadi
You can also search for this author in PubMed Google Scholar
Contributions
AQ: Student. Author of the article. SH: Supervisor, the editor, preparation of educational packages. AF.: Consultant professor, the editor, preparation of educational packages. RB: Consultant professor of statistics department, the editor.
Corresponding author
Correspondence to Shahnaz Pouladi .
Ethics declarations
Ethical approval and consent to participate.
The research has an ethics code number of IR.BPUMS.REC.1399.042 from the Research Vice-Chancellor of Bushehr University of Medical Sciences. All study participants provided written informed consent.
Consent for publication
Not Applicable.
Competing interests
The authors declare no competing interests.
Additional information
Publisher’s note.
Springer Nature remains neutral with regard to jurisdictional claims in published maps and institutional affiliations.
Rights and permissions
Open Access This article is licensed under a Creative Commons Attribution-NonCommercial-NoDerivatives 4.0 International License, which permits any non-commercial use, sharing, distribution and reproduction in any medium or format, as long as you give appropriate credit to the original author(s) and the source, provide a link to the Creative Commons licence, and indicate if you modified the licensed material. You do not have permission under this licence to share adapted material derived from this article or parts of it. The images or other third party material in this article are included in the article’s Creative Commons licence, unless indicated otherwise in a credit line to the material. If material is not included in the article’s Creative Commons licence and your intended use is not permitted by statutory regulation or exceeds the permitted use, you will need to obtain permission directly from the copyright holder. To view a copy of this licence, visit http://creativecommons.org/licenses/by-nc-nd/4.0/ .
Reprints and permissions
About this article
Cite this article.
Qorbani, A., Pouladi, S., Farhadi, A. et al. The impact of religious spiritual care training on the spiritual health and care burden of elderly family caregivers during the COVID-19 pandemic: a field trial study. BMC Nurs 23 , 584 (2024). https://doi.org/10.1186/s12912-024-02268-2
Download citation
Received : 29 May 2024
Accepted : 16 August 2024
Published : 24 August 2024
DOI : https://doi.org/10.1186/s12912-024-02268-2
Share this article
Anyone you share the following link with will be able to read this content:
Sorry, a shareable link is not currently available for this article.
Provided by the Springer Nature SharedIt content-sharing initiative
- Caregiver burden
- Spirituality
BMC Nursing
ISSN: 1472-6955
- General enquiries: [email protected]
share this!
August 28, 2024
This article has been reviewed according to Science X's editorial process and policies . Editors have highlighted the following attributes while ensuring the content's credibility:
fact-checked
peer-reviewed publication
trusted source
Study shows pollution affects the growth and behavior of aquatic organisms
by University of Jyväskylä

Research at the University of Jyväskylä, Finland, reveals that size-based selection, as happens in fishing, may impact the stress tolerance of fish, which in turn has a significant impact on the condition and coping of fish in changing environments.
The study proved that long-term exposure to manganese sulfate (MnSO 4 ), which is a common aquatic pollutant from mining, hampers the growth and alters the behavior of zebra fish. The study is published in Ecology and Evolution .
As we know, fishing is focused on middle-sized and large individuals through lower limits and fishing equipment restrictions, which often favors a fast life-cycle in target species. JYU researchers found that zebra fish with different life-history traits react differently to chronic exposure to manganese sulfate (MnSO 4 ).
"In our research, we concentrated on two life-history types: fast-growing fish characterized by rapid juvenile growth, early maturation, and smaller size, versus slowly growing fish characterized by slower juvenile growth, later maturation and greater size," explains Academy Research Fellow Silva Uusi-Heikkilä from the University of Jyväskylä.
Considering fish life-history types in conservation
The findings show that MnSO 4 exposure slows down the growth of zebra fish and lowers their condition factor, which is an indicator of well-being. However, the scope of impacts varies by life-history types.
Fish with the fast life-history strategy were more sensitive to MnSO 4 , as they grew more slowly when exposed to high concentrations. In addition, they had a lower condition factor, and they ate less than their conspecifics with a slow life-history strategy.
"Our findings show that manganese sulfate may deteriorate the growth and stress coping of fish, and that variation in life-history traits may play a crucial role in modifying the stress tolerance of individuals," Uusi-Heikkilä says.
"This study underlines that it is important to take population life-history traits into account in environmental risk assessments and protection strategies and also to consider the combined effects of fishing and environmental factors on fish populations."
The study provides valuable information on how pollutants like manganese sulfate ending up in the aquatic environment can affect aquatic organisms in various ways based on their life-history strategies.
"These findings have significant effects when we wish to get insight into the viability and adaptability of fish populations in polluted habitats," Uusi-Heikkilä states.
Journal information: Ecology and Evolution
Provided by University of Jyväskylä
Explore further
Feedback to editors

Labor day crowds temporarily impact local streams, research shows
43 minutes ago

New Sisyphus cooling technique could enhance precision of atomic clocks

Researchers map 50,000 of DNA's mysterious 'knots' in the human genome
3 hours ago

Land-sea 'tag-team' devastated ocean life millions of years ago, reveal scientists

Can fungi turn food waste into the next culinary sensation?

Global timber supply threatened as climate change pushes cropland northwards

Team using AI finds a cheaper way to make green hydrogen
7 hours ago

Ancient sea cow that was attacked by both a primeval crocodile and shark sheds new light on prehistoric food chains
8 hours ago

How beetle juice led to the discovery of a virus and solved the mystery of a superworm die-off
15 hours ago

Framework for solving parabolic partial differential equations could guide computer graphics and geometry processing
Relevant physicsforums posts, will cryosleep ever be a reality, any suggestions to dampen the sounds of a colostomy bag.
16 hours ago
The predictive brain (Stimulus-Specific Error Prediction Neurons)
Aug 27, 2024
Any stereo audio learning resources for other languages?
Aug 25, 2024
Cannot find a comfortable side-sleeping position
Therapeutic interfering particle.
Aug 24, 2024
More from Biology and Medical
Related Stories

Antidepressant pollution is rewiring fish behavior and reproduction, biologists reveal

Fishing can disrupt mating systems
May 8, 2020

Polar fish are less likely to die early, so they prioritize growth over reproduction
May 25, 2023

Commercial fishing in reduced fish populations may cause genetic changes
Dec 14, 2021

Artificial light is a deadly siren song for young fish
Jul 3, 2024

Unselective fishing could reduce fish diversity and homogenize assemblage structure in lakes
Jun 22, 2022
Recommended for you

A price on their heads? Implications of international trade for African hornbills in Cameroon
21 hours ago

Love is blind for male fruit flies, who will choose sex over safety

Field observations reveal microplastic transfer in marine planktonic food chains
22 hours ago

New insights into Little Penguins' sensitivity to noise
23 hours ago

Some bats are surviving and thriving with blood sugar levels that would be lethal for other mammals
Aug 28, 2024
Let us know if there is a problem with our content
Use this form if you have come across a typo, inaccuracy or would like to send an edit request for the content on this page. For general inquiries, please use our contact form . For general feedback, use the public comments section below (please adhere to guidelines ).
Please select the most appropriate category to facilitate processing of your request
Thank you for taking time to provide your feedback to the editors.
Your feedback is important to us. However, we do not guarantee individual replies due to the high volume of messages.
E-mail the story
Your email address is used only to let the recipient know who sent the email. Neither your address nor the recipient's address will be used for any other purpose. The information you enter will appear in your e-mail message and is not retained by Phys.org in any form.
Newsletter sign up
Get weekly and/or daily updates delivered to your inbox. You can unsubscribe at any time and we'll never share your details to third parties.
More information Privacy policy
Donate and enjoy an ad-free experience
We keep our content available to everyone. Consider supporting Science X's mission by getting a premium account.
E-mail newsletter

IMAGES
COMMENTS
The impact score (IS), also denoted as Journal impact score (JIS), of an academic journal is a measure of the yearly average number of citations to recent articles published in that journal. It is based on Scopus data. Impact Score is defined as the ratio of the number of citations a journal receives in the latest two years (Including the year ...
The impact factor (IF) is a measure of the frequency with which the average article in a journal has been cited in a particular year. It is used to measure the importance or rank of a journal by calculating the times its articles are cited.
The impact factor (IF) or journal impact factor (JIF) of an academic journal is a scientometric index calculated by Clarivate that reflects the yearly mean number of citations of articles published in the last two years in a given journal, as indexed by Clarivate's Web of Science.. As a journal-level metric, it is frequently used as a proxy for the relative importance of a journal within its ...
<link rel="stylesheet" href="/public/styles.29276a7c2f2290b7.css">
They are used to evaluate the quality of a journal, as well as to determine the influence of your research in your field. In this series of free modules, we walk you through some of the key players in metrics. Measure article, author and journal influences using CiteScore metrics, h-index, article-level metrics, SNIP, SJR, impact factor and more.
Google Scholar Metrics provide an easy way for authors to quickly gauge the visibility and influence of recent articles in scholarly publications. Scholar Metrics summarize recent citations to many publications, to help authors as they consider where to publish their new research. To get started, you can browse the top 100 publications in ...
The journal impact factor (JIF), as calculated by Clarivate Analytics, is a measure of the average number of times articles from a two-year time frame have been cited in a given year, according to citations captured in the Web of Science database. The 2023 JIF (released in 2024), for example, was calculated as follows:
Journal Impact Factor (JIF) Journal Impact Factor (JIF) is calculated by Clarivate Analytics as the average of the sum of the citations received in a given year to a journal's previous two years of publications (linked to the journal, but not necessarily to specific publications) divided by the sum of "citable" publications in the previous two years.
A Journal Impact Factor of 2.5 means that, on average, the articles published one or two years ago have been cited two and a half times. The citing works may be articles published in the same journal.
The impact factor (IF) is a measure of the frequency with which the average article in a journal has been cited in a particular year. It is used to measure the importance or rank of a journal by calculating the times its articles are cited.
The journal Impact Factor is an index that measures how often a journal's articles are cited in other research. This is calculated by the number of citations received by articles published in that journal during the two preceding years, divided by the total number of articles published in that journal during the two preceding years.
Impact factor, or Journal Impact Factor, is a measure of the frequency with which the "average article" published in a given scholarly journal has been cited in a particular year or period and is often used to measure or describe the importance of a particular journal to its field.Impact factor was originally developed by Eugene Garfield, the founder of Institute of Scientific Information ...
Metric Name. Metric Source. Metric Description. Journal Impact Factor. Clarivate - Web of Science. The Journal Impact Factor is defined as all citations to the journal in the current JCR year to items published in the previous two years, divided by the total number of scholarly items (these comprise articles, reviews, and proceedings papers) published in the journal in the previous two years.
How. Follow these steps to find the Impact Factor of a journal: Search for a journal using the 'Journal/book title' field on the ScienceDirect homepage or browse journal titles by selecting ' Journals & Books ' in the top right corner. Click the journal title to navigate to the journal's home page. The Impact Factor and Journal CiteScore ...
Impact factor is commonly used to evaluate the relative importance of a journal within its field and to measure the frequency with which the "average article" in a journal has been cited in a particular time period. Journal which publishes more review articles will get highest IFs. Journals with higher IFs believed to be more important than ...
Disciplines vary widely in the amount of research output, the number of citations that are normally included in papers, and the tendency of a discipline to cite recent articles. 6 For example, Acta Poetica focuses on literary criticism. Its impact factor would be a poor measure of the journal's influence.
Scopus provide citation counts for articles indexed within it (limited to article written in 1996 and after). It indexes o ver 15,000 journals from over 4,000 international publishers across the disciplines. To find the citation counts to your own articles: Once in Scopus, click on the Author search tab. Enter the name of the author in the ...
Journal metrics are used to identify key journals in a research field. This identification may be most useful to authors who are considering which journals to submit manuscripts to for future publication. The Impact Factor may be the most familiar metric in academics. Eugene Garfield of Thomson Scientific first introduced this idea in the 1950s.
How impact factors are calculated. The IF for a particular year is calculated as the ratio of the total times the journal's articles were cited in the previous 2 years to the total citable items it published in those 2 years. For example, in 2018, Nature had an IF of 43.070. That's a good journal impact factor.
B: Number of articles published in 2016 & 2017. C: A/B= 2018 Impact Factor. For instance, the current impact factor of Journal of the Academy of Marketing Science is 9.36. This means, on average, the papers published in the journal in 2016 and 2017 received roughly 9 citations each in 2018. See our introductory guide to Research Impact Metrics.
According to Journal Citation Reports (JCR), an impact factor is a ratio focusing on original research.. Impact factor = # of citations to all items published in that journal in the past two years (divided by) # of articles and reviews published over those past two years referencing those citations
Physical Therapy, Sports Therapy and Rehabilitation. Public Health, Environmental and Occupational Health. Renewable Energy, Sustainability and the Environment. Only Open Access Journals Only SciELO Journals Only WoS Journals. Display journals with at least. Citable Docs. (3years) Apply. Download data. 1 - 50 of 29165.
Article. The Impact Factor is calculated by dividing the number of citations in the Journal Citation Reports year (the numerator) by the total number of citable items published in the two previous years (the denominator). The 2021 Journal Citation Reports equation reads: Number of citable items published in 2018 and 2019.
Understanding Impact Factors. Clarivate determines journal impact factors using a ratio that measures how often an article is cited in a year against the total number of citable content piece published in the previous two-year period. The ISI uses a quality-driven process to determine which journals receive impact factors.
To the best of our knowledge, only one two-sample MR study investigated the effect of childhood maltreatment on ADHD indicating an increasing risk [12]. Evidence from MR studies for other ...
The research employs the Tobit model to examine the factors that impact energy efficiency. The precise methods and processes are depicted in Figure 1. 2.1. Three-Stage DEA Model Construction ... Su, Y.; Lin, Y.N. Research on the impact of government subsidies on R&D investment of new energy enterprises. Sci. Manag. Res. 2021, 39, 102-110 ...
Global research shows that study habits impact academic performance and are the most important predictor of it . It is difficult for medical students to organize and learn a lot of information, and they need to employ study skills to succeed [1, 2, 5, 13]. Different lifestyle and social factors could affect students' academic performance.
This first article in a series presents the development of the method and a cross-country comparison of the contextual factors that enable PAEHR access and use. Methods: The dimensions of the framework for sociotechnical analysis were thoroughly discussed and extended in a series of workshops with international stakeholders, all being eHealth ...
Family caregiving is associated with many physical and psychological problems for caregivers, but the effect of spiritual support on reducing their issues during a crisis is also the subject of research. The study aims to examine the impact of religious spiritual care training on the spiritual health and care burdens of elderly family caregivers during the COVID-19 pandemic.
Research at the University of Jyväskylä, Finland, reveals that size-based selection, as happens in fishing, may impact the stress tolerance of fish, which in turn has a significant impact on the ...