Thank you for visiting nature.com. You are using a browser version with limited support for CSS. To obtain the best experience, we recommend you use a more up to date browser (or turn off compatibility mode in Internet Explorer). In the meantime, to ensure continued support, we are displaying the site without styles and JavaScript.
- View all journals
- Explore content
- About the journal
- Publish with us
- Sign up for alerts
- Review Article
- Published: 20 July 2020

Diet and exercise in the prevention and treatment of type 2 diabetes mellitus
- Faidon Magkos ORCID: orcid.org/0000-0002-1312-7364 1 ,
- Mads F. Hjorth ORCID: orcid.org/0000-0001-9440-2737 1 &
- Arne Astrup ORCID: orcid.org/0000-0001-8968-8996 1
Nature Reviews Endocrinology volume 16 , pages 545–555 ( 2020 ) Cite this article
18k Accesses
224 Citations
120 Altmetric
Metrics details
- Type 2 diabetes
Evidence from observational studies and randomized trials suggests that prediabetes and type 2 diabetes mellitus (T2DM) can develop in genetically susceptible individuals in parallel with weight (that is, fat) gain. Accordingly, studies show that weight loss can produce remission of T2DM in a dose-dependent manner. A weight loss of ~15 kg, achieved by calorie restriction as part of an intensive management programme, can lead to remission of T2DM in ~80% of patients with obesity and T2DM. However, long-term weight loss maintenance is challenging. Obesity and T2DM are associated with diminished glucose uptake in the brain that impairs the satiating effect of dietary carbohydrate; therefore, carbohydrate restriction might help maintain weight loss and maximize metabolic benefits. Likewise, increases in physical activity and fitness are an important contributor to T2DM remission when combined with calorie restriction and weight loss. Preliminary studies suggest that a precision dietary management approach that uses pretreatment glycaemic status to stratify patients can help optimize dietary recommendations with respect to carbohydrate, fat and dietary fibre. This approach might lead to improved weight loss maintenance and glycaemic control. Future research should focus on better understanding the individual response to dietary treatment and translating these findings into clinical practice.
Studies show that weight loss can produce remission of type 2 diabetes mellitus (T2DM) in a dose-dependent manner.
In patients with T2DM and obesity, weight loss of ~15 kg, achieved by an intensive management programme involving calorie restriction, can lead to remission of T2DM in ~80% of individuals.
Long-term maintenance of weight loss and metabolic health in people who have undergone intensive lifestyle intervention is challenging.
Carbohydrate restriction might help maintain weight loss and maximize metabolic benefits.
When combined with calorie restriction and weight loss, increases in physical activity and fitness are an important contributor to T2DM remission.
Preliminary work suggests that pretreatment glycaemic status could be used to stratify patients in order to optimize dietary recommendations.
This is a preview of subscription content, access via your institution
Access options
Access Nature and 54 other Nature Portfolio journals
Get Nature+, our best-value online-access subscription
24,99 € / 30 days
cancel any time
Subscribe to this journal
Receive 12 print issues and online access
195,33 € per year
only 16,28 € per issue
Buy this article
- Purchase on SpringerLink
- Instant access to full article PDF
Prices may be subject to local taxes which are calculated during checkout
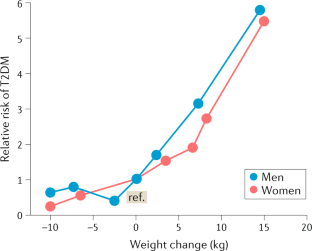
Similar content being viewed by others
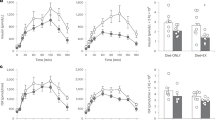
Dietary weight loss-induced improvements in metabolic function are enhanced by exercise in people with obesity and prediabetes
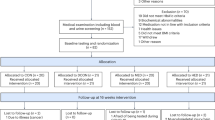
Effects of different doses of exercise and diet-induced weight loss on beta-cell function in type 2 diabetes (DOSE-EX): a randomized clinical trial
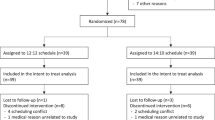
Effect of time restricted eating on body weight and fasting glucose in participants with obesity: results of a randomized, controlled, virtual clinical trial
International Diabetes Federation. IDF Diabetes Atlas 9th edn (International Diabetes Federation, 2019).
Zhu, Y. et al. Racial/ethnic disparities in the prevalence of diabetes and prediabetes by BMI: patient outcomes research to advance learning (PORTAL) multisite cohort of adults in the U.S. Diabetes Care 42 , 2211–2219 (2019).
Article PubMed PubMed Central Google Scholar
Magkos, F. Metabolically healthy obesity: what’s in a name? Am. J. Clin. Nutr. 110 , 533–539 (2019). A review of the dissociation between excess body weight and metabolic dysfunction .
Article PubMed Google Scholar
Willett, W. C., Dietz, W. H. & Colditz, G. A. Guidelines for healthy weight. N. Engl. J. Med. 341 , 427–434 (1999).
Article CAS PubMed Google Scholar
Prospective Studies Collaboration. Body-mass index and cause-specific mortality in 900,000 adults: collaborative analyses of 57 prospective studies. Lancet 373 , 1083–1096 (2009).
Article PubMed Central Google Scholar
Chan, J. M., Rimm, E. B., Colditz, G. A., Stampfer, M. J. & Willett, W. C. Obesity, fat distribution, and weight gain as risk factors for clinical diabetes in men. Diabetes Care 17 , 961–969 (1994).
Colditz, G. A., Willett, W. C., Rotnitzky, A. & Manson, J. E. Weight gain as a risk factor for clinical diabetes mellitus in women. Ann. Intern. Med. 122 , 481–486 (1995).
Hu, F. B. et al. Diet, lifestyle, and the risk of type 2 diabetes mellitus in women. N. Engl. J. Med. 345 , 790–797 (2001).
Kendall, D. M., Cuddihy, R. M. & Bergenstal, R. M. Clinical application of incretin-based therapy: therapeutic potential, patient selection and clinical use. Am. J. Med. 122 , S37–S50 (2009).
Mittendorfer, B., Magkos, F., Fabbrini, E., Mohammed, B. S. & Klein, S. Relationship between body fat mass and free fatty acid kinetics in men and women. Obesity 17 , 1872–1877 (2009).
Conte, C. et al. Multiorgan insulin sensitivity in lean and obese subjects. Diabetes Care 35 , 1316–1321 (2012).
Article CAS PubMed PubMed Central Google Scholar
Wilman, H. R. et al. Characterisation of liver fat in the UK Biobank cohort. PLoS One 12 , e0172921 (2017).
Article PubMed PubMed Central CAS Google Scholar
Pienkowska, J. et al. MRI assessment of ectopic fat accumulation in pancreas, liver and skeletal muscle in patients with obesity, overweight and normal BMI in correlation with the presence of central obesity and metabolic syndrome. Diabetes Metab. Syndr. Obes. 12 , 623–636 (2019).
Tabak, A. G. et al. Trajectories of glycaemia, insulin sensitivity, and insulin secretion before diagnosis of type 2 diabetes: an analysis from the Whitehall II study. Lancet 373 , 2215–2221 (2009). A prospective study of the temporal changes in metabolic function and glucose control along the natural history of T2DM .
Weir, G. C. & Bonner-Weir, S. Five stages of evolving beta-cell dysfunction during progression to diabetes. Diabetes 53 (Suppl. 3), 16–21 (2004).
Article Google Scholar
Astrup, A. & Finer, N. Redefining type 2 diabetes: ‘diabesity’ or ‘obesity dependent diabetes mellitus’? Obes. Rev. 1 , 57–59 (2000).
Leitner, D. R. et al. Obesity and type 2 diabetes: two diseases with a need for combined treatment strategies — EASO can lead the way. Obes. Facts 10 , 483–492 (2017).
Sjostrom, L. Review of the key results from the Swedish Obese Subjects (SOS) trial — a prospective controlled intervention study of bariatric surgery. J. Intern. Med. 273 , 219–234 (2013).
Jans, A. et al. Duration of type 2 diabetes and remission rates after bariatric surgery in Sweden 2007–2015: a registry-based cohort study. PLoS Med. 16 , e1002985 (2019).
Davies, M. J. et al. Efficacy of liraglutide for weight loss among patients with type 2 diabetes: the SCALE diabetes randomized clinical trial. JAMA 314 , 687–699 (2015).
Madsbad, S. & Holst, J. J. GLP-1 as a mediator in the remission of type 2 diabetes after gastric bypass and sleeve gastrectomy surgery. Diabetes 63 , 3172–3174 (2014).
MacDonald, P. E. et al. The multiple actions of GLP-1 on the process of glucose-stimulated insulin secretion. Diabetes 51 (Suppl. 3), 434–442 (2002).
Magkos, F. et al. Effects of moderate and subsequent progressive weight loss on metabolic function and adipose tissue biology in humans with obesity. Cell Metab. 23 , 591–601 (2016). A randomized controlled trial of the effects of progressive diet-induced weight loss on body composition and metabolic function .
Wing, R. R. et al. Long-term effects of modest weight loss in type II diabetic patients. Arch. Intern. Med. 147 , 1749–1753 (1987).
Henry, R. R., Wallace, P. & Olefsky, J. M. Effects of weight loss on mechanisms of hyperglycemia in obese non-insulin-dependent diabetes mellitus. Diabetes 35 , 990–998 (1986).
Markovic, T. P. et al. The determinants of glycemic responses to diet restriction and weight loss in obesity and NIDDM. Diabetes Care 21 , 687–694 (1998).
Henry, R. R., Scheaffer, L. & Olefsky, J. M. Glycemic effects of intensive caloric restriction and isocaloric refeeding in noninsulin-dependent diabetes mellitus. J. Clin. Endocrinol. Metab. 61 , 917–925 (1985).
Hughes, T. A., Gwynne, J. T., Switzer, B. R., Herbst, C. & White, G. Effects of caloric restriction and weight loss on glycemic control, insulin release and resistance, and atherosclerotic risk in obese patients with type II diabetes mellitus. Am. J. Med. 77 , 7–17 (1984).
Steven, S. & Taylor, R. Restoring normoglycaemia by use of a very low calorie diet in long- and short-duration type 2 diabetes. Diabet. Med. 32 , 1149–1155 (2015).
Lim, E. L. et al. Reversal of type 2 diabetes: normalisation of beta cell function in association with decreased pancreas and liver triacylglycerol. Diabetologia 54 , 2506–2514 (2011).
Taylor, R. et al. Remission of human type 2 diabetes requires decrease in liver and pancreas fat content but is dependent upon capacity for beta cell recovery. Cell Metab. 28 , 547–556.e3 (2018).
Al-Mrabeh, A. et al. Hepatic lipoprotein export and remission of human type 2 diabetes after weight loss. Cell Metab. 31 , 233–249 (2020). A prospective study evaluating the potential mechanisms of T2DM remission and relapse following lifestyle modification .
Taylor, R. Pathogenesis of type 2 diabetes: tracing the reverse route from cure to cause. Diabetologia 51 , 1781–1789 (2008).
Taylor, R. & Barnes, A. C. Can type 2 diabetes be reversed and how can this best be achieved? James Lind Alliance research priority number one. Diabet. Med. 36 , 308–315 (2019).
Brown, A. et al. Low-energy total diet replacement intervention in patients with type 2 diabetes mellitus and obesity treated with insulin: a randomized trial. BMJ Open Diabetes Res. Care 8 , e001012 (2020).
Gregg, E. W. et al. Association of an intensive lifestyle intervention with remission of type 2 diabetes. JAMA 308 , 2489–2496 (2012).
Annuzzi, G., Rivellese, A. A., Bozzetto, L. & Riccardi, G. The results of Look AHEAD do not row against the implementation of lifestyle changes in patients with type 2 diabetes. Nutr. Metab. Cardiovasc. Dis. 24 , 4–9 (2014).
Raynor, H. A. et al. Partial meal replacement plan and quality of the diet at 1 year: action for health in diabetes (Look AHEAD) trial. J. Acad. Nutr. Diet. 115 , 731–742 (2015).
Lean, M. E. et al. Primary care-led weight management for remission of type 2 diabetes (DiRECT): an open-label, cluster-randomised trial. Lancet 391 , 541–551 (2018).
Lean, M. E. J. et al. Durability of a primary care-led weight-management intervention for remission of type 2 diabetes: 2-year results of the DiRECT open-label, cluster-randomised trial. Lancet Diabetes Endocrinol. 7 , 344–355 (2019). A randomized controlled trial of diet-induced weight loss demonstrating that remission of T2DM depends on the amount of weight loss .
Heymsfield, S. B., Gonzalez, M. C., Shen, W., Redman, L. & Thomas, D. Weight loss composition is one-fourth fat-free mass: a critical review and critique of this widely cited rule. Obes. Rev. 15 , 310–321 (2014).
DeFronzo, R. A. et al. The effect of insulin on the disposal of intravenous glucose. Results from indirect calorimetry and hepatic and femoral venous catheterization. Diabetes 30 , 1000–1007 (1981).
Ferrannini, E. et al. The disposal of an oral glucose load in healthy subjects. A quantitative study. Diabetes 34 , 580–588 (1985).
American Diabetes Association. Standards of medical care in diabetes — 2020. Diabetes Care 43 , S1–S212 (2020).
Ajala, O., English, P. & Pinkney, J. Systematic review and meta-analysis of different dietary approaches to the management of type 2 diabetes. Am. J. Clin. Nutr. 97 , 505–516 (2013).
Hjorth, M. F., Zohar, Y., Hill, J. O. & Astrup, A. Personalized dietary management of overweight and obesity based on measures of insulin and glucose. Annu. Rev. Nutr. 38 , 245–272 (2018). A review of evidence supporting baseline glycaemia as a major predictor of weight loss success in response to dietary interventions .
Snorgaard, O., Poulsen, G. M., Andersen, H. K. & Astrup, A. Systematic review and meta-analysis of dietary carbohydrate restriction in patients with type 2 diabetes. BMJ Open. Diabetes Res. Care 5 , e000354 (2017).
Kirk, E. et al. Dietary fat and carbohydrates differentially alter insulin sensitivity during caloric restriction. Gastroenterology 136 , 1552–1560 (2009).
Wing, R. R. et al. Caloric restriction per se is a significant factor in improvements in glycemic control and insulin sensitivity during weight loss in obese NIDDM patients. Diabetes Care 17 , 30–36 (1994).
Look Ahead Research Group. Cardiovascular effects of intensive lifestyle intervention in type 2 diabetes. N. Engl. J. Med. 369 , 145–154 (2013).
Article CAS Google Scholar
Sjostrom, L. et al. Association of bariatric surgery with long-term remission of type 2 diabetes and with microvascular and macrovascular complications. JAMA 311 , 2297–2304 (2014).
Article PubMed CAS Google Scholar
Wing, R. R., Blair, E., Marcus, M., Epstein, L. H. & Harvey, J. Year-long weight loss treatment for obese patients with type II diabetes: does including an intermittent very-low-calorie diet improve outcome? Am. J. Med. 97 , 354–362 (1994).
Samkani, A. et al. A carbohydrate-reduced high-protein diet acutely decreases postprandial and diurnal glucose excursions in type 2 diabetes patients. Br. J. Nutr. 119 , 910–917 (2018).
Skytte, M. J. et al. A carbohydrate-reduced high-protein diet improves HbA1c and liver fat content in weight stable participants with type 2 diabetes: a randomised controlled trial. Diabetologia 62 , 2066–2078 (2019). A cross-over study showing that low-carbohydrate diets can improve metabolic risk factors in patients with T2DM without much weight loss .
Taylor, R., Al-Mrabeh, A. & Sattar, N. Understanding the mechanisms of reversal of type 2 diabetes. Lancet Diabetes Endocrinol. 7 , 726–736 (2019). A review of the mechanisms of T2DM remission .
Hellerstein, M. K. De novo lipogenesis in humans: metabolic and regulatory aspects. Eur. J. Clin. Nutr. 53 (Suppl. 1), 53–65 (1999).
van Wyk, H. J., Davis, R. E. & Davies, J. S. A critical review of low-carbohydrate diets in people with type 2 diabetes. Diabet. Med. 33 , 148–157 (2016).
Kodama, S. et al. Influence of fat and carbohydrate proportions on the metabolic profile in patients with type 2 diabetes: a meta-analysis. Diabetes Care 32 , 959–965 (2009).
Hamdy, O. et al. Fat versus carbohydrate-based energy-restricted diets for weight loss in patients with type 2 diabetes. Curr. Diab Rep. 18 , 128 (2018).
Forouhi, N. G., Misra, A., Mohan, V., Taylor, R. & Yancy, W. Dietary and nutritional approaches for prevention and management of type 2 diabetes. BMJ 361 , k2234 (2018).
Shan, Z., Guo, Y., Hu, F. B., Liu, L. & Qi, Q. Association of low-carbohydrate and low-fat diets with mortality among US adults. JAMA Intern. Med. 180 , 513–523 (2020).
Livesey, G. et al. Dietary glycemic index and load and the risk of type 2 diabetes: a systematic review and updated meta-analyses of prospective cohort studies. Nutrients 11 , 1280 (2019).
Article CAS PubMed Central Google Scholar
Livesey, G. et al. Dietary glycemic index and load and the risk of type 2 diabetes: assessment of causal relations. Nutrients 11 , 1436 (2019).
Hwang, J. J. et al. Blunted rise in brain glucose levels during hyperglycemia in adults with obesity and T2DM. JCI Insight 2 , e95913 (2017). A study showing that patients with obesity and T2DM have a blunted rise in brain blood glucose levels in response to carbohydrate ingestion, and this associates with their feelings of appetite and hunger .
Astrup, A. & Hjorth, M. F. Classification of obesity targeted personalized dietary weight loss management based on carbohydrate tolerance. Eur. J. Clin. Nutr. 72 , 1300–1304 (2018).
Frost, G. et al. The short-chain fatty acid acetate reduces appetite via a central homeostatic mechanism. Nat. Commun. 5 , 3611 (2014).
Trajkovski, M. & Wollheim, C. B. Physiology: microbial signals to the brain control weight. Nature 534 , 185–187 (2016).
Hjorth, M. F. et al. Pretreatment prevotella-to-bacteroides ratio and salivary amylase gene copy number as prognostic markers for dietary weight loss. Am. J. Clin. Nutr. 111 , 1079–1086 (2020).
Sanna, S. et al. Causal relationships among the gut microbiome, short-chain fatty acids and metabolic diseases. Nat. Genet. 51 , 600–605 (2019).
Yamada, Y. et al. A non-calorie-restricted low-carbohydrate diet is effective as an alternative therapy for patients with type 2 diabetes. Intern. Med. 53 , 13–19 (2014).
Tay, J. et al. A very low-carbohydrate, low-saturated fat diet for type 2 diabetes management: a randomized trial. Diabetes Care 37 , 2909–2918 (2014).
Balducci, S. et al. Physical exercise as therapy for type 2 diabetes mellitus. Diabetes Metab. Res. Rev. 30 (Suppl 1), 13–23 (2014).
Boule, N. G., Haddad, E., Kenny, G. P., Wells, G. A. & Sigal, R. J. Effects of exercise on glycemic control and body mass in type 2 diabetes mellitus: a meta-analysis of controlled clinical trials. JAMA 286 , 1218–1227 (2001).
Snowling, N. J. & Hopkins, W. G. Effects of different modes of exercise training on glucose control and risk factors for complications in type 2 diabetic patients: a meta-analysis. Diabetes Care 29 , 2518–2527 (2006).
Balducci, S. et al. Effect of an intensive exercise intervention strategy on modifiable cardiovascular risk factors in subjects with type 2 diabetes mellitus: a randomized controlled trial: the Italian Diabetes and Exercise Study (IDES). Arch. Intern. Med. 170 , 1794–1803 (2010).
Di Loreto, C. et al. Make your diabetic patients walk: long-term impact of different amounts of physical activity on type 2 diabetes. Diabetes Care 28 , 1295–1302 (2005).
Balducci, S. et al. Changes in physical fitness predict improvements in modifiable cardiovascular risk factors independently of body weight loss in subjects with type 2 diabetes participating in the Italian Diabetes and Exercise Study (IDES). Diabetes Care 35 , 1347–1354 (2012).
Balducci, S. et al. Effect of high- versus low-intensity supervised aerobic and resistance training on modifiable cardiovascular risk factors in type 2 diabetes: the Italian Diabetes and Exercise Study (IDES). PLoS One 7 , e49297 (2012).
Eriksson, K. F. & Lindgarde, F. Prevention of type 2 (non-insulin-dependent) diabetes mellitus by diet and physical exercise. The 6-year Malmo feasibility study. Diabetologia 34 , 891–898 (1991).
Saltin, B. et al. Physical training and glucose tolerance in middle-aged men with chemical diabetes. Diabetes 28 (Suppl. 1), 30–32 (1979).
Nagi, D. Diabetes in Practice 2nd edn (John Wiley & Sons, 2005).
Ades, P. A., Savage, P. D., Marney, A. M., Harvey, J. & Evans, K. A. Remission of recently diagnosed type 2 diabetes mellitus with weight loss and exercise. J. Cardiopulm. Rehabil. Prev. 35 , 193–197 (2015).
Ried-Larsen, M. et al. Type 2 diabetes remission 1 year after an intensive lifestyle intervention: a secondary analysis of a randomized clinical trial. Diabetes Obes. Metab. 21 , 2257–2266 (2019).
Johansen, M. Y. et al. Effect of an intensive lifestyle intervention on glycemic control in patients with type 2 diabetes: a randomized clinical trial. JAMA 318 , 637–646 (2017).
Vetter, M. L., Ritter, S., Wadden, T. A. & Sarwer, D. B. Comparison of bariatric surgical procedures for diabetes remission: efficacy and mechanisms. Diabetes Spectr. 25 , 200–210 (2012).
Bray, G. A., Krauss, R. M., Sacks, F. M. & Qi, L. Lessons learned from the POUNDS Lost Study: genetic, metabolic, and behavioral factors affecting changes in body weight, body composition, and cardiometabolic risk. Curr. Obes. Rep. 8 , 262–283 (2019).
Franz, M. J. & Evert, A. B. American Diabetes Association Guide to Nutrition Therapy for Diabetes 2 edn (American Diabetes Association, 2012).
Rowley, W. R., Bezold, C., Arikan, Y., Byrne, E. & Krohe, S. Diabetes 2030: insights from yesterday, today, and future trends. Popul. Health Manag. 20 , 6–12 (2017).
Gillies, C. L. et al. Pharmacological and lifestyle interventions to prevent or delay type 2 diabetes in people with impaired glucose tolerance: systematic review and meta-analysis. BMJ 334 , 299 (2007).
Knowler, W. C. et al. Reduction in the incidence of type 2 diabetes with lifestyle intervention or metformin. N. Engl. J. Med. 346 , 393–403 (2002).
Lindstrom, J. et al. Sustained reduction in the incidence of type 2 diabetes by lifestyle intervention: follow-up of the Finnish Diabetes Prevention study. Lancet 368 , 1673–1679 (2006).
Pan, X. R. et al. Effects of diet and exercise in preventing NIDDM in people with impaired glucose tolerance. The Da Qing IGT and Diabetes study. Diabetes Care 20 , 537–544 (1997).
Li, G. et al. The long-term effect of lifestyle interventions to prevent diabetes in the China Da Qing Diabetes Prevention study: a 20-year follow-up study. Lancet 371 , 1783–1789 (2008).
Poulsen, S. K. et al. Health effect of the New Nordic Diet in adults with increased waist circumference: a 6-mo randomized controlled trial. Am. J. Clin. Nutr. 99 , 35–45 (2014).
Hjorth, M. F. et al. Pretreatment fasting plasma glucose and insulin modify dietary weight loss success: results from 3 randomized clinical trials. Am. J. Clin. Nutr. 106 , 499–505 (2017).
Ritz, C., Astrup, A., Larsen, T. M. & Hjorth, M. F. Weight loss at your fingertips: personalized nutrition with fasting glucose and insulin using a novel statistical approach. Eur. J. Clin. Nutr. 73 , 1529–1535 (2019). This article uses a novel statistical approach to model and estimate diet-induced weight loss according to baseline levels of glycaemia .
Due, A. et al. Comparison of 3 ad libitum diets for weight-loss maintenance, risk of cardiovascular disease, and diabetes: a 6-mo randomized, controlled trial. Am. J. Clin. Nutr. 88 , 1232–1241 (2008).
CAS PubMed Google Scholar
Hjorth, M. F., Due, A., Larsen, T. M. & Astrup, A. Pretreatment fasting plasma glucose modifies dietary weight loss maintenance success: results from a stratified RCT. Obesity 25 , 2045–2048 (2017).
Larsen, T. M. et al. Diets with high or low protein content and glycemic index for weight-loss maintenance. N. Engl. J. Med. 363 , 2102–2113 (2010).
Greenway, F. L. et al. A randomized, double-blind, placebo-controlled study of Gelesis100: a novel nonsystemic oral hydrogel for weight loss. Obesity 27 , 205–216 (2019).
Dansinger, M. L., Gleason, J. A., Griffith, J. L., Selker, H. P. & Schaefer, E. J. Comparison of the Atkins, Ornish, Weight Watchers, and Zone diets for weight loss and heart disease risk reduction: a randomized trial. JAMA 293 , 43–53 (2005).
Greenberg, I., Stampfer, M. J., Schwarzfuchs, D., Shai, I. & Group, D. Adherence and success in long-term weight loss diets: the dietary intervention randomized controlled trial (DIRECT). J. Am. Coll. Nutr. 28 , 159–168 (2009).
Sacks, F. M. et al. Comparison of weight-loss diets with different compositions of fat, protein, and carbohydrates. N. Engl. J. Med. 360 , 859–873 (2009). The largest and longest (to date) randomized study comparing the weight loss effectiveness of diets differing in macronutrient composition shows no differences among diets .
Download references
Author information
Authors and affiliations.
Department of Nutrition, Exercise and Sports, Faculty of Science, University of Copenhagen, Frederiksberg Campus, Copenhagen, Denmark
Faidon Magkos, Mads F. Hjorth & Arne Astrup
You can also search for this author in PubMed Google Scholar
Contributions
The authors contributed equally to all aspects of the article.
Corresponding author
Correspondence to Arne Astrup .
Ethics declarations
Competing interests.
M.F.H. and A.A. are co-inventors on a pending provisional patent application on the use of biomarkers for prediction of weight loss responses and co-founders/owners of the University of Copenhagen spin-out company Personalized Weight Management Research Consortium ApS (Gluco-diet.dk). A.A. is a consultant or advisory board member for Basic Research, USA, Beachbody, USA, BioCare Copenhagen, Denmark, Gelesis, USA, Groupe Éthique et Santé, France, McCain Foods Limited, USA, Nestlé Research Center, Switzerland, and Weight Watchers, USA. A.A. and M.F.H. are co-authors of a number of diet/cookery books, including personalized nutrition for weight loss, published in several languages. F.M. declares no competing interests.
Additional information
Peer review information.
Nature Reviews Endocrinology thanks P. Clifton, R. Taylor and the other, anonymous, reviewer(s) for their contribution to the peer review of this work.
Publisher’s note
Springer Nature remains neutral with regard to jurisdictional claims in published maps and institutional affiliations.
An intermediate condition between normoglycaemia and type 2 diabetes mellitus, characterized by moderately elevated fasting or postprandial blood glucose or HbA 1c .
A relative ranking of foods according to their ability to increase blood glucose levels relative to a reference food (glucose or white bread) for the same amount of bioavailable carbohydrate.
An extension of the glycaemic index that takes into account the actual amount of available carbohydrate present in one serving of a food or in the whole diet.
Rights and permissions
Reprints and permissions
About this article
Cite this article.
Magkos, F., Hjorth, M.F. & Astrup, A. Diet and exercise in the prevention and treatment of type 2 diabetes mellitus. Nat Rev Endocrinol 16 , 545–555 (2020). https://doi.org/10.1038/s41574-020-0381-5
Download citation
Accepted : 12 June 2020
Published : 20 July 2020
Issue Date : October 2020
DOI : https://doi.org/10.1038/s41574-020-0381-5
Share this article
Anyone you share the following link with will be able to read this content:
Sorry, a shareable link is not currently available for this article.
Provided by the Springer Nature SharedIt content-sharing initiative
This article is cited by
Comorbidities and use of health services in people with diabetes mellitus according to risk levels by adjusted morbidity groups.
- Jaime Barrio-Cortes
- María Pilar Mateos-Carchenilla
- Montserrat Ruiz-López
BMC Endocrine Disorders (2024)
Hypothalamic POMC neuron-specific knockout of MC4R affects insulin sensitivity by regulating Kir2.1
- Haohao Zhang
Molecular Medicine (2024)
Knowledge, attitude, and practice toward weight management among diabetic patients in Qidong City, Jiangsu Province
- Xiaofeng Li
- Shengnan Cai
BMC Public Health (2024)
Metrnl: a promising biomarker and therapeutic target for cardiovascular and metabolic diseases
- Wen-sheng Dong
Cell Communication and Signaling (2024)
Advances in secondary prevention mechanisms of macrovascular complications in type 2 diabetes mellitus patients: a comprehensive review
- Huifang Guan
- Jiaxing Tian
- Xiaolin Tong
European Journal of Medical Research (2024)
Quick links
- Explore articles by subject
- Guide to authors
- Editorial policies
Sign up for the Nature Briefing newsletter — what matters in science, free to your inbox daily.

Advertisement
Public Health Approaches to Type 2 Diabetes Prevention: the US National Diabetes Prevention Program and Beyond
- Diabetes Epidemiology (E Selvin and K Foti, Section Editors)
- Open access
- Published: 05 August 2019
- Volume 19 , article number 78 , ( 2019 )
Cite this article
You have full access to this open access article
- Stephanie M. Gruss 1 ,
- Kunthea Nhim 1 ,
- Edward Gregg 2 ,
- Miriam Bell 1 ,
- Elizabeth Luman 1 &
- Ann Albright 1
29k Accesses
91 Citations
Explore all metrics
A Correction to this article was published on 27 June 2020
This article has been updated
Purpose of Review
This article highlights foundational evidence, translation studies, and current research behind type 2 diabetes prevention efforts worldwide, with focus on high-risk populations, and whole-population approaches as catalysts to global prevention.
Recent Findings
Continued focus on the goals of foundational lifestyle change program trials and their global translations, and the targeting of those at highest risk through both in-person and virtual modes of program delivery, is critical. Whole-population approaches (e.g., socioeconomic policies, healthy food promotion, environmental/systems changes) and awareness raising are essential complements to efforts aimed at high-risk populations.
Successful type 2 diabetes prevention strategies are being realized in the USA through the National Diabetes Prevention Program and elsewhere in the world. A multi-tiered approach involving appropriate risk targeting and whole-population efforts is essential to curb the global diabetes epidemic.
Similar content being viewed by others
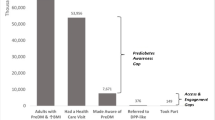
Evidence and Challenges for Translation and Population Impact of the Diabetes Prevention Program
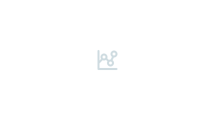
Diabetes Prevention Amongst South Asians: Current Evidence, Challenges, and a Way Forward
Lifestyle interventions for diabetes prevention in south asians: current evidence and opportunities.
Avoid common mistakes on your manuscript.
Introduction
Worldwide, it is estimated that 425 million adults (20–79 years) have diabetes, projected to reach 629 million by 2045 [ 1 •]. In the USA, about 30 million adults (18 years and older) have diabetes, or 12.2% of the adult population [ 2 ]. Diabetes was estimated to cost $727 billion in 2017 in health expenditures worldwide [ 1 ] and $327 billion in 2017 in total economic costs in the USA [ 3 •]. Increases in diabetes prevalence have led to increases in related complications, such as cardiovascular disease, visual impairment and vision loss, lower extremity amputations, end-stage renal disease, disability, and premature mortality [ 2 ].
The majority of diabetes is type 2, which generally follows a period of prediabetes, a condition where blood glucose levels are higher than normal, but not high enough for a type 2 diabetes diagnosis [ 2 ]. In the USA, risk factors for prediabetes and type 2 diabetes include being overweight or having obesity; having a racial/ethnic background that is African-American, Hispanic/Latino, American Indian, Asian American, or Pacific Islander; having a parent or sibling with diabetes; having hypertension; having a history of gestational diabetes; and living a sedentary lifestyle. An estimated 353 million adults worldwide [ 1 ]—84.1 million in the USA (33.9% of all adults) [ 2 ]—have prediabetes diabetes. Given the magnitude of these numbers, identifying those at risk and preventing or delaying onset of type 2 diabetes are critical to ending the pandemic.
Type 2 diabetes can be prevented or delayed through mitigation of modifiable risk factors, such as healthier eating, weight loss, and increased physical activity. Studies show lifestyle intervention as a sole modality, lifestyle intervention in combination with therapeutics, and whole-population approaches are all promising for type 2 diabetes prevention. Public health interventions using glycemic risk stratification to target individuals at “very high” risk (having obesity and impaired glucose tolerance (IGT)) and “high” risk (being overweight with IGT) have proven to significantly reduce conversion to type 2 diabetes [ 4 ]. Individuals in these categories have a fasting plasma glucose (FPG) > 100 mg/dL, HbA1c levels > 5.7%, and a 10-year diabetes incidence of 20–30% or more [ 5 ]. Individuals in lower risk tiers may be more appropriately targeted via risk counseling and whole-population strategies [ 5 , 6 , 7 ]. Population-level policies, systems, and environmental approaches, along with lifestyle intervention for those at high risk, are likely optimal to achieve the greatest level of impact [ 8 ].
The purpose of this review is to highlight the foundational and current research and translation studies which underlie high-risk population and whole-population strategies for type 2 diabetes prevention worldwide, with particular focus on the U.S. National Diabetes Prevention Program, as catalysts for global prevention efforts.
Worldwide Evidence for Type 2 Diabetes Prevention Through Lifestyle Change
Foundational research.
The scientific evidence for prevention through lifestyle changes is compelling based on a series of randomized control trials (RCTs), which found through intensive, structured, yearlong educational programs focused on moderate weight loss (5–7%), increasing self-efficacy around engagement in one’s health, and moderate increases in physical activity over time, it is possible to prevent or delay type 2 diabetes among those at very high and high risk. RCTs have been conducted in various settings among diverse racial and ethnic populations. Common elements include utilizing a group-based intervention and evaluating effectiveness in terms of increased physical activity and healthy eating, and improved clinical metrics, such as weight, body mass index (BMI), waist circumference, HbA1c, and blood glucose.
The Chinese Da Qing group-based RCT was the first and longest running of such studies. It began in 1986 [ 9 ] with a follow-up study conducted in 1997–2006. The study demonstrated a 43% incidence reduction in the intervention group after 14 years when compared to the control group. Type 2 diabetes was delayed by an average of 3.6 years [ 10 ], and the incidence of severe retinopathy and cardiovascular-related disease and events were reduced [ 10 , 11 ]. After 30 years, 577 adults with IGT were followed from the original trial; the intervention group had a median delay in type 2 diabetes incidence of 3.96 years, an average increase in life expectancy of 1.44 years, and fewer cardiovascular disease and all-cause deaths, reduced incidence of cardiovascular events, and lower incidence of microvascular complications compared to the control group [ 12 ].
The U.S. Diabetes Prevention Program (DPP) was a three-arm RCT, which began in 1996 [ 13 ••]. The study found that a lifestyle change intervention focused on a 5–7% weight loss and a moderate increase in physical activity over one year achieved a 58% relative risk reduction in type 2 diabetes, and that use of metformin achieved a 31% reduction, when compared to a placebo [ 13 ••]. The DPP used intensive one-on-one counseling with a minimum of 50% racially and ethnically diverse individuals across both male and female genders at high risk for type 2 diabetes (elevated plasma glucose of 95 to 125 mmol/L or fasting glucose of 7.8 to 11.0 mmol/L) [ 13 ••]. A follow-up study, the U.S. Diabetes Prevention Program Outcomes Study (DPPOS), reported a 34% reduction in type 2 diabetes incidence 10 years after the completion of the DPP trial for the lifestyle change intervention arm [ 14 ], and a 27% reduction after 15 years (18% for the metformin arm), compared to the placebo group [ 15 ]. Furthermore, the study found that lifestyle change was cost-effective compared to a placebo, and that cumulative quality-adjusted life years (QALYs) gained over a 3-year timeframe were greater for lifestyle (6.81) than either metformin (6.69) or a placebo (6.67) [ 16 ].
The Finnish Diabetes Prevention Study (Finnish DPS), which began in 2001 [ 17 ], tested lifestyle intervention in a community-based primary health care setting, and designed and implemented a high-risk screening assessment for type 2 diabetes that is used worldwide called the Finnish Type 2 Diabetes Risk Score [ 18 ]. The study demonstrated a risk reduction of 58%, as well as a legacy effect of 43% reduction in type 2 diabetes incidence three years after completion of the study. The study has also been successfully translated in Greece with similar results [ 19 ].
The Japanese DPP, conducted in 2005 in male participants with IGT > 140 mg/dL, found a cumulative 4-year incidence of diabetes of 9.3% in the control group, versus 3.0% in the intervention group [ 20 ]. The Indian DPP-1 trial, conducted in 2006, was a community-based RCT involving 531 subjects with IGT across three intervention arms (one was given advice on lifestyle modification (LSM), one was treated with metformin (MET), and another was given LSM plus MET) and one control arm. A relative risk reduction of 26–29% after 30 months was similar in all three intervention arms [ 21 ]. Additional 3-year results suggest that both metformin and lifestyle change were cost-effective in preventing type 2 diabetes [ 22 ].
Translational Research
Translational research, which examines how to best tailor key research findings into policy, program, or practice [ 23 ], further demonstrates that lifestyle interventions are feasible and effective in real-world settings [ 24 ]. A systematic review and meta-analysis of 28 US-based DPP translation studies found a mean weight loss of over 4% across 3797 high-risk participants and demonstrated cost-effectiveness in terms of program, materials, and staff costs [ 24 ].
The European DE-PLAN study (“Diabetes in Europe – Prevention using Lifestyle, Physical Activity, and Nutritional Intervention”), implemented in 17 countries, was a community-based 10-month translation of the Finnish DPS targeting those at high risk for type 2 diabetes [ 19 ]. DE-PLAN began in 2008 and used the Finnish Diabetes Risk Score to determine eligibility [ 19 ]. In 2018, study participants in Poland ( n = 175) with increased risk for type 2 diabetes received 10 months of lifestyle counseling sessions, physical activity, and self-efficacy sessions [ 25 ••]. Participants with a higher starting BMI and a history of increased glucose were more likely to achieve the goal weight loss of ≥ 5% of initial body weight compared to those with lower risk [ 25 ]. A UK study had similar findings, also determining adults with obesity and higher HbA1c levels (6–6.4%) not only met the weight loss outcomes of the US DPP trial but also gained more QALYs than those with lower risk; the intervention was also determined to be cost-saving [ 26 ].
The Australian Good Ageing in Lahti Region Lifestyle (GOAL) Implementation program, based on the Finnish DPS, found positive associations between changes in self-efficacy and dietary behaviors and improvement in waist circumference, cholesterol levels, triglycerides, diastolic blood pressure, and FPG in the lifestyle intervention arm compared to the control group [ 27 ]. GOAL was further scaled up with over 10,000 participants via the Melbourne DPS with significant improvements in cardiovascular risk factors, waist circumference, BMI, and weight loss [ 28 ].
Alternative modalities of lifestyle change program delivery, such as virtual program delivery and telehealth, have the potential to reach millions of people, even in remote areas. Shortly after publication of the 2002 DPP research study, Tate et al. (2003) conducted the first RCT of a yearlong Internet-based diabetes prevention lifestyle change weight loss program alone vs. one with the addition of e-behavioral counseling [ 29 ]. The group receiving e-behavioral counseling submitted calorie and exercise information and received weekly e-mail behavioral counseling and feedback from a counselor for 12 months and lost 4.8% of original body weight compared to 2.2% among those receiving the Internet program only [ 29 ]. A 2013 text messaging study in India showed that mobile technology can have an impact on clinical outcomes, with cumulative incidence of type 2 diabetes at 2 years of 18% in the text messaging counseling group vs. 27% in the control group [ 30 ], and a sustained reduction in incidence after 5 years [ 31 ]. Similar studies have been conducted (Supplemental Table 1 ) to determine the effectiveness of various forms of virtual delivery, including delivery via television, social networking sites, and online. These published studies on virtual delivery found ~ 4% to 10% weight loss after virtual implementation of a yearlong diabetes prevention lifestyle change program. A summary of results is included in Supplemental Table 1 .
The evidence is clear: lifestyle interventions can prevent or delay type 2 diabetes among various races, ethnicities, genders, and regions. Additionally, type 2 diabetes prevention program interventions continue to demonstrate cost-effectiveness/cost savings in real-world settings over time [ 32 , 33 ].
The US National DPP—a Case Study
Large-scale implementation of the US DPP trial’s lifestyle change program began in 2010, when Congress authorized the US Centers for Disease Control and Prevention (CDC) to establish and lead the National DPP in an effort to make the intervention broadly available to individuals at high risk [ 34 ]. The National DPP is a partnership of public and private organizations working to build a delivery system for the lifestyle intervention. It consists of four core elements: a trained workforce of lifestyle coaches; national quality standards supported by the CDC Diabetes Prevention Recognition Program (DPRP); a network of program delivery organizations sustained through coverage; and participant referral and engagement [ 35 ]. The National DPP lifestyle change program is based on evidence and key features of the US DPP trial that were shown to be successful: realistic weight loss goal after 12 months (minimum 5% of initial body weight), documentation of physical activity minutes (≥ 150 min per week), and attendance throughout the 12-month program, with an emphasis on self-efficacy that focuses on improving problem-solving skills, social supports, the use of built environments, and strategies to adapt to change [ 35 ]. The National DPP is the world’s largest translation of the US DPP study, having reached over 324,000 participants across > 3,000 organizations as of April 12, 2019 [ 36 ]. The DPRP is the quality assurance arm of the National DPP, developing evidence-based standards (DPRP Standards) and monitoring and evaluating participating organizations for fidelity and effectiveness of intervention delivery [ 37 ]. The DPRP Standards are updated every three years based on new dietary, physical activity, self-efficacy, delivery modality, and other type 2 diabetes prevention evidence. Through the DPRP, CDC awards either preliminary (based on participant attendance rather than outcomes) or full (meeting all DPRP Standards) recognition to successful organizations [ 37 ]. CDC recognition is widely accepted in the USA as an assurance of a quality type 2 diabetes prevention program and can result in insurance coverage for participants and reimbursement for program delivery organizations.
Public and private insurance coverage for type 2 diabetes prevention interventions is crucial to widespread adoption and participation in National DPP lifestyle change programs. Insurance coverage expands payment options, thereby reducing the burden of cost for participants. Currently, over 3.8 million public employees and dependents in 20 states have the National DPP lifestyle change program as a covered benefit and over 100 private employers and commercial plans include it as a covered benefit for their employees [ 38 ]. In 2018, the US Centers for Medicare & Medicaid Services (CMS) began coverage for eligible Medicare beneficiaries who participate in CDC-recognized programs and meet performance goals of the National DPP [ 40 ]. CMS provides reimbursement for participants meeting program goals such as 5% weight loss in organizations that have achieved either preliminary or full CDC recognition [ 39 ]. Eight states in the USA also provide Medicaid coverage for eligible beneficiaries [ 38 ].
Based on published translational research, feedback from stakeholder organizations, and gray literature scans (stakeholder materials and policies not found in peer-reviewed journals), CDC concluded that there was sufficient evidence to allow organizations delivering the National DPP lifestyle change program virtually to apply for CDC recognition. Thus, in order to expand availability and increase program participation (especially for those in rural or remote locations), the DPRP Standards were amended in January 2015 to allow online modes of delivery in addition to in-person. The Standards were amended again in February 2018 to also include telehealth and combination (in-person/virtual) delivery and new participant-level variables that include education level, enrollment source, and payer source. All virtual providers are held accountable to the same quality standards as in-person delivery organizations, including the provision of coaching services [ 37 ]. As of April 2019, CDC had 121 recognized virtual providers delivering the yearlong lifestyle change program to over 193,000 people [ 36 ].
Key Findings from In-Person National DPP Delivery in the USA
DPRP data from the first 4 years (February 2012–January 2016) of the National DPP, describing the experience of 14,747 participants who attended 4 or more sessions of the lifestyle change program in 220 organizations, showed an average weight loss of 4.2%, with 35.5% of participants achieving ≥ 5% weight loss [ 40 ]. Participants reported an average 152 min per week of physical activity, with 41.8% meeting the physical activity goal of 150 min per week. Participants with longer retention and greater participation in the program and who were more physically active were more likely to have higher weight loss [ 40 ].
Implementation of the National DPP: New Insights
Analysis sample.
To further assess National DPP implementation success, we examined recent DPRP data and analyzed new variables (education level, enrollment source, and payer source) to assess their relationship with participant outcomes. More than 297,000 participants attended one or more lifestyle change program sessions between February 2012 and January 2019. For the purpose of this new analysis, 143,489 eligible participants across all program modalities (in-person, online, distance learning, and combination) who completed the yearlong lifestyle change program and attended ≥ 3 sessions in the first 6 months (analyzed participants) were included in descriptive analyses. Of those, 29,069 eligible participants who started the program in 2018 and reported new variables (education level, enrollment source, and payer source) were included in the multivariable analysis (Fig. 1 ).
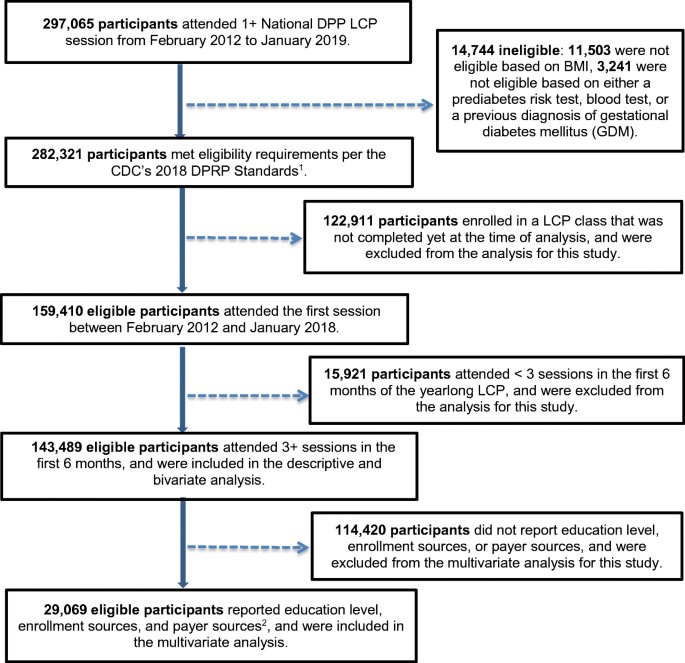
Flow chart for analysis sample. CDC = Centers for Disease Control and Prevention; DPRP = Diabetes Prevention Recognition Program; LCP = CDC-recognized lifestyle change program; National DPP = National Diabetes Prevention Program. 1 Participant eligibility was based on BMI (≥ 25 kg/m 2 , or ≥ 23 kg/m 2 if Asian American), a blood test indicating prediabetes, a CDC Prediabetes Screening Test or American Diabetes Association Type 2 Diabetes Risk Test, or a previous diagnosis of gestational diabetes mellitus. 2 Education level, enrollment sources, and payer sources were not collected before February 2018
Measures and Methods
Percent body weight change was the primary outcome of this analysis, calculated among participants with at least 2 documented body weights using the first (baseline) and last recorded weights. Average weight loss and physical activity minutes per week were calculated among participants who attended ≥ 3 sessions in the first 6 months and whose time from first session attended to last session attended was ≥ 9 months. CDC considers this threshold to be the minimum dose to begin seeing lifestyle and weight change that can impact type 2 diabetes [ 40 ].
Mantel-Haenszel chi-square tests of difference were used to assess bivariate associations between participants’ characteristics and duration of participation. Results were stratified by duration of participation (≥ 9 vs. < 9 months). Multiple logistic regression models were used to estimate the association between participants’ attendance and duration of participation and the likelihood of meeting the minimum 5% weight loss goal conditional on other factors. Adjusted odds ratios (AORs) in relation to a reference category were reported with their respective 95% confidence intervals (CIs). Results with p < 0.05 were considered statistically significant. All analyses were conducted using SAS, version 9.4.
Overall, about 60% of analyzed participants attended the lifestyle change via an online-only modality, 40% via an in-person only modality, and < 1% via distance learning only or combination modality (Table 1 ). About 40% of analyzed participants attended at least 17 lifestyle change sessions; 31% met the minimum 5% weight loss goal, and 45% met the average 150+ min per week of physical activity goal. Three quarters were females; over half were aged 45–64 years; over 60% were non-Hispanic whites; about half reported having 4 years of college or more; and over 70% had obesity (body mass index (BMI) ≥ 30 kg/m 2 ).
Weight loss success was significantly higher among those who attended ≥ 17 sessions (AOR 3.2), those who stayed in the program for ≥ 9 months (AOR 1.3), and those with ≥ 150 min of physical activity per week (AOR 1.7) (Table 2 ). Participants aged 45–64 and ≥ 65 years had 3.2 and 1.6 times, respectively, the odds of meeting the 5% weight loss goal than those aged 18–44. Females, non-Hispanic blacks, and those with obesity were slightly less likely to meet the 5% weight loss goal than males, non-Hispanic whites, and those overweight (BMI between 25 and 29.9 kg/m 2 ).
This analysis is subjected to some limitations. First, biometric data (weight and physical activity minutes) was self-reported either by CDC-recognized organizations or participants themselves. However, lifestyle coaches were provided guidance to use the same scale at each session for recording participants’ body weight to ensure consistency. Second, calculation of percent weight loss was based on first and last recorded weight. Participants who lost more weight might be more likely to stay in the program longer and continue to lose more weight by the end of the program than those who dropped out early. Third, CDC’s DPRP began requiring organizations to submit information on participants’ education level, enrollment source, and payer source in February 2018; thus, our analyses were limited to a small sample of participants who started the program in 2018 (~ 10% of the total National DPP participants), as the remaining participants had not yet had the opportunity to participate in the program for a year prior to our study. Lastly, the analysis only included eligible participants based on CDC’s DPRP Standards, so it may not be generalizable to ineligible participants who may also benefit from this program.
Participation in Lifestyle Change Intervention
Successful expansion of lifestyle change interventions relies on sufficient enrollment and retention. A recent analysis of 2016–2017 U.S. National Health Interview Survey data showed that 73.5% of adults with overweight or obesity with diagnosed prediabetes and 50.6% of adults with overweight or obesity and elevated American Diabetes Association (ADA) risk scores reported receiving risk reduction advice or referrals to risk reduction activities from their health care providers [ 41 •]. Of those referred, only one third reported engagement in risk-reducing activities in the past year, and less than 3% reported participating in a type 2 diabetes prevention program. The key drivers for engagement in risk-reducing activities and programs included receiving advice from a health professional, having higher education, having insurance, being non-white race/ethnicity, and being middle aged. This study underscores the need for research from fields such as behavioral economics, human-centered design, and habit formation, to understand how to best engage people at high risk in type 2 diabetes prevention programs. Other approaches for systems change include the establishment of referral processes from clinical health care providers to community-based implementation programs, and media and marketing strategies to drive traffic to such programs. A recent study found that primary care providers in the USA who were aware of the National DPP lifestyle change program and the Prevent Diabetes STAT: Screen, Test, and Act Today™ Toolkit developed by CDC and the American Medical Association (AMA) were more likely to screen patients for prediabetes and make referrals to CDC-recognized organizations offering the National DPP. Those who used electronic health records were also more likely to screen, test, and refer [ 42 ].
Offering diabetes prevention programs via businesses and worksites could help lower employee health care costs and increase QALYs, and is an important systems approach still in need of broader consideration among employers [ 43 ]. Evidence-based programs within worksite wellness programs and health-based interventions within worksites are increasing in scope in the USA, and seem to be slowly gaining traction elsewhere in the world as well. To assist employers and insurers in determining the feasibility of providing the National DPP lifestyle change program or including it as part of their employees’ insurance benefits, CDC developed the Diabetes Prevention Impact Toolkit [ 43 ]. The toolkit helps estimate the cost per employee and the associated cost savings related to offering the National DPP lifestyle change program, including estimating the employee’s QALYs gained. A summary of recent peer-reviewed literature on employer-based diabetes prevention programs in the USA found that greater weight loss and maintenance of weight loss were achieved among worksites that implemented the National DPP lifestyle change program compared to worksites that implemented other interventions [ 44 ].
Another key approach to increasing participation focuses on raising awareness of both prediabetes as a serious condition and of type 2 diabetes prevention activities. This is a challenge, as many providers are not screening or testing patients for prediabetes when considering its risk factors (obesity, overweight, glycemic range, and cardiovascular risks) In the USA, CDC, ADA, and AMA partnered with the Ad Council to launch the nation’s first national public service campaign about prediabetes [ 45 ] which encourages people to take a short online test at DoIHavePrediabetes.org to learn their prediabetes risk. From the campaign’s launch on January 21, 2016, through March 31, 2019, 2.5 million people have completed the online risk test [ 46 ].
Whole-Population Approaches
Because of the widespread prevalence of type 2 diabetes and its risk factors, lifestyle change programs for the highest-risk people alone cannot sufficiently impact the diabetes pandemic without approaches that support prevention efforts across whole systems and communities. Larger-scale, population-wide prevention strategies, such as environmental, policy, cost reimbursement, and health marketing/awareness efforts, are therefore needed. Several whole-population approaches have been implemented in the USA. Many focus on socioeconomic policy involving nutrition regulation such as menu labeling; subsidies to increase the affordability of fruits and vegetables, particularly in rural or hard-to-reach areas [ 47 , 48 ]; sugar-sweetened beverage tax and decreased added sugars; and increased whole grains, fibers, nuts, and legumes and elimination of trans fats (or trans fatty acids) as recommended by the American Heart Association [ 49 ]. Globally, supported by WHO, clear nutrition “front-of-pack labeling” has been found to improve dietary habits and reduce cardiovascular complications [ 50 ].
A systematic review found strong evidence in Europe for implementing multiple policies simultaneously, including taxes on unhealthy food, subsidies for healthy food, trans fat elimination, and trade agreements with supportive countries who implement similar taxation and food policies [ 51 ••]. A 2014 systematic review of the evidence behind food taxation and food subsidies (government/local investments in healthy food) found that both should be implemented in tandem at a minimum rate of 10 to 15% to ensure the unhealthy foods are less accessible and healthy foods/beverages are more accessible to purchasers [ 52 ]. Similarly, Colchero et al. studied the effect of taxing sugar-sweetened beverages in stores in Mexico and found reductions in purchases of the taxed beverages associated with increases in purchases of untaxed beverages [ 53 ].
Environmental changes such as targeting the built environment and community planning efforts also show promise in reaching large populations. These approaches include the expansion or building of walking, biking, and hiking trials, and other “safe” routes [ 54 ]. A systematic review evaluating the effect of built environment policies on obesity-related outcomes across the USA, Canada, Chile, the UK, and New Zealand found that physical activity-related policies had a stronger impact when they involved improvements to transportation infrastructure. These improvements included creating more structural access such as building cycling lanes and park trails [ 55 ].
A possible limitation of whole-population approaches is that researchers have to rely largely on modeling studies or intermediate outcomes to determine impact. Short-term and longitudinal impact testing involving actual health and economic trends is more difficult, but critical to assessing intervention success. Also critical is understanding the contexts in which whole-population approaches are most effective.
To achieve large-scale type 2 diabetes prevention, interventions directed to both high-risk populations and the general population are necessary. There is strong evidence for the prevention of type 2 diabetes from RCTs and subsequent translation studies in which people at high risk engage in a structured lifestyle intervention that addresses nutrition, physical activity, and behavior change strategies resulting in a weight loss of ≥ 5%. Whole-population strategies also show promise in reaching large numbers of people and include multi-sector and multi-policy approaches, most successfully in combination. These approaches include taxation of unhealthy foods, enhancing the built environment, addressing food accessibility, offering worksite wellness, and raising awareness of prediabetes among health care providers and the general public.
Large-scale implementation of what has been proven to work, including alternate delivery approaches for type 2 diabetes prevention programs to reach disparate and geographically isolated populations, is needed. Continued examination of outcomes and program effectiveness is needed to refine global prevention efforts. Fortunately, there is much evidence worldwide that type 2 diabetes prevention or delay is attainable, and that prevention strategies can be adapted across cultures and environments. Success will require a combination of policy, systems, environmental, and health marketing/awareness approaches with effective interventions for high-risk populations and partnerships across sectors. Based on the data showing the impact of diabetes it warrants being prioritized to protect the public’s health around the world and curb the increasing burden of diabetes.
Change history
27 june 2020.
The sentence should read: ��� Average weight loss and physical activity minutes per week were calculated among participants who attended���������3 sessions in the first 6��months and whose time from first session attended to last session attended was���������9��months.
Papers of particular interest, published recently, have been highlighted as: • Of importance •• Of major importance
• International Diabetes Federation. Diabetes atlas 8 th edition 2017 global fact sheet. 2017. Available at https://www.idf.org/aboutdiabetes/what-is-diabetes/facts-figures.html . Accessed April 14, 2019. Findings indicate the significant and costly economic and disease burden that diabetes has across the world.
Centers for Disease Control and Prevention. Diabetes 2017 report card. 2017. Available at https://www.cdc.gov/diabetes/pdfs/library/diabetesreportcard2017-508.pdf . Accessed April 14, 2019.
• American Diabetes Association. Economic costs of diabetes in the U.S. in 2017. Diabetes Care. 2018;41(5):917–28. https://doi.org/10.2337/dci18-0007 Findings indicate the significant and costly economic and productivity burden that diabetes has in the USA.
Article PubMed Central Google Scholar
Tuomilehto J, Lindstrom J, Eriksson JG, Valle TT, Hamalainen H, Ilanne-Parikka P, et al. Prevention of type 2 diabetes mellitus by changes in lifestyle among subjects with impaired glucose tolerance. N Engl J Med. 2001;344(18):1343–50. https://doi.org/10.1056/nejm200105033441801.
Article CAS PubMed Google Scholar
Noble D, Mathur R, Dent T, Meads C, Greenhalgh T. Risk models and scores for type 2 diabetes: systematic review. Br Med J (Clin Res Ed). 2011;343:d7163.
Article Google Scholar
Modesti PA, Galanti G, Cala P, Calabrese M. Lifestyle interventions in preventing new type 2 diabetes in Asian populations. Intern Emerg Med. 2016;11(3):375–84. https://doi.org/10.1007/s11739-015-1325-2 .
Article PubMed Google Scholar
Pearson-Stuttard J, Bandosz P, Rehm CD, Penalvo J, Whitsel L, Gaziano T, et al. Reducing US cardiovascular disease burden and disparities through national and targeted dietary policies: a modelling study. PLoS Med. 2017;14(6):e1002311. https://doi.org/10.1371/journal.pmed.1002311 .
Article PubMed PubMed Central Google Scholar
Konchak JN, Moran MR, O'Brien MJ, Kandula NR, Ackermann RT. The state of diabetes prevention policy in the USA following the Affordable Care Act. Curr Diab Rep. 2016;16(6):55. https://doi.org/10.1007/s11892-016-0742-6.
Pan XR, Li GW, Hu YH, Wang JX, Yang WY, An ZX, et al. Effects of diet and exercise in preventing NIDDM in people with impaired glucose tolerance. The Da Qing IGT and Diabetes Study. Diabetes Care. 1997;20(4):537–44.
Article CAS Google Scholar
Li G, Zhang P, Wang J, Gregg EW, Yang W, Gong Q, et al. The long-term effect of lifestyle interventions to prevent diabetes in the China Da Qing Diabetes Prevention Study: a 20-year follow-up study. Lancet. 2008;371(9626):1783–9. https://doi.org/10.1016/s0140-6736(08)60766-7 .
Gong Q, Gregg EW, Wang J, An Y, Zhang P, Yang W, et al. Long-term effects of a randomised trial of a 6-year lifestyle intervention in impaired glucose tolerance on diabetes-related microvascular complications: the China Da Qing Diabetes Prevention Outcome Study. Diabetologia. 2011;54(2):300–7. https://doi.org/10.1007/s00125-010-1948-9 .
Gong Q, Zhang P, Wang J, Ma J, An Y, Chen Y, et al. Morbidity and mortality after lifestyle intervention for people with impaired glucose tolerance: 30-year results of the Da Qing Diabetes Prevention Outcome Study. Lancet Diabetes Endocrinol. 2019. https://doi.org/10.1016/S2213-8587(19)30093-2 .
•• Knowler WC, Barrett-Connor E, Fowler SE, Hamman RF, Lachin JM, Walker EA, et al. Reduction in the incidence of type 2 diabetes with lifestyle intervention or metformin. N Engl J Med. 2002;346(6):393–403. https://doi.org/10.1056/NEJMoa012512 Findings from this study demonstrate that diabetes incidence reduction in those at high risk for type 2 diabetes is possible with both lifestyle interventions and metformin use, but greater through lifestyle intervention overall.
Knowler WC, Fowler SE, Hamman RF, Christophi CA, Hoffman HJ, Brenneman AT, et al. 10-year follow-up of diabetes incidence and weight loss in the Diabetes Prevention Program Outcomes Study. Lancet. 2009;374(9702):1677–86. https://doi.org/10.1016/s0140-6736(09)61457-4 .
Diabetes Prevention Program Research Group. Long-term effects of lifestyle intervention or metformin on diabetes development and microvascular complications over 15-year follow-up: the Diabetes Prevention Program Outcomes Study. Lancet Diabetes Endocrinol. 2015;3(11):866–75. https://doi.org/10.1016/s2213-8587(15)00291-0 .
Diabetes Prevention Program Research Group. The 10-year cost-effectiveness of lifestyle intervention or metformin for diabetes prevention: an intent-to-treat analysis of the DPP/DPPOS. Diabetes Care. 2012;35(4):723–30. https://doi.org/10.2337/dc11-1468 .
The Diabetes Prevention Program Research Group. The Diabetes Prevention Program: design and methods for a clinical trial in the prevention of type 2 diabetes. Diabetes Care. 1999;22(4):623–34.
Lindstrom J, Ilanne-Parikka P, Peltonen M, Aunola S, Eriksson JG, Hemio K, et al. Sustained reduction in the incidence of type 2 diabetes by lifestyle intervention: follow-up of the Finnish Diabetes Prevention Study. Lancet. 2006;368(9548):1673–9. https://doi.org/10.1016/s0140-6736(06)69701-8 .
Makrilakis K, Liatis S, Grammatikou S, Perrea D, Katsilambros N. Implementation and effectiveness of the first community lifestyle intervention programme to prevent type 2 diabetes in Greece. The DE-PLAN study. Diabet Med. 2010;27(4):459–65. https://doi.org/10.1111/j.1464-5491.2010.02918.x .
Kosaka K, Noda M, Kuzuya T. Prevention of type 2 diabetes by lifestyle intervention: a Japanese trial in IGT males. Diabetes Res Clin Pract. 2005;67(2):152–62. https://doi.org/10.1016/j.diabres.2004.06.010 .
Ramachandran A, Snehalatha C, Mary S, Mukesh B, Bhaskar AD, Vijay V. The Indian Diabetes Prevention Programme shows that lifestyle modification and metformin prevent type 2 diabetes in Asian Indian subjects with impaired glucose tolerance (IDPP-1). Diabetologia. 2006;49(2):289–97. https://doi.org/10.1007/s00125-005-0097-z .
Ramachandran A, Snehalatha C, Yamuna A, Mary S, Ping Z. Cost-effectiveness of the interventions in the primary prevention of diabetes among Asian Indians: within-trial results of the Indian Diabetes Prevention Programme (IDPP). Diabetes Care. 2007;30(10):2548–52. https://doi.org/10.2337/dc07-0150 .
Davidson A. Translational research: what does it mean? Anesthesiology. 2011;115(5):909–11. https://doi.org/10.1097/ALN.0b013e3182337a5e .
Ali MK, Echouffo-Tcheugui J, Williamson DF. How effective were lifestyle interventions in real-world settings that were modeled on the Diabetes Prevention Program? Health Aff (Millwood). 2012;31(1):67–75. https://doi.org/10.1377/hlthaff.2011.1009 .
•• Gilis-Januszewska A, Piwonska-Solska B, Lindstrom J, Wojtowicz E, Tuomilehto J, Schwarz PEH, et al. Determinants of weight outcomes in type 2 diabetes prevention intervention in primary health care setting (the DE-PLAN project). BMC Public Health. 2018;18(1):97. https://doi.org/10.1186/s12889-017-4977-1 Findings from this study indicate that persons with a higher body mass index, increased glucose, and lower education (i.e., at higher risk for type 2 diabetes) achieved successful weight loss of 5% or more after a yearlong lifestyle change intervention as compared to those with lower risk for type 2 diabetes.
Thomas C, Sadler S, Breeze P, Squires H, Gillett M, Brennan A. Assessing the potential return on investment of the proposed UK NHS diabetes prevention programme in different population subgroups: an economic evaluation. Br Med J Open. 2017;7(8):e014953. https://doi.org/10.1136/bmjopen-2016-014953 .
Laatikainen T, Dunbar JA, Chapman A, Kilkkinen A, Vartiainen E, Heistaro S, et al. Prevention of type 2 diabetes by lifestyle intervention in an Australian primary health care setting: Greater Green Triangle (GGT) Diabetes Prevention Project. BMC Public Health. 2007;7:249. https://doi.org/10.1186/1471-2458-7-249 .
Janus ED, Best JD, Davis-Lameloise N, Philpot B, Hernan A, Bennett CM, et al. Scaling-up from an implementation trial to state-wide coverage: results from the preliminary Melbourne Diabetes Prevention Study. Trials. 2012;13:152. https://doi.org/10.1186/1745-6215-13-152 .
Tate DF, Jackvony EH, Wing RR. Effects of internet behavioral counseling on weight loss in adults at risk for type 2 diabetes: a randomized trial. JAMA. 2003;289(14):1833–6. https://doi.org/10.1001/jama.289.14.1833 .
Ramachandran A, Snehalatha C, Ram J, Selvam S, Simon M, Nanditha A, et al. Effectiveness of mobile phone messaging in prevention of type 2 diabetes by lifestyle modification in men in India: a prospective, parallel-group, randomised controlled trial. Lancet Diabetes Endocrinol. 2013;1(3):191–8. https://doi.org/10.1016/s2213-8587(13)70067-6 .
Nanditha A, Snehalatha C, Raghavan A, Vinitha R, Satheesh K, Susairaj P, et al. The post-trial analysis of the Indian SMS diabetes prevention study shows persistent beneficial effects of lifestyle intervention. Diabetes Res Clin Pract. 2018;142:213–21. https://doi.org/10.1016/j.diabres.2018.05.042 .
Centers for Medicare & Medicaid Services. 2016 annual report of the Boards of Trustees of the Federal Hospital Insurance and Federal Supplementary Medical Insurance Trust Funds. Available at https://www.cms.gov/Research-Statistics-Data-and-Systems/Statistics-Trends-and-Reports/ReportsTrustFunds/downloads/tr2016.pdf . Accessed April 14, 2019.
Herman WH. The economics of diabetes prevention. Med Clin North Am. 2011;95(2):373–84, viii . https://doi.org/10.1016/j.mcna.2010.11.010 .
US Congress. H.R.4124 - Diabetes Prevention Act of 2009. Available at https://www.congress.gov/bill/111th-congress/house-bill/4124 . Accessed April 14, 2019.
Albright AL, Gregg EW. Preventing type 2 diabetes in communities across the U.S.: the National Diabetes Prevention Program. Am J Prev Med. 2013;44(4 Suppl 4):S346–51. https://doi.org/10.1016/j.amepre.2012.12.009 .
Centers for Disease Control and Prevention. Diabetes Prevention Recognition Program data dashboard. Accessed April 11, 2019.
Centers for Disease Control and Prevention. 2018 Diabetes Prevention Recognition Program standards and operating procedures. Available at https://www.cdc.gov/diabetes/prevention/pdf/dprp-standards.pdf . Accessed April 14, 2019.
Centers for Disease Control and Prevention. Insurance coverage for the US National Diabetes Prevention Program State reported data. Accessed May 14, 2019.
Centers for Medicare & Medicaid Services. Medicare Diabetes Prevention Program (MDPP) expanded model. Available at https://innovation.cms.gov/initiatives/medicare-diabetes-prevention-program/ . Accessed April 14, 2019.
Ely EK, Gruss SM, Luman ET, Gregg EW, Ali MK, Nhim K, et al. A national effort to prevent type 2 diabetes: participant-level evaluation of CDC’s National Diabetes Prevention Program. Diabetes Care. 2017;40(10):1331–41. https://doi.org/10.2337/dc16-2099 .
• Ali MK, McKeever Bullard K, Imperatore G, Benoit SR, Rolka DB, Albright AL, et al. Reach and use of diabetes prevention services in the United States, 2016-2017. JAMA Netw Open. 2019;2(5):e193160. https://doi.org/10.1001/jamanetworkopen.2019.3160 Findings from this study offer the basis by which to assess future diabetes prevention lifestyle change programs and coverage for such programs, strategies for identification of prediabetes, and referral practices into such programs that lead to retention.
Nhim K, Khan T, Gruss SM, Wozniak G, Kirley K, Schumacher P, et al. Primary care providers’ prediabetes screening, testing, and referral behaviors. Am J Prev Med. 2018;55(2):e39–47. https://doi.org/10.1016/j.amepre.2018.04.017 .
Centers for Disease Control and Prevention. Diabetes prevention impact toolkit. Available at https://nccd.cdc.gov/toolkit/diabetesimpact . Accessed April 14, 2019.
Hafez D, Fedewa A, Moran M, O'Brien M, Ackermann R, Kullgren JT. Workplace interventions to prevent type 2 diabetes mellitus: a narrative review. Curr Diab Rep. 2017;17(2):9. https://doi.org/10.1007/s11892-017-0840-0.
Ad Council. Do I have prediabetes Available at DoIHavePrediabetes.org . Accessed April 14, 2019.
Centers for Disease Control and Prevention. Prediabetes campaign results report, April 2019 Accessed April 4, 2019.
Andreyeva T, Long MW, Brownell KD. The impact of food prices on consumption: a systematic review of research on the price elasticity of demand for food. Am J Public Health. 2010;100(2):216–22. https://doi.org/10.2105/ajph.2008.151415 .
Calancie L, Leeman J, Jilcott Pitts SB, Khan LK, Fleischhacker S, Evenson KR, et al. Nutrition-related policy and environmental strategies to prevent obesity in rural communities: a systematic review of the literature, 2002-2013. Prev Chronic Dis. 2015;12:E57. https://doi.org/10.5888/pcd12.140540 .
Olstad DL, Teychenne M, Minaker LM, Taber DR, Raine KD, Nykiforuk CI, et al. Can policy ameliorate socioeconomic inequities in obesity and obesity-related behaviours? A systematic review of the impact of universal policies on adults and children. Obes Rev. 2016;17(12):1198–217. https://doi.org/10.1111/obr.12457 .
Lichtenstein AH, Carson JS, Johnson RK, Kris-Etherton PM, Pappas A, Rupp L, et al. Food-intake patterns assessed by using front-of-pack labeling program criteria associated with better diet quality and lower cardiometabolic risk. Am J Clin Nutr. 2014;99(3):454–62. https://doi.org/10.3945/ajcn.113.071407 .
•• Hyseni L, Atkinson M, Bromley H, Orton L, Lloyd-Williams F, McGill R, et al. The effects of policy actions to improve population dietary patterns and prevent diet-related non-communicable diseases: scoping review. Eur J Clin Nutr. 2017;71(6):694–711. https://doi.org/10.1038/ejcn.2016.234 Findings are from a large, worldwide systematic and non-systematic review that highlights successful whole-population strategies for preventing and reducing non-communicable diseases.
Niebylski ML, Redburn KA, Duhaney T, Campbell NR. Healthy food subsidies and unhealthy food taxation: a systematic review of the evidence. Nutrition. 2015;31(6):787–95. https://doi.org/10.1016/j.nut.2014.12.010 .
Colchero MA, Popkin BM, Rivera JA, Ng SW. Beverage purchases from stores in Mexico under the excise tax on sugar sweetened beverages: observational study. Br Med J (Clin Res Ed). 2016;352:h6704.
Google Scholar
Albright A, Devlin H, and Zhang X: Integrating Nutrition Therapy into Community-Based Diabetes Prevention Programs (pp 579). In: Franz MJ, Evert AB, editors. American Diabetes Association Guide to Nutrition Therapy for Diabetes, 3rd Edition; 2012.
Mayne SL, Auchincloss AH, Michael YL. Impact of policy and built environment changes on obesity-related outcomes: a systematic review of naturally occurring experiments. Obes Rev. 2015;16(5):362–75. https://doi.org/10.1111/obr.12269 .
Article CAS PubMed PubMed Central Google Scholar
Download references
The findings and conclusions in this report are those of the authors and do not necessarily represent the official position of the Centers for Disease Control and Prevention. No fınancial disclosures were reported by the authors of this paper.
Author information
Authors and affiliations.
Division of Diabetes Translation, Centers for Disease Control and Prevention, 4770 Buford Hwy., Mailstop F75, Atlanta, GA, 30341, USA
Stephanie M. Gruss, Kunthea Nhim, Miriam Bell, Elizabeth Luman & Ann Albright
Department of Epidemiology and Biostatistics, School of Public Health, Imperial College London, London, UK
Edward Gregg
You can also search for this author in PubMed Google Scholar
Corresponding author
Correspondence to Stephanie M. Gruss .
Ethics declarations
Conflict of interest.
Stephanie M. Gruss, Kunthea Nhim, Edward Gregg, Miriam Bell, Elizabeth Luman, and Ann Albright declare that they have no conflict of interest.
Human and Animal Rights and Informed Consent
This article does not contain any studies with human or animal subjects performed by any of the authors.
Additional information
Publisher’s note.
Springer Nature remains neutral with regard to jurisdictional claims in published maps and institutional affiliations.
This article is part of the Topical Collection on Diabetes Epidemiology
Electronic supplementary material
(DOCX 49 kb)
Rights and permissions
Open Access This article is distributed under the terms of the Creative Commons Attribution 4.0 International License (http://creativecommons.org/licenses/by/4.0/), which permits unrestricted use, distribution, and reproduction in any medium, provided you give appropriate credit to the original author(s) and the source, provide a link to the Creative Commons license, and indicate if changes were made.
Reprints and permissions
About this article
Gruss, S.M., Nhim, K., Gregg, E. et al. Public Health Approaches to Type 2 Diabetes Prevention: the US National Diabetes Prevention Program and Beyond. Curr Diab Rep 19 , 78 (2019). https://doi.org/10.1007/s11892-019-1200-z
Download citation
Published : 05 August 2019
DOI : https://doi.org/10.1007/s11892-019-1200-z
Share this article
Anyone you share the following link with will be able to read this content:
Sorry, a shareable link is not currently available for this article.
Provided by the Springer Nature SharedIt content-sharing initiative
- Diabetes prevention
- National Diabetes Prevention Program
- Type 2 diabetes
- Diabetes policy
- Diabetes prevention program
- Find a journal
- Publish with us
- Track your research
Information
- Author Services
Initiatives
You are accessing a machine-readable page. In order to be human-readable, please install an RSS reader.
All articles published by MDPI are made immediately available worldwide under an open access license. No special permission is required to reuse all or part of the article published by MDPI, including figures and tables. For articles published under an open access Creative Common CC BY license, any part of the article may be reused without permission provided that the original article is clearly cited. For more information, please refer to https://www.mdpi.com/openaccess .
Feature papers represent the most advanced research with significant potential for high impact in the field. A Feature Paper should be a substantial original Article that involves several techniques or approaches, provides an outlook for future research directions and describes possible research applications.
Feature papers are submitted upon individual invitation or recommendation by the scientific editors and must receive positive feedback from the reviewers.
Editor’s Choice articles are based on recommendations by the scientific editors of MDPI journals from around the world. Editors select a small number of articles recently published in the journal that they believe will be particularly interesting to readers, or important in the respective research area. The aim is to provide a snapshot of some of the most exciting work published in the various research areas of the journal.
Original Submission Date Received: .
- Active Journals
- Find a Journal
- Proceedings Series
- For Authors
- For Reviewers
- For Editors
- For Librarians
- For Publishers
- For Societies
- For Conference Organizers
- Open Access Policy
- Institutional Open Access Program
- Special Issues Guidelines
- Editorial Process
- Research and Publication Ethics
- Article Processing Charges
- Testimonials
- Preprints.org
- SciProfiles
- Encyclopedia
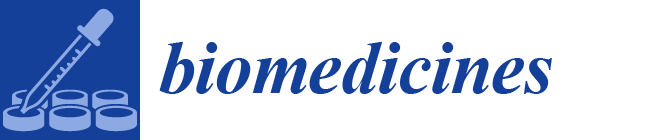
Article Menu
- Subscribe SciFeed
- Recommended Articles
- PubMed/Medline
- Google Scholar
- on Google Scholar
- Table of Contents
Find support for a specific problem in the support section of our website.
Please let us know what you think of our products and services.
Visit our dedicated information section to learn more about MDPI.
JSmol Viewer
Current advances in the management of diabetes mellitus.
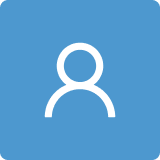
1. Introduction
3. risk factors of diabetes, 4. management of diabetes, 4.1. internet intervention for lifestyle modification in diabetes, 4.2. nanotechnology and diabetes, 4.3. medical nutrition therapy in diabetes, 4.4. gene therapy and diabetes mellitus, 4.5. stem cell therapy in diabetes, 4.6. latest inventions in diabetes management, 4.6.1. drugs recently introduced, 4.6.2. drugs in the pipeline, 5. discussion of major findings, 6. conclusions, author contributions, institutional review board statement, informed consent statement, data availability statement, conflicts of interest.
- Giovannini, P.; Howes, M.J.R.; Edwards, S.E. Medicinal plants used in the traditional management of diabetes and its sequelae in Central America: A review. J. Ethnopharmacol. 2016 , 184 , 58–71. [ Google Scholar ] [ CrossRef ] [ PubMed ]
- International Diabetes Federation. Data from: IFD Diabetes Altas Globally. IDF Diabetes Atlas, 8th ed, 2017. Available online: https://diabetesatlas.org/upload/resources/previous/files/8/IDF_DA_8e-EN-final.pdf (accessed on 11 May 2022).
- WHO. Global Report on Diabetes ; WHO: Geneva, Switzerland, 2016; Available online: http://www.who.int/diabetes/global-report/en/ (accessed on 11 May 2022).
- Wu, Y.; Ding, Y.; Tanaka, Y.; Zhang, W. Risk Factors Contributing to Type 2 Diabetes and Recent Advances in the Treatment and Prevention. Int. J. Med. Sci. 2014 , 11 , 1185–1200. [ Google Scholar ] [ CrossRef ]
- Group, T.D.P. Incidence and trends of childhood Type 1 diabetes worldwide 1990–1999. Diabet. Med. 2006 , 23 , 857–866. [ Google Scholar ] [ CrossRef ]
- Rask-Madsen, C.; King, G.L. Vascular complications of diabetes: Mechanisms of injury and protective factors. Cell Metab. 2013 , 17 , 20–33. [ Google Scholar ] [ CrossRef ] [ PubMed ]
- Diabetes Control and Complications Trial Research Group; Nathan, D.M.; Genuth, S.; Lachin, J.; Cleary, P.; Crofford, O.; Davis, M.; Rand, L.; Siebert, C. The effect of Intensive Treatment of diabetes on the development and progression of ling-term complications in insulin-dependent diabetes mellitus. N. Engl. J. Med. 1993 , 329 , 977–986. [ Google Scholar ] [ PubMed ]
- Callejas, D.; Mann, C.J.; Ayuso, E.; Lage, R.; Grifoll, I.; Roca, C.; Andaluz, A.; Ruiz-de Gopegui, R.; Montané, J.; Muñoz, S.; et al. Treatment of diabetes and long-term survival after insulin and glucokinase gene therapy. Diabetes 2013 , 62 , 1718–1729. [ Google Scholar ] [ CrossRef ] [ PubMed ]
- Palmer, S.C.; Mavridis, D.; Nicolucci, A.; Johnson, D.W.; Tonelli, M.; Craig, J.C.; Maggo, J.; Gray, V.; De Berardis, G.; Ruospo, M.; et al. Comparison of clinical outcomes and adverse events associated with glucose-lowering drugs in patients with type 2 diabetes a meta-analysis. J. Am. Med. Assoc. 2016 , 316 , 313–324. [ Google Scholar ] [ CrossRef ] [ PubMed ]
- Defronzo, R.; Fleming, G.A.; Chen, K.; Bicsak, T.A. Metformin-associated lactic acidosis: Current perspectives on causes and risk. Metab. Clin. Exp. 2016 , 65 , 20–29. [ Google Scholar ] [ CrossRef ]
- Banting, F.G.; Best, C.H. Pancreatic extracts. J. Lab. Clin. Med. 1990 , 115 , 254–272. [ Google Scholar ] [ PubMed ]
- Control, T.D.; Trial, C. Hypoglycemia in the diabetes control and complications trial. Diabetes 1997 , 46 , 271–286. [ Google Scholar ] [ CrossRef ]
- Group, T.D.R. Weight gain associated with intensive therapy in the diabetes control and complications trial. Diabetes Care 1988 , 11 , 567–573. [ Google Scholar ] [ CrossRef ]
- Meek, T.H.; Morton, G.J. The role of leptin in diabetes: Metabolic effects. Diabetologia 2016 , 59 , 928–932. [ Google Scholar ] [ CrossRef ] [ PubMed ]
- Noh, J.-W.; Jung, J.H.; Park, J.E.; Lee, J.H.; Sim, K.H.; Park, J.; Kim, M.H.; Yoo, K.-B. The relationship between age of onset and risk factors including family history and life style in Korean population with type 2 diabetes mellitus. J. Phys. Ther. Sci. 2018 , 30 , 201–206. [ Google Scholar ] [ CrossRef ]
- Asiimwe, D.; Mauti, G.O.; Kiconco, R. Prevalence and Risk Factors Associated with Type 2 Diabetes in Elderly Patients Aged 45–80 Years at Kanungu District. J. Diabetes Res. 2020 , 2020 , 5152146. [ Google Scholar ] [ CrossRef ]
- Mordarska, K.; Godziejewska-Zawada, M. Diabetes in the elderly. Prz. Menopauzalny 2017 , 16 , 38–43. [ Google Scholar ] [ CrossRef ] [ PubMed ]
- Ye, J. Mechanisms of insulin resistance in obesity. Front. Med. China 2013 , 7 , 14–24. [ Google Scholar ] [ CrossRef ]
- FDA. Cigarette Smoking: A Risk Factor for Type 2 Diabetes. 2020. Available online: https://www.fda.gov/tobacco-products/health-effects-tobacco-use/cigarette-smoking-risk-factor-type-2-diabetes (accessed on 20 July 2022).
- Herman, W.H.; Ye, W.; Griffin, S.J.; Simmons, R.K.; Davies, M.J.; Khunti, K.; Rutten, G.E.H.M.; Sandbaek, A.; Lauritzen, T.; Borch-Johnsen, K.; et al. Early detection and treatment of type 2 diabetes reduce cardiovascular morbidity and mortality: A simulation of the results of the Anglo-Danish-Dutch study of intensive treatment in people with screen-detected diabetes in primary care (ADDITION-Europe). Diabetes Care 2015 , 38 , 1449–1455. [ Google Scholar ] [ CrossRef ] [ PubMed ]
- Franz, M.J. Lifestyle modifications for diabetes management. Endocrinol. Metab. Clin. North Am. 1997 , 26 , 499–510. [ Google Scholar ] [ CrossRef ]
- Chong, S.; Ding, D.; Byun, R.; Comino, E.; Bauman, A.; Jalaludin, B. Lifestyle changes after a diagnosis of type 2 diabetes. Diabetes Spectr. 2017 , 30 , 43–50. [ Google Scholar ] [ CrossRef ]
- Cotter, A.P.; Durant, N.; Agne, A.A.; Cherrington, A.L. Internet interventions to support lifestyle modification for diabetes management: A systematic review of the evidence. J. Diabetes Its Complicat. 2014 , 28 , 243–251. [ Google Scholar ] [ CrossRef ] [ Green Version ]
- Veiseh, O.; Tang, B.C.; Whitehead, K.A.; Anderson, D.G.; Langer, R. Managing diabetes with nanomedicine: Challenges and opportunities. Nat. Rev. Drug Discov. 2014 , 14 , 45–57. [ Google Scholar ] [ CrossRef ]
- Disanto, R.M.; Subramanian, V.; Gu, Z. Recent advances in nanotechnology for diabetes treatment. Wiley Interdiscip. Rev. Nanomed. Nanobiotechnol. 2015 , 7 , 548–564. [ Google Scholar ] [ CrossRef ] [ PubMed ]
- Lemmerman, L.R.; Das, D.; Higuita-Castro, N.; Mirmira, R.G.; Gallego-Perez, D. Nanomedicine-Based Strategies for Diabetes: Diagnostics, Monitoring, and Treatment. Trends Endocrinol. Metab. 2020 , 31 , 448–458. [ Google Scholar ] [ CrossRef ] [ PubMed ]
- Weir, G.C.; Bonner-Weir, S. Five stages of evolving beta-cell dysfunction during progression to diabetes. Diabetes 2004 , 53 (Suppl. 3). [ Google Scholar ] [ CrossRef ] [ PubMed ]
- Tamborlane, W.; Beck, R.; Bode, B.; Buckingham, B.; Chase, H.; Clemons, R.; Fiallo-Scharer, R.; Fox, L.; Gilliam, L.; Hirsch, I.; et al. Continuous glucose monitoring and intensive treatment of type 1 diabetes. N. Engl. J. Med. 2008 , 359 , 1464–1476. [ Google Scholar ] [ CrossRef ]
- Edelman, S.V.; Argento, N.B.; Pettus, J.; Hirsch, I.B. Clinical implications of real-time and intermittently scanned continuous glucose monitoring. Diabetes Care 2018 , 41 , 2265–2274. [ Google Scholar ] [ CrossRef ]
- Hovorka, R.; Nodale, M.; Haidar, A.; Wilinska, M.E. Assessing performance of closed-loop insulin delivery systems by continuous glucose monitoring: Drawbacks and way forward. Diabetes Technol. Ther. 2013 , 15 , 4–12. [ Google Scholar ] [ CrossRef ] [ PubMed ]
- Scognamiglio, V. Nanotechnology in glucose monitoring: Advances and challenges in the last 10 years. Biosens. Bioelectron. 2013 , 47 , 12–25. [ Google Scholar ] [ CrossRef ]
- Grunberger, G. The need for better insulin therapy. Diabetes Obes. Metab. 2013 , 15 (Suppl. 1), 1–5. [ Google Scholar ] [ CrossRef ]
- Lagopati, N.; Pavlatou, E. Nanotechnology in Diabetes Management. Interv. Obes. Diabetes 2021 , 5 , 419–424. [ Google Scholar ] [ CrossRef ]
- Garber, A.J.; Abrahamson, M.J.; Barzilay, J.I.; Blonde, L.; Bloomgarden, Z.T.; Bush, M.A.; Dagogo-Jack, S.; DeFronzo, R.A.; Einhorn, D.; Fonseca, V.A.; et al. Consensus statement by the American association of clinical endocrinologists and American college of endocrinology on the comprehensive type 2 diabetes management algorithm—2018 executive summary. Endocr. Pract. 2018 , 24 , 91–120. [ Google Scholar ] [ CrossRef ]
- Viswanathan, V.; Krishnan, D.; Kalra, S.; Chawla, R.; Tiwaskar, M.; Saboo, B.; Baruah, M.; Chowdhury, S.; Makkar, B.M.; Jaggi, S. Insights on Medical Nutrition Therapy for Type 2 Diabetes Mellitus: An Indian Perspective. Adv. Ther. 2019 , 36 , 520–547. [ Google Scholar ] [ CrossRef ]
- Institute of Medicine. Dietary Reference Intakes: Energy, Carbohydrate, Fiber, Fat, Fatty Acids, Cholesterol, Protein, and Amino Acids (Macronutrients) ; National Academy Press: Washington, DC, USA, 2005. [ Google Scholar ]
- Moreno-Castilla, C.; Mauricio, D.; Hernandez, M. Role of Medical Nutrition Therapy in the Management of Gestational Diabetes Mellitus. Curr. Diabetes Rep. 2016 , 16 , 22. [ Google Scholar ] [ CrossRef ]
- Ley, S.H.; Hamdy, O.; Mohan, V.; Hu, F.B. Prevention and management of type 2 diabetes: Dietary components and nutritional strategies. Lancet 2014 , 383 , 1999–2007. [ Google Scholar ] [ CrossRef ]
- Dunbar, C.E.; High, K.A.; Joung, J.K.; Kohn, D.B.; Ozawa, K.; Sadelain, M. Gene therapy comes of age. Science 2018 , 175. [ Google Scholar ] [ CrossRef ]
- Xu, R.; Li, H.; Lai-yin, T.; Hsiang-fu, K.; Lu, H.; Lam, K. Diabetes Gene Therapy: Potential and Challenges. Curr. Gene Ther. 2003 , 3 , 65–82. [ Google Scholar ] [ CrossRef ]
- Wong, M.S.; Hawthorne, W.J.; Manolios, N. Gene therapy in diabetes. Self/Nonself 2010 , 1 , 165–175. [ Google Scholar ] [ CrossRef ]
- Mali, S. Delivery systems for gene therapy. Indian J. Hum. Genet. 2013 , 19 , 3–8. [ Google Scholar ] [ CrossRef ]
- Kaufmann, K.B.; Büning, H.; Galy, A.; Schambach, A.; Grez, M. Gene therapy on the move. EMBO Mol. Med. 2013 , 5 , 1642–1661. [ Google Scholar ] [ CrossRef ]
- Tsokos, G.C.; Nepom, G.T. Gene therapy in the treatment of autoimmune diseases. J. Clin. Investig. 2000 , 106 , 181–183. [ Google Scholar ] [ CrossRef ] [ Green Version ]
- Liu, X.; Zhang, S.; Li, X.; Zheng, P.; Hu, F.; Zhou, Z. Vaccination with a co-expression DNA plasmid containing GAD65 fragment gene and IL-10 gene induces regulatory CD4+ T cells that prevent experimental autoimmune diabetes. Diabetes/Metab. Res. Rev. 2016 , 32 , 522–533. [ Google Scholar ] [ CrossRef ]
- Chellappan, D.K.; Yap, W.S.; Bt Ahmad Suhaimi, N.A.; Gupta, G.; Dua, K. Current therapies and targets for type 2 diabetes mellitus. Panminerva Med. 2018 , 60 , 117–131. [ Google Scholar ] [ CrossRef ] [ PubMed ]
- Bakay, M.; Pandey, R.; Hakonarson, H. Genes involved in type 1 diabetes: An update. Genes 2013 , 4 , 499–521. [ Google Scholar ] [ CrossRef ] [ PubMed ]
- Kwak, S.H.; Park, K.S. Recent progress in genetic and epigenetic research on type 2 diabetes. Exp. Mol. Med. 2016 , 48 , e220–e228. [ Google Scholar ] [ CrossRef ]
- Florez, J.C. Pharmacogenetics in type 2 diabetes: Precision medicine or discovery tool? Diabetologia 2017 , 60 , 800–807. [ Google Scholar ] [ CrossRef ]
- Abderrazak, A.; El Hadri, K.; Bosc, E.; Blondeau, B.; Slimane, M.N.; Büchele, B.; Simmet, T.; Couchie, D.; Rouis, M. Inhibition of the inflammasome NLRP3 by arglabin attenuates inflammation, protects pancreatic β-cells from apoptosis, and prevents type 2 diabetes mellitus development in ApoE2Ki mice on a chronic high-fat diet. J. Pharmacol. Exp. Ther. 2016 , 357 , 487–494. [ Google Scholar ] [ CrossRef ]
- Yue, Z.; Zhang, L.; Li, C.; Chen, Y.; Tai, Y.; Shen, Y.; Sun, Z. Advances and potential of gene therapy for type 2 diabetes mellitus. Biotechnol. Biotechnol. Equip. 2019 , 33 , 1150–1157. [ Google Scholar ] [ CrossRef ]
- Mccall, M.D.; Toso, C.; Baetge, E.E.; Shapiro, A.M.J. Are stem cells a cure for diabetes? Clin. Sci. 2009 , 118 , 87–97. [ Google Scholar ] [ CrossRef ]
- Abdulazeez, S.S. Diabetes treatment: A rapid review of the current and future scope of stem cell research. Saudi Pharm. J. 2015 , 23 , 333–340. [ Google Scholar ] [ CrossRef ]
- Bonner-Weir, S.; Baxter, L.A.; Schuppin, G.T.; Smith, F.E. A second pathway for regeneration of adult exocrine and endocrine pancreas: A possible recapitulation of embryonic development. Diabetes 1993 , 42 , 1715–1720. [ Google Scholar ] [ CrossRef ]
- Bonner-Weir, S.; Taneja, M.; Weir, G.C.; Tatarkiewicz, K.; Song, K.H.; Sharma, A.; O’Neil, J.J. In vitro cultivation of human islets from expanded ductal tissue. Proc. Natl. Acad. Sci. USA 2000 , 97 , 7999–8004. [ Google Scholar ] [ CrossRef ] [ PubMed ]
- Gao, R.; Ustinov, J.; Pulkkinen, M.A.; Lundin, K.; Korsgren, O.; Otonkoski, T. Characterization of endocrine progenitor cells and critical factors for their differentiation in human adult pancreatic cell culture. Diabetes 2003 , 52 , 2007–2015. [ Google Scholar ] [ CrossRef ] [ PubMed ]
- Brazelton, T.R.; Rossi, F.M.; Keshet, G.I.; Blau, H.M. From Marrow to Brain: Expression of Neuronal Phenotypes in Adult Mice Timothy. Science 2000 , 290 , 1775–1779. [ Google Scholar ] [ CrossRef ] [ PubMed ]
- Krause, D.S.; Theise, N.D.; Collector, M.I.; Henegariu, O.; Hwang, S.; Gardner, R.; Neutzel, S.; Sharkis, S.J. Multi-organ, multi-lineage engraftment by a single bone marrow-derived stem cell. Cell 2001 , 105 , 369–377. [ Google Scholar ] [ CrossRef ]
- Jiang, Y.; Jahagirdar, B.N.; Reinhardt, R.L.; Schwartz, R.E.; Keene, C.D.; Ortiz-Gonzalez, X.R.; Reyes, M.; Lenvik, T.; Lund, T.; Blackstad, M.; et al. Pluripotency of mesenchymal stem cells derived from adult marrow. Nature 2002 , 418 , 41–49. [ Google Scholar ] [ CrossRef ] [ PubMed ]
- Ianus, A.; Holz, G.G.; Theise, N.D.; Hussain, M.A. In vivo derivation of glucose-competent pancreatic endocrine cells from bone marrow without evidence of cell fusion. J. Clin. Investig. 2003 , 111 , 843–850. [ Google Scholar ] [ CrossRef ]
- Hess, D.; Li, L.; Martin, M.; Sakano, S.; Hill, D.; Strutt, B.; Thyssen, S.; Gray, D.A.; Bhatia, M. Bone marrow-derived stem cells initiate pancreatic regeneration. Nat. Biotechnol. 2003 , 21 , 763–770. [ Google Scholar ] [ CrossRef ]
- Couri, C.E.B.; Oliveira, M.C.B.; Stracieri, A.B.P.L.; Moraes, D.A.; Madeira, M.I.A.; Malmegrim, K.C.R.; Simo, B.P.; Foss, M.C.; Burt, R.K. C-Peptide Levels and Insulin Independence Following Autologous Nonmyeloablative Hematopoietic Stem Cell Transplantation in Newly Diagnosed Type 1 Diabetes Mellitus. JAMA 2009 , 301 , 1573–1579. [ Google Scholar ] [ CrossRef ]
- Estrada, E.J.; Valacchi, F.; Nicora, E.; Brieva, S.; Esteve, C.; Echevarria, L.; Froud, T.; Bernetti, K.; Cayetano, S.M.; Velazquez, O.; et al. Combined treatment of intrapancreatic autologous bone marrow stem cells and hyperbaric oxygen in type 2 diabetes mellitus. Cell Transplant. 2008 , 17 , 1295–1304. [ Google Scholar ] [ CrossRef ]
- FDA. FDA Approves Novel, Dual-Targeted Treatment for Type 2 Diabetes. 2022. Available online: https://www.fda.gov/news-events/press-announcements/fda-approves-novel-dual-targeted-treatment-type-2-diabetes (accessed on 16 September 2022).
- Bertsch, T. An Introduction to Tirzepatide. Clin. Diabetes 2022 , 40 , 371–372. [ Google Scholar ] [ CrossRef ]
- Kawai, T.; Sun, B.; Yoshino, H.; Feng, D.; Suzuki, Y.; Fukazawa, M.; Nagao, S.; Wainscott, D.B.; Showalter, A.D.; Droz, B.A.; et al. Structural basis for GLP-1 receptor activation by LY3502970, an orally active nonpeptide agonist. Proc. Natl. Acad. Sci. USA 2020 , 117 , 29959–29967. [ Google Scholar ] [ CrossRef ]
- SCOHIA. SCO-094 GLP-1R/GIPR Dual Agonist. 2022. Available online: https://www.scohia.com/eng/sys/pipeline/sco-094/ (accessed on 16 September 2022).
- Piemonti, L.; Keymeulen, B.; Gillard, P.; Linn, T.; Bosi, E.; Rose, L.; Pozzilli, P.; Giorgino, F.; Cossu, E.; Daffonchio, L.; et al. Ladarixin, an inhibitor of the interleukin-8 receptors CXCR1 and CXCR2, in new-onset type 1 diabetes: A multicentre, randomized, double-blind, placebo-controlled trial. Diabetes Obes. Metab. 2022 , 24 , 1840–1849. [ Google Scholar ] [ CrossRef ] [ PubMed ]
- Zimmet, P.; Alberti, K.; Shaw, J. Global and societal implications of the diabetes epidemic. Nature 2001 , 414 , 782–787. [ Google Scholar ] [ CrossRef ] [ PubMed ]
- American Diabetes Association Task Force for Writing Nutrition Principles and Recommendations for the Management of Diabetes and Related Complications. American Diabetes Association position statement: Evidence-based nutrition principles and recommendations for the treatment and prevention of diabetes and related complications. J. Am. Diet. Assoc. 2002 , 102 , 109–118. [ Google Scholar ] [ CrossRef ]
- Casares, S.; Hurtado, A.; McEvoy, R.C.; Sarukhan, A.; von Boehmer, H.; Brumeanu, T. Down-regulation of diabetogenic CD4+ T cells by a soluble dimeric peptide-MHC class II chimera. Nature Inmmunol. 2002 , 3 , 383–391. [ Google Scholar ] [ CrossRef ]
- Dong, H.; Altomonte, J.; Morral, N.; Meseck, M.; Thung, S.N.; Woo, S.L.C. Basal insulin gene expression significantly improves conventional insulin therapy in type 1 diabetic rats. Diabetes 2002 , 51 , 130–138. [ Google Scholar ] [ CrossRef ]
- Sharma, N.R.; Rao, G.H.R. Diabetes Management: Expectations and Limitations. J. Diabetes Metab. 2016 , 7 , 4. [ Google Scholar ] [ CrossRef ]
- Touchefeu, Y.; Harrington, K.J.; Galmiche, J.P.; Vassaux, G. Review article: Gene therapy, recent developments and future prospects in gastrointestinal oncology. Aliment. Pharmacol. Ther. 2010 , 32 , 953–968. [ Google Scholar ] [ CrossRef ]
- Varga, C.M.; Hong, K.; Lauffenburger, D.A. Quantitative analysis of synthetic gene delivery vector design properties. Mol. Ther. 2001 , 4 , 438–446. [ Google Scholar ] [ CrossRef ]
- Samulski, R.J.; Sally, M.; Muzyczka, N. Adenoassociated viral vectors. In Development of Human Gene Therapy ; Cold Spring Habour Laboratory Press: Cold Spring Harbor, NY, USA, 1999; pp. 131–172. [ Google Scholar ]
- León-Quinto, T.; Jones, J.; Skoudy, A.; Burcin, M.; Soria, B. In vitro directed differentation of mouse embryonic stem cells into insulin-producing cells. Diabetologia 2004 , 47 , 1442–1451. [ Google Scholar ] [ CrossRef ] [ PubMed ] [ Green Version ]
- Holm, S. Time to reconsider stem cell ethics—The importance of induced pluripotent cells. J. Med. Ethics 2008 , 34 , 63–64. [ Google Scholar ] [ CrossRef ] [ PubMed ] [ Green Version ]
Class | Genes | Main Function |
---|---|---|
Genes modulating homeostasis of glucose | GLUTs | Involved in the re-absorption of filtered glucose from the kidney into the bloodstream |
SGLTs | Partake profoundly in muscle and hepatic glucose fluxes | |
FGFs | Functions significantly in the homeostasis of glucose | |
SIRT6 | Connected with an expression of GLUTs and increased glycolysis | |
Genes enhancing the secretion of insulin and/or sensitivity | GLP-1 and its analogs/agonists | Boost the survival of beta-cell, provoke the expression of the insulin gene, and synthesis |
GPGRs and their agonists | Enhances the secretion of insulin and GLP-1 | |
CTB-APSL | Enhances secretion of insulin and insulin resistance | |
IKK E, TBK1 | Linked with diminution in weight, insulin resistance, fatty liver as well as inflammation | |
Genes attenuating diabetic induced complications | IL-1b | Linked with inflammation and b-cell failure |
ADPN | Attenuates diabetic nephropathy | |
TGF-a | Has a function in DKD linked with nephron reduction | |
NLRP3 | Attenuates diabetic cardiomyopathy | |
CDKN2A/2B | connected with modulation of T-cell phenotype and chronic inflammation | |
HSP70 | Connected with bioenergetics of mitochondrion and diabetic sensory neuropathy | |
MicroRNAs | Implicated in the modulation of diabetic microvasculature |
MDPI stays neutral with regard to jurisdictional claims in published maps and institutional affiliations. |
Share and Cite
Aloke, C.; Egwu, C.O.; Aja, P.M.; Obasi, N.A.; Chukwu, J.; Akumadu, B.O.; Ogbu, P.N.; Achilonu, I. Current Advances in the Management of Diabetes Mellitus. Biomedicines 2022 , 10 , 2436. https://doi.org/10.3390/biomedicines10102436
Aloke C, Egwu CO, Aja PM, Obasi NA, Chukwu J, Akumadu BO, Ogbu PN, Achilonu I. Current Advances in the Management of Diabetes Mellitus. Biomedicines . 2022; 10(10):2436. https://doi.org/10.3390/biomedicines10102436
Aloke, Chinyere, Chinedu Ogbonnia Egwu, Patrick Maduabuchi Aja, Nwogo Ajuka Obasi, Jennifer Chukwu, Blessing Oluebube Akumadu, Patience Nkemjika Ogbu, and Ikechukwu Achilonu. 2022. "Current Advances in the Management of Diabetes Mellitus" Biomedicines 10, no. 10: 2436. https://doi.org/10.3390/biomedicines10102436
Article Metrics
Article access statistics, further information, mdpi initiatives, follow mdpi.
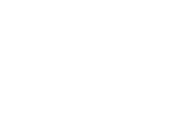
Subscribe to receive issue release notifications and newsletters from MDPI journals

An official website of the United States government
The .gov means it’s official. Federal government websites often end in .gov or .mil. Before sharing sensitive information, make sure you’re on a federal government site.
The site is secure. The https:// ensures that you are connecting to the official website and that any information you provide is encrypted and transmitted securely.
- Publications
- Account settings
- My Bibliography
- Collections
- Citation manager
Save citation to file
Email citation, add to collections.
- Create a new collection
- Add to an existing collection
Add to My Bibliography
Your saved search, create a file for external citation management software, your rss feed.
- Search in PubMed
- Search in NLM Catalog
- Add to Search
Intermittent fasting: is there a role in the treatment of diabetes? A review of the literature and guide for primary care physicians
Affiliations.
- 1 Central Michigan University College of Medicine, 1200 S. Franklin St., Mount Pleasant, MI, 48858, USA. [email protected].
- 2 , Saginaw, MI, 48602, USA. [email protected].
- 3 Central Michigan University College of Medicine, 1200 S. Franklin St., Mount Pleasant, MI, 48858, USA.
- PMID: 33531076
- PMCID: PMC7856758
- DOI: 10.1186/s40842-020-00116-1
Background: Type 2 Diabetes is a metabolic disorder characterized by hyperglycemia that causes numerous complications with significant long-term morbidity and mortality. The disorder is primarily due to insulin resistance particularly in liver, skeletal muscle, and adipose tissue. In this review, we detail the hormonal mechanisms leading to the development of diabetes and discuss whether intermittent fasting should be considered as an alternative, non-medicinal treatment option for patients with this disorder.
Methods: We searched PubMed, Ovid MEDLINE, and Google Scholar databases for review articles, clinical trials, and case series related to type 2 diabetes, insulin resistance, and intermittent fasting. Articles were carefully reviewed and included based on relevance to our topic. We excluded abstracts and any non-English articles.
Results: The majority of the available research demonstrates that intermittent fasting is effective at reducing body weight, decreasing fasting glucose, decreasing fasting insulin, reducing insulin resistance, decreasing levels of leptin, and increasing levels of adiponectin. Some studies found that patients were able to reverse their need for insulin therapy during therapeutic intermittent fasting protocols with supervision by their physician.
Conclusion: Current evidence suggests that intermittent fasting is an effective non-medicinal treatment option for type 2 diabetes. More research is needed to delineate the effects of intermittent fasting from weight loss. Physicians should consider educating themselves regarding the benefits of intermittent fasting. Diabetic patients should consult their physician prior to beginning an intermittent fasting regimen in order to allow for appropriate oversight and titration of the patients medication regimen during periods of fasting.
Keywords: Diabetes; Health; Intermittent fasting; Nutrition.
PubMed Disclaimer
Conflict of interest statement
The authors declare that they have no competing interests.
Effects of Insulin on Various…
Effects of Insulin on Various Tissues [7, 8]
Effects of Leptin [9, 10]
Effects of Adiponectin [10]
Intermittent Fasting Regimens
Example of Intermittent Fasting Prescription
Similar articles
- Multifaceted Effects of Intermittent Fasting on the Treatment and Prevention of Diabetes, Cancer, Obesity or Other Chronic Diseases. Tagde P, Tagde S, Bhattacharya T, Tagde P, Akter R, Rahman MH. Tagde P, et al. Curr Diabetes Rev. 2022;18(9):e131221198789. doi: 10.2174/1573399818666211213103315. Curr Diabetes Rev. 2022. PMID: 34961463 Review.
- Intermittent Fasting as Part of the Management for T2DM: from Animal Models to Human Clinical Studies. Muñoz-Hernández L, Márquez-López Z, Mehta R, Aguilar-Salinas CA. Muñoz-Hernández L, et al. Curr Diab Rep. 2020 Mar 12;20(4):13. doi: 10.1007/s11892-020-1295-2. Curr Diab Rep. 2020. PMID: 32166554 Review.
- Knockdown of angiopoietin-like 2 mimics the benefits of intermittent fasting on insulin responsiveness and weight loss. Martel C, Pinçon A, Bélanger AM, Luo X, Gillis MA, de Montgolfier O, Thorin-Trescases N, Thorin É. Martel C, et al. Exp Biol Med (Maywood). 2018 Jan;243(1):45-49. doi: 10.1177/1535370217745505. Epub 2017 Dec 1. Exp Biol Med (Maywood). 2018. PMID: 29192516 Free PMC article.
- Steroidal contraceptives: effect on carbohydrate metabolism in women without diabetes mellitus. Lopez LM, Grimes DA, Schulz KF. Lopez LM, et al. Cochrane Database Syst Rev. 2019 Nov 12;2019(11). doi: 10.1002/14651858.CD006133.pub5. Cochrane Database Syst Rev. 2019. PMID: 31711271
- Suggested insulin regimens for patients with type 1 diabetes mellitus who wish to fast during the month of Ramadan. Kobeissy A, Zantout MS, Azar ST. Kobeissy A, et al. Clin Ther. 2008 Aug;30(8):1408-15. doi: 10.1016/j.clinthera.2008.08.007. Clin Ther. 2008. PMID: 18803984 Review.
- The potential of the Mediterranean diet to improve metabolic control and body composition in youths with Type 1 Diabetes Mellitus. Güneş Kaya D, Arslan N, Ayyıldız F, Bayramoğlu E, Turan H, Ercan O. Güneş Kaya D, et al. BMC Endocr Disord. 2024 May 9;24(1):63. doi: 10.1186/s12902-024-01593-6. BMC Endocr Disord. 2024. PMID: 38724988 Free PMC article.
- Effect of Intermittent Fasting on Cardiometabolic Health in the Chinese Population: A Meta-Analysis of Randomized Controlled Trials. Cheung K, Chan V, Chan S, Wong MMH, Chung GK, Cheng WY, Lo K, Zeng F. Cheung K, et al. Nutrients. 2024 Jan 25;16(3):357. doi: 10.3390/nu16030357. Nutrients. 2024. PMID: 38337642 Free PMC article.
- Intermittent fasting protects β-cell identity and function in a type-2 diabetes model. Patel S, Yan Z, Remedi MS. Patel S, et al. Metabolism. 2024 Apr;153:155813. doi: 10.1016/j.metabol.2024.155813. Epub 2024 Feb 1. Metabolism. 2024. PMID: 38307325
- Intermittent Fasting: Does It Affect Sports Performance? A Systematic Review. Conde-Pipó J, Mora-Fernandez A, Martinez-Bebia M, Gimenez-Blasi N, Lopez-Moro A, Latorre JA, Almendros-Ruiz A, Requena B, Mariscal-Arcas M. Conde-Pipó J, et al. Nutrients. 2024 Jan 4;16(1):168. doi: 10.3390/nu16010168. Nutrients. 2024. PMID: 38201996 Free PMC article. Review.
- Effect of intermittent fasting on lipid biokinetics in obese and overweight patients with type 2 diabetes mellitus: prospective observational study. Mohamed YA, Abouelmagd M, Elbialy A, Elwassefy M, Kyrillos F. Mohamed YA, et al. Diabetol Metab Syndr. 2024 Jan 3;16(1):4. doi: 10.1186/s13098-023-01234-3. Diabetol Metab Syndr. 2024. PMID: 38172970 Free PMC article.
- Powers AC, Niswender KD, Evans-Molina C. Diabetes mellitus: diagnosis, classification, and pathophysiology. In: Jameson JL, Fauci AS, Kasper DL, Hauser SL, Longo DL, Loscalzo J, editors. Harrison's principles of internal medicine, 20e. New York: McGraw-Hill Education; 2018.
- National Diabetes Statistics Report . CDC.gov : U.S. Department of Health and Human Services. 2020.
- Powers AC, Stafford JM, Rickels MR. Diabetes mellitus: complications. In: Jameson JL, Fauci AS, Kasper DL, Hauser SL, Longo DL, Loscalzo J, editors. Harrison's principles of internal medicine, 20e. New York: McGraw-Hill Education; 2018.
- Davies MJ, D'Alessio DA, Fradkin J, et al. Management of Hyperglycemia in type 2 diabetes, 2018. A consensus Report by the American Diabetes Association (ADA) and the European Association for the Study of diabetes (EASD) Diabetes Care. 2018;41(12):2669–2701. doi: 10.2337/dci18-0033. - DOI - PMC - PubMed
- American Diabetes Association Standards of medical care for patients with diabetes mellitus. Diabetes Care. 2003;26(Suppl 1):S33–S50. - PubMed
Publication types
- Search in MeSH
Related information
Linkout - more resources, full text sources.
- BioMed Central
- Europe PubMed Central
- PubMed Central
Other Literature Sources
- scite Smart Citations
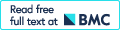
- Citation Manager
NCBI Literature Resources
MeSH PMC Bookshelf Disclaimer
The PubMed wordmark and PubMed logo are registered trademarks of the U.S. Department of Health and Human Services (HHS). Unauthorized use of these marks is strictly prohibited.
Log in using your username and password
- Search More Search for this keyword Advanced search
- Latest content
- BMJ Journals
You are here
- Volume 13, Issue 2
- Using the Translating Research into Practice framework to develop a diabetes prevention intervention in primary care: a mixed-methods study
- Article Text
- Article info
- Citation Tools
- Rapid Responses
- Article metrics

- http://orcid.org/0000-0003-4001-2869 Eva Tseng 1 , 2 ,
- Katherine Smith 3 ,
- Jeanne M Clark 1 , 2 , 4 ,
- Jodi B Segal 1 , 2 , 5 , 6 , 7 ,
- Jill A Marsteller 2 , 6 ,
- Nisa M Maruthur 1 , 2 , 4
- 1 Johns Hopkins University School of Medicine , Baltimore , Maryland , USA
- 2 Welch Center for Prevention, Epidemiology, & Clinical Research, Johns Hopkins University , Baltimore , MD , USA
- 3 Department of Health, Behavior and Society , Johns Hopkins Bloomberg School of Public Health , Baltimore , Maryland , USA
- 4 Department of Epidemiology , Johns Hopkins University Bloomberg School of Public Health , Baltimore , Maryland , USA
- 5 Johns Hopkins Bloomberg School of Public Health , Baltimore , Maryland , USA
- 6 Health Policy and Management , Johns Hopkins University Bloomberg School of Public Health , Baltimore , Maryland , USA
- 7 Center for Drug Safety and Effectiveness, Johns Hopkins University , Baltimore , MD , USA
- Correspondence to Dr Eva Tseng; etseng3{at}jhmi.edu
Background Pre-diabetes affects one-third of US adults and increases the risk of type 2 diabetes. Effective evidence-based interventions, such as the Diabetes Prevention Program, are available, but a gap remains in effectively translating and increasing uptake of these interventions into routine care.
Methods We applied the Translating Research into Practice (TRiP) framework to guide three phases of intervention design and development for diabetes prevention: (1) summarise the evidence, (2) identify local barriers to implementation and (3) measure performance. In phase 1, we conducted a retrospective cohort analysis of linked electronic health record claims data to evaluate current practices in the management of pre-diabetes. In phase 2, we conducted in-depth interviews of 16 primary care physicians, 7 payor leaders and 31 patients to elicit common barriers and facilitators for diabetes prevention. In phase 3, using findings from phases 1 and 2, we developed the core elements of the intervention and performance measures to evaluate intervention uptake.
Results In phase 1 (retrospective cohort analysis), we found few patients with pre-diabetes received diabetes prevention interventions. In phase 2 (stakeholder engagement), we identified common barriers to include a lack of knowledge about pre-diabetes among patients and about the Diabetes Prevention Program among clinicians. In phase 3 (intervention development), we developed the START Diabetes Prevention Clinical Pathway as a systematic change package to address barriers and facilitators identified in phases 1 and 2, performance measures and a toolkit of resources to support the intervention components.
Conclusions The TRiP framework supported the identification of evidence-based care practices for pre-diabetes and the development of a well-fitted, actionable intervention and implementation plan designed to increase treatment uptake for pre-diabetes in primary care settings. Our change package can be adapted and used by other health systems or clinics to target prevention of diabetes or other related chronic conditions.
- PRIMARY CARE
- DIABETES MELLITUS
- Chronic disease management
- Decision support, clinical
- Implementation science
Data availability statement
All data relevant to the study are included in the article or uploaded as supplemental information.
This is an open access article distributed in accordance with the Creative Commons Attribution Non Commercial (CC BY-NC 4.0) license, which permits others to distribute, remix, adapt, build upon this work non-commercially, and license their derivative works on different terms, provided the original work is properly cited, appropriate credit is given, any changes made indicated, and the use is non-commercial. See: http://creativecommons.org/licenses/by-nc/4.0/ .
https://doi.org/10.1136/bmjoq-2024-002752
Statistics from Altmetric.com
Request permissions.
If you wish to reuse any or all of this article please use the link below which will take you to the Copyright Clearance Center’s RightsLink service. You will be able to get a quick price and instant permission to reuse the content in many different ways.
What is already known on this topic
Although effective evidence-based interventions for pre-diabetes, such as the Diabetes Prevention Program lifestyle change programme, are available, there remains a gap in effectively translating and increasing uptake of these interventions into routine care.
What this study adds
This study describes how the Translating Research into Practice (TRiP) framework supported our mixed-methods development process to design and implement a systematic approach to diabetes prevention in primary care.
How this study might affect research, practice or policy
The TRiP framework is a suitable approach to supporting the identification of evidence-based care practices and the development of a well-fitted, actionable intervention and implementation plan. This change package can be adapted and used by other health systems or clinics to target prevention of diabetes or other related chronic conditions.
Diabetes prevention is crucial for addressing the public health burden of type 2 diabetes in the USA. Pre-diabetes affects 96 million US adults 1 and increases the risk of type 2 diabetes with a 5-year risk of up to 50%. 2 Although effective evidence-based interventions, such as the year-long intensive Diabetes Prevention Program (DPP) lifestyle change programme, are available, there remains a gap in effectively translating and increasing uptake of these interventions into routine care. 3
The DPP and metformin are effective treatments for preventing type 2 diabetes. In the DPP clinical trial, compared with the placebo group, diabetes incidence was reduced by 58% at 3 years and 27% at 15 years in the lifestyle intervention group and by 31% at 3 years and 18% at 15 years in the metformin group. 4 5 Similar results have been observed in lifestyle intervention trials globally. 6–8 Individualised medical nutrition therapy (MNT) has also been demonstrated to improve glycaemic control in people with pre-diabetes. 9 10 Based on this evidence, the American Diabetes Association recommends adults with overweight or obesity and at high risk of type 2 diabetes be referred to a DPP and/or start metformin 11 along with other guidelines also recommending individualised MNT. 9 12
Despite strong evidence that diabetes can be prevented, less than 5% of eligible persons report being referred to a DPP, 13 and the prevalence of metformin use for pre-diabetes has remained low at <5%. 14–16 There are a number of factors influencing the low uptake of pre-diabetes treatment, including low knowledge among primary care physicians (PCPs) about the DPP, the lack of availability of DPPs, and low patient awareness and understanding of the importance of pre-diabetes and its management. 17–25
The gap between known effective strategies and successful implementation in practice is explicitly addressed by the Translating Research into Practice (TRiP) framework 26 created by Johns Hopkins researchers in 2008 to guide the process of gathering what is known to be best practice and moving this evidence into day-to-day use in patient encounters. The TRiP framework grew out of real-world implementation experience 27 28 and was informed by both quality improvement science and implementation science. Although somewhat under-recognised in survey articles from the implementation science literature, 29 the TRiP framework is a viable process model that both lays solid groundwork for clinical change and offers detailed steps guiding implementation that is applicable to both inpatient and ambulatory settings.
Our objective is to describe the mixed-methods development process we undertook to design and develop a systematic intervention for diabetes prevention in primary care using the TRiP framework. 26 The three phases described here include the following: (1) summarise existing evidence, (2) identify local barriers to implementation and (3) measure performance.
We used the TRiP framework, an established implementation method for translating evidence into real-world practice ( figure 1 ). The TRiP model features four stages: (1) summarise the evidence, (2) identify local barriers to implementation, (3) measure performance and (4) ensure all patients receive the intervention by implementing the six Es (engage, educate, execute, evaluate, embed and expand). The TRiP framework was originally developed in the hospital setting, but has been successfully applied to the ambulatory setting for conditions like hypertension. 30 31
- Download figure
- Open in new tab
- Download powerpoint
Integration of Translating Evidence into Practice model and implementation framework (blue) into intervention design and development (black). EHR, electronic health record; START, Screen, Test, Act, Refer and Treat.
The TRiP framework provides actionable steps which align with the three phases of intervention design and development we describe in this paper ( figure 1 ): (1) summarising the existing evidence by conducting a retrospective cohort analysis of linked electronic health record (EHR) claims data from our health system to evaluate current practices in the management of pre-diabetes; (2) identifying local barriers to implementation through stakeholder engagement, including insurance plan leaders, physicians and patients; and (3) measuring performance. These three phases were integral to intervention design and refinement as a systematic change package. We also describe how the six Es approach was used for intervention implementation. 32 The results of the effectiveness of the intervention, as tested in the START Diabetes Prevention clinical trial ( NCT05265312 ), will be reported in a separate publication.
Phase 1: summarise existing evidence
The first stage of the TRiP framework involves summarising the evidence for interventions to improve pre-diabetes care. To gather this information, we first conducted a retrospective cohort analysis of linked EHR claims data from our health system to evaluate current practices in the management of pre-diabetes. Our objectives were to describe the order rates of pre-diabetes clinical care activities by PCPs and their completion by patients, establish patient factors associated with this care and understand the incidence of diabetes within our cohort. Using the linked EHR claims dataset, we created a cohort of patients aged 18 years and older with ≥2 outpatient primary care encounters during the 5-year window (February 2016–February 2021). Pre-diabetes was defined based on laboratory criteria using haemoglobin A1c or fasting glucose. We conducted descriptive analyses and used multiple logistic regression models for association analyses. The full details regarding inclusion/exclusion criteria, outcome definition and analysis are published elsewhere. 33
Phase 2: identify local barriers to implementation through stakeholder engagement
In accordance with the second stage of the TRiP framework, we conducted stakeholder engagement including with insurance plan leaders, physicians and patients, to identify barriers and facilitators for implementation of a systematic diabetes prevention intervention in the primary care clinic setting. From May 2020 to February 2022, we conducted remote one-on-one semistructured interviews lasting 0.5–1 hour with several stakeholders: (1) PCPs (defined as internal medicine or family medicine physicians) from primary care practices affiliated with an academic system in the mid-Atlantic region of the USA; (2) payors from regional commercial, Medicare and Medicaid plans; and (3) adult patients with pre-diabetes from the same large academic health system. The full details regarding inclusion/exclusion criteria, methods and analysis from the PCP and payor interviews are published elsewhere. 19
A common interview guide was developed by the research team with key themes for all three stakeholders: (1) barriers to diabetes prevention, (2) facilitators for diabetes prevention and (3) potential intervention components for a systematic intervention directed at diabetes prevention. Modifications were made to the guide to accommodate different foci of the three groups. All participants provided verbal informed consent and were offered a $50 gift card for their participation. Each interview was audio-recorded using Zoom or digital recorder then professionally transcribed. Two reviewers cleaned and double-coded the transcripts using the framework analytical approach. 34 We created a coding framework based on the main questions from the interview guide and later refined by the reviewers through a consensus process. Both reviewers compared their coding and differences in coding were resolved through consensus-focused discussions. All interviews were organised and analysed using MAXQDA V.2020.
Phase 3: measure performance
Intervention design and development began during phases 1 and 2 and were finalised in phase 3 where we developed performance measures to evaluate the effectiveness of the intervention. Based on the Centers for Disease Control and Prevention (CDC) guidelines for addressing pre-diabetes (Screen, Test, Refer), 35 we developed a diabetes prevention clinical pathway called START (Screen, Test, Act, Refer and Treat) ( table 1 ). To ensure successful implementation of the clinical pathway, we developed and evaluated a toolkit of resources to support the intervention components and to address findings from phases 1 and 2. During regular team meetings (ET, JMC, JAM and NMM) that occurred during 2021–2022, we discussed, developed and finalised the START Diabetes Prevention Clinical Pathway and toolkit. Then, we developed performance measures to evaluate our intervention, focusing on both process and implementation outcomes.
- View inline
Process of translating findings from phases 1 and 2 into action using the Translating Research into Practice (TRiP) framework resulting in the START Diabetes Prevention Clinical Pathway
Patient and public involvement
Patients and members of the public were not involved in the design, conduct, reporting or dissemination plans of our research.
Our retrospective cohort analysis summarised the evidence in our population concerning treatment and referral for pre-diabetes care. 33 Our cohort included 3888 patients with a laboratory diagnosis of pre-diabetes. The mean age was 63 years with the majority of patients of female sex (65%) and white (55%) or black (35%). Mean body mass index (BMI) at baseline was 30 kg/m² with nearly half of the patients having a haemoglobin A1c of 6–6.4%. Major findings and how they directly informed the overall design and development of the START Diabetes Prevention Clinical Pathway during phase 3 are elaborated in table 1 . Other detailed findings are reported elsewhere. 33
In the 12 months after cohort entry, 63% had repeat glycaemic testing ordered, but only 13% with a visit had a diagnosis of pre-diabetes coded. Only 1% of patients were referred to nutrition services and 5% were prescribed metformin. These findings highlighted the need for increased clinician education about pre-diabetes management, which led to the development of a printed clinician treatment algorithm that summarises guideline recommendations on the treatment and follow-up of pre-diabetes ( online supplemental appendix 1 ). In response to the findings, we also developed an EHR clinical decision support tool, specifically a pre-diabetes orders smartset which included specific instructions, such as criteria for the DPP and dosing instructions on metformin, orders (metformin, laboratory tests) and referrals (DPP, MNT) relevant to pre-diabetes.

Supplemental material
In our cohort, most patients (80%) had at least one visit with their PCP within 12 months of cohort entry, but only 50% of patients completed repeat glycaemic testing in that period. To address the low follow-up testing, we emphasised in the clinician treatment algorithm the importance of interval follow-up visits and testing to monitor for progression to type 2 diabetes. We also designed the pre-diabetes orders smartset to offer laboratory test orders with specified frequencies of testing (ie, 3 months, 6 months, 12 months).
We held in-depth interviews of 16 PCPs (56% female, 56% white, aged 35–67 years, in practice for 2–27 years) representing 13 community-based primary care clinics. We also interviewed seven payor leaders representing six insurance plans (commercial, Medicare and Medicaid). Finally, we interviewed 31 patients (55% female, 45% black, 35% white, aged 31–74 years). Table 1 highlights common barriers and facilitators identified during stakeholder engagement and the core intervention elements that we selected to address these barriers and facilitators. Major themes and how they directly informed the overall design and development of the START Diabetes Prevention Clinical Pathway during phase 3 are elaborated below and in table 1 . Other detailed findings are reported elsewhere. 19
A theme that emerged from the patient interviews was a lack of knowledge about pre-diabetes. While discussing their diagnosis of pre-diabetes, some patients expressed that they had not received much information about pre-diabetes, and few knew much about the DPP and/or metformin. Many participants wanted more detailed information about recommended nutrition changes to prevent diabetes and how to get medical nutrition therapy covered for patients with pre-diabetes. Therefore, we developed a print and digital patient handout with information about what is pre-diabetes, how to prevent diabetes through lifestyle change, and what the DPP and metformin are ( online supplemental appendix 2 ). We also included links to the American Diabetes Association website, which provides information on recipes and healthy food choices to prevent diabetes. Finally, we gathered information from our nutrition department to understand how MNT visits may be covered for individuals with pre-diabetes and provided it to clinicians during the training session and in the treatment algorithm ( online supplemental appendix 1 ) described in phase 3 below.
A theme arising from PCP participants was a lack of knowledge about the DPP in general, insurance coverage of the DPP and the referral process for the DPP. Based on this discussion, we included in the treatment algorithm for PCPs ( online supplemental appendix 1 ) details on the insurance coverage of and referral process for the DPP. Additionally, we developed and refined a DPP referral order in the EHR. The referral order includes instructions on who is eligible for the DPP (ie, laboratory and BMI criteria) and automatically pulls in the patient’s most recent laboratory results and BMI to facilitate clinicians in determining their patient’s eligibility. To encourage PCPs to engage with their patients in discussions about pre-diabetes and joining the DPP, we promoted an online module, developed by a clinical psychologist, that teaches clinicians how to use motivational interviewing techniques to facilitate these discussions. 36
Using our evidence summary from phase 1 to identify gaps in pre-diabetes care and stakeholder engagement about barriers and facilitators in phase 2 to identify sticking points in the implementation process, we shaped the CDC guidelines for addressing pre-diabetes 35 into an actionable clinical pathway called START Diabetes Prevention ( table 1 ). To ensure successful implementation of the clinical pathway, we developed and evaluated a toolkit of resources to support the intervention components and address learning from phases 1 and 2. Then, we developed performance measures to evaluate our intervention as described further below.
The START Diabetes Prevention Clinical Pathway steps and toolkit included:
Screen: identify patients at risk and eligible for screening for pre-diabetes/diabetes. We developed an EHR clinical decision support tool (‘Diabetes Screening Best Practice Advisory’ alert) that flags eligible patients using criteria from the 2021 US Preventive Services Task Force guidelines. 37
Test: screen and test eligible patients for pre-diabetes/diabetes. The ‘Diabetes Screening Best Practice Advisory’ prompts clinicians to order one of several laboratory test options (fasting glucose, haemoglobin A1c or point-of-care haemoglobin A1c).
Act: educate clinicians and patients on the diagnosis and prompt shared decision-making through use of a pre-diabetes decision aid. We developed a clinician treatment algorithm ( online supplemental appendix 1 ) and patient handout ( online supplemental appendix 2 ), each containing information about pre-diabetes and treatment options. Both materials included the pre-diabetes decision aid developed by O’Brien et al which was designed to facilitate discussion of treatment options for pre-diabetes, specifically usual care versus starting metformin versus participating in a DPP. 38 Additionally, we promoted an online educational module for clinicians on how to engage with patients in discussions about diabetes prevention using established motivational interviewing techniques. 36 Recognising that a single conversation may not be sufficient, especially if the patient is not ready to discuss how to manage pre-diabetes, we encouraged clinicians to have regular follow-up with their patients (see Follow-up step).
Refer/test: based on the shared decision-making conversation, clinicians may refer patients to evidence-based diabetes prevention interventions (DPP and/or MNT) and/or prescribe metformin. These orders are streamlined through the EHR orders set (‘pre-diabetes orders smartset’) we developed.
Follow-up: ensure regular follow-up to reassess treatment and evaluate progress. In the PCP treatment algorithm, we provided a suggested time frame of PCP visits every 3–6 months to continue discussing pre-diabetes, recognising that there may be insufficient time for this topic to be addressed at all visits. We also recommended clinicians recheck laboratory tests at least every 12 months consistent with guidelines, 11 and we built these standing orders into the pre-diabetes orders smartset.
We developed several performance measures to evaluate our intervention. Our primary outcome was referral to a DPP, referral to MNT and/or metformin prescription. Secondary outcomes included enrolment in a DPP, MNT visit completion, follow-up PCP visits, glycaemic laboratory testing and weight loss. Additional process and implementation outcomes are described below where we elaborate on the six Es approach from the TRiP framework 32 to ensure implementation of the intervention as follows:
Engage: we engaged clinicians and staff in our intervention clinic by describing the high prevalence of pre-diabetes and showing them evidence of current practices, including external data on screening rates 39–41 and local data from phases 1 and 2. 19 33 We provided an in-person training session that was attended by 4 out of the 16 physicians in the clinic. The training slides and recording of the training session were shared with all clinicians.
Educate: during the training session, we educated clinicians and staff of the scientific literature supporting the proposed intervention. The training slides described above reviewed the evidence behind the DPPs, MNT and metformin for preventing progression of pre-diabetes to type 2 diabetes.
Execute: we designed an implementation toolkit to address learning from phases 1 and 2 as described above. During the training session, we described to clinicians the resources in our implementation toolkit ( table 1 and online supplemental appendices 1 and 2 ).
Evaluate: we evaluated whether the intervention was successful by examining several primary and secondary outcomes collected during and after implementation of the intervention. Additionally, we collected several patient outcomes, including satisfaction with PCP discussion about pre-diabetes, confidence with and motivation for managing pre-diabetes and engagement in lifestyle change. Among patients who reported that their PCP discussed pre-diabetes with them, more patients in the intervention clinic versus control clinic felt they understood what their doctor was telling them about pre-diabetes (76% vs 62%, p=0.04) and felt their opinion was valued (78% vs 69%, p=0.13).
Embed: we elicited feedback from clinicians to ensure the intervention is sustainable. We surveyed clinicians at baseline, 6 months and 12 months after the intervention to gather data on several implementation outcomes (eg, acceptability, actionability and adoption). At 12 months, 9 out of 11 PCPs agreed that the START Diabetes Prevention Clinical Pathway was acceptable to their patients and 10 out of 11 PCPs agreed that the pathway is actionable and has encouraged them to implement changes in their practices with patients with pre-diabetes. Regarding adoption of the pathway components, all PCPs reported use of the pre-diabetes decision aid and patient education materials on pre-diabetes.
Expand: we created a plan to disseminate our intervention to the other primary care practices within our academic network while also incorporating changes based on clinician feedback.
We tested the effectiveness of the START Diabetes Prevention Clinical Pathway in a clinical trial ( NCT05265312 ) comparing an intervention clinic with a control clinic providing usual care. Full results will be reported in a separate dedicated publication.
This paper summarises the mixed-methods development process we undertook using the TRiP framework to develop and implement a systematic approach to diabetes prevention in primary care. This design enabled us to develop an intervention from the existing evidence of current practices that is tailored to the needs of the patient population being served, thus facilitating the translation of research into routine clinical care with the goal of improving treatment uptake for pre-diabetes.
Despite the large number of diabetes prevention trials demonstrating efficacy of evidence-based interventions such as the DPP for preventing diabetes, there remains a gap in effectively translating these interventions into routine care. 3 There have only been several studies examining the systematic identification and referral of patients with pre-diabetes to treatment, including uptake of the DPP in primary care settings. At Intermountain Health, quality improvement work was conducted to create a process for identifying patients with pre-diabetes using the EHR and the creation of an education and referral process to diabetes prevention interventions (2-hour introductory class called Prediabetes 101, MNT, 6-month Weigh to Health behavioural programme or online DPP). 42 Although the authors mentioned in their paper the use of plan–do–study–act cycles of the quality improvement process, it was not otherwise well described. In another study, the American Medical Association collaborated with Henry Ford Health System to pilot a pre-diabetes clinical programme designed to include clinical decision support, patient engagement and population health management tools to increase DPP referrals. 43 The authors also did not describe any systematic process for engaging stakeholders and for intervention development.
There are several advantages to using the TRiP framework for translating evidence into real-world practice. 26 First, it provides several straightforward, actionable procedures. Using this framework, we undertook three clear phases of intervention design and development. Second, the model focuses on systems and not just on individuals, which is important since clinicians work within healthcare teams. Our intervention was not focused on just changing clinician behaviours, but understanding how we can organise care through a clinical pathway supported by a toolkit of resources. Third, the model promotes a sustainable approach since it focuses on engagement of the local team in adapting the intervention to fit local work processes. We engaged input from a wide range of stakeholders relevant to diabetes prevention to guide intervention design and development to ensure acceptability, appropriateness and feasibility of our intervention.
Challenges with using the TRiP framework, which also apply to other implementation science models, include the time and resources required to develop, implement and evaluate programmes. The time needed to design and develop an effective intervention must be balanced by the fact that clinical changes are occurring simultaneously in the health system that may affect the intervention or outcomes. As we started this study, there was a new health system initiative focused on diabetes prevention that ultimately impacted some of the intervention components. For example, one area of focus of this health system initiative was on clinician education about pre-diabetes and a push to increase the referral of patients to the DPP, a primary outcome of the aforementioned clinical trial testing the START Diabetes Prevention Clinical Pathway. Nonetheless, the intervention arising from this work addresses many of the barriers that have arisen with the health system initiative. Future consideration might be a hybrid effectiveness–implementation design 44 that would allow timely assessment of both effectiveness and implementation outcomes for this type of work.
Limitations to our approach include the potential lack of generalisability of our findings from phase 1 (summarising existing evidence) and 2 (identify local barriers to implementation through stakeholder engagement). The intervention developed over the course of the three phases may not be applicable to all clinical settings, particularly ones caring for populations experiencing a large burden of adverse social determinants of health. We were fortunate to be able to widely offer the DPP to all our patients due to grant funding supporting the DPP, which may not be possible in many settings. In general, the DPP is covered by Medicare, Medicaid in certain states and some commercial plans.
We anticipate our future research will include adaptation and dissemination of our approach to populations where there may be barriers to transportation, healthy food, accessing regular medical care and engaging in healthy behaviours. Our multistakeholder engagement process will be particularly important to determine how to adapt our diabetes prevention intervention to address common barriers and social determinants of health. To that end, we may need to involve and use other clinical staff members in our intervention recognising that PCPs have many issues to address in a single visit. During the design and development of the START Diabetes Prevention Clinical Pathway, we engaged stakeholders about using other clinical staff members besides PCPs to deliver the intervention, but we did not ultimately include them because of significant short-staffing of nurses and medical assistants in the clinic as a result of the COVID-19 pandemic. We recognise that other diabetes prevention interventions have focused on the use of pharmacists 45 or medical assistants 25 to lead shared decision-making treatment discussions with primary care patients, and this could be incorporated in the future if clinic staffing is sufficient.
Conclusions
The TRiP framework provided a suitable approach to support the identification of evidence-based care practices for pre-diabetes and the development of a well-fitted, actionable intervention and implementation plan designed to increase treatment uptake for pre-diabetes in primary care. This approach addresses the current gap in effectively translating diabetes prevention interventions into routine care, and our change package can be adapted and used by other health systems or clinics to target prevention of diabetes or other related chronic conditions.
Ethics statements
Patient consent for publication.
Not applicable.
Ethics approval
This study involves human participants and was approved by the Johns Hopkins School of Medicine Institutional Review Board (IRB). Each phase had a separate IRB review (phase 1: IRB00203278; phase 2: IRB00232415; phase 3: IRB00313490). Participants gave informed consent to participate in the study before taking part.
- Sebrié EM ,
- Williamson DF , et al
- Patorno E ,
- Karter AJ , et al
- Diabetes Prevention Program Research Group
- Knowler WC ,
- Barrett-Connor E ,
- Fowler SE , et al
- Hu YH , et al
- Tuomilehto J ,
- Lindström J ,
- Eriksson JG , et al
- Ramachandran A ,
- Snehalatha C ,
- Mary S , et al
- Dennison M ,
- Gardner CD , et al
- Raynor HA ,
- Davidson PG ,
- Burns H , et al
- ElSayed NA ,
- Bannuru RR , et al
- Briggs Early K ,
- Venkataramani M ,
- Pollack CE ,
- Yeh H-C , et al
- Maruthur NM
- Grams ME , et al
- Duru OK , et al
- O’Rourke P , et al
- Marsteller JA , et al
- Mainous AG ,
- Tanner RJ ,
- Scuderi CB , et al
- Thomas AR ,
- Hieronymus L , et al
- Skoglund G ,
- Nilsson BB ,
- Olsen CF , et al
- O’Brien MJ ,
- Tang JW , et al
- Vargas MC ,
- Lopez A , et al
- Pronovost PJ ,
- Berenholtz SM ,
- Caffrey J ,
- Krout K , et al
- Pronovost P ,
- Needham D ,
- Berenholtz S , et al
- Boonyasai RT ,
- Rakotz MK ,
- Lubomski LH , et al
- Murakami L ,
- Daniel D , et al
- Marsteller JA ,
- Gurses AP ,
- Ozok AA , et al
- Clark JM , et al
- Srivastava A ,
- Centers for Disease Control and Prevention
- Johns Hopkins Medicine
- Davidson KW ,
- Barry MJ , US Preventive Services Task Force
- Cameron KA ,
- Vargas MC , et al
- Shealy KM ,
- Waites J , et al
- Brunisholz KD ,
- Conroy MB ,
- Belnap T , et al
- Lingvay I , et al
- Hashibe M , et al
- Aquino G , et al
- Curran GM ,
- Mittman B , et al
- Turk N , et al
Supplementary materials
Supplementary data.
This web only file has been produced by the BMJ Publishing Group from an electronic file supplied by the author(s) and has not been edited for content.
- Data supplement 1
- Data supplement 2
Contributors ET—study concept and design; oversight of recruitment and data collection; data analysis; manuscript writing; guarantor. KS, JMC, JAM and NMM—study concept and design, advise and review of data analysis, manuscript writing. All authors have commented and approved the manuscript.
Funding ET is supported by the National Institute of Diabetes and Digestive and Kidney Diseases (K23DK118205).
Competing interests None declared.
Patient and public involvement Patients and/or the public were not involved in the design, or conduct, or reporting, or dissemination plans of this research.
Provenance and peer review Not commissioned; externally peer reviewed.
Supplemental material This content has been supplied by the author(s). It has not been vetted by BMJ Publishing Group Limited (BMJ) and may not have been peer-reviewed. Any opinions or recommendations discussed are solely those of the author(s) and are not endorsed by BMJ. BMJ disclaims all liability and responsibility arising from any reliance placed on the content. Where the content includes any translated material, BMJ does not warrant the accuracy and reliability of the translations (including but not limited to local regulations, clinical guidelines, terminology, drug names and drug dosages), and is not responsible for any error and/or omissions arising from translation and adaptation or otherwise.
Read the full text or download the PDF:
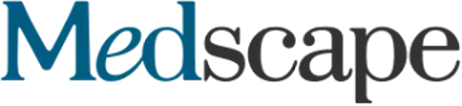
- Allergy & Immunology
- Anesthesiology
- Critical Care
- Dermatology
- Diabetes & Endocrinology
- Emergency Medicine
- Family Medicine
- Gastroenterology
- General Surgery
- Hematology - Oncology
- Hospital Medicine
- Infectious Diseases
- Internal Medicine
- Multispecialty
- Ob/Gyn & Women's Health
- Ophthalmology
- Orthopedics
- Pathology & Lab Medicine
- Plastic Surgery
- Public Health
- Pulmonary Medicine
- Rheumatology
- Transplantation
- Today on Medscape
- Business of Medicine
- Medical Lifestyle
- Science & Technology
- Medical Students
- Pharmacists
Inhaled Insulin Helps Patients Overcome Fear of Needles
Akshay B. Jain, MD; James W. Kim, MBBCh, PgDip, MScCH
Authors and Disclosures
Disclosure: Akshay B. Jain, MD, has disclosed the following relevant financial relationships: Serve(d) as a speaker or a member of a speakers bureau for: Abbott; Acerus; AstraZeneca; Amgen; Bausch Healthcare; Bayer; Boehringer Ingelheim; Care to Know; CCRN; Connected in Motion; CPD Network; Dexcom; Diabetes Canda; Eli Lilly; GSK; HLS Therapeutics; Janssen; Master Clinician Alliance; MDBriefcase; Merck; Medtronic; Moderna; Novartis; Novo Nordisk; Partners in Progressive Medical Education; Pfizer; Sanofi Aventis; Timed Right; WebMD Received research grants/research support from: Abbott; Amgen; Novo Nordisk Received consulting fees from: Abbott; Acerus; AstraZeneca; Amgen; Bausch Healthcare; Bayer; Boehringer Ingelheim; Dexcom; Eli Lilly; Gilead Sciences; GSK; HLS Therapeutics; Insulet; Janssen; Medtronic; Novo Nordisk; Partners in Progressive Medical Education; PocketPills; Roche; Sanofi Aventis; Takeda
Disclosure: James W. Kim, MBBCh, PgDip, MScCH, has disclosed the following relevant financial relationships: Serve(d) as a director, officer, partner, employee, advisor, consultant, or trustee for: Abbott; AbbVie; AstraZeneca; Bayer; Boehringer Ingelheim; Eisai; Embecta; Eli Lilly; GSK; Janssen; Novo Nordisk; Miravo; Teva; Takeda; Sanofi Serve(d) as a speaker or a member of a speakers bureau for: AbbVie; AstraZeneca; Boehringer Ingelheim; Eisai; Embecta; Eli Lilly; GSK; Janssen; Novo Nordisk; Miravo; Pfizer Takeda; Sanofi; Otsuka Received research grant from: Novo Nordisk Received income in an amount equal to or greater than $250 from: AbbVie; AstraZeneca; Boehringer Ingelheim; Eisai; Embecta; Eli Lilly; GSK; Janssen; Novo Nordisk; Miravo; Linpharma; Pfizer Takeda; Sanofi; Otsuka Serve(d) as Co-director: Partners in Progressive Medical Education
This transcript has been edited for clarity.
Akshay B. Jain, MD: Welcome back to Medscape, from ADA 2024. I'm Dr Akshay Jain, an endocrinologist from Vancouver, and I'm joined by Dr James Kim, a primary care physician from Calgary, Canada.
Both Dr Kim and I attended ADA 2024. We went over all our learnings and decided that there was a whole heap of clinical pearls that we learned from the conference. We thought it would be awesome if we could share our learnings with all of you, both from a primary care lens and from an endocrinology perspective.
One study Dr Kim and I learned about, and we think has some definite nuances in management of people living with diabetes, regards inhaled insulin . When we have patients in our clinic who have perhaps failed multiple oral agents or have very high blood sugars, we obviously want to consider starting them on insulin for type 2 diabetes .
Sometimes there is a significant barrier, which is related to the needles. There's an actual term for this: trypanophobia — a fear of needles. For the longest time, people have not wanted to take insulin or injectables because there's only one way of administering it, which is subcutaneous.
Enter now inhaled insulin. We saw studies at the ADA 2024 conference that looked at a new inhaled insulin called Afrezza . Afrezza essentially is a short-acting insulin, so it's kind of like a prandial insulin derivative, where it can be inhaled by an individual and it will work for mealtime control of blood sugars.
Dr Kim, in your practice, how often do you see people not wanting to take shots, and has this been a big barrier for you in starting insulin?
James W. Kim, MBBCh, PgDip, MScCH: Thank you for having me. To answer your question, absolutely I encounter this on a weekly basis — and I'm not even an endocrinologist. I just have an interest in diabetes. There are a number of patients that I think will benefit massively with insulin but they're needle-phobic. You taught me that word, but I can never pronounce it, so my apologies for not remembering that phobia . I'm just going to call it needle phobia because I'm a simple-minded person.
The needle phobia is massive. I think there's a definite fear of the needle, but there's also a fear of failure. As soon as an injection is mentioned, many patients feel they failed miserably. There's an emotional roller coaster that happens.
I'm sure, Dr Jain, you have seen many patients, especially from Asia, who would say, "Oh, my auntie got on insulin and 3 months later, she got a kidney transplant." "My uncle started on insulin and he unfortunately passed away a couple of months later." Unfortunately, they're blaming many of those things on insulin.
I also have a number of patients who said they were on insulin before many years ago, and they experienced some severe hypoglycemic events, and they don't want to get on the insulin ever again. This is unfortunate because you know that if those patients, those aunties and uncles, were on insulin long before, maybe we could have saved their legs and kidneys, and potentially death.
Now we have advanced so much with insulin that hypoglycemia does occur, but much less than before. We still have many barriers when it comes to insulin initiations. Therefore, having this idea of inhaled insulin is fantastic, and I think we can get many more patients on insulin — the medication they actually need.
Jain: Absolutely. From the studies on inhaled insulin at ADA 2024, the key thing I found very interesting, regarding the pharmacokinetics of the insulin, was that it's working very quickly. It starts working within minutes of administering it.
Additionally, it lasts in the body only for a shorter duration of time compared with other injectable short-acting insulins, so it lasts in the body. The active insulin time is roughly about 2 hours or so, based on the studies, which in my mind opens up a whole world of possibilities because it means that people can take another correctional insulin if the blood sugars are still high after taking their first inhaled dose. You can take another dose subsequently without worrying about stacking of insulin.
Many of us are familiar with this term, which is if you take two shots of short-acting insulin too close to each other, the insulin doses might add up and there can be a big drop in the blood sugars; it's called stacking of insulin. This can be potentially avoided.
Similarly, if you take your dinnertime inhaled insulin and the sugars are still high around bedtime, you could take a smaller dose of the inhaled insulin and not worry about middle-of-the-night hypoglycemia because the effect of the insulin would be only for a little while.
That's one key learning that I found very helpful. The other important thing that I found was that this is not for everyone, so there are some restrictions. Essentially, the contraindication is that people who have asthma or COPD cannot be prescribed an inhaled insulin.
What are your thoughts, Dr Kim, based on this for your practice in primary care?
Kim: It is very fascinating, for sure. I cannot wait to get hold of this insulin. I can already think of some patients who may benefit. You've mentioned the asthma and COPD patients, and that makes more sense because there is an actual airway problem.
I also wonder what will happen to patients who have restrictive airway disease, where asthma and COPD fall under obstructive airway disease. What if they have obesity , where it's really pressing into the diaphragm, and where they may not be able to take the deep breath in? How will they react?
What about someone who's got a cold, someone who has postnasal drip, or someone who tends to cough frequently? What about egg allergies? There are many question marks around this insulin before initiating these medications. There is excitement, but there are also many questions at the same time.
Jain: I think these are very important, practical considerations that we'll uncover as we start using more of this in clinical practice. The other important thing to note is that the presenters told us it's important to monitor pulmonary function tests. It's important to get a baseline pulmonary function test, and then we have to do another one in 6 months, followed by annually thereafter.
If, at any point of time, the FEV 1 drops by 20% or more, then that would be an indication for discontinuation of the inhaled insulin. The pulmonary function test does not need to be one of those fancier ones. The study group would just do office spirometries. I'm wondering, Dr Kim, in primary care, do you think this could potentially be a rate-limiting factor?
Kim: In Alberta, where I reside, no. Spirometry is very easily accessible in the province. For example, in Calgary alone, we have a population of about 1.3 million people. We have over 13 or 15 companies that can do this spirometry. We can get these things done literally within a week or two.
However, I am aware that in other provinces in Canada, it can definitely be a huge rate-limiting factor. Not everyone has the office-based spirometry, and definitely not within the primary care office. It has to be referred out to these private companies, most likely, and some of the rural areas will have to rely on the provincial hospitals, where the access can be even more challenging.
On the day of the actual spirometry, if the person has a cough or is not feeling well, it's going to be a problem because you don't want the spirometry to be infected with a whole bunch of viruses. You'll have to cancel that and it can be a bit of an issue.
Jain: Many of our viewers are from the United States and other parts of the world, and spirometry is quite easily accessible in most places. As an endocrinologist, I must confess that it's been a long time since I've even ordered a spirometry or any clinical form of pulmonary function test. Once I start using the inhaled insulin, I'll need to start brushing up on my pulmonary function test knowledge.
I think these are exciting times. At least we've got something to offer to people who would have otherwise not taken any insulin at all. There's certainly that hope that now there's a different way to administer this, and hopefully it can only get better from here on.
Thanks for joining us again, Dr Kim. This is Dr Akshay Jain with Medscape, from ADA 2024. Don't forget to check out our other videos on Medscape for more coverage of the conference.
TOP PICKS FOR YOU
- Perspective
- Drugs & Diseases
- Global Coverage
- Additional Resources
- Biosimilars May Finally Stop the Rocketing Cost of Insulin
- Reducing Albumin Improves Kidney and Heart Function in People With Type 2 Diabetes
- Atherosclerosis and Diabetes Synergistic, Deadlier in South Asians
- Diseases & Conditions Type 2 Diabetes Mellitus
- Diseases & Conditions Pediatric Type 2 Diabetes Mellitus
- Diseases & Conditions Type 2 Diabetes Mellitus and TCF7L2
- Drugs exenatide injectable suspension
- Type 2 Diabetes Mellitus
- Pediatric Type 2 Diabetes Mellitus
- Type 2 Diabetes Mellitus and TCF7L2
- Pediatric Type 1 Diabetes Mellitus
- Fast Five Quiz: Atrial Fibrillation and Diabetes
- Fast Five Quiz: How Much Do You Know About Diabetic Neuropathy?
- Bedridden Breakdown: Classifying Pressure Injuries
- Diabetes Mellitus Type 2 News & Perspectives
- What's the Goal in Treating Type 2 Diabetes?
- Denosumab for Osteoporosis May Prevent Type 2 Diabetes
- - Google Chrome
Intended for healthcare professionals
- My email alerts
- BMA member login
- Username * Password * Forgot your log in details? Need to activate BMA Member Log In Log in via OpenAthens Log in via your institution

Search form
- Advanced search
- Search responses
- Search blogs
- Risk of dementia after...
Risk of dementia after initiation of sodium-glucose cotransporter-2 inhibitors versus dipeptidyl peptidase-4 inhibitors in adults aged 40-69 years with type 2 diabetes: population based cohort study
Linked editorial.
SGLT-2 inhibitors and dementia
- Related content
- Peer review
- Anna Shin , senior researcher 1 ,
- Bo Kyung Koo , professor 2 ,
- Jun Young Lee , professor 3 ,
- 1 Medical Research Collaborating Centre, Department of Internal Medicine, Seoul National University Bundang Hospital, Seongnam, Korea
- 2 Division of Endocrinology, Department of Internal Medicine, Seoul National University College of Medicine, SMG-SNU Boramae Medical Centre, Seoul, Korea
- 3 Department of Psychiatrics, Seoul National University College of Medicine, SMG-SNU Boramae Medical Centre, Seoul, Korea
- 4 Department of Internal Medicine, Seoul National University College of Medicine, Seoul National University Bundang Hospital, Seongnam, Korea
- Correspondence to: E H Kang kangeh{at}snubh.org
- Accepted 4 July 2024
Objective To compare the risk of dementia associated with sodium-glucose cotransporter-2 (SGLT-2) inhibitors versus dipeptidyl peptidase-4 (DPP-4) inhibitors in adults aged 40-69 years with type 2 diabetes.
Design Population based cohort study.
Setting Korean National Health Insurance Service data, 2013-21.
Participants 110 885 propensity score matched pairs of adults with type 2 diabetes aged 40-69 years who were initiators of either an SGLT-2 inhibitor or a DPP-4 inhibitor.
Main outcome measures The primary outcome was new onset dementia. Secondary outcomes were dementia requiring drug treatment and individual types of dementia, including Alzheimer’s disease and vascular dementia. Control outcomes were genital infections (positive), and osteoarthritis related clinical encounters and cataract surgery (negative). Hazard ratios and 95% confidence intervals (CIs) were estimated using Cox models. Follow-up time stratified analyses (>2 years and ≤2 years) and subgroup analyses by age, sex, concomitant use of metformin, and baseline cardiovascular risk were performed.
Results 110 885 propensity score matched pairs of initiators of an SGLT-2 inhibitor or a DPP-4 inhibitor were followed-up for a mean 670 (standard deviation 650) days, generating 1172 people with newly diagnosed dementia: incidence rate 0.22 per 100 person years in initiators of SGLT-2 inhibitors and 0.35 per 100 person years in initiators of DPP-4 inhibitors, with hazard ratios of 0.65 (95% CI 0.58 to 0.73) for dementia, 0.54 (0.46 to 0.63) for dementia requiring drugs, 0.61 (0.53 to 0.69) for Alzheimer’s disease, and 0.48 (0.33 to 0.70) for vascular dementia. The hazard ratios for the control outcomes were 2.67 (2.57 to 2.77) for genital infections, 0.97 (0.95 to 0.98) for osteoarthritis related encounters, and 0.92 (0.89 to 0.96) for cataract surgery. When calibrated for residual confounding measured by cataract surgery, the hazard ratio for dementia was 0.70 (0.62 to 0.80). The association was greater for more than two years of treatment (hazard ratio of dementia 0.57, 95% CI 0.46 to 0.70) than for two years or less (0.52, 0.41 to 0.66) and persisted across subgroups.
Conclusion SGLT-2 inhibitors might prevent dementia, providing greater benefits with longer treatment. As this study was observational and therefore prone to residual confounding and informative censoring, the effect size could have been overestimated. Randomised controlled trials are needed to confirm these findings.
Introduction
Dementia concerns damage to the brain parenchyma, resulting in a permanent degradation of higher cortical functions, mood, and even behaviour. 1 According to a World Health Organization (WHO) report in 2021, the number of people with dementia globally is expected to reach 78 million by 2030. 2 Despite the severe consequences of dementia, the success rate of the development for dementia drugs has been markedly low in the past two decades, leaving only extremely limited options for disease modifying treatment. 3 Evidence has, however, emerged to support the importance of modifiable risk factors for dementia, including diabetes. 4 According to a pooled analysis, type 2 diabetes is associated with a 60% greater risk of dementia, 5 predisposing such people to both Alzheimer’s disease and vascular dementia. 6 The mechanisms linking type 2 diabetes and dementia are multifactorial, involving insulin resistance, hypoglycaemic episodes, and vascular compromise. 7 In line with this, meta-analyses on observational studies have shown that certain antiglycaemic drugs may have neuroprotective effects in people with diabetes. 8 9 10
Sodium-glucose cotransporter-2 (SGLT-2) inhibitors are a newer class of antiglycaemic drugs that inhibit reabsorption of glucose in the proximal tubule. Key randomised controlled trials have shown significant cardiorenal protection from use of SGLT-2 inhibitors beyond glucose lowering effects. 11 SGLT-2 inhibitors are now considered one of the drug repurposing candidates for disease modifying treatment of dementia. 12 Recent evidence suggests neuroprotective effects of SGLT-2 inhibitors based on penetration of the drug through the blood-brain barrier, SGLT-2 expression in brain tissue, and direct inhibition of acetylcholinesterase, as well as indirect cardiometabolic benefits. 13
Previous observational studies have suggested better preservation of cognitive function among people with type 2 diabetes treated with SGLT-2 inhibitors than other treatments, including dipeptidyl peptidase-4 (DPP-4) inhibitors, 14 15 16 17 another newer class of antiglycaemic drugs found to have no effect on cognitive performance in recent randomised controlled trials compared with sulfonylurea and placebo. 18 19 The methodological approaches of these observational studies were often limited, however, and did not meet the active comparator new user design, leaving concerns about confounding or bias. 15 16 A recent well designed study on residents in Ontario, Canada compared new users of SGLT-2 inhibitors with new users of DPP-4 inhibitors and found that the former were associated with a 20-34% reduced risk of dementia among people older than 66 years. 14 The effects on younger populations and specific types of dementia (eg, Alzheimer’s disease, vascular dementia) were not, however, examined. Moreover, it is unclear whether different patient characteristics such as concomitant treatment or comorbidity status would modify such drug effects. We therefore compared the risk of dementia among adults with diabetes younger than 70 years who initiated an SGLT-2 inhibitor or DPP-4 inhibitor using the nationally representative Korea National Health Insurance Service database.
Data source
We conducted a cohort study using data from the Korea National Health Insurance Service database during 2013-21. This database covers the entire population of Korea and provides longitudinal patient data, including personal characteristics, ICD-10 (international classification of diseases, 10th revision) diagnosis codes, procedures, prescription and dispensing records (drug names, prescription and dispensing dates, days’ supply, dose, and route of administration), and type of healthcare utilisation (outpatient, inpatient, or emergency department). 20
Study design and population
We emulated a target trial for the outcomes of interest (see supplemental table S1 for the framework of the target trial emulation) using a propensity score matched active comparator new user cohort study design (see supplemental figure S1 for the detailed study design).
Adults aged 40-69 years with an ICD-10 code for type 2 diabetes who had initiated an SGLT-2 inhibitor or DPP-4 inhibitor were eligible for inclusion in the study (see supplemental figure S2 for the participant selection process and supplemental table S2 for ICD-10 codes used in this selection process). To implement a new user active comparator design, we only included initiators of the two competitive study drugs, an SGLT-2 inhibitor and a DPP-4 inhibitor, who had not been dispensed either drug for at least 365 days (the baseline period) before the first dispensing date of the study drug (the index date). To be included, individuals were required to be free of any dementia and related drugs ever before the index date. We also excluded those with ICD-10 diagnosis codes for type 1 diabetes mellitus, HIV, or end stage renal disease (or dialysis service) during the baseline period, and those who concomitantly used glucagon-like peptide-1 receptor agonists or thiazolidinedione on the index date.
Outcome measurement
Our primary outcome was incident dementia based on ICD-10 diagnosis codes in a primary position recorded on inpatient or outpatient claims (see supplemental table S3 for ICD-10 codes used to define outcomes). 21 To improve specificity of outcome ascertainment, we examined dementia defined by the diagnosis codes along with dispensing of dementia drugs (donepezil, rivastigmine, galantamine, or memantine) as a secondary outcome. In Korea, dementia drugs are reimbursed by the Rare and Intractable Diseases programme, where beneficiaries should qualify for a diagnosis certificate of dementia based on brain imaging and cognitive function testing. Other secondary outcomes were individual types of dementia (eg, Alzheimer’s disease, vascular dementia) in a primary position.
Control outcomes
To assess reproducibility of established relations and unmeasured systematic bias, we also compared the risk of positive and negative control outcomes between the two treatment groups (see supplemental table S3). Given the higher risk of genital infections associated with SGLT-2 inhibitors compared with DPP-4 inhibitors in randomised controlled trials, we examined genital infections as a positive control outcome. 22 We also examined osteoarthritis related encounters and cataract surgery as negative control outcomes. A null association with treatment is expected for appropriate negative control outcomes, which share unmeasured confounders with the outcome and are unaffected by treatment. 23 As with dementia, osteoarthritis and cataract are degenerative diseases of older people. Therefore, osteoarthritis related encounters and cataract surgery would share with dementia unmeasured confounders such as frailty, lifestyle, and healthcare system usage patterns associated with ageing, and cataract surgery would also share smoking and alcohol consumption. 24 25 Osteoarthritis related encounters would be expected for symptomatic or advanced osteoarthritis. Thus we considered such encounters to be minimally affected by the study drugs despite mild weight reduction effect of SGLT-2 inhibitors. 11 Also, two meta-analyses reported a null association between the development of cataract and treatment with SGLT-2 inhibitors. 26 27 Using a deviation from the null association between a negative control outcome and treatment, we estimated corrected hazard ratios and corresponding 95% confidence intervals (CIs) adjusting for residual confounding. 23 28
We identified covariates related to diabetes severity and risk of dementia for the 365 day pre-index baseline period (see supplemental table S2 for ICD-10 codes used to ascertain covariates). The covariates included personal characteristics, sociodemographic factors, complications from diabetes (retinopathy, nephropathy, neuropathy, and diabetic foot), classes and number of antiglycaemic drugs, risk factors for dementia (ie, cardiometabolic risk factors, hearing loss, head trauma, fracture history, mood or mental disorders, and anticholinergic drugs), other comorbidities and related drugs, Charlson-Deyo comorbidity index, 29 and healthcare service use patterns such as hospital admissions, emergency department visits, and outpatient clinic visits.
Statistical analysis
We used propensity score matching to account for confounding. The propensity score was estimated for each comparison using a multivariable logistic regression model that included >110 baseline covariates (see supplemental table S4 for the full list). Nearest neighbour matching for SGLT-2 inhibitor versus DPP-4 inhibitor was done in a ratio of 1:1, with a caliper of 0.025 on the propensity score scale. Balance between covariates after propensity score matching was considered to have been achieved when the absolute standardised difference was <0.1 between the two treatment groups. 30 Propensity score matched incidence rates of primary and secondary outcomes were calculated per 100 person years.
We primarily used Cox proportional hazard models to estimate the hazard ratios and corresponding 95% CIs. Owing to the discrete difference in mortality between the two treatments, 11 we also presented hazard ratios (95% CIs) from Fine-Gray models, adjusting for competing risk of death. 31 The proportional hazard assumption was tested by adding the interaction term between treatment and follow-up time in the model. When the interaction was statistically significant, we performed a follow-up time stratified analysis to examine the time varying treatment effect. We sorted propensity score matched study participants into two groups according to their follow-up times (≤2 years or >2 years), then estimated a matched set stratified hazard ratio (95% CI) within the two groups.
In our primary as treated analysis, patients were followed from the day after the index date up to the first occurrence of the censoring events (outcome event, disenrollment, death, end of database (31 December 2021), or treatment change through discontinuation, switching, or adding). Drug discontinuation was defined as no dispensing within 90 days from the expected refill date. The expected refill date was calculated by adding days’ supply to the last dispensing date of the study drug. Participants who discontinued the study drug were followed up until the last expected refill date plus a 30 day grace period. Although switching between different SGLT-2 inhibitors or between different DPP-4 inhibitors was not a censoring event, adding or switching to other classes of antiglycaemic treatments resulted in immediate censoring. We performed an intention-to-treat analysis as our secondary analysis, where participants were followed up until censoring events except for treatment change to deal with concerns of informative censoring.
Sensitivity analyses —Firstly, to avoid reverse causation from delayed diagnosis of dementia, we started follow-up after 365 days from the index date in both as treated and intention-to-treat analyses (up to three years and the whole follow-up). Secondly, we applied a grace period of 180 or 365 days for the censoring by treatment change to capture delayed diagnoses made after the change of treatment. Thirdly, to eliminate the effect of hypoglycaemic episodes during treatment, analyses were done excluding those who concurrently used drugs with hypoglycaemia potential (insulin, sulfonylurea, or glinides) on the index date. Fourthly, we adjusted for the duration of diabetes mellitus for those who had an ascertainable type 2 diabetes diagnosis date, defined as the first date of an ICD-10 code for type 2 diabetes diagnosis in the primary position free of such codes for at least 365 days before the diagnosis date. Lastly, we utilised the entirety of new users of SGLT-2 inhibitors and DPP-4 inhibitors using propensity score based fine stratification and weighting to achieve greater generalisability. 32
Subgroup analyses —Prespecified propensity score matched subgroup analyses were done based on participants’ age (≥60 years and <60 years), sex, concurrent metformin use, and baseline cardiovascular risk. The estimation of propensity score and matching were done separately for individual subgroups. The subgroup with high cardiovascular risk was defined as men aged ≥50 years and women aged ≥55 years who had at least one diagnosis of angina, myocardial infarction, stroke, or peripheral vascular disease during the one year pre-index period. 20 We tested interaction terms between the treatment and individual stratifying factors.
Patient and public involvement
This study analysed secondary data without patient involvement. Patients were not invited to be involved in the study design, development of outcomes, interpretation of the results, or drafting of the manuscript. The primary barrier against patient and public involvement was use of an administrative database, which requires a specific study design and pharmacoepidemiological method to ensure internal validity, leaving minimal potential for the patient and public to be engaged.
Baseline patient characteristics
Supplemental figure S2 shows the selection process of the study cohort. We identified 112 663 new users of SGLT-2 inhibitors and 847 999 new users of DPP-4 inhibitors who were free of known dementia and did not use either of the study drugs at baseline. Before propensity score matching, most baseline covariates, including diabetes complications and number of antiglycaemic drugs, were overall relatively well balanced, reflecting the effectiveness of the active comparator new user design ( table 1 , also see supplemental table S4 for the distribution of the full list of covariates between the two groups). Some covariates showed imbalance, with standardised differences >0.1, particularly cardiovascular comorbidities, which were more prevalent among initiators of SGLT-2 inhibitors than among initiators of DPP-4 inhibitors (16.8% v 10.6% for angina pectoris, 3.1% v 1.6% for myocardial infarction, 7.8% v 4.2% for heart failure, 66.6% v 59.8% for hypertension, 78.8% v 70.9% for hyperlipidaemia). After propensity score matching in a 1:1 ratio, 110 885 pairs of initiators of SGLT-2 inhibitors and DPP-4 inhibitors were included in the analysis (mean age 61.9 years, 55.7% men) ( table 1 , also see supplemental table S4). All propensity score matched baseline covariates, including psychiatric disorders, cardiovascular diseases, other comorbidities, use of drugs with anticholinergic activity, and use of other drugs, were well balanced (standardised differences <0.1). The study participants’ mean comorbidity score was 2.4 (standard deviation (SD) 1.8). Cardiometabolic factors were highly common, with 66.5% of participants having hypertension and 78.6% having hyperlipidaemia. Established cardiovascular diseases were observed in 16.7% of participants with angina, 6.4% with stroke, and 3.1% with myocardial infarction. The most common oral antiglycaemic agents used during the baseline period were biguanide (52.2%), followed by sulfonylurea (27.8%) and thiazolidinedione (8.2%). The most common index SGLT-2 inhibitor was dapagliflozin (58.6%), followed by empagliflozin (35.4%), and the most common index DPP-4 inhibitors were gemigliptin (22.7%), linagliptin (22.4%), and sitagliptin (20.4%) (see supplemental table S5).
Select baseline characteristics of propensity score matched cohort. Values are number (percentage) unless stated otherwise
- View inline
Comparative risk of dementia between initiators of SGLT-2 inhibitors and DPP-4 inhibitors
The mean follow-up time of patients was 670 (SD 650) days, with 612 (SD 613) days for initiators of SGLT-2 inhibitors and 728 (SD 679) days for initiators of DPP-4 inhibitors (see supplemental table S6 for distribution of censoring events). A total of 1172 participants with newly diagnosed dementia were identified, with incidence rates per 100 person years of 0.22 for initiators of SGLT-2 inhibitors and 0.35 for initiators of DPP-4 inhibitors. The corresponding hazard ratio was 0.65 (95% CI 0.58 to 0.73; table 2 ). The lowered risk of dementia associated with use of SGLT-2 inhibitors compared with DPP-4 inhibitors was similarly observed for secondary outcomes: hazard ratio 0.54 (0.46 to 0.63) for dementia requiring drugs, 0.61 (0.53 to 0.69) for Alzheimer’s disease, and 0.48 (0.33 to 0.70) for vascular dementia. The results were consistent with those of intention-to-treat analyses: 0.65 (0.60 to 0.71) for dementia, 0.60 (0.54 to 0.67) for dementia requiring drugs, 0.63 (0.57 to 0.69) for Alzheimer’s disease, and 0.62 (0.49 to 0.79) for vascular dementia. Estimates for the Fine-Gray models were also similar. We found a 2.67-fold risk (95% CI 2.57-fold to 2.77-fold) of genital infections associated with SGLT-2 inhibitors versus DPP-4 inhibitors. The hazard ratios for association between treatment and negative control outcomes were 0.97 (95% CI 0.95 to 0.98) for osteoarthritis related encounters and 0.92 (0.89 to 0.96) for cataract surgery. When corrected using the association between treatment and cataract surgery, the hazard ratios for dementia increased by about 7.7% (see supplemental table S7), to 0.70 (0.62 to 0.80).
Comparative risk of dementia between initiators of SGLT-2 inhibitors and DPP-4 inhibitors in main propensity score matched cohort
Follow-up time stratified analysis
A significant interaction (P<0.05) was observed between treatment and follow-up time for all outcomes except vascular dementia in the as treated analysis. The Kaplan-Meier curve diverged more in the later follow-up period for these outcomes ( fig 1 ), indicating that the effect would be greater with longer treatment. According to the follow-up time stratified analyses (46 767 propensity score matched pairs treated for two or less years, 16 827 pairs treated for more than two years; see supplemental table S8 for the distribution of baseline covariates for individual stratified groups), the magnitude of association modestly increased with more than two years of treatment compared with two years or less for these outcomes (see supplemental table S9): hazard ratio for more than two years versus two years or less of treatment was 0.52 (95% CI 0.41 to 0.66) v 0.57 (0.46 to 0.70) for dementia, 0.41 (0.29 to 0.57) v 0.45 (0.33 to 0.61) for dementia requiring drugs, and 0.48 (0.37 to 0.63) v 0.53 (0.41 to 0.68) for Alzheimer’s disease.

Kaplan-Meier curves for dementia-free survival comparing propensity score matched initiators of SGLT-2 inhibitors with initiators of DPP-4 inhibitors. CI=confidence interval; DPP-4=dipeptidyl peptidase-4; SGLT-2=sodium-glucose cotransporter-2
- Download figure
- Open in new tab
- Download powerpoint
Sensitivity analyses
The results were highly consistent even after accounting for the 365 day lag time from the index date ( table 3 ), with hazard ratios in as treated analyses of 0.57 (0.48 to 0.68) for dementia, 0.48 (0.38 to 0.61) for dementia requiring drugs, 0.55 (0.45 to 0.67) for Alzheimer’s disease, and 0.46 (0.26 to 0.80) for vascular dementia. In the intention-to-treat analyses with lag time applied, the hazard ratios were 0.80 (0.75 to 0.86) for dementia, 0.84 (0.77 to 0.91) for dementia requiring drugs, 0.80 (0.74 to 0.86) for Alzheimer’s disease, and 0.80 (0.66 to 0.98) for vascular dementia.
Lag time analyses on comparative risk of dementia in main propensity score matched cohort, with follow-up starting after 365 days from index date
For as treated analyses with longer grace periods after treatment change, a slightly increased incidence rate of dementia was noted in both treatment groups but to a greater degree among initiators of SGLT-2 inhibitors, with a hazard ratio of 0.72 (0.65 to 0.80) for dementia for a grace period of 180 days and 0.76 (0.69 to 0.83) for a grace period of 365 days (see supplemental table S10). Decreased incidence rates of genital infections were also noted among initiators of SGLT-2 inhibitors.
The results were consistent regardless of concurrent use of a drug with hypoglycaemic potential (see supplemental tables S11 and S12), with a hazard ratio of 0.69 (0.60 to 0.80) for dementia. The duration of type 2 diabetes was identified for 45 088 propensity score matched pairs (1008 v 925 days for initiators of SGLT-2 inhibitors and DPP-4 inhibitors, respectively, with a standardised difference of 0.10). Consistent results were observed after adjusting for duration of type 2 diabetes (see supplemental tables S13 and S14), with a hazard ratio of 0.60 (0.50 to 0.72) for dementia. We also observed similar results in propensity score based fine stratification weighted analyses (see supplemental tables S15 and S16), with a hazard ratio of 0.68 (0.62 to 0.75) for dementia.
Subgroup analysis
Supplemental table S17 presents the baseline characteristics of the subgroups. The lower risk associated with SGLT-2 inhibitors was overall consistent across subgroups stratified by age, sex, concurrent metformin use, and baseline cardiovascular risk ( fig 2 , also see supplemental table S18). However, statistical significance was not achieved for the subgroups with relatively small outcome numbers (eg, those aged <60 years). We did not find any interaction between the treatment and individual stratifying factors.

Comparative risk of dementia between initiators of sodium-glucose cotransporter-2 inhibitors and initiators of dipeptidyl peptidase-4 inhibitors in individual propensity score matched subgroups (as treated analysis). CI=confidence interval
This large population based cohort study among adults aged 40-69 years with type 2 diabetes found a 35% reduced risk of dementia associated with use of SGLT-2 inhibitors compared with DPP-4 inhibitors. This finding persisted regardless of dementia type and across subgroups of populations with diverse characteristics. Highly consistent results over a range of secondary and sensitivity analyses supported the robustness of our study findings. Our findings also suggest that the treatment effect of SGLT-2 inhibitors escalated with time.
Relevance of study design to internal validity
An active comparator new user design is a powerful pharmacoepidemiological approach that effectively copes with both measured and unmeasured confounding in observational studies. 33 One of the key advantages of this approach would be that similar disease (type 2 diabetes in our example) severity and related comorbidity profile can be expected between the two treatment groups because the participants in both groups are at the beginning of a similar stage of a given treatment. International guidelines had equally recommended SGLT-2 inhibitors and DPP-4 inhibitors as second line treatment until December 2018 34 when the revised guideline preferentially recommended use of SGLT-2 inhibitors in the presence of atherosclerotic cardiovascular disease, heart failure, or chronic kidney disease. 35 This approach also ensures that selection bias associated with depletion of susceptible people (to inefficacy or intolerance, or both) is avoided, allowing all individuals initiating the study drug to contribute to the follow-up from the start of the treatment. In this context, our study design offered greater internal validity than in previous studies. 15 16
Interpretation of results and comparison with other studies
We observed a known association between a positive control outcome and treatment. 22 The association for osteoarthritis related encounters was close to null (hazard ratio 0.97, 95% CI 0.95 to 0.98), which achieved statistical significance owing to excess power from a highly frequent outcome. A slight deviation (0.92, 0.89 to 0.96) from the null association was observed for cataract surgery. A bias measure (7.7% increased hazard ratio) based on this deviation indicated that the association between treatment and dementia was largely unexplained solely by residual confounding.
In preclinical studies, SGLT-2 inhibitors have shown direct neuroprotective effects through multiple pathways. 13 36 37 38 These drugs exhibited anticholinergic activity, 13 prevented ultrastructural changes of neurovascular units associated with cognitive decline in mice with diabetes, 36 and ameliorated amyloid β deposition and tau phosphorylation in the brain tissue of mice with Alzheimer’s disease and type 2 diabetes. 37 Diurnal catabolism induced by SGLT-2 inhibitors restored autophagy by downregulating the mTOR (mechanistic target of rapamycin) pathway, which is chronically activated in Alzheimer’s disease. 38 Based on these preclinical findings, SGLT-2 inhibitors may delay the progression of dementia in people with type 2 diabetes both for Alzheimer’s disease and for vascular dementia, independent of the cardiorenal benefits exerted by SGLT-2 inhibitors.
A considerable effect estimate found within a relatively short period (≤2 years) of follow-up needs attention. Dementia develops through a continuum of accumulated molecular and structural changes. 7 Heterogeneous states of disease progression yet to reach definitive dementia are likely to exist among people with type 2 diabetes at baseline or even after applying a one year lag time. This is likely true since mild cognitive impairment, a transitional state between normal ageing and dementia, 7 is prevalent among 12-18% and 23% of people aged ≥60 years in the US and Korea, respectively, with 10-15% of the annual conversion to dementia. 39 40 Notably, mild cognitive impairment is 1.4~2.0 times more prevalent among people with type 2 diabetes with accelerated progression. 41 42 43 44 Because the time span between mild cognitive impairment and dementia has already been shortened, and progression is particularly rapid among people with type 2 diabetes, early risk reduction against dementia could be seen in the presence of effective treatment (see supplementary figure S3 for a schematic explanation). This scenario also complies with the finding that the cognitive benefits of SGLT-2 inhibitor use versus non-use were better noted for those with mild cognitive impairment than with normal cognitive function at baseline. 17 Moreover, the visible action of SGLT-2 inhibitors versus DPP-4 inhibitors was rapid, based on the time elapsed until the first statistically significant result as early as day 5 for the benefits on death and worsening heart failure. 45
A recent prospective cohort study found that use of SGLT-2 inhibitors for more than three years improved cognitive function scores compared with non-use. 46 Although this finding suggests that longer treatment might generate more benefits, the study was subject to confounding by indication and immortal time bias owing to the comparison between users (eg, prevalent users) and non-users of SGLT-2 inhibitors. 33 Our study comparing new users of two competing drugs, SGLT-2 inhibitors and DPP-4 inhibitors, further supports favourable results for early initiation of the drug and prolonged treatment.
We observed attenuated results with lag time applied in intention-to-treat analyses and with longer grace periods. Since incidence rates of genital infections continually decreased among users of SGLT-2 inhibitors in these analyses, loss of treatment effect associated with misclassification of drug use played a role in driving the results towards null. Initiators of SGLT-2 inhibitors, however, were more frequently censored by treatment change than initiators of DPP-4 inhibitors. Because patients with risk factors for treatment change (non-adherence, inefficacy, or adverse events) can be more prone to develop dementia than patients without these risk factors, informative censoring may have overestimated the results in our as treated analysis. Nevertheless, the overall results between as treated and intention-to-treat analyses were similar ( table 2 ), suggesting non-substantial informative censoring.
In subgroup analyses, we observed highly consistent results, but did not find an interaction between treatment and individual characteristics of the study population. Unlike the expectation that SGLT-2 inhibitors might be associated with greater benefits against the risk of vascular dementia than Alzheimer’s disease, the magnitude of association was accompanied by widely overlapping 95% CIs between the two types of dementia for all analyses. Thus, it is not surprising to observe no interaction between treatment and baseline cardiovascular risk. A recent meta-analysis also reported that the pooled beneficial association between dementia and use of SGLT-2 inhibitors versus other antiglycaemic treatments was not affected by cardiovascular diseases. 10 These findings suggest that the underlying mechanisms are not limited to cardiorenal pathways, possibly involving direct neuroprotective pathways observed in preclinical studies. 13 36 37 38 According to previous studies on metformin monotherapy versus no treatment, metformin was not associated with incident dementia. 47 48 Based on these findings, concurrent use of metformin is unlikely to interact with SGLT-2 inhibitors in modifying the risk of dementia.
Strengths and limitations of this study
Several important strengths of this study deserve comment. Firstly, we used rigorous pharmacoepidemiological approaches, in particular we adopted an active comparator new user design and extensive propensity score matching. 33 The diagnosis codes in the primary position and applying disease specific drugs would increase the specificity of the outcome. The sensitivity analyses and control outcomes add relevant internal validity to this study. Secondly, compared with a previous study, 14 we included relatively younger people (aged 40-69 years) with type 2 diabetes, broadening the target population of benefits associated with use of SGLT-2 inhibitors. Thirdly, we used a nationally representative database, providing high generalisability. Fourthly, we performed comprehensive analyses for time varying comparisons of SGLT-2 inhibitors versus DPP-4 inhibitors, diverse subgroups, and individual types of dementia, presenting highly consistent results.
This study also has limitations. Firstly, owing to the observational nature of our study, it is inherently subject to residual or unmeasured confounding. Although we balanced many proxies of type 2 diabetes severity and comorbidities and used negative control outcomes, direct test results on serum glucose levels, renal function, severity of other comorbidities, health behaviours (eg, smoking and alcohol consumption), and duration of type 2 diabetes were not fully ascertainable from the claims data. Secondly, diagnoses of dementia are commonly delayed, rendering studies on dementia risk particularly susceptible to informative censoring, reverse causation, and outcome misclassification, which may have resulted in overestimation of our results. Thirdly, our study did not provide exact mechanisms of neuroprotection.
Conclusions
This large population based cohort study found that initiation of SGLT-2 inhibitors was associated with a 35% lower risk of dementia compared with initiation of DPP-4 inhibitors in people with type 2 diabetes aged 40-69 years. This association was similarly observed for Alzheimer’s disease and vascular dementia and was also consistent across subgroups. We observed a greater association with treatment duration longer than two years. These findings underscore the need for future randomised controlled trials.
What is already known on this topic
Despite increasing numbers of people with dementia globally, current options for disease modifying treatments are limited
Type 2 diabetes substantially predisposes people to Alzheimer’s disease and vascular dementia through multiple pathways
A previous study suggested a decreased risk of dementia associated with sodium-glucose cotransporter-2 (SGLT-2) inhibitors versus dipeptidyl peptidase-4 (DPP-4) inhibitors among people with type 2 diabetes aged >66 years
What this study adds
This large population based cohort study among people with type 2 diabetes aged 40-69 years found a 35% lower risk of dementia associated with use of SGLT-2 inhibitors compared with DPP-4 inhibitors
This finding persisted regardless of dementia type and across subgroups of diverse population characteristics such as age, sex, concomitant use of metformin, and baseline cardiovascular risk
The treatment effect of SGLT-2 inhibitors compared with DPP-4 inhibitors increased with time
Ethics statements
Ethical approval.
The Institutional Review Board of the Seoul National University Bundang Hospital exempted the study protocol (X-2206-762-901) and waived written patient consent based on the fully deidentified database.
Data availability statement
Patient level data are not publicly allowed according to data use agreement. Aggregate level data can be requested from the corresponding author.
Acknowledgments
We thank Joongyub Lee (Department of Preventive Medicine, Seoul National University College of Medicine, Seoul, Korea) for comments on the pharmacoepidemiological design of this study.
Contributors: EHK conceived and designed the study and drafted the manuscript. AS did the analyses. EHK and AS had full access to all the data in the study and take responsibility for the integrity of the data and accuracy of the data analysis. All authors interpreted the data, critically revised the manuscript for important intellectual content, and approved the final version. EHK is the guarantor. The corresponding author attests that all listed authors meet authorship criteria and that no others meeting the criteria have been omitted.
Funding: This study was supported by the Korea Health Industry Development Institute (KHIDI)-AZ Diabetes Research programme (grant No 08-2022-0261). The funders had no role in considering the study design or in the collection, analysis, interpretation of data, writing of the report, or decision to submit the article for publication.
Competing interests: All authors have completed the ICMJE uniform disclosure form at www.icmje.org/disclosure-of-interest/ and declare: support from the Korea Health Industry Development Institute (KHIDI)-AZ Diabetes Research programme; no financial relationships with any organisations that might have an interest in the submitted work in the previous three years; no other relationships or activities that could appear to have influenced the submitted work. EHK receives grants from Celltrion and SK Chemicals to Seoul National University Bundang Hospital for unrelated studies. BKK receives grants from KHIDI to SMG-SNU Boramae Medical Centre for unrelated studies.
Transparency: The guarantor (EHK) affirms that this manuscript is an honest, accurate, and transparent account of the study being reported; that no important aspects of the study have been omitted; and that any discrepancies from the study as planned (and, if relevant, registered) have been explained.
Dissemination to participants and related patient and public communities: The use of deidentified data preclude direct dissemination to participants, and we have no plans to involve patients in the dissemination of study results. Study findings will be disseminated by all coauthors through their own institutions. The article will be distributed within the corresponding author’s institution.
Provenance and peer review: Not commissioned; externally peer reviewed.
This is an Open Access article distributed in accordance with the Creative Commons Attribution Non Commercial (CC BY-NC 4.0) license, which permits others to distribute, remix, adapt, build upon this work non-commercially, and license their derivative works on different terms, provided the original work is properly cited and the use is non-commercial. See: http://creativecommons.org/licenses/by-nc/4.0/ .
- American Psychiatric Association
- ↵ World Health Organization. Fact sheets of dementia. Geneva, Switzerland: WHO; 2021. www.who.int/news-room/fact-sheets/detail/dementia .
- Gauthier S ,
- Livingston G ,
- Huntley J ,
- Sommerlad A ,
- Chatterjee S ,
- Peters SA ,
- Woodward M ,
- Biessels GJ ,
- Staekenborg S ,
- Brunner E ,
- Scheltens P
- Shaaban CE ,
- Kuate Defo A ,
- Pisaturo A ,
- Daskalopoulou SS
- Gunawan PY ,
- Gunawan PA ,
- Hariyanto TI
- Heerspink HJL ,
- Cuthbertson DJ ,
- Wilding JPH
- Katsenos AP ,
- Iskander C ,
- Verhagen C ,
- Janssen J ,
- CARMELINA Investigators
- Taylor DH Jr . ,
- Plassman BL
- Lipsitch M ,
- Tchetgen Tchetgen E ,
- Lindblad BE ,
- Håkansson N ,
- Philipson B ,
- Richardson DB ,
- Laurier D ,
- Schubauer-Berigan MK ,
- Sundararajan V ,
- Henderson T ,
- Muggivan A ,
- Austin PC ,
- Rothman KJ ,
- Bateman BT ,
- Hernandez-Diaz S ,
- Huybrechts KF
- Yoshida K ,
- Solomon DH ,
- Inzucchi SE ,
- Bergenstal RM ,
- Davies MJ ,
- D’Alessio DA ,
- Fradkin J ,
- Hayden MR ,
- Hierro-Bujalance C ,
- Infante-Garcia C ,
- Del Marco A ,
- Esterline RL ,
- Oscarsson J ,
- ↵ 2022 Alzheimer’s disease facts and figures . Alzheimers Dement 2022 ; 18 : 700 - 89 . pmid: 35289055 OpenUrl CrossRef PubMed
- Luchsinger JA ,
- Winkler A ,
- Cukierman T ,
- Gerstein HC ,
- Williamson JD
- Patorno E ,
- Everett BM ,
- Morley JE ,
- Scherrer JF ,
Interdisciplinary Centre for Black Health named finalist in Type 2 Diabetes Prevention Challenge
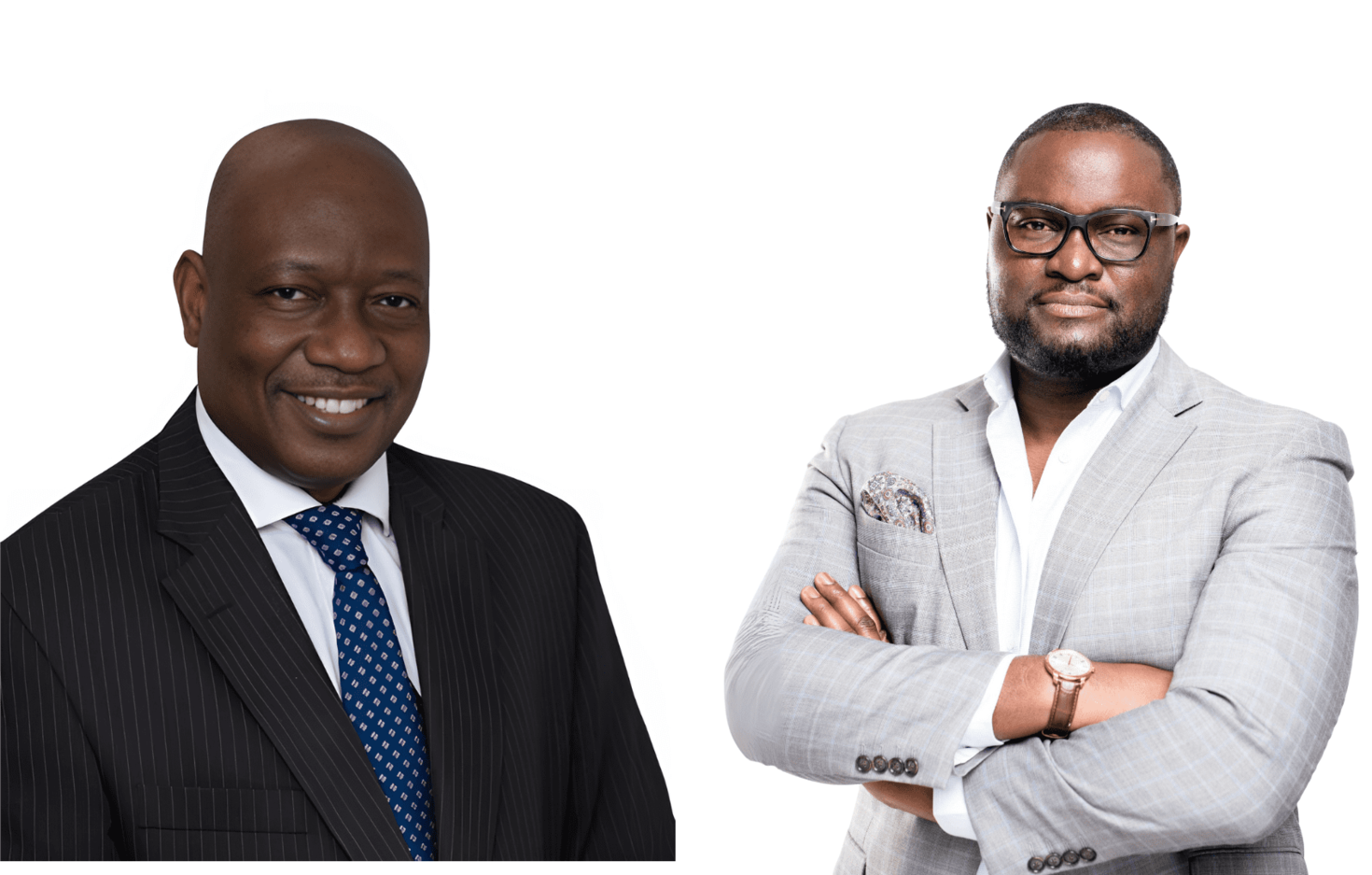
The ICBH’s project titled “ Train, Inform, Involve and Transform to Reduce the Risk of Type 2 Diabetes in Black Communities in Canada ” aims to train students from various disciplines to become peer educators. They in turn will inform religious leaders and encourage Black communities to take part in diabetes prevention activities, thereby helping to raise awareness and reduce the risks associated with the disease. “This funding will enable us to implement tangible, sustainable solutions to improve the health of Black communities disproportionately affected by Type 2 diabetes,” Cénat said.
Cénat will be leading the project along with Professor Idrissa Beogo from the School of Nursing in the Faculty of Health Sciences . Beogo, currently ICBH acting director, will work with Cénat, who will use his sabbatical year to step up his research into the health of Black populations in North America. The work of the ICBH and its team has enjoyed wide recognition, for example, through awards and ample media coverage, making the organization a key player in guiding the efforts of government agencies in the fight against health inequalities.
Read the full article on Collabzium .
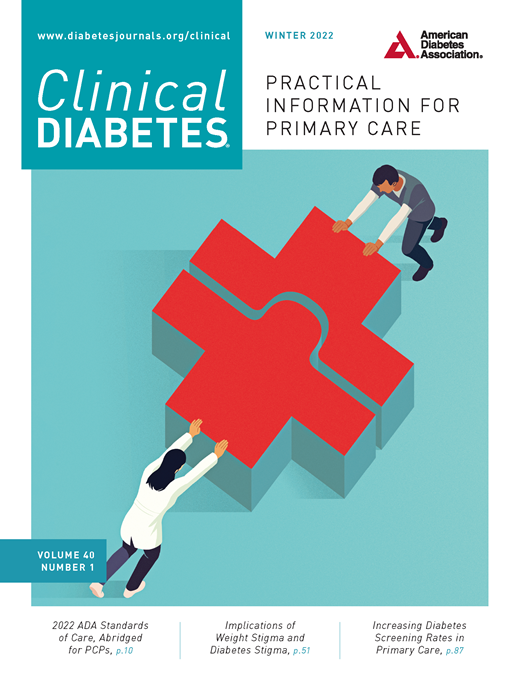
- Previous Article
- Next Article
Describe your practice setting and location.
Describe the specific quality gap addressed through the initiative., how did you identify this quality gap in other words, where did you get your baseline data, summarize the initial data for your practice (before the improvement initiative)., what was the time frame from initiation of your qi initiative to its completion, describe your core qi team. who served as project leader, and why was this person selected who else served on the team, describe the structural changes you made to your practice through this initiative., describe the most important changes you made to your process of care delivery., if you used the “plan, do, study, act” (pdsa) change model, provide details for one example., summarize your final outcome data (at the end of the improvement initiative) and how it compared with your baseline data., what are your next steps, what lessons did you learn through your qi process that you would like to share with others, article information, increasing diabetes screening in a primary care setting.

- Split-Screen
- Article contents
- Figures & tables
- Supplementary Data
- Peer Review
- Open the PDF for in another window
- Cite Icon Cite
- Get Permissions
Jennifer J. Iyengar , Mary E.K. Fisher , Julie E. Ziegler , Douglas W. Wright , Meghan C. Monson , Cheryl L. Rhodes Dehmlow , Keturah P. Schacht , Jennifer A. Wyckoff , Audrey L. Fan; Increasing Diabetes Screening in a Primary Care Setting. Clin Diabetes 1 January 2022; 40 (1): 87–91. https://doi.org/10.2337/cd21-0039
Download citation file:
- Ris (Zotero)
- Reference Manager
Quality Improvement Success Stories are published by the American Diabetes Association in collaboration with the American College of Physicians and the National Diabetes Education Program. This series is intended to highlight best practices and strategies from programs and clinics that have successfully improved the quality of care for people with diabetes or related conditions. Each article in the series is reviewed and follows a standard format developed by the editors of Clinical Diabetes . The following article describes an initiative to increase rates of diabetes screening in a large multisite academic health system in the greater Ann Arbor, MI, area.
Michigan Medicine (MM) is a large academic medical center located in Ann Arbor, MI. There are 14 primary care sites within the MM system, with six locations in Ann Arbor and eight locations in surrounding communities in southeast Michigan. Approximately 60–70 general internal medicine physicians and 40–50 family medicine physicians provide primary care within the health system, with additional primary care services provided by geriatrics and obstetrics/gynecology clinicians ( 1 ). In total, there are ∼2.4 million outpatient visits to the health care system each year, including both primary and specialty care services ( 2 ).
The American Diabetes Association (ADA) estimates that 7.3 million adults in the United States have undiagnosed diabetes ( 3 ). Undiagnosed diabetes can lead to treatment delays and the potential for acute hyperglycemic complications, as well as long-term micro- and macrovascular complications. An additional 88 million adults in the United States have prediabetes, of whom 84% may not realize they have it ( 4 ). Identification of prediabetes is important because there are proven lifestyle interventions that can prevent or delay the progression of prediabetes to type 2 diabetes.
The focus of this quality improvement (QI) initiative was to identify and screen patients in primary care who are at risk for prediabetes or diabetes and thereby minimize the number of patients with undiagnosed diabetes. The goal was to have a simple yet large-scale intervention that would have a measurable population-level impact across the health care system. Our intervention involved the use of an alert in the electronic medical record (EMR) system called a best practice advisory (BPA) to notify health care providers (HCPs) of patients who met criteria for diabetes screening.
The MM Diabetes Mellitus Quality Improvement Committee was established in 2002 to support diabetes-related clinical operations in the ambulatory care setting. As part of this effort, the QI committee regularly monitors the institution’s performance on key diabetes-related quality metrics such as glycemic control, monitoring of microvascular complications, and statin use.
A prerequisite to appropriately monitoring diabetes care is to correctly identify which adult patients in the health system have a diagnosis of diabetes. The population of people with diabetes managed by MM endocrinology and/or primary care clinicians has been steadily increasing over time and had risen from 12,141 patients in 2013 to 13,327 patients in 2016. However, we still noted a significant number of patients at MM with risk factors for diabetes who had not had a recent screening test. This situation was first noted anecdotally by the QI team leaders and later confirmed by baseline measurement in December 2016, which found that there were 16,044 patients at MM who were 40–70 years of age and without a current diagnosis of diabetes who had had no A1C or glucose testing in the past 3 years. Hence, there was concern that there could still be patients with unrecognized diabetes in our institution. This discovery prompted further investigation to identify how many of these patients would meet criteria for screening based on current guidelines.
We applied the U.S. Preventative Services Task Force (USPSTF) recommendations for type 2 diabetes screening to the MM population to determine the number of patients at our institution who would meet screening criteria. The USPSTF recommends screening patients who are 40–70 years of age with overweight or obesity every 3 years ( 5 ). Screening can be performed using an A1C, fasting plasma glucose, or oral glucose tolerance test. The USPSTF guidelines indicate that physicians can consider earlier screening for patients with certain risk factors for diabetes ( Supplementary Figure S1 ). For the purposes of our project, we used just the main criteria, which results in a lower sensitivity but higher specificity compared with the expanded criteria ( 6 ). Similarly, use of the ADA criteria ( Supplementary Figure S1 ) would result in more patients being eligible for screening and a higher sensitivity; however, this strategy was not felt to be feasible for our project, as some of the criteria (e.g., physical activity) are not available in the EMR system, and others (e.g., family history or personal history of gestational diabetes) may not be reliably recorded there ( 7 , 8 ). The lower sensitivity of the USPSTF criteria is a limitation of this project ( 9 ).
As of 31 December 2016, 109,680 patients aged 40–70 years had been seen at MM in the past 3 years in either primary care, endocrinology, nephrology, or cardiology clinics. Primary care clinics included general medicine, medicine/pediatrics, family medicine, and geriatrics. A total of 10,427 unique patients met USPSTF criteria for screening based on having a BMI ≥25 kg/m 2 , current diagnosis of diabetes, and an absence of A1C or glucose test results in the past 3 years. Of these, 7,303 were seen in primary care, 2,280 in cardiology, 996 in endocrinology, and 48 in nephrology (although some patients were seen in multiple clinics in the 3-year period examined). Patients seen in nephrology were most likely to have had A1C or glucose testing, followed by those seen in endocrinology. Patients seen in a cardiology clinic were least likely to have had A1C or glucose testing.
There was concern about the potential volume of patients who would qualify for screening, so it was important to identify a manageable target population. We also needed to ensure adequate resources and support to manage the influx of new diagnoses. The decision was made to target primary care for the initial screening intervention, with the option to roll out the initiative in specialty clinics later.
The initial pilot for the intervention was launched in two primary care clinic locations on 15 November 2017. Data from the pilot were reviewed in February 2018. The intervention was then rolled out in all MM general medicine and family medicine clinics in March 2018. Geriatrics was added in August 2018, and obstetrics/gynecology clinics were added in January 2020. These clinics were selected because both geriatrics and obstetrics/gynecology can provide primary care services within our health system.
The MM Diabetes QI Committee is led by one physician representative from general medicine and one physician representative from endocrinology. Both have extensive experience in QI, with the general medicine lead also serving as the associate medical director for the University of Michigan Medical Group Quality Department and the endocrinology lead also serving as the associate clinical chief of the Division of Metabolism, Endocrinology & Diabetes/Podiatry, as well as the medical director for the Adult Diabetes Education Program. Quality Analytics, a division of the MM Quality Department, played a crucial role by collecting and analyzing the data at all stages of the project, and two members of Quality Analytics serve on the MM Diabetes QI Committee. Representatives of Health Information Technology Services also sit on the committee and provided assistance with the creation of the BPA and its implementation in the EMR system. The committee also includes two additional physicians (one from primary care and one from endocrinology), a pharmacist, a diabetes educator, a registered dietitian, an administrative director, a project manager, and others, as noted in acknowledgments .
A BPA is an EMR-based point-of-care alert that facilitates patient care aligned with evidence-based practices and institutional initiatives. These alerts can be linked to “smart sets,” which allow for laboratory test ordering, referrals, health maintenance documentation, and other resources.
Age 40–70 years
BMI ≥25 kg/m 2
No A1C or blood glucose test in the past 3 years
No current diagnosis of diabetes (based on the institution’s diabetes registry)
Although the USPSTF recommends A1C or fasting blood glucose testing to satisfy the screening requirement, our EMR system does not identify whether a patient was fasting for a given blood draw. The decision was made to use any glucose measurement to satisfy the requirement. This decision would result in fewer inappropriate BPA activations but might miss screening some patients who had a recent nonfasting glucose measurement. When the BPA triggered, it recommended either a point-of-care or a laboratory A1C test for the initial screening, which would allow screening to occur on the same day as the visit rather than waiting for a fasting laboratory test.
To have a successful BPA rollout, we had to consider how this EMR alert would integrate with existing clinic workflows and what action steps would be required by physicians and staff when it triggered. The recommended workflow was for the medical assistant (MA) to review all activated BPAs at the time of rooming. When activated, the BPA was linked to an order set allowing the MA to prepare a pending A1C order, either at the point of care or at the laboratory, based on clinic preference. The physician would review the pending order during the clinic visit and sign it if the patient was agreeable to screening. Before rollout at each clinic location, an e-mail was sent to the clinic leads detailing this workflow, along with EMR screenshots and tip sheets.
Patient education materials, in both electronic and print formats
Referral to group classes, including a type 2 diabe tes education class and a lifestyle change program based on the Diabetes Prevention Program
Referral to one-on-one nutrition counseling
Referral to a pharmacist for chronic care management
Referral to endocrinology for specialized diabetes management
Plan. The BPA alert was initially piloted at a general medicine clinic in Northville, MI, and a family medicine clinic in Chelsea, MI.
Do. The BPA pilot went live on 15 November 2017, and data were analyzed for the period from 21 November 2017 to 15 January 2018.
Study. During the pilot, the BPA activated for 470 unique patients. Of these, 81 had an A1C ordered as part of the BPA “smart” order set. It is possible that more patients had an A1C ordered during their visit; however, only orders that were part of the order set were captured during the pilot period. Of the patients who had an A1C test ordered via the order set, 34.6% ( n = 28) had an A1C in the prediabetes range, and 7.4% ( n = 6) had an A1C >6.4%, indicative of diabetes.
Act. Based on these data, it was felt that the BPA had activated in a sufficiently small/targeted population to make it manageable for HCPs, but also resulted in a high enough positive screen rate to justify rolling it out more broadly. The intervention was subsequently rolled out in all MM general medicine and family medicine clinics (in March 2018) and in geriatrics (in August 2018).
Before the start of the intervention in December 2016, 7,303 patients seen in primary care in the previous 3 years were eligible for the BPA on the basis of being 40–70 years of age, having a BMI ≥25 kg/m 2 , and having no current diagnosis of diabetes. As of December 2019, the BPA-eligible population was reduced to 5,377 patients. This reduction occurred despite an increase in the overall patient population ages 40–70 years who were followed by primary care during the same time frame, from 88,138 to 92,865, and a similar increase in the subset of those patients who also had a BMI ≥25 kg/m 2 from 63,666 to 67,439.
The BPA activated during an office visit for 6,703 patients between the time it was introduced and December 2019. Of those for whom the BPA activated, 77% ( n = 5,181) had subsequent A1C or glucose testing performed, and 927 had either diabetes or prediabetes added to their EMR problem list as a result of the BPA. Supplementary Figure S2 depicts the change in the reportable diabetes population at MM from December 2013 to December 2019.
In January 2020, there was an additional rollout of the BPA in the obstetrics/gynecology clinics. Looking at the total BPA utilization for the first 6 months (through June 2020), there were 2,884 patients for whom the BPA activated, of whom 55% ( n = 1,574) had subsequent diabetes screening, and 274 had diabetes or prediabetes added to their EMR problem list.
The lower rate of A1C testing may have been caused, in part, by the coronavirus disease 2019 pandemic and related transition to virtual visits. It is generally easier and faster to obtain laboratory testing for patients who are physically present in the clinic. Depending on the ongoing need for virtual visits, we may need to make further adjustments to accommodate this new and rapidly evolving visit format. Breaking down the screening data based on visit type (in person vs. virtual) may provide further insights to inform these next steps.
Future updates to the USPSTF guidelines may expand the age criteria for screening eligibility, and we anticipate updating the BPA accordingly. We are also considering expanding the BPA screening criteria to add other groups not included in the current version such as women with a history of gestational diabetes or polycystic ovary syndrome and patients with a family history of diabetes.
Finally, this project did not address disparities in diabetes screening rates within our patient population. Identifying any possible disparities in screening rates would be an important future direction to ensure equitable health care delivery.
Our results illustrate how an EMR system can be used to change screening behavior on a population level across multiple primary care specialties in a large health system. Key to our success was the ability of the QI committee to bring together clinician leadership, analytic support, and EMR expertise to enact these changes. It was also important to have buy-in from the primary care clinical sites and the individual providers.
By rolling out the intervention first on a pilot basis in a small number of clinics and then more broadly in phases, we were able to see how it could be incorporated into existing workflows and assess its functioning at regular intervals to ensure that the BPA was having the desired result. The pilot data indicated that the BPA was actionable without being overburdensome and allowed us to obtain approval from the physician leads at all the general medicine clinical sites for the broader rollout.
Primary care providers already understand the clinical importance of identifying patients with diabetes, but to get their support, we needed to ensure that the BPA identified a manageable target population and that the recommended action when the BPA triggered (in this case, ordering A1C testing) was something readily achievable in a busy practice environment. Providers also had access to a robust set of resources to manage patients with a new diagnosis of prediabetes or diabetes, allowing them to respond appropriately to positive screening results. Finally, the intervention selected was low maintenance and thus could be continued over the long term for lasting impact.
Acknowledgments
The authors acknowledge the other members of the MM Diabetes QI Committee who served during the time this project took place: Diabetes Education – Emily Weatherup, RD, CDE; Ambulatory Care Services – Emily Collins, RD, Dietitian Manager; Ambulatory Care Services – Elizabeth Seif, Project Manager; Quality Department – Heather Fazio, RDN, PMP, Quality Improvement Committee Project Manager; Family Medicine – Jenna Greenburg, MD; Pharmacy – Heidi Diez, PharmD; and Learning Health Sciences – Van Harrison, PhD.
Duality of Interest
No potential conflicts of interest relevant to this article were reported.
Author Contributions
J.J.I., M.E.K.F., J.E.Z., M.C.M., C.L.R.D., K.P.S., J.A.W., and A.L.F. all serve on the MM Diabetes QI Committee. J.J.I. drafted the manuscript. M.E.K.F. collected and analyzed data and wrote a poster on which the manuscript was based. J.E.Z. collected and analyzed data. D.W.W. queried the data. C.L.R.D. designed the clinical decision-support BPA used in the project. M.C.M. and K.P.S. reviewed and edited the manuscript. J.A.W. and A.L.F. contributed to the conception and design of the work, interpretation of the data, and critical revision of the manuscript. J.J.I. is the guarantor of this work and as such, had full access to all of the data and takes responsibility for the integrity of data and the accuracy of the report.
J.A.W. and A.L.F. are co-senior authors.
This series is published by the American Diabetes Association in collaboration with the American College of Physicians, Inc., and the National Diabetes Education Program. The American College of Physicians and the American College of Physicians logos are trademarks or registered trademarks of American College of Physicians, Inc., in the United States and shall not be used otherwise by any third party without the prior express written consent of the American College of Physicians, Inc. Likewise, products and materials that are not developed by or in partnership with the National Diabetes Education Program are prohibited from using the National Diabetes Education Program logo.
This article contains supplementary material online at https://doi.org/10.2337/figshare.16564221
Email alerts
- Online ISSN 1945-4953
- Print ISSN 0891-8929
- Diabetes Care
- Clinical Diabetes
- Diabetes Spectrum
- Standards of Medical Care in Diabetes
- Scientific Sessions Abstracts
- BMJ Open Diabetes Research & Care
- ShopDiabetes.org
- ADA Professional Books
Clinical Compendia
- Clinical Compendia Home
- Latest News
- DiabetesPro SmartBrief
- Special Collections
- DiabetesPro®
- Diabetes Food Hub™
- Insulin Affordability
- Know Diabetes By Heart™
- About the ADA
- Journal Policies
- For Reviewers
- Advertising in ADA Journals
- Reprints and Permission for Reuse
- Copyright Notice/Public Access Policy
- ADA Professional Membership
- ADA Member Directory
- Diabetes.org
- X (Twitter)
- Cookie Policy
- Accessibility
- Terms & Conditions
- Get Adobe Acrobat Reader
- © Copyright American Diabetes Association
This Feature Is Available To Subscribers Only
Sign In or Create an Account
- Open access
- Published: 24 August 2024
User engagement in clinical trials of digital mental health interventions: a systematic review
- Jack Elkes 1 ,
- Suzie Cro 1 ,
- Rachel Batchelor 2 ,
- Siobhan O’Connor 3 ,
- Ly-Mee Yu 2 ,
- Lauren Bell 4 ,
- Victoria Harris 2 ,
- Jacqueline Sin 5 na1 &
- Victoria Cornelius 1 na1
BMC Medical Research Methodology volume 24 , Article number: 184 ( 2024 ) Cite this article
209 Accesses
1 Altmetric
Metrics details
Introduction
Digital mental health interventions (DMHIs) overcome traditional barriers enabling wider access to mental health support and allowing individuals to manage their treatment. How individuals engage with DMHIs impacts the intervention effect. This review determined whether the impact of user engagement was assessed in the intervention effect in Randomised Controlled Trials (RCTs) evaluating DMHIs targeting common mental disorders (CMDs).
This systematic review was registered on Prospero (CRD42021249503). RCTs published between 01/01/2016 and 17/09/2021 were included if evaluated DMHIs were delivered by app or website; targeted patients with a CMD without non-CMD comorbidities (e.g., diabetes); and were self-guided. Databases searched: Medline; PsycInfo; Embase; and CENTRAL. All data was double extracted. A meta-analysis compared intervention effect estimates when accounting for engagement and when engagement was ignored.
We identified 184 articles randomising 43,529 participants. Interventions were delivered predominantly via websites (145, 78.8%) and 140 (76.1%) articles reported engagement data. All primary analyses adopted treatment policy strategies, ignoring engagement levels. Only 19 (10.3%) articles provided additional intervention effect estimates accounting for user engagement: 2 (10.5%) conducted a complier-average-causal effect (CACE) analysis (principal stratum strategy) and 17 (89.5%) used a less-preferred per-protocol (PP) population excluding individuals failing to meet engagement criteria (estimand strategies unclear). Meta-analysis for PP estimates, when accounting for user engagement, changed the standardised effect to -0.18 95% CI (-0.32, -0.04) from − 0.14 95% CI (-0.24, -0.03) and sample sizes reduced by 33% decreasing precision, whereas meta-analysis for CACE estimates were − 0.19 95% CI (-0.42, 0.03) from − 0.16 95% CI (-0.38, 0.06) with no sample size decrease and less impact on precision.
Discussion
Many articles report user engagement metrics but few assessed the impact on the intervention effect missing opportunities to answer important patient centred questions for how well DMHIs work for engaged users. Defining engagement in this area is complex, more research is needed to obtain ways to categorise this into groups. However, the majority that considered engagement in analysis used approaches most likely to induce bias.
Peer Review reports
One in four people experience a mental health problem every year [ 1 ]. However, an estimated 70% with mental ill health are unable to access treatment [ 2 ]. App and web-based tools, collectively digital mental health interventions (DMHIs), are low cost, scalable [ 3 ], and have potential for overcoming traditional barriers to treatment access, such as physical access (flexibility in treatment location), confidentiality (providing anonymity), and stigma [ 4 ]. In recent years, the number of available DMHIs has rapidly increasd [ 5 ], the Apple App Store alone has over 10,000 behavioural apps [ 6 ]. This rapid increase combined with the complex nature of DMHIs has meant safety and effectiveness regulations have lagged behind [ 7 ]. Additionally, many DMHIs are developed for commercial purposes and marketed to the public without scientific evidence [ 8 ]. The current National Institute for Health and Care Excellence (NICE) guidelines [ 9 ] for digital health technologies, advocate for use of randomised controlled trials (RCTs) to evaluate the effectiveness of digital interventions in specific conditions such as mental health. Promisingly, the number of digital interventions evaluated in RCTs over the last decade has more than doubled [ 10 ].
Many DMHIs are developed through the digitalisations of existing services, such as online self-led formats of conventional therapist-delivered treatments. However, in contrary to conventional therapist-led treatments, DMHIs offer flexible anytime access for individuals [ 11 ]. This change in delivery means existing evidence of risk-benefit balance from structured therapist-delivered interventions is not translatable. DMHIs are potential solutions to provide more individuals with much needed treatment access, but they are not without challenges. In 2018 the James Lind Alliance (JLA) patient priority setting group for DMHIs set out the top 10 challenges to address [ 12 ]. Overcoming these challenges is essential for DMHIs to successfully improve treatment access and health outcomes in mental health [ 13 , 14 ]. One theme that emerged from across the priorities was the importance of improving methods for evaluating DMHIs including the impact of user engagement.
The impact user engagement has on DMHIs efficacy is poorly understood [ 6 , 15 , 16 ]. Although DMHIs are widely available, user engagement with DMHIs is typically low [ 17 ]. For multi-component DMHIs (commonly including psychoeducation, cognitive exercises, self-monitoring diary), a minimally sufficient engagement in DMHIs is often crucial for establishing behavioural changes and thus improved health outcomes [ 18 ]. However, achieved sustained behavioural changes by engaging with DMHIs is a multidimensional construct that is both challenging to assess and the pathway for patients to achieve this is complex [ 19 , 20 ]. Unlike other interventions, DMHIs are unique in that web-based or app-based interventions can capture interactions from individuals. User engagement can be measured and recorded using automatically captured indicators (e.g., pageviews, proportion of content/modules completed, or number of logins). However, the large variety in measurable indicators across different DMHIs [ 16 , 21 ] further compounds challenges to understanding pathways to sustained behaviour changes.
For RCTS, the latest estimand framework in the ICH E9 R1 addendum [ 22 ] provides guidance on defining different estimands, which enables trialists to ensure the most important research questions of interest are evaluated. This includes guidance on handling post-randomisation events, such as user engagement with the DMHI, in efficacy analysis. For example, policy makers are likely to be most interested in a treatment policy estimand which provides an assessment of the benefit received on average under the new policy of prescribing the DMHI regardless of how it’s engaged with. For DMHIs typically engagement is poor, which means treatment policy estimands may underestimate the true intervention efficacy for those who engaged [ 23 ], so alternative estimands that address this may also be of interest to target. For example, the benefit received on average for individuals who would actively engage with the DMHI (a principal stratification estimand). However, to utilise available methods post-randomisation variables need to be clearly defined, but this is difficult for engagement with DMHIs because it is multifaceted with many different engagement indicators available to use.
This systematic review aimed to assess the current landscape of how RCTs for DMHIs are reported and analysed. The review primarily assessed how user engagement is described, what engagement indicators are reported and how, if at all, researchers assessed the impact of user engagement on efficacy. As the number of DMHIs evaluated in RCTs is ever increasing, this review is essential to identify current practice in trial reporting to inform further research to improve the quality of future trials. The specific research aims of interest were to: (1) examine trial design and characteristics of DMHIs; (2) summarise how user engagement had been defined and measured in RCTs of DMHIs; and (3) assess how often intervention efficacy was adjusted for user engagement and the impact of user engagement on efficacy estimates.
The protocol for this systematic review was prospectively published in Prospero [ 24 ], and PRISMA guidance was followed in reporting of this review.
Study selection
We included RCTs examining the efficacy of DMHIs, excluding pilot and feasibility studies [ 25 ]. Search terms for RCT designs followed guidance from Glanville et al. [ 26 ]. We included trials of participants with common mental disorders (CMD) defined by Cochrane [ 27 ] excluding populations with non-CMD comorbidities, such as patients with depression and comorbid diabetes. Populations with multiple CMDs were not excluded as there were many transdiagnostic interventions targeting overlapping symptoms of different conditions. Both trials requiring a confirmed clinical diagnosis and trials where participants self-referred were included. For consistency in DMHIs included interventions must meet any criteria from items 1.1 (targeted communication on health information), 1.3 (client to client communication, e.g., peer forums), 1.4 (health tracking or self-monitoring) or 1.6 (access to own health information) from the WHO Classification of Digital Health Interventions [ 28 ]. DMHIs must have been delivered on a mobile app or through a web-browser and where the intervention was self-guided by participants, defined as an intervention where participants have full autonomy over how this is used. Search terms for interventions followed guidance from Ayiku et al. [ 29 ]. All publications must have been reported in English.
The search was performed on the 17th September 2021 and included trials published between 1st January 2016 to 17th September 2021. Search terms were adapted for each database: MEDLINE, Embase, PsycINFO and Cochrane CENTRAL (see supplemental table S1 for search strategy). Title and abstracts were independently screened by two reviewers (JE, RB, SO, LB, LM & VH), and again at the full text review stage. Covidence [ 30 ] was used to manage all stages, remove duplicates and resolve disagreements.
As a methodology review to examine how user engagement was described and analysed a risk of bias tool to assess trial quality was not undertaken [ 31 ]. However, key CONSORT items [ 32 ] were extracted to determine adherence to reporting guidance, including reporting of a protocol or trial registration (item 23/24), planned sample size (item 7a) and amendments to the primary analysis (item 3b). For all items self-reported data from articles was extracted.
Data extraction
A data extraction form was developed by the lead author (JE) and reviewed by VC, SC and JS. Summary data extracted covered: trial characteristics (e.g., design and sample size); intervention and comparator descriptions (e.g., delivery method or primary function); participant demographics (e.g., age or gender); reporting of user engagement (e.g., indicators reported); and point estimates, confidence intervals and P-values of analysis results unadjusted and adjusted for user engagement. In trials with multiple arms the first active arm mentioned was included. No restriction was applied to the control arm in the trial. The full extraction sheet, including CONSORT items, is in the table S2 of the supplementary material.
The analysis was predominantly descriptive and used mean and standard deviations, or medians and interquartile ranges (IQRs) to describe continuous variables. Frequencies and percentages summarized categorical variables. User engagement captured through engagement indicators (e.g., pageviews and total logins) and methods to encourage user engagement (e.g., automatic notifications) were summarised descriptively. Indicator data was summarised in four categories: duration of use (e.g., length of session), frequency of use (e.g., number of logins), milestone achieved (e.g., modules completed) and communication (e.g., messages to therapist). Descriptive summaries also assessed both the recommended user engagement definitions, the pre-specified minimum engagement level investigators told participants to use DMHIs, and active user definitions, the pre-specified engagement level of most interest to investigators for intervention effects accounting for user engagement. Both were summarised by indicators used in definitions.
To determine the impact of user engagement on intervention efficacy, restricted maximum likelihood random effects meta-analyses were conducted for articles that reported both intervention effect when user engagement was accounted for and when it wasn’t. Standardised effects were used due to outcomes and measures varying between articles. These were taken directly, where reported, otherwise calculated using guidance from Cochrane [ 33 ], and Cohen’s d formula for the standard deviation [ 34 ]. Articles were grouped by outcome domains (e.g., depression, anxiety or eating disorders) based on the reported primary clinical outcome used to evaluate efficacy. Analyses also group articles based on the analytical approach used for adjustment, those using statistical methods that retained all participants formed one group (recommend approaches) and those using statistical methods only retaining conventional per-protocol populations, i.e., exclude the data from those who did not comply, formed the other group (per-protocol approaches). All analysis was performed using Stata 17.
From a total of 6,042 articles identified, 184 were eligible and included in this review (see Fig. 1 ) randomising 43,529 participants. The most evaluated outcome domain was Depression, 74 (40.2%) articles, followed by Anxiety, 29 (15.8%) articles, and PTSD, 12 (6.5%) articles, see supplementary table S3 for full list. At least 123 unique interventions were assessed, however some interventions ( n = 39) were only described in general terms, such as internet delivered cognitive behaviour therapy for depression, so could not be distinguished as separate interventions and are excluded from the count. On average 30.7 (SD 7.7) articles were published each year, a more detailed breakdown by outcome domain is in supplementary figures s1 and s2 .
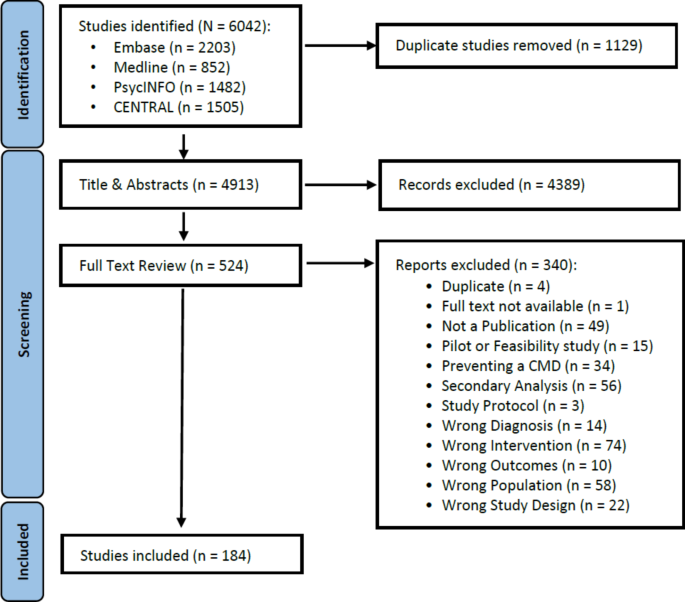
PRISMA flowchart for studies included in the systematic review
Extracted CONSORT items assessed trial reporting quality, 51 articles (27.7%) did not report their planned sample size and 36 articles (19.7%) did not clearly reference a trial protocol or trial registration number. For the 133 articles that reported both the planned and actual sample size, 43 (32.3%) failed to recruit to their target. The planned analysis approach was reportedly changed in 3 (1.6%) articles, one due to changes in the intervention [ 35 ] and the others due to high attrition [ 36 , 37 ].
Most articles used “traditional” trial designs with 170 (92.4%) opting for a parallel arm design and the majority assessed only one new intervention ( n = 134, 78.8%). Four articles (2.2%) used a factorial design allowing for the simultaneous evaluation of multiple treatments providing statistical efficiency by reducing the number of participants required in the trial. Two articles (1.1%) in the Body Dysmorphic Disorder outcome domain reported using a crossover design. However, the first had no wash-out period and instead those in the intervention arm were asked to stop engaging with the app after 16 days [ 38 ]. The second actually used a parallel arm design, where the control group received the intervention after 3 weeks [ 39 ]. Median delivery period for DMHIs was 56 days (IQR 42–84) post-randomisation and the median total follow-up time for primary outcome collection was 183 days post-randomisation (IQR 84–365).
Participants average age was 34.1 years (SD 11.1), and most participants were female (70.7%), see Table 1 . Ethnicity data was not extractable in 133 (72.3%) articles. Most trials required a confirmed diagnosis of a CMD, such as through a structured interview, for inclusion ( n = 110, 59.8%). Symptom severity could not be extracted in 97 (52.7%) trials, but where available the most common (49 trials, 56.3%) severity was a combination of both mild and moderate. Only 12 (6.5%) articles assessed participants with severe symptomatology in the depression domain ( n = 7, 58.3%), anxiety ( n = 1, 8.3%), psychological distress ( n = 1, 8.3%), general fatigue ( n = 1, 8.3%), post-traumatic stress disorder ( n = 1, 8.3%), or psychosis ( n = 1, 8.3%).
Most interventions were delivered through a website, 145 (78.8%), see Table 2 . There were 76 (41.3%) trials that adapted interventions from existing in-person therapist led interventions, and 84 (45.7%) interventions were newly developed. App delivered interventions were more likely to be newly developed, 23 (71.9%), compared to website interventions, 57 (39.3%). Most common choice of control arm was usual care, 126 (68.5%). For articles with usual care as control, most opted to use wait-lists, 94 (74.6%), where intervention access was provided either immediately after the intervention period, 62/94 (66.0%), or after the total follow-up period, 32/94 (34.0%).
Most articles, 136 (73.9%), reported using at least one approach to encourage participants to engage with the intervention. Methods of encouragement were automatic notifications, n = 49/136 (32.5%), contacting participants by telephone or email, n = 68/136 (45.0%), or automated feedback on homework exercises, n = 76/136 (50.3%). Most used only one method of encouragement, n = 85 (62.5%), with 6 (4.4%) articles using all 3 methods of encouragement. Although many articles encouraged engagement, only 23.9% ( n = 44) provided a recommended level of engagement to participants. Recommendations varied from using a rate to progress through content (e.g., one module per week or maximum of two modules per week), a specified duration to use the intervention (e.g., 1.5 h per week or 4 to 6 h per week), or specifying milestones to complete (e.g., complete one lesson every 1–2 weeks or complete daily homework assignments), a full list is in table s5 of the supplementary material.
User engagement data captured through indicators was reported in many articles, 76.1% ( n = 140), Fig. 2 . Typically, this included only reporting only one indicator ( n = 41, 29.3%) ranging up to eight indicators for one (0.7%) trial [ 40 ]. Across the 140 studies reporting user engagement data, most commonly indicators described the frequency of use, 150 (40.7%), followed by indicators to capture milestones achieved, 124 (33.6%), further detail is found in table s4 of the supplemental. A total of 150 unique indicators were reported across the 140 articles, the most popular measure used was modules completed, 51.3% ( n = 77), followed by the number of logins, 25.3% ( n = 38). In website only delivered interventions there were 102 unique indicators compared to 41 unique indicators reported in app-based interventions, and 7 unique indicators in interventions delivered as both an app and website.
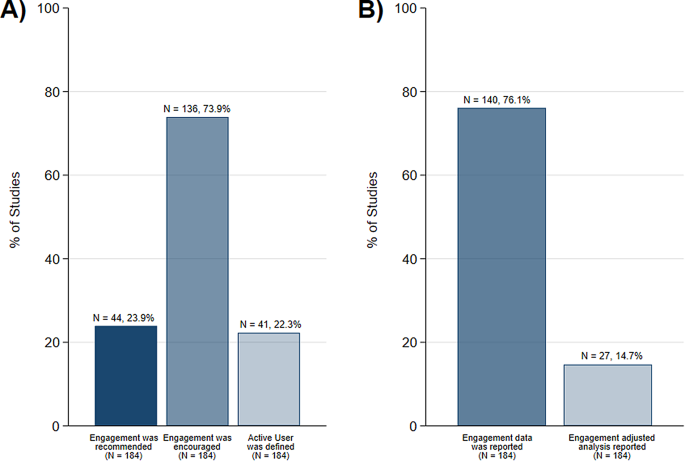
Proportion of trials describing user engagement in methods section ( A ) or in results section ( B )
A) – How user engagement was reported in the methods section
Recommended – the participant was told how to use the intervention by the study team
Encouraged – reminders (e.g., notifications or emails) were sent to the participant
Active User – participants meeting a pre-specified engagement level set by the study team
B) – How user engagement data was reported in the results section
Reported – results describe activity for at least one engagement indicator
Analysis – results report an intervention effect where user engagement has been considered
Active user definitions, the engagement level of most interest to trial teams, was stated in the methods sections for 20.1% ( n = 37) of articles. Digital components of active user definitions included setting a minimum number of modules completed (e.g., 4 out of 5 modules), a proportion of content accessed (e.g., at least 25% of pages viewed), or the total time accessed (e.g., used app for 30 min per week), a full list of active user definitions is in table s6 of the supplemental. From the 37 articles reporting active user definitions, 27 (14.7%) described statistical methods to perform an analysis accounting for user engagement but only 19 (10.3%) reported intervention effect estimates.
All articles reporting effects from the analysis accounting for user engagement also reported effects not accounting for engagement so were included a meta-analysis, Table 3 . All articles used a treatment policy estimand (including all participants randomised regardless of the level of user engagement) for their primary outcome, where user engagement was not accounted for. In articles reporting an analysis accounting for user engagement, all outcome domains reported an increase in overall effect size favouring the intervention in comparison to estimates from analysis not accounting for user engagement. The largest increase in intervention efficacy was in the distress domain ( n = 1) where the standardised mean effect size increased from − 0.61 (95% CI -0.86 to -0.36) to -0.88 (95% CI -1.17 to -0.59).
The results comparing changes in the intervention effect by the analysis approach used (recommended versus per-protocol) is in Table 4 . From the 19 articles included in the analysis, 17 (89.5%) used a conventional per-protocol (i.e., exclude the data from those who did not comply) approach for the analysis accounting for user engagement [ 41 ]. A consequence of which is that the average sample size decreased to 76.9% (IQR 67.7–87.6%) of the original size, in the active arm the average size decreased by 61.8% (IQR 38.1–75.4%). The overall standardised intervention effect increased from − 0.14 (95% CI -0.24 to -0.03, n = 17), p = .01, to -0.18 (95% CI -0.32 to -0.04, n = 17), p = .01, but was also less precise. Two trials used a Complier Average Causal Effect (CACE) analysis [ 42 ], a recommended approach where assumptions hold, with all participants randomised included in the analysis. The overall standardised intervention effect increased in the meta-analysis with an overall change from − 0.16 (95% CI -0.38 to 0.06, n = 2), p = .16, to -0.19 (95%CI -0.42 to 0.03, n = 2), p = .09, with no decrease in sample size and slightly less impact on the precision of the estimate.
This systematic review found that in trials of DMHIs for CMDs, promisingly many articles reported user engagement as summaries of automatically captured indicators, but the reported intervention effect rarely accounted for this. Overall, trials were not well reported, almost 30% did not reference a trial protocol and only 27% of articles had available data on ethnicity. The JLA patient priority group set user engagement as a research priority in 2018 and this review, including publications between 2016 and 2021, supports evidence that engagement data has been poorly utilised where only 10% ( n = 19) of articles had available estimates to evaluate the impact of user engagement on intervention efficacy. Many (> 70%) articles reported summarised engagement data highlighting plenty of opportunities to better utilise this data and understand the relationship between user engagement and efficacy, a question of particular interest to the individual using DMHIs to know the true intervention efficacy.
Many articles reported at least one method used to encourage participants to engage with the intervention, however very few articles were able to specify what the recommended level of engagement should be for individuals. Additionally, only a small proportion of trials assessed the impact of user engagement on the intervention efficacy through active user definitions, but these were broad ranging and used a variety of different engagement indicators. This highlights the complex and challenging task to properly assess user engagement where currently there is little guidance available. This also shows how difficult it is for researchers to identify what the minimum required engagement to the intervention, active user definitions, should be due to the heterogeneity in both the individuals being treated and how the intervention is being delivered (e.g., timeliness and access to other support).
Most articles performing an analysis that accounted for engagement used a conventional per-protocol population. Although the per-protocol population can be unbiased under the strong assumption that user engagement is independent from treatment allocation [ 43 ], typically use of this population causes bias in the estimated intervention effect [ 44 ] and the underlying estimand cannot be determined, i.e. unclear precisely what is being estimated. User engagement is a post-randomisation variable and the estimand framework [ 22 ] suggests using more appropriate strategies for handling post-randomisation events. For example, conducting a complier average causal effect analysis [ 42 ] under the principal stratification strategy estimated using instrumental variable regression [ 45 ] with randomised treatment allocation used as the instrumental variable. Alternative statistical methods can also be used to implement the estimand framework [ 46 ], but due to large variation in the reported engagement indicators and therefore difficulties in how engagement as a post-randomisation variable should be defined comparisons between trials remain challenging.
Establishing better methods in how user groups are defined, based on all available engagement measures, for example by using clustering algorithms combining all engagement measures, are needed. Secondly, once groups are defined existing statistical methods available to implement the estimand framework need to be assessed to determine the optimal approach to analyse the impact of engagement on the efficacy analysis. This is now the focus of our future work.
Future implications
The JLA priority setting partnership occurred in 2018, meaning this review of publications between 2016 and 2021, includes very few trials recruiting after 2018. Therefore, implementation of the JLA priorities cannot be assessed. However, this review has shown user engagement data was available, showing potential for more trials to explore engagement in efficacy analysis. An update of this systematic review should be performed for the next 5 years (2021–2026) to assess whether issues identified in this review around user engagement have been improved. More trials exploring engagement in efficacy analysis will mean the pathway of sustained behaviour changes through engagement with DMHIs is better understood. Additionally, reporting of user engagement varied greatly, and although the CONSORT extension of e-health [ 47 ] outlines some detail on engagement reporting, more directed guidance is needed. Improvements should include reporting what and how many indicators were available and better guidance on how indicator data should be summarised. Additionally, trial publications varied greatly in quality of reported results and particularly for key demographic information such as ethnicity. CONSORT trial reporting guidance has been around since 1996 and more journals should enforce its implemented to ensure robust reporting of trials.
Finally, where data was available, participants were mostly female, white ethnicity and young, demographics consistent with another systematic review of DMHI trials [ 48 ] and the most recent 2014 Adult Psychiatric Morbidity Survey (APMS) for who is most likely to receive treatment [ 49 ]. However, the APMS 2014 also shows that individuals from black or mixed ethnicities are more likely to experience a CMD than those from white ethnicities. This supports other literature [ 50 , 51 ] and highlights differences in those recruited into trials and those who experience a CMD and not represented in DMHI efficacy estimates.
Strengths and limitations of the review
This systematic review assessed a wide-ranging number of outcome domains, providing an overview for all current DMHIs evaluated, including articles from CMDs with lots of active research, such as anxiety and depression, to CMDs with very few published results. Additionally, this review collected detailed information on engagement indicators, how these were reported, and how they were utilised in the analysis of the intervention effect, providing a rich database of the typical indicators available across a wide range of DMHIs.
As the focus of this review was to assess user engagement the review does not analyse the temporal differences of when primary outcome data for the intervention effect were collected. This means the review ignores that differences of the intervention effects across articles could partly be due to temporal differences in when they were collected, assuming the intervention effect changes over time. However, comparisons of adjusted and unadjusted intervention effects are measured at the same timepoints within each article. Additionally, as very few studies reported analysis adjusted for user engagement there was limited data to assess the impact of user engagement on the intervention efficacy in most outcome domains. Further, as most studies assessing engagement used a similar approach, per-protocol population, a formal comparison of methods was not possible. Finally, as this review only focused on appraising how engagement was reported and statistical methods used to analyse engagement, we don’t consider the impact of loss to follow-up has on the efficacy of interventions but must acknowledge that DMHIs typically have high drop-out rates from studies with very low proportions of individuals completing the intervention [ 52 ].
This review assessed reporting of user engagement and how authors considered engagement in the efficacy analysis of digital mental health interventions. While many articles reported at least one measure of engagement, very few articles used the data to analyse how engagement affects intervention efficacy, making it difficult to draw conclusions on the impact of engagement. In the small proportion of articles that reported this analysis, nearly all used statistical methods at high risk of bias. There is a clear need to improve the methods used to define active users by using all available engagement measures. This will help ensure a more consistent approach to how user engagement as a post-randomisation variable is defined. Once these methods are established trialists can then utilise existing statistical methods to target alternative estimands, such as principal stratification, that mean the impact of user engagement with the intervention efficacy can be explored.
Data availability
The study protocol is already available on Prospero (CRD42021249503), datasets used are available from the corresponding author on reasonable request after the NIHR fellowship from which this project comes from is completed (April 2025). Any researchers interested in using the data extracted can contact the lead author using the shared correspondence information.
England N. The Five Year Forward View For Mental Health. 82NHS, NHS England, 2016.
Henderson C, Evans-Lacko S, Thornicroft G. Mental Illness Stigma, help seeking, and Public Health Programs. Am J Public Health. 2013;103:777–80.
Article PubMed PubMed Central Google Scholar
Muñoz RF, et al. Massive Open Online interventions: a Novel Model for delivering behavioral-health services Worldwide. Clin Psychol Sci. 2015;4:194–205.
Article Google Scholar
Ferwerda M, et al. What patients think about E-health: patients’ perspective on internet-based cognitive behavioral treatment for patients with rheumatoid arthritis and psoriasis. 2013.
Koh J, Tng GA-O, Hartanto A. Potential and Pitfalls of Mobile Mental Health Apps in traditional treatment: an Umbrella Review. LID – https://doi.org/10.3390/jpm12091376 LID – 1376. J Personalized Med. 2022;12.
Torous J, et al. Towards a consensus around standards for smartphone apps and digital mental health. World Psychiatry. 2019;18:97–8.
Capital FC. NICE and MHRA will review regulation of digital mental health tools. Volume 2023. Future Care Capital Website; 2022.
Torous J, Haim A. Dichotomies in the Development and Implementation of Digital Mental Health Tools. 2018.
NICE. NICE Evidience Standards For Digital Health. NICE, https://www.nice.org.uk/ , 2019.
Koneska EA-O, Appelbe DA-O, Williamson PA-O, Dodd SA. -O. usage Metrics of web-based interventions evaluated in Randomized controlled trials. Systematic Review; 2020.
Patel SA-O, et al. The Acceptability and Usability of Digital Health Interventions for Adults With Depression, Anxiety, and Somatoform Disorders: Qualitative Systematic Review and Meta-Synthesis. 2020.
Hollis C, et al. Identifying research priorities for digital technology in mental health care: results of the James Lind Alliance Priority setting Partnership. Lancet Psychiatry. 2018;5:845–54.
Article PubMed Google Scholar
Torous JB, et al. A hierarchical Framework for evaluation and informed decision making regarding smartphone apps for Clinical Care. Technol Mental Health. 2018;69:498–500.
Google Scholar
Donker T, et al. Smartphones for smarter delivery of mental health programs: a systematic review. 2013.
Torous J, Nicholas J, Larsen ME, Firth J, Christensen H. Clinical review of user engagement with mental health smartphone apps: evidence, theory and improvements. Evid Based Mental Health. 2018;21:116.
Doherty K, Doherty G. Engagement in HCI: Conception, Theory and Measurement. ACM Comput Surv. 2018;51:99.
Lipschitz J, et al. Adoption of mobile apps for depression and Anxiety: cross-sectional survey study on patient interest and barriers to Engagement. JMIR Ment Health. 2019;6:e11334.
Michie SA-O, Yardley LA-OX, West RA-O, Patrick KA-O, Greaves FA-O. Developing and Evaluating Digital Interventions to Promote Behavior Change in Health and Health Care: Recommendations Resulting From an International Workshop. Journal of Medical Internet Research. 2017;19.
Haine-Schlagel R, Walsh NE. A review of parent participation engagement in child and family mental health treatment. Clin Child Fam Psychol Rev 2015.
Saleem MA-O et al. Understanding Engagement strategies in Digital Interventions for Mental Health Promotion: scoping review. JMIR Mental Health. 2021;8.
Perski O, Blandford A, West R, Michie S. Conceptualising engagement with digital behaviour change interventions: a systematic review using principles from critical interpretive synthesis. Transl Behav Med. 2017;7:254–67.
Use C. f.M.P.f.H. ICH E9 (R1) addendum on estimands and sensitivity analysis in clinical trials to the guideline on statistical principles for clinical trials. European Medicines Agency; 2020.
Eysenbach G. The law of attrition. J Med Internet Res. 2005;7.
Elkes J. A systematic review to evaluate how user engagement is described and analysed in randomised controlled trials for digital mental health interventions. PROSPERO Int Prospective Register Syst Reviews. 2021.
Eldridge SM, et al. Defining feasibility and Pilot studies in Preparation for Randomised controlled trials: development of a conceptual Framework. PLoS ONE. 2016;11:e0150205.
Glanville J, et al. Translating the Cochrane EMBASE RCT filter from the Ovid interface to Embase.com: a case study. Health Inform Libr J. 2019;36:264–77.
Cochrane. Glossary of Cochrane Common Mental Disorders. Vol. 2021 A glossary of the definitions of common mental disorders. 2021.
World Health O. Classification of digital health interventions v1.0: a shared language to describe the uses of digital technology for health. Geneva: World Health Organization; 2018.
Ayiku L, et al. The NICE MEDLINE and Embase (Ovid) health apps search filters: development of validated filters to retrieve evidence about health apps. Int J Technol Assess Health Care. 2021;37:e16.
Innovation VH. Covidence systematic review software, Veritas Health Innovation, Melbourne, Australia. www.covidence.org . 2022.
Murad MH, Wang Z. Guidelines for reporting meta-epidemiological methodology research. Evid Based Med. 2017;22:139.
Schulz KF, Altman DG, Moher D. CONSORT 2010 Statement: updated guidelines for reporting parallel group randomised trials. BMJ. 2010;340:c332.
Cochrane. Cochrane Handbook for Systematic Reviews of Interventions. Cochrane. 2023.
Larry V, Hedges, Olkin I. Statistical methods for meta-analysis. 2014.
Fitzsimmons-Craft EE, et al. Effectiveness of a Digital Cognitive Behavior Therapy-guided self-help intervention for eating disorders in College Women. A Cluster Randomized Clinical Trial; 2020.
Salamanca-Sanabria AA-O, et al. A culturally adapted cognitive behavioral internet-delivered intervention for depressive symptoms. Randomized Controlled Trial; 2020.
Richards D, et al. Effectiveness of an internet-delivered intervention for generalized anxiety disorder in routine care: A randomised controlled trial in a student population. 2016.
Cerea S, et al. Cognitive behavioral training using a Mobile Application reduces body image-related symptoms in high-risk Female University students: a randomized controlled study. Behav Ther. 2021;52:170–82.
Glashouwer KA, Neimeijer RAM, de Koning ML, Vestjens M, Martijn C. Evaluative conditioning as a body image intervention for adolescents with eating disorders. 2018.
Milgrom JA-O, et al. Internet Cognitive Behavioral Therapy for Women With Postnatal Depression: A Randomized Controlled Trial of MumMoodBooster. 2016.
Hernán MA, Hernández-Díaz S. Beyond the intention-to-treat in comparative effectiveness research. Clin Trails. 2011;9:48–55.
Dunn G, Maracy M, Fau - Tomenson B, Tomenson B. Estimating treatment effects from randomized clinical trials with noncompliance and loss to follow-up: the role of instrumental variable methods. 2005.
Kahan BC, White IR, Edwards M, Harhay MO. Using modified intention-to-treat as a principal stratum estimator for failure to initiate treatment. Clin Trails. 2023;20:269–75.
Ranganathan P, Pramesh CS, Aggarwal R. Common pitfalls in statistical analysis: Intention-to-treat versus per-protocol analysis. 2016.
Lipkovich I, et al. Using principal stratification in analysis of clinical trials. Stat Med. 2022;41:3837–77.
Parra CO, Rhian M, Daniel, Bartlett JW. Hypothetical estimands in clinical trials: a unification of causal inference and missing data methods. Arxiv - Stat Methodol. 2021.
Eysenbach G, Group C-E. CONSORT-EHEALTH: improving and standardizing evaluation reports of web-based and mobile health interventions. J Med Internet Res. 2011;13:e126.
Sin J, et al. Digital Interventions for Screening and Treating Common Mental disorders or symptoms of Common Mental illness in adults: systematic review and Meta-analysis. J Med Internet Res. 2020;22:e20581.
McManus S, Jenkins BP, Brugha R T, editors. Mental health and wellbeing in England: Adult Psychiatric Morbidity Survey 2014. in Adult Psychiatric Morbidity Survey 405 (Leeds, NHS Digital) 2016.
Iflaifel M, et al. Widening participation - recruitment methods in mental health randomised controlled trials: a qualitative study. 2023.
Coss NA, et al. Does clinical research account for diversity in deploying digital health technologies? Npj Digit Med. 2023;6:187.
Karyotaki E, et al. Predictors of treatment dropout in self-guided web-based interventions for depression: an ‘individual patient data’ meta-analysis. Psychol Med. 2015;45:2717–26.
Article CAS PubMed Google Scholar
Download references
Acknowledgements
This work was funded by the NIHR Doctoral Fellowship (NIHR301810). The views expressed are those of the author(s) and not necessarily those of the NIHR or the Department of Health and Social Care. The funder had no role in study design, data collection, data analysis, data interpretation, or writing of the report. The TMRP Health Informatics working group, which the lead author is a member of, was essential in finding members (SOC, LY, LB) to join this project and support the work.
This work was funded by the NIHR Doctoral Fellowship (NIHR301810).
Author information
Jacqueline Sin and Victoria Cornelius contributed equally to this work.
Authors and Affiliations
Imperial Clinical Trials Unit, Imperial College London, White City Campus, Stadium House, 68 Wood Lane, London, W12 7RH, UK
Jack Elkes, Suzie Cro & Victoria Cornelius
University of Oxford, Oxford, UK
Rachel Batchelor, Ly-Mee Yu & Victoria Harris
Florence Nightingale Faculty of Nursing, Midwifery and Palliative Care, King’s College London, London, UK
Siobhan O’Connor
Leeds Institute of Clinical Trials Research, University of Leeds, Leeds, LS2 9JT, UK
Lauren Bell
City St Geroge’s, University of London, London, UK
Jacqueline Sin
You can also search for this author in PubMed Google Scholar
Contributions
Conceptualization, JE, VC, SC and JS.; Methodology, JE, VC, SC and JS.; Software, JE.; Validation, JE, VC, SC, JS, SO, LB, RB, LY, and VH.; Formal Analysis, JE.; Investigation, JE, VC, SC, JS, SO, LB, RB, LY, and VH.; Resources, JE, VC, SC, and JS.; Data Curation, JE.; Writing – Original Draft, JE, VC, SC, and JS.; Writing – Reviewing & Editing, JE, VC, SC, JS, SO, LB, RB, LY, and VH.; Visualisation, JE, VC, SC, and JS.; Supervision, VC, SC, and JS.; Project Administration, JE, VC, and SC.; Funding Acquisition, JE, VC, SC, and JS.
Corresponding author
Correspondence to Jack Elkes .
Ethics declarations
Ethics approval and consent to participate.
Not applicable as all data was publicly available.
Consent for publication
Not applicable as no participants were recruited for this research.
Competing interests
JE was recently a collaborator on a NIHR HTA grant (NIHR132896) for long term effectiveness of a video feedback intervention for parents. JE is also on the trial steering committee for a trial (NIHR302349) that is part of an NIHR Doctoral Fellowship called Restore-B. JE is also on the programme steering committee (NIHR204413) for a trial called ATTEND. SC was previously awarded funding for an NIHR advanced fellowship (NIHR300593) between Stepember 2020 and December 2023. VC was also involved in the NIHR HTA (NIHR132896) funded trial of long-term follow-up of the video feedback intervention for parents. VC is also on the trial steering committee for a problem solving intervention for adults with dementia and depression, a steering committee member for a trial called ADVANCE and the chair of a NIHR HTA funded data monitoring committee (NIHR132808) called BAY. No other competing interests are reported for all other authors (RB, SOC, LMY, LB, VH and JS).
Additional information
Publisher’s note.
Springer Nature remains neutral with regard to jurisdictional claims in published maps and institutional affiliations.
Electronic supplementary material
Below is the link to the electronic supplementary material.
Supplementary Material 1
Rights and permissions.
Open Access This article is licensed under a Creative Commons Attribution 4.0 International License, which permits use, sharing, adaptation, distribution and reproduction in any medium or format, as long as you give appropriate credit to the original author(s) and the source, provide a link to the Creative Commons licence, and indicate if changes were made. The images or other third party material in this article are included in the article’s Creative Commons licence, unless indicated otherwise in a credit line to the material. If material is not included in the article’s Creative Commons licence and your intended use is not permitted by statutory regulation or exceeds the permitted use, you will need to obtain permission directly from the copyright holder. To view a copy of this licence, visit http://creativecommons.org/licenses/by/4.0/ . The Creative Commons Public Domain Dedication waiver ( http://creativecommons.org/publicdomain/zero/1.0/ ) applies to the data made available in this article, unless otherwise stated in a credit line to the data.
Reprints and permissions
About this article
Cite this article.
Elkes, J., Cro, S., Batchelor, R. et al. User engagement in clinical trials of digital mental health interventions: a systematic review. BMC Med Res Methodol 24 , 184 (2024). https://doi.org/10.1186/s12874-024-02308-0
Download citation
Received : 02 May 2024
Accepted : 14 August 2024
Published : 24 August 2024
DOI : https://doi.org/10.1186/s12874-024-02308-0
Share this article
Anyone you share the following link with will be able to read this content:
Sorry, a shareable link is not currently available for this article.
Provided by the Springer Nature SharedIt content-sharing initiative
- Randomised controlled trials
- Digital mental health interventions
- Mental health
- User engagement
- Digital health
- Systematic review
- Meta-analysis
BMC Medical Research Methodology
ISSN: 1471-2288
- General enquiries: [email protected]

An official website of the United States government
The .gov means it’s official. Federal government websites often end in .gov or .mil. Before sharing sensitive information, make sure you’re on a federal government site.
The site is secure. The https:// ensures that you are connecting to the official website and that any information you provide is encrypted and transmitted securely.
- Publications
- Account settings
Preview improvements coming to the PMC website in October 2024. Learn More or Try it out now .
- Advanced Search
- Journal List
- J Public Health Res
- v.4(1); 2015 Feb 20

School-Based Diabetes Interventions and Their Outcomes: A Systematic Literature Review
Contributions: BP, conception and design of the systematic literature review, search strategy, screening and selection of the articles, analysis and interpretation of data, drafting of the article; PJS, critical evaluation of the article.
Type 1 diabetes is one of the most common chronic childhood diseases, while type 2 diabetes in children is increasing at alarming rates globally. Against this backdrop, the school is a critical environment for children with diabetes. They continue to face barriers to education that may lead to depression, poor academic performance, and poor quality of life. To address these challenges, diabetes interventions have been implemented in school and the goal was to systematically review these interventions and their outcomes between 2000 and 2013. Fifteen studies were included in the narrative synthesis. Education of school personnel was the main focus before 2006. Studies reported gains in knowledge and perceived confidence of school staff. Since 2006, more comprehensive interventions have been developed to promote better care coordination and create a safe school environment. These studies reported improved diabetes management and quality of life of students. Assessment tools varied and study design included randomized controlled trials, quantitative and qualitative methods. Although many of the studies reported a significant difference in the parameters measured, it was not possible to determine optimal ways to improve the health, quality of life and academic performance of children with diabetes, given the disparity in scope, assessment tools and measured outcomes. Experimental designs, longer follow-up studies, larger sample sizes, and a higher number of participating schools are critical issues to consider in future studies. Most of the research was conducted in North America and further research is needed in other parts of the world.
Significance for public health
Diabetes is one of the most common chronic childhood diseases; both type 1 and type 2 diabetes are increasing in children globally. Against this backdrop, the school is a critical environment for children with diabetes. This systematic literature review on school-based diabetes interventions and their outcomes demonstrates that increasing efforts are being made to improve diabetes care and create a safe school environment. Studies reported gains in knowledge and confidence of school staff, as well as improved health and quality of life of students. Given the disparity of the assessment tools used, it was not possible to determine optimal ways to improve the health, quality of life and academic performance of children with diabetes. Future evaluations should include experimental designs, longer follow-up studies, and larger sample sizes. School-based diabetes interventions and solid evaluations will contribute to improving diabetes school policies and ensuring children with diabetes have the same educational opportunities as other children.
Introduction
Type 1 diabetes is one of the most common chronic childhood diseases, affecting an estimated 497,100 children under 15 years globally. 1 The incidence of type 1 diabetes among children is increasing in many countries, and more than 79,000 children under 15 years are estimated to develop type 1 diabetes annually worldwide. 1-3 Young people today also face another danger that threatens their ability to attain a healthy and successful future as evidence shows that type 2 diabetes is increasing in children and adolescents around the world at alarming rates. 1 , 4-10 With rising levels of childhood obesity and physical inactivity in many countries, type 2 diabetes in childhood has the potential to become a global public health issue leading to serious health outcomes and a significant burden on the family and society.
Children with diabetes spend between 6 and 10 hours a day in school and doing school-related activities. Diabetes management requires intensive resources; blood glucose monitoring, insulin administration and the treatment of low blood glucose are essential for children with diabetes during the school day, and the need for assistance varies across age groups. As a result, the school system is a critical environment for children with diabetes. 11-18
Position statements and guidelines for diabetes in children and adolescents issued by diabetes organizations call for all children with diabetes to have the right to manage their diabetes without being excluded or discriminated against in school, and the right to participate fully and safely in all school activities. 19-21 Resources have been made available to improve the management of diabetes at school. 22-27 However, many school children with diabetes continue to face barriers to education and the effective management of their disease in school. The main obstacles include a lack of informed and trained staff, poor or limited knowledge of, and misconceptions about diabetes, a lack of equipment and communication, the absence of a school nurse on site daily, and a lack of diabetes management policies. 15 , 17 , 28-45
The literature has shown that children with diabetes are still confronted with many challenges and issues in school: they may have a limited ability to monitor and treat blood glucose levels and be at risk of diabetes complications; they may be denied access to school and extracurricular activities; they may face a lack of support or stigma and discrimination; they may hide their condition and feel that they are treated differently in school because of their diabetes. All of these factors are leading to absenteeism, depression, stress, poor academic performance and poor quality of life. 13 , 15 , 18 , 28 , 35 , 38 , 40 , 41 , 44-46 School-based diabetes interventions become critical to improve support, increase knowledge and confidence, protect against discrimination, and ensure a safe environment for children with diabetes.
In response to the rising obesity trends in children and adolescents, innovative multi-faceted school-based diabetes prevention programs have been implemented, such as the HEALTHY study, 47 Bienestar school-based diabetes mellitus prevention programmes, 48 the Jump into Action school-based diabetes prevention programme, 49 the medical education for children/adolescents for realistic prevention of obesity and diabetes intervention study, 50 and the NDKK-funded NEEMA school-based diabetes risk prevention programme. 51 In the past few years, several literature reviews have been conducted to review existing school-based prevention interventions focusing on type 2 diabetes risk factors and promoting healthy lifestyles. 52-58
Focusing on the impact of school-based diabetes interventions, a systematic literature review, which was conducted in 2002, assessed the effectiveness of diabetes education for school personnel and concluded that the literature was very scant, the methodology was inadequate and results were mixed. 38 The review concluded that further research was needed to define effective diabetes interventions for school personnel. Two additional integrative reviews focused only on type 1 diabetes management in the school setting. 17 , 45 They identified gaps for effective diabetes management and areas for improvement in communication, education of staff and peers, and school nurse availability.
It is time again to review the literature about school-based diabetes interventions and their outcomes over the past decade (2000-2013). In the early 2000s’, the management of diabetes considerably changed, and ever since, technological advances have grown: children have started to use improved blood glucose meters, insulin pumps that deliver rapid-acting insulin and pen devices. 42 , 45 In addition, both the UN Resolution on World Diabetes Day in December 2006 and the UN Political Declaration of the High-level Meeting of the General Assembly on the Prevention and Control of Non-communicable Diseases in September 2011 have put diabetes on the global health agenda. 59 , 60 On the basis of the findings of earlier studies as well as recent policy developments, we have formulated two research questions. The first one is: what school-based diabetes interventions have been implemented since 2000?
According to the literature, the evaluation of health interventions is essential for two main reasons: i) improving programmes; and ii) improving policy. 61 , 62 Evaluation may help improve programmes and their outcomes by adjusting programme content, identifying the best strategies for increasing participation and adherence, addressing problems and identifying the most effective methods. Evaluation may also help advocate for the programme and mobilize health authorities’ support to implement policies and trigger action. This leads to the formulation of the second research question: what were the outcomes of these school-based diabetes interventions?
The present systematic literature review aims to provide a comprehensive overview of school-based diabetes interventions and their outcomes between 2000 and 2013. The ultimate goal is to determine effective school-based interventions to enhance the health, quality of life and academic results of children with diabetes.
Search strategy and information sources
Using multiple electronic databases including PubMed, CINAHL, PsychInfo and the Psychology and Behavioral Sciences Collection, we conducted a systematic literature review of articles written in English and published in peer-reviewed journals between 2000 and 2013. The databases were searched using the following key words in titles or abstracts: diabetes and school and intervention, campaign, program, project or promotion , as well as the following Medical Subject Headings (Mesh) terms: diabetes mellitus combined with schools and health campaign or health education.
Titles and abstracts of articles extracted by this search were reviewed for relevance and, if potentially relevant, the full-text article was retrieved and reviewed. Only full articles of original studies were considered for final inclusion. We also conducted a manual search by reviewing the reference lists of relevant articles. However, this did not result in additional studies eligible for inclusion.
Inclusion criteria
The inclusion criteria for the articles reviewed were the following: i) primary studies; ii) published in English; iii) between 2000 and 2013; iv) a school-based intervention included; and v) intervention focusing on diabetes.
Descriptive studies focusing on the needs of school children with diabetes or identifying the gaps in diabetes care in school were excluded if there had not been an intervention. They were, however, used to provide baseline information in the introduction. Studies focusing on children with diabetes outside the school, such as summer camps or paediatric centres, were also excluded. Studies focusing on type 2 diabetes prevention, with interventions comprised of nutrition and exercise components and evaluation measured by food choices, physical activity or anthropometric characteristics were beyond the scope of this review.
Study selection
We followed the PRISMA Statement to conduct the study selection (Preferred Reporting Items for Systematic Reviews and Meta-Analyses). 63 All references were downloaded to RefWorks and duplicates were automatically removed. The first investigator checked all titles and abstracts of references generated by the extensive search for relevance. A second screening was undertaken based on the full text analysis to select eligible studies. Two independent coders reviewed the full articles to ensure the validity of the review. Ten articles were subject to discussion and were thereby resolved.
Data collection process and data items
The following data for each study has been taken into consideration: date and country of study, intervention (objectives, design, duration, theory-grounded) and evaluation (study population, study design, measurement tools, scales, indicators, results), implications and limitations. The results are summarized below using narrative synthesis. 64
As can be seen in the PRISMA 2009 Flow Diagram in Figure 1 , 63 the database search resulted in 1473 records; 246 duplicates were removed by the RefWorks automatic duplicates tool; 1227 titles and abstracts were reviewed and 1107 records were removed as they did not meet the inclusion criteria. Out of the 120 full-text articles reviewed, a total of 15 full papers that met all criteria were included in the final study selection. The breakdown of the full-text article screening (n=120) is the following: i) 15 articles were eligible studies; ii) 15 were baseline studies with no intervention focusing on diabetes knowledge and the needs of children with diabetes in school; iii) 34 were focusing on diabetes prevention programmes (risk factors/lifestyle interventions); iv) 17 were focusing on children with diabetes outside the school environment; v) 13 were non-scientific articles or communication statements; and vi) 26 were duplicates or irrelevant (not focusing on school children with diabetes).

PRISMA 2009 flow diagram. 63
Study characteristics
Fifteen studies were included into this systematic literature review ( Table 1 and Supplementary Table S1). 42 , 46 , 65-77 The majority of studies were conducted after 2006 (n=9), and six were conducted before 2005. All studies were undertaken in North America (n=14 in the USA and n=1 in Canada). Most of the studies lasted one year (n=7); four studies lasted between 3-5 years, while four studies lasted three months or less. The majority of studies focused on type 1 diabetes but four studies conducted after 2005 addressed both type 1 and type 2 diabetes in school.
List of papers included in our literature review.
First author | Title | Date | Country |
---|---|---|---|
Bachman | Evaluation of online education about diabetes management in the school setting | 2008 | US |
Bobo | Diabetes management at school: application of the Healthy Learner Model | 2011 | US |
Bobo | A collaborative approach to diabetes management: the choice made for Colorado schools | 2011 | US |
Bullock | Continuing education: improving perceived competence in school nurses | 2002 | US |
Engelke | School nurse case management for children with chronic illness: health, academic, and quality of life outcomes | 2008 | US |
Engelke | School nurses and children with diabetes: a descriptive study | 2011 | US |
Faro | Improving students’ diabetes management through school-based diabetes care | 2005 | US |
Husband | The effectiveness of a CD-rom in educating teachers who have a student with diabetes | 2000 | Canada |
Izquierdo | School-centered telemedicine for children with type 1 diabetes mellitus | 2009 | US |
Nguyen | Targeting blood glucose management in school improves glycemic control in children with poorly controlled type 1 diabetes mellitus | 2008 | US |
Nimsgrem | Implementing a new diabetes resource for Wisconsin schools and families | 2005 | US |
Peery | Parent and teacher perceptions of the impact of school nurse interventions on children’s self management of diabetes | 2012 | US |
Radjenovic | Computer-based remote diabetes education for school personnel | 2001 | US |
Siminerio | A diabetes education program for school personnel | 2000 | US |
Smith | Evaluation of the impact of a diabetes education curriculum for school personnel on disease knowledge and confidence in caring for students | 2012 | US |
Synthesis of results
Interventions.
The first research question was: what school-based diabetes interventions have been implemented since 2000? In order to answer this, we have considered the objectives, the target audience and the components of the interventions, as well as the theory used (Supplementary Table S1).
Two main types of intervention can be distinguished: i) studies targeting school personnel focusing on diabetes education; and ii) comprehensive studies focusing on children with diabetes aiming to improving their health, academic performance, and well-being.
Seven studies focused on diabetes education targeting school personnel, mainly school nurses or school teachers. 42 , 65 , 67 , 71 , 74 , 76 , 77 Their main objective was to increase knowledge about diabetes and confidence in caring for school children with diabetes. Interventions included diabetes education programmes, continuing education, creation of a resource for school personnel, on-line education and CD-ROM, and computer-based education. They were mainly conducted between 2000 and 2005; only two were conducted after, respectively in 2008 and 2012. 65 , 77 After 2005, eight studies proposed a more comprehensive approach to improve diabetes management in school and create a safe environment for children with diabetes. 46 , 66 , 68-70 , 72 , 73 , 75 The interventions consisted of the application of the Healthy Learner Model, a collaborative approach to diabetes care and prevention, School Nurse Case Management programmes, paediatric nurses’ school visits, school nurse supervision of diabetes management and school-centered telemedicine. They included components such as direct care, education/counselling, and care coordination, in order to improve diabetes management in school.
Nine studies were theory-based. Case Management (n=3), 68 , 69 , 75 the Healthy Learner Model (n=2) 46 , 66 and the Social Cognitive Theory (n=2) 70 , 77 served as main theoretical frameworks for the development of the study interventions. Two other theories (Roger’s theory of diffusion of innovation and Mantel and Teorey’s usability framework) 65 , 76 were also used to support interventions. None of the studies focusing on school personnel’s training before 2005 were theory-based, while the two education interventions after 2006 were theory-grounded. 65 , 77 All the comprehensive studies focusing on diabetes care in school after 2005 were theory-based, apart from two. 71 , 73
Among all interventions, the study populations can be classified into three groups: i) school personnel (n=10), comprising school nurses (n=7), teachers (n=3) or other school staff (n=2); ii) school children with diabetes (n=6); and iii) parents of children with diabetes (n=6).
The second research question was: what were the outcomes of these school-based diabetes interventions? To answer this, we have considered the study design, measurement tools used, and reported results in the studies (Supplementary Table S1). The outcomes of the interventions have been reported using multiple and diverse tools, and a combination of study designs. Six studies were assessed by randomized controlled trials (RCTs) measuring diabetes knowledge, perceived competence/confidence, Quality of Life (QoL), Haemoglobin A1c (HbA1c) levels or satisfaction. Ten studies used quantitative and qualitative methods to assess the satisfaction with the intervention, the diabetes knowledge or confidence, perceived improvement in diabetes care, health outcomes/QoL, student self-efficacy or follow-up impact. In five studies, other complementary tools such as individual goal attainment, activity logs and student academic outcomes were also used.
Eight studies used a pre/post- evaluation design and three studies were longitudinal with measurements at different points in time during the intervention. As can be seen in Supplementary Table S1, nine studies reported statistically significant improvements. Significantly higher knowledge of diabetes in school personnel has been shown by Radjenovic and Layne Wallace (P<0.033), 76 Siminerio and Koerbel (P<0.004), 42 and Smith and colleagues (P<0.001), 77 in diabetes education programmes focusing on school personnel (face-to-face or computer-based training). There is also evidence of higher self-perceived competence in school nurses who have completed continuing education (P=.0001), 67 CD-ROM based training (P=0.016), 71 or advanced training programmes (P<0.001). 77 Only in the study of the effectiveness of a CD-ROM training, the increase in knowledge, measured by a RCT, was not statistically significant. 71 In two studies focusing on children with diabetes, Engelke and colleagues reported a significant increase in QoL of children with diabetes as a result of Case Management interventions in the school setting. 68 , 69 Similarly, significant improvements in QoL were reported by Izquierdo and colleagues as outcomes of a combination of usual care and telemedicine. 72 Nguyen and colleagues showed a significant decrease in HbA1c in children with poorly controlled type 1 diabetes who were supervised by school nurses for glucose checks and insulin injection (P<0.0001). 73 Peery and colleagues also showed the perceived positive outcome by parents and teachers of school nurse interventions on children’s self-management. 75 Even though some positive trends were observed, no statistically significant differences in student with diabetes self-efficacy were seen by Faro and colleagues as a result of monthly school visits by a paediatric nurse in schools. 70 All authors who conducted satisfaction surveys reported high satisfaction rates with the programmes. 46 , 65 , 66 , 70 , 72 , 74 , 76
An increasing number of school-based diabetes interventions have been implemented in developed countries over the past 14 years (2000-2013). These interventions are varied in terms of scope, duration and measured outcomes.
All studies in this review were conducted in North America which can be explained by US federal legislation, the central role of the school nurse in diabetes management and the provision of school diabetes policies and diabetes management plans in the US. Some studies examined the outcomes of interventions on teachers’, school nurses’ and other school personnel’s diabetes knowledge and confidence in delivering care. Interventions consisted of diabetes training and education of school personnel about diabetes, including face-to-face training, continuing education, resource guides and also online education, CD-ROM or computer-based training. Most of these studies were conducted before 2006 (five out of seven studies). All studies reported overall satisfaction with the training provided. In addition, some studies used knowledge and confidence questionnaires and demonstrated gains in knowledge and perceived competence (although in one instance, the gain in knowledge was not significant). Four RCTs were conducted and showed a significant increase in nurses’ confidence. None of these studies measured the effects on practices and behaviour change. These interventions, however, showed the importance of on-going education of school personnel to stay aware of developing knowledge and new technological advances in diabetes management, and gain confidence in managing children with diabetes. 42 , 65 , 67 , 71 , 74 , 76 , 77
New comprehensive approaches focusing on the care of children with diabetes have been implemented in the school setting since 2005. Compared to the interventions described previously, these campaigns lasted longer (usually one school year, but lasting up to five years). These theory-based programmes offered an integrative and collaborative approach of care to meet the needs of the child with diabetes in school. The main goal was to offer a standardized approach to care in order to improve diabetes management and promote a safe and healthy school environment for children with diabetes. Interventions based on Case Management, telemedicine and collaborative approaches to diabetes management included a full set of services such as education, direct care, counselling, meetings, coordination and communication with the families. Most of these interventions were theory-grounded and, apart from one, 76 all theory-based interventions have been conducted since 2005. 46 , 65 , 66 , 68-70 , 75 , 77 They illustrate a positive trend in theory-based interventions, in line with the literature that has shown the benefits of theory-grounded interventions in health education and communication. 78 Evaluations involved multiple tools and indicators. In addition to satisfaction surveys about the programme, indicators such as HbA1c levels and QoL were used as these studies aimed to improve the diabetes management and well-being of students with diabetes at school. Activity/intervention logs, individualized goals, as well as students’ academic measures, were also complementary assessment tools. These studies all included a pre and post evaluation design and, in three studies, 46 , 66 , 72 a longitudinal evaluation was conducted at several points in time. RCTs were used to assess the effectiveness of two interventions and showed significant improvements in HbA1c levels as a result of school nurse supervision in diabetes care 73 or school-centered telemedicine. 72 School-centered diabetes management interventions provided evidence of the critical role of a supportive school environment to improve the health and quality of life of children with diabetes.
Limitations
Some limitations could be observed regarding the evaluation of the studies. Although many of the studies reported a significant difference in the parameters measured, it was not possible to determine optimal ways to improve the health, quality of life and academic performance of children with diabetes given the disparity in scope, assessment tools and measured outcomes. Only six studies used RCTs, and both quantitative and qualitative methods have been used. In several cases, the evaluation was limited to satisfaction surveys or perceived improved knowledge, and confidence levels. Behaviour change and practice were not assessed. Scales were not always validated. The samples and the number of schools were rather limited in most cases, making impossible to generalize results. Only one study reported a follow-up study and the long-term impact after one year was never assessed.
The large heterogeneity of school-based diabetes interventions, measurement tools and measured outcomes among the reviewed studies did not allow a statistical pooling of results. Therefore no single value can be presented to demonstrate the effectiveness of school-based diabetes interventions. Small samples, the use of non-validated scales and qualitative studies do not provide adequate data for supporting evidence on the effectiveness of school-based diabetes interventions and replicability. All studies were conducted in North America, making difficult to generalize findings to other parts of the world. Lastly, the cost-effectiveness of the interventions was never assessed and should be integrated in future evaluation.
Conclusions
This systematic literature review has shown an increase in school-based diabetes interventions since 2005 in response to the rising prevalence of diabetes in children (both type 1 and type 2 diabetes) and the higher global attention given to the diabetes burden. The education of school personnel was the main focus before 2006. Interventions aimed to address the lack of informed and trained staff, limited knowledge and misconception about diabetes, and to increase knowledge and confidence of school personnel. Interventions such as diabetes training and continuing education of school nurses and teachers have reported school personnel’s gained knowledge and improved self-perceived competence. More comprehensive approaches focusing on children with diabetes have been developed since 2005. These integrative interventions have aimed to promote better care coordination, collaboration between all care givers and the provision of a safe school environment. These studies have measured HbA1c levels and quality of life of students and, in pre/post tests, have shown significant improvements.
This review highlights the importance of conducting thorough evaluations of school-based diabetes interventions. Additional thinking about long-term outcomes that reflect the nature of the interventions, and how they can be measured best, is needed. Comprehensive evaluation of school-based diabetes projects will contribute to adjusting and improving the interventions. It will also serve as a powerful advocacy tool for improving school policies on diabetes.
This systematic literature review does not provide definitive guidance toward the optimal school-based diabetes interventions, given the large heterogeneity of the assessment tools used and limited evaluation in some cases. It does, however, demonstrate that increasing efforts are being made, and it does provide evidence that can be used for developing future school-based interventions. Experimental designs, longer follow-up studies, larger sample sizes, and higher numbers of participating schools are critical issues that should be taken account of before work is begun. Further research is needed and studies should be conducted in other parts of the world than North America in order to promote a safe school environment and ensure children with diabetes have the same educational opportunities as other children everywhere in the world.
Acknowledgments
The authors are grateful to Teresa Cafaro for her review of the full-text articles. They also thank Dr David Chaney, Dr Nanon Labrie and Sean Taylor for their critical reviews of the document.

IMAGES
VIDEO
COMMENTS
However, type 2 diabetes makes up more than 90% of diagnosed diabetes cases in the United States. 35 Thus, our findings largely reflect risk-factor treatment and control in those with type 2 diabetes.
I. Introduction. Diabetes mellitus, a metabolic disease defined by elevated fasting blood glucose levels due to insufficient insulin production, has reached epidemic proportions worldwide (World Health Organization, 2020).Type 1 and type 2 diabetes (T1D and T2D, respectively) make up the majority of diabetes cases with T1D characterized by autoimmune destruction of the insulin-producing ...
The diagnosis of incident diabetes was based on an oral glucose tolerance test (OGTT). The overall risk reduction of T2D by the lifestyle interventions was 0.53 (95% CI 0.41; 0.67). Most of the trials aimed to reduce weight, increase physical activity, and apply a diet relatively low in saturated fat and high in fiber.
It is estimated that the annual cost of diagnosed diabetes in the U.S. in 2017 was $327 billion, including $237 billion in direct health care costs and $90 billion in reduced productivity. After adjusting for inflation, the economic costs of diabetes increased by 26% from 2012 to 2017 ( 8 ).
Although nationally standardized quality measures have demonstrated improvement in diabetes care delivery, progress has been slow (1,2).The redesign of primary care delivery models provides an important focus for enhancing the quality of diabetes care provided in communities throughout the country (3,4).New models of care delivery, such as the patient-centered medical home (PCMH), promote ...
Primary Care Diabetes is the official journal of Primary Care Diabetes Europe. The journal publishes original research articles and high quality reviews in the fields of clinical care, diabetes education, nutrition, health services, psychosocial research and epidemiology and other areas as far as …. View full aims & scope.
Common ground on dietary approaches for the prevention, management, and potential remission of type 2 diabetes can be found, argue Nita G Forouhi and colleagues Dietary factors are of paramount importance in the management and prevention of type 2 diabetes. Despite progress in formulating evidence based dietary guidance, controversy and confusion remain. In this article, we examine the ...
The worldwide prevalence of type 2 diabetes mellitus (T2DM) in adults has increased from ~150 million affected people in 2000 to >450 million in 2019 and is projected to rise further to ~700 ...
Purpose of Review This article highlights foundational evidence, translation studies, and current research behind type 2 diabetes prevention efforts worldwide, with focus on high-risk populations, and whole-population approaches as catalysts to global prevention. Recent Findings Continued focus on the goals of foundational lifestyle change program trials and their global translations, and the ...
Altogether seven RCTs (N = 4090) fulfilled the eligibility criteria and were included in the meta-analysis. The diagnosis of incident diabetes was based on an oral glucose tolerance test (OGTT). The overall risk reduction of T2D by the lifestyle interventions was 0.53 (95% CI 0.41; 0.67). Most of the trials aimed to reduce weight, increase ...
Type 2 diabetes (T2D) affects an estimated 10.5% of adults in the US, 1 with increasing prevalence in younger age groups 2,3 and approximately 21% of those individuals with diabetes being undiagnosed. 1 Without adequate treatment and management, the condition can result in blindness, kidney disease, cardiovascular diseases including atherosclerosis and heart failure, and other comorbidities ...
New and emerging medical therapies and evidence have changed the landscape for managing people with type 2 diabetes (T2D) with established cardiovascular disease (CVD) and those with cardiovascular risk factors. Previously, guidelines gauged good diabetes care primarily based on glycated haemoglobin (HbA1c) targets [1], but recent updates have represented a major shift, now recommending a ...
The prevalence of diagnosed diabetes is projected to increase in the U.S. from 22.3 million (9.1% of the total population) in 2014, to 39.7 million (13%) in 2030, and to 60.6 million (17%) in 2060 ().Approximately 90-95% of those with diabetes have type 2 diabetes ().Diabetes is an expensive disease, and the medical costs of health care alone for a person with diabetes are 2.3 times more ...
The prevalence of diagnosed diabetes is projected to increase in the U.S. from 22.3 million (9.1% of the total population) in 2014, to 39.7 million (13%) in 2030, and to 60.6 million (17%) in 2060. 3 Approximately 90-95% of those with diabetes have type 2 diabetes. 4 Diabetes is an expensive disease, and the medical costs of health care alone for a person with diabetes are 2.3 times more than ...
Diabetes mellitus (DM) underscores a rising epidemic orchestrating critical socio-economic burden on countries globally. Different treatment options for the management of DM are evolving rapidly because the usual methods of treatment have not completely tackled the primary causes of the disease and are laden with critical adverse effects. Thus, this narrative review explores different ...
Diabetes and Diabetes Care publish primary research findings and have impact factors of 8.9 and 7.1, respectively, making them the highest ranking journals exclusively publishing diabetes research. The ADA scientific and medical resources are also disseminated in the community, and patient education materials that the Association develops are ...
Different classes of diabetes mellitus, type 1, type 2, gestational diabetes and other types of diabetes mellitus are compared in terms of diagnostic criteria, etiology and genetics. The molecular genetics of diabetes received extensive attention in recent years by many prominent investigators and research groups in the biomedical field.
Today, diabetes mellitus (DM) is one of the most prevalent diseases globally. The prevalence is 8.5% of the adult population, and it will likely grow every year (World Health Organization [WHO], 2016a).The International Diabetes Federation (IDF; 2017) has estimated that the rate will be 10% in 2045.Ninety percent of people with DM around the world have diabetes type 2 (IDF, 2017).
Background: Type 2 Diabetes is a metabolic disorder characterized by hyperglycemia that causes numerous complications with significant long-term morbidity and mortality. The disorder is primarily due to insulin resistance particularly in liver, skeletal muscle, and adipose tissue. In this review, we detail the hormonal mechanisms leading to the development of diabetes and discuss whether ...
Background Pre-diabetes affects one-third of US adults and increases the risk of type 2 diabetes. Effective evidence-based interventions, such as the Diabetes Prevention Program, are available, but a gap remains in effectively translating and increasing uptake of these interventions into routine care. Methods We applied the Translating Research into Practice (TRiP) framework to guide three ...
I'm Dr Akshay Jain, an endocrinologist from Vancouver, and I'm joined by Dr James Kim, a primary care physician from Calgary, Canada. Both Dr Kim and I attended ADA 2024.
In particular, there is value in integrating DCESs in the management of patients with type 1 diabetes in primary care settings, where clinical experience is primarily in the management of type 2 diabetes. For organizations supported by outcomes-based payment models, these are particularly essential contributions.
Objective To compare the risk of dementia associated with sodium-glucose cotransporter-2 (SGLT-2) inhibitors versus dipeptidyl peptidase-4 (DPP-4) inhibitors in adults aged 40-69 years with type 2 diabetes. Design Population based cohort study. Setting Korean National Health Insurance Service data, 2013-21. Participants 110 885 propensity score matched pairs of adults with type 2 diabetes aged ...
Abstract. Type 1 diabetes is a chronic autoimmune disease characterised by insulin deficiency and resultant hyperglycaemia. Knowledge of type 1 diabetes has rapidly increased over the past 25 years, resulting in a broad understanding about many aspects of the disease, including its genetics, epidemiology, immune and β-cell phenotypes, and ...
The ICBH's project titled "Train, Inform, Involve and Transform to Reduce the Risk of Type 2 Diabetes in Black Communities in Canada" aims to train students from various disciplines to become peer educators. They in turn will inform religious leaders and encourage Black communities to take part in diabetes prevention activities, thereby helping to raise awareness and reduce the risks ...
Primary care providers already understand the clinical importance of identifying patients with diabetes, but to get their support, we needed to ensure that the BPA identified a manageable target population and that the recommended action when the BPA triggered (in this case, ordering A1C testing) was something readily achievable in a busy ...
The JLA patient priority group set user engagement as a research priority in 2018 and this review, including publications between 2016 and 2021, supports evidence that engagement data has been poorly utilised where only 10% (n = 19) of articles had available estimates to evaluate the impact of user engagement on intervention efficacy. Many ...
Introduction. Type 1 diabetes is one of the most common chronic childhood diseases, affecting an estimated 497,100 children under 15 years globally. 1 The incidence of type 1 diabetes among children is increasing in many countries, and more than 79,000 children under 15 years are estimated to develop type 1 diabetes annually worldwide. 1-3 Young people today also face another danger that ...