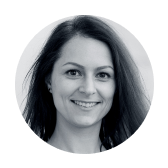

Supply chain analytics examples - 18 modern use cases
Leveraging data analytics has become a critical differentiator for any business that seeks to optimize its supply chain operations. Modern supply chain analytics, a transformative approach that harnesses the power of data-driven insights, has become a true game-changer in the field. The ability to make informed decisions is necessary to not stay behind your competitors.
This blog post delves into the world of modern supply chain analytics, exploring its definition, key components, and the remarkable capabilities it offers. Whether you're a business leader, supply chain manager, or data enthusiast, this guide will provide you with a comprehensive understanding of how to unlock the potential of your supply chain data and drive your organization towards greater success.
From demand forecasting and inventory optimization to risk mitigation and supply chain visibility, we'll examine a range of real-world use cases that showcase the transformative power of modern supply chain analytics. By the end of this post, you'll be equipped with the knowledge and inspiration to harness the power of data and revolutionize your supply chain operations.
So, let's dive in and uncover the secrets to unlocking the full potential of your supply chain with modern data analytics.
What is supply chain analytics?
Supply chain analytics refers to the use of data to gain insights and make informed decisions about the various components and processes within a company's supply chain. The insights are extracted through statistical analysis and advanced analytics techniques (AI and machine learning).
Supply chain data is inherently vast and diverse, encompassing both internal and external sources. It can include the following internal data:
- Inventory data
- Production data
- Financial data
- Customer data
- Employee data
External data:
- Market data
- Weather data
- Transportation data
- Geopolitical data
- Supplier data
And real-time data from:
- IoT devices
Supply chain analytics leverages all the data to optimize performance at every stage of the supply chain:
- Planning and demand management : accurate demand forecasting today is the foundation of effective supply chain management. Companies typically have to consider factors such as labor hours, costs, production feasibility, and profit expectations should be meticulously considered during the planning phase.
- Procurement : securing the necessary resources to meet projected demand is crucial. This involves finding reliable suppliers, ensuring material compliance with product specifications, negotiating prices, and procuring the right quantities.
- Production planning : transforming raw materials into finished products, which encompasses manufacturing, quality control, and packaging. Minimizing waste and inefficiencies at this stage is vital to preserving product quality, operational efficiency, and profit margins.
- Inventory management : once the products are ready, they must be carefully managed as they move from manufacturing to warehousing and ultimately to the point of sale. Maintaining accurate and real-time inventory levels is critical to avoiding disruptions in the supply chain. Ensuring the availability of raw materials when and where they are needed is a key aspect of effective inventory management.
- Transportation : this applies to both raw materials and finished goods. In today's global economy, partnering with a logistics provider in close proximity can simplify logistics, especially in the event of any issues. I’ve also written a detailed article about the use cases of data analytics in logistics only.
- Delivery and order fulfillment : The final step in the supply chain is the delivery of the goods to the customer. Selecting the appropriate transportation method and ensuring timely and reliable delivery are essential for customer satisfaction and maintaining a competitive edge.
Why is supply chain analytics important?
In today's fiercely competitive market, it’s crucial to make data-driven decisions. This is especially true for supply chain management, where even subtle changes can significantly impact costs, customer satisfaction, and ultimately, profitability.
Analytics provides a powerful lens for understanding historical data, identifying emerging trends, and forecasting future demand. This insight empowers supply chain managers to make informed decisions about inventory levels, production schedules, and transportation routes, leading to optimized operations and enhanced efficiency. Supply chain analytics is crucial for the following reasons:
- Making data-driven decisions : supply chain analytics provides valuable insights that enable companies to make more informed, data-driven decisions about their supply chain strategies. With the help of analytical tools and techniques, companies can better understand the impact of various factors, such as supplier performance, lead times, and market trends, on their supply chain operations.
- Understanding your customers : supply chain analytics allows businesses to glean valuable insights into customer behavior. By analyzing historical data, they can uncover buying patterns, preferences, and needs, leading to improved product offerings, targeted marketing campaigns, and enhanced customer service.
- Optimizing your supply chain : identifying inefficiencies and bottlenecks within the supply chain is key to maximizing efficiency. Analytics can pinpoint these areas, allowing for process improvements that save time and money. Furthermore, predicting future demand helps businesses avoid stockouts and excess inventory, ensuring a smooth flow of goods.
- Ensuring regulatory compliance : many industries require businesses to collect and manage supply chain data. Analytics enables businesses to analyze this data and ensure compliance with all relevant regulations, mitigating potential risks and ensuring smooth operations.
- Competitive advantage : companies that invest in supply chain analytics can gain a competitive edge by optimizing their supply chain performance, reducing costs, and improving customer satisfaction. By leveraging data-driven insights, companies can make more strategic decisions, adapt to changing market conditions, and differentiate themselves from their competitors.
- Risk mitigation : analyzing historical data and trends helps companies proactively plan for and respond to potential disruptions, reducing the impact on their operations. Supply chain analytics plays a critical role in identifying and mitigating potential risks, such as supply disruptions, quality issues, or regulatory changes, before they occur.
- Sustainability and environmental impact : supply chain analytics can help companies identify opportunities to reduce their environmental footprint, such as optimizing transportation routes, reducing waste, and improving energy efficiency. By analyzing data on emissions, resource consumption, and waste, companies can make more informed decisions to enhance the sustainability of their supply chain operations.
What are the components of modern supply chain data analytics?
The shift from traditional to modern supply chain analytics represents a significant transformation in how supply chain businesses leverage data and insights to drive their operations.
Whereas traditional approaches relied on limited, internal data sources, modern analytics harnesses a much broader range of data, including external, unstructured, and real-time information. This enables a more holistic, contextual understanding of supply chain dynamics. The analytical techniques have also advanced, moving from basic descriptive methods to sophisticated predictive modeling, machine learning, and prescriptive algorithms. This allows for more insightful and actionable recommendations to anticipate challenges and optimize operations.
The scope of supply chain analytics has expanded from siloed, function-specific views to a more integrated, end-to-end approach across the entire ecosystem. This provides deeper insights into interdependencies and opportunities for improvement. The timeliness and responsiveness of analytics has also improved, with modern approaches leveraging real-time data streams to enable rapid decision-making, in contrast to the lags of traditional methods.
Additionally, the role of automation and optimization has become more prominent, with autonomous, self-learning algorithms enhancing efficiency and driving continuous improvement.
Finally, the flexibility and adaptability of modern analytics stand out, allowing organizations to rapidly adapt to changing business needs and market conditions, a crucial capability in today's dynamic environment.
ELT data integration process
Supply chain data is highly siloed across different functional areas and different datasets are used across each stage of the ecosystem. Integrating and consolidating this fragmented data into a cohesive, end-to-end view is a significant challenge.
This is where the power of ELT (Extract, Load, Transform) data integration comes into play, particularly advantageous in the logistics context. ELT allows for the rapid ingestion and transformation of data from these diverse sources, without the need for the more complex and time-consuming ETL (Extract, Transform, Load) processes (you can refer to my earlier article for a detailed comparison of ETL vs ELT). This agility is crucial for enabling real-time analytics and other advanced analytical techniques that can provide a modern boost to your logistics analytics capabilities.
Unlike traditional ETL, which requires data to be transformed before loading into the target system, ELT first loads the raw data and then performs the necessary transformations. This approach offers several benefits:
- Faster time-to-insight: by eliminating the upfront transformation step, ELT enables the rapid ingestion of data, allowing for quicker access to insights and accelerating decision-making. If you come across data-related terms that are unclear, I encourage you to refer to our comprehensive data glossary for definitions.
- Flexibility: the ELT model provides greater flexibility to adjust and refine data transformations over time, as business requirements or data sources evolve.
- Scalability and performance: ELT architectures are often more scalable and can handle larger data volumes with improved performance, as the transformation workload is distributed across the data warehouse or analytics platform.
- Reduced Complexity: ELT simplifies the overall data integration process, as it eliminates the need for maintaining complex ETL pipelines and reduces the risk of data loss or corruption during the transformation stage.
Modern data platform
The indispensable capability of this platform is integrated data management - the ability to collect, integrate, and manage data from diverse sources across the supply chain, including ERP systems, TMS systems, external data sources, real-time data and more. A modern data platform is easily scalable, so it leverages advanced data integration techniques and technologies like data lakes and data warehouses.
Real-time supply chain visibility
Providing end-to-end supply chain visibility through the use of IoT sensors, GPS tracking, and other real-time data sources. Enabling proactive monitoring and alerting to identify and respond to supply chain disruptions or performance issues in a timely manner.
Predictive analytics
Applying machine learning and advanced statistical modeling techniques to forecast demand, predict supply chain disruptions, and optimize inventory levels. Leveraging historical data, market trends, and external factors to generate accurate and actionable predictions. Predictive analytics is critical for unlocking scenario planning and simulations , which are critical for optimizing the performance of the modern supply chain.
Prescriptive analytics
Employing optimization algorithms and decision-support tools to recommend the best course of action based on the insights generated from predictive analytics. Enabling supply chain professionals to make more informed decisions on inventory management, transportation planning, and supplier selection.
Descriptive analytics
Analyzing historical data to understand past performance, identify patterns, and uncover insights about the supply chain's operations.
By embracing these core components, modern supply chain analytics can enable organizations to achieve greater operational efficiency, enhance decision-making, and improve overall supply chain performance in an increasingly complex and dynamic business environment.
Business Intelligence
Modern supply chain analytics must provide robust visualization and reporting tools that allow supply chain professionals to access and interpret data-driven insights easily. Business intelligence solutions offer interactive dashboards, reports, and data visualization features help supply chain managers make informed decisions and communicate insights effectively across the organization – but that’s not where the role of BI for the supply chain ends.
Advanced analytics and ML
Modern data platforms typically provide advanced analytics capabilities, including AI-powered predictive modeling, optimization algorithms, and machine learning techniques. These capabilities enable supply chain companies to leverage historical data and real-time data to forecast demand, optimize inventory levels, identify supply chain risks, and automate decision-making processes.
In fact, the examples of applications of AI in the supply chain can go as far as your imagination does. I’ve gathered 28 examples on how to boost the supply chain with artificial intelligence in an earlier article.
Cognitive supply chain is a new concept growing in popularity thanks to these technologies. It’s about leveraging AI and ML to automate decision-making and optimize supply chain processes, as well as enabling self-learning and self-correcting supply chain systems that can adapt to changing conditions without human intervention.
Secure data sharing
Facilitating seamless collaboration and information sharing among all supply chain stakeholders is critical for smooth end-to-end performance. Modern data platforms can facilitate secure data sharing and collaboration among supply chain partners, enabling them to share information, coordinate activities, and make joint decisions based on a shared understanding of the supply chain. Advanced security and access control features ensure the protection of sensitive supply chain data.
Modern supply chain analytics examples
Supply chain analytics is a rapidly evolving field that holds immense potential for businesses of all sizes and industries. Supply chain analytics examples are vast, limited only by the creativity of those who seek to leverage its powerful insights.
From optimizing inventory management and forecasting demand to identifying supply chain bottlenecks and enhancing customer service, the use cases for supply chain analytics are as diverse as the challenges faced by modern organizations. In this section, I’m exploring a selection of innovative supply chain analytics use cases that illustrate the transformative impact this discipline can have on organizational efficiency, profitability, and resilience.
Read on to understand the breadth of possibilities.
1. End-to-end supply chain visibility dashboards
The importance of being able to monitor the flow of goods throughout the entire supply chain in real-time cannot be overstated. It's about having a clear picture of where products are, what their status is, and what potential disruptions might be on the horizon.
With real-time supply chain visibility into the movement of goods, companies can make more informed decisions about production, inventory levels, transportation routes, and potential disruptions. Better visibility allows for better coordination and collaboration among supply chain partners, reducing delays, optimizing logistics, and minimizing waste.
Achieving supply chain visibility requires combining data from various sources, such as ERP systems, transportation management systems, warehouse management systems, and supplier portals, to create a unified view of the supply chain. Utilizing technologies like GPS tracking, RFID tags, and IoT sensors allows for real-time monitoring of goods in transit and within warehouses.
2. Demand forecasting with ML algorithms
Demand forecasting, when implemented with the power of supply chain analytics, transforms from a guesstimate into a strategic tool for optimizing operations and maximizing profitability. Instead of relying on gut feelings or historical trends alone, companies can leverage data from diverse sources to predict future demand with greater accuracy.
Data from various sources like point-of-sale systems, customer relationship management (CRM) systems, social media, weather data, and economic indicators are integrated into a centralized platform. This allows for a comprehensive view of past and present demand patterns. Machine learning algorithms, statistical modeling, and predictive analytics are applied to the integrated data to identify trends, seasonality, and other factors influencing demand. This helps create more accurate forecasts.
Different scenarios, like economic downturns, competitor actions, or new product launches, are modeled to assess their potential impact on demand. This allows for more robust and flexible forecasting. The forecasts are constantly monitored and adjusted based on real-time data, ensuring they remain accurate and responsive to changing market conditions.
3. Route optimization for transportation networks
Route optimization for transportation networks involves designing and improving efficient routes to move goods cost-effectively. One of the key benefits of route optimization is cost reduction. By optimizing transportation routes, businesses can minimize expenses such as fuel costs, labor costs, and vehicle maintenance costs, resulting in increased profitability.
Another important aspect of route optimization is improved delivery performance. By optimizing routes, businesses can ensure faster delivery times, which can lead to increased customer satisfaction and a competitive advantage in the marketplace. This can be achieved by minimizing travel time, reducing transit delays, and optimizing the use of transportation resources.
Additionally, route optimization can also lead to increased capacity utilization. By optimizing routes, businesses can make the most efficient use of their transportation resources, such as vehicles and drivers, resulting in a reduced need for additional resources and lower costs.
4. Supply chain network design and optimization
Supply chain network design is a strategic planning activity that focuses on two key aspects: determining the footprint of the supply chain (where to locate facilities and how to size them) and deciding on the product flow through the supply chain.
The design of the supply chain network will dictate the capacity of the business facilities, as well as the movement of raw materials, intermediates, and finished goods from source to consumption. Decision-makers must consider numerous complex variables, such as labor costs, customer locations, and available transportation networks. Due to the scale and complexity of modern supply chains, these decisions are typically supported by prescriptive analytics.
5. Predictive maintenance for manufacturing equipment
Predictive maintenance is a game-changer for supply chains, using data to anticipate equipment failures before they occur. Imagine sensors constantly monitoring machinery like forklifts, conveyor belts, and automated storage systems. This data is analyzed using algorithms and machine learning to identify patterns and predict potential breakdowns. Instead of waiting for a breakdown, companies can schedule maintenance during off-peak periods, minimizing disruption to operations.
According to McKinsey Global Institute , “Predictive maintenance typically reduces machine downtime by 30 to 50 percent and increases machine life by 20 to 40 percent.” This proactive approach not only prevents costly repairs and unscheduled downtime but also optimizes maintenance efforts by focusing on components with the highest risk of failure. Furthermore, predictive maintenance allows for more accurate forecasting of spare parts needs, minimizing stockouts and reducing inventory costs.
6. Dynamic pricing with real-time analytics
Dynamic pricing is a strategy where prices for goods and services are adjusted in real-time based on various and diverse factors, such as fluctuations in customer demand, influenced by seasonality, holidays, or market trends, as well as external factors like fuel prices, raw material costs, or competitor pricing. Real-time data on inventory levels, transportation capacity, and delivery routes also plays a crucial role in dynamic pricing, allowing for adjustments to optimize resource allocation and pricing.
Dynamic pricing is used in various aspects of the supply chain. Logistics companies can adjust their shipping rates based on fuel prices, traffic conditions, and demand for specific routes. Warehousing companies can dynamically adjust storage rates based on available space, demand for specific storage types, and the cost of labor. Manufacturers can adjust pricing based on raw material costs, production capacity, and demand for specific products.
7. Risk assessment modeling and mitigation
Risk assessment modeling has become a strategic necessity for businesses seeking to thrive in an increasingly complex and volatile world. A proactive approach to identifying, assessing, and mitigating risks is necessary for companies to build supply chains that are resilient and adaptable – a critical advantage in the ever-evolving global marketplace.
Through advanced predictive modeling, companies can quantify the likelihood and impact of these risks, enabling them to make informed decisions and develop targeted mitigation strategies. This might involve diversifying supplier networks, implementing redundancy measures, or optimizing inventory levels – all informed by the insights gleaned from in-depth analytics.
But the value of data analytics in supply chain extends beyond mere risk identification. Organizations are leveraging supply chain analytics to simulate various disruption scenarios, allowing them to test and validate their mitigation plans. This scenario planning not only enhances preparedness but also fosters a culture of agility, where supply chain teams can adapt swiftly to emerging challenges.
8. Warehouse layout optimization
Warehouse layout is about leveraging technology to gather real-time data, analyze it to gain valuable insights, and optimize warehouse operations.
Automated systems like robots, conveyors, and automated guided vehicles (AGVs) constantly collect data on inventory levels, location, movement, and performance metrics. This data is then analyzed using advanced analytics tools to identify trends, bottlenecks, and areas for improvement. For example, analyzing data on picking times and travel distances can optimize picking routes and improve efficiency.
https://www.youtube.com/watch?v=8huwyHfHR0g
E-commerce retailers like Walmart and Amazon are heavily investing in warehouse automation to handle the increasing volume of online orders. Manufacturers are using supply chain analytics and automation to optimize their warehousing operations and streamline the supply chain for their products.
9. Customer segmentation for targeted supply chain strategies
Customer segmentation divides a company's customer base into distinct groups based on shared characteristics and behaviors. In the context of supply chain analytics examples, this method allows businesses to tailor their supply chain operations to meet the specific needs of each customer segment more effectively.
The process involves analyzing customer data such as purchasing patterns, order frequency, volume, and service level requirements. Using advanced analytics techniques, businesses identify meaningful segments and develop targeted strategies for each group. For instance, high-volume customers might receive priority in inventory allocation, while those with unpredictable demand patterns could be managed with more flexible supply chain arrangements.
This segmentation approach can be applied to various aspects of the supply chain, including inventory management, distribution network design, transportation planning, and production scheduling. Companies often see improved customer satisfaction due to more tailored service, cost optimization through efficient resource allocation, and enhanced demand forecasting accuracy. It also allows businesses to focus on their most valuable customer segments, potentially increasing profitability and market share.
Ultimately, customer segmentation for targeted supply chain strategies enables businesses to move away from a one-size-fits-all approach, creating a more nuanced, efficient, and customer-centric supply chain.
10. Demand sensing using social media and external data
Demand sensing using social media and external data is a supply chain analytics example that leverages real-time information from various sources to improve demand forecasting accuracy and responsiveness. It works by collecting data from social media, search trends, news, weather forecasts, and economic indicators, then processing and analyzing this data using NLP and machine learning algorithms. These insights are integrated with existing supply chain systems to update demand forecasts in near real-time.
Businesses can apply this technique to improve demand forecasting accuracy, optimize inventory management, implement dynamic pricing strategies, inform new product launches, enhance marketing and promotion planning, improve risk management, and conduct competitor analysis.
The potential benefits include improved forecast accuracy, reduced inventory levels, fewer stockouts, increased agility in responding to market changes, significant cost savings, and potential revenue growth. However, successful implementation requires investment in data infrastructure, analytics capabilities, and organizational change. The extent of the benefits depends on how well a business integrates these insights into its decision-making processes and overall supply chain strategy.
11. Inventory optimization through predictive analytics
Inventory optimization through predictive analytics is a data-driven approach to managing stock levels in supply chain management. This method uses advanced analytics techniques to forecast demand and determine optimal inventory levels, reorder points, and order quantities.
The process involves collecting historical sales data, inventory levels, and other relevant information, then applying statistical methods and machine learning algorithms to identify patterns and predict future demand. These predictions are then used to create mathematical models that optimize inventory across the supply chain.
Ultimately, inventory optimization through predictive analytics is one of those supply chain analytics examples that enable companies to achieve more efficient and cost-effective processes.
12. Price elasticity analysis for raw materials
Businesses that rely on commodities like oil or grain face a constant challenge: fluctuating prices. These swings are often driven by big economic forces beyond their control, like global demand, political instability, or even weather patterns.
To manage this uncertainty, many companies opt for price elasticity analysis for raw materials. It helps them understand how price changes affect the demand or supply of materials essential to a business. This approach involves analyzing historical data on prices and quantities to calculate elasticity coefficients, which measure the sensitivity of demand or supply to price fluctuations.
While predicting commodity prices isn't foolproof, using these strategies can help businesses gain a degree of control over their costs, allowing them to plan effectively and avoid being caught off guard by market volatility. For instance, if a raw material is highly elastic, companies might focus on bulk purchases when prices are low.
13. Scenario planning and simulation for supply chain disruptions
Scenario planning and simulation is one of those supply chain analytics examples that helps businesses prepare for potential risks. This method uses advanced analytics to model and evaluate various future scenarios that could impact a company's supply chain.
The process involves collecting historical data, developing hypothetical disruption scenarios, and creating mathematical models of the supply chain network. Key variables like lead times, capacity, demand, and costs are incorporated into these models. Using analytics tools, businesses simulate how different scenarios would affect their supply chain and analyze the potential consequences on key performance indicators.
This approach allows companies to identify vulnerable areas, develop contingency plans, and optimize resource allocation. It informs decisions about supplier diversification, network redesign, and technology investments to improve resilience.
To mitigate disruptions, businesses can implement early warning systems, maintain flexible capacity, optimize inventory levels, and diversify suppliers. They can also enhance collaboration with partners, develop agile decision-making frameworks, and prepare financial buffers.
14. Real-time supply chain visibility into shipments and assets
Real-time tracking of assets is a technology-driven approach that provides continuous monitoring and reporting of the location, condition, and status of goods and equipment as they move through the supply chain.
This system leverages a combination of GPS, IoT sensors, RFID or barcode technology, and cellular or satellite networks to collect and transmit data, which is then processed and presented through cloud-based platforms and user-friendly dashboards.
Businesses can utilize real-time supply chain visibility to gain unprecedented visibility into their supply chain operations. It allows them to monitor shipments and assets throughout their journey, enabling proactive problem-solving and more efficient inventory management.
Companies can provide accurate ETAs and status updates to customers, enhancing their service quality. It also aids in risk management by allowing close monitoring of sensitive or high-value shipments and ensures compliance with regulations, especially for goods with specific handling requirements.
15. Carbon footprint tracking and reduction
Carbon footprint tracking and reduction involves measuring and minimizing greenhouse gas emissions across a company's entire supply chain. It works by establishing a baseline of emissions, using data on energy consumption, transportation, manufacturing, and waste management. Advanced analytics tools process this data to calculate the carbon footprint and identify areas for improvement.
Businesses can use data analytics in supply chain to set and track emissions reduction targets, optimize operations, inform supplier selection, and enhance sustainability reporting. It can be applied to transportation route optimization, energy source selection, product redesign, and supplier engagement.
Results often include reduced operational costs, improved brand image, easier regulatory compliance, and contribution to climate change mitigation. Benefits of carbon footprint tracking go beyond environmental advantages: they can help businesses save costs, offer a competitive advantage in environmentally conscious markets, and access to sustainability-linked opportunities.
However, the extent of these benefits depends on the comprehensiveness of implementation and the company's commitment to acting on the insights gained. While initial costs can be high, many businesses find long-term value in this investment for efficiency, risk management, and reputation.
16. Supplier relationship management analytics
Supplier relationship management (SRM) is a data-driven approach to optimizing interactions with suppliers. It works by integrating data from various sources, including procurement systems, quality control reports, delivery performance metrics, and financial data. Advanced analytics tools and machine learning algorithms are then applied to generate insights and actionable recommendations.
Businesses can use SRM analytics to assess supplier performance, identify risks, inform negotiations, and make strategic decisions about supplier selection and development. This approach enables companies to improve supplier performance, reduce costs, mitigate risks, and align supplier capabilities with long-term business goals.
While the extent of benefits depends on factors such as data quality and the organization's ability to act on insights, many businesses find that SRM analytics provides a competitive advantage in managing increasingly complex global supply chains. Despite the initial investment required, the long-term benefits in cost savings, risk reduction, and strategic advantage often make it a worthwhile endeavor for companies looking to build more resilient and efficient supply chains.
17. Quality control analytics using statistical process control
Quality control analytics using statistical process control (SPC) in supply chain analytics is a data-driven approach to monitoring and improving product quality throughout the manufacturing process. It applies statistical methods to identify, analyze, and reduce variations in production, ensuring consistent quality and minimizing defects.
SPC works by collecting data on various quality parameters during production and analyzing it using statistical tools like control charts and process capability analysis. This helps determine if processes are stable and predictable or if they require intervention. The system continuously monitors production, enabling early detection of issues and facilitating root-cause analysis when problems occur.
18. Sales and operations planning (S&OP) analytics
S&OP is a cross-functional business process that aligns supply and demand to optimize overall performance. It involves forecasting sales and demand, planning production and resource requirements, balancing inventory levels and supply chain constraints, and integrating financial and operational plans.
The key benefits of S&OP include improved demand forecasting, better inventory management, enhanced resource utilization, increased responsiveness to market changes, and improved financial performance.
Implementing S&OP requires a cross-functional team and clear communication. It starts with establishing the S&OP process and gathering data, and can be refined over time to suit the business's unique needs.
Ready to implement specific supply chain analytics use cases?
Modern supply chain analytics bring remarkable, transformative capabilities to the sector. From demand forecasting and inventory optimization to risk mitigation and supply chain visibility, we've examined a range of real-world use cases that showcase the power of data-driven insights in revolutionizing supply chain operations.
The shift to modern data analytics in the supply chain represents a significant transformation, with a broader range of data sources, advanced analytical techniques, and a more integrated, end-to-end approach.
If you're ready to harness the power of modern supply chain analytics and drive your organization towards greater success, we invite you to book a free data strategy session with our team of experts through this contact form . Together, we'll explore the unique challenges and opportunities within your supply chain and develop a customized plan to leverage data and analytics for optimal performance.
People also ask
Want to read more.
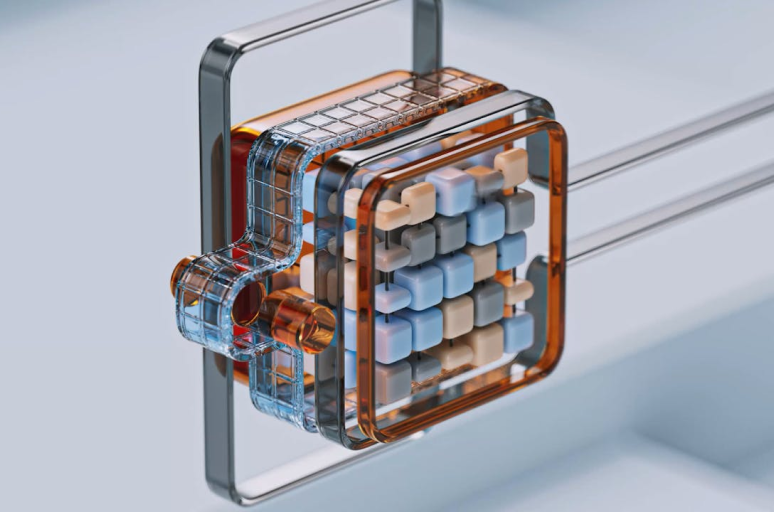
ELT Process: unlock the future of data integration with Extract, Load, Transform
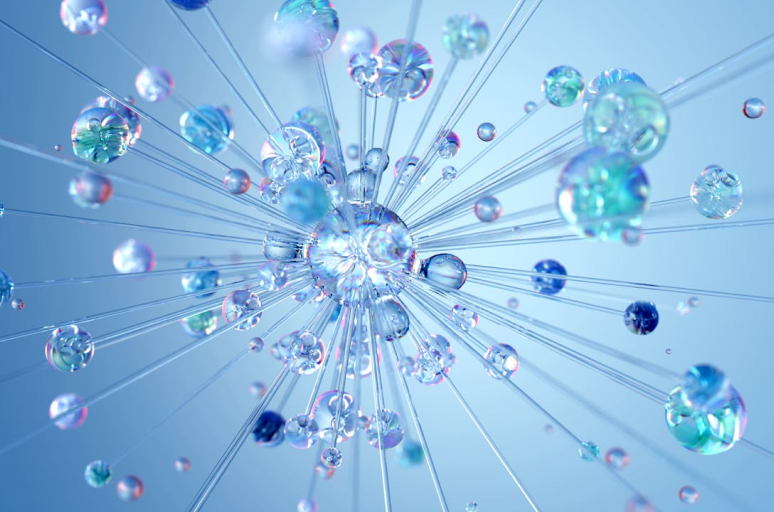
Data integration: different techniques, tools and solutions
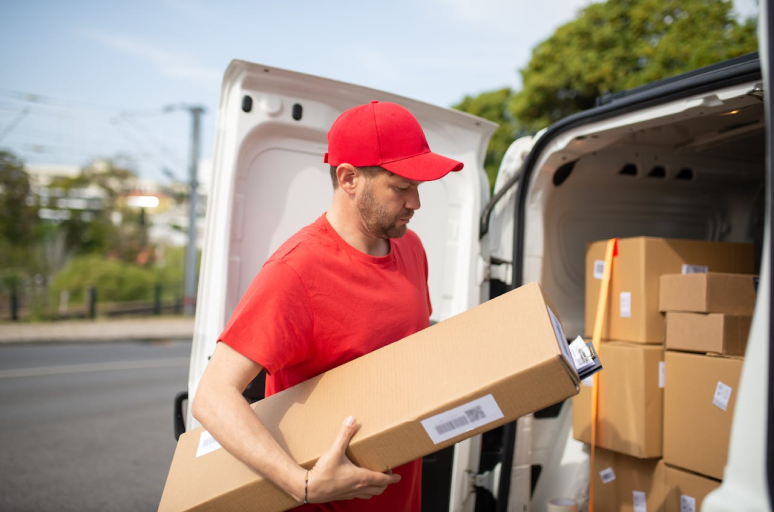
Logistics analytics: driving operational efficiency in the supply chain
Topic: Supply Chain Management
How real-time analytics is used to optimize supply chain and inventory control.
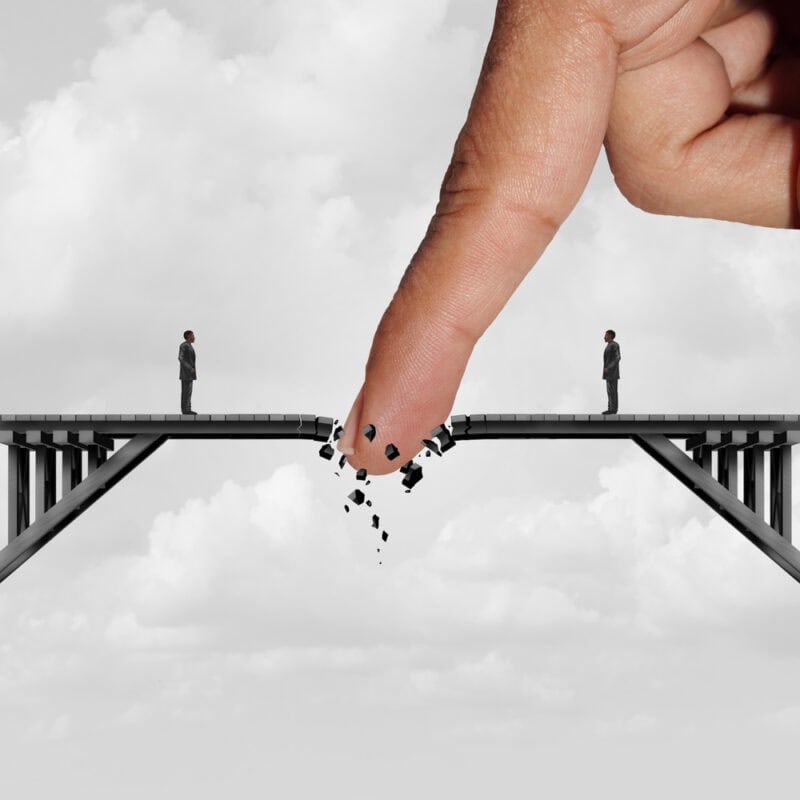
Baltimore Bridge Collapse: The Urgent Need for Real-Time Supply Chain Interoperability
Interoperability may be the key to enabling real-time supply chain end-to-end visibility, enhanced collaboration, and heightened customer
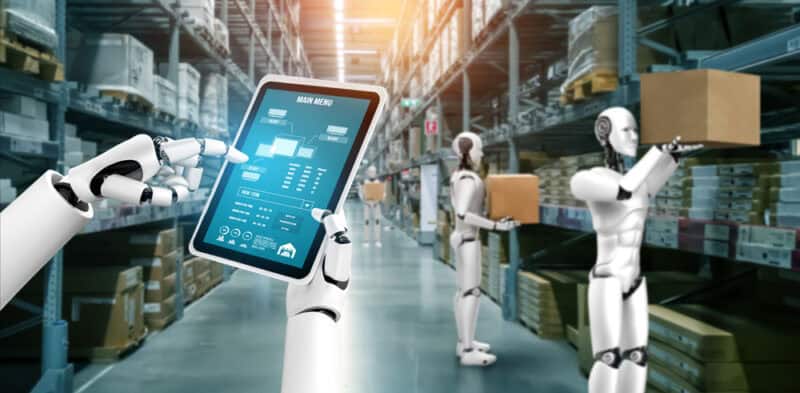
Generative AI Brings Real-Time Supply Chains Closer to Reality
Embedding Generative AI into supply chains isn't just a technology project, it also requires a profound shift in the way organizations think about creating …
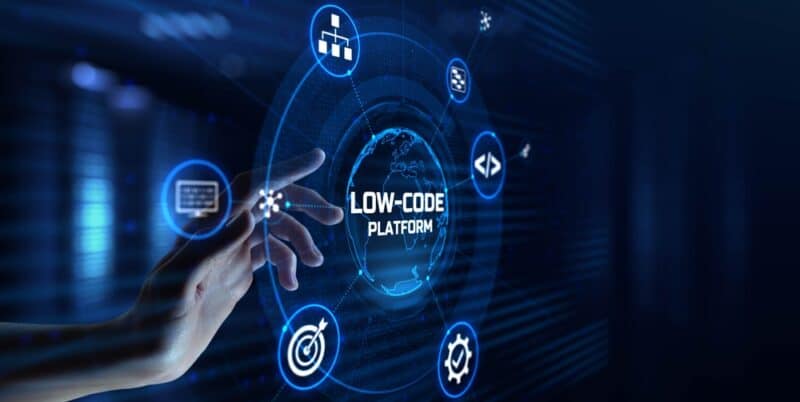
6 Benefits to Embracing No-Code/Low-Code Enabled Solutions Across the Supply Chain
No-code and low-code solutions used throughout the supply chain empower businesses to integrate data seamlessly, streamline custom workflows, and optimize …
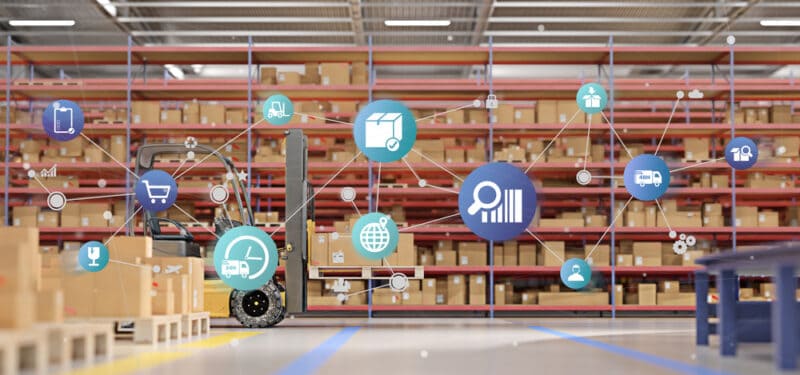
Can Real-Time Analytics Solve the Supply Chain Crisis?
The integration of IoT devices and their real-time data into transportation services offers unprecedented visibility and control over the supply
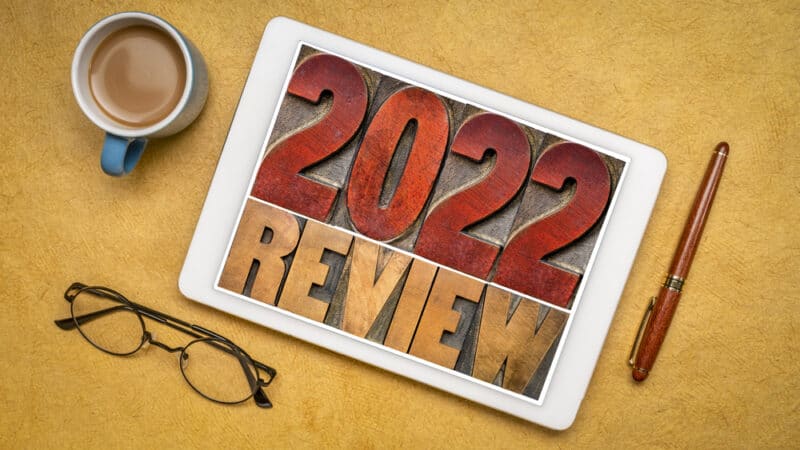
RTInsights 2022: A Look Back at the Year’s Top Stories
Major themes that persisted throughout the year included applying analytics to supply chain problems, mainstream use of AI, and infrastructure to access data.
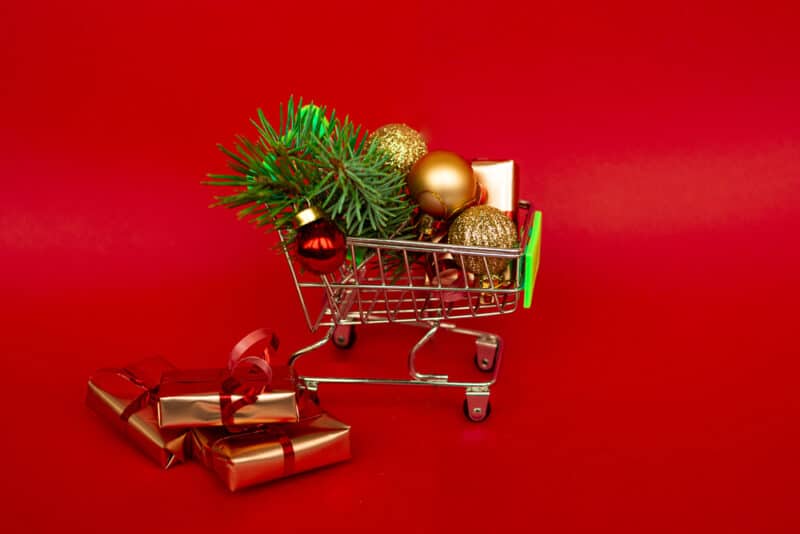
Holiday Gift Returns: Another Chance to Engage the Customer
Retailers are using end-to-end return management systems to successfully execute reverse logistics and grow brand loyalty amid the busy holiday shopping
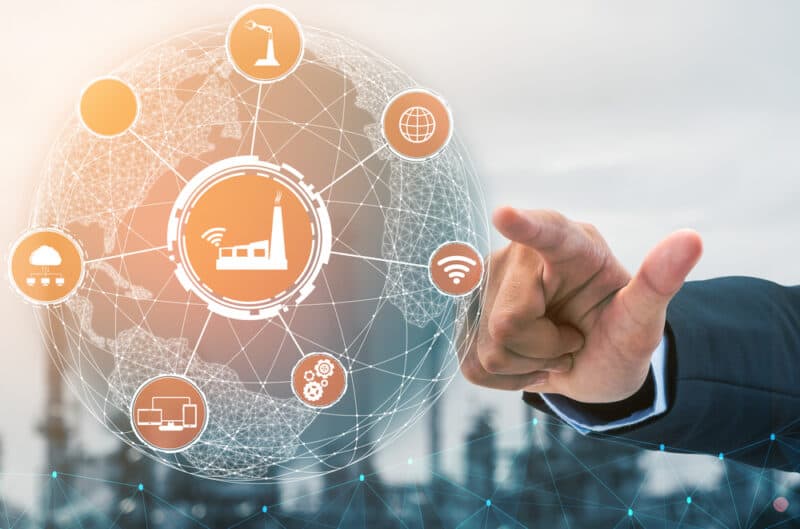
Intelligent Digital Supply Chain Trends to Watch
Companies are trying to address supply chain challenges by leveraging cutting-edge technologies including robotics, edge computing, and artificial
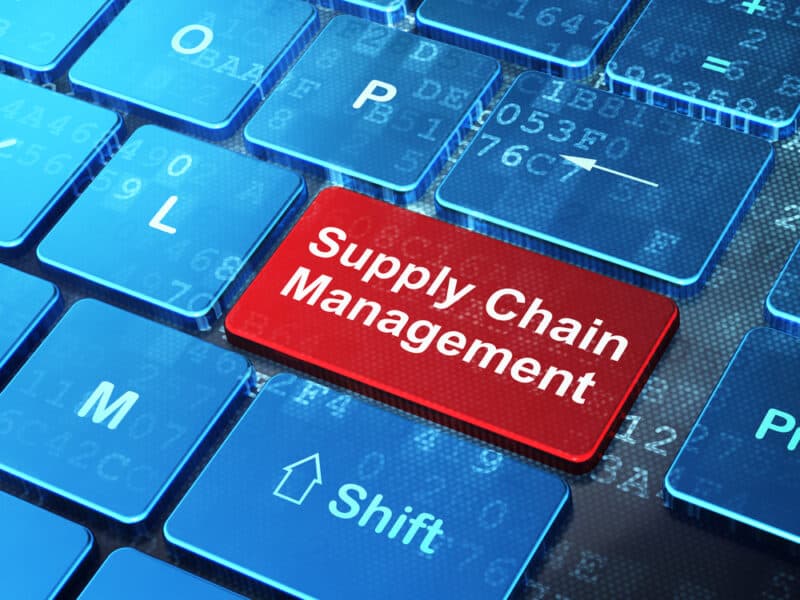
5 Ways Analytics Are Disrupting Supply Chain Management
Harmonizing supply chain management analytics will put organizations on a path to automating their
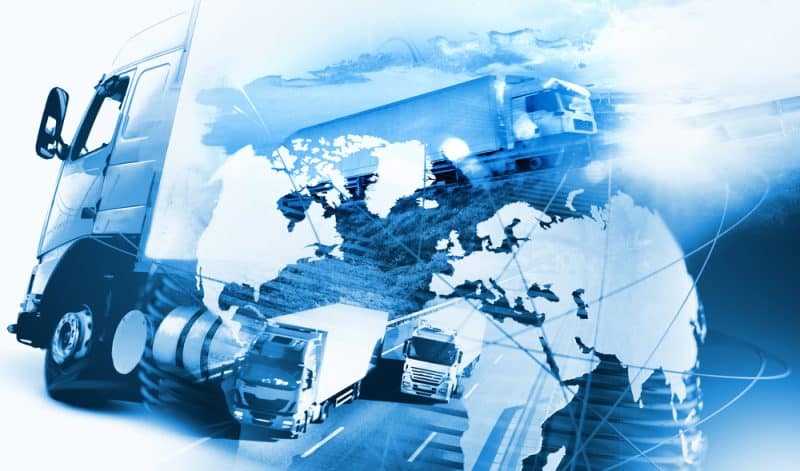
How IoT in Transportation Makes Big Data Valuable For Businesses
IoT data in transportation combined with machine learning and artificial intelligence can help make a supply chain transparent and
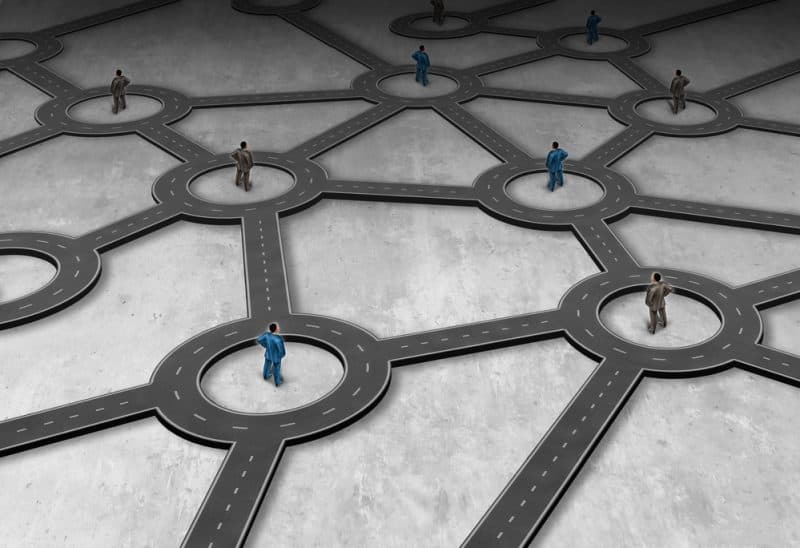
Dissecting a Supply Chain’s Digital Twin
At the heart of the supply chain digital twin development lies a flexible supply chain simulation model that is data-driven and
A more resilient supply chain from optimized operations planning
Across industries, companies that rely on transported materials for their operations have gained hard-earned knowledge from major disruptions over the past couple of decades, most notably the financial crisis of 2008, the COVID-19 pandemic, and geopolitical developments. During times of sudden upheaval, companies must quickly ramp down operations and then ramp up again once demand bounces back, adapt their execution, and revisit long-term plans. The supply chain has a special role to play, as companies rapidly shift their focus from cost-effectiveness to maximized throughput—all with the same assets and infrastructure.
About the authors
A poor response can have cascading effects, such as facility shutdowns or missed delivery obligations. In recent years, approaches that use data and analytics to identify answers and make recommendations to specific business problems have proved to be particularly relevant in bringing clarity to operations planning and thus improving supply chain resilience. However, the vast majority of companies have yet to implement such approaches in their supply chain, leaving a serious gap in their planning capabilities.
A recent McKinsey article 1 “ Building value-chain resilience with AI ,” November 26, 2021. examined three value chain approaches that can address this supply chain gap —simulations of reality, optimization of plans, and real-time control-tower monitoring (see sidebar, “How analytics supports different planning approaches”). Here, we examine the second approach in more detail, as it ranks among the most powerful tools businesses can use to navigate complex and changing environments, especially disruptions.
How analytics supports different planning approaches
Supply chain analytics can support planning efforts that broadly fall into three types. While optimization has been at the center of this article, two other tools are simulation and monitoring. All three require expert knowledge of the system, but simulation also relies on large historic data sets (exhibit).
Optimization models are prescriptive analytics tools. The main output is an optimal plan for the current environment. Optimization is most useful when an organization must create an ideal plan from scratch that factors in complex rules and constraints. A typical use case for optimization is building a monthly plan for operational production as part of the sales and operations planning process.
Simulations are descriptive analytics tools. In a simulation, a digital twin is constructed with uncertainty measures and operational inputs. Simulation is more appropriate for comparing or modifying existing plans in the face of uncertainty. Whereas optimization is more of a “black box” process, simulation produces explainable KPI-driven reports. A typical simulation use case involves examining an existing material network with uncertain production quantities, demand, and transit times to identify first- and second-order bottlenecks.
The third analytics approach is real-time monitoring. Most companies have a control tower serving as an operating nerve center. However, fully digital end-to-end control towers can increase resilience by expanding the scope of real-time monitoring to anticipate and respond to upcoming or potential supply chain disruption. Real-time monitoring is the most useful when seamless communication between various functions and integrated decision making are critical.
A combined approach is often best. For example, consider detailed railway scheduling. The complex rules and interactions require optimization methods to create valid plans, while simulation can then validate the performance and robustness of plans and determine the most effective delay-mitigation policies. Real-time monitoring ensures seamless communication between individual hubs in case of unexpected disruptions.
Organizations that want to get the most out of this powerful approach design their associated optimization tools and processes along five best practices: improve information flows between teams, elevate customer centricity, bridge the gap between long-term planning and day-to-day operations, understand true operational constraints, and use scenario analysis to ask critical “what if” questions.
As companies in manufacturing industries have discovered, following these design practices can increase supply chain throughput by 10 to 15 percent in the short term, with no change in assets or overall configuration. Organizations can also reduce costs by 5 to 10 percent and CO 2 emissions by 10 to 15 percent over the long term while increasing operational flexibility and resilience toward disruptions. This article details the design best practices that support this effort and how companies can get started integrating the necessary capabilities into their business.
Optimization in sales and operations planning
Optimization in operations planning involves determining the optimal choices for a set of decisions in a given business environment and business target. This type of optimization generally works best with prescriptive models that provide the ideal set of decisions as an output. The elegance of optimization is its ability to adapt to not only changing business environments but also shifts in the business target—for example, from minimum cost to maximum throughput, highest yield, zero environmental impact, or a combination of multiple factors.
For these reasons, optimization is the ideal approach to readjust a company’s operations as outside factors or strategic priorities change. For example, an agricultural company recently experienced dramatic rises in production costs combined with limited transport capacity, creating significant gaps in its ability to manage existing resource constraints. The company was able to respond by shifting its operations planning to an optimization approach that almost completely closed this gap.
Would you like to learn more about our Manufacturing & Supply Chain practice?
In essence, organizations can embrace optimization to support better, faster planning and thus increase value capture and resilience. Five best practices can sustainably improve supply chain decision making across the full coordination process (exhibit). To do so, the design of the corresponding optimization tools and processes must address all decision layers—from strategic orientation to operational execution. The best practices for optimization design explicitly address distinct layers and can thus enhance transparency and planning effectiveness.
1. Elevate customer centricity by enabling a pull principle for demand-driven operations
Customer-centric thinking is a foundational element for any type of operations planning. Tools and processes must be able to quickly translate demand into sequence and volume of production units and share this information with individual production sites. Optimization tools and processes can make these decisions rapidly and objectively, enabling both automated plan adjustments based on changing customer demand and faster responses by contact center agents to customer requests. Satisfying those requests can have a significant impact on operations. A commodity metals company was able to accelerate its decision-making process and thus more quickly react to urgent customer requests resulting from demand fluctuations caused by rapidly changing spot prices.
2. Bridge the gap between long-term planning and day-to-day operations
Short-term plans are typically shaped by current constraints, while long-term plans depend on outside factors that are likely to change. Effective operations planning addresses the in-between period, in which value can be lost in the gap between tactical vision and concrete implementation. Optimization tools and processes can translate mid- and long-term plans into detailed operational schedules, automatically accounting for dynamic conditions and complex operational constraints while allowing users to refine and explore possibilities. The gap is bridged in the other direction by feeding information on actual operations and deviations from control-tower monitoring back to optimization or simulation tools to continually minimize any loss in value. The agricultural company mentioned above was able to ensure its day-to-day operations remained in line with the long-term strategic goals and tactical monthly plans despite significant changes in the overall business environment.
3. Improve information flows between operations and marketing and sales
Organizations need to enable a consistent flow of information among stakeholders. Integrated supply chain planning tools already bring together information from multiple systems and business functions, creating transparency and empowering decision makers while enabling analytics tools. Optimization tools and processes can be added to improve decision making. A tangible example of this interplay can be a sudden change in production capacity due to an unforeseen dumper breakdown. Integrated planning tools ensure all relevant stakeholders are aware of this change, but only optimization tools will actively steer and synchronize the decisions of the entire organization toward the ideal target in this new environment. Actions could include modified marketing and pricing of the respective products or the realignment of the supply chain. Comprehensive control-tower dashboards are then used by teams from various functions to support real-time decision making.
For instance, a mining company was able to quickly and regularly make updates to its product portfolio based on recommendations from the marketing and sales team. This coordinated rapid response enabled the company to keep its portfolio closely aligned with market demand at all times.
4. Understand true operational constraints by dissecting the infeasible plans
The exercise of identifying and avoiding infeasible plans often leads planners to review and adjust their operations. This infeasibility can come in the form of forced stoppages when operators are tasked with following an impossible plan or situations in which plans are unable to meet all known operational constraints. Control-tower dashboards can aid in understanding these constraints and providing feedback. Optimization tools can then progressively integrate all these constraints into actual operations planning, thus providing consistently feasible solutions. In this way, the impact of optimization tools results in higher adoption, as they reduce the overall frustration level across the organization associated with these infeasible solutions. For one automotive company facing a shortage of semiconductor chips, a control-tower tool in combination with optimization-based processes generated more than $100 million in margin improvement.
5. Use scenario analysis to ask critical what-if questions
Planners must have an operational process that can run, understand, and evaluate scenarios for planning and scheduling. This process produces what-if questions that can inform discussions with sales, customers, and third parties and support better decision making. Optimization tools allow decision makers to focus on the “what if?” and receive immediate and risk-free feedback on the consequences, thus streamlining and lowering the barriers to asking insightful questions. Recently, a pharmaceutical company was able to improve overall throughput and on-time delivery by using optimization tools that enabled asking what-if questions regarding rush orders, staffing shortfalls, and capital expenditure investments.
Get started with an appropriate business opportunity
Many companies have yet to make significant investments in optimization for their operations planning. Often, organizations have not even undertaken the analysis to select the business opportunity. In addition, discussions around optimal business targets that cover the most relevant trade-offs are often ignored, as they reside between functions and are thus considered “off limits.” Picking the wrong operations element or business target for optimization diverts finite resources from what truly matters and represents the biggest risk of failure.
The key is to identify a process that satisfies three criteria: first, the overall decision space must be so large that an individual planner can’t explore and understand all the possibilities at once; second, the quality of outcomes must be objectively measured and assessed according to a well-defined and accepted target; and third, the potential for improvement must be measurable and ideally quantifiable—for example, costs, throughput, CO 2 emissions, profit, or a combination of multiple factors. The mining company focused on profit by adjusting the supply chain in line with the optimal product portfolio, and the agriculture company emphasized the marginal costs of incremental production. The metals company selected a combination of throughput and yield while maintaining high customer satisfaction. The automotive company minimized lost revenue coming from supply chain disruptions. And the pharmaceutical maximized on-time, in-full delivery.
Companies must ensure their optimization program identifies business opportunities and associated targets that are not properly covered by the existing manual processes. However, any optimization-based approach must retain an adequate level of human judgment and expertise to account for unexpected situations and outliers. Optimization should aim to augment and empower human decision makers but not replace them.
Common pitfalls in data gathering and infrastructure
Companies should avoid directing time and resources to elements that aren’t necessary for high-functioning operations planning. For example, contrary to conventional wisdom, large longitudinal data sets are not required. Optimization for supply chain planning can typically be built by drawing on knowledge gained through expert interviews as well as snapshots using a small amount of the latest business data. Both elements are present today, as organizations need access to this information to monitor supply chain performance in the first place.
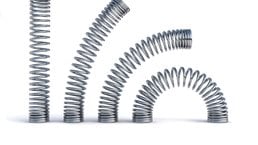
Future-proofing the supply chain
Similarly, companies should avoid focusing on building new data-centric infrastructure as an initial enabler for operations planning—either to capture and ingest data more frequently or to host new, complex analytics solutions. Instead, operations planning solutions can run infrequently, use small-scale data, and typically be implemented alongside and connected to existing tools and systems. In this way, these solutions are complementary to common end-to-end planning cloud solutions and maximize the value organizations can extract from existing resources.
In a recent case study, the large mining player developed an optimization-based process to improve the supply chain of its trains, vessels, and mine operations over short- and medium-term horizons. The process was aligned with the company’s strategic target regarding customer specifications and overall production goals. Embedding the optimization process into overall decision making allowed the company to improve operations significantly. This move alone captured many millions of dollars in cost savings.
In a world characterized by increasing volatility and major disruptions, the maturity of operations planning has the potential to increase the performance spread between first movers and laggards. So far, bigger corporations have taken the lead in implementing approaches to optimize their operations planning, given the resources and capabilities required. Whether optimization is within the reach of all companies is still an open question.
Hossein Aghai-Khozani is a consultant in McKinsey’s Munich office, where Sebastian Reiter is a partner; Simon Bull is a consultant in the Copenhagen office; Valerio Dilda is a senior partner in the Paris office; and Lapo Mori is a partner in the Denver office.
The authors wish to thank Eddie Elizondo, Shailesh Lekhwani, and Tarusha Moonsamy for their contributions to this article.
Explore a career with us
Related articles.
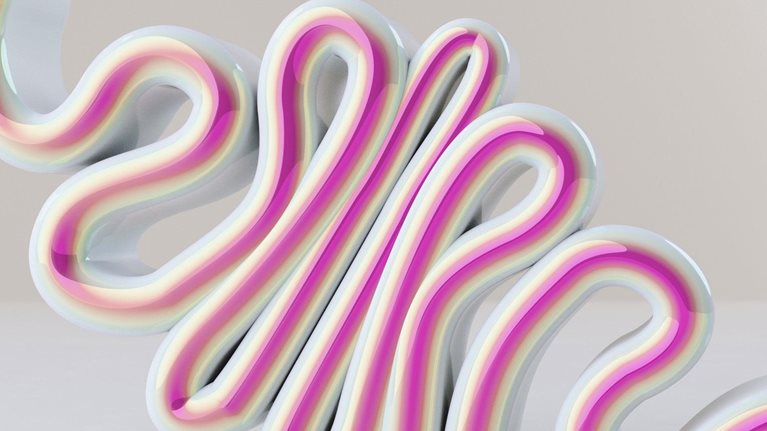
Building value-chain resilience with AI
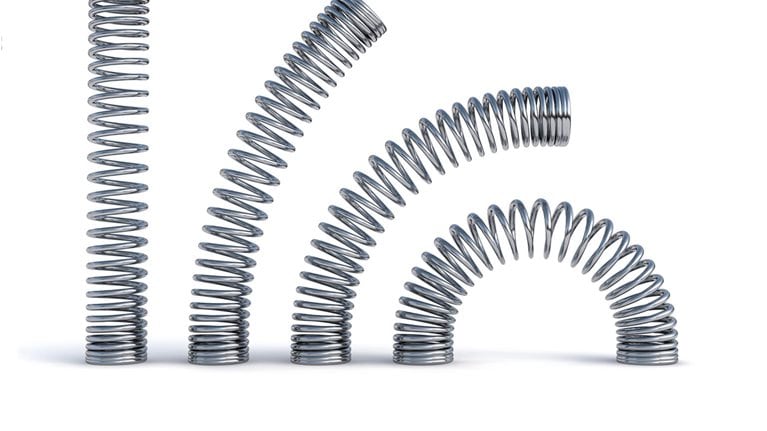
A better way to drive your business
ThoughtSpot Analytics
Deliver insights 10x faster for your employees
AI-Powered Analytics for your modern data stack
Create insights in seconds with AI-powered search
Create insights in seconds using natural language search
Live-query your data cloud or lake in a few clicks
Build search data models in ThoughtSpot
Govern & Secure
Balance self-service with enterprise-scale control
Keep a finger on the pulse of your business with Liveboards
Augmented Analytics
Surface actionable insights fast with AI
- Operationalize
Schedule and sync cloud data directly to business apps
Know what's happening and why, wherever you are
Spreadsheets
Search to create visualizations from data in Microsoft Excel and Google Sheets
New Features
Stay up to date with the latest product news and releases
ThoughtSpot Embedded
Drive higher app engagement with embedded analytics
Embed engaging self-service analytics experiences into any app
Embed analytics with a few lines of code
Get a jumpstart to building ThoughtSpot apps and API services
Developer Playground
Explore ThoughtSpot Embedded’s SDK and APIs
Get the latest new features and enhancements in ThoughtSpot Embedded
Generate more revenue with embedded analytics

Gartner 2024 Magic Quadrant™ for Analytics and BI Platforms

Interactive Product Tour
Experience the power of Search and Liveboards for yourself
- Business Leader
- Data Leader
- Product Leader
By Industry
- Financial Services
Retail & E-commerce
Manufacturing & Logistics
Healthcare & Life Sciences
Media & Communications
- Procurement
- Public Sector
By Department
- ServiceNow Analytics

See Mode and ThoughtSpot bring data and business teams together
Demo Videos
The Data Chief
Data Trends
- Documentation
Analyst Reports
White Papers
Case Studies
Solution Briefs
User Groups
- Professional Services

Meet the future of analytics in our live weekly demo sessions.
Support Center
- Atlas Marketplace
In The News
Press Releases
Technology Alliances
Channel Partners
System Integrators
All Partners

Experience AI Analytics yourself with our interactive product tour.
Supply chain analytics explained: how it works and top use cases.

Supply chain analytics can be complex and difficult to understand for some companies. For any business producing a product or service, they must procure various raw materials and component parts, manage their inventories and production processes, and ultimately get a high-quality product to their end customer in a timely manner.
However, a supply chain is rarely static, and things can change fast. This is something we’re all keenly aware of coming out of COVID-19 induced market instability—a period that saw unprecedented supply chain failures and shipping delays across almost every industry. This situation highlights the importance of supply chain data. Those who had a view of their entire supply chain could make data-driven decisions, pulling away from their competition and driving success for their organization.
But what exactly is supply chain analytics, and how can you use them to join the ranks of successful businesses? Keep reading to find out.
What is supply chain data analytics?
Supply chain data analytics is the computational analysis of the systems and processes involved in a company’s supply chain. This involves integrating and evaluating data from all parties involved in producing a good of service—including suppliers, distributors, shipping providers, warehouses, and more.
By integrating data from various data sources such as your inventory management and procurement systems, analysts can study how materials or component parts are being sourced, assembled, transported, and ultimately provided to an end consumer as a finished product or service.
Once integrated and centralized, your data can then be processed and displayed through data visualizations. These visualizations help users understand the relationship between these systems and processes, showing what’s happening, why it’s happening, and potential actions that will make the overall supply chain more efficient.
Why is supply chain analytics important?
We’ve already discussed the complexity, unpredictability, and volatility of supply chains. With supply chain data analytics, companies can monitor and analyze these complex structures, adjusting processes and operations to optimize the supply and demand relationship with high-quality, on-time, and lean production.
For example, many manufacturing companies are dependent on raw materials that fluctuate in price and supply over time due to broader macroeconomic, environmental, and weather conditions. Due to elements like seasonality, weather, or other factors that are out of the control of the manufacturer, customer demand for the end product can also fluctuate independently of the raw materials.
Having supply chain data analytics systems in place can help businesses understand how these different supply and demand conditions affect factors such as inventory levels, delivery timeliness, and labor needs so that management can adjust operations in response to — or ideally in preparation for — these changes.
How supply chain analytics works
Supply chain data analytics is a process that involves:
Capturing data from multiple sources
Integrating that disparate data
Analyzing the results through ad hoc analyses , visualizations, and reports.
Since most supply chains involve a network of different organizations and business departments, there are typically numerous different systems that are producing relevant data.
For example: Let’s say your business orders raw materials from different providers. You will need to reconcile and link your internal inventory data with your external vendors’ various systems and the relative shipping providers. These connections will empower you to track how component parts and raw materials are flowing into your warehouses and production centers.
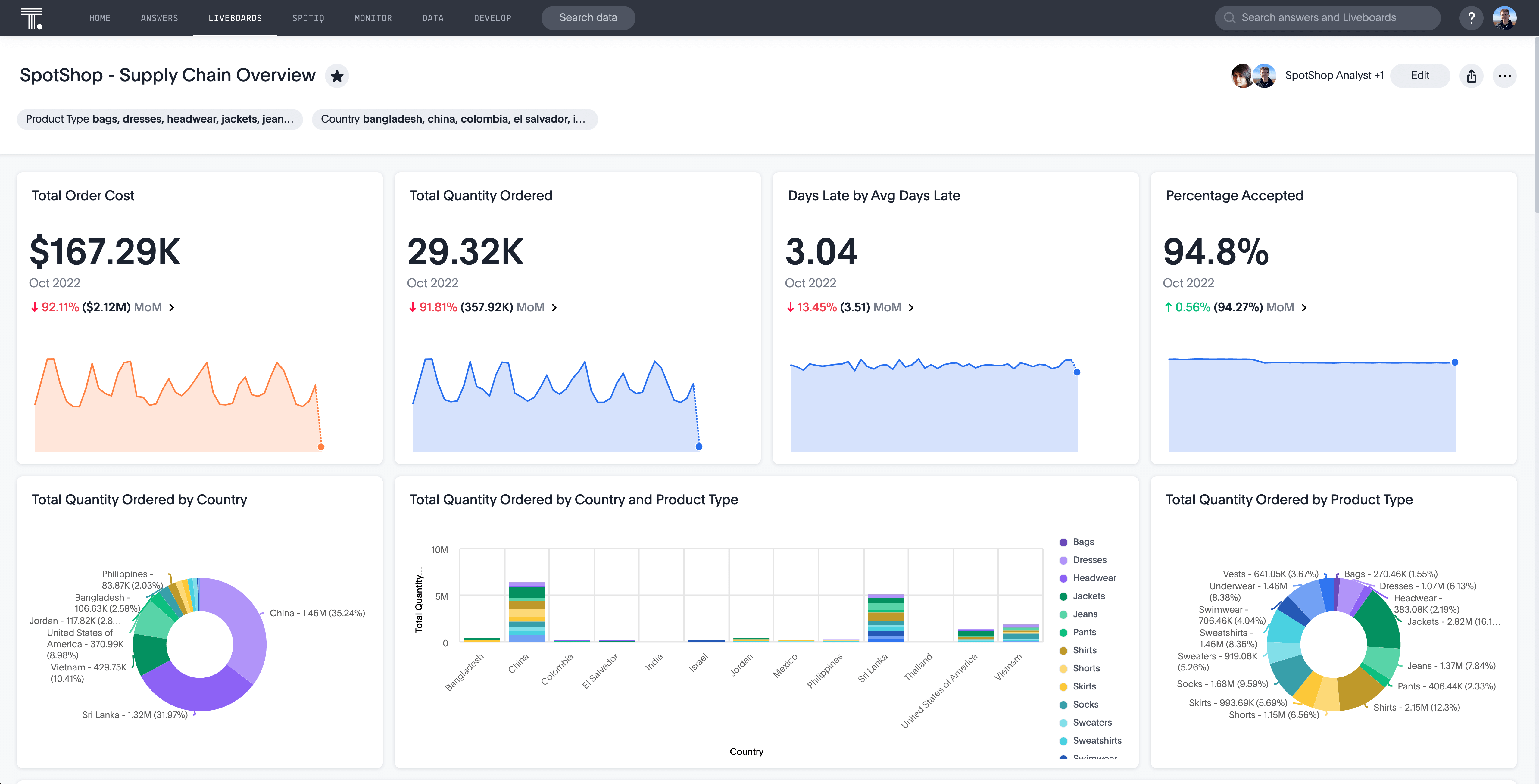
However, integrations alone are rarely sufficient for starting an analysis. In order to provide value, data needs to be cleaned , standardized, and integrated so that it can be easily queried and reported on. This is particularly the case when pulling data from different companies, as they likely have different standards for how data is structured, how products are identified, and how the data interfaces are exposed. Data processing pipelines allow businesses to take these varying standards and bring them into a consistent format for analysis.
Once the data is integrated and processed into a centralized repository, analysts can build out models, visualizations, and reports—giving supply chain professionals the opportunity to explore the data directly with modern business intelligence tools. Now that both teams have visibility into their data, they can draw inferences about the health of their supply chain.
5 types of supply chain analytics
Supply chain analytics is a broad stroke—using data to find out what happened, why it happened, and to predict the future state if you do, or don’t, take a specific action. That said, you can actually break supply chain analytics into five different types of data analytics :
Descriptive analytics
Descriptive analytics is the process of interpreting historical data. The result is often used to answer the questions “what happened?” or “what is happening?” in a business’s operations.
In the context of the supply chain, this involves understanding the historical state of everything from inventory levels to delivery times to consumer reviews. These different pieces can be viewed over time to see how they fluctuate or are changing over time.
Diagnostic analytics
Diagnostic analytics takes descriptive analytics one step further and tries to understand the relationship between various data points. More broadly, it answers the “why” of the historical data that descriptive analytics surfaces.
For example, consider if your descriptive analytics shows low inventory levels unable to meet customer demand and slow supplier deliveries, Your diagnostic analytics would try to determine if the slow deliveries caused a shortage in some key component, resulting in insufficient product production.
Predictive analytics
Predictive analytics uses statistical analysis, often computing correlations between various datasets to determine some type of causal relationship, to make projections about the future.
To continue with our supply chain example, let’s say the diagnostic analytics determined that the slow deliveries did cause the low inventory. Predictive analytics would build on key indicators from this example in order to flag potential problems in the future.
Maybe, for example, colder months of the year cause road problems that lead to frequent delivery delays. By running a statistical analysis between the delays and local weather data, analysts can determine if there’s a strong correlation between the two and decide whether it warrants a further investigation to determine causality.
Prescriptive analytics
Prescriptive analytics uses the analysis of predictive analytics to determine potential actions based on the projections. Rather than just showing the plain facts, this type of analysis draws broader meaning about the data so business leaders can solve issues before they arise.
Let’s continue with our previous example. After confirming the strong correlation between cold weather and delivery delays due to road problems, the next question is how we fix this. Analysts can run simulations with proposed solutions to see how they affect key metrics , like delivery timeliness.
Perhaps another shipping method is more reliable in the winter, or the company could boost inventory in preparation for winter delays. Data analysis and modeling can help to automate and test these scenarios before operators decide to invest in the ultimate solution.
Cognitive analytics
Cognitive analytics is the use of machine learning and AI to make sense of complex and interconnected data. This type of analytics effectively runs predictive and prescriptive analytics models on wide ranges of datasets, trying to understand relationships that would otherwise be infeasible for a human to manually experiment on.
For instance, a cognitive analytics model may try to map the delivery delay and inventory planning data from various data sources to find unexpected relationships. This analysis could uncover a strong correlation with rising natural gas prices in the winter. This discovery might lead analysts to hypothesize that the suppliers’ input costs are putting a seasonal strain on their businesses. Without being able to run these broad types of regression analyses, it would be very difficult for a human analyst to navigate these seemingly random relationships.
Features of effective supply chain analytics
While there are so many different types of businesses and supply chain structures, there are a few standard features that almost every effective supply chain analytics system has::
Data visualizations
Data visualizations are used by analysts to represent raw datasets in an easy-to-grasp, visual format. For example, bar charts, histograms, and line graphs are all examples of visualizations that you can create to show how different parts of a supply chain are operating.
Supply chains consist of sensitive operational data about a business. Therefore, it’s important to use industry-standard security practices and ensure your data is access controlled.
Comprehensive analysis
Supply chains aren’t just about the raw materials and shipping providers. Any data-producing element of the process should be integrated to ensure that analyses calculate all relationships and correlations that ultimately impact strategic business decisions.
Digital modeling of the supply chain
The physical supply chain processes should be replicated in a digital modeling system—often referred to as the “ digital twin ”—so analysts can easily iterate on and experiment before the business makes material investments in adjusting the physical systems.
Internal and external data integration
In addition to internal integrations that pull data from things like inventory management systems, analysts should pull information from external sources that affect their supply chain, such as public weather datasets or feeds from social media APIs.
Intuitive, collaborative access
While data needs to be secured and access controlled, it should also be easy to share amongst analysts to help with the collaborative and iterative process of improving models and visualizations. In addition to increasing creativity, it’ll reduce the duplication of efforts, particularly with data pipelines that are often generalizable.
Uses of supply chain analytics
Supply chain analytics is relevant to nearly all types of businesses, as its purpose is simply to understand and drive action for operations around producing a product or service. Here we’ll explore some common examples of how businesses can leverage supply chain analytics to optimize inventory , improve production quality, reduce stockout costs , and hedge raw material costs.
Project future demand to optimize inventory levels
Consumer demand for products can vary widely between businesses. For instance, some businesses are highly seasonal and can have big spikes in different times of year or around holidays, while others may be much more consistent over time.
At scale, there’s often sufficient data to project demand into the future using statistical analyses of sales data. By doing so, the business can understand what upcoming sales forecasts may look like to ensure there’s sufficient inventory in place while keeping costs low by not producing more than is practically needed.
That was also the case for new-comer airline, Flyer. As they looked to disrupt a legacy industry, they knew that understanding and executing on demand would be the key to their success. That’s why they invested in supply chain analytics early, and that investment paid off. In just one quarter, they were able to increase revenue by 313%.
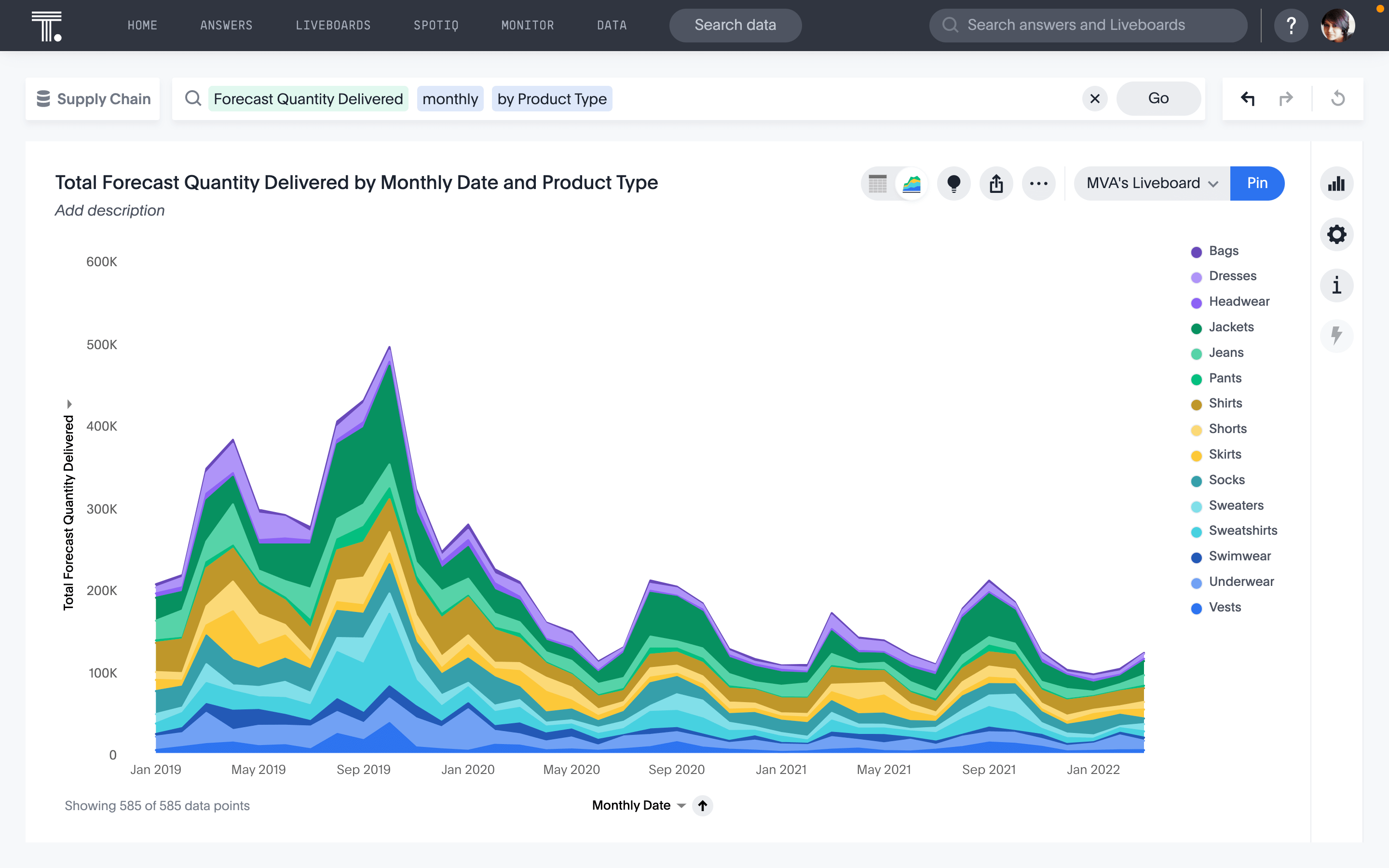
Understand production quality
End customers provide a lot of actionable data through online reviews, support phone calls, social media posts, and much more. Much of this data is available to companies to integrate and aggregate so that analysts can start to stitch together common complaints or feedback.
This customer feedback data can then be used to understand potential issues with product quality or even ways the product could be modified to better fit consumer needs. Ultimately, the end consumer’s opinion on a product or service is what matters most. So, understanding their experience is invaluable.
As a SaaS provider, you can also leverage this data as an added value for your customers. That’s the case for OrderPay, a London-based hospitality and restaurant payment solution trusted by over 2600 businesses in the UK. With inflation, soaring energy costs, price hikes, and staffing shortages, OrderPay’s customers wanted to get answers to detailed data questions about performance and customer behavior to make smart, profitable decisions.
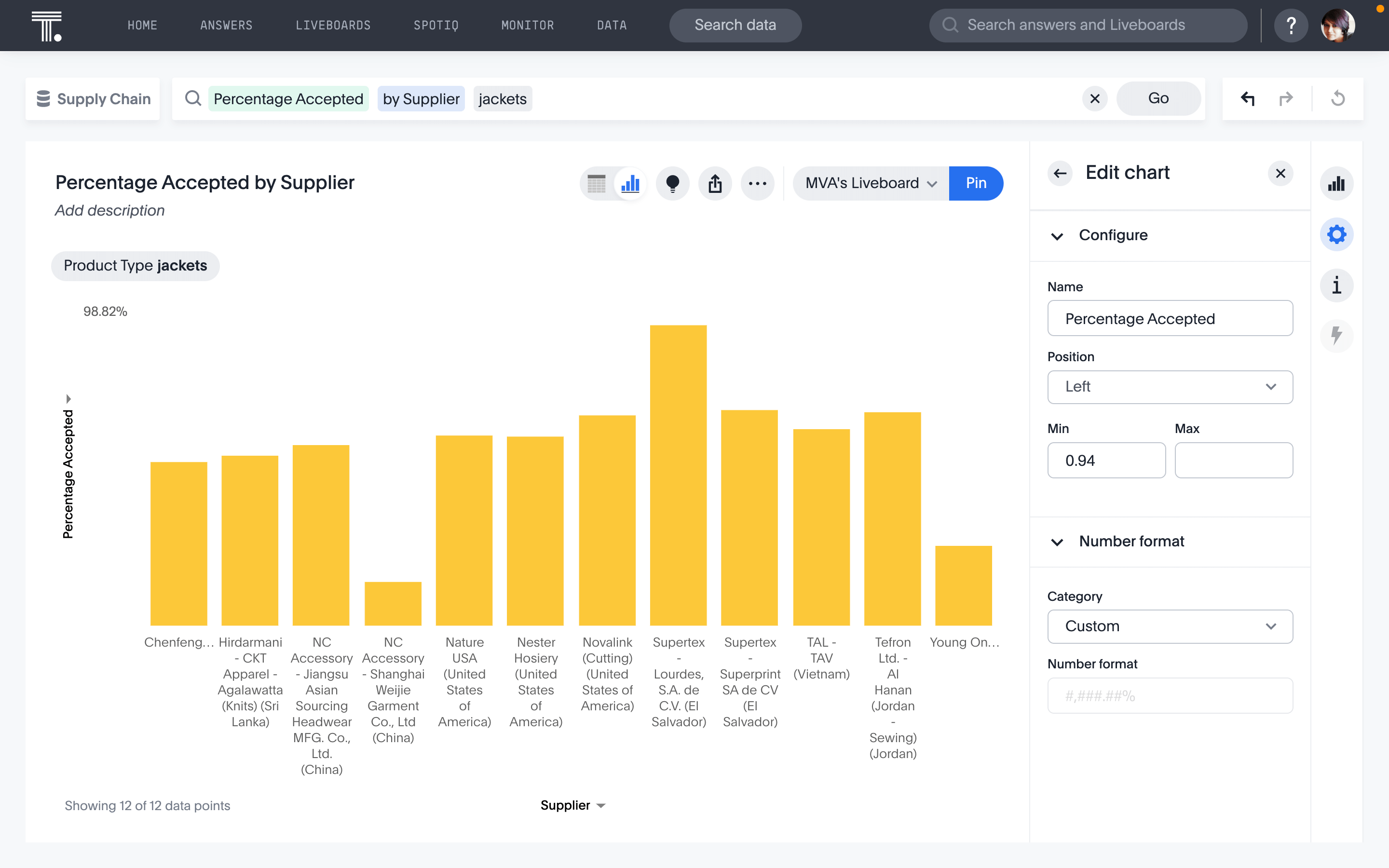
Model commodity pricing
For many businesses that rely on standard commodities like oil or grain, there can be a lot of fluctuation in pricing of necessary raw materials due to macroeconomic conditions that are out of the control of the business. Many businesses use historical data about things like prices and weather to predict what may happen to their costs so that they can hedge them with financial instruments.
For instance, it’s very common for airlines to lock-in their oil prices using futures contracts — where they commit to buy at a specified price and time in the future. While it’s certainly difficult to predict the way that a commodity is going to move, this practice has the added benefit of allowing businesses to know exactly what their costs are going to be over the duration of that contract and plan accordingly rather than being exposed to market fluctuations.
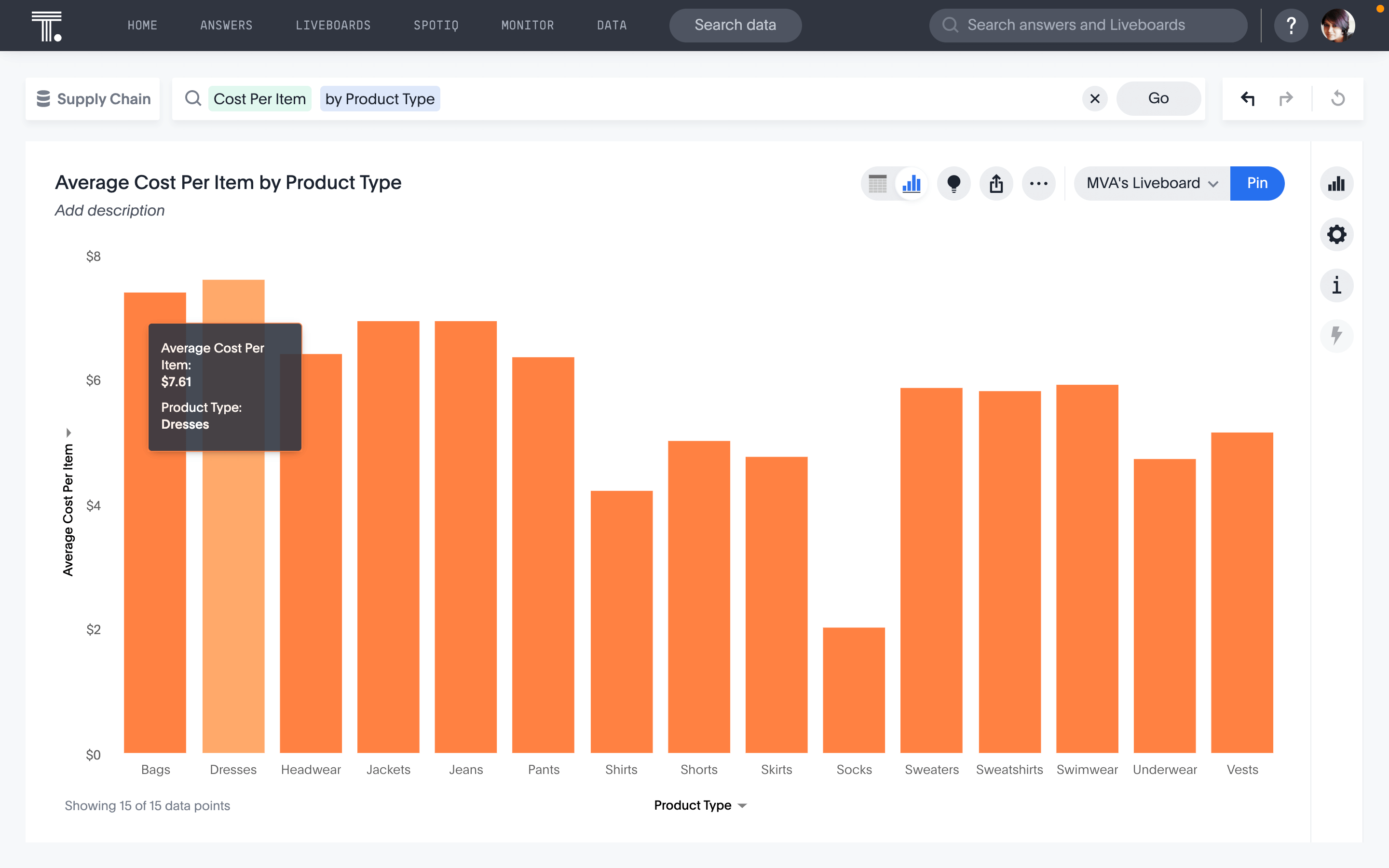
Improve your supply chain today
Supply chain analytics allows businesses to integrate and analyze all of the data related to their supply chains to better understand what is going on and how it can be improved to drive better efficiencies. However, doing so requires a robust analytic stack that can integrate disparate data sources, aggregate them using secure data pipelines , and build reports and data visualizations in a collaborative manner.
ThoughtSpot can power your supply chain analytics efforts. See for yourself—try a free trial of ThoughtSpot today.
ThoughtSpot is the AI-Powered Analytics company that lets everyone create personalized insights to drive decisions and take action.
- ThoughtSpot Sage
- Auto Analyze
- Govern & Secure
- New features
- Retail & E-commerce
- Manufacturing & Logistics
- Healthcare & Life Sciences
- Media & Communications
- Partner Directory
- Request Demo
(800) 508-7008
Stay in Touch
Get the latest from ThoughtSpot
Exclusive One-Time Offer: Act Now and Save $413.8
Already on SCMDOJO? Log in
I accept SCMDOJO'S Terms of Use and Privacy Notice .

Walmart Supply Chain: Building a Successful Integrated Supply Chain for Sustainable Competitive Advantage
- Case Studies
Introduction
The global business landscape has witnessed an increasingly fierce competition, pushing companies to seek effective strategies to maintain and enhance their competitiveness. Among these strategies, the role of supply chain capability stands out as a key factor in driving success. A well-optimized supply chain not only ensures efficient delivery and cost-effectiveness but also provides companies with a competitive advantage in the market. In this context, Walmart, the world’s largest retailer, has demonstrated a highly successful and integrated Walmart supply chain, propelling its growth and dominance in the retail industry.
This case study aims to delve into the significance of supply chain capability for enhancing a company’s competitiveness and how it serves as a competitive advantage for companies. Additionally, we will explore the imperative need for supply chain redesign in the global economy to adapt to the challenges of the modern era of globalization. Focusing on Walmart’s exemplary supply chain practices, the purpose of this case study is to analyze the features of its successful integrated supply chain while identifying relevant issues in the context of the current globalized market.
[Read More: Rivian: Navigating Supply Chain and Operational Challenges and Embracing Growth ]
Walmart’s Supply Chain: Integrated Supply Chain Success
Data-driven success factors.
In the realm of modern supply chain management, data-driven strategies play a pivotal role in enhancing a company’s competitiveness. Walmart’s remarkable success as the world’s largest retailer can be attributed to its astute utilization of data analysis and advanced technologies within its integrated supply chain. This section delves into the key data-driven success factors that have propelled Walmart’s supply chain to the forefront of the retail industry.
[Read More: ERP Master Data: A Guide to Improve Quality & Governance ]
Role of Data Analysis through Barcode Scanning and Point-of-Sale Systems
Data analysis is at the core of Walmart’s supply chain prowess. The company has implemented sophisticated barcode scanning and point-of-sale systems to collect real-time data from its stores. By employing these technologies, Walmart gains valuable insights into customer buying behavior, sales trends, and inventory levels. The ability to analyze this data enables the retail giant to make informed decisions on product procurement, inventory management, and demand forecasting.
Efficient Supply Chain Practices: Automated Distribution Centers and Computerized Inventory Systems
Automation is a key component of Walmart’s efficient supply chain practices. The company has strategically invested in automated distribution centers, streamlining the flow of products from manufacturers to stores. These automated facilities not only optimize the handling and movement of goods but also enable faster order fulfillment and replenishment. Additionally, computerized inventory systems provide Walmart with accurate and up-to-date information about stock levels, allowing for precise inventory control and reducing the risk of stockouts or excess inventory.

Utilizing Walmart’s Own Trucking System and Cross-Docking Logistics
Another critical factor contributing to Walmart’s supply chain success is the utilization of its private trucking system and cross-docking logistics. By maintaining its own trucking fleet, Walmart gains greater control over transportation and delivery schedules, leading to improved efficiency and timely product replenishment. Furthermore, the adoption of cross-docking logistics techniques has enabled Walmart to minimize the need for intermediate storage, leading to reduced handling costs and faster product movement through the supply chain.
[Read More: The Ultimate Guide to Contract Logistics: What You Need to Know ]
Information Technologies Driving Efficiency
In Walmart’s journey towards becoming a global leader, information technologies have played a pivotal role in driving efficiency within the integrated Walmart supply chain. The retail giant has strategically adopted various IT initiatives to optimize its operations, enhance collaboration with suppliers, and achieve real-time inventory targeting. These technologies have contributed significantly to Walmart’s supply chain success, allowing them to maintain a competitive edge in the retail industry.

Collaborative Planning, Forecasting, and Replenishment (CPFR)
One of the key information technologies that have bolstered Walmart’s supply chain efficiency is the implementation of Collaborative Planning, Forecasting, and Replenishment (CPFR). This system facilitates seamless communication and coordination between Walmart and its supply chain partners, including suppliers and distributors. By sharing real-time sales data and demand information, CPFR enables accurate forecasting and demand planning, minimizing information distortion, and promoting synchronized inventory replenishment. The CPFR program has been instrumental in enhancing overall supply chain visibility and efficiency, allowing Walmart to respond promptly to fluctuations in demand and supply, reducing stockouts, and optimizing inventory levels.
Vendor-Managed Inventory (VMI) and Its Benefits
Walmart’s adoption of Vendor-Managed Inventory (VMI) has been another critical information technology-driven initiative. Through VMI, Walmart empowers its suppliers to take on the responsibility of managing their inventory stored in Walmart’s warehouses. By granting suppliers access to real-time inventory data and sales information, Walmart facilitates efficient inventory tracking and replenishment. This hands-on approach by suppliers results in streamlined inventory management, reduced delays in replenishment, and lower stockouts. The VMI model has proved particularly advantageous for Walmart due to its vast product range and numerous suppliers, making inventory management complex and costly if managed solely by the retailer.
[Read More: Vendor Managed Inventory: A Comprehensive Guide ]
Leveraging RFID Technology for Real-Time Inventory Targeting
RFID (Radio Frequency Identification) technology has been a game-changer in Walmart’s pursuit of real-time inventory targeting and enhanced supply chain visibility. By employing RFID tags on products, Walmart can track the movement of inventory throughout the supply chain in real-time. RFID enables accurate and automated inventory tracking, reducing the need for manual counting and minimizing errors in inventory management. The technology also provides crucial details, such as production time, location, and expiry dates of goods, allowing for efficient inventory targeting and better control over inventory turnover. RFID technology has been instrumental in Walmart’s cost reduction efforts, ensuring optimal stock levels while avoiding overstocking and unnecessary inventory holding costs.
Achieving Competitive Advantage through Strategy
Walmart’s competitive strategy: “everyday low prices” (edlp).
Walmart’s competitive advantage is deeply rooted in its strategic focus on offering “Everyday Low Prices” (EDLP) to its customers. The EDLP strategy revolves around providing high-quality products and services at the lowest possible prices, ensuring that customers can benefit from affordable prices every day. This approach sets Walmart apart from its competitors and has been instrumental in establishing the company as a dominant force in the retail industry.
Implementing the “Everyday Low Costs” (EDLC) Policy through Direct Procurement
To support its EDLP strategy, Walmart follows an “Everyday Low Costs” (EDLC) policy in its supply chain management. One of the key elements of the EDLC policy is the direct procurement of items from suppliers, eliminating intermediaries in the process. By procuring directly from manufacturers, Walmart can negotiate and understand their cost structure, enabling them to make informed purchasing decisions and obtain the best prices for their products.
Walmart’s emphasis on direct procurement is further bolstered by the use of technology and information systems. The company has implemented a central database, store-level point-of-sale systems, and a satellite network, along with barcodes and RFID technology as previously mentioned. These technologies allow Walmart to gather and analyze real-time store-level information, including sales data and external factors like weather forecasts, to enhance the accuracy of purchasing predictions. This integration of information technology helps Walmart optimize its procurement process and maintain low costs throughout the supply chain.
Utilizing Information Systems for Better Inventory Management
Effective inventory management is critical for Walmart to sustain its competitive advantage through the EDLP strategy. The company relies on information systems and information technology (IT) capabilities to control inventory levels efficiently. By capturing customers’ demand information, Walmart can identify popular products and stock them adequately, leading to an overall reduction in inventory.
One notable example of Walmart’s successful utilization of information systems is its collaboration with Procter & Gamble (P&G) through the Collaborative Planning, Forecasting, and Replenishment (CPFR) program. This program links all computers of P&G to Walmart’s stores and warehouses, allowing for efficient replenishment orders based on real-time inventory needs. Additionally, Walmart’s Retail Link , developed in the early 1990s, serves as another vital IT application for storing data, sharing it with vendors, and aiding in shipment routing assignments.

Challenges and Opportunities
Supplier cooperation and collaboration.
Walmart’s supply chain success can be attributed to its strong relationships with suppliers, but achieving and maintaining supplier cooperation and collaboration is not without challenges. Let’s explore the challenges and opportunities in this area:
Challenges in Obtaining Suppliers’ Cooperation
- Supplier Resistance to Direct Procurement: Walmart follows an “Everyday Low Costs” (EDLC) policy by directly procuring items from suppliers, eliminating intermediaries. However, some suppliers may be reluctant to cooperate with this approach as it can disrupt existing distribution channels and potentially reduce their bargaining power.
- Complex Supplier Networks: With thousands of suppliers across various product categories, managing diverse supplier networks can be challenging. Each supplier may have different production and delivery schedules, making coordination difficult.
- Balancing Profit Margins: As Walmart emphasizes low prices, maintaining a balance between cost savings and ensuring suppliers’ profitability can be a delicate task. Suppliers may resist pressure to reduce prices further to maintain their margins.
Opportunities for Enhanced Supplier Cooperation and Collaboration
- Establishing Transparent Communication Channels: Walmart can create transparent and open communication channels with its suppliers to foster better cooperation. Clear communication regarding demand forecasts, inventory levels, and potential disruptions can help suppliers plan their production and deliveries more efficiently.
- Supplier Incentive Programs: Introducing incentive programs that reward suppliers for meeting certain performance metrics, such as on-time delivery or cost reduction, can motivate suppliers to actively collaborate and improve their supply chain capabilities.
- Collaborative Planning, Forecasting, and Replenishment (CPFR): Walmart can leverage technology, such as CPFR, to share real-time sales data and demand forecasts with its suppliers. This collaborative approach allows suppliers to align their production and inventory management with actual market demand, reducing the bullwhip effect and optimizing the supply chain.
- Sharing Inventory Visibility: Providing suppliers with access to inventory data, including stock levels and sales information, can help them plan production and deliveries more effectively. This visibility can prevent stockouts and overstocking issues.
- Long-term Partnerships: Building long-term strategic partnerships with key suppliers can create a sense of mutual commitment and trust. By assuring consistent business over an extended period, Walmart can foster stronger relationships and supplier loyalty.
[Read More: 3 Types of Supplier Segmentation Matrix You Can Use to Classify Suppliers ]
Importance of Collaboration to Enhance Supply Chain Efficiency
- Reducing Lead Times: Effective collaboration with suppliers can help shorten lead times by streamlining production and transportation processes. Faster lead times enables Walmart to respond quickly to changes in demand, reducing the risk of stockouts.
- Efficient Inventory Management: Collaborative efforts with suppliers enable better inventory planning and management. Suppliers can adjust production based on actual demand, reducing excess inventory and associated costs.
- Supply Chain Flexibility: Collaboration fosters agility and adaptability in the supply chain. When Walmart and its suppliers work together closely, they can quickly adjust to market changes, supply disruptions, or new opportunities.
- Cost Reduction: Improved supplier collaboration can lead to cost-saving opportunities. By eliminating unnecessary intermediaries and optimizing production and transportation, overall supply chain costs can be minimized.

The Incentives Alignment Issue
In any supply chain, maintaining a balance of profit margins among different parties is essential for efficient collaboration and sustained success. However, achieving incentives alignment can be challenging, and this issue is particularly relevant in the case of Walmart supply chain. Addressing misalignment of interests between Walmart and its suppliers is crucial for optimizing the overall performance of the supply chain and ensuring long-term success. The following points highlight the incentives alignment issue faced by Walmart:
1. Balancing Profit Margins Among Different Supply Chain Parties:
Walmart’s success is attributed to its ability to offer high-quality products and services at the lowest affordable prices. To achieve this, Walmart employs various cost-cutting strategies, such as direct procurement from suppliers and streamlined distribution practices. While these strategies help Walmart maintain competitive prices, they can create challenges for suppliers who may face pressure to lower their own profit margins to meet Walmart’s demands. This misalignment of profit margins can lead to strained relationships and potentially impact the overall efficiency of the supply chain.
2. Misalignment of Interests Between Walmart and Suppliers:
Walmart’s size and market dominance can lead to power imbalances in supplier relationships. Suppliers may feel compelled to comply with Walmart’s demands to maintain access to its large customer base. However, this can lead to situations where suppliers may not have enough leverage to negotiate favorable terms, impacting their own profitability. As a result, suppliers may be less inclined to invest in innovations or improvements that would benefit the supply chain as a whole.
3. Conflict Between Inventory Growth and Sales Growth:
Walmart faced inventory growth issues in the past, with the inventory growth rate outpacing the sales growth rate. This can be indicative of conflicting incentives between Walmart and its suppliers. Suppliers may prioritize producing and delivering more inventory to ensure they meet Walmart’s demands, even if the sales growth does not keep up with the increased inventory. This misalignment can lead to excess inventory, increased carrying costs, and potential stockouts.
4. The Need for a New Triple-A Supply Chain:
Addressing the incentives alignment issue requires a fundamental shift in the supply chain strategy. Lee (2004) proposed the concept of a new Triple-A supply chain for Walmart and other companies in the 21st century. The Triple-A supply chain emphasizes agility, adaptability, and alignment to create a sustainable competitive advantage. Achieving alignment among all participating parties is crucial to optimize supply chain performance and ensure that risks and rewards are distributed fairly.
The Triple-A Supply Chain Approach
In today’s competitive business landscape, companies like Walmart recognize that a successful supply chain is not just about having a fast and cost-effective system. To maintain a sustainable competitive advantage and address the challenges of the global economy, it is essential to redesign supply chains that incorporate agility, adaptability, and alignment. This section explores the concept of the Triple-A Supply Chain Approach, which emphasizes these three key qualities that an ideal supply chain should possess: agility, adaptability, and alignment of interests among all participating parties.
The Three Qualities of an Ideal Supply Chain
Agility for quick and cost-effective responses:.
Agility refers to a supply chain’s ability to respond quickly and cost-effectively to sudden changes in demand, supply, and external disruptions. In the fast-paced business environment, companies must be able to adapt swiftly to fluctuations in customer preferences, market conditions, and unforeseen events. For Walmart, agility has been a critical factor in maintaining its leadership position in the retail industry. The company’s investments in technology and supply chain optimization strategies have allowed them to optimize inventory levels and respond rapidly to changing customer demands, ensuring the availability of products while minimizing inventory costs.
Adaptability to Handle Changes in Demand and Supply:
Supply chains should be adaptable and flexible enough to handle variations in demand and supply patterns. Demand forecasts can be uncertain, and unexpected supply chain disruptions may occur, making adaptability a vital quality. Walmart’s focus on omnichannel and various fulfillment options, such as in-store pickup and ship from store, demonstrates their commitment to adaptability. By utilizing multiple channels, Walmart can cater to diverse customer preferences, ensuring an uninterrupted flow of products to meet demand.
Alignment of Interests among All Participating Parties:
One of the significant challenges in supply chain management is ensuring alignment of interests among all parties involved, including suppliers, manufacturers, distributors, and retailers. Walmart’s scale and dominance in the retail market have allowed them to establish strong relationships with vendors, enabling strategic partnerships with vendors who can meet their high-volume demands. Additionally, Walmart’s adoption of Vendor Managed Inventory (VMI) allows suppliers to manage their own inventory stored in Walmart’s warehouses. This collaboration aligns the incentives of suppliers and Walmart, streamlining inventory management and ensuring timely replenishment.

In conclusion, Walmart’s integrated supply chain has been a crucial factor in the company’s global dominance and sustained competitive advantage. By strategically investing in technology and optimizing its supply chain, Walmart has managed to maintain its position as the world’s largest retailer with over $572 billion in revenue in 2022.
Walmart’s success serves as a compelling example of the importance of a well-integrated supply chain in achieving and sustaining competitive advantage in the global market. As businesses continue to navigate the complexities of the 21st-century economy, building and enhancing supply chain capabilities will remain a critical aspect of ensuring sustainable growth and profitability. By prioritizing agility, adaptability, and alignment, companies can follow in Walmart’s footsteps and position themselves for continued success in the dynamic and ever-evolving global marketplace.
References:
- Lee H.L. (2004): The triple A supply chain. “Harvard Business Review”, Vol. 82, No. 10, pp. 102-112.
- Nguyen T.T.H. (2017): Wal-Mart’s successfully integrated supply chain and the necessity of establishing the Triple-A supply chain in the 21st century. “Journal of Economics and Management”, Vol. 29(3), pp. 102-117
About the Author – Dr Muddassir Ahmed
Dr MuddassirAhmed is the Founder & CEO of SCMDOJO. He is a global speaker , vlogger and supply chain industry expert with 17 years of experience in the Manufacturing Industry in the UK, Europe, the Middle East and South East Asia in various Supply Chain leadership roles. Dr. Muddassir has received a PhD in Management Science from Lancaster University Management School. Muddassir is a Six Sigma black belt and founded the leading supply chain platform SCMDOJO to enable supply chain professionals and teams to thrive by providing best-in-class knowledge content, tools and access to experts.
You can follow him on LinkedIn , Facebook , Twitter or Instagram .
- Walmart Walmart Supply Chain

Download Indirect Procurement Assessment
An Indirect Procurement Best Practices Assessment is a valuable process for organisations to evaluate and optimise their indirect procurement functions.
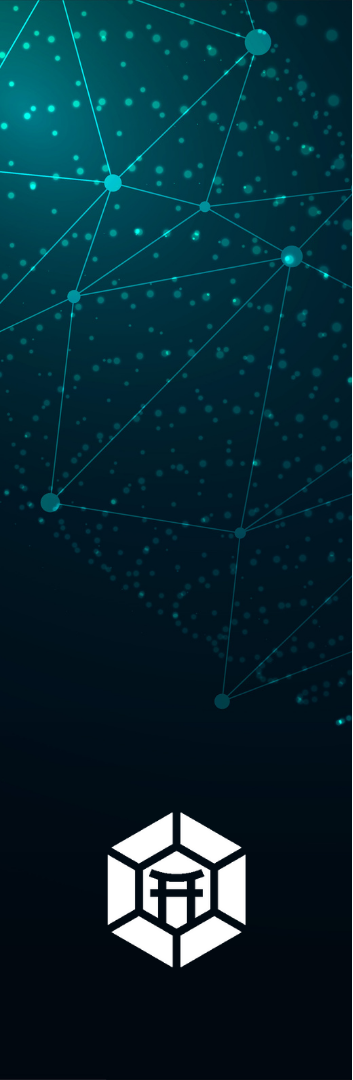
Discover the Top 75 Free Courses for August
Udemy Announces Layoffs Without Saying ‘Layoffs’
Udemy’s latest ‘Strategic Business Update’ uses corporate euphemisms to signal job cuts while pivoting to enterprise clients.
- 7 Best Sketch Courses for 2024
- 8 Best Free Geology Courses for 2024
- 7 Best Climate Change Courses for 2024: Exploring the Science
- [2024] 110+ Hours of Free LinkedIn Learning Courses with Free Certification
- 7 Best Free Haskell Courses for 2024
600 Free Google Certifications
Most common
- data science
Popular subjects
Cybersecurity
Digital Skills
Popular courses
Bioseguridad y equipo de protección para la prevención de COVID-19
Happier Employees and Return-On-Investment Course
Understanding the Brain: The Neurobiology of Everyday Life
Organize and share your learning with Class Central Lists.
View our Lists Showcase
Class Central is learner-supported. When you buy through links on our site, we may earn an affiliate commission.
Case Study: Supply Chain Analytics in Power BI
via DataCamp Help
- The Buy Option
- In this first chapter, you will learn about the make and buy options in a supply chain. Then you will become acquainted with the concepts of extended cost and full cost. You will learn how to calculate these with a basic quote dataset.
- Creating a Volume Parameter and Scenario Analysis
- In this chapter, you will explore building a scenario analysis tool to examine how your cost calculations change as volumes change. You will create visualizations of how the full cost changes across the different potential production volumes.
- The Make Option
- You will learn how to assess internal costs manufacturing costs - the "Make" option in the Make versus Buy. You will also learn how to communicate your results.
Nicholas Switzer
Related Courses
Supply chain analytics, case study: ecommerce analysis in power bi, case study: supply chain analytics in tableau, supply chain management and analytics, supply chain analytics essentials, case study: hr analytics in power bi.
Select rating
Start your review of Case Study: Supply Chain Analytics in Power BI
Never Stop Learning.
Get personalized course recommendations, track subjects and courses with reminders, and more.

Supply Chain Analysis: Data Analysis Case Study Using Excel
A supply chain analysis project using microsoft excel and microsoft power bi..

Supply chain analytics plays a crucial role in driving data-driven decision-making across industries, including manufacturing, retail, healthcare, and logistics. In this report, I presented an analysis of a Fashion and Beauty startup’s supply chain data, specifically focused on the movement of makeup products. By collecting, analyzing, and interpreting this dataset, I aim to gain insights that can inform strategic decisions and optimize supply chain operations.
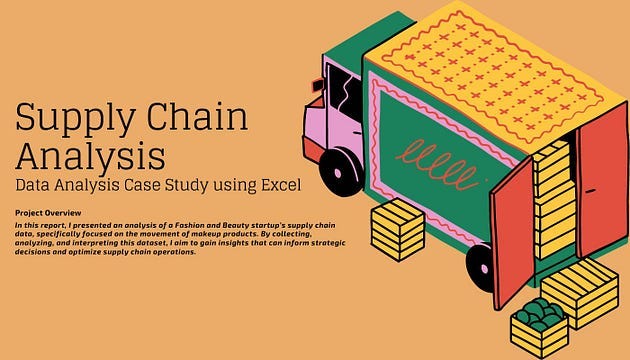
Dataset Overview
The dataset comprises various features related to the fashion and makeup product supply chain. These features provide valuable information for understanding the flow of products from suppliers to customers. The following features include:
Product Type
Availability
Number of products sold
Revenue generated
Customer demographics
Stock levels
Order quantities
Shipping times
Shipping carriers
Shipping costs
Supplier name
Production volumes
Manufacturing lead time
Manufacturing costs
Inspection results
Defect rates
Transportation modes
Supply Chain Analysis using Excel
The supply chain represents a network of interconnected processes involved in the production and delivery of goods to customers. Analyzing the various components of a supply chain is crucial for identifying opportunities to enhance its effectiveness and generate greater value for customers. In this report, I conducted a supply chain analysis using Excel and gave valuable insights into optimizing supply chain operations.
Dataset Source
To perform a supply chain analysis on this company, it is crucial to gather data related to various stages of the supply chain. This includes information on sourcing, manufacturing, transportation, inventory management, sales, and customer demographics.
Fortunately, I have come across this excellent dataset that provides comprehensive data about the supply chain of a Fashion and Beauty startup.
In the following section, I will guide you through the process I use to conduct a supply chain analysis using Excel.
Dataset from Github
Dataset Analysis using Excel
The first step is to import the file into Excel.
After importing the file, the next task is to remove any duplicate entries. To do this, I selected all the data by clicking on cell A1 and then pressed Ctrl+A. Then click on the “Data” tab and choose “Remove Duplicates” under the “Data Tools” section. Once duplicates are removed, the next step is to filter the data to identify any spelling errors or unwanted names. To do this, I can click on the “Filter” tool under the “Sort & Filter” section. While filtering, I noticed that some entries under the “Customer Demographics” category are labelled as “Unknown” instead of specific demographics like Male, Female or Non-Binary. Since I won’t be using this data, I proceeded with the existing information. At this stage, the data cleaning process is considered complete, and I have moved on to the next steps.
To facilitate further analysis, I converted the data into a table. I achieved this by selecting the entire data range (Ctrl+A) and then pressing Ctrl+T to convert it into a table. The next step is to create a pivot table. Navigated to the “Insert” tab and select the “PivotTable” tool. Choose the location for the pivot table (such as a new worksheet) and specify the data range (Table 1 or the relevant table name). Once the pivot table was created, I formatted the numbers to display as whole numbers and removed any decimal places for better readability.
A. Now, I’m ready to analyze the data and provide insights based on the following requirements or objectives.
I begin the analysis of the Supply Chain by examining the correlation between product prices and the corresponding revenue they generate.
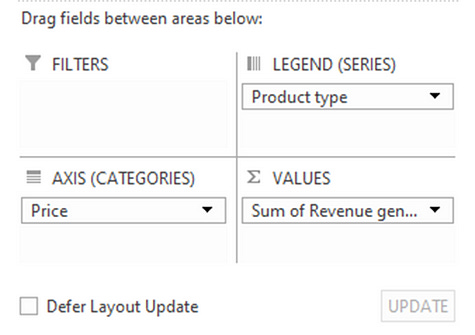
Therefore, the company generates a higher revenue from skincare products, and there is a positive relationship between the price of skincare products and the revenue they generate.
B. Now, I examined the sales based on different product types.
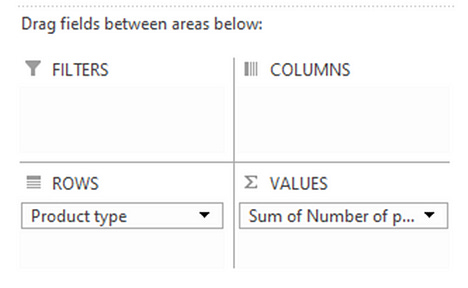
Skincare products account for 45% of the company’s business, while haircare products contribute to 29.5% of the revenue, and cosmetics make up 25.5% of the total sales.
C. Now, I analyzed the total revenue generated from shipping carriers.
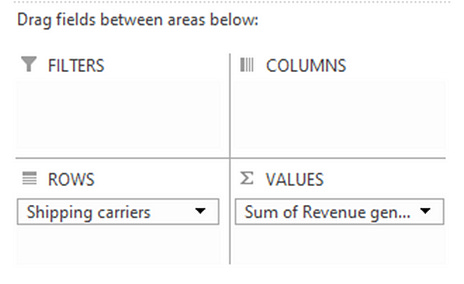
The company utilizes three shipping carriers for transportation, and among them, Carrier B contributes significantly to the company’s revenue generation.
D. Now, I examined the average lead time and average manufacturing costs for all products of the company.
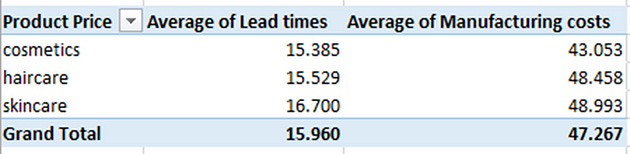
Analyzing SKUs
In the dataset, there is a column labelled SKUs, which stands for Stock Keeping Units. SKUs are unique codes assigned to products to facilitate inventory management and tracking. They serve as a means to identify and differentiate individual items, ensuring accurate monitoring of stock levels. For instance, in a toy store with a diverse range of toys, each toy would be assigned a unique SKU as a secret number known only to the store, enabling efficient inventory control.
A. Now, I analyzed the revenue generated by each SKU.
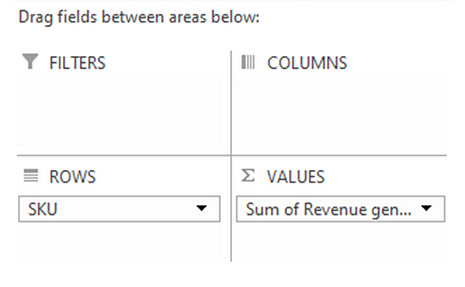
Another column in the dataset is labelled Stock levels. Stock levels indicate the quantity of products available in a store or business’s inventory at a given time
B. Now, I examined the stock levels of each SKU.
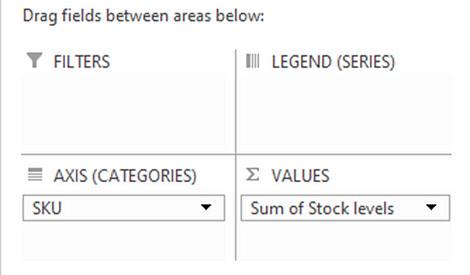
C. Now, I analyzed the order quantity of each SKU.
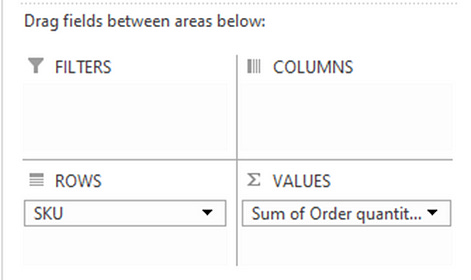
Cost Analysis
A. Now, I analyzed the shipping costs associated with different carriers.
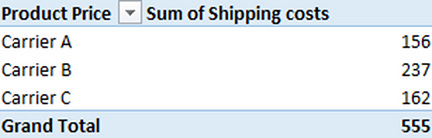
The above visualizations revealed that Carrier B contributes significantly to the company’s revenue. However, it is also the most expensive carrier among the three options.
B. Now, I examined the distribution of costs by transportation mode.
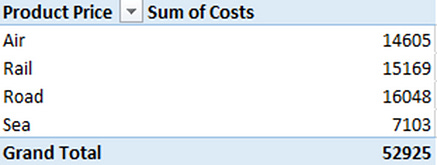
The company allocates a larger portion of its transportation expenses to the Road and Rail modes for the transportation of goods.
Analyzing Defect Rate
The defect rate in the supply chain refers to the percentage of products that are found to have issues or are damaged after being shipped.
A. Now, I analyzed the average defect rate across all product types.
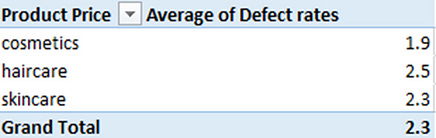
Haircare products have a higher defect rate compared to other product types.
B. Now, I examined the defect rates based on the mode of transportation.
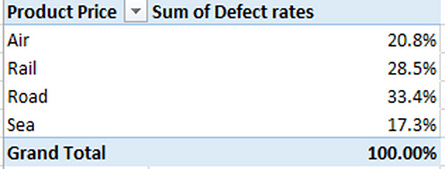
Road transportation exhibits a higher defect rate, while Air transportation demonstrates the lowest defect rate. This showcases how Excel programming language can be utilized to analyze a company’s supply chain.
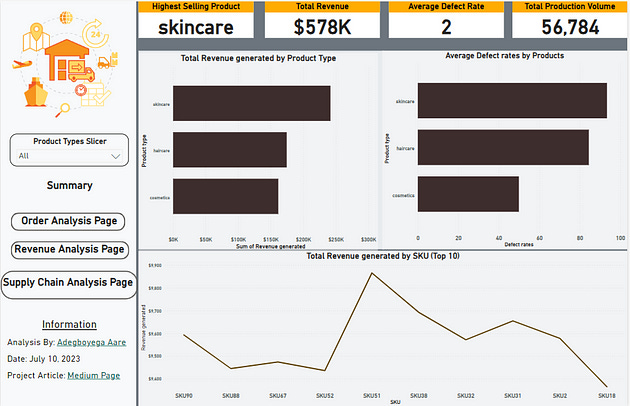
I successfully created a dashboard using Power BI that presents the insights mentioned earlier. The dashboard effectively highlights essential revenue-related information, including:
1. Revenue generated from each supplier. 2. Revenue generated from each location. 3. Revenue generated from each company product. 4. Total products delivered by each carrier.
These insightful visualizations offer valuable data-driven perspectives, enabling informed decision-making and strategic planning.
Supply Chain Analysis involves examining different aspects of a supply chain to identify areas for improvement and enhance the overall efficiency of the supply chain, ultimately delivering greater value to customers. This report provided an overview of conducting a supply chain analysis using Excel, highlighting key steps and techniques and revenue generated.
Thank you for reading, for more guides like this follow me on;
Twitter || LinkedIn
Buy Me a Coffee
If you find this article insightful you can support me by buying me a coffee (Click HERE ).
Remember to like, share, comment and subscribe.

Ready for more?
- Survey Paper
- Open access
- Published: 25 July 2020
Predictive big data analytics for supply chain demand forecasting: methods, applications, and research opportunities
- Mahya Seyedan 1 &
- Fereshteh Mafakheri ORCID: orcid.org/0000-0002-7991-4635 1
Journal of Big Data volume 7 , Article number: 53 ( 2020 ) Cite this article
124k Accesses
149 Citations
23 Altmetric
Metrics details
Big data analytics (BDA) in supply chain management (SCM) is receiving a growing attention. This is due to the fact that BDA has a wide range of applications in SCM, including customer behavior analysis, trend analysis, and demand prediction. In this survey, we investigate the predictive BDA applications in supply chain demand forecasting to propose a classification of these applications, identify the gaps, and provide insights for future research. We classify these algorithms and their applications in supply chain management into time-series forecasting, clustering, K-nearest-neighbors, neural networks, regression analysis, support vector machines, and support vector regression. This survey also points to the fact that the literature is particularly lacking on the applications of BDA for demand forecasting in the case of closed-loop supply chains (CLSCs) and accordingly highlights avenues for future research.
Introduction
Nowadays, businesses adopt ever-increasing precision marketing efforts to remain competitive and to maintain or grow their margin of profit. As such, forecasting models have been widely applied in precision marketing to understand and fulfill customer needs and expectations [ 1 ]. In doing so, there is a growing attention to analysis of consumption behavior and preferences using forecasts obtained from customer data and transaction records in order to manage products supply chains (SC) accordingly [ 2 , 3 ].
Supply chain management (SCM) focuses on flow of goods, services, and information from points of origin to customers through a chain of entities and activities that are connected to one another [ 4 ]. In typical SCM problems, it is assumed that capacity, demand, and cost are known parameters [ 5 ]. However, this is not the case in reality, as there are uncertainties arising from variations in customers’ demand, supplies transportation, organizational risks and lead times. Demand uncertainties, in particular, has the greatest influence on SC performance with widespread effects on production scheduling, inventory planning, and transportation [ 6 ]. In this sense, demand forecasting is a key approach in addressing uncertainties in supply chains [ 7 , 8 , 9 ].
A variety of statistical analysis techniques have been used for demand forecasting in SCM including time-series analysis and regression analysis [ 10 ]. With the advancements in information technologies and improved computational efficiencies, big data analytics (BDA) has emerged as a means of arriving at more precise predictions that better reflect customer needs, facilitate assessment of SC performance, improve the efficiency of SC, reduce reaction time, and support SC risk assessment [ 11 ].
The focus of this meta-research (literature review) paper is on “demand forecasting” in supply chains. The characteristics of demand data in today’s ever expanding and sporadic global supply chains makes the adoption of big data analytics (and machine learning) approaches a necessity for demand forecasting. The digitization of supply chains [ 12 ] and incoporporation Blockchain technologies [ 13 ] for better tracking of supply chains further highlights the role of big data analytics. Supply chain data is high dimensional generated across many points in the chain for varied purposes (products, supplier capacities, orders, shipments, customers, retailers, etc.) in high volumes due to plurality of suppliers, products, and customers and in high velocity reflected by many transactions continuously processed across supply chain networks. In the sense of such complexities, there has been a departure from conventional (statistical) demand forecasting approaches that work based on identifying statistically meannignful trends (characterized by mean and variance attributes) across historical data [ 14 ], towards intelligent forecasts that can learn from the historical data and intelligently evolve to adjust to predict the ever changing demand in supply chains [ 15 ]. This capability is established using big data analytics techniques that extract forecasting rules through discovering the underlying relationships among demand data across supply chain networks [ 16 ]. These techniques are computationally intensive to process and require complex machine-programmed algorithms [ 17 ].
With SCM efforts aiming at satisfying customer demand while minimizing the total cost of supply, applying machine-learning/data analytics algorithms could facilitate precise (data-driven) demand forecasts and align supply chain activities with these predictions to improve efficiency and satisfaction. Reflecting on these opportunities, in this paper, first a taxonmy of data sources in SCM is proposed. Then, the importance of demand management in SCs is investigated. A meta-research (literature review) on BDA applications in SC demand forecasting is explored according to categories of the algorithms utilized. This review paves the path to a critical discussion of BDA applications in SCM highlighting a number of key findings and summarizing the existing challenges and gaps in BDA applications for demand forecasting in SCs. On that basis, the paper concludes by presenting a number of avenues for future research.
Data in supply chains
Data in the context of supply chains can be categorized into customer, shipping, delivery, order, sale, store, and product data [ 18 ]. Figure 1 provides the taxonomy of supply chain data. As such, SC data originates from different (and segmented) sources such as sales, inventory, manufacturing, warehousing, and transportation. In this sense, competition, price volatilities, technological development, and varying customer commitments could lead to underestimation or overestimation of demand in established forecasts [ 19 ]. Therefore, to increase the precision of demand forecast, supply chain data shall be carefully analyzed to enhance knowledge about market trends, customer behavior, suppliers and technologies. Extracting trends and patterns from such data and using them to improve accuracy of future predictions can help minimize supply chain costs [ 20 , 21 ].
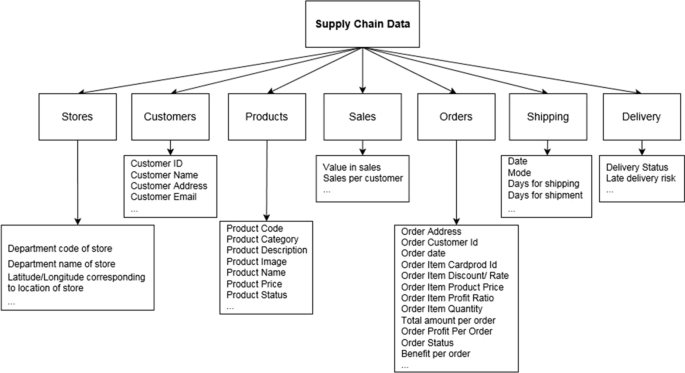
Taxonomy of supply chain data
Analysis of supply chain data has become a complex task due to (1) increasing multiplicity of SC entities, (2) growing diversity of SC configurations depending on the homogeneity or heterogeneity of products, (3) interdependencies among these entities (4) uncertainties in dynamical behavior of these components, (5) lack of information as relate to SC entities; [ 11 ], (6) networked manufacturing/production entities due to their increasing coordination and cooperation to achieve a high level customization and adaptaion to varying customers’ needs [ 22 ], and finally (7) the increasing adoption of supply chain digitization practices (and use of Blockchain technologies) to track the acitivities across supply chains [ 12 , 13 ].
Big data analytics (BDA) has been increasingly applied in management of SCs [ 23 ], for procurement management (e.g., supplier selection [ 24 ], sourcing cost improvement [ 25 ], sourcing risk management [ 26 ], product research and development [ 27 ], production planning and control [ 28 ], quality management [ 29 ], maintenance, and diagnosis [ 30 ], warehousing [ 31 ], order picking [ 32 ], inventory control [ 33 ], logistics/transportation (e.g., intelligent transportation systems [ 34 ], logistics planning [ 35 ], in-transit inventory management [ 36 ], demand management (e.g., demand forecasting [ 37 ], demand sensing [ 38 ], and demand shaping [ 39 ]. A key application of BDA in SCM is to provide accurate forecasting, especially demand forecasting, with the aim of reducing the bullwhip effect [ 14 , 40 , 41 , 42 ].
Big data is defined as high-volume, high-velocity, high-variety, high value, and high veracity data requiring innovative forms of information processing that enable enhanced insights, decision making, and process automation [ 43 ]. Volume refers to the extensive size of data collected from multiple sources (spatial dimension) and over an extended period of time (temporal dimension) in SCs. For example, in case of freight data, we have ERP/WMS order and item-level data, tracking, and freight invoice data. These data are generated from sensors, bar codes, Enterprise resource planning (ERP), and database technologies. Velocity can be defined as the rate of generation and delivery of specific data; in other words, it refers to the speed of data collection, reliability of data transferring, efficiency of data storage, and excavation speed of discovering useful knowledge as relate to decision-making models and algorithms. Variety refers to generating varied types of data from diverse sources such as the Internet of Things (IoT), mobile devices, online social networks, and so on. For instance, the vast data from SCM are usually variable due to the diverse sources and heterogeneous formats, particularly resulted from using various sensors in manufacturing sites, highways, retailer shops, and facilitated warehouses. Value refers to the nature of the data that must be discovered to support decision-making. It is the most important yet the most elusive, of the 5 Vs. Veracity refers to the quality of data, which must be accurate and trustworthy, with the knowledge that uncertainty and unreliability may exist in many data sources. Veracity deals with conformity and accuracy of data. Data should be integrated from disparate sources and formats, filtered and validated [ 23 , 44 , 45 ]. In summary, big data analytics techniques can deal with a collection of large and complex datasets that are difficult to process and analyze using traditional techniques [ 46 ].
The literature points to multiple sources of big data across the supply chains with varied trade-offs among volume, velocity, variety, value, and veracity attributes [ 47 ]. We have summarized these sources and trade-offs in Table 1 . Although, the demand forecasts in supply chains belong to the lower bounds of volume, velocity, and variety, however, these forecasts can use data from all sources across the supply chains from low volume/variety/velocity on-the-shelf inventory reports to high volume/variety/velocity supply chain tracking information provided through IoT. This combination of data sources used in SC demand forecasts, with their diverse temporal and spatial attributes, places a greater emphasis on use of big data analytics in supply chains, in general, and demand forecasting efforts, in particular.
The big data analytics applications in supply chain demand forecasting have been reported in both categories of supervised and unsupervised learning. In supervised learning, data will be associated with labels, meaning that the inputs and outputs are known. The supervised learning algorithms identify the underlying relationships between the inputs and outputs in an effort to map the inputs to corresponding outputs given a new unlabeled dataset [ 48 ]. For example, in case of a supervised learning model for demand forecasting, future demand can be predicted based on the historical data on product demand [ 41 ]. In unsupervised learning, data are unlabeled (i.e. unknown output), and the BDA algorithms try to find the underlying patterns among unlabeled data [ 48 ] by analyzing the inputs and their interrelationships. Customer segmentation is an example of unsupervised learning in supply chains that clusters different groups of customers based on their similarity [ 49 ]. Many machine-learning/data analytics algorithms can facilitate both supervised learning (extracting the input–output relationships) and unsupervised learning (extracting inputs, outputs and their relationships) [ 41 ].
Demand management in supply chains
The term “demand management” emerged in practice in the late 1980s and early 1990s. Traditionally, there are two approaches for demand management. A forward approach which looks at potential demand over the next several years and a backward approach that relies on past or ongoing capabilities in responding to demand [ 50 ].
In forward demand management, the focus will be on demand forecasting and planning, data management, and marketing strategies. Demand forecasting and planning refer to predicting the quantities and timings of customers’ requests. Such predictions aim at achieving customers’ satisfaction by meeting their needs in a timely manner [ 51 ]. Accurate demand forecasting could improve the efficiency and robustness of production processes (and the associated supply chains) as the resources will be aligned with requirements leading to reduction of inventories and wastes [ 52 , 53 ].
In the light of the above facts, there are many approaches proposed in the literature and practice for demand forecasting and planning. Spreadsheet models, statistical methods (like moving averages), and benchmark-based judgments are among these approaches. Today, the most widely used demand forecasting and planning tool is Excel. The most widespread problem with spreadsheet models used for demand forecasting is that they are not scalable for large-scale data. In addition, the complexities and uncertainties in SCM (with multiplicity and variability of demand and supply) cannot be extracted, analyzed, and addressed through simple statistical methods such as moving averages or exponential smoothing [ 50 ]. During the past decade, traditional solutions for SC demand forecasting and planning have faced many difficulties in driving the costs down and reducing inventories [ 50 ]. Although, in some cases, the suggested solutions have improved the day’s payable, they have pushed up the SC costs as a burden to suppliers.
The era of big data and high computing analytics has enabled data processing at a large scale that is efficient, fast, easy, and with reduced concerns about data storage and collection due to cloud services. The emergence of new technologies in data storage and analytics and the abundance of quality data have created new opportunities for data-driven demand forecasting and planning. Demand forecast accuracy can be significantly improved with data-mining algorithms and tools that can sift through data, analyze the results, and learn about the relationships involved. This could lead to highly accurate demand forecasting models that learn from data and are scalable for application in SCM. In the following section, a review of BDA applications in SCM is presented. These applications are categorized based on the employed techniques in establishing the data-drive demand forecasts.
BDA for demand forecasting in SCM
This survey aims at reviewing the articles published in the area of demand and sales forecasting in SC in the presence of big data to provide a classification of the literature based on algorithms utilized as well as a survey of applications. To the best of our knowledge, no comprehensive review of the literature specifically on SC demand forecasting has been conducted with a focus on classification of techniques of data analytics and machine learning. In doing so, we performed a thorough search of the existing literature, through Scopus, Google Scholar, and Elsevier, with publication dates ranging from 2005 to 2019. The keywords used for the search were supply chain, demand forecasting, sales forecasting, big data analytics, and machine learning.
Figure 2 shows the trend analysis of publications in demand forecasting for SC appeared from 2005 to 2019. There is a steadily increasing trend in the number of publications from 2005 to 2019. It is expected that such growth continues in 2020. Reviewing the past 15 years of research on big data analysis/machine learning applications in SC demand forecasting, we identified 64 research papers (excluding books, book chapters, and review papers) and categorized them with respect to the methodologies adopted for demand forecasting. The five most frequently used techniques are listed in Table 2 that includes “Neural Network,” “Regression”, “Time-series forecasting (ARIMA)”, “Support Vector Machine”, and “Decision Tree” methods. This table implies the growing use of big data analysis techniques in SC demand forecasting. It shall be mentioned that there were a few articles using multiple of these techniques.
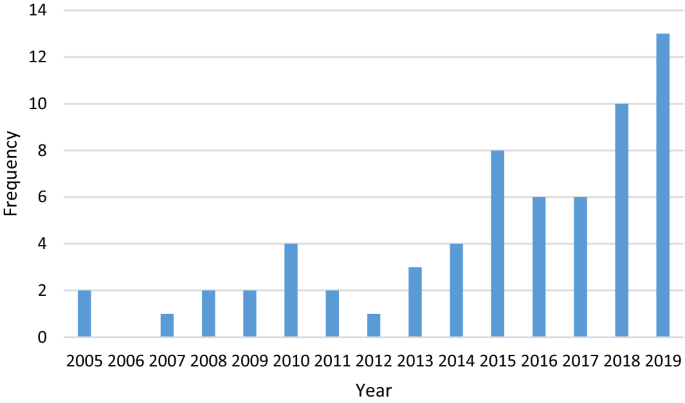
Distribution of literature in supply chain demand forecasting from 2005 to 2019
It shall be mentioned that there are literature review papers exploring the use of big data analytics in SCM [ 10 , 16 , 23 , 54 , 55 , 56 , 57 , 58 , 59 , 60 , 61 , 62 , 63 , 64 , 65 , 66 , 67 ]. However, this study focuses on the specific topic of “demand forecasting” in SCM to explore BDA applications in line with this particular subtopic in SCM.
As Hofmann and Rutschmann [ 58 ] indicated in their literature review, the key questions to answer are why, what and how big data analytics/machine-learning algorithms could enhance forecasts’ accuracy in comparison to conventional statistical forecasting approaches.
Conventional methods have faced a number of limitations for demand forecasting in the context of SCs. There are a lot of parameters influencing the demand in supply chains, however, many of them were not captured in studies using conventional methods for the sake of simplicity. In this regard, the forecasts could only provide a partial understanding of demand variations in supply chains. In addition, the unexplained demand variations could be simply considered as statistical noise. Conventional approaches could provide shorter processing times in exchange for a compromise on robustness and accuracy of predictions. Conventional SC demand forecasting approaches are mostly done manually with high reliance on the planner’s skills and domain knowledge. It would be worthwhile to fully automate the forecasting process to reduce such a dependency [ 58 ]. Finally, data-driven techniques could learn to incorporate non-linear behaviors and could thus provide better approximations in demand forecasting compared to conventional methods that are mostly derived based on linear models. There is a significant level of non-linearity in demand behavior in SC particularly due to competition among suppliers, the bullwhip effect, and mismatch between supply and demand [ 40 ].
To extract valuable knowledge from a vast amount of data, BDA is used as an advanced analytics technique to obtain the data needed for decision-making. Reduced operational costs, improved SC agility, and increased customer satisfaction are mentioned among the benefits of applying BDA in SCM [ 68 ]. Researchers used various BDA techniques and algorithms in SCM context, such as classification, scenario analysis, and optimization [ 23 ]. Machine-learning techniques have been used to forecast demand in SCs, subject to uncertainties in prices, markets, competitors, and customer behaviors, in order to manage SCs in a more efficient and profitable manner [ 40 ].
BDA has been applied in all stages of supply chains, including procurement, warehousing, logistics/transportation, manufacturing, and sales management. BDA consists of descriptive analytics, predictive analytics, and prescriptive analytics. Descriptive analysis is defined as describing and categorizing what happened in the past. Predictive analytics are used to predict future events and discover predictive patterns within data by using mathematical algorithms such as data mining, web mining, and text mining. Prescriptive analytics apply data and mathematical algorithms for decision-making. Multi-criteria decision-making, optimization, and simulation are among the prescriptive analytics tools that help to improve the accuracy of forecasting [ 10 ].
Predictive analytics are the ones mostly utilized in SC demand and procurement forecasting [ 23 ]. In this sense, in the following subsections, we will review various predictive big data analytics approaches, presented in the literature for demand forecasting in SCM, categorized based on the employed data analytics/machine learning technique/algorithm, with elaborations of their purpose and applications (summarized in Table 3 ).
Time-series forecasting
Time series are methodologies for mining complex and sequential data types. In time-series data, sequence data, consisting of long sequences of numeric data, recorded at equal time intervals (e.g., per minute, per hour, or per day). Many natural and human-made processes, such as stock markets, medical diagnosis, or natural phenomenon, can generate time-series data. [ 48 ].
In case of demand forecasting using time-series, demand is recorded over time at equal size intervals [ 69 , 70 ]. Combinations of time-series methods with product or market features have attracted much attention in demand forecasting with BDA. Ma et al. [ 71 ] proposed and developed a demand trend-mining algorithm for predictive life cycle design. In their method, they combined three models (a) a decision tree model for large-scale historical data classification, (b) a discrete choice analysis for present and past demand modeling, and (c) an automated time-series forecasting model for future trend analysis. They tested and applied their 3-level approach in smartphone design, manufacturing and remanufacturing.
Time-series approach was used for forecasting of search traffic (service demand) subject to changes in consumer attitudes [ 37 ]. Demand forecasting has been achieved through time-series models using exponential smoothing with covariates (ESCov) to provide predictions for short-term, mid-term, and long-term demand trends in the chemical industry SCs [ 7 ]. In addition, Hamiche et al. [ 72 ] used a customer-responsive time-series approach for SC demand forecasting.
In case of perishable products, with short life cycles, having appropriate (short-term) forecasting is extremely critical. Da Veiga et al. [ 73 ] forecasted the demand for a group of perishable dairy products using Autoregressive Integrated Moving Average (ARIMA) and Holt-Winters (HW) models. The results were compared based on mean absolute percentage error (MAPE) and Theil inequality index (U-Theil). The HW model showed a better goodness-of-fit based on both performance metrics.
In case of ARIMA, the accuracy of predictions could diminish where there exists a high level of uncertainty in future patterns of parameters [ 42 , 74 , 75 , 76 ]. HW model forecasting can yield better accuracy in comparison to ARIMA [ 73 ]. HW is simple and easy to use. However, data horizon could not be larger than a seasonal cycle; otherwise, the accuracy of forecasts will decrease sharply. This is due to the fact that inputs of an HW model are themselves predicted values subject to longer-term potential inaccuracies and uncertainties [ 45 , 73 ].
Clustering analysis
Clustering analysis is a data analysis approach that partitions a group of data objects into subgroups based on their similarities. Several applications of clustering analysis has been reported in business analytics, pattern recognition, and web development [ 48 ]. Han et al. [ 48 ] have emphasized the fact that using clustering customers can be organized into groups (clusters), such that customers within a group present similar characteristic.
A key target of demand forecasting is to identify demand behavior of customers. Extraction of similar behavior from historical data leads to recognition of customer clusters or segments. Clustering algorithms such as K-means, self-organizing maps (SOMs), and fuzzy clustering have been used to segment similar customers with respect to their behavior. The clustering enhances the accuracy of SC demand forecasting as the predictions are established for each segment comprised of similar customers. As a limitation, the clustering methods have the tendency to identify the customers, that do not follow a pattern, as outliers [ 74 , 77 ].
Hierarchical forecasts of sales data are performed by clustering and categorization of sales patterns. Multivariate ARIMA models have been used in demand forecasting based on point-of-sales data in industrial bakery chains [ 19 ]. These bakery goods are ordered and clustered daily with a continuous need to demand forecasts in order to avoid both shortage or waste [ 19 ]. Fuel demand forecasting in thermal power plants is another domain with applications of clustering methods. Electricity consumption patterns are derived using a clustering of consumers, and on that basis, demand for the required fuel is established [ 77 ].
K-nearest-neighbor (KNN)
KNN is a method of classification that has been widely used for pattern recognition. KNN algorithm identifies the similarity of a given object to the surrounding objects (called tuples) by generating a similarity index. These tuples are described by n attributes. Thus, each tuple corresponds to a point in an n-dimensional space. The KNN algorithm searches for k tuples that are closest to a given tuple [ 48 ]. These similarity-based classifications will lead to formation of clusters containing similar objects. KNN can also be integrated into regression analysis problems [ 78 ] for dimensionality reduction of the data [ 79 ]. In the realm of demand forecasting in SC, Nikolopoulos et al. [ 80 ] applied KNN for forecasting sporadic demand in an automotive spare parts supply chain. In another study, KNN is used to forecast future trends of demand for Walmart’s supply chain planning [ 81 ].
Artificial neural networks
In artificial neural networks, a set of neurons (input/output units) are connected to one another in different layers in order to establish mapping of the inputs to outputs by finding the underlying correlations between them. The configuration of such networks could become a complex problem, due to a high number of layers and neurons, as well as variability of their types (linear or nonlinear), which needs to follow a data-driven learning process to be established. In doing so, each unit (neuron) will correspond to a weight, that is tuned through a training step [ 48 ]. At the end, a weighted network with minimum number of neurons, that could map the inputs to outputs with a minimum fitting error (deviation), is identified.
As the literature reveals, artificial neural networks (ANN) are widely applied for demand forecasting [ 82 , 83 , 84 , 85 ]. To improve the accuracy of ANN-based demand predictions, Liu et al. [ 86 ] proposed a combination of a grey model and a stacked auto encoder applied to a case study of predicting demand in a Brazilian logistics company subject to transportation disruption [ 87 ]. Amirkolaii et al. [ 88 ] applied neural networks in forecasting spare parts demand to minimize supply chain shortages. In this case of spare parts supply chain, although there were multiple suppliers to satisfy demand for a variety of spare parts, the demand was subject to high variability due to a varying number of customers and their varying needs. Their proposed ANN-based forecasting approach included (1) 1 input demand feature with 1 Stock-Keeping Unit (SKU), (2) 1 input demand feature with all SKUs, (3) 16 input demand features with 1 SKU, and (4) 16 input demand features with all SKUs. They applied neural networks with back propagation and compared the results with a number of benchmarks reporting a Mean Square Error (MSE) for each configuration scenario.
Huang et al. [ 89 ] compared a backpropagation (BP) neural network and a linear regression analysis for forecasting of e-logistics demand in urban and rural areas in China using data from 1997 to 2015. By comparing mean absolute error (MAE) and the average relative errors of backpropagation neural network and linear regression, they showed that backpropagation neural networks could reach higher accuracy (reflecting lower differences between predicted and actual data). This is due to the fact that a Sigmoid function was used as the transfer function in the hidden layer of BP, which is differentiable for nonlinear problems such as the one presented in their case study, whereas the linear regression works well with linear problems.
ANNs have also been applied in demand forecasting for server models with one-week demand prediction ahead of order arrivals. In this regard, Saha et al. [ 90 ] proposed an ANN-based forecasting model using a 52-week time-series data fitted through both BP and Radial Basis Function (RBF) networks. A RBF network is similar to a BP network except for the activation/transfer function in RBF that follows a feed-forward process using a radial basis function. RBF results in faster training and convergence to ANN weights in comparison with BP networks without compromising the forecasting precision.
Researchers have combined ANN-based machine-learning algorithms with optimization models to draw optimal courses of actions, strategies, or decisions for future. Chang et al. [ 91 ] employed a genetic algorithm in the training phase of a neural network using sales/supply chain data in the printed circuit board industry in Taiwan and presented an evolving neural network-forecasting model. They proposed use of a Genetic Algorithms (GA)-based cost function optimization to arrive at the best configuration of the corresponding neural network for sales forecast with respect to prediction precision. The proposed model was then compared to back-propagation and linear regression approaches using three performance indices of MAPE, Mean Absolute Deviation (MAD), and Total Cost Deviation (TCD), presenting its superior prediction precision.
Regression analysis
Regression models are used to generate continuous-valued functions utilized for prediction. These methods are used to predict the value of a response (dependent) variable with respect to one or more predictor (independent) variables. There are various forms of regression analysis, such as linear, multiple, weighted, symbolic (random), polynomial, nonparametric, and robust. The latter approach is useful when errors fail to satisfy normalcy conditions or when we deal with big data that could contain significant number of outliers [ 48 ].
Merkuryeva et al. [ 92 ] analyzed three prediction approaches for demand forecasting in the pharmaceutical industry: a simple moving average model, multiple linear regressions, and a symbolic regression with searches conducted through an evolutionary genetic programming. In this experiment, symbolic regression exhibited the best fit with the lowest error.
As perishable products must be sold due to a very short preservation time, demand forecasting for this type of products has drawn increasing attention. Yang and Sutrisno [ 93 ] applied and compared regression analysis and neural network techniques to derive demand forecasts for perishable goods. They concluded that accurate daily forecasts are achievable with knowledge of sales numbers in the first few hours of the day using either of the above methods.
Support vector machine (SVM)
SVM is an algorithm that uses a nonlinear mapping to transform a set of training data into a higher dimension (data classes). SVM searches for an optimal separating hyper-plane that can separate the resulting class from another) [ 48 ]. Villegas et al. [ 94 ] tested the applicability of SVMs for demand forecasting in household and personal care SCs with a dataset comprised of 229 weekly demand series in the UK. Wu [ 95 ] applied an SVM, using a particle swarm optimization (PSO) to search for the best separating hyper-plane, classifying the data related to car sales and forecasting the demand in each cluster.
Support vector regression (SVR)
Continuous variable classification problems can be solved by support vector regression (SVR), which is a regression implementation of SVM. The main idea behind SVR regression is the computation of a linear regression function within a high-dimensional feature space. SVR has been applied in financial/cost prediction problems, handwritten digit recognition, and speaker identification, object recognition, etc. [ 48 ].
Guanghui [ 96 ] used the SVR method for SC needs prediction. The use of SVR in demand forecasting can yield a lower mean square error than RBF neural networks due to the fact that the optimization (cost) function in SVR does not consider the points beyond a margin of distance from the training set. Therefore, this method leads to higher forecast accuracy, although, similar to SVM, it is only applicable to a two-class problem (such as normal versus anomaly detection/estimation problems). Sarhani and El Afia [ 97 ] sought to forecast SC demand using SVR and applied Particle swarm optimization (PSO) and GA to optimize SVR parameters. SVR-PSO and SVR-GA approaches were compared with respect to accuracy of predictions using MAPE. The results showed a superior performance by PSO in terms time intensity and MAPE when configuring the SVR parameters.
Mixed approaches
Some works in the literature have used a combination of the aforementioned techniques. In these studies, the data flow into a sequence of algorithms and the outputs of one stage become inputs of the next step. The outputs are explanatory in the form of qualitative and quantitative information with a sequence of useful information extracted out of each algorithm. Examples of such studies include [ 15 , 98 , 99 , 100 , 101 , 102 , 103 , 104 , 105 ].
In more complex supply chains with several points of supply, different warehouses, varied customers, and several products, the demand forecasting becomes a high dimensional problem. To address this issue, Islek and Oguducu [ 100 ] applied a clustering technique, called bipartite graph clustering, to analyze the patterns of sales for different products. Then, they combined a moving average model and a Bayesian belief network approaches to improve the accuracy of demand forecasting for each cluster. Kilimci et al. [ 101 ] developed an intelligent demand forecasting system by applying time-series and regression methods, a support vector regression algorithm, and a deep learning model in a sequence. They dealt with a case involving big amount of data accounting for 155 features over 875 million records. First, they used a principal component analysis for dimension reduction. Then, data clustering was performed. This is followed by demand forecasting for each cluster using a novel decision integration strategy called boosting ensemble. They concluded that the combination of a deep neural network with a boosting strategy yielded the best accuracy, minimizing the prediction error for demand forecasting.
Chen and Lu [ 98 ] combined clustering algorithms of SOM, a growing hierarchical self-organizing mapping (GHSOM), and K-means, with two machine-learning techniques of SVR and extreme learning machine (ELM) in sales forecasting of computers. The authors found that the combination of GHSOM and ELM yielded better accuracy and performance in demand forecasts for their computer retailing case study. Difficulties in forecasting also occur in cases with high product variety. For these types of products in an SC, patterns of sales can be extracted for clustered products. Then, for each cluster, a machine-learning technique, such as SVR, can be employed to further improve the prediction accuracy [ 104 ].
Brentan et al. [ 106 ] used and analyzed various BDA techniques for demand prediction; including support vector machines (SVM), and adaptive neural fuzzy inference systems (ANFIS). They combined the predicted values derived from each machine learning techniques, using a linear regression process to arrive at an average prediction value adopted as the benchmark forecast. The performance (accuracy) of each technique is then analyzed with respect to their mean square root error (RMSE) and MAE values obtained through comparing the target values and the predicted ones.
In summary, Table 3 provides an overview of the recent literature on the application of Predictive BDA in demand forecasting.
Discussions
The data produced in SCs contain a great deal of useful knowledge. Analysis of such massive data can help us to forecast trends of customer behavior, markets, prices, and so on. This can help organizations better adapt to competitive environments. To forecast demand in an SC, with the presences of big data, different predictive BDA algorithms have been used. These algorithms could provide predictive analytics using time-series approaches, auto-regressive methods, and associative forecasting methods [ 10 ]. The demand forecasts from these BDA methods could be integrated with product design attributes as well as with online search traffic mapping to incorporate customer and price information [ 37 , 71 ].
Predictive BDA algorithms
Most of the studies examined, developed and used a certain data-mining algorithm for their case studies. However, there are very few comparative studies available in the literature to provide a benchmark for understanding of the advantages and disadvantages of these methodologies. Additionally, as depicted by Table 3 , there is no clear trend between the choice of the BDA algorithm/method and the application domain or category.
Predictive BDA applicability
Most data-driven models used in the literature consider historical data. Such a backward-looking forecasting ignores the new trends and highs and lows in different economic environments. Also, organizational factors, such as reputation and marketing strategies, as well as internal risks (related to availability of SCM resources), could greatly influence the demand [ 107 ] and thus contribute to inaccuracy of BDA-based demand predictions using historical data. Incorporating existing driving factors outside the historical data, such as economic instability, inflation, and purchasing power, could help adjust the predictions with respect to unseen future scenarios of demand. Combining predictive algorithms with optimization or simulation can equip the models with prescriptive capabilities in response to future scenarios and expectations.
Predictive BDA in closed-loop supply chains (CLSC)
The combination of forward and reverse flow of material in a SC is referred to as a closed-loop supply chain (CLSC). A CLSC is a more complex system than a traditional SC because it consists of the forward and reverse SC simultaneously [ 108 ]. Economic impact, environmental impact, and social responsibility are three significant factors in designing a CLSC network with inclusion of product recycling, remanufacturing, and refurbishment functions. The complexity of a CLSC, compared to a common SC, results from the coordination between backward and forward flows. For example, transportation cost, holding cost, and forecasting demand are challenging issues because of uncertainties in the information flows from the forward chain to the reverse one. In addition, the uncertainties about the rate of returned products and efficiencies of recycling, remanufacturing, and refurbishment functions are some of the main barriers in establishing predictions for the reverse flow [ 5 , 6 , 109 ]. As such, one key finding from this literature survey is that CLSCs particularly deal with the lack of quality data for remanufacturing. Remanufacturing refers to the disassembly of products, cleaning, inspection, storage, reconditioning, replacement, and reassembling. As a result of deficiencies in data, optimal scheduling of remanufacturing functions is cumbersome due to uncertainties in the quality and quantity of used products as well as timing of returns and delivery delays.
IoT-based approaches can overcome the difficulties of collecting data in a CLSC. In an IoT environment, objects are monitored and controlled remotely across existing network infrastructures. This enables more direct integration between the physical world and computer-based systems. The results include improved efficiency, accuracy, and economic benefit across SCs [ 50 , 54 , 110 ].
Radio frequency identification (RFID) is another technology that has become very popular in SCs. RFID can be used for automation of processes in an SC, and it is useful for coordination of forecasts in CLSCs with dispersed points of return and varied quantities and qualities of returned used products [ 10 , 111 , 112 , 113 , 114 ].
Conclusions
The growing need to customer behavior analysis and demand forecasting is deriven by globalization and increasing market competitions as well as the surge in supply chain digitization practices. In this study, we performed a thorough review for applications of predictive big data analytics (BDA) in SC demand forecasting. The survey overviewed the BDA methods applied to supply chain demand forecasting and provided a comparative categorization of them. We collected and analyzed these studies with respect to methods and techniques used in demand prediction. Seven mainstream techniques were identified and studied with their pros and cons. The neural networks and regression analysis are observed as the two mostly employed techniques, among others. The review also pointed to the fact that optimization models or simulation can be used to improve the accuracy of forecasting through formulating and optimizing a cost function for the fitting of the predictions to data.
One key finding from reviewing the existing literature was that there is a very limited research conducted on the applications of BDA in CLSC and reverse logistics. There are key benefits in adopting a data-driven approach for design and management of CLSCs. Due to increasing environmental awareness and incentives from the government, nowadays a vast quantity of returned (used) products are collected, which are of various types and conditions, received and sorted in many collection points. These uncertainties have a direct impact on the cost-efficiency of remanufacturing processes, the final price of the refurbished products and the demand for these products [ 115 ]. As such, design and operation of CLSCs present a case for big data analytics from both supply and demand forecasting perspectives.
Availability of data and materials
The paper presents a review of the literature extracted from main scientific databases without presenting data.
Abbreviations
Adaptive neural fuzzy inference systems
Auto regressive integrated moving average
Artificial neural network
- Big data analytics
Backpropagation
Closed-loop supply chain
Extreme learning machine
Enterprise resource planning
Genetic algorithms
Growing hierarchical self-organizing map
Holt-winters
Internet of things
K-nearest-neighbor
Mean absolute deviation
Mean absolute error
Mean absolute percentage error
Mean square error
Mean square root error
Radial basis function
Particle swarm optimization
Self-organizing maps
Stock-keeping unit
Supply chain analytics
Supply chain
- Supply chain management
Support vector machine
Support vector regression
Total cost deviation
Theil inequality index
You Z, Si Y-W, Zhang D, Zeng X, Leung SCH, Li T. A decision-making framework for precision marketing. Expert Syst Appl. 2015;42(7):3357–67. https://doi.org/10.1016/J.ESWA.2014.12.022 .
Article Google Scholar
Guo ZX, Wong WK, Li M. A multivariate intelligent decision-making model for retail sales forecasting. Decis Support Syst. 2013;55(1):247–55. https://doi.org/10.1016/J.DSS.2013.01.026 .
Wei J-T, Lee M-C, Chen H-K, Wu H-H. Customer relationship management in the hairdressing industry: an application of data mining techniques. Expert Syst Appl. 2013;40(18):7513–8. https://doi.org/10.1016/J.ESWA.2013.07.053 .
Lu LX, Swaminathan JM. Supply chain management. Int Encycl Soc Behav Sci. 2015. https://doi.org/10.1016/B978-0-08-097086-8.73032-7 .
Gholizadeh H, Tajdin A, Javadian N. A closed-loop supply chain robust optimization for disposable appliances. Neural Comput Appl. 2018. https://doi.org/10.1007/s00521-018-3847-9 .
Tosarkani BM, Amin SH. A possibilistic solution to configure a battery closed-loop supply chain: multi-objective approach. Expert Syst Appl. 2018;92:12–26. https://doi.org/10.1016/J.ESWA.2017.09.039 .
Blackburn R, Lurz K, Priese B, Göb R, Darkow IL. A predictive analytics approach for demand forecasting in the process industry. Int Trans Oper Res. 2015;22(3):407–28. https://doi.org/10.1111/itor.12122 .
Article MathSciNet MATH Google Scholar
Boulaksil Y. Safety stock placement in supply chains with demand forecast updates. Oper Res Perspect. 2016;3:27–31. https://doi.org/10.1016/J.ORP.2016.07.001 .
Article MathSciNet Google Scholar
Tang CS. Perspectives in supply chain risk management. Int J Prod Econ. 2006;103(2):451–88. https://doi.org/10.1016/J.IJPE.2005.12.006 .
Wang G, Gunasekaran A, Ngai EWT, Papadopoulos T. Big data analytics in logistics and supply chain management: certain investigations for research and applications. Int J Prod Econ. 2016;176:98–110. https://doi.org/10.1016/J.IJPE.2016.03.014 .
Awwad M, Kulkarni P, Bapna R, Marathe A. Big data analytics in supply chain: a literature review. In: Proceedings of the international conference on industrial engineering and operations management, 2018(SEP); 2018, p. 418–25.
Büyüközkan G, Göçer F. Digital Supply Chain: literature review and a proposed framework for future research. Comput Ind. 2018;97:157–77.
Kshetri N. 1 Blockchain’s roles in meeting key supply chain management objectives. Int J Inf Manage. 2018;39:80–9.
Michna Z, Disney SM, Nielsen P. The impact of stochastic lead times on the bullwhip effect under correlated demand and moving average forecasts. Omega. 2019. https://doi.org/10.1016/J.OMEGA.2019.02.002 .
Zhu Y, Zhao Y, Zhang J, Geng N, Huang D. Spring onion seed demand forecasting using a hybrid Holt-Winters and support vector machine model. PLoS ONE. 2019;14(7):e0219889. https://doi.org/10.1371/journal.pone.0219889 .
Govindan K, Cheng TCE, Mishra N, Shukla N. Big data analytics and application for logistics and supply chain management. Transport Res Part E Logist Transport Rev. 2018;114:343–9. https://doi.org/10.1016/J.TRE.2018.03.011 .
Bohanec M, Kljajić Borštnar M, Robnik-Šikonja M. Explaining machine learning models in sales predictions. Expert Syst Appl. 2017;71:416–28. https://doi.org/10.1016/J.ESWA.2016.11.010 .
Constante F, Silva F, Pereira A. DataCo smart supply chain for big data analysis. Mendeley Data. 2019. https://doi.org/10.17632/8gx2fvg2k6.5 .
Huber J, Gossmann A, Stuckenschmidt H. Cluster-based hierarchical demand forecasting for perishable goods. Expert Syst Appl. 2017;76:140–51. https://doi.org/10.1016/J.ESWA.2017.01.022 .
Ali MM, Babai MZ, Boylan JE, Syntetos AA. Supply chain forecasting when information is not shared. Eur J Oper Res. 2017;260(3):984–94. https://doi.org/10.1016/J.EJOR.2016.11.046 .
Bian W, Shang J, Zhang J. Two-way information sharing under supply chain competition. Int J Prod Econ. 2016;178:82–94. https://doi.org/10.1016/J.IJPE.2016.04.025 .
Mourtzis D. Challenges and future perspectives for the life cycle of manufacturing networks in the mass customisation era. Logist Res. 2016;9(1):2.
Nguyen T, Zhou L, Spiegler V, Ieromonachou P, Lin Y. Big data analytics in supply chain management: a state-of-the-art literature review. Comput Oper Res. 2018;98:254–64. https://doi.org/10.1016/J.COR.2017.07.004 .
Choi Y, Lee H, Irani Z. Big data-driven fuzzy cognitive map for prioritising IT service procurement in the public sector. Ann Oper Res. 2018;270(1–2):75–104. https://doi.org/10.1007/s10479-016-2281-6 .
Huang YY, Handfield RB. Measuring the benefits of erp on supply management maturity model: a “big data” method. Int J Oper Prod Manage. 2015;35(1):2–25. https://doi.org/10.1108/IJOPM-07-2013-0341 .
Miroslav M, Miloš M, Velimir Š, Božo D, Đorđe L. Semantic technologies on the mission: preventing corruption in public procurement. Comput Ind. 2014;65(5):878–90. https://doi.org/10.1016/J.COMPIND.2014.02.003 .
Zhang Y, Ren S, Liu Y, Si S. A big data analytics architecture for cleaner manufacturing and maintenance processes of complex products. J Clean Prod. 2017;142:626–41. https://doi.org/10.1016/J.JCLEPRO.2016.07.123 .
Shu Y, Ming L, Cheng F, Zhang Z, Zhao J. Abnormal situation management: challenges and opportunities in the big data era. Comput Chem Eng. 2016;91:104–13. https://doi.org/10.1016/J.COMPCHEMENG.2016.04.011 .
Krumeich J, Werth D, Loos P. Prescriptive control of business processes: new potentials through predictive analytics of big data in the process manufacturing industry. Bus Inform Syst Eng. 2016;58(4):261–80. https://doi.org/10.1007/s12599-015-0412-2 .
Guo SY, Ding LY, Luo HB, Jiang XY. A Big-Data-based platform of workers’ behavior: observations from the field. Accid Anal Prev. 2016;93:299–309. https://doi.org/10.1016/J.AAP.2015.09.024 .
Chuang Y-F, Chia S-H, Wong J-Y. Enhancing order-picking efficiency through data mining and assignment approaches. WSEAS Transactions on Business and Economics. 2014;11(1):52–64.
Google Scholar
Ballestín F, Pérez Á, Lino P, Quintanilla S, Valls V. Static and dynamic policies with RFID for the scheduling of retrieval and storage warehouse operations. Comput Ind Eng. 2013;66(4):696–709. https://doi.org/10.1016/J.CIE.2013.09.020 .
Alyahya S, Wang Q, Bennett N. Application and integration of an RFID-enabled warehousing management system—a feasibility study. J Ind Inform Integr. 2016;4:15–25. https://doi.org/10.1016/J.JII.2016.08.001 .
Cui J, Liu F, Hu J, Janssens D, Wets G, Cools M. Identifying mismatch between urban travel demand and transport network services using GPS data: a case study in the fast growing Chinese city of Harbin. Neurocomputing. 2016;181:4–18. https://doi.org/10.1016/J.NEUCOM.2015.08.100 .
Shan Z, Zhu Q. Camera location for real-time traffic state estimation in urban road network using big GPS data. Neurocomputing. 2015;169:134–43. https://doi.org/10.1016/J.NEUCOM.2014.11.093 .
Ting SL, Tse YK, Ho GTS, Chung SH, Pang G. Mining logistics data to assure the quality in a sustainable food supply chain: a case in the red wine industry. Int J Prod Econ. 2014;152:200–9. https://doi.org/10.1016/J.IJPE.2013.12.010 .
Jun S-P, Park D-H, Yeom J. The possibility of using search traffic information to explore consumer product attitudes and forecast consumer preference. Technol Forecast Soc Chang. 2014;86:237–53. https://doi.org/10.1016/J.TECHFORE.2013.10.021 .
He W, Wu H, Yan G, Akula V, Shen J. A novel social media competitive analytics framework with sentiment benchmarks. Inform Manage. 2015;52(7):801–12. https://doi.org/10.1016/J.IM.2015.04.006 .
Marine-Roig E, Anton Clavé S. Tourism analytics with massive user-generated content: a case study of Barcelona. J Destination Market Manage. 2015;4(3):162–72. https://doi.org/10.1016/J.JDMM.2015.06.004 .
Carbonneau R, Laframboise K, Vahidov R. Application of machine learning techniques for supply chain demand forecasting. Eur J Oper Res. 2008;184(3):1140–54. https://doi.org/10.1016/J.EJOR.2006.12.004 .
Article MATH Google Scholar
Munir K. Cloud computing and big data: technologies, applications and security, vol. 49. Berlin: Springer; 2019.
Rostami-Tabar B, Babai MZ, Ali M, Boylan JE. The impact of temporal aggregation on supply chains with ARMA(1,1) demand processes. Eur J Oper Res. 2019;273(3):920–32. https://doi.org/10.1016/J.EJOR.2018.09.010 .
Beyer MA, Laney D. The importance of ‘big data’: a definition. Stamford: Gartner; 2012. p. 2014–8.
Benabdellah AC, Benghabrit A, Bouhaddou I, Zemmouri EM. Big data for supply chain management: opportunities and challenges. In: Proceedings of IEEE/ACS international conference on computer systems and applications, AICCSA, no. 11, p. 20–26; 2016. https://doi.org/10.1109/AICCSA.2016.7945828 .
Kumar M. Applied big data analytics in operations management. Appl Big Data Anal Oper Manage. 2016. https://doi.org/10.4018/978-1-5225-0886-1 .
Zhong RY, Huang GQ, Lan S, Dai QY, Chen X, Zhang T. A big data approach for logistics trajectory discovery from RFID-enabled production data. Int J Prod Econ. 2015;165:260–72. https://doi.org/10.1016/J.IJPE.2015.02.014 .
Varela IR, Tjahjono B. Big data analytics in supply chain management: trends and related research. In: 6th international conference on operations and supply chain management, vol. 1, no. 1, p. 2013–4; 2014. https://doi.org/10.13140/RG.2.1.4935.2563 .
Han J, Kamber M, Pei J. Data mining: concepts and techniques. Burlington: Morgan Kaufmann Publishers; 2013. https://doi.org/10.1016/B978-0-12-381479-1.00001-0 .
Book MATH Google Scholar
Arunachalam D, Kumar N. Benefit-based consumer segmentation and performance evaluation of clustering approaches: an evidence of data-driven decision-making. Expert Syst Appl. 2018;111:11–34. https://doi.org/10.1016/J.ESWA.2018.03.007 .
Chase CW. Next generation demand management: people, process, analytics, and technology. Hoboken: Wiley; 2016.
Book Google Scholar
SAS Institute. Demand-driven forecasting and planning: take responsiveness to the next level. 13; 2014. https://www.sas.com/content/dam/SAS/en_us/doc/whitepaper2/demand-driven-forecasting-planning-107477.pdf .
Acar Y, Gardner ES. Forecasting method selection in a global supply chain. Int J Forecast. 2012;28(4):842–8. https://doi.org/10.1016/J.IJFORECAST.2011.11.003 .
Ma S, Fildes R, Huang T. Demand forecasting with high dimensional data: the case of SKU retail sales forecasting with intra- and inter-category promotional information. Eur J Oper Res. 2016;249(1):245–57. https://doi.org/10.1016/J.EJOR.2015.08.029 .
Addo-Tenkorang R, Helo PT. Big data applications in operations/supply-chain management: a literature review. Comput Ind Eng. 2016;101:528–43. https://doi.org/10.1016/J.CIE.2016.09.023 .
Agrawal S, Singh RK, Murtaza Q. A literature review and perspectives in reverse logistics. Resour Conserv Recycl. 2015;97:76–92. https://doi.org/10.1016/J.RESCONREC.2015.02.009 .
Gunasekaran A, Kumar Tiwari M, Dubey R, Fosso Wamba S. Big data and predictive analytics applications in supply chain management. Comput Ind Eng. 2016;101:525–7. https://doi.org/10.1016/J.CIE.2016.10.020 .
Hazen BT, Skipper JB, Ezell JD, Boone CA. Big data and predictive analytics for supply chain sustainability: a theory-driven research agenda. Comput Ind Eng. 2016;101:592–8. https://doi.org/10.1016/J.CIE.2016.06.030 .
Hofmann E, Rutschmann E. Big data analytics and demand forecasting in supply chains: a conceptual analysis. Int J Logist Manage. 2018;29(2):739–66. https://doi.org/10.1108/IJLM-04-2017-0088 .
Jain A, Sanders NR. Forecasting sales in the supply chain: consumer analytics in the big data era. Int J Forecast. 2019;35(1):170–80. https://doi.org/10.1016/J.IJFORECAST.2018.09.003 .
Jin J, Liu Y, Ji P, Kwong CK. Review on recent advances in information mining from big consumer opinion data for product design. J Comput Inf Sci Eng. 2018;19(1):010801. https://doi.org/10.1115/1.4041087 .
Kumar R, Mahto D. Industrial forecasting support systems and technologies in practice: a review. Glob J Res Eng. 2013;13(4):17–33.
MathSciNet Google Scholar
Mishra D, Gunasekaran A, Papadopoulos T, Childe SJ. Big Data and supply chain management: a review and bibliometric analysis. Ann Oper Res. 2016;270(1):313–36. https://doi.org/10.1007/s10479-016-2236-y .
Ren S, Zhang Y, Liu Y, Sakao T, Huisingh D, Almeida CMVB. A comprehensive review of big data analytics throughout product lifecycle to support sustainable smart manufacturing: a framework, challenges and future research directions. J Clean Prod. 2019;210:1343–65. https://doi.org/10.1016/J.JCLEPRO.2018.11.025 .
Singh Jain AD, Mehta I, Mitra J, Agrawal S. Application of big data in supply chain management. Mater Today Proc. 2017;4(2):1106–15. https://doi.org/10.1016/J.MATPR.2017.01.126 .
Souza GC. Supply chain analytics. Bus Horiz. 2014;57(5):595–605. https://doi.org/10.1016/J.BUSHOR.2014.06.004 .
Tiwari S, Wee HM, Daryanto Y. Big data analytics in supply chain management between 2010 and 2016: insights to industries. Comput Ind Eng. 2018;115:319–30. https://doi.org/10.1016/J.CIE.2017.11.017 .
Zhong RY, Newman ST, Huang GQ, Lan S. Big Data for supply chain management in the service and manufacturing sectors: challenges, opportunities, and future perspectives. Comput Ind Eng. 2016;101:572–91. https://doi.org/10.1016/J.CIE.2016.07.013 .
Ramanathan U, Subramanian N, Parrott G. Role of social media in retail network operations and marketing to enhance customer satisfaction. Int J Oper Prod Manage. 2017;37(1):105–23. https://doi.org/10.1108/IJOPM-03-2015-0153 .
Coursera. Supply chain planning. Coursera E-Learning; 2019. https://www.coursera.org/learn/planning .
Villegas MA, Pedregal DJ. Supply chain decision support systems based on a novel hierarchical forecasting approach. Decis Support Syst. 2018;114:29–36. https://doi.org/10.1016/J.DSS.2018.08.003 .
Ma J, Kwak M, Kim HM. Demand trend mining for predictive life cycle design. J Clean Prod. 2014;68:189–99. https://doi.org/10.1016/J.JCLEPRO.2014.01.026 .
Hamiche K, Abouaïssa H, Goncalves G, Hsu T. A robust and easy approach for demand forecasting in supply chains. IFAC-PapersOnLine. 2018;51(11):1732–7. https://doi.org/10.1016/J.IFACOL.2018.08.206 .
Da Veiga CP, Da Veiga CRP, Catapan A, Tortato U, Da Silva WV. Demand forecasting in food retail: a comparison between the Holt-Winters and ARIMA models. WSEAS Trans Bus Econ. 2014;11(1):608–14.
Murray PW, Agard B, Barajas MA. Forecasting supply chain demand by clustering customers. IFAC-PapersOnLine. 2015;48(3):1834–9. https://doi.org/10.1016/J.IFACOL.2015.06.353 .
Ramos P, Santos N, Rebelo R. Performance of state space and ARIMA models for consumer retail sales forecasting. Robot Comput Integr Manuf. 2015;34:151–63. https://doi.org/10.1016/J.RCIM.2014.12.015 .
Schaer O, Kourentzes N. Demand forecasting with user-generated online information. Int J Forecast. 2019;35(1):197–212. https://doi.org/10.1016/J.IJFORECAST.2018.03.005 .
Pang Y, Yao B, Zhou X, Zhang Y, Xu Y, Tan Z. Hierarchical electricity time series forecasting for integrating consumption patterns analysis and aggregation consistency; 2018. In: IJCAI international joint conference on artificial intelligence; 2018, p. 3506–12.
Goyal R, Chandra P, Singh Y. Suitability of KNN regression in the development of interaction based software fault prediction models. IERI Procedia. 2014;6:15–21. https://doi.org/10.1016/J.IERI.2014.03.004 .
Runkler TA. Data analytics (models and algorithms for intelligent data analysis). In: Revista Espanola de las Enfermedades del Aparato Digestivo (Vol. 26, Issue 4). Springer Fachmedien Wiesbaden; 2016. https://doi.org/10.1007/978-3-658-14075-5 .
Nikolopoulos KI, Babai MZ, Bozos K. Forecasting supply chain sporadic demand with nearest neighbor approaches. Int J Prod Econ. 2016;177:139–48. https://doi.org/10.1016/j.ijpe.2016.04.013 .
Gaur M, Goel S, Jain E. Comparison between nearest Neighbours and Bayesian network for demand forecasting in supply chain management. In: 2015 international conference on computing for sustainable global development, INDIACom 2015, May; 2015, p. 1433–6.
Burney SMA, Ali SM, Burney S. A survey of soft computing applications for decision making in supply chain management. In: 2017 IEEE 3rd international conference on engineering technologies and social sciences, ICETSS 2017, 2018, p. 1–6. https://doi.org/10.1109/ICETSS.2017.8324158 .
González Perea R, Camacho Poyato E, Montesinos P, Rodríguez Díaz JA. Optimisation of water demand forecasting by artificial intelligence with short data sets. Biosyst Eng. 2019;177:59–66. https://doi.org/10.1016/J.BIOSYSTEMSENG.2018.03.011 .
Vhatkar S, Dias J. Oral-care goods sales forecasting using artificial neural network model. Procedia Comput Sci. 2016;79:238–43. https://doi.org/10.1016/J.PROCS.2016.03.031 .
Wong WK, Guo ZX. A hybrid intelligent model for medium-term sales forecasting in fashion retail supply chains using extreme learning machine and harmony search algorithm. Int J Prod Econ. 2010;128(2):614–24. https://doi.org/10.1016/J.IJPE.2010.07.008 .
Liu C, Shu T, Chen S, Wang S, Lai KK, Gan L. An improved grey neural network model for predicting transportation disruptions. Expert Syst Appl. 2016;45:331–40. https://doi.org/10.1016/J.ESWA.2015.09.052 .
Yuan WJ, Chen JH, Cao JJ, Jin ZY. Forecast of logistics demand based on grey deep neural network model. Proc Int Conf Mach Learn Cybern. 2018;1:251–6. https://doi.org/10.1109/ICMLC.2018.8527006 .
Amirkolaii KN, Baboli A, Shahzad MK, Tonadre R. Demand forecasting for irregular demands in business aircraft spare parts supply chains by using artificial intelligence (AI). IFAC-PapersOnLine. 2017;50(1):15221–6. https://doi.org/10.1016/J.IFACOL.2017.08.2371 .
Huang L, Xie G, Li D, Zou C. Predicting and analyzing e-logistics demand in urban and rural areas: an empirical approach on historical data of China. Int J Performabil Eng. 2018;14(7):1550–9. https://doi.org/10.23940/ijpe.18.07.p19.15501559 .
Saha C, Lam SS, Boldrin W. Demand forecasting for server manufacturing using neural networks. In: Proceedings of the 2014 industrial and systems engineering research conference, June 2014; 2015.
Chang P-C, Wang Y-W, Tsai C-Y. Evolving neural network for printed circuit board sales forecasting. Expert Syst Appl. 2005;29(1):83–92. https://doi.org/10.1016/J.ESWA.2005.01.012 .
Merkuryeva G, Valberga A, Smirnov A. Demand forecasting in pharmaceutical supply chains: a case study. Procedia Comput Sci. 2019;149:3–10. https://doi.org/10.1016/J.PROCS.2019.01.100 .
Yang CL, Sutrisno H. Short-term sales forecast of perishable goods for franchise business. In: 2018 10th international conference on knowledge and smart technology: cybernetics in the next decades, KST 2018, p. 101–5; 2018. https://doi.org/10.1109/KST.2018.8426091 .
Villegas MA, Pedregal DJ, Trapero JR. A support vector machine for model selection in demand forecasting applications. Comput Ind Eng. 2018;121:1–7. https://doi.org/10.1016/J.CIE.2018.04.042 .
Wu Q. The hybrid forecasting model based on chaotic mapping, genetic algorithm and support vector machine. Expert Syst Appl. 2010;37(2):1776–83. https://doi.org/10.1016/J.ESWA.2009.07.054 .
Guanghui W. Demand forecasting of supply chain based on support vector regression method. Procedia Eng. 2012;29:280–4. https://doi.org/10.1016/J.PROENG.2011.12.707 .
Sarhani M, El Afia A. Intelligent system based support vector regression for supply chain demand forecasting. In: 2014 2nd world conference on complex systems, WCCS 2014; 2015, p. 79–83. https://doi.org/10.1109/ICoCS.2014.7060941 .
Chen IF, Lu CJ. Sales forecasting by combining clustering and machine-learning techniques for computer retailing. Neural Comput Appl. 2017;28(9):2633–47. https://doi.org/10.1007/s00521-016-2215-x .
Fasli M, Kovalchuk Y. Learning approaches for developing successful seller strategies in dynamic supply chain management. Inf Sci. 2011;181(16):3411–26. https://doi.org/10.1016/J.INS.2011.04.014 .
Islek I, Oguducu SG. A retail demand forecasting model based on data mining techniques. In: IEEE international symposium on industrial electronics; 2015, p. 55–60. https://doi.org/10.1109/ISIE.2015.7281443 .
Kilimci ZH, Akyuz AO, Uysal M, Akyokus S, Uysal MO, Atak Bulbul B, Ekmis MA. An improved demand forecasting model using deep learning approach and proposed decision integration strategy for supply chain. Complexity. 2019;2019:1–15. https://doi.org/10.1155/2019/9067367 .
Loureiro ALD, Miguéis VL, da Silva LFM. Exploring the use of deep neural networks for sales forecasting in fashion retail. Decis Support Syst. 2018;114:81–93. https://doi.org/10.1016/J.DSS.2018.08.010 .
Punam K, Pamula R, Jain PK. A two-level statistical model for big mart sales prediction. In: 2018 international conference on computing, power and communication technologies, GUCON 2018; 2019. https://doi.org/10.1109/GUCON.2018.8675060 .
Puspita PE, İnkaya T, Akansel M. Clustering-based Sales Forecasting in a Forklift Distributor. In: Uluslararası Muhendislik Arastirma ve Gelistirme Dergisi, 1–17; 2019. https://doi.org/10.29137/umagd.473977 .
Thomassey S. Sales forecasts in clothing industry: the key success factor of the supply chain management. Int J Prod Econ. 2010;128(2):470–83. https://doi.org/10.1016/J.IJPE.2010.07.018 .
Brentan BM, Ribeiro L, Izquierdo J, Ambrosio JK, Luvizotto E, Herrera M. Committee machines for hourly water demand forecasting in water supply systems. Math Probl Eng. 2019;2019:1–11. https://doi.org/10.1155/2019/9765468 .
Mafakheri F, Breton M, Chauhan S. Project-to-organization matching: an integrated risk assessment approach. Int J IT Project Manage. 2012;3(3):45–59. https://doi.org/10.4018/jitpm.2012070104 .
Mafakheri F, Nasiri F. Revenue sharing coordination in reverse logistics. J Clean Prod. 2013;59:185–96. https://doi.org/10.1016/J.JCLEPRO.2013.06.031 .
Bogataj M. Closed Loop Supply Chain (CLSC): economics, modelling, management and control. Int J Prod Econ. 2017;183:319–21. https://doi.org/10.1016/J.IJPE.2016.11.020 .
Hopkins J, Hawking P. Big Data Analytics and IoT in logistics: a case study. Int J Logist Manage. 2018;29(2):575–91. https://doi.org/10.1108/IJLM-05-2017-0109 .
de Oliveira CM, Soares PJSR, Morales G, Arica J, Matias IO. RFID and its applications on supply chain in Brazil: a structured literature review (2006–2016). Espacios. 2017;38(31). https://www.scopus.com/inward/record.uri?eid=2-s2.0-85021922345&partnerID=40&md5=f062191611541391ded4cdb73eea55cb .
Griva A, Bardaki C, Pramatari K, Papakiriakopoulos D. Retail business analytics: customer visit segmentation using market basket data. Expert Syst Appl. 2018;100:1–16. https://doi.org/10.1016/J.ESWA.2018.01.029 .
Lee CKM, Ho W, Ho GTS, Lau HCW. Design and development of logistics workflow systems for demand management with RFID. Expert Syst Appl. 2011;38(5):5428–37. https://doi.org/10.1016/J.ESWA.2010.10.012 .
Mohebi E, Marquez L. Application of machine learning and RFID in the stability optimization of perishable foods; 2008.
Jiao Z, Ran L, Zhang Y, Li Z, Zhang W. Data-driven approaches to integrated closed-loop sustainable supply chain design under multi-uncertainties. J Clean Prod. 2018;185:105–27.
Levis AA, Papageorgiou LG. Customer demand forecasting via support vector regression analysis. Chem Eng Res Des. 2005;83(8):1009–18. https://doi.org/10.1205/CHERD.04246 .
Chi H-M, Ersoy OK, Moskowitz H, Ward J. Modeling and optimizing a vendor managed replenishment system using machine learning and genetic algorithms. Eur J Oper Res. 2007;180(1):174–93. https://doi.org/10.1016/J.EJOR.2006.03.040 .
Sun Z-L, Choi T-M, Au K-F, Yu Y. Sales forecasting using extreme learning machine with applications in fashion retailing. Decis Support Syst. 2008;46(1):411–9. https://doi.org/10.1016/J.DSS.2008.07.009 .
Efendigil T, Önüt S, Kahraman C. A decision support system for demand forecasting with artificial neural networks and neuro-fuzzy models: a comparative analysis. Expert Syst Appl. 2009;36(3):6697–707. https://doi.org/10.1016/J.ESWA.2008.08.058 .
Lee CC, Ou-Yang C. A neural networks approach for forecasting the supplier’s bid prices in supplier selection negotiation process. Expert Syst Appl. 2009;36(2):2961–70. https://doi.org/10.1016/J.ESWA.2008.01.063 .
Chen F-L, Chen Y-C, Kuo J-Y. Applying Moving back-propagation neural network and Moving fuzzy-neuron network to predict the requirement of critical spare parts. Expert Syst Appl. 2010;37(9):6695–704. https://doi.org/10.1016/J.ESWA.2010.04.037 .
Wu Q. Product demand forecasts using wavelet kernel support vector machine and particle swarm optimization in manufacture system. J Comput Appl Math. 2010;233(10):2481–91. https://doi.org/10.1016/J.CAM.2009.10.030 .
Babai MZ, Ali MM, Boylan JE, Syntetos AA. Forecasting and inventory performance in a two-stage supply chain with ARIMA(0,1,1) demand: theory and empirical analysis. Int J Prod Econ. 2013;143(2):463–71. https://doi.org/10.1016/J.IJPE.2011.09.004 .
Kourentzes N. Intermittent demand forecasts with neural networks. Int J Prod Econ. 2013;143(1):198–206. https://doi.org/10.1016/J.IJPE.2013.01.009 .
Lau HCW, Ho GTS, Zhao Y. A demand forecast model using a combination of surrogate data analysis and optimal neural network approach. Decis Support Syst. 2013;54(3):1404–16. https://doi.org/10.1016/J.DSS.2012.12.008 .
Arunraj NS, Ahrens D. A hybrid seasonal autoregressive integrated moving average and quantile regression for daily food sales forecasting. Int J Prod Econ. 2015;170:321–35. https://doi.org/10.1016/J.IJPE.2015.09.039 .
Di Pillo G, Latorre V, Lucidi S, Procacci E. An application of support vector machines to sales forecasting under promotions. 4OR. 2016. https://doi.org/10.1007/s10288-016-0316-0 .
da Veiga CP, da Veiga CRP, Puchalski W, dos Coelho LS, Tortato U. Demand forecasting based on natural computing approaches applied to the foodstuff retail segment. J Retail Consumer Serv. 2016;31:174–81. https://doi.org/10.1016/J.JRETCONSER.2016.03.008 .
Chawla A, Singh A, Lamba A, Gangwani N, Soni U. Demand forecasting using artificial neural networks—a case study of American retail corporation. In: Applications of artificial intelligence techniques in wind power generation. Integrated Computer-Aided Engineering; 2018, p. 79–90. https://doi.org/10.3233/ica-2001-8305 .
Pereira MM, Machado RL, Ignacio Pires SR, Pereira Dantas MJ, Zaluski PR, Frazzon EM. Forecasting scrap tires returns in closed-loop supply chains in Brazil. J Clean Prod. 2018;188:741–50. https://doi.org/10.1016/J.JCLEPRO.2018.04.026 .
Fanoodi B, Malmir B, Jahantigh FF. Reducing demand uncertainty in the platelet supply chain through artificial neural networks and ARIMA models. Comput Biol Med. 2019;113:103415. https://doi.org/10.1016/J.COMPBIOMED.2019.103415 .
Sharma R, Singhal P. Demand forecasting of engine oil for automotive and industrial lubricant manufacturing company using neural network. Mater Today Proc. 2019;18:2308–14. https://doi.org/10.1016/J.MATPR.2019.07.013 .
Tanizaki T, Hoshino T, Shimmura T, Takenaka T. Demand forecasting in restaurants using machine learning and statistical analysis. Procedia CIRP. 2019;79:679–83. https://doi.org/10.1016/J.PROCIR.2019.02.042 .
Wang C-H, Chen J-Y. Demand forecasting and financial estimation considering the interactive dynamics of semiconductor supply-chain companies. Comput Ind Eng. 2019;138:106104. https://doi.org/10.1016/J.CIE.2019.106104 .
Download references
Acknowledgements
The authors are very much thankful to anonymous reviewers whose comments and suggestion were very helpful in improving the quality of the manuscript.
Author information
Authors and affiliations.
Concordia Institute for Information Systems Engineering (CIISE), Concordia University, Montreal, H3G 1M8, Canada
Mahya Seyedan & Fereshteh Mafakheri
You can also search for this author in PubMed Google Scholar
Contributions
The authors contributed equally to the writing of the paper. First author conducted the literature search. Both authors read and approved the final manuscript.
Corresponding author
Correspondence to Fereshteh Mafakheri .
Ethics declarations
Ethics approval.
Not applicable.
Competing interests
The authors declare no competing or conflicting interests.
Additional information
Publisher's note.
Springer Nature remains neutral with regard to jurisdictional claims in published maps and institutional affiliations.
Rights and permissions
Open Access This article is licensed under a Creative Commons Attribution 4.0 International License, which permits use, sharing, adaptation, distribution and reproduction in any medium or format, as long as you give appropriate credit to the original author(s) and the source, provide a link to the Creative Commons licence, and indicate if changes were made. The images or other third party material in this article are included in the article's Creative Commons licence, unless indicated otherwise in a credit line to the material. If material is not included in the article's Creative Commons licence and your intended use is not permitted by statutory regulation or exceeds the permitted use, you will need to obtain permission directly from the copyright holder. To view a copy of this licence, visit http://creativecommons.org/licenses/by/4.0/ .
Reprints and permissions
About this article
Cite this article.
Seyedan, M., Mafakheri, F. Predictive big data analytics for supply chain demand forecasting: methods, applications, and research opportunities. J Big Data 7 , 53 (2020). https://doi.org/10.1186/s40537-020-00329-2
Download citation
Received : 05 April 2020
Accepted : 17 July 2020
Published : 25 July 2020
DOI : https://doi.org/10.1186/s40537-020-00329-2
Share this article
Anyone you share the following link with will be able to read this content:
Sorry, a shareable link is not currently available for this article.
Provided by the Springer Nature SharedIt content-sharing initiative
- Demand forecasting
- Closed-loop supply chains
- Machine-learning

During the last decade, a cascading series of unpredictable events—including earthquakes, volcanic eruptions, catastrophic storms, disease outbreaks and armed conflicts—has exposed deep fragilities in global supply chains. These events served as initial alarm bells for much greater challenges to come.
Intricately woven supply chains were built on concepts such as just-in-time manufacturing and designed to reduce labor and operating costs. Over the years, companies relentlessly optimized their supply chains to serve markets with relatively predictable supply and demand patterns. However, recent and unprecedented events have shown how these choices have created inflexible supply chains that are brittle under stress.
Breaking a single link in a globalized supply chain can have a ripple effect, impacting customers thousands of miles away from the point of disruption. “Supply chain issues” has become a catchphrase for economic dislocation.
“In recent years, supply chain has gone from the background, something people did not think about, to a boardroom-level topic,” says Rob Cushman, Senior Partner, IBM Supply Chain Transformation. “It’s a concept that people have had very painful personal experiences with. And that’s why thinking about supply chain is pivoting from cost to being about resilience and agility, and ultimately driving growth.”
Cost savings
By deploying a cognitive supply chain, IBM reduced supply chain costs by USD 160 million and built in more resilience and agility
100% order fulfillment
Even during the peak of the covid-19 pandemic, IBM maintained a 100% order fulfillment rate of its products to clients
The worldwide reach, size and complexity of its supply chain organization represented a significant challenge as IBM began exploring transformation strategies for delivering a differentiated customer experience to promote customer loyalty and growth. IBM employs supply chain staff in 40 countries and makes hundreds of thousands of customer deliveries and service calls in over 170 nations. IBM also collaborates with hundreds of suppliers across its multi-tier global network to build highly configurable and customized products to customer specifications.
Previously, the IBM supply chain ran on legacy systems spread across different organizational silos, making information sharing slow and incomplete. Employees also performed much of their work on spreadsheets, which impeded collaboration and real-time data transparency.
However, at the same time the IBM supply chain was re-thinking business processes and transforming its technology platforms, IBM was making major strides in AI, cloud, data fabric, IoT, edge computing and other tools. “We saw the advances IBM was making in all these new technologies,” says Ron Castro, Vice President of IBM Supply Chain. “So, we asked, ‘Why not leverage our own technology to move our own supply chain forward?’”
“The principle behind why we embarked on this journey was to answer the question, ‘How can we best react to disruptions to manage resiliency and our client experience?’” says Castro. “We needed to identify disruptions quickly, analyze the data, get insights and decide on the best course of action.”
IBM supply chain management set out a bold vision to build its first cognitive supply chain. The aim was to have an agile supply chain that extensively uses data and AI to lower costs, exceed customer expectations, ruthlessly eliminate or automate non-value add work and exponentially improve the experience of supply chain colleagues.
IBM Consulting® was brought in at the beginning to help develop the processes required to drive the transformation. “We consider ourselves ‘Client Zero’ for IBM Consulting,” says Debbie Powell, IBM Digital Supply Chain Transformation Leader. “We have the technology to do what we need to do. It’s the culture and the processes where change was needed. We also realized that a lot of our knowledge was tribal and often depended on one person. We needed to digitize and democratize knowledge to support decision-making throughout the organization.”
IBM Consulting helped the IBM supply chain team use Design Thinking methods to plan its digital transformation and move from sequential to continuous planning. “We put a lot of effort into agility and a cultural shift to empower people and adjust workflows in a controlled way,” says Matthias Gräfe, Director of IBM Supply Chain Transformation. “We went from a top-down approach to identifying personas from the bottom up, the people that actually make the decisions.”
“Successful digital transformation required us to challenge traditional ways of working that were held sacred for decades and win the hearts and minds of supply chain colleagues for change to stick,” says Takshay Aggarwal, Partner, IBM Supply Chain Transformation.
At a high level, the IBM supply chain digital transformation revolves around building sense-and-respond capabilities. This was accomplished by democratizing data and automating and augmenting decisions achieved by combining cognitive control tower, cognitive advisor, demand-supply planning and risk-resilience solutions. “We view the cognitive control tower as the single source of truth where you have access to all the data and it helps advise the best course of action,” says Castro. “It also helps gather insights from the information quickly across the end-to-end supply chain.”
The cognitive control tower is powered by the IBM® Cognitive Supply Chain Advisor 360 Solution, which runs on IBM Hybrid Cloud and on Red Hat® OpenShift® (link resides outside of ibm.com) software. Cognitive Advisor 360 enables real-time, intelligent supply chain visibility and transparency. It also senses and responds to changes in demand as they happen and simplifies the automation of supplier management.
The system uses IBM Watson® technology to enable natural language queries and responses, which accelerates the speed of decision-making and offers more options to correct issues. “I can ask—in natural language—about part shortages, order impacts, risks to revenues and trade-offs,” says Cushman. “There’s a button that recommends actions to solve issues — that’s what Watson does. It’s augmented intelligence so we empower people with better information to make data-driven decisions very quickly.”
“With the cognitive supply chain, we have the benefit of bringing in all these data from legacy systems and internal and external sources, as well as unstructured data, to apply advanced analytics and different elements of AI,” says Castro. “And since the system responds to natural language, think about the power of being able to extract data and get insights and recommendations without having to be an expert in a legacy system or an ERP platform.”
The IBM cognitive supply chain technology architecture also includes IBM Edge Application Manager , IBM Maximo® Visual Inspection and IBM Track and Trace IoT —an integrated stack of solutions that connect data end-to-end across the supply chain. “Our procurement, planning, manufacturing and logistics data are connecting in close to real time,” says Cushman. “That’s how we can share inbound information from suppliers, manufacturing status updates with our external manufacturing partners and delivery information with our customers.”
“We’ve added demand sensing, so that the solution pulses the market for changes in demand, predicting the future. We’ve also embedded a cloud-based risk management tool called Resilinc into our procurement and inbound parts management process,” says Cushman. “It essentially uses AI to crawl the web and if there is a disruption, we can take action quickly to secure a second supply source.”
On a minute-by-minute basis, one of the biggest advantages of IBM’s cognitive supply chain is that it provides employees with immediate access to the information they need to read and mitigate disruptions. “There is unbelievable power that comes from taking lots of disparate data and putting it where people can see and understand it,” says Cushman.
“The real-time, single-view of the truth increases the velocity of decisions and leverages rapid response,” says Castro. “It helps us develop ‘what-if’ scenario analysis from a planning perspective all the way through to the execution team and suppliers.”
In fast-moving, real-world situations, quick, informed decisions provide a competitive advantage. “In the past, a major disruption—such as the closing of a major airport—would take days for us to understand the immediate impacts. With our current solution, we have ‘what-if’ capability that brings this analysis down to minutes,” says Powell. “In a supply constrained environment, whoever gets the information first wins.”
Since its cognitive supply chain became operational, IBM has saved USD 160 million related to reduced inventory costs, optimized shipping costs, better decision-making and time savings. “When mitigating a part shortage, it used to take four to six hours per part number,” says Powell. “We’ve brought that down to minutes and made further improvements to seconds.”
“Where’s my stuff?” is a common question in the supply chain industry. Finding an answer can entail hours of phone calls, emails and ERP queries across different geographies. “We’ve built a solution where you can log in and enter an order and you’ll have an answer in about 17 seconds,” says Cushman. “That was an enormous pivot and a powerful change in how we do business.”
By using its cognitive supply chain platform, the IBM supply chain team is also able to create new capabilities much faster. “Years ago, when we started this journey, we needed a long, looping roadmap with one or two years required for major capability upgrades,” says Castro. “With this digital enterprise, we now have teams that complete deployments in two or three weeks. We’ve moved to much more agile development.”
Despite dislocations caused by the COVID-19 pandemic, IBM fulfilled 100% of its orders by using its cognitive supply chain to quickly re-source and re-route parts as necessary. “During the last two years, the IBM supply chain did not fall behind. We met our commitments. Everyone else was screaming supply chain issues and we’re shipping products,” says Daniel Thomas, IBM Business Optimization Manager and Chief of Staff. “We delivered on our promises during the height of the disruptive era we live in.”
“Guaranteed supply is important, but many of our clients are also looking for predictability of supply,” says Castro. “The tools we have now help us address both issues. They enable us to manage the demand side to meet the right client expectations.”
“We have a responsibility to inspire younger supply chain leaders who will keep the IBM supply chain at the cutting edge and beyond for years to come,” says Aggarwal. “People entering the work force today have different experiences than previous generations. They are digital natives and expect a consumer-grade experience when managing their work. As we embarked on our journey, we actively engaged them in designing workflows and digital capabilities. There were trials and tribulations and we had multiple failures in design and rollout. Architecting the cognitive supply chain, and learning from failures and successes, made our young leaders champions of the cognitive supply chain and constant innovators of new capabilities.”
“IBM is the only global services company with its own multibillion-dollar supply chain, and we’ve transformed it into a data-driven architecture to drive our business. There’s a richness of experience that we bring to client conversations because we’ve done this work for ourselves,” says Cushman. “It’s all about how a supply chain delivers a differentiated customer experience to enable stickiness and growth.”
“The collaboration between IBM Systems and IBM Consulting teams to transform our own business and demonstrate the power of exponential technologies in supply chain has been one of our finest moments as a company,” says Cushman. “We look forward to sharing our real-world experience and learnings with our worldwide community of customers, partners and clients.”
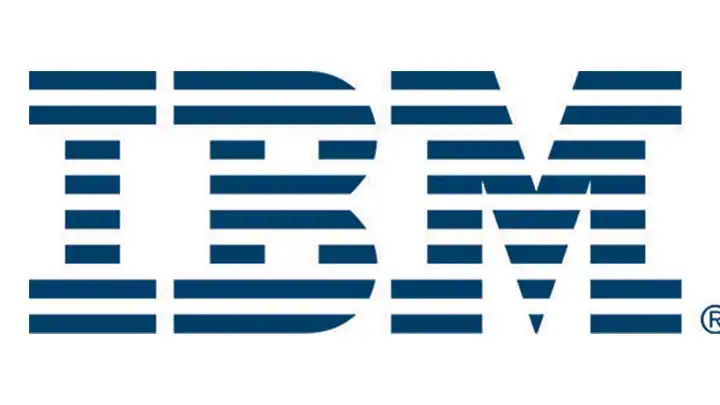
IBM is an information technology company based in Armonk, New York. Founded in 1911, the company offers hardware, software and services in cloud computing, AI, commerce, data and analytics, IoT, mobile and cybersecurity, as well as business resiliency, strategy and design solutions. IBM has a global workforce of more than 280,000 employees serving clients in over 175 countries through IBM Consulting, IBM Software and IBM Infrastructure.
To learn more about the IBM solutions featured in this story, please contact your IBM representative or IBM Business Partner.
Build AI-enabled, sustainable supply chains that prepare your business for the future of work, create greater transparency and improve employee and customer experiences
IBM Sterling Supply Chain Insights with Watson provides visibility across the entire supply chain.
Sourcing minerals responsibly with blockchain technology
© Copyright IBM Corporation 2022. IBM Corporation, New Orchard Road, Armonk, NY 10504
Produced in the United States of America, July 2022.
IBM, the IBM logo, ibm.com, IBM Consulting, IBM Watson and Maximo are trademarks of International Business Machines Corp., registered in many jurisdictions worldwide. Other product and service names might be trademarks of IBM or other companies. A current list of IBM trademarks is available on the web at ibm.com/legal/copyright-trademark .
Red Hat®, JBoss®, OpenShift®, Fedora®, Hibernate®, Ansible®, CloudForms®, RHCA®, RHCE®, RHCSA®, Ceph®, and Gluster® are trademarks or registered trademarks of Red Hat, Inc. or its subsidiaries in the United States and other countries.
This document is current as of the initial date of publication and may be changed by IBM at any time. Not all offerings are available in every country in which IBM operates.
The performance data and client examples cited are presented for illustrative purposes only. Actual performance results may vary depending on specific configurations and operating conditions. THE INFORMATION IN THIS DOCUMENT IS PROVIDED “AS IS” WITHOUT ANY WARRANTY, EXPRESS OR IMPLIED, INCLUDING WITHOUT ANY WARRANTIES OF MERCHANTABILITY, FITNESS FOR A PARTICULAR PURPOSE AND ANY WARRANTY OR CONDITION OF NON-INFRINGEMENT. IBM products are warranted according to the terms and conditions of the agreements under which they are provided.
- Artificial Intelligence
- Generative AI
- Business Operations
- Cloud Computing
- Data Center
- Data Management
- Emerging Technology
- Enterprise Applications
- IT Leadership
- Digital Transformation
- IT Strategy
- IT Management
- Diversity and Inclusion
- IT Operations
- Project Management
- Software Development
- Vendors and Providers
- Enterprise Buyer’s Guides
- United States
- Middle East
- España (Spain)
- Italia (Italy)
- Netherlands
- United Kingdom
- New Zealand
- Data Analytics & AI
- Newsletters
- Foundry Careers
- Terms of Service
- Privacy Policy
- Cookie Policy
- Copyright Notice
- Member Preferences
- About AdChoices
- Your California Privacy Rights

Our Network
- Computerworld
- Network World

Supply chain analytics: 3 success stories
With supply chain disruptions ongoing, organizations are leaning on analytics to glean insights into their supply chains and operations, as these three supply chain analytics examples attest..

The past year has seen a multitude of industries around the globe suffering supply chain disruptions, with little sign of that letting up in 2022. To navigate these supply chain issues, organizations are increasingly turning to analytics to gain better insight into their supply chains and operations.
Supply chain analytics draw data from procurement, inventory management, order management, warehouse management and fulfillment, transportation management, and other operations applications to provide the organization with insight into every step of its supply chain. These insights can be used to quick adjustments in the moment but can also be used to support long-term strategic decision-making.
Here are three examples of how organizations are using supply chain analytics effectively today.
Predictive analytics gives UPS insight into its logistics network
On average, UPS delivers roughly 21 million packages on any given day. That number gets far bigger in December. In the past, the shipping multinational has relied on historical data and know-how from expert planners to track package status. Today, it uses the Harmonized Enterprise Analytics Tool (HEAT) , a business intelligence platform, to capture and analyze customer data, operational data, and planning data to track the real-time status of every package as it moves across the company’s shipping network.
“HEAT helps us make better decisions in the way that we move packages across our network, the way that we plan our network, and the way that we provide information to our customers,” says Juan Perez, chief information and engineering officer of UPS. “It analyzes millions and millions of data points every single day to ensure that we are constantly providing the most-up-to-date information as to the status of a package, which then feeds all kinds of other systems that allow us to do better planning and better management of the network, better support in the way that we process packages across the organization.”
The HEAT platform analyzes more than 5.3 petabytes of data per week. It leverages predictive analytics, machine learning, and multi-model forecasting with proprietary randomness and seasonality growth factors to support forecasting, operations visibility, optimization, and reporting.
Perez’s advice: Think of your data strategy as a journey, rather than a destination.
“As big as we are and as good as we are with using data, one thing that I know is that the journey towards having a solid data strategy doesn’t really have an immediate end here for us,” Perez says. “We have to be constantly and constructively dissatisfied with the state of our technology and the state of our data, so we can be constantly making improvements to support the business.”
PepsiCo turns to predictive analytics to predict out-of-stocks
Food and beverage company PepsiCo is using analytics and machine learning to predict out-of-stocks and alert retailers to reorder.
“Certain products were flying off the shelves for various reasons early in the pandemic,” says Jason Fertel, ecommerce engineering head at PepsiCo. “Folks wanted to get as much oatmeal as possible, for example.”
Fortunately for PepsiCo, Fertel and his engineering organization within PepsiCo eCommerce had already been working on providing workflow automation for managing search marketing operations in the form of the company’s Sales Intelligence Platform. The platform combines retailer data with PepsiCo’s supply chain data to predict when items will go out of stock and prompt users to make purchases to replenish them.
Fertel’s advice: Find early adopters who are excited about your project and adopt a laser focus on a particular business problem.
“We want to do a lot of things, but we very much focused on out-of-stocks,” Fertel says. “There’s a whole slew of different verticals and sales intelligence that we go into, but we’ve been highly focused initially on out-of-stocks and I think that’s helped us find success.”
Pfizer’s digital transformation helps it manage its supply chain
Pharmaceutical titan Pfizer says its Global Supply – Digital Operations Center (DOC) project, has been critical to the company’s ability to manufacture and supply the Pfizer-BioNTech Covid-19 vaccine around the world.
The project is a “cockpit” for Pfizer operations, providing a shared view of end-to-end manufacturing and supply operational performance data for the company. Pfizer says the data has helped it identify opportunities to reduce up to 10% of cycle-time in some manufacturing areas and to maintain critical supply continuity for patients reliant on Pfizer pharmaceuticals.
“This solution has transformed how manufacturing colleagues collaborate and make decisions, providing tools to enable them to predict an issue before it happens and adjust in real time,” says Lidia Fonseca, executive vice president and chief digital and technology officer at Pfizer. “The DOC allows teams to mine data to provide analysis on variations compared to previously estimated standard lead-times, enabling further improvement opportunities.”
Fonseca’s advice: It’s all about culture. Pfizer’s move toward supply chain analytics has helped it transform into a leaner, more science-driven, more patient-focused organization. Success has required clearly communicating the company’s digital strategy to inspire employees’ support and participation.
“Our culture was instrumental in encouraging our employees to be courageous and think differently to accomplish what we previously would not have imagined possible.”
Related content
Zoho updates its collaboration tools to help with asynchronous work, possibilities at the edge: putting intelligence where your data is, genai demand helps aws accelerate revenue growth, is pc-as-a-service part of your hybrid work strategy, from our editors straight to your inbox.

Thor Olavsrud covers data analytics, business intelligence, and data science for CIO.com. He resides in New York.
More from this author
Data literacy, governance keys to transformation at dow, mulesoft unveils composability solution for gen ai, salesforce debuts gen ai benchmark for crm, team liquid tackles esports data with ai, ai is key player in texas rangers’ winning formula, porsche carrera cup brasil gets real-time data boost, sap customers see s/4hana and ai as top digital transformation drivers, red hat seeks to shrink it skills gap with lightspeed gen ai, most popular authors.
- Robert Mitchell Author

- Martin Bayer Editor in Chief B2B COMPUTERWOCHE, CIO, CSO in Germany
Show me more
Data transformation takes flight at atlanta’s hartsfield-jackson airport.

Making the gen AI and data connection work

Five generative AI tips for every business leader

CIO Leadership Live Australia with Alan Sharvin, Chief Information Officer, Tabcorp

CIO Leadership Live India with Irshad Saifi, Director IT and Digitization (CDIO), Shardul Amarchand Mangaldas & Co

CIO Leadership Live ASEAN with Sandeep Pandey, Group Chief Technology and Operations Officer, FWD Insurance

GitGuardian scans code to detect, fix secrets leaks

Sponsored Links
- The cloud shouldn’t be complicated. Unlock its potential with SAS.
- Everyone’s moving to the cloud. Are they realizing expected value?
- Everybody's ready for AI except your data. Unlock the power of AI with Informatica
- The future of identity is here. Unlock brand growth with Merkury
Top 5 Manufacturing Supply Chain Analytics Use Cases

- Manufacturing
- Supply Chain
- Data Analytics
Start with these 5 Manufacturing analytics use cases for supply chain
1. production analytics for operational efficiency, 2. supply chain analytics & risk management, 3. demand planning & forecasting, 4. inventory analytics for smarter stock management, 5. cost & overhead tracking with analytics, how polestar solutions can help you in supply chain management analytics.
Sign up to get the latest news and developments in technology, business analytics, data science and Polestar
Editor’s Note: From port congestion, spillover impacts from geopolitical conflicts, and freight transportation issues and more manufacturing and supply chain has become more challenging (like it wasn’t before) in the past few years. Understand impact of analytics in manufacturing specially for supply chain processes with these use cases.
For any successful manufacturing firm, it is very important to find a new way to streamline its operations. Starting from the raw materials to WIPs, logistics and of course, the final product, manufacturing is an intricate process with countless moving parts. For example, an average car engine would have more than 2000 individual components – think about the scale to extrapolate it for multiple vehicles and functions.
Besides the tangible aspects of the manufacturing industry, there are financial and managerial aspects to oversee, not to mention a perpetually changing market demand and aggressive competition.
Moving from pressure to profit through automation and innovation
The manufacturing industry is undergoing a lot of automation, cost pressure is always high and the margins thin. Bringing in efficiency and productivity gain is important to ensure you are competitive as well as profitable. Hence analysing different moving parts spread across functions to make sure they seamlessly work in tandem to bring down the cost, push up the utilization, and increase the margins.
Learn how powerful visualization and data analytics can help optimize your processes right from raw material sourcing to final product delivery
It takes months and extreme due diligence in examining each stage, coming up with innovative ideas and finally implementing them. The recent technological disruptions are not only good for manufacturers’ internal processes but are also a way to remain competitive and achieving organisation goals.
A World Economic Forum (WEF) and A.T. Kearney’s study of the future of production find that manufacturers are evaluating how combining emerging technologies including IoT, AI, and machine learning can improve asset tracking accuracy, supply chain visibility, and inventory optimization.

Source: WEF & A.T. Kearney Report
But, how can these organisations improve their underlying manufacturing processes and practices and practices before the enterprise-wide tech adoption?
There are a series of processes going on in parallel and it generates a huge volume of data ranging from machine run-time to no. of units produced. When all this information is decoupled, analyzed, and resynched together in a system, the results can be very powerful.
Machinery and systems are constantly operating for long stretches under heavy loads and any fault can significantly impact your production. As a reactive approach is not sustainable, by using predictive analytics systems, factory supervisors can predict failures in advance and avoid downtime. A practice that is catching trend is self-correcting machines that warn once such a threshold is achieved.
Machine utilisation and effectiveness data can lead to some crucial insights like
- The average run time for each machine and the reasons for any deviations from the schedule (human error, raw-material scarcity, technical issues, etc)
- Predictive Asset Maintenance - for which IoT data from sensors can be pulled and analysed to understand the pain areas and help in improving machine efficiency
- Monitor and predict the quality of goods based on machine sensors, quality control charts, and more.
These can show benefit by reducing the maintenance costs, improve machine efficiency, reliability and more. It is estimated that Predictive maintenance has the potential to reduce maintenance costs by 19%.
In addition to the manufacturing production line, the use of analytics is often limited to warehouse optimisation and forward logistics. However, while performing Reverse Logistics Analytics on numerous occasions organisations have dug up some hidden insights into sunk costs and inefficiencies cropping up from certain activities.
Learn how powerful visualization and data analytics can help optimize your processes right from raw material sourcing to final product delivery.
Analysis of returned items provide insights related to which stage of the production process is generating the maximum volume of faulty pieces or end products. It leads to avoiding loss emanating due to customers dissatisfaction as well as the sunk cost associated with manufacturing them. Further, it also adds to optimizing the existing processes, updating the vendor scorecard and ratings.
Advanced data analytics in manufacturing maximizes operational efficiency through three key applications: Predictive Asset Maintenance, Yield/throughput analysis and Supply chain optimization with advanced modelling.
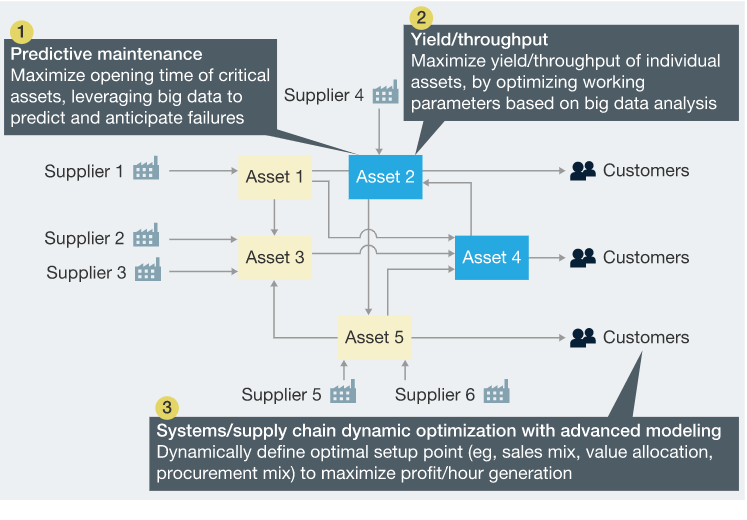
There are several areas of supply chain management where data analytics can be of significant help.
Now, Suppliers & Manufacturers have a choice to share their production data with their partners and customers to bring in transparency and gain trust. This way the manufacturer can see exactly whether the supplier is delayed with production just in time to avoid any additional lead time. At the same time, the suppliers can pre-empt any such incident and modify their production output accordingly.
A report by World Economic Forum , estimates the potential value of data sharing to be over $100 billion, by focusing on manufacturing process optimization based on best practices. The top 5 application domains include: Enhancing asset optimization, tracking products across value chain, tracing process conditions, exchanging digital product characteristics, and verifying provenance.
With greater visibility into supplier quality levels and their other performance metrics, manufacturers can:
- Have clear visibility on their supplier portfolio and have insightful data in their hands when it comes to supplier contract negotiations & improve supplier collaboration .
- Ensure consistency by tracking key quality metrics like dimensional accuracy and material composition across batches and suppliers to maintain product consistency.
- Anomaly detection to identify suppliers with higher defect rates to collaborate on improvement plans.
- Perform vendor evaluation on key metrics like quality, cost, and delivery times to negotiate better contracts.
Though supplier management is a vital part of any supply chain analytics solution there are a lot of KPIs that can be tracked, if interested you can find more procurement KPIs here .
Having supplier production and quality information available can also provide all the insight needed for better risk management. Supplier dependencies are quantifiable and with timely analysis of this information, the manufacturer can make fact-based decisions for Strategic Risk Management. Take a look at what have had the most impact on manufacturer’s supply chains:
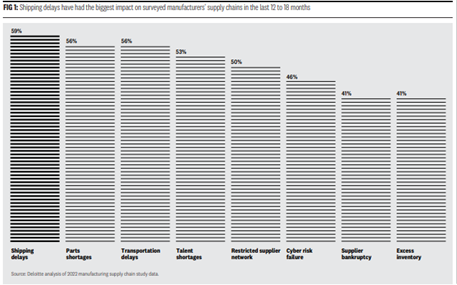
Source: Delloite
Mapping such delays to your supply chain and working on the controllable actions like shortages, restricted supply chain networks, excess inventories, etc. can tie combine data analytics and manufacturing operations efficiencies.
Manufacturers not only create products for their current customers only, but also for the perceived demand that they expect to emerge soon. Demand forecasting is key because it suggests an optimum capacity for a production chain and that can be the difference between strong sales or a warehouse full of unpurchased inventory.
Traditionally, forecasts are based on previous years’ historic values, and not on more actionable forward-looking data. By combining existing data with predictive analytics in manufacturing to build a more precise projection of what purchasing trends, seasonality, fads and disruptions could be, they can gain significant competitive advantage.
Additionally with demand forecasting as input, organizations can perform demand planning by incorporating the market and competition data as well as how well the production lines are operating i.e. by unconstrained and constrained demand , leading to better sales and operations planning, smarter risk management and lesser cost emerging due to access inventory storage space.
Demand planning and Demand forecasting are like the Yin-yang of S&OP one is the input for the other. Though demand planning remains at the top of the list, supply chain leaders are already trying to use other AI use cases in manufacturing, few of them include:
In addition to these manufacturing analytics examples, some other use cases include:
- Capacity Planning: To define the capacity and optimal units of the goods to manufacture for each production cycle, based on technology and human capital for a specific period. Considerations: capacity, sales forecasts, and parallel schedules.
- New Product demand: Forecast potential demand for new products based on market research and historical data and mitigate risks beforehand.
- Pricing and Promotion strategies: To create informed pricing strategies by understanding market demand, competitor pricing or real-time demand fluctuations.
Roping in the daily manufacturing data, the BI analytics dashboards can project a clear picture of the actual number of units manufactured over-reporting month in each production unit, making it easy for stakeholders to streamline the workflow and concentrate on the required areas.
With ever-growing SKUs, a limited shelf space, massive competitions, and thin margins manufacturers are always faced with pressure. This means that they have to ensure they have the right inventory at multiple levels right from warehouses to the retail shelfs. We’ve previously spoken about how sharing data can create more value synergy – this applies to inventory too as tracking products across value chain can lead to a potential gain of ~ $40 billion .
Additionally, it can help create true end-to-end visibility and bring more transparency. Depending on the number of companies participating you can create new inventory models upon which analytics can be done easily.

A few types of diagnostic inventory analytics techniques in manufacturing include:
- ABC Analysis – inventory is classified based on categories based on consumption value and contribution to profit.
- HML Analysis – inventory is classified based on cost.
- VED Analysis – inventory is classified based on user perception and experience.
- SDE Analysis - classified based on inventory availability and lead time.
- Inventory Aging analysis – classified based on the age of inventory
Additionally, manufacturers can leverage predictive analytics use cases like Collaborative Filtering, Causal Inference, Scenario Planning, Neural networks, multi-variate regression models, demand shaping techniques, exponential smoothening for short-term forecasting, etc., to find inferences and forecast data for the future.
Manufacturers must deal with a number of vendors and distribution partners to procure or distribute different parts or finished goods. It is very important to keep a check on the associated costs and the profitability numbers. Having a cost analysis solution that integrates all the information on a single platform and allows you to get actionable insights, can really bring efficiencies across these activities.
There are a lot of associated costs when manufacturers deal with vendors and distribution partners to procure or distribute different parts or finished goods. It is very important to keep a check on these costs and the profitability numbers.
Having a cost and spend analysis solution that integrates all the information on a single platform and allows you to get actionable insights, can really bring efficiencies across these activities.
Keeping track on the cost per unit of the item is important for a production manager as it impacts the pricing decisions and promotions as well . Calculating it requires data on costs like:
- Direct Labour & Direct Material (the cost of raw materials used)
- Overhead costs (Rent and utilities)
- Equipment and machinery allocated costs (depreciation)
- Licences and permits
- Packaging and shipping costs
- Cost of materials lost in production (waste and scrap)
Having a BI solution for reporting and visualisation can significantly reduce the risk and suggest timely corrections.
The excessive Overhead costs could be detrimental to manufacturing profitability. To have real control and visibility over these costs, connected data sources and advanced analytics capabilities are needed. Labor cost forms a major chunk of overhead costs. Hence, it is critical to link not only job roles and wages to certain processes but individuals. Employee badges can be tracked with sensors placed on the shop floor. This data can be analysed to assign the exact cost of each task in a process, broken down to an individual’s level. We are observing a growing adoption of these smart IoT devices in the factories .
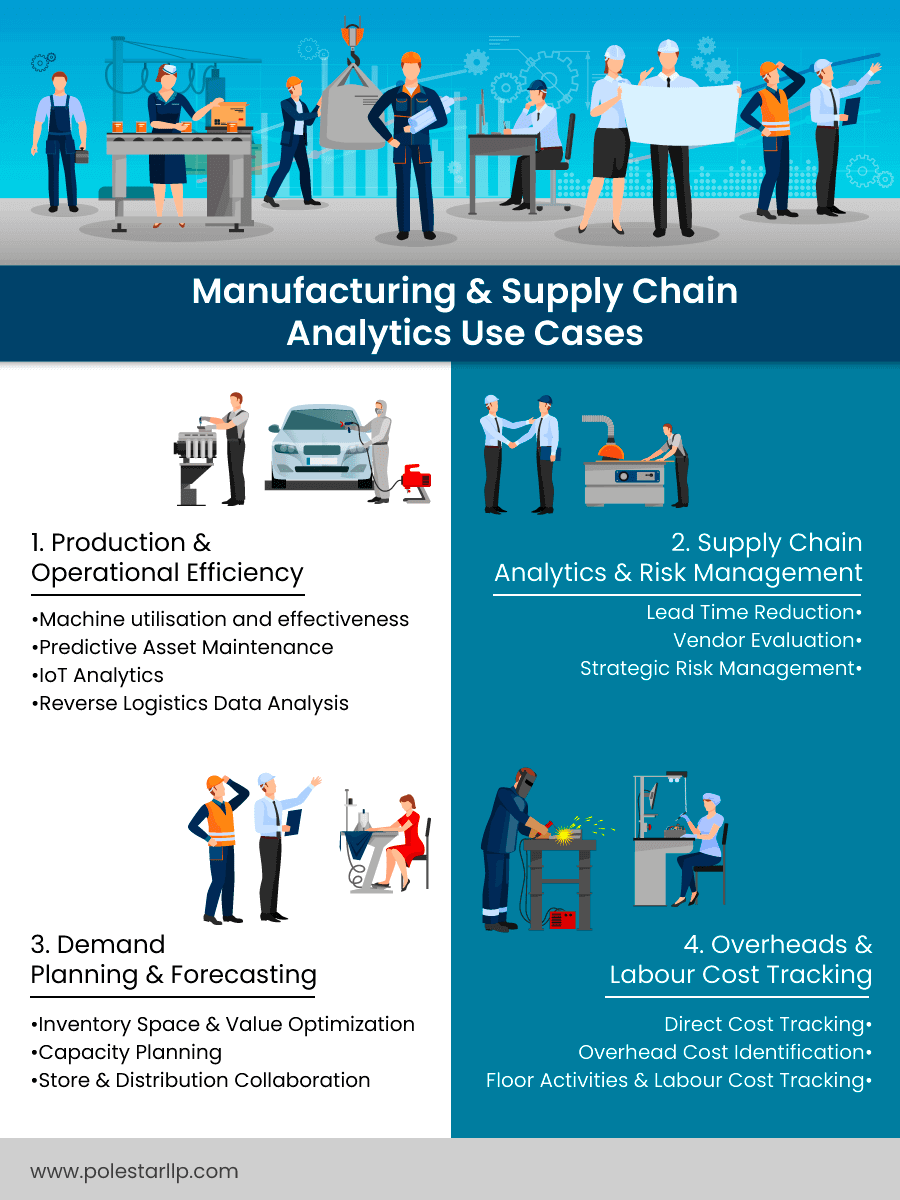
At Polestar Solutions , we help companies in becoming a connected enterprise where the data sources are connected and everyone in the company has access to the right data, at the right time to achieve operational efficiencies.
If you are looking for a data analytics partner in India or an offshore engagement, our data science teams can help you set up a robust data management and analytics platform. Why not today and let’s get started?
About Author

Data and Tech enthusiast
You can't hire somebody else to do your pushups for you.
Generally Talks About
Related blog

Supply Chain Analytics in Manufacturing – Everything You Need to Know
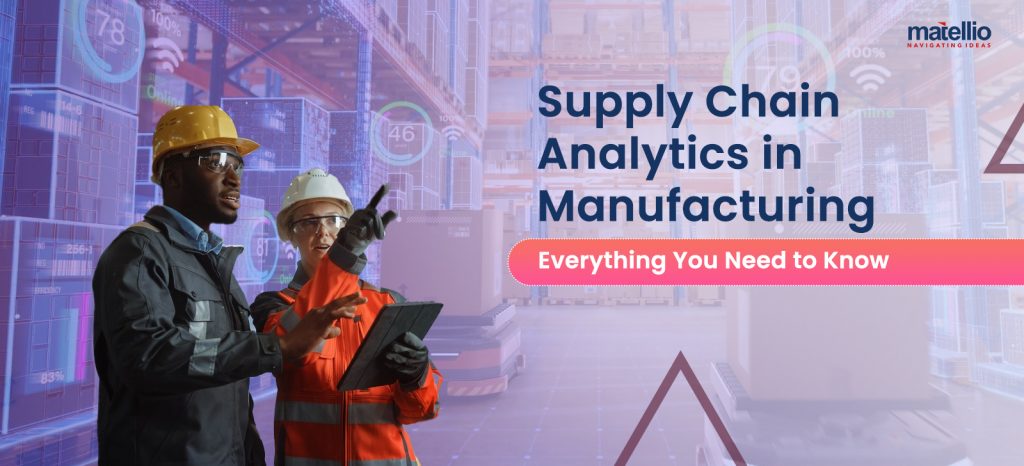
Picture this: It’s Monday morning, and you’re staring at a report showing missed deliveries, excess inventory, and rising production costs. Sound familiar? If you’re nodding your head, you’re not alone. But what if there was a way to turn this nightmare into a dream come true? Enter supply chain analytics in manufacturing – your secret weapon for operational excellence.
In today’s fast-paced, data-driven world, supply chain analytics in manufacturing is revolutionizing how companies operate, make decisions, and stay competitive. It’s not just a buzzword; it’s a game-changer that’s reshaping the entire manufacturing landscape. But what exactly is supply chain analytics in manufacturing, why should you care, and how can you implement it effectively? Let’s dive in and explore this transformative technology.
- Unleash the power of your supply chain data to drive operational excellence and profitability.
- Transform supply chain challenges into strategic opportunities through data-driven insights.
- Optimize inventory, production, and logistics for maximum efficiency and cost savings.
- Make informed, data-backed decisions to stay ahead of the competition.
- Partner with Matellio to embark on your supply chain analytics journey.
Table of Contents
Issues in Traditional Manufacturing Methods
Before we explore the role of manufacturing analytics, let’s take a moment to acknowledge the pain points you’re likely facing with traditional manufacturing methods:
- Inventory Mismanagement: The constant struggle of having too much stock tying up capital or too little leading to stockouts and dissatisfied customers.
- Production Inefficiencies: Unexpected machine downtime, quality issues, and inefficient processes eating into your profits and productivity.
- Demand Forecasting Challenges: The ongoing battle to predict what your customers will want next, leaving you constantly playing catch-up and missing opportunities.
- Supplier Relationship Struggles: Late deliveries, quality issues, and lack of visibility into your supplier network causing endless frustration and delays.
- Logistics Nightmares: Shipping delays, rising transportation costs, and lack of real-time tracking leaving you in the dark about your products’ whereabouts.
- Data Silos: Valuable information trapped in different departments or systems, preventing a holistic view of your operations.
- Reactive Decision Making: Constantly putting out fires instead of preventing them, leading to higher costs and missed opportunities.
These challenges aren’t just inconveniences – they’re roadblocks to growth, profitability, and competitiveness in an increasingly global market. But there’s good news: supply chain analytics in manufacturing offer a powerful solution to these age-old problems.
The Advent of Supply Chain Analytics in Manufacturing
So, what exactly is supply chain analytics in manufacturing? Think of it as a digital crystal ball for your operations. It’s the art and science of collecting, analyzing, and interpreting data from every nook and cranny of your supply chain to make smarter, faster, and more profitable decisions.
Supply chain analytics in manufacturing isn’t just about crunching numbers – it’s about turning those numbers into actionable insights that can transform your business. It’s like having a team of genius analysts working 24/7 to optimize every aspect of your operation.
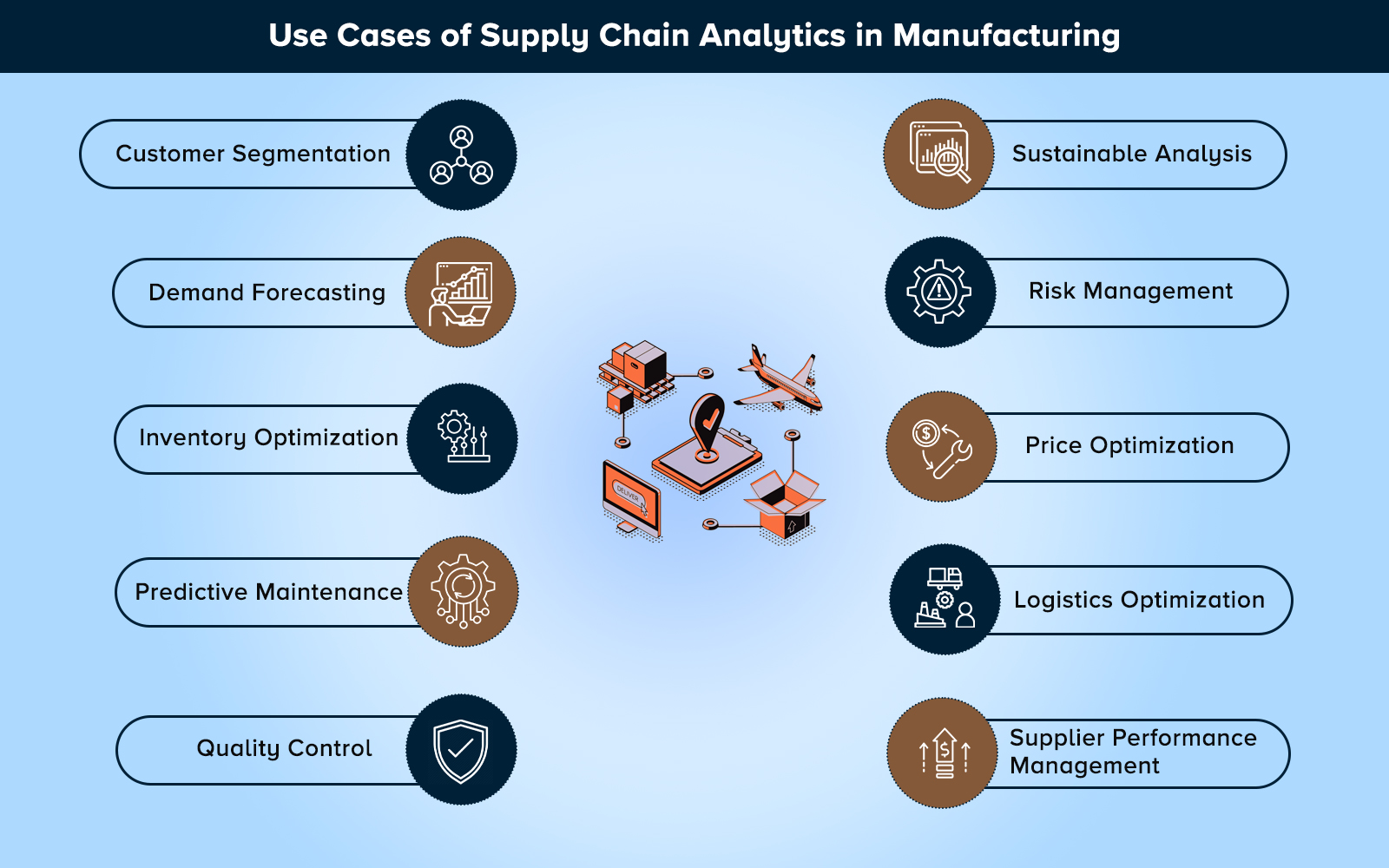
This revolutionary approach of analytics in manufacturing combines advanced technologies like artificial intelligence, machine learning, and big data analytics with traditional supply chain management principles. The result? A powerful tool that can predict trends, identify inefficiencies, and suggest optimizations in real-time.
Benefits of Supply Chain Analytics in Manufacturing: Your Ticket to Operational Excellence
Well, if you think that supply chain analytics is only about numbers and stats; well, you need to rethink! The benefits of implementing supply chain analytics in manufacturing are far-reaching and transformative.
As per a trusted source , 79% of companies with high-performing supply chains achieve greater revenue growth than the average for their industry. And that’s not all!
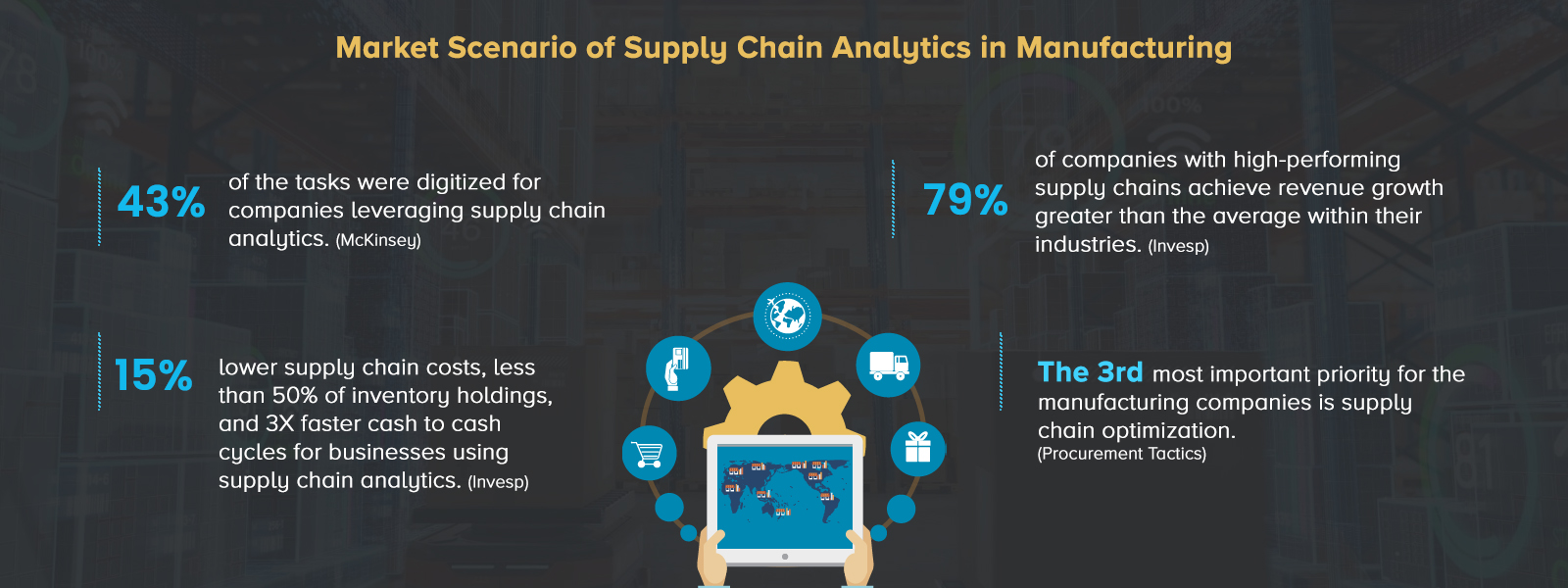
Here’s how supply chain analytics can revolutionize your operations:
Enhanced Visibility
Imagine having x-ray vision for your entire supply chain. That’s what supply chain analytics in manufacturing offers. No more guessing where your raw materials are, or when they’ll arrive. No more wondering about production status or delivery schedules. With real-time data at your fingertips, you’ll have a crystal-clear view of every aspect of your operation.
- Spot potential issues before they become problems
- Identify opportunities for improvement
- Make informed decisions based on a complete picture of your supply chain.
It’s like turning on the lights in a dark room – suddenly, everything becomes clear.
Improved Forecasting
Say goodbye to the crystal ball and hello to precision forecasting. Supply chain analytics in manufacturing doesn’t just look at your historical sales data; it considers market trends, economic indicators, social media sentiment, and even weather patterns to predict demand with uncanny accuracy.
- Imagine knowing exactly how much of each product you’ll need to produce next month, next quarter, or next year.
- No more overproduction leading to excess inventory, no more underproduction leading to lost sales.
- You’ll be able to align your production perfectly with demand, maximizing profits and minimizing waste.
Optimized Inventory Management
Picture this: you have the right amount of inventory – not too much tying up capital or too little risking stockouts. That’s the power of supply chain analytics in manufacturing.
- By analyzing demand patterns, lead times, and even supplier reliability, you can determine the optimal inventory levels for each product
- Reduce your carrying costs while ensuring you always have what you need on hand
It’s like having a just-in-time inventory system on steroids, perfectly balanced to meet your needs and your customers’ demands.
Streamlined Production
Imagine your production line running like a well-oiled machine, with no hiccups, no bottlenecks, and no wasted time. Supply chain analytics in manufacturing makes this a reality. By analyzing production data in real-time, you can identify inefficiencies, optimize schedules, and improve overall equipment effectiveness.
- Spot bottlenecks before they slow you down
- Optimize your production schedules to maximize efficiency
- Ensure that every minute of production time is used to its fullest potential
It’s like having a super-efficient production manager who never sleeps, always spotting ways to improve.
Proactive Maintenance
Picture a world where your machines never break down unexpectedly. With supply chain analytics in manufacturing, this becomes possible. By analyzing data from sensors on your equipment, you can predict when a machine will fail before it does.
- Schedule maintenance at the most opportune times
- Prevent unexpected breakdowns
- Extend the life of your assets
It’s like having a crystal ball for your machinery, allowing you to act before problems occur, not after.
Enhanced Quality Control
Imagine catching every quality issue before your products leave the factory. Supply chain analytics in manufacturing makes this possible. By analyzing production data in real-time, you can spot quality issues as they emerge, not after your products have been shipped.
- Identify the root causes of quality problems
- Make adjustments on the fly
- Ensure that only top-quality products reach your customers
It’s like having a thousand eagle-eyed quality inspectors working 24/7, catching every imperfection.
Improved Supplier Management
Picture having a scorecard for every supplier, based on objective data rather than gut feel. With supply chain analytics in manufacturing, you can:
- Track supplier performance in real-time
- Measure factors like on-time delivery, quality, price competitiveness, and more
- Use this data to have more productive conversations with your suppliers, identifying areas for improvement and recognizing top performers
It’s like having a relationship counselor for your supplier relationships, helping you build stronger, more productive partnerships.
Optimized Logistics
Imagine your products always taking the fastest, most cost-effective route with your customers. Analytics in manufacturing makes this a reality. By analyzing shipping data, traffic patterns, and even weather forecasts, you can optimize your logistics operations in real-time.
- Plan the most efficient routes
- Improve load planning to maximize truck capacity
- Track shipments in real-time to provide accurate ETAs to your customers
It’s like having a logistics superhero, ensuring your products always arrive on time and at the lowest possible cost.
Better Decision Making
Picture making every decision with the confidence of having all the facts at your fingertips. Custom manufacturing analytics software provides you with data-driven insights for every aspect of your operation.
- No more relying on gut feeling or outdated reports
- Leverage real-time, accurate data to inform every decision
- Get analytics on strategic choices about new product lines and tactical decisions about daily production schedules
It’s like having a team of expert advisors by your side 24/7, always ready with the insights you need to make the best possible decisions.
In short, in today’s fast-paced, ever-changing manufacturing landscape, supply chain analytics isn’t just a nice-to-have – it’s a must-have for survival and success.
It’s the key to unlocking efficiencies, reducing costs, improving quality, and staying ahead of the competition. With supply chain analytics in manufacturing, you’re not just keeping up with the future of manufacturing – you’re leading the way.
You don’t believe us? Here’s a stellar case study that shows the transformative power of analytics in manufacturing!
Case Study: Transforming AMZ Prep’s Supply Chain with Analytics
AMZ Prep, a leading FBA prep service provider, faced significant challenges in managing their supply chain due to manual processes. These outdated methods, including paper records and spreadsheets, led to labeling and packing errors, inefficiencies, and a lack of real-time visibility. These issues hindered operational efficiency and affected customer satisfaction.
Solution:
Matellio implemented a comprehensive digital transformation solution, emphasizing supply chain analytics. This involved replacing manual methods with a centralized digital system for managing merchant onboarding, inbound consignments, inventory management, and order fulfillment.
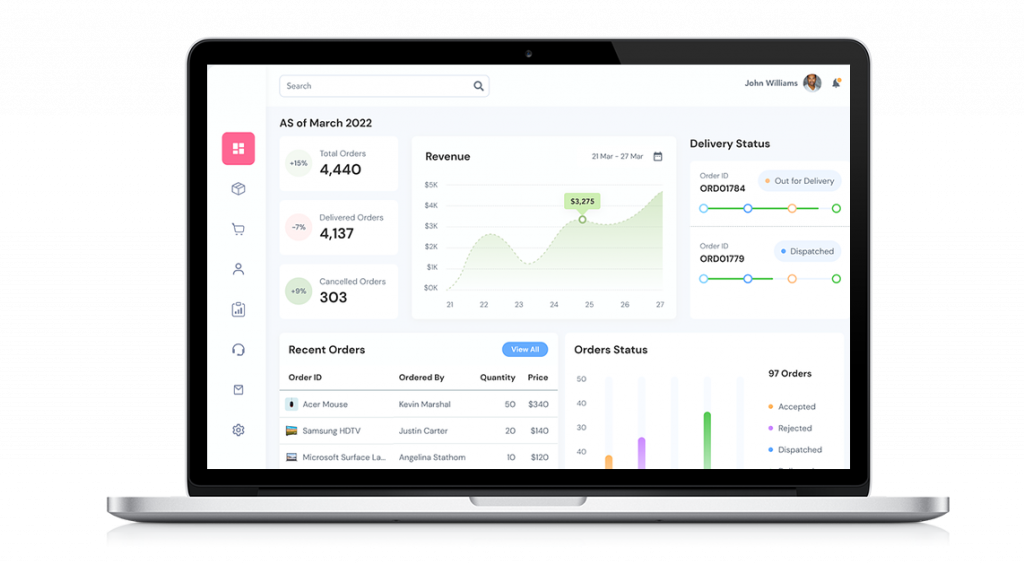
The new system integrated supply chain analytics, providing real-time insights into operations, enabling better decision-making, and ensuring compliance with Amazon’s evolving guidelines.
The solution’s scalability allowed AMZ Prep to accommodate more merchants and streamline operations. Additionally, the system facilitated automated retrieval and printing of FNSKU codes and shipment labels, further enhancing operational efficiency.
The integration of supply chain analytics transformed AMZ Prep’s operations, significantly reducing errors and processing times, leading to cost savings and enhanced customer satisfaction.
Real-time updates and data analytics kept AMZ Prep compliant with Amazon’s guidelines, avoiding penalties and disruptions. Most importantly, supply chain analytics empowered AMZ Prep to continuously monitor performance, make informed decisions, and optimize their supply chain, setting the stage for sustained success in the competitive FBA market.
Want a Similar Transformation for Your Company Using Supply Chain Analytics?
What is 5 + 3 =
Technology Trends Enhancing Supply Chain Analytics in Manufacturing
Now that you’ve seen how supply chain analytics can revolutionize manufacturing operations, let’s explore the cutting-edge technologies that are taking this power to the next level. These innovations are not just futuristic concepts – they’re tools that forward-thinking manufacturers are already using to gain a competitive edge.
Artificial Intelligence (AI) and Machine Learning (ML)
Imagine having a virtual data scientist working tirelessly to uncover insights from your supply chain data. That’s what AI and ML bring to the table. As per a trusted source, AI-powered supply chains are 67% more efficient than traditional supply chains . But what can AI integration services offer to your business?
- Predictive analytics on steroids: AI algorithms can process vast amounts of data to forecast demand, predict maintenance needs, and optimize inventory with unprecedented accuracy.
- Autonomous decision-making: Advanced AI systems can make real-time decisions, adjusting production schedules or reordering supplies without human intervention.
- Continuous improvement: ML models learn from each decision and outcome, constantly refining their predictions and recommendations.
Read More: How Can AI Help in Enhancing Supply Chain Management?
Internet of Things (IoT)
Picture your entire supply chain coming alive with data. That’s possible with IoT development services ! Connected devices and sensors provide real-time data from across the supply chain, enabling more accurate and timely analytics.
- Real-time visibility: Track the location and condition of raw materials, work-in-progress, and finished goods in real-time.
- Predictive maintenance: Sensors on machinery can detect early signs of wear, allowing you to schedule maintenance before breakdowns occur.
- Environmental monitoring: Ensure optimal conditions for sensitive materials or products throughout the supply chain.
5G Networks
Imagine having lightning-fast, ultra-reliable connectivity across your entire operation. That’s what 5G in supply chain analytics offer to your business! Faster, more reliable connectivity enables the transmission of larger volumes of data in real-time, enhancing analytics capabilities.
- Real-time data processing: Enable instantaneous data transmission from IoT devices, supporting split-second decision-making.
- Enhanced remote monitoring: Conduct detailed, real-time inspections of distant facilities or in-transit goods.
- Improved collaboration: facilitate seamless communication and data sharing across your global supply network.
Digital Twins
Picture having a virtual replica of your entire supply chain that you can experiment with risk-free. That’s what digital twin, a part of IIoT services , offers to your business! Virtual replicas of physical assets or processes allow for more accurate simulations and scenario planning.
- Scenario planning: Test different strategies and see their impact before implementing them in the real world.
- Process optimization: Identify inefficiencies and bottlenecks in a virtual environment.
- Training and simulation: Prepare your team for various scenarios without disrupting actual operations.
Edge Computing
By processing data closer to its source, edge computing enables faster, more efficient analytics, especially for real-time decision making. Imagine processing data right where it’s generated, without the delay of sending it to a central server:
- Faster response times: Make split-second decisions based on real-time data.
- Reduced bandwidth needs: Process data locally, sending only relevant information to the cloud.
- Enhanced security: Keep sensitive data close to its source, reducing transmission-related risks.
Advanced Analytics Platforms
Picture having a user-friendly interface that puts the power of complex analytics into the hands of every decision-maker. That’s what analytics in manufacturing offers for your business!
- Democratized data access: Enable team members at all levels to leverage data-driven insights.
- Customizable dashboards: Tailor data visualizations to specific roles and needs.
- Integrated analytics: Combine data from multiple sources for a holistic view of your supply chain.
Read More: Manufacturing Analytics Software Development – From Concept to Implementation
By leveraging these technologies, you’re not just implementing supply chain analytics – you’re future-proofing your manufacturing operations. These tools work synergistically with analytics to provide deeper insights, faster responses, and more accurate predictions.
Contact us to implement these technologies in your manufacturing business!
The Implementation Process: Bringing Supply Chain Analytics to Your Manufacturing Operations
Now that you’re excited about the potential of supply chain analytics in manufacturing, you might be wondering, “How do I actually implement this in my factory?” Don’t worry – we’ve got you covered. Here’s a step-by-step guide to bringing the power of supply chain analytics to your manufacturing operations:
Assess Your Current Supply Chain Landscape
Before diving into supply chain analytics, it’s crucial to understand your starting point. This assessment phase involves:
- Mapping your supply chain: Identify all key players, processes, and data points across your entire supply network.
- Auditing existing data sources: Pinpoint where you’re currently collecting data, from supplier performance metrics to production line sensors and logistics tracking systems.
- Evaluating data quality: Assess the accuracy, completeness, and timeliness of your current supply chain data.
- Reviewing your IT infrastructure: Examine your current hardware and software capabilities. Do you have the necessary computing power and integration capabilities to handle supply chain analytics?
- Identifying data silos: Look for isolated pockets of supply chain data that aren’t currently integrated with other systems.
This assessment will help you identify strengths to build upon and weaknesses to address in your supply chain analytics strategy. You can leverage technology consulting services to streamline this step!
Define Clear Objectives and KPIs
With a clear understanding of your current state, it’s time to set your goals. Consider:
- Specific supply chain challenges: What problems do you want supply chain analytics to solve? This could range from reducing lead times to optimizing inventory levels across your network.
- Measurable outcomes: Set concrete, quantifiable goals. For example, “Reduce supply chain costs by 20%” or “Improve on-time delivery performance by 15%.”
- Alignment with business strategy: Ensure your supply chain analytics objectives support your overall business goals.
- Time-bound targets: Set realistic timelines for achieving your objectives.
Remember, it’s often better to start with a few well-defined objectives rather than trying to solve everything at once.
Choose a Trusted Digital Transformation Services Company
Implementing supply chain analytics in manufacturing is a complex undertaking. Partnering with the right digital transformation services company can make all the difference. Look for a partner that:
- Has specific experience in custom manufacturing software development and data analytics services
- Offers end-to-end services, from strategy consulting to system integration and support
- Demonstrates a track record of successful supply chain analytics projects in manufacturing
- Can provide case studies and references from satisfied clients
- Has expertise in the latest supply chain technologies and analytics methodologies
A trusted partner will guide you through the complexities of supply chain analytics implementation, helping you avoid common pitfalls and accelerate your journey to data-driven manufacturing.
Develop a Comprehensive Supply Chain Data Strategy
Work with your chosen partner to create a robust data strategy that includes:
- Identifying critical data points: Determine what data you need to collect across your supply chain to meet your objectives.
- Data collection methods: Implement IoT devices, sensors, and integration with supplier systems to capture real-time supply chain data.
- Data integration plan: Develop a strategy to bring together data from various systems – ERP, warehouse management, transportation management, supplier portals, and even external sources like market trends or weather data.
- Data governance framework: Establish policies and procedures for data quality, security, and compliance across your supply chain.
- Scalability considerations: Ensure your data strategy can grow and adapt as your supply chain analytics needs evolve.
You can easily streamline this step by using data consultation services!
Implement the Right Technology Stack
Based on your data strategy, work with your digital transformation partner to:
- Select and implement a supply chain analytics platform: Choose a solution that can handle the volume, velocity, and variety of your supply chain data.
- Integrate with existing systems: Ensure seamless data flow between your analytics platform and other critical systems like ERP, MES, and CRM.
- Set up data visualization tools: Implement user-friendly dashboards that present key supply chain insights in an easily digestible format.
- Enable real-time analytics: Deploy systems that can process and analyze supply chain data as it’s generated, allowing for immediate action when issues arise.
- Incorporate advanced analytics capabilities: Implement predictive and prescriptive analytics models to forecast future supply chain scenarios and recommend optimal actions.
Develop and Deploy Analytics Models
With your data infrastructure in place, it’s time to turn that data into actionable insights:
- Create predictive models: Develop algorithms that can forecast demand, predict supply disruptions, or optimize inventory levels based on historical and real-time data.
- Implement machine learning: Use advanced analytics techniques to uncover hidden patterns in your supply chain and continuously improve your models over time.
- Design scenario planning tools: Build capabilities to simulate different supply chain scenarios and their potential impacts.
- Establish alert systems: Set up automated notifications for potential supply chain issues or opportunities.
APIs | GraphQL, RESTful APIs, EDI (Electronic Data Interchange) |
ETL Tools | Apache NiFi, Talend |
Databases | PostgreSQL, MongoDB, AWS S3, Azure Data Lake Storage |
Data Warehouses | Amazon Redshift, Google BigQuery, Snowflake |
Big Data Tools | Apache Hadoop, Apache Spark |
Machine Learning | Tensor, PyTorch, scikit-learn |
Business Intelligence Tools | Tableau, Looker, PowerBI |
Stream Processing Tools | Apache Kafka, Apache Flink, Apache Kinesis |
Monitoring Tools | Grafana, Prometheus |
Encryption Tools | OpenSSL, AWS KMS |
Compliance Frameworks | GDPR, ISO/IEC 27001 |
Cloud Platforms | AWS, Microsoft Azure, Google Cloud Platform (GCP) |
Foster a Data-Driven Supply Chain Culture
The final step is ensuring your team can effectively use and benefit from your new supply chain analytics capabilities:
- Provide comprehensive training: Offer workshops and hands-on sessions to familiarize employees with new supply chain analytics tools and dashboards.
- Develop data literacy: Help all employees understand the basics of supply chain data analysis and interpretation, not just your data scientists.
- Encourage data-driven decision making: Lead by example and incentivize the use of data in daily supply chain operations and strategic planning.
- Create cross-functional teams: Bring together individuals from different departments (procurement, production, logistics, sales) to collaborate on data-driven supply chain projects.
- Establish a continuous improvement mindset: Regularly review and refine your supply chain analytics processes based on feedback and results.
Remember, implementing supply chain analytics in manufacturing is an ongoing process. Start with a pilot project, learn from your experiences, and gradually expand your capabilities. With persistence and the right technology partner, you’ll transform your manufacturing operations into a data-driven powerhouse, setting new standards for efficiency, responsiveness, and innovation in your industry.
Ready to Implement Supply Chain Analytics in Your Business? Get a Free Consultation!
What is 5 + 2 =
Navigating the Challenges: Why Matellio is Your Ideal Supply Chain Analytics Partner
While the benefits of supply chain analytics in manufacturing are undeniable, the journey to implementation isn’t without its hurdles. But here’s the good news: with the right technology partner by your side, these challenges become opportunities for growth and innovation.
That’s Where Matellio Comes In!
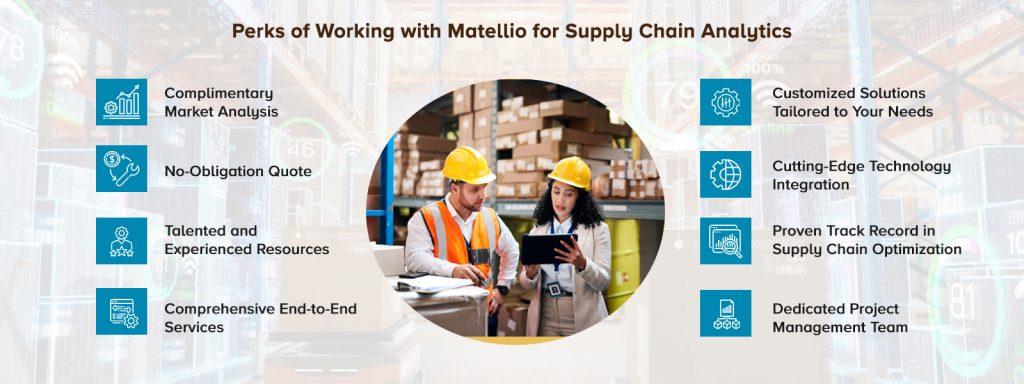
Let’s explore the potential roadblocks and how Matellio’s expertise can help you overcome them:
Taming the Data Beast
Challenge: Ensuring data quality, consistency, and integration across your entire supply chain can feel like herding cats.
Matellio’s Solution: Our data consultation services bring order to chaos. We’ll help you clean, organize, and integrate data from every corner of your supply chain, ensuring you’re working with a single source of truth.
Bridging the Skills Gap
Challenge: Finding professionals who understand both advanced analytics and manufacturing processes is like searching for unicorns.
Matellio’s Advantage: Our team of data scientists and industry experts bring the perfect blend of analytical prowess and manufacturing know-how. Plus, we’ll train your team, building in-house capabilities for long-term success.
Winning Hearts and Minds
Challenge: Overcoming resistance to new technologies and data-driven decision-making can be an uphill battle.
Matellio’s Approach: We don’t just implement technology; we drive cultural change. Our change management expertise ensures your team embraces supply chain analytics, from the factory floor to the C-suite.
Untangling the Technology Web
Challenge: Integrating new analytics tools with existing systems can feel like solving a Rubik’s cube blindfolded.
Matellio’s Expertise: Our custom solution design and technology implementation services ensure seamless integration. We’ll make your new analytics tools play nicely with your existing systems, creating a harmonious tech ecosystem.
Fortifying Your Data Fortress
Challenge: With increased data collection comes increased security risks. Protecting your supply chain data can keep you up at night.
Matellio’s Shield: Our cloud integration services , and cybersecurity expertise ensure your data is locked down tighter than Fort Knox, while still being accessible to those who need it.
Proving the Payoff
Challenge: Demonstrating ROI, especially in the short term, can be as tricky as nailing jelly to a wall.
Matellio’s Approach: We help you identify and track the right KPIs from day one. Our user-friendly dashboards make it easy to visualize and communicate the value of your analytics investment.
Scaling New Heights
Challenge: Ensuring your analytics solution can grow with your business is like planning for a child’s future – you need to think ahead.
Matellio’s Vision: Our scalable solutions and continuous improvement services ensure your analytics capabilities evolve as your business grows. We’re not just solving today’s problems; we’re preparing you for tomorrow’s opportunities.
Conclusion– Navigate the Challenges with Matellio
Implementing supply chain analytics in manufacturing isn’t just about overcoming challenges – it’s about seizing opportunities. With Matellio as your partner, you’re not just getting a service provider; you’re gaining a collaborator committed to your success.
Our end-to-end approach means we’re with you every step of the way:
- We’ll design a custom solution tailored to your unique needs and challenges.
- Our technical expertise ensures smooth implementation and integration.
- We’ll develop advanced analytics models that turn your data into actionable insights.
- Our user-friendly dashboards make complex data accessible to everyone in your organization.
- We’ll train your team and provide ongoing support, ensuring long-term success.
- Our continuous improvement services keep you at the cutting edge of supply chain analytics.
But perhaps most importantly, we understand that supply chain analytics is just one part of your broader digital transformation journey. Our expertise extends beyond analytics to encompass your entire digital strategy, ensuring you’re prepared for the future of manufacturing.
With Matellio, you’re not just implementing a tool; you’re transforming your entire approach to supply chain management. We’re here to help you turn data into decisions, insights into action, and challenges into competitive advantages.
Ready to revolutionize your supply chain? Let’s embark on this journey together. Contact us today for a free 30-minute consultation!
FAQ’s
Q1. what specific technologies do you integrate for supply chain analytics in manufacturing.
Matellio integrates technologies such as AI, machine learning, IoT, edge computing, and advanced analytics platforms. These technologies enable real-time data processing, predictive analytics, and comprehensive data integration.
Q2. How does Matellio ensure data security and compliance during digital transformation?
We implement robust security protocols, including encryption, secure data transmission, and regular compliance audits. Our cloud integration services, and cybersecurity measures are designed to protect sensitive supply chain data.
Q3. Can you customize analytics solutions for different manufacturing industries?
Yes, Matellio provides tailored analytics solutions based on the specific needs and challenges of each manufacturing sector. Our approach includes customizing data models, dashboards, and analytics tools to align with industry-specific requirements.
Q4. What kind of support do you offer post-implementation?
We offer continuous support and maintenance services, including system updates, troubleshooting, and performance monitoring. Additionally, we provide training to ensure your team can effectively utilize the analytics tools and insights.
Q5. How does Matellio help in scaling analytics solutions as our business grows?
Matellio designs scalable solutions that can evolve with your business. We plan for future growth by building flexible systems that accommodate increased data volumes, additional data sources, and expanding analytics capabilities, ensuring long-term scalability and efficiency.
Related articles
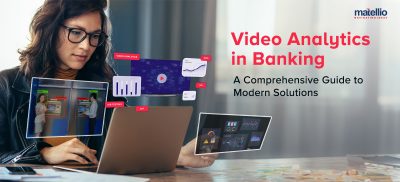
Video Analytics in Banking: A Comprehensive Guide to Modern Solutions
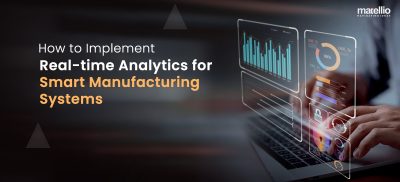
How to Implement Real-time Analytics for Smart Manufacturing Systems
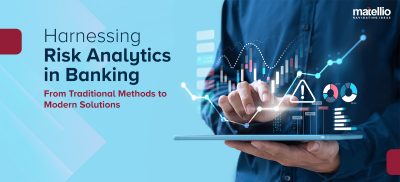
Harnessing Risk Analytics in Banking: From Traditional Methods to Modern Solutions
Enquire now.
Facing Development Hurdles? Let’s Conquer Them Together. Our expert team is ready to tackle your challenges, from streamlining processes to scaling your tech.
This site uses cookies to improve your experience. By viewing our content, you are accepting the use of cookies. To help us insure we adhere to various privacy regulations, please select your country/region of residence. If you do not select a country we will assume you are from the United States. View our privacy policy and terms of use.
- Inventory Management Software
- Forecasting
- Sustainability
- Supply Chain Visibility

How Technology Makes Continuous Innovation Possible: A Case Study with Unilever
Logistics Viewpoints
OCTOBER 5, 2023
Automatic data diagnostics ensures the quality of the data it receives, fixes the inconsistencies where it can, and alerts the planners in case their attention is required. Currently, she is COO and Head of Customer Success at Solvoyo, a leading supply chain planning and analytics SaaS company based in Boston.

AI in the Retail Industry: Benefits, Case Studies & Examples
MARCH 27, 2024
While on one hand, predictive analytics analyzes historical data and market trends for accurate demand forecasting, on the other, customers are being offered personalized experiences based on their preferences, purchase history and behavior. Such detailed analytics also reduce retrieval times for faster order processing.
This site is protected by reCAPTCHA and the Google Privacy Policy and Terms of Service apply.
Trending Sources
- The Logistics of Logistics
- Enchange Supply Chain Consultancy
- Enterra Insights
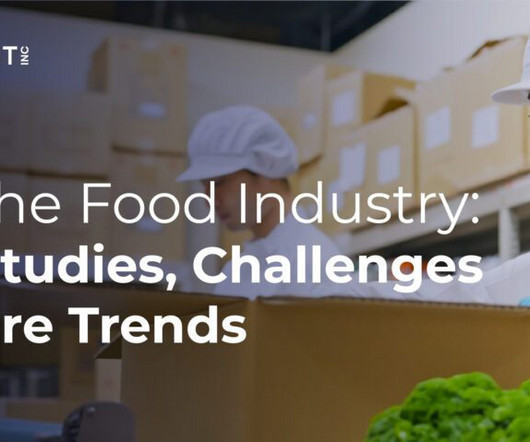
AI in the Food Industry: Case Studies, Challenges & Future Trends
MARCH 28, 2024
Food Production AI tools can drive advanced predictive analytics with precision forecasting for weather and crop yield predictions . Food Retail In the food retail sector, AI has been helping reduce wastage as the tools can more accurately predict the demand for products. All for the good.
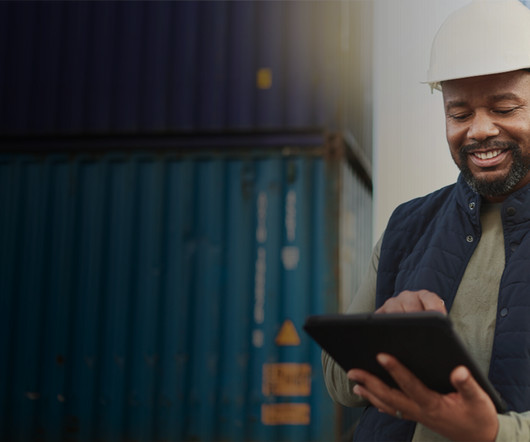
Supply Chain Predictive Analytics: Benefits, Use Cases and Growth Potentials
DECEMBER 6, 2023
Thanks to the more advanced forms of supply chain analytics like predictive analytics , supply chains are proactively looking into the future and prepping for “what is to come” rather than only ruminating over “what already happened.” What Is Predictive Analytics for Supply Chain?
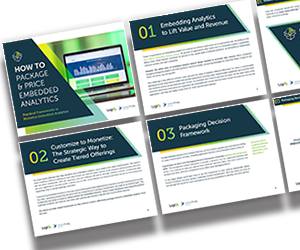
How to Package and Price Embedded Analytics
Advertisement
Just by embedding analytics , application owners can charge 24% more for their product. Brought to you by Logi Analytics . How much value could you add? This framework explains how application enhancements can extend your product offerings.
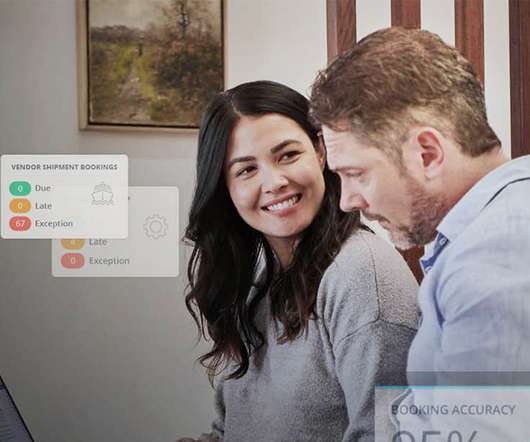
Case Study: Röhlig Logistics and Gravity Supply Chain Solutions
Gravity Supply Chain
JUNE 6, 2022
Receive live tracking and predictive analytics on their goods, from origin to destination. Download full case study here. The post Case Study : Röhlig Logistics and Gravity Supply Chain Solutions appeared first on Gravity Supply Chain. Easily place orders. Monitor the production process.
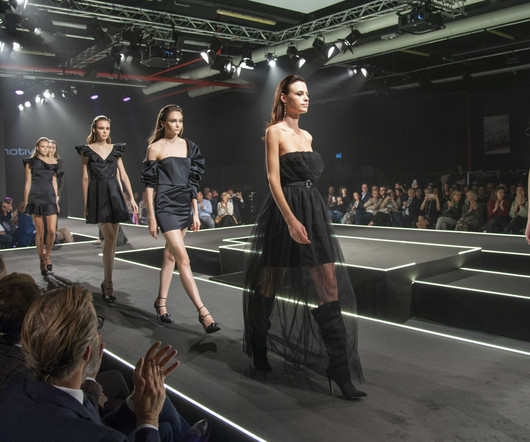
Miroglio Fashion: AI-Powered In-Season Inventory Optimization
FEBRUARY 28, 2024
This case study details how Miroglio used EvoAI to respond quickly to the smallest changes in demand and dynamically rebalanced inventory across the network to maximize GMROI. But fashion is inherently hard to predict . Read the case on Harvard Business School website Read here →
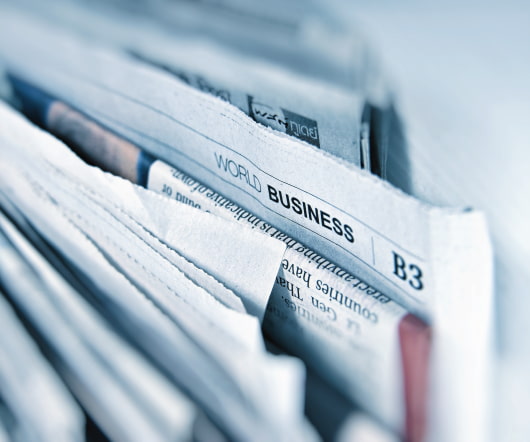
S&OP in Agribusiness: How to Harvest the Benefits with Advanced Analytics
JULY 23, 2019
But supporting the process with advanced analytics goes even further, contributing to higher levels of productivity and profitability. Like many organizations, Tereos recognizes the use of advanced analytics as an imperative. Many of these factors are difficult to control and predict . Advanced analytics as enabling technology.
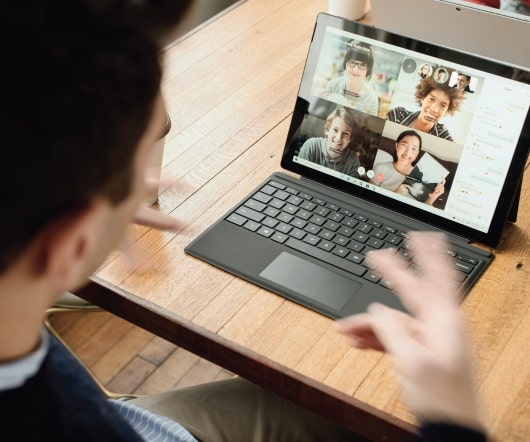
Catch up on Supply Chain Analytics news with our Summer Reading List
JULY 19, 2017
The list includes the best of our blog and news items, our latest webinars and most recent case studies . Looking for a software provider for Business Analytics , S&OP, Inventory Optimization, Production Planning & Scheduling or SC Network Design? It’s the perfect companion for an inspiring holiday break! .
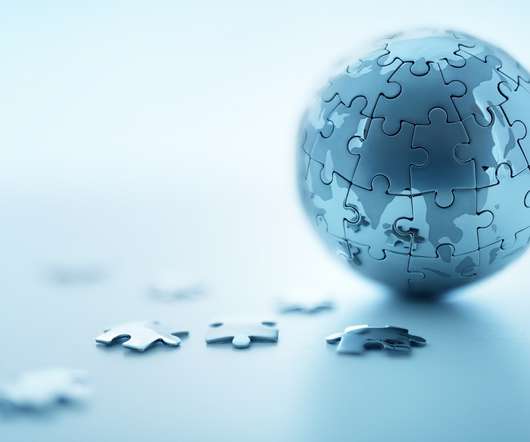
The list includes our best supply chain analytics blogs and news items, our latest webinars and most recent case studies . Looking for a software provider for Business Analytics , S&OP, Inventory Optimization, Production Planning & Scheduling or SC Network Design?
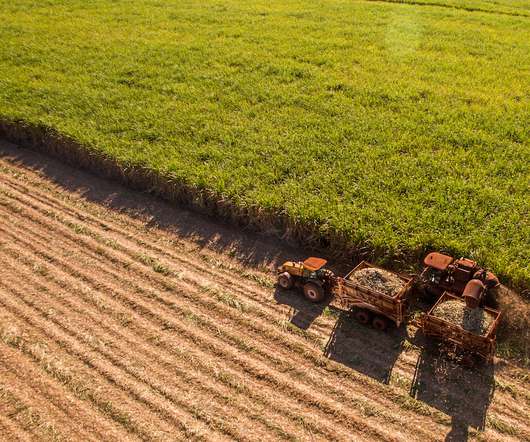
Prescriptive Analytics for Supply Chain Optimization: 6 Powerful Use Cases
DECEMBER 8, 2023
If there’s any piece of technology or analytics that can help with the most advanced data-driven decision-making in the supply chain right now, that’s prescriptive analytics . It is the most promising form of analytics in the market currently. What Is Prescriptive Analytics in Supply Chain? How should the supplier perform?

How to Accurately Predict Demand in the F&B Industry?
JUNE 19, 2024
Inaccurate predictions , especially for seasonal and short-lifecycle products, can severely impact operations. To mitigate these risks, the F&B sector must harness advanced analytics and machine learning. How to Accurately Predict Demand in the F&B Industry with ThroughPut AI?
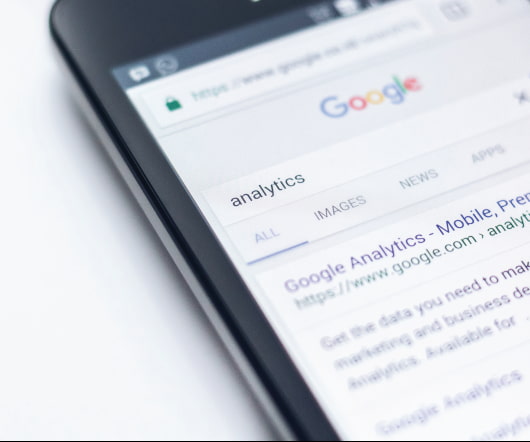
Supply Chain Innovation: The Latest Gartner Supply Chain Predictions
OCTOBER 13, 2015
This is a compilation of predictions and recommendations from various presentations. They see the highest spending priorities in e-commerce software, supply chain optimization and cost analytics . Cognitive learning and analytics – Burkett predicted that cognitive or machine learning will have a big impact on supply chains.
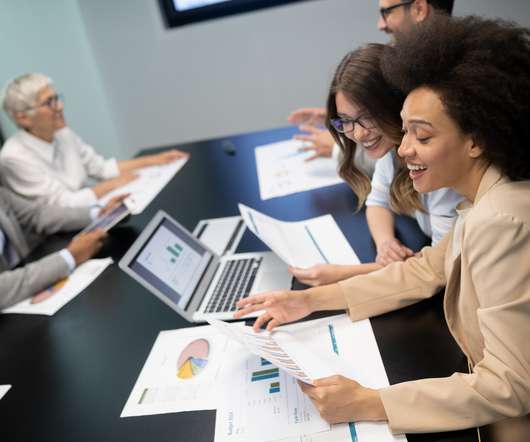
3 Compelling Reasons to Start Using Prescriptive Analytics
FEBRUARY 9, 2017
If you’ve read up on the latest topics in the field of data analysis, then you’ve probably encountered the term Prescriptive Analytics . Prescriptive Analytics is a type of Advanced Analytics that results in a recommended action. Supply chain teams are curious about adopting Prescriptive Analytics and exploring the benefits.
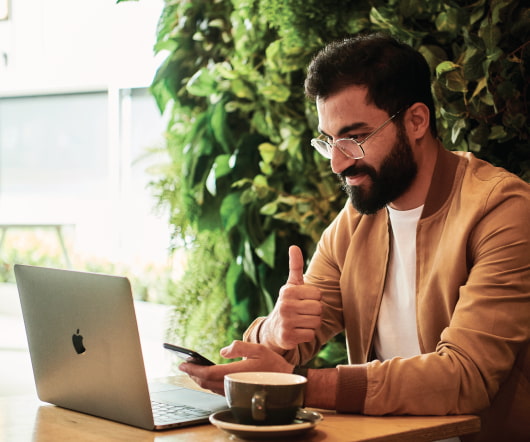
Coming Now: The Age of Advanced Demand Analytics
AUGUST 11, 2015
In her recent report, Making the Case for Increased Adoption of Advanced Supply Chain Analytics , Gartner analyst Noha Tohamy says that it’s no longer realistic to expect planners alone to analyze trends and underlying drivers, predict future scenarios and devise action plans. Danone is a case in point.
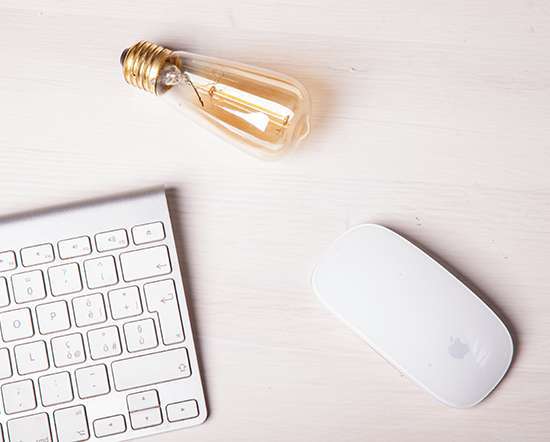
If you’ve read up on the latest topics in the field of data analysis, then you’ve probably encountered the term Prescriptive Analytics . Prescriptive Analytics is a type of Advanced Analytics that results in a recommended action. According to Gartner’s Forecast Snapshot , the Prescriptive Analytics software market will reach $1.1
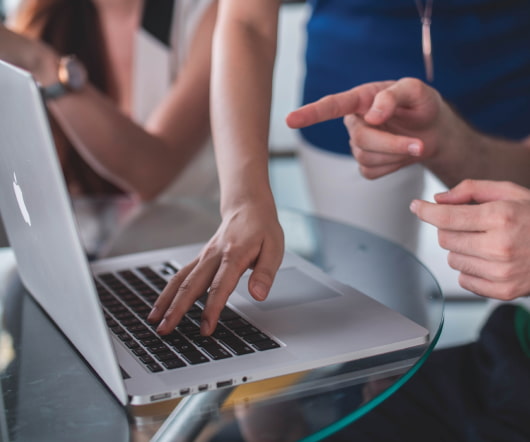
Supply Chain Analytics: The Times They Are A-Changin’
Supply Chain Shaman
FEBRUARY 5, 2015
My focus in this post is to highlight changes in supply chain analytics , and what this can mean for your business. Today, if you ask a supply chain leader about analytics , their response is all about reporting. If you ask an IT professional about analytics , their response is also usually centered in reporting.
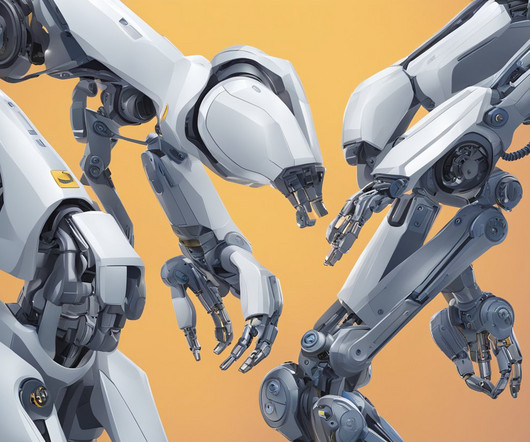
AI Business Process Automation: Revolutionizing Efficiency in the Corporate World
DECEMBER 14, 2023
As intelligent systems learn from data, they become better at predicting outcomes, streamlining operations, and enhancing customer experiences. By leveraging machine learning algorithms and data analytics , businesses are able to automate complex tasks that were previously manual, enhancing efficiency and productivity.
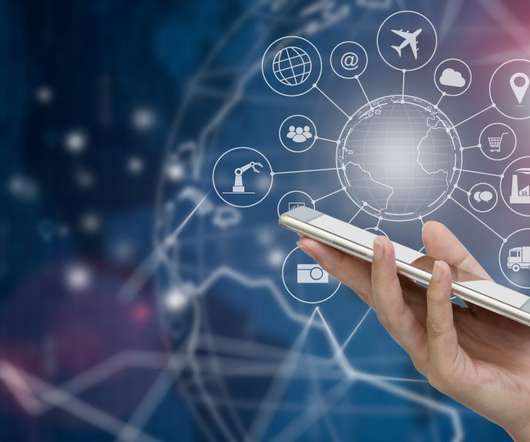
Importance of Digitalisation to Improve Supply Chains: Helping Businesses Navigate Through Supply Chain Disruptions
The Logistics & Supply Chain Management Society
AUGUST 19, 2022
Applying innovation to supply chains, combines innovative technologies like the Internet of Things (IoT), analytics , and robotics to supply chain management to improve performance and meet customer demands. brings innovations like IoT, robotics, data analytics , and other technologies to improve supply chain management and improve performance.

The Critical Path: Navigating Supply Chain Efficiency in the Oil Industry
JUNE 27, 2024
Artificial Intelligence (AI) : Improves decision-making through predictive analytics and machine learning. Advancements in technology are pivotal in reshaping the efficiency of oil supply chains: Internet of Things (IoT) : Enhances monitoring and management of assets. Blockchain : Ensures transparency and security in transactions.
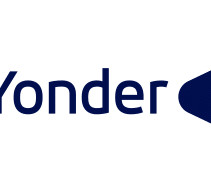
Blue Yonder ICON: Preparing for a New Tomorrow
MAY 10, 2023
Snowflake is a cloud computing–based data cloud company that offers a cloud-based data storage and analytics service, generally termed “data-as-a-service.” Both predictive and generative artificial intelligence play a role in supplying infinite intelligence, as Mr. Angove pointed out.
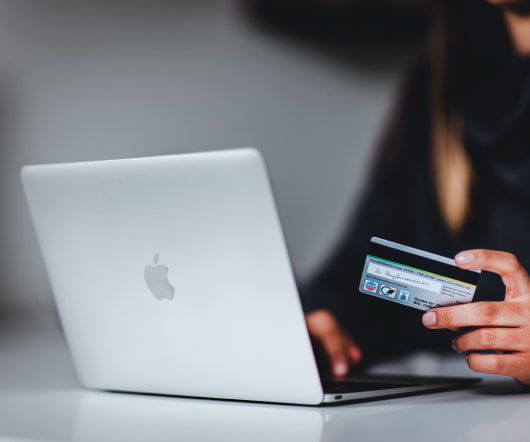
Google Will Acquire a 3PL – And Other Supply Chain and Logistics Predictions for 2015
Talking Logistics
DECEMBER 11, 2014
made that prediction in 2008 (see the Barron’s article What $300-a-Barrel Oil Will Mean for You ). Three years later, he stayed with his $300-a-barrel prediction , but shifted the timeframe to 2020 (see the CBS News article, Another $300 Oil Prediction — and Why This One Matters ). million bbl/d in 2015.” .
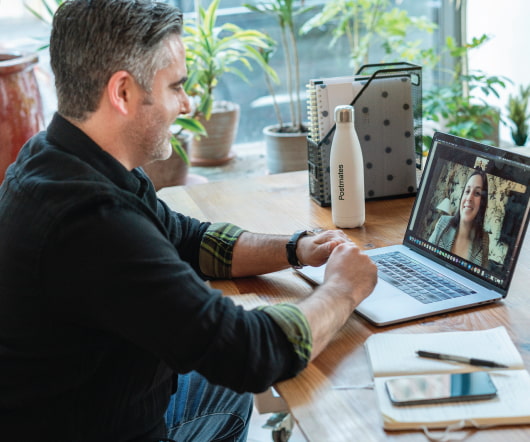
Tackling Item Complexity
JANUARY 9, 2019
Long tail products do not flow well through the traditional supply chain designed for high volume, predictable demand. In Figure 1, I share a case study from a client engagement. The demand patterns of the tail are different requiring a change in planning analytics . The reason? the organizational dynamics were tough.
Cognitive Computing: Getting Clear on Definitions
AUGUST 7, 2017
The decision support technologies that we use today–price management, trade promotion management, network design, supply chain planning, transportation planning, supplier risk management–are on the cusp of redefinition through new forms of analytics . Evolution of Analytics in Supply Chain Management. Predictive Analytics .
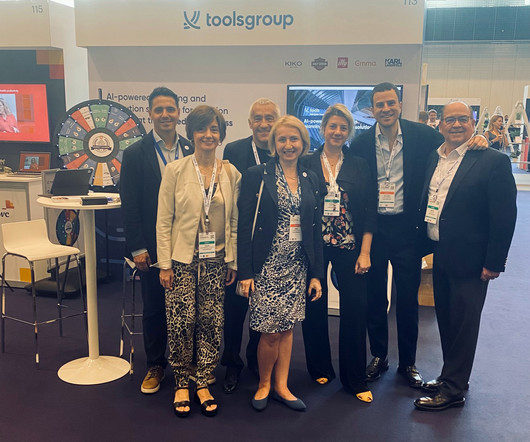
Your Must-Have Gartner EU Debrief: The TopTakeaways from Gartner Supply Chain Symposium/Xpo EU 2023
JUNE 15, 2023
With more visibility and predictive forecasting. Several use case sessions focused on the Importance of Real-Time Data to capture signals quickly, enabling better decision making faster within the supply chain. Analytics in the past were backward looking. Prescriptive analytics . Does not require real-time data.
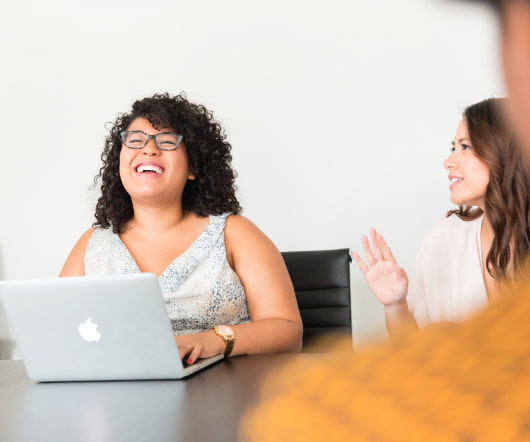
IBM Case Study Webinar: How AI can revolutionize your supply chain NOW
NC State SCRC
NOVEMBER 15, 2017
The focus was on the impact of AI on supply chain decision-making, and I spoke about a recent case study on IBM’s application of Watson to their own supply chain. Supply chain problems were always recognized after the fact, and were not being predicted ahead of time.
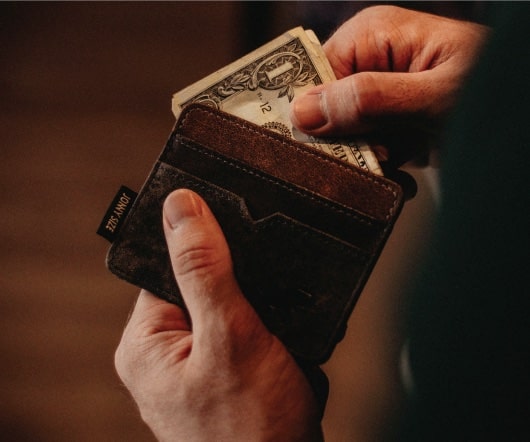
Supply Chain and Logistics Predictions for 2016
DECEMBER 16, 2015
Well, my big audacious prediction for 2015 did not come true. But some of my other predictions did hit the mark or came close. Making supply chain and logistics predictions is like throwing darts at a moving target. When making predictions , it’s easy to look at recent trends and simply project them forward.
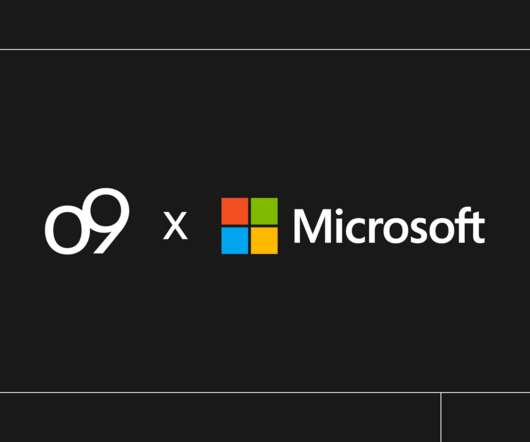
o9 Solutions enables global enterprises to transform disparate data into market knowledge with Microsoft Azure Synapse Analytics
o9 Solutions
JUNE 27, 2021
Microsoft Data, Analytics , and AI Partner Case Study Program. The o9 solution integrates multiple technology innovations into one platform, including graph-based enterprise modelling, big data analytics , advanced algorithms for scenario planning, collaborative portals, easy-to-use interfaces, and cloud-based delivery.
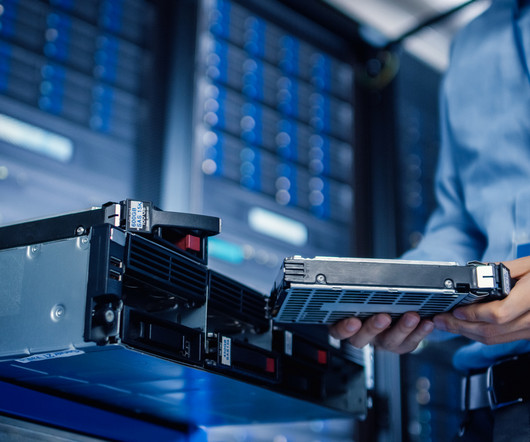
Electronics Kitting and Assembly with 3PL Solutions
ModusLink Corporation
APRIL 22, 2024
Continuous improvement initiatives driven by data analytics and feedback mechanisms. Predictive analytics to anticipate supply chain disruptions and mitigate risks. Case Studies and Success Stories: Real-world examples showcasing the transformative impact of global 3PL solutions, including ModusLink.
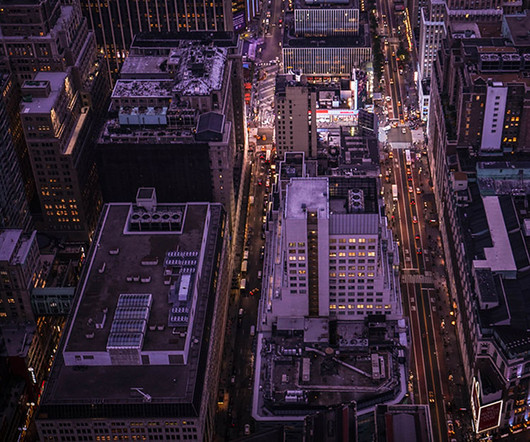
Exiger is thrilled to be a Gartner Supply Chain Symposium | Xpo™ 2024 sponsor.
Exiger Perspectives
APRIL 26, 2024
Save the Day With Exiger Empowering customers with Supply Chain AI Article 1Exiger Ending Forced Labor Case Studies Technology Platform Proactive Intelligence One platform. 1Exiger leverages advanced AI and real-time analytics to provide end-to-end visibility. End-to-end visibility into your entire supply chain and risk.
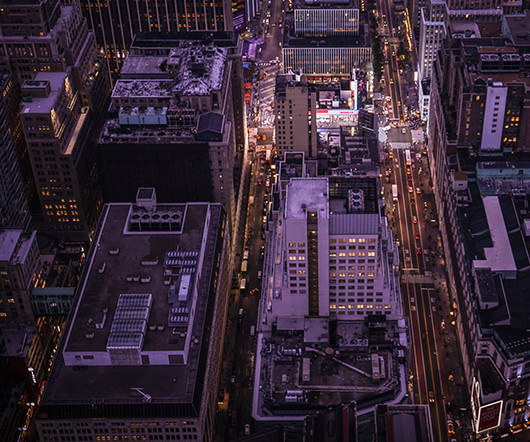
MAY 28, 2024
This session explores compelling narratives with dynamic case studies and real-world examples, illuminating forced labor in even sophisticated supply chains and surfacing the innovative solutions that are empowering organizations to uphold ethical standards. Access platform preview Seamless.
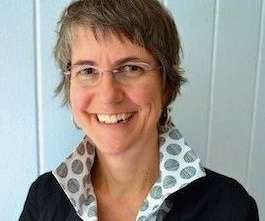
Can Improving Forecast Accuracy Address Our Demand Planning Woes?
AUGUST 4, 2022
In times that continue to defy our ability to predict them, the words of famous statistician George Box have never been more right: “All models are wrong, but some are useful.” If “the forecast is always wrong,” is improving forecast accuracy even the solution to our demand planning woes? So what can we do to make models more useful?
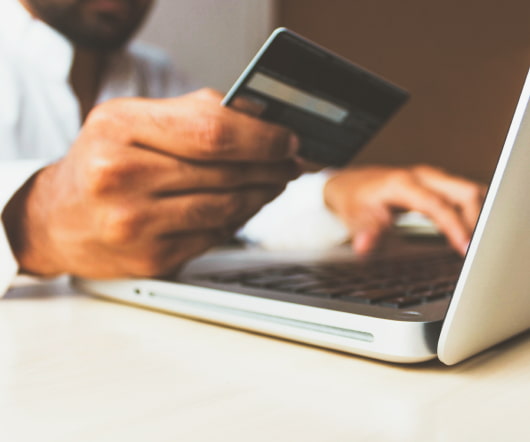
Sorry, I’m Not Sure: Supply Chain and Logistics Predictions for 2018
DECEMBER 20, 2017
I will be wrong again,” I stated last year before making my supply chain and logistics predictions for 2017 , and I was right. But I was right with some of my other predictions , especially my prediction that we would see a rise in cyber attacks in 2017, with supply chain and logistics operations becoming new targets.
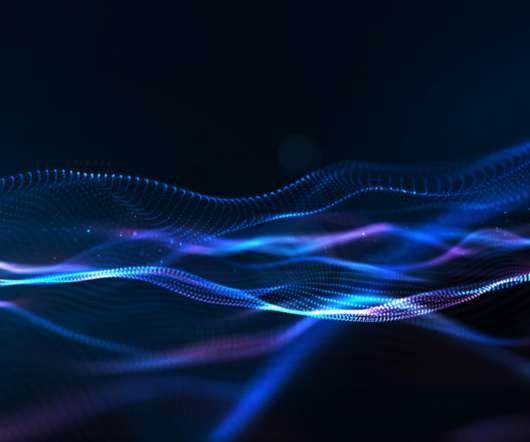
Digital Transformation Journey in Supply Chain Planning
MARCH 4, 2021
The First Step: Bring all the data together and ensure analytics and planning can happen on the same platform. . You can start with daily/operational decisions and work your way to tactical and strategic decisions to evaluate opportunities for integrating the data sources into your planning and analytics platform. . and Europe.
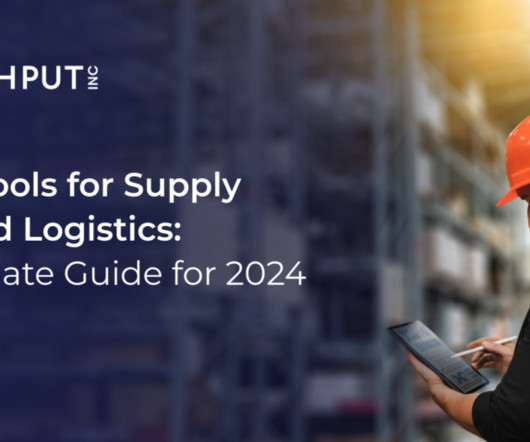
Best AI Tools for Supply Chain and Logistics: The Ultimate Guide for 2024
APRIL 30, 2024
ThroughPut AI: For Making Intelligent Decisions Across the Supply Chain Watch On-demand Demo ThroughPut AI has been recognized as a Leading Vendor in the prestigious 2023 Gartner Market Guide for Analytics and Decision Intelligence Platforms in Supply Chain. The platform primarily helps predict demand and improve customer experience.
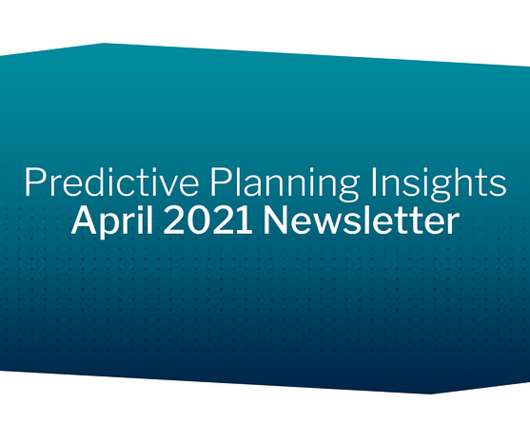
Supply Chain Simulation: What You Need to Know
Vanguard Software
APRIL 16, 2021
While the transformational power of AI is evident, too much faith is put into supply chain simulation platforms that are neither accurate nor predictive . This last factor, also known as predictive analytics , is the basis for successful supply chain simulation software. Scenario Planning During COVID-19.
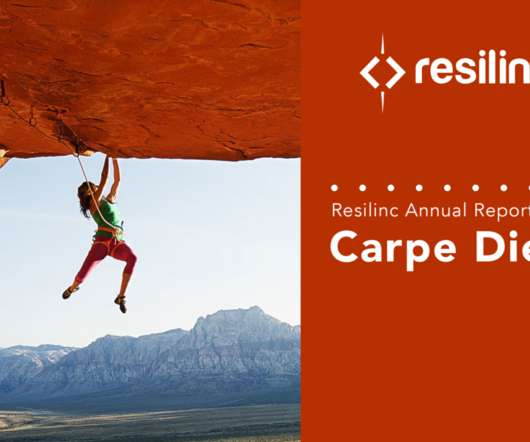
Download Now: Resilinc 2020 Annual Report
APRIL 22, 2021
Customer Case Studies using Mapping and Predictive Analytics . A Q&A with Bindiya Vakil on navigating the early days of COVID-19. Expert Perspective: Trade Agreements, Geopolitics, and Reshoring . Commentary: Why Supplier Collaboration is a Win-Win Strategy. Download Report .
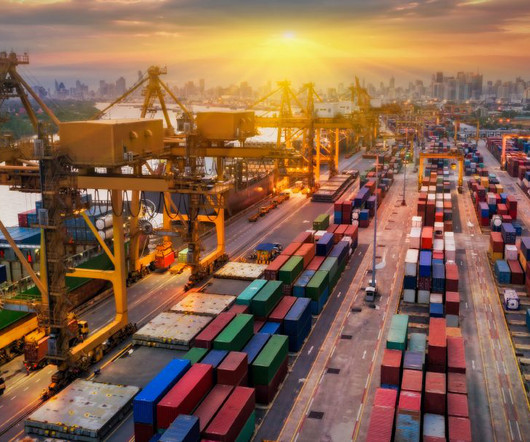
Supply Chain Forecasting with AI
It has the power to analyze vast datasets, predict future trends with remarkable accuracy, and adapt to changes in real time, offering businesses a competitive edge. Supply chain forecasting predicts future demand for products and services, inventory needs, and production planning based on historical data, market analysis, and current trends.
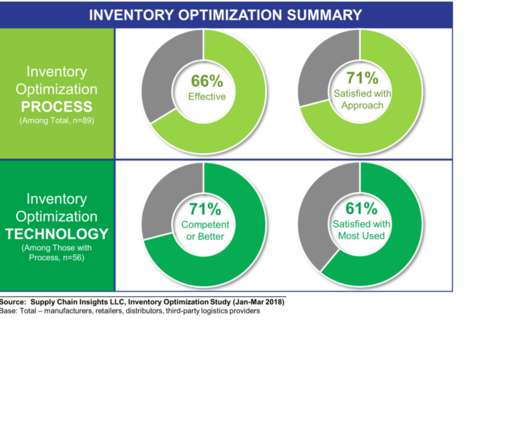
Strong Supply Chains Required For an Economic Rebound: Six Steps To Take
APRIL 15, 2020
No doubt about it, we are characters in a supply chain case study searching to define a new normal. Today, we find ourselves in the middle of a risk management case study . Another is looking at hospital bed utilization to predict market baskets. Don’t expect demand to be predictable . Bottom line?
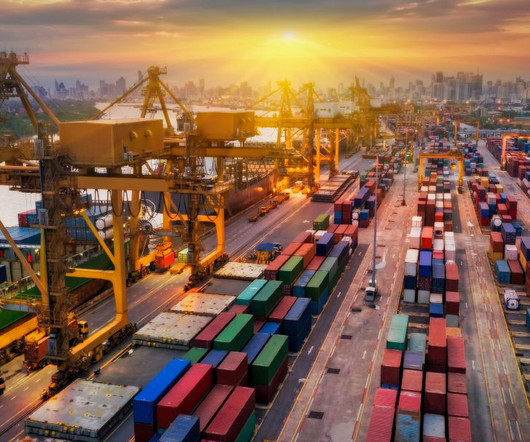
APRIL 15, 2024
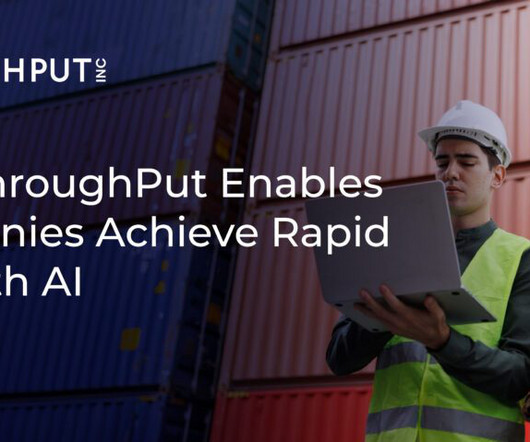
ROI in Supply Chain: Achieving Rapid Value with ThroughPut AI in Just 90 Days
MAY 22, 2024
With time running short, businesses need to build a clear business case for AI with a concrete plan of action that rapidly – and incrementally – achieves ROI. ThroughPut AI, a Gartner-recognized Supply Chain Decision Intelligence and Analytics Platform, does just that. Global Cement Manufacturer Achieves $1.5M
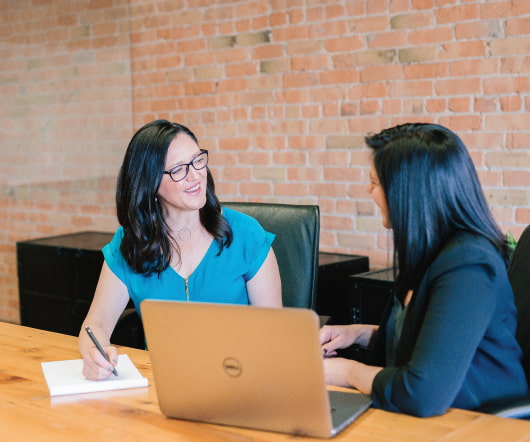
AI and All That – Ivalua Now Looks to the Future
APRIL 12, 2019
Anyway, there were also a couple of very good customer cases studies , but we’ll save those for a future discussion and focus on AI here. More support in terms of predictive analytics is the dream. (Now there’s an interesting question – if AI had a flavour, what exactly would it be? Fresh mint, maybe?
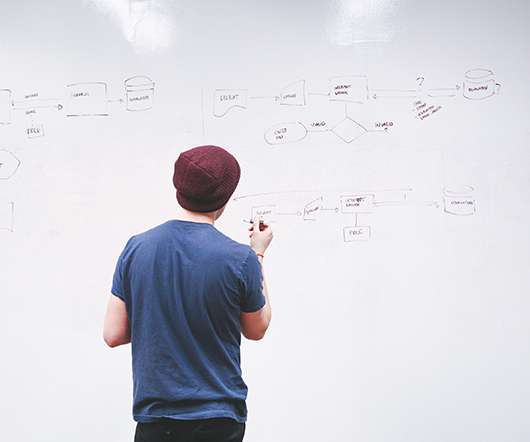
Trade promotion forecasting: the present and the near future
JANUARY 30, 2018
The next step is to accurately predict the uplift from baseline expected to be generated by the promotion so that supply chains can optimize inventory to properly support the promotion. As you move into the higher stages of maturity, you increasingly rely on advanced analytics and machine learning for demand shaping and promotion forecasting.
Stay Connected
Join 136,000+ Insiders by signing up for our newsletter
- Add a Source
- Add a Resource
- Fri. Aug 09
- Thu. Aug 08
- Wed. Aug 07
- Tue. Aug 06
- Jul 27 - Aug 02
- Warehousing
- Procurement
- Transportation
- Supply Chain
- More Topics

Input your email to sign up, or if you already have an account, log in here!
Enter your email address to reset your password. a temporary password will be e‑mailed to you., be in the know on.
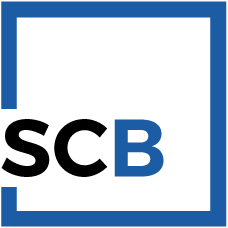
Supply Chain Brief
Expert insights. Personalized for you.
We organize all of the trending information in your field so you don't have to. Join 136,000+ users and stay up to date on the latest articles your peers are reading.

Get the good stuff
Subscribe to the following Supply Chain Brief newsletters:
You must accept the Privacy Policy and Terms & Conditions to proceed.

You know about us, now we want to get to know you!
Check your mail, we've sent an email to . please verify that you have received the email..
We have resent the email to
Let's personalize your content
Use social media to find articles.
We can use your profile and the content you share to understand your interests and provide content that is just for you.
Turn this off at any time. Your social media activity always remains private.
Let's get even more personalized
Choose topics that interest you., so, what do you do.
Are you sure you want to cancel your subscriptions?
Cancel my subscriptions
Don't cancel my subscriptions
Changing Country?
Accept terms & conditions.
It looks like you are changing your country/region of residence. In order to receive our emails, you must expressly agree. You can unsubscribe at any time by clicking the unsubscribe link at the bottom of our emails.
You appear to have previously removed your acceptance of the Terms & Conditions.

We noticed that you changed your country/region of residence; congratulations! In order to make this change, you must accept the Aggregage Terms and Conditions and Privacy Policy. Once you've accepted, then you will be able to choose which emails to receive from each site .
You must choose one option
Please choose which emails to receive from each site .
- Update All Sites
- Update Each Site
Please verify your previous choices for all sites
Sites have been updated - click Submit All Changes below to save your changes.
We recognize your account from another site in our network , please click 'Send Email' below to continue with verifying your account and setting a password.
You must accept the Privacy Policy and Terms & Conditions to proceed.
This is not me
Information
- Author Services
Initiatives
You are accessing a machine-readable page. In order to be human-readable, please install an RSS reader.
All articles published by MDPI are made immediately available worldwide under an open access license. No special permission is required to reuse all or part of the article published by MDPI, including figures and tables. For articles published under an open access Creative Common CC BY license, any part of the article may be reused without permission provided that the original article is clearly cited. For more information, please refer to https://www.mdpi.com/openaccess .
Feature papers represent the most advanced research with significant potential for high impact in the field. A Feature Paper should be a substantial original Article that involves several techniques or approaches, provides an outlook for future research directions and describes possible research applications.
Feature papers are submitted upon individual invitation or recommendation by the scientific editors and must receive positive feedback from the reviewers.
Editor’s Choice articles are based on recommendations by the scientific editors of MDPI journals from around the world. Editors select a small number of articles recently published in the journal that they believe will be particularly interesting to readers, or important in the respective research area. The aim is to provide a snapshot of some of the most exciting work published in the various research areas of the journal.
Original Submission Date Received: .
- Active Journals
- Find a Journal
- Proceedings Series
- For Authors
- For Reviewers
- For Editors
- For Librarians
- For Publishers
- For Societies
- For Conference Organizers
- Open Access Policy
- Institutional Open Access Program
- Special Issues Guidelines
- Editorial Process
- Research and Publication Ethics
- Article Processing Charges
- Testimonials
- Preprints.org
- SciProfiles
- Encyclopedia
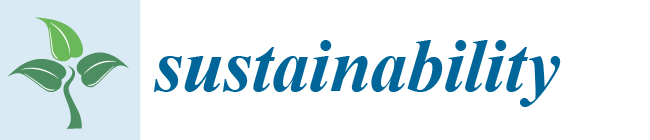
Article Menu
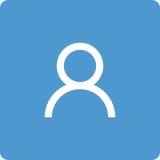
- Subscribe SciFeed
- Recommended Articles
- Google Scholar
- on Google Scholar
- Table of Contents
Find support for a specific problem in the support section of our website.
Please let us know what you think of our products and services.
Visit our dedicated information section to learn more about MDPI.
JSmol Viewer
Enhancing sustainable supply chain management through digital transformation: a comparative case study analysis.
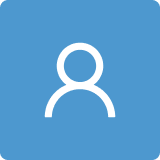
1. Introduction
2. theoretical background, 2.1. sustainable development and supply chain management, 2.2. digital transformation.
- Data collection and monitoring [ 40 ].
- Supplier assessment and compliance [ 41 ].
- Inventory and demand management [ 42 ].
- Transport optimization [ 43 ].
- Waste reduction [ 44 ].
- Carbon footprint monitoring [ 45 ].
- Product traceability [ 15 ].
- Stakeholder communication [ 15 ].
- Decision-making [ 46 ].
- Continuous improvement and adaptation [ 47 ].
2.3. Supply Chain Management Resources and Capabilities
2.4. business performance, sustainable impact, and business excellence.
- Enhanced reputation and image [ 52 ].
- Strategic supplier relationships [ 53 ].
- Improved efficiency [ 54 ].
- Optimized inventory management [ 55 ].
- Data-driven decision-making [ 56 ].
2.5. Sustainable Strategies
- The sustainable technology development strategy, which aims to develop IT capabilities that can contribute to sustainable development through innovation.
- The networking strategy, which aims to develop collaborative skills within the company and between all stakeholders.
- The sustainability strategy, which includes investments in feasibility-related technologies and aims to provide financial incentives for employees to propose and implement innovative ideas.
- The sustainable product marketing and promotion strategy, which aims to develop marketing and promote the benefits of sustainable products to customers in order to increase demand for products and services.
- The research and development strategy, which aims to develop research units within the company in order to improve products and processes.
- The sustainable skills and competencies development strategy, which aims to create an enabling environment for employees to develop green and sustainable skills.
2.6. Conceptual Framework
3. research method, 3.1. research design, 3.2. case selection, 3.3. data collection procedures, 3.4. data coding and analysis, 4. case studies’ description and findings, 4.1. case study—3pl company, 4.1.1. resources and capabilities.
- Efficient warehouse management through the “Mantis WMS”, which enabled the company to automate and effectively manage its processes, achieving accuracy and speed in the storage and preparation of orders.
- Automation, quick product identification, and information management through the use of “barcode” labels on each product and pallet and connection to wireless terminals, allowing operators to quickly access and alter information.
- Employee development through investment in staff training.
4.1.2. Sustainable Impact
4.1.3. business performance, excellence and sustainable strategies, 4.2. case study—retailer, 4.2.1. resources and capabilities.
- Driver information.
- Location at all times.
- Temperature in storage areas for the better and safer transport and monitoring of products.
4.2.2. Sustainable Impact
4.2.3. business performance, excellence and sustainable strategies, 4.3. case study—producer, 4.3.1. resources.
- Financial Supply Chain Management (FSCM);
- Logistics Execution (LE).
4.3.2. Capabilities and Sustainable Impact
- Commitment to sustainability.
- Resilience in financial performance.
- Social contribution and community support.
- Digital transformation.
- Ethics and compliance training.
- Effective management of distribution centers.
4.3.3. Sustainable Strategies
- Research and development.
- Networking.
- Marketing and promotion.
5. Results and Discussion
- Increasing productivity.
- Reducing operating costs.
- Efficient management of supply chain processes.
- Automation.
- Knowledge transfer and training.
- Customer satisfaction and loyalty.
5.1. Theoretical Implications
5.2. managerial implications, 6. conclusions, author contributions, institutional review board statement, informed consent statement, data availability statement, conflicts of interest.
- Verhoef, P.C.; Broekhuizen, T.; Bart, Y.; Bhattacharya, A.; Dong, J.Q.; Fabian, N.; Haenlein, M. Digital Transformation: A Multidisciplinary Reflection and Research Agenda. J. Bus. Res. 2021 , 122 , 889–901. [ Google Scholar ] [ CrossRef ]
- Gao, P.; Wu, W.; Yang, Y. Discovering Themes and Trends in Digital Transformation and Innovation Research. J. Theor. Appl. Electron. Commer. Res. 2022 , 17 , 1162–1184. [ Google Scholar ] [ CrossRef ]
- Schmidt, C.G.; Wagner, S.M. Blockchain and Supply Chain Relations: A Transaction Cost Theory Perspective. J. Purch. Supply Manag. 2019 , 25 , 100552. [ Google Scholar ] [ CrossRef ]
- Farahani, P.; Meier, C.; Wilke, J. Digital Supply Chain Management Agenda for the Automotive Supplier Industry. In Shaping the Digital Enterprise ; Oswald, G., Kleinemeier, M., Eds.; Springer International Publishing: Cham, Switzerland, 2017; pp. 157–172. ISBN 978-3-319-40966-5. [ Google Scholar ]
- Duong Thi Binh, A.; Akbari, M.; Le Thi Cam, H.; Nguyen Canh, L.; Truong Quang, H. Forging Pathways to Circular Economy Excellence: Integrating Industry 4.0 with Quality Management. Sustainability 2024 , 16 , 3053. [ Google Scholar ] [ CrossRef ]
- Zhang, S.; Sun, L.; Sun, Q.; Dong, H. Impact of Novel Information Technology on IT Alignment and Sustainable Supply Chain Performance: Evidence from Chinese Manufacturing Industry. J. Bus. Ind. Mark. 2022 , 37 , 461–473. [ Google Scholar ] [ CrossRef ]
- Ahmed, Z.; Ahmad, M.; Alvarado, R.; Sinha, A.; Shah, M.I.; Abbas, S. Towards Environmental Sustainability: Do Financial Risk and External Conflicts Matter? J. Clean. Prod. 2022 , 371 , 133721. [ Google Scholar ] [ CrossRef ]
- Nozari, H.; Fallah, M.; Szmelter-Jarosz, A. A Conceptual Framework of Green Smart IoT-Based Supply Chain Management. Int. J. Res. Ind. Eng. 2021 , 10 , 22–34. [ Google Scholar ]
- Birkel, H.; Müller, J.M. Potentials of Industry 4.0 for Supply Chain Management within the Triple Bottom Line of Sustainability–A Systematic Literature Review. J. Clean. Prod. 2021 , 289 , 125612. [ Google Scholar ] [ CrossRef ]
- Mani, V.; Gunasekaran, A.; Papadopoulos, T.; Hazen, B.; Dubey, R. Supply Chain Social Sustainability for Developing Nations: Evidence from India. Resour. Conserv. Recycl. 2016 , 111 , 42–52. [ Google Scholar ] [ CrossRef ]
- Kosasih, W.; Pujawan, I.N.; Karningsih, P.D. Integrated Lean-Green Practices and Supply Chain Sustainability for Manufacturing SMEs: A Systematic Literature Review and Research Agenda. Sustainability 2023 , 15 , 12192. [ Google Scholar ] [ CrossRef ]
- Van Zanten, J.A.; Van Tulder, R. Multinational Enterprises and the Sustainable Development Goals: An Institutional Approach to Corporate Engagement. J. Int. Bus. Policy 2018 , 1 , 208–233. [ Google Scholar ] [ CrossRef ]
- Akbari, M.; Kok, S.; Hopkins, J.; Frederico, G.; Nguyen, H.; Alonso, A. The Changing Landscape of Digital Transformation in Supply Chains: Impacts of Industry 4.0 in Vietnam. Int. J. Logist. Manag. 2023 , 35 , 1040–1072. [ Google Scholar ] [ CrossRef ]
- Akbari, M. Revolutionizing Supply Chain and Circular Economy with Edge Computing: Systematic Review, Research Themes and Future Directions. Manag. Decis. 2023 . ahead-of-print . [ Google Scholar ] [ CrossRef ]
- Stroumpoulis, A.; Kopanaki, E. Theoretical Perspectives on Sustainable Supply Chain Management and Digital Transformation: A Literature Review and a Conceptual Framework. Sustainability 2022 , 14 , 4862. [ Google Scholar ] [ CrossRef ]
- Kilibarda, M.; Andrejić, M.; Popović, V. Research in Logistics Service Quality: A Systematic Literature Review. Transport 2020 , 35 , 224–235. [ Google Scholar ] [ CrossRef ]
- Agatz, N.A.H.; Fleischmann, M.; van Nunen, J.A.E.E. E-Fulfillment and Multi-Channel Distribution—A Review. Eur. J. Oper. Res. 2008 , 187 , 339–356. [ Google Scholar ] [ CrossRef ]
- Chichilnisky, G. What Is Sustainable Development? Land Econ. 1997 , 467–491. [ Google Scholar ] [ CrossRef ]
- Emas, R. The Concept of Sustainable Development: Definition and Defining Principles. Brief GSDR 2015 , 2015 , 10–13140. [ Google Scholar ]
- Robert, K.W.; Parris, T.M.; Leiserowitz, A.A. What Is Sustainable Development? Goals, Indicators, Values, and Practice. Environ. Sci. Policy Sustain. Dev. 2005 , 47 , 8–21. [ Google Scholar ] [ CrossRef ]
- Purvis, B.; Mao, Y.; Robinson, D. Three Pillars of Sustainability: In Search of Conceptual Origins. Sustain. Sci. 2019 , 14 , 681–695. [ Google Scholar ] [ CrossRef ]
- Hansmann, R.; Mieg, H.A.; Frischknecht, P. Principal Sustainability Components: Empirical Analysis of Synergies between the Three Pillars of Sustainability. Int. J. Sustain. Dev. World Ecol. 2012 , 19 , 451–459. [ Google Scholar ] [ CrossRef ]
- Weiland, S.; Hickmann, T.; Lederer, M.; Marquardt, J.; Schwindenhammer, S. The 2030 Agenda for Sustainable Development: Transformative Change through the Sustainable Development Goals? Politics Gov. 2021 , 9 , 90–95. [ Google Scholar ] [ CrossRef ]
- Miles, M.P.; Munilla, L.S. The Potential Impact of Social Accountability Certification on Marketing: A Short Note. J. Bus. Ethics 2004 , 50 , 1–11. [ Google Scholar ] [ CrossRef ]
- Mani, V.; Gunasekaran, A.; Delgado, C. Supply Chain Social Sustainability: Standard Adoption Practices in Portuguese Manufacturing Firms. Int. J. Prod. Econ. 2018 , 198 , 149–164. [ Google Scholar ] [ CrossRef ]
- Bhuniya, S.; Pareek, S.; Sarkar, B. A Supply Chain Model with Service Level Constraints and Strategies under Uncertainty. Alex. Eng. J. 2021 , 60 , 6035–6052. [ Google Scholar ] [ CrossRef ]
- Seuring, S.; Müller, M. From a Literature Review to a Conceptual Framework for Sustainable Supply Chain Management. J. Clean. Prod. 2008 , 16 , 1699–1710. [ Google Scholar ] [ CrossRef ]
- Kumar, A.; Shrivastav, S.K.; Shrivastava, A.K.; Panigrahi, R.R.; Mardani, A.; Cavallaro, F. Sustainable Supply Chain Management, Performance Measurement, and Management: A Review. Sustainability 2023 , 15 , 5290. [ Google Scholar ] [ CrossRef ]
- Al-Emran, M.; Griffy-Brown, C. The Role of Technology Adoption in Sustainable Development: Overview, Opportunities, Challenges, and Future Research Agendas. Technol. Soc. 2023 , 73 , 102240. [ Google Scholar ] [ CrossRef ]
- Andriole, S.J.; Cox, T.; Khin, K.M. The Innovator’s Imperative: Rapid Technology Adoption for Digital Transformation ; Auerbach Publications: Boca Raton, FL, USA, 2017. [ Google Scholar ]
- Yin, W. Identifying the Pathways through Digital Transformation to Achieve Supply Chain Resilience: An fsQCA Approach. Environ. Sci. Pollut. Res. 2022 , 30 , 10867–10879. [ Google Scholar ] [ CrossRef ]
- Fitzgerald, M.; Kruschwitz, N.; Bonnet, D.; Welch, M. Embracing Digital Technology: A New Strategic Imperative. MIT Sloan Manag. Rev. 2014 , 55 , 1. [ Google Scholar ]
- de Camargo Fiorini, P.; Jabbour, C.J.C. Information Systems and Sustainable Supply Chain Management towards a More Sustainable Society: Where We Are and Where We Are Going. Int. J. Inf. Manag. 2017 , 37 , 241–249. [ Google Scholar ] [ CrossRef ]
- Wang, Y.; Chen, Y.; Benitez-Amado, J. How Information Technology Influences Environmental Performance: Empirical Evidence from China. Int. J. Inf. Manag. 2015 , 35 , 160–170. [ Google Scholar ] [ CrossRef ]
- Kagermann, H.; Helbig, J.; Hellinger, A.; Wahlster, W. Recommendations for Implementing the Strategic Initiative INDUSTRIE 4.0: Securing the Future of German Manufacturing Industry ; Final Report of the Industrie 4.0 Working Group; acatech—National Academy of Science and Engineering: Munich, Germany, 2013; Available online: https://www.din.de/resource/blob/76902/e8cac883f42bf28536e7e8165993f1fd/recommendations-for-implementing-industry-4-0-data.pdf (accessed on 6 March 2024).
- Moeuf, A.; Pellerin, R.; Lamouri, S.; Tamayo-Giraldo, S.; Barbaray, R. The Industrial Management of SMEs in the Era of Industry 4.0. Int. J. Prod. Res. 2018 , 56 , 1118–1136. [ Google Scholar ] [ CrossRef ]
- Bigliardi, B.; Filippelli, S.; Petroni, A.; Tagliente, L. The Digitalization of Supply Chain: A Review. Procedia Comput. Sci. 2022 , 200 , 1806–1815. [ Google Scholar ] [ CrossRef ]
- Schallmo, D.; Williams, C.A.; Boardman, L. Digital transformation of business models—Best practice, enablers, and roadmap. Int. J. Innov. Mgt. 2017 , 21 , 1740014. [ Google Scholar ] [ CrossRef ]
- Shee, H.; Miah, S.J.; Fairfield, L.; Pujawan, N. The Impact of Cloud-Enabled Process Integration on Supply Chain Performance and Firm Sustainability: The Moderating Role of Top Management. Supply Chain Manag. Int. J. 2018 , 23 , 500–517. [ Google Scholar ] [ CrossRef ]
- Jahani, H.; Jain, R.; Ivanov, D. Data Science and Big Data Analytics: A Systematic Review of Methodologies Used in the Supply Chain and Logistics Research. Ann. Oper. Res. 2023 . [ Google Scholar ] [ CrossRef ]
- Sharma, M.; Joshi, S. Digital Supplier Selection Reinforcing Supply Chain Quality Management Systems to Enhance Firm’s Performance. TQM J. 2020 , 35 , 102–130. [ Google Scholar ] [ CrossRef ]
- Verma, A.; Tripathy, S.; Singhal, D. The Significance of Warehouse Management in Supply Chain: An ISM Approach. Decis. Mak. Appl. Manag. Eng. 2023 , 6 , 92–110. [ Google Scholar ] [ CrossRef ]
- Shamsuddoha, M.; Kashem, M.; Nasir, T. Smart Transportation Logistics: Achieving Supply Chain Efficiency with Green Initiatives. In Data Analytics for Supply Chain Networks ; Springer International Publishing: Berlin/Heidelberg, Germany, 2023; pp. 243–258. ISBN 978-3-031-29822-6. [ Google Scholar ]
- Debnath, A.; Sarkar, B. Effect of Circular Economy for Waste Nullification under a Sustainable Supply Chain Management. J. Clean. Prod. 2023 , 385 , 135477. [ Google Scholar ] [ CrossRef ]
- Wang, M.; Wang, B.; Abareshi, A. Blockchain Technology and Its Role in Enhancing Supply Chain Integration Capability and Reducing Carbon Emission: A Conceptual Framework. Sustainability 2020 , 12 , 10550. [ Google Scholar ] [ CrossRef ]
- Kabadurmus, O.; Kayikci, Y.; Demir, S.; Koc, B. A Data-Driven Decision Support System with Smart Packaging in Grocery Store Supply Chains during Outbreaks. Socio-Econ. Plan. Sci. 2023 , 85 , 101417. [ Google Scholar ] [ CrossRef ]
- Aldhaheri, R.T.; Ahmad, S.Z. Factors Affecting Organisations’ Supply Chain Agility and Competitive Capability. Bus. Process Manag. J. 2023 , 29 , 505–527. [ Google Scholar ] [ CrossRef ]
- Chan, H.-L.; Shen, B.; Cai, Y. Quick Response Strategy with Cleaner Technology in a Supply Chain: Coordination and Win-Win Situation Analysis. Int. J. Prod. Res. 2018 , 56 , 3397–3408. [ Google Scholar ] [ CrossRef ]
- Dao, V.; Langella, I.; Carbo, J. From Green to Sustainability: Information Technology and an Integrated Sustainability Framework. J. Strateg. Inf. Syst. 2011 , 20 , 63–79. [ Google Scholar ] [ CrossRef ]
- Dyer, J.H.; Singh, H. The Relational View: Cooperative Strategy and Sources of Interorganizational Competitive Advantage. Acad. Manag. Rev. 1998 , 23 , 660–679. [ Google Scholar ] [ CrossRef ]
- Hart, S.L.; Dowell, G. Invited Editorial: A Natural-Resource-Based View of the Firm: Fifteen Years After. J. Manag. 2011 , 37 , 1464–1479. [ Google Scholar ] [ CrossRef ]
- Mahmood, A.; Bashir, J. How Does Corporate Social Responsibility Transform Brand Reputation into Brand Equity? Economic and Noneconomic Perspectives of CSR. Int. J. Eng. Bus. Manag. 2020 , 12 , 1847979020927547. [ Google Scholar ] [ CrossRef ]
- Alshurideh, M.; Kurdi, B.; Alzoubi, H.; Obeidat, B.; Hamadneh, S.; Ahmad, A. The Influence of Supply Chain Partners’ Integrations on Organizational Performance: The Moderating Role of Trust. Uncertain Supply Chain Manag. 2022 , 10 , 1191–1202. [ Google Scholar ] [ CrossRef ]
- Qrunfleh, S.; Tarafdar, M. Supply Chain Information Systems Strategy: Impacts on Supply Chain Performance and Firm Performance. Int. J. Prod. Econ. 2014 , 147 , 340–350. [ Google Scholar ] [ CrossRef ]
- Saragih, J.; Tarigan, A.; Silalahi, E.F.; Wardati, J.; Pratama, I. Supply Chain Operational Capability and Supply Chain Operational Performance: Does the Supply Chain Management and Supply Chain Integration Matters? Int. J. Supply Chain Manag. 2020 , 9 , 1222–1229. [ Google Scholar ] [ CrossRef ]
- Bhatti, S.H.; Hussain, W.M.H.W.; Khan, J.; Sultan, S.; Ferraris, A. Exploring Data-Driven Innovation: What’s Missing in the Relationship between Big Data Analytics Capabilities and Supply Chain Innovation? Ann. Oper. Res. 2024 , 333 , 799–824. [ Google Scholar ] [ CrossRef ]
- El Khatib, M.; Al Falasi, A. Effects of Artificial Intelligence on Decision Making in Project Management. Am. J. Ind. Bus. Manag. 2021 , 11 , 251–260. [ Google Scholar ] [ CrossRef ]
- Madakam, S.; Holmukhe, R.M.; Jaiswal, D.K. The Future Digital Work Force: Robotic Process Automation (RPA). JISTEM-J. Inf. Syst. Technol. Manag. 2019 , 16 , e201916001. [ Google Scholar ] [ CrossRef ]
- Al-Htaybat, K.; von Alberti-Alhtaybat, L. Big Data and Corporate Reporting: Impacts and Paradoxes. Account. Audit. Account. J. 2017 , 30 , 850–873. [ Google Scholar ] [ CrossRef ]
- Mahapatra, S.K.; Narasimhan, R.; Barbieri, P. Strategic Interdependence, Governance Effectiveness and Supplier Performance: A Dyadic Case Study Investigation and Theory Development. J. Oper. Manag. 2010 , 28 , 537–552. [ Google Scholar ] [ CrossRef ]
- Kazancoglu, I.; Sagnak, M.; Kumar Mangla, S.; Kazancoglu, Y. Circular Economy and the Policy: A Framework for Improving the Corporate Environmental Management in Supply Chains. Bus. Strategy Environ. 2021 , 30 , 590–608. [ Google Scholar ] [ CrossRef ]
- Hart, S.L. A Natural-Resource-Based View of the Firm. Acad. Manag. Rev. 1995 , 20 , 986–1014. [ Google Scholar ] [ CrossRef ]
- Dev, N.K.; Shankar, R.; Qaiser, F.H. Industry 4.0 and Circular Economy: Operational Excellence for Sustainable Reverse Supply Chain Performance. Resour. Conserv. Recycl. 2020 , 153 , 104583. [ Google Scholar ] [ CrossRef ]
- Biswas, D.; Jalali, H.; Ansaripoor, A.H.; De Giovanni, P. Traceability vs. Sustainability in Supply Chains: The Implications of Blockchain. Eur. J. Oper. Res. 2023 , 305 , 128–147. [ Google Scholar ] [ CrossRef ]
- Campbell, D.J. Legitimacy Theory or Managerial Reality Construction? Corporate Social Disclosure in Marks and Spencer Plc Corporate Reports, 1969–1997. Account. Forum 2000 , 24 , 80–100. [ Google Scholar ] [ CrossRef ]
- Deegan, C.M. Legitimacy Theory: Despite Its Enduring Popularity and Contribution, Time Is Right for a Necessary Makeover. Account. Audit. Account. J. 2019 , 32 , 2307–2329. [ Google Scholar ] [ CrossRef ]
- Alsaedi, B.S.; Alamri, O.A.; Jayaswal, M.K.; Mittal, M. A Sustainable Green Supply Chain Model with Carbon Emissions for Defective Items under Learning in a Fuzzy Environment. Mathematics 2023 , 11 , 301. [ Google Scholar ] [ CrossRef ]
- Myovella, G.; Karacuka, M.; Haucap, J. Digitalization and Economic Growth: A Comparative Analysis of Sub-Saharan Africa and OECD Economies. Telecommun. Policy 2020 , 44 , 101856. [ Google Scholar ] [ CrossRef ]
- Sezhiyan, D.M.; Page, T.; Iskanius, P. The Impact of Supply Effort Management, Logistics Capability, and Supply Chain Management Strategies on Firm Performance. Int. J. Electron. Transp. 2011 , 1 , 26–44. [ Google Scholar ] [ CrossRef ]
- Degraeve, Z.; Labro, E.; Roodhooft, F. An Evaluation of Vendor Selection Models from a Total Cost of Ownership Perspective. Eur. J. Oper. Res. 2000 , 125 , 34–58. [ Google Scholar ] [ CrossRef ]
- Gupta, H.; Kusi-Sarpong, S.; Rezaei, J. Barriers and Overcoming Strategies to Supply Chain Sustainability Innovation. Resour. Conserv. Recycl. 2020 , 161 , 104819. [ Google Scholar ] [ CrossRef ]
- Rigó, L.; Fabianová, J.; Lokšík, M.; Mikušová, N. Utilising Digital Twins to Bolster the Sustainability of Logistics Processes in Industry 4.0. Sustainability 2024 , 16 , 2575. [ Google Scholar ] [ CrossRef ]
- Walsham, G.; Han, C.K. Information Systems Strategy Formation and Implementation: The Case of a Central Government Agency. Account. Manag. Inf. Technol. 1993 , 3 , 191–209. [ Google Scholar ] [ CrossRef ]
- Kopanaki, E. Examining Organisational Flexibility in an Interorganisational Context: A Case Study of a Grocery Retail Supply Chain ; London School of Economics and Political Science: London, UK, 2008. [ Google Scholar ]
- Eisenhardt, K.M. Building Theories from Case-Study Research. Acad. Manag. Rev. 1989 , 14 , 532–550. [ Google Scholar ] [ CrossRef ]
- Cavaye, A.L.M. Case Study Research: A Multi-faceted Research Approach for IS. Inf. Syst. J. 1996 , 6 , 227–242. [ Google Scholar ] [ CrossRef ]
- Saunders, M.; Lewis, P.; Thornhill, A. Research Methods for Business Students , 8th ed.; Pearson Education: New York, NY, USA, 2019. [ Google Scholar ]
- Jick, T.D. Mixing Qualitative and Quantitative Methods: Triangulation in Action. Adm. Sci. Q. 1979 , 24 , 602–611. [ Google Scholar ] [ CrossRef ]
- Shih, F. Triangulation in Nursing Research: Issues of Conceptual Clarity and Purpose. J. Adv. Nurs. 1998 , 28 , 631–641. [ Google Scholar ] [ CrossRef ]
- Magaldi, D.; Berler, M. Semi-Structured Interviews. In Encyclopedia of Personality and Individual Differences ; Zeigler-Hill, V., Shackelford, T.K., Eds.; Springer International Publishing: Cham, Switzerland, 2020; pp. 4825–4830. ISBN 978-3-319-24610-9. [ Google Scholar ]
- Cohen, L.; Manion, L.; Morrison, K. Research Methods in Education ; Routledge: London, UK, 2002. [ Google Scholar ]
- Daymon, C.; Holloway, I. Qualitative Research Methods in Public Relations and Marketing Communications ; Routledge: London, UK, 2010. [ Google Scholar ]
- Oakley, A. Gender, Methodology and People’s Ways of Knowing: Some Problems with Feminism and the Paradigm Debate in Social Science. Sociology 1998 , 32 , 707–731. [ Google Scholar ] [ CrossRef ]
- Miles, M.B.; Huberman, A.M. Qualitative Data Analysis: An Expanded Sourcebook ; Sage: Los Angeles, CA, USA, 1994. [ Google Scholar ]
- Braun, V.; Clarke, V. Using Thematic Analysis in Psychology. Qual. Res. Psychol. 2006 , 3 , 77–101. [ Google Scholar ] [ CrossRef ]
- Campbell, D.T. III. “Degrees of Freedom” and the Case Study. Comp. Political Stud. 1975 , 8 , 178–193. [ Google Scholar ] [ CrossRef ]
- Baxter, P.; Jack, S. Qualitative Case Study Methodology: Study Design and Implementation for Novice Researchers. Qual. Rep. 2008 , 13 , 544–559. [ Google Scholar ] [ CrossRef ]
- Khadka, K.; Maharjan, S. Customer Satisfaction and Customer Loyalty: Case Trivsel Städtjänster (Trivsel Siivouspalvelut). Centria University of Applied Sciences: Kokkola, Finland, 2017. Available online: https://www.theseus.fi/bitstream/handle/10024/139650/khadka_kabu%20%20and%20%20maharjan_%20soniya.pdf (accessed on 6 March 2024).
- Ozkan, M.; Cek, K.; Eyupoglu, S.Z. Sustainable Development and Customer Satisfaction and Loyalty in North Cyprus: The Mediating Effect of Customer Identification. Sustainability 2022 , 14 , 5196. [ Google Scholar ] [ CrossRef ]
- Abdi, Y.; Li, X.; Càmara-Turull, X. Impact of Sustainability on Firm Value and Financial Performance in the Air Transport Industry. Sustainability 2020 , 12 , 9957. [ Google Scholar ] [ CrossRef ]
- Bafas, S.A.; Alexandropoulou, A.P.; Fousteris, A.E.; Didaskalou, E.A.; Georgakellos, D.A. Sustainable Development and Business Strategies: An Exploratory Study of Greek Businesses. Businesses 2023 , 3 , 441–459. [ Google Scholar ] [ CrossRef ]
- Ersoy, P.; Börühan, G.; Kumar Mangla, S.; Hormazabal, J.H.; Kazancoglu, Y.; Lafcı, Ç. Impact of Information Technology and Knowledge Sharing on Circular Food Supply Chains for Green Business Growth. Bus. Strategy Environ. 2022 , 31 , 1875–1904. [ Google Scholar ] [ CrossRef ]
- Kalyar, M.N.; Shafique, I.; Saleem, S.; Humayun, S. Role of Leadership for Blockchain-Driven Supply Chain Management. In Blockchain Driven Supply Chain Management ; Mubarik, M.S., Shahbaz, M., Eds.; Management for Professionals; Springer Nature: Singapore, 2023; pp. 87–100. ISBN 978-981-9906-98-7. [ Google Scholar ]
- Shee, H. Internet of Things: Applications and Challenges for Supply Chain Management. In The Palgrave Handbook of Supply Chain Management ; Sarkis, J., Ed.; Springer International Publishing: Cham, Switzerland, 2024; pp. 1197–1215. ISBN 978-3-031-19883-0. [ Google Scholar ]
- Schoenherr, T. Supply Chain Management Professionals’ Proficiency in Big Data Analytics: Antecedents and Impact on Performance. Transp. Res. Part E Logist. Transp. Rev. 2023 , 169 , 102972. [ Google Scholar ] [ CrossRef ]
- Thottoli, M.M.; Ahmed, E.R. Information Technology and E-Accounting: Some Determinants among SMEs. J. Money Bus. 2022 , 2 , 1–15. [ Google Scholar ] [ CrossRef ]
Click here to enlarge figure
Company Main Activities Size Region | 3PL Warehousing, Distribution, Consulting Small and Medium Attica, Greece | Retailer Warehousing, Distribution, Sales Large Attica, Greece | Producer Producing Construction Materials Large Evia, Greece |
---|---|---|---|
Industry Representation | It illustrates the logistical backbone of supply chains, crucial for understanding the operational changes enabled by digital technologies. | Interfacing directly with consumers, it offers insights into consumer behaviour and the implementation of sustainability practices in a retail setting. | Being a resource-intensive manufacturing sector entity, it was selected for its significant environmental impacts and potential for sustainability improvements through technology. |
Leadership in Innovation and Sustainability | It has been notable for its rapid adoption of innovative logistics technologies and sustainable growth practices since its recent inception. | It has implemented several initiatives emphasizing sustainability across its extensive network, effectively integrating digital platforms to enhance consumer engagement and sustainable consumption. | It is at the forefront of digitalizing production processes, which has substantially improved its resource efficiency and waste management. |
Commitment to Sustainability and Digital Transformation | Its use of technology has not only streamlined operations but also significantly reduced its environmental footprint. | Its initiatives, such as recycling programs and energy-efficient operations, are supported by digital tools that track and enhance their effectiveness. | It has invested in advanced digital systems to monitor and optimize resource use, reflecting a strong commitment to environmental sustainability. |
Geographical and Economic Context | All companies operate within Greece, providing a consistent economic and regulatory context that influences their approaches to sustainability and digitalization. This context is particularly relevant given the European Union’s strict regulations on both digital innovation and sustainability practices. |
Participant Position | Company | Gender | Age Group | Highest Academic Qualification | Expertise | Experience (Years) | Interview Date | Interview Duration (min) | Interview Mode |
---|---|---|---|---|---|---|---|---|---|
CEO | 3PL | Male | 40–45 | PhD | Supply Chain Management | 10–15 | 12/09/2022 | 45 | Face-to-face |
Warehouse Manager | 3PL | Male | 40–45 | Bachelor’s degree | Logistics | 10–15 | 12/09/2022 | 45 | Face-to-face |
Warehouse Manager | Retailer | Male | 40–45 | Bachelor’s degree | Warehouse Management | 10–15 | 09/05/2023 | 120 | Face-to-face |
IT Senior Manager | Retailer | Male | 55–60 | Master’s degree | Information Technology | 20–25 | 07/07/2023 | 60 | Face-to-face |
IT Junior Manager | Retailer | Female | 35–40 | Master’s degree | Information Technology | 5–10 | 07/07/2023 | 60 | Face-to-face |
Procurement Manager | Producer | Male | 45–50 | Master’s degree | Logistics | 15–20 | 02/10/2023 | 30 | Face-to-face |
IT Senior Manager | Producer | Male | 40–45 | Bachelor’s degree | Information Technology | 10–15 | 03/10/2023 | 45 | Video call |
Head of Sustainable Development Department | Producer | Male | 50–55 | Master’s degree | Sustainability | 20–25 | 10/10/2023 | 45 | Video call |
3PL | Retailer | Producer | ||||||||
---|---|---|---|---|---|---|---|---|---|---|
Themes | Codes | Interviews | Interview Confirmation Method | Interviews | Interview Confirmation Method | Interviews | Interviews Confirmation Method | |||
Two Key Officers | Review of Documents and Records | On-Site Observation | Three Key Officers | Review of Documents and Records | On-Site Observation | Three Key Officers | Review of Documents and Records | On-Site Observation | ||
Sustainable Policies | Environmental Pillar | |||||||||
Social Pillar | ||||||||||
Economic Pillar | ||||||||||
Organizational Context | IT & Digital Transformation | |||||||||
SCM Resources/Processes | ||||||||||
Capabilities | Capabilities | |||||||||
Sustainable Impact | Environmental | |||||||||
Social | ||||||||||
Economic Results | ||||||||||
Excellence | Sustainable Development | |||||||||
Digital Transformation | ||||||||||
Business Performance | ||||||||||
Sustainable Strategies | Sustainable Strategies |
Themes | Codes | Company | ||
---|---|---|---|---|
3PL | Retailer | Producer | ||
Sustainable Policies | Environmental Pillar | emissions | ||
Social Pillar | ||||
Economic Pillar | ||||
Organizational Context | IT and Digital Transformation | |||
SCM Resources/ Processes | ||||
Capabilities | Capabilities | |||
Sustainable Impact | Environmental | |||
Social | ||||
Economic Results | ||||
Excellence | Sustainable Development | |||
Digital Transformation | ||||
Business Performance | ||||
Sustainable Strategies | Sustainable Strategies |
The statements, opinions and data contained in all publications are solely those of the individual author(s) and contributor(s) and not of MDPI and/or the editor(s). MDPI and/or the editor(s) disclaim responsibility for any injury to people or property resulting from any ideas, methods, instructions or products referred to in the content. |
Share and Cite
Stroumpoulis, A.; Kopanaki, E.; Chountalas, P.T. Enhancing Sustainable Supply Chain Management through Digital Transformation: A Comparative Case Study Analysis. Sustainability 2024 , 16 , 6778. https://doi.org/10.3390/su16166778
Stroumpoulis A, Kopanaki E, Chountalas PT. Enhancing Sustainable Supply Chain Management through Digital Transformation: A Comparative Case Study Analysis. Sustainability . 2024; 16(16):6778. https://doi.org/10.3390/su16166778
Stroumpoulis, Asterios, Evangelia Kopanaki, and Panos T. Chountalas. 2024. "Enhancing Sustainable Supply Chain Management through Digital Transformation: A Comparative Case Study Analysis" Sustainability 16, no. 16: 6778. https://doi.org/10.3390/su16166778
Article Metrics
Article access statistics, further information, mdpi initiatives, follow mdpi.
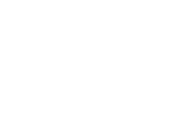
Subscribe to receive issue release notifications and newsletters from MDPI journals
Partnerships in the Real World: Great Supply Chain Partners 2024 Case Studies
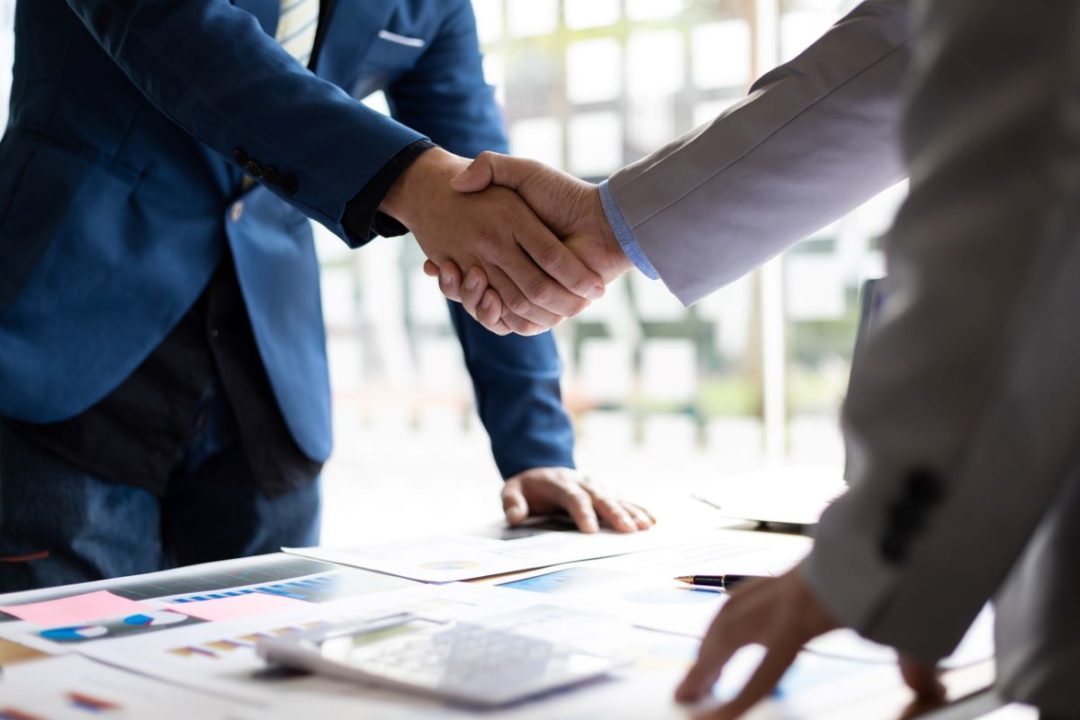
Photo: iStock.com/Wasan Tita
Our annual 100 Great Supply Chain Partners issue is dedicated to showing what supply chain excellence looks like in the real world — through partnership. Technology is critical, of course, but it requires expert help to use it to make supply chains hum.
The best way to illustrate the importance of excellent supply chain partnership is through real-world case studies, and this August’s issue is chock full of them.
See the Full List of 100 Great Supply Chain Partners 2024 Here.
You’ll read how almond producer Blue Diamond developed a strategy to acquire all of the software it needed to run a digitized global supply chain with end-to-end control, one piece at a time.
You’ll learn how BD Biosciences worked with a technology vendor to reduce delivery lead times at a global scale.
We’ll also tell you optimized storage capacity allowed security hardware firm Levata ’s new warehouse to efficiently manage a wide range of SKUs, maximizing storage utilization, and giving the company room to grow.
Read SupplyChainBrain’s latest 100 Great Supply Chain Partners issue now!
View the Digital Flip Book here.
Then, we’ll dig into how freight brokerage and transportation management company TI & NTG found a way to streamline multiple legacy systems for interacting with customers and carriers.
Next, you’ll discover how a golf equipment manufacturer, TaylorMade , took advantage of the fact that leases were expiring on its customization and distribution facilities to find a smart way to rejig its distribution network.
Another great partnership success story comes from kitchen cabinet manufacturer, American Woodmark , which deployed voice technology to help workers assembling cabinets perform better, and new employees get up to speed much faster than before.
Love Twinkies? Find out how snack giant Hostess significantly increased the accuracy and speed of data available regarding movement of goods in its warehouse.
Another intriguing case study comes from 3PL Langham Logistics , which deployed drones for inventory counts, meaning employees no longer spend long, tedious hours doing manual inventory with forklifts, and there is now less likelihood of misplacing product.
A well-designed supply chain should function like a precision Swiss watch. And timepiece brand Breitling saw sales increase by 20% in the U.S. the month after it introduced an omnichannel inventory management technology, with online sales revenue increasing by 15% to 30%.
Keeping workers happy is important. International shipping and mailing company Pitney Bowe s found a huge opportunity to improve operational efficiency, as well as employee retention, with a lively, ongoing feedback loop between workers and management.
Finally, we plunge into the world of swimming pools, with pool liner and cover vendor Tara Manufacturing , which reduced its downtime by 75%, saving the company $100,000 in the first year, after implementing computerized maintenance management system.
Running through these case studies is the need for new tools that automate manual processes and supplant legacy systems that were designed for a simpler and less volatile time. Artificial intelligence, in particular, comes to the fore as a means of overseeing just about every aspect of the supply chain, with its ability to make sense of more data than humans could ever manage.
But humans remain firmly in the loop, and here again we’re reminded of the value of partnership.
RELATED CONTENT
RELATED VIDEOS
Related Articles
100 great supply chain partners 2011, 100 great supply chain partners secrets to success 2006, related directories, tecsys, inc., u.s. battery manufacturers form coalition to boost domestic supply chain, subscribe to our daily newsletter.
Timely, incisive articles delivered directly to your inbox.
Popular Stories

ILA Sets Date to Prep for Potential East Coast, Gulf Port Strike

Long-Feared Canada Rail Strike Could Arrive by End of August

Canada Rail Union, Railways Agree to Restart Labor Negotiations

100 Great Supply Chain Partners 2024

Surviving in an Age of Permanent Disruption: We’re All in This Together
Digital edition.

100 Great Supply Chain Partners 2024: We're All in This Together
Case studies, recycled tagging fasteners: small changes make a big impact.
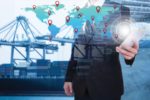
Enhancing High-Value Electronics Shipment Security with Tive's Real-Time Tracking
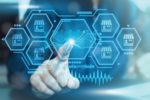
Moving Robots Site-to-Site
Jll finds perfect warehouse location, leading to $15m grant for startup, robots speed fulfillment to help apparel company scale for growth.
Embodied carbon flows in resource-based cities based on multiregional input–output model: a case study of Hohhot-Baotou-Ordos-Yulin urban agglomeration in China
- Original Paper
- Published: 08 August 2024
Cite this article
- Penghui Li 1 , 2 , 3 , 4 ,
- Chunyang He 1 , 2 , 3 , 5 ,
- Tao Qi 1 , 2 , 3 , 4 &
- Yida Wang 1 , 2 , 3 , 4
Explore all metrics
Managing embodied carbon flows in the supply chains of resource-based cities is crucial to China’s carbon neutrality. However, the embodied carbon flows in these regions are still unclear. Taking the Hohhot-Baotou-Ordos-Yulin (HBOY) urban agglomeration as a case, this study reveals the process and critical pathways of embodied carbon flows in resource-based cities by using environmentally extended multiregional input–output model and structural path analysis. The results indicated that embodied carbon flows from high-to-low emission intensity along the supply chain in HBOY. Spatially, Ordos located upstream in the supply chain, exhibited a net carbon outflow of 11.24 Mt to midstream and downstream areas, while Hohhot situated downstream, demonstrated a net carbon inflow of 6.87 Mt from upstream and midstream areas. Sectorally, a significant flow of embodied carbon from the electricity and heat supply industry to the construction industry and other service industry was observed, with 11.55 Mt and 10.08 Mt, respectively. The embodied carbon flows in HBOY were mainly driven by fixed capital formation, with 44.40% of the emissions in the top 20 critical paths attributed to it. To ensure the achievement of extensive carbon emission reduction targets in resource-based cities, it is essential to complement emission reduction measures on the production side with differentiated regional and sectoral policies that leverage the characteristics of embodied carbon flows.
Graphical abstract
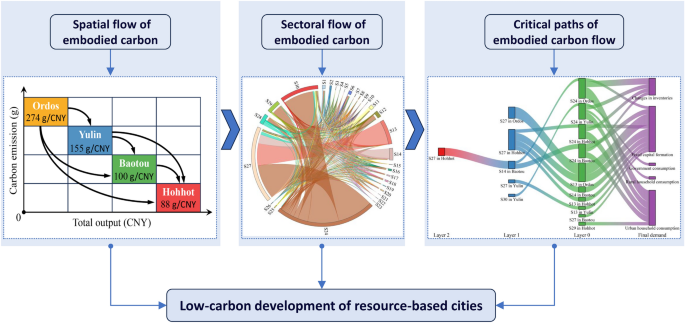
This is a preview of subscription content, log in via an institution to check access.
Access this article
Subscribe and save.
- Get 10 units per month
- Download Article/Chapter or eBook
- 1 Unit = 1 Article or 1 Chapter
- Cancel anytime
Price includes VAT (Russian Federation)
Instant access to the full article PDF.
Rent this article via DeepDyve
Institutional subscriptions
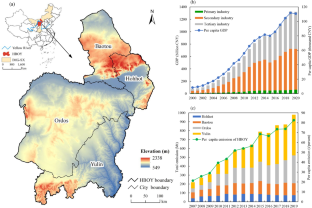
Similar content being viewed by others
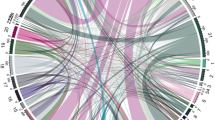
A study on carbon transfer and carbon emission critical paths in China: I-O analysis with multidimensional analytical framework
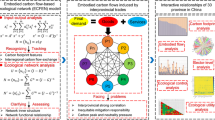
Exploring the embodied carbon flow interactive relationships in China from an ecological network perspective: a model framework and application at provincial level

China’s CO2 emissions embodied in fixed capital formation and its spatial distribution
Data availability.
Data and materials are available on reasonable request.
Bai Y, Zheng H, Shan Y, Meng J, Li Y (2021) The consumption based carbon emissions in the Jing Jin Ji urban agglomeration over China’s economic transition. Earth’s Future 9:e2021EF002132. https://doi.org/10.1029/2021ef002132
Article CAS Google Scholar
Chen W, Chen W, Ning S, Liu E, Zhou X, Wang Y, Zhao M (2019) Exploring the industrial land use efficiency of China’s resource-based cities. Cities 93:215–223. https://doi.org/10.1016/j.cities.2019.05.009
Article Google Scholar
Davis SJ, Caldeira K (2010) Consumption-based accounting of CO 2 emissions. Proc Natl Acad Sci 107(12):5687–5692. https://doi.org/10.1073/pnas.0906974107
Defourny J, Thorbecke E (1984) Structural path analysis and multiplier decomposition within a social accounting matrix framework. Econ J 94(373):111–136. https://doi.org/10.2307/2232220
Fang C, Zhang G, Xue D (2021) High-quality development of urban agglomerations in China and construction of science and technology collaborative innovation community. Acta Geogr Sin 76(12):2898–2908. https://doi.org/10.11821/dlxb202112003
Fry J, Geschke A, Langdon S, Lenzen M, Li M, Malik A, Sun YY, Wiedmann T (2021) Creating multi-scale nested MRIO tables for linking localized impacts to global consumption drivers. J Ind Ecol 26(1):281–293. https://doi.org/10.1111/jiec.13165
He D, Deng X, Wang X, Zhang F (2023a) Livestock greenhouse gas emission and mitigation potential in China. J Environ Manage 348:119494. https://doi.org/10.1016/j.jenvman.2023.119494
He T, Song H, Chen W (2023b) Recognizing the transformation characteristics of resource-based cities using night-time light remote sensing data: evidence from 126 cities in China. Resour Policy 85:104013. https://doi.org/10.1016/j.resourpol.2023.104013
Hou J, Wang Z, Zhang J, Yu S, Liu L (2022) Revealing energy and water hidden in Chinese regional critical carbon supply chains. Energy Policy 165:112979. https://doi.org/10.1016/j.enpol.2022.112979
Hou Y, Yang M, Li Y (2024) Coordinated effect of green expansion and carbon reduction: Evidence from sustainable development of resource-based cities in China. J Environ Manage 349:119534. https://doi.org/10.1016/j.jenvman.2023.119534
Li J, Ma Z, Sun H, Chen W (2023a) Driving factor analysis and dynamic forecast of industrial carbon emissions in resource-dependent cities: a case study of Ordos. China Environ Sci Pollut Res 30(40):92146–92161. https://doi.org/10.1007/s11356-023-28872-4
Li K, Zhou Y, Huang X, Xiao H, Shan Y (2023b) Low-carbon development pathways for resource-based cities in China under the carbon peaking and carbon neutrality goals. Environ Sci Pollut Res. https://doi.org/10.1007/s11356-023-28349-4
Li Y, Zhong H, Shan Y, Hang Y, Wang D, Zhou Y, Hubacek K (2023c) Changes in global food consumption increase GHG emissions despite efficiency gains along global supply chains. Nature Food 4(6):483–495. https://doi.org/10.1038/s43016-023-00768-z
Liu Z, Guan D, Wei W, Davis SJ, Ciais P, Bai J, Peng S, Zhang Q, Hubacek K, Marland G, Andres RJ, Crawford-Brown D, Lin J, Zhao H, Hong C, Boden TA, Feng K, Peters GP, Xi F, Liu J, Li Y, Zhao Y, Zeng N, He K (2015) Reduced carbon emission estimates from fossil fuel combustion and cement production in China. Nature 524(7565):335–338. https://doi.org/10.1038/nature14677
Liu Q, Long Y, Wang C, Wang Z, Wang Q, Guan D (2019) Drivers of provincial SO 2 emissions in China—Based on multi-regional input-output analysis. J Clean Prod 238:117893. https://doi.org/10.1016/j.jclepro.2019.117893
Liu Z, Huang Q, He C, Wang C, Wang Y, Li K (2021) Water-energy nexus within urban agglomeration: An assessment framework combining the multiregional input-output model, virtual water, and embodied energy. Resour Conserv Recycl 164:105113. https://doi.org/10.1016/j.resconrec.2020.105113
Liu Z, Dou X, Yu Y, Tan J, Sun T, Meng J (2023) China’s embodied carbon transfer in global trade. China J Economet 3(4):1225–1242. https://doi.org/10.12012/CJoE2022-0048(inChinese)
Long Y, Yoshida Y, Liu Q, Zhang H, Wang S, Fang K (2020) Comparison of city-level carbon footprint evaluation by applying single- and multi-regional input-output tables. J Environ Manage 260:110108. https://doi.org/10.1016/j.jenvman.2020.110108
Ma R, Li K, Guo Y, Zhang B, Zhao X, Linder S, Guan C, Chen G, Gan Y, Meng J (2021) Mitigation potential of global ammonia emissions and related health impacts in the trade network. Nat Commun 12:6308. https://doi.org/10.1038/s41467-021-25854-3
Meng B, Peters GP, Wang Z, Li M (2018a) Tracing CO 2 emissions in global value chains. Energy Econ 73:24–42. https://doi.org/10.1016/j.eneco.2018.05.013
Meng J, Mi Z, Guan D, Li J, Tao S, Li Y, Feng K, Liu J, Liu Z, Wang X, Zhang Q, Davis SJ (2018b) The rise of South-South trade and its effect on global CO 2 emissions. Nat Commun 9:1871. https://doi.org/10.1038/s41467-018-04337-y
Meng J, Huo J, Zhang Z, Liu Y, Mi Z, Guan D, Feng K (2023) The narrowing gap in developed and developing country emission intensities reduces global trade’s carbon leakage. Nat Commun 14:3775. https://doi.org/10.1038/s41467-023-39449-7
Mi Z, Meng J, Zheng H, Shan Y, Wei Y-M, Guan D (2018) A multi-regional input-output table mapping China’s economic outputs and interdependencies in 2012. Sci Data 5:180155. https://doi.org/10.1038/sdata.2018.155
Minx JC, Wiedmann T, Wood R, Peters GP, Lenzen M, Owen A, Scott K, Barrett J, Hubacek K, Baiocchi G, Paul A, Dawkins E, Briggs J, Guan D, Suh S, Ackerman F (2009) Input-output analysis and carbon footprinting: an overview of applications. Econ Syst Res 21(3):187–216. https://doi.org/10.1080/09535310903541298
NDRCC (National Development and Reform Commission of China) (2018) Development Plan for the Hohhot-Baotou-Ordos-Yulin Urban Agglomeration. http://www.gov.cn/xinwen/2018-03/07/5271788/files/d186cc88913b48039197494c40773021.pdf/ , Accessed 9th Nov 2022
Pehl M, Arvesen A, Humpenöder F, Popp A, Hertwich EG, Luderer G (2017) Understanding future emissions from low-carbon power systems by integration of life-cycle assessment and integrated energy modelling. Nat Energy 2(12):939–945. https://doi.org/10.1038/s41560-017-0032-9
Pu Y, Wang P, Wang Y, Qiao W, Wang L, Zhang Y (2021) Environmental effects evaluation of photovoltaic power industry in China on life cycle assessment. J Clean Prod 278:123993. https://doi.org/10.1016/j.jclepro.2020.123993
Qi T, Ren Q, Zhang D, Lu W, He C (2023) Impacts of urban expansion on vegetation in drylands: a multiscale analysis based on the vegetation disturbance index. Ecol Ind 147:109984. https://doi.org/10.1016/j.ecolind.2023.109984
Qian Y, Zheng H, Meng J, Shan Y, Zhou Y, Guan D (2022) Large inter-city inequality in consumption-based CO 2 emissions for China’s pearl river basin cities. Resour Conserv Recycl 176:105923. https://doi.org/10.1016/j.resconrec.2021.105923
Shan Y, Liu J, Liu Z, Shao S, Guan D (2019) An emissions-socioeconomic inventory of Chinese cities. Sci Data 6:190027. https://doi.org/10.1038/sdata.2019.27
Shan Y, Huang Q, Guan D, Hubacek K (2020) China CO 2 emission accounts 2016–2017. Sci Data 7:54. https://doi.org/10.1038/s41597-020-0393-y
Shan Y (2018) China emissions accounts and low-carbon development in cities. Doctoral thesis, University of East Anglia. https://ueaeprints.uea.ac.uk/id/eprint/67787
Song J, Qi Z, Gai Y, Chen S (2022) Shared network and supply chain features for synergetic control of carbon and air pollutant emissions. Sci Total Environ 827:154391. https://doi.org/10.1016/j.scitotenv.2022.154391
Sun Y, Wang Y, Zheng H, Zhang Z, Wang Y, Li H, Lu Y (2022) Critical supply chains of NOx emissions in the Beijing-Tianjin-Hebei urban agglomeration. J Clean Prod 362:132379. https://doi.org/10.1016/j.jclepro.2022.132379
Tian C, Qi L (2023) Empirical decomposition and peaking path of carbon emissions in resource-based areas. J Clean Prod. https://doi.org/10.1016/j.jclepro.2023.136372
Wang Z, Cui C, Peng S (2018) Critical sectors and paths for climate change mitigation within supply chain networks. J Environ Manage 226:30–36. https://doi.org/10.1016/j.jenvman.2018.08.018
Wang W, Hu Y, Lu Y (2023) Driving forces of China’s provincial bilateral carbon emissions and the redefinition of corresponding responsibilities. Sci Total Environ 857:159404. https://doi.org/10.1016/j.scitotenv.2022.159404
Wen L, Zhang Y (2020) A study on carbon transfer and carbon emission critical paths in China: I–O analysis with multidimensional analytical framework. Environ Sci Pollut Res 27(9):9733–9747. https://doi.org/10.1007/s11356-019-07549-x
Wu H, Zeng X, Zhang L, Liu X, Jiang S, Dong Z, Meng X, Wang Q (2023) Water-energy nexus embedded in coal supply chain of a coal-based city. China Resour Policy 85:103812. https://doi.org/10.1016/j.resourpol.2023.103812
Xia C, Zheng H, Meng J, Li S, Du P, Shan Y (2022) The evolution of carbon footprint in the yangtze river delta city cluster during economic transition 2012–2015. Resour Conserv Recycl 181:106266. https://doi.org/10.1016/j.resconrec.2022.106266
Xing Z, Jiao Z, Wang H (2022) Carbon footprint and embodied carbon transfer at city level: a nested MRIO analysis of Central Plain urban agglomeration in China. Sustain Cities Soc 83:103977. https://doi.org/10.1016/j.scs.2022.103977
Xu D, Zhang Y, Chen B, Bai J, Liu G, Zhang B (2022) Identifying the critical paths and sectors for carbon transfers driven by global consumption in 2015. Appl Energy 306:118137. https://doi.org/10.1016/j.apenergy.2021.118137
Yang M, Liang X, Ma Y, Lu W, Zhao R (2023) Exploring the carbon inequality embodied in China’s interregional trade based on a human well-being equity perspective. J Clean Prod 420:138367. https://doi.org/10.1016/j.jclepro.2023.138367
Yuan X, Sheng X, Chen L, Tang Y, Li Y, Jia Y, Qu D, Wang Q, Ma Q, Zuo J (2022) Carbon footprint and embodied carbon transfer at the provincial level of the Yellow River Basin. Sci Total Environ 803:149993. https://doi.org/10.1016/j.scitotenv.2021.149993
Zhang B, Qiao H, Chen ZM, Chen B (2016) Growth in embodied energy transfers via China’s domestic trade: Evidence from multi-regional input–output analysis. Appl Energy 184:1093–1105. https://doi.org/10.1016/j.apenergy.2015.09.076
Zhang H, Zhang W, Lu Y, Wang Y, Shan Y, Ping L, Li H, Lee L-C, Wang T, Liang C, Jiang H, Cao D (2023) Worsening carbon inequality embodied in trade within China. Environ Sci Technol 57(2):863–873. https://doi.org/10.1021/acs.est.2c05990
Zheng H, Meng J, Mi Z, Song M, Shan Y, Ou J, Guan D (2019) Linking city-level input–output table to urban energy footprint: construction framework and application. J Ind Ecol 23(4):781–795. https://doi.org/10.1111/jiec.12835
Zheng H, Többen J, Dietzenbacher E, Moran D, Meng J, Wang D, Guan D (2021) Entropy-based Chinese city-level MRIO table framework. Econ Syst Res 34(4):519–544. https://doi.org/10.1080/09535314.2021.1932764
Zheng H, Zhang Z, Dietzenbacher E, Zhou Y, Többen J, Feng K, Moran D, Jiang M, Shan Y, Wang D, Liu X, Li L, Zhao D, Meng J, Ou J, Guan D (2023) Leveraging opportunity of low carbon transition by super-emitter cities in China. Science Bulletin 68(20):2456–2466. https://doi.org/10.1016/j.scib.2023.08.016
Zhou S, Li W, Lu Z, Lu Z (2022) A technical framework for integrating carbon emission peaking factors into the industrial green transformation planning of a city cluster in China. J Clean Prod 344:131091. https://doi.org/10.1016/j.jclepro.2022.131091
Download references
Acknowledgements
We thank the editors and anonymous reviewers for their valuable comments and suggestions, which have improved the quality of the manuscript.
This research was supported by the National Natural Science Foundation of China (grant No. 42371296) and BNU-FGS Global Environmental Change Program (grant No. 2023-GC-ZYTS-08). It was also funded by a project supported by State Key Laboratory of Earth Surface Processes and Resource Ecology (grant No. 2022-GS-02) and Key Laboratory of Environmental Change and Natural Disaster of Ministry of Education, Beijing Normal University (grant No. 2022-KF-03).
Author information
Authors and affiliations.
Key Laboratory of Environmental Change and Natural Disasters of Chinese Ministry of Education, Beijing Normal University, Beijing 100875, China
Penghui Li, Chunyang He, Tao Qi & Yida Wang
State Key Laboratory of Earth Surface Processes and Resource Ecology (ESPRE), Beijing Normal University, Beijing 100875, China
Academy of Disaster Reduction and Emergency Management, Ministry of Emergency Management and Ministry of Education, Beijing 100875, China
Faculty of Geographical Science, Beijing Normal University, Beijing 100875, China
Penghui Li, Tao Qi & Yida Wang
Academy of Plateau Science and Sustainability, People’s Government of Qinghai Province and Beijing Normal University, Xining, China
Chunyang He
You can also search for this author in PubMed Google Scholar
Contributions
PL presented conceptualization, methodology, visualization, and writing—original draft. CH provided supervision. TQ and YW participated in investigation. All authors contributed to writing—review & editing.
Corresponding author
Correspondence to Chunyang He .
Ethics declarations
Conflict of interest.
The authors declare no competing interests.
Additional information
Publisher's note.
Springer Nature remains neutral with regard to jurisdictional claims in published maps and institutional affiliations.
Supplementary Information
Below is the link to the electronic supplementary material.
Supplementary file1 (DOCX 6139 KB)
Rights and permissions.
Springer Nature or its licensor (e.g. a society or other partner) holds exclusive rights to this article under a publishing agreement with the author(s) or other rightsholder(s); author self-archiving of the accepted manuscript version of this article is solely governed by the terms of such publishing agreement and applicable law.
Reprints and permissions
About this article
Li, P., He, C., Qi, T. et al. Embodied carbon flows in resource-based cities based on multiregional input–output model: a case study of Hohhot-Baotou-Ordos-Yulin urban agglomeration in China. Clean Techn Environ Policy (2024). https://doi.org/10.1007/s10098-024-02974-0
Download citation
Received : 13 March 2024
Accepted : 16 July 2024
Published : 08 August 2024
DOI : https://doi.org/10.1007/s10098-024-02974-0
Share this article
Anyone you share the following link with will be able to read this content:
Sorry, a shareable link is not currently available for this article.
Provided by the Springer Nature SharedIt content-sharing initiative
- Resource-based cities
- Embodied carbon flows
- Multiregional input–output
- Structural path analysis
- Hohhot-Baotou-Ordos-Yulin urban agglomeration
- Find a journal
- Publish with us
- Track your research
Supply chain management performance analysis using SCOR method: A case of coffee agroindustry in Indonesia
- Suryaningrat, Ida Bagus
- Wibowo, Yuli
- Ansori, Ludfi
- Kuswardhani, Nita
- Purnomo, Bambang Herry
Competition between industries is getting tighter, so it becomes a challenge to carry out production activities. The company realizes that to be able to compete with competitors, it must produce quality products, cheaply, quickly, and by the wishes and needs of consumers. Kahyangan Coffee Agroindustry is a diversified business from PDP (District Plantation Company) Kahyangan whose main product is coffee. PDP Kahyangan conducts coffee agro-industry activities due to the unstable selling price of ose (ground) coffee. Indications of problems in the industry are low market share and relatively poor performance. The company must have the right strategy in facing competition by optimizing supply chain management. This study uses the SCOR (Supply Chain Operation Reference). The SCOR model can map supply chain activities through 5 core processes of plan, source, make, deliver, and return with dimensions of reliability, responsiveness, flexibility, cost, and assets that have indicators so that they can be used as benchmarks in measuring supply chain management performance. Then identify Key Performance Indicators (KPI) to adjust company conditions and normalize Snorm de Boer to equalize the KPI scale. Furthermore, weighting is carried out to determine the priority of each performance indicator using AHP (Analytical Hierarchy Process). The results showed that in the process of producing ground coffee in the Kahyangan coffee agroindustry, 26 KPIs were produced. The value of supply chain performance in this industry is 79.16 which indicates that the performance achievement is categorized as good (good) but needs to be improved on performance indicators that have low scores. 4 KPIs have the lowest values and must be prioritized for improvement, namely the suitability of the production plan with demand (53.85), quality of raw materials (58.33), efficiency of use of raw materials and machinery in the production process (54.55), and the average marketing staff who received marketing training (50).
- APPLIED MATHEMATICS

IMAGES
COMMENTS
In this section, I'm exploring a selection of innovative supply chain analytics use cases that illustrate the transformative impact this discipline can have on organizational efficiency, profitability, and resilience. Read on to understand the breadth of possibilities. 1. End-to-end supply chain visibility dashboards.
Support on supply chain analytics. Gartner provides trusted insights and objective advice to help its 2,500+ supply chain leader clients build a strong supply chain analytics strategy. Supply chain analytics refers to gaining insight from the large amounts of data associated with the procurement, processing, and distribution of goods.
by Matt Lowe, G V Nadhanael, and Benjamin N. Roth. Policy makers in the developing world face important tradeoffs in reacting to a pandemic. The quick and complete recovery of India's food supply chain suggests that strict lockdown measures at the onset of pandemics need not cause long-term economic damage.
Your supply chains generate big data. Big supply-chain analytics turn that data into real insights. The explosive impact of e-commerce on traditional brick and mortar retailers is just one notable example of the data-driven revolution that is sweeping many industries and business functions today. Few companies, however, have been able to apply ...
Dissecting a Supply Chain's Digital Twin. At the heart of the supply chain digital twin development lies a flexible supply chain simulation model that is data-driven and. Case studies of real-time supply chain analytics and inventory control.
Supply chain analytics can support planning efforts that broadly fall into three types. While optimization has been at the center of this article, two other tools are simulation and monitoring. ... In a recent case study, the large mining player developed an optimization-based process to improve the supply chain of its trains, vessels, and mine ...
Supply chain data analytics is a process that involves: Capturing data from multiple sources. Integrating that disparate data. Analyzing the results through ad hoc analyses, visualizations, and reports. Since most supply chains involve a network of different organizations and business departments, there are typically numerous different systems ...
Supply chain 4.0 is all about the application of the Internet of Things, robotics, big data and predictive analytics in supply chain management. The main goal is to create a 'smart' supply chain that utilizes data from various types of sensors and all the available sources in order to optimize the processes. "Smart manufacturing processes ...
In this context, Walmart, the world's largest retailer, has demonstrated a highly successful and integrated Walmart supply chain, propelling its growth and dominance in the retail industry. This case study aims to delve into the significance of supply chain capability for enhancing a company's competitiveness and how it serves as a ...
Learn how to use Power BI for supply chain analytics in this case study. Create a make vs. buy analysis tool, calculate costs, and analyze production volumes. This course focuses on creating a powerful make versus buy analysis tool using Power BI. Through this case study, you will develop a quote analysis tool, calculate costs for various ...
A Supply Chain Analysis Project using Microsoft Excel and Microsoft Power BI. Supply chain analytics plays a crucial role in driving data-driven decision-making across industries, including manufacturing, retail, healthcare, and logistics. In this report, I presented an analysis of a Fashion and Beauty startup's supply chain data ...
Big data analytics (BDA) in supply chain management (SCM) is receiving a growing attention. This is due to the fact that BDA has a wide range of applications in SCM, including customer behavior analysis, trend analysis, and demand prediction. ... Hopkins J, Hawking P. Big Data Analytics and IoT in logistics: a case study. Int J Logist Manage ...
IBM employs supply chain staff in 40 countries and makes hundreds of thousands of customer deliveries and service calls in over 170 nations. IBM also collaborates with hundreds of suppliers across its multi-tier global network to build highly configurable and customized products to customer specifications. Previously, the IBM supply chain ran ...
Abstract. Although predictive machine learning for supply chain data analytics has recently been reported as a significant area of investigation due to the rising popularity of the AI paradigm in industry, there is a distinct lack of case studies that showcase its application from a practical point of view.
Pfizer's move toward supply chain analytics has helped it transform into a leaner, more science-driven, more patient-focused organization. Success has required clearly communicating the company ...
Start with these 5 Manufacturing analytics use cases for supply chain. 1. Production Analytics for Operational Efficiency. There are a series of processes going on in parallel and it generates a huge volume of data ranging from machine run-time to no. of units produced.
Explore how supply chain analytics in manufacturing can transform your operations, increase efficiency, and boost revenues! Schedule a Call. Search for: Services. ... Here's a stellar case study that shows the transformative power of analytics in manufacturing! Case Study: Transforming AMZ Prep's Supply Chain with Analytics ...
These demand-driven supply chains are driven by analytics. She outlined five predictions: We will build outside-in processes using channel data to improve demand signals (See Costa case study ). Analytics will enable these supply chains to simulate the profitability of responses and learn from successes and failures.
This study aims to explore the relationship between supply chain digitalization and the adoption of comprehensive sustainability practices. The research employs a qualitative methodology, including a comparative case study analysis of a modern 3PL company, a retailer, and a producer of construction materials.
Another intriguing case study comes from 3PL Langham Logistics, which deployed drones for inventory counts, meaning employees no longer spend long, tedious hours doing manual inventory with forklifts, and there is now less likelihood of misplacing product. A well-designed supply chain should function like a precision Swiss watch.
Managing embodied carbon flows in the supply chains of resource-based cities is crucial to China's carbon neutrality. However, the embodied carbon flows in these regions are still unclear. Taking the Hohhot-Baotou-Ordos-Yulin (HBOY) urban agglomeration as a case, this study reveals the process and critical pathways of embodied carbon flows in resource-based cities by using environmentally ...
The company must have the right strategy in facing competition by optimizing supply chain management. This study uses the SCOR (Supply Chain Operation Reference). The SCOR model can map supply chain activities through 5 core processes of plan, source, make, deliver, and return with dimensions of reliability, responsiveness, flexibility, cost ...