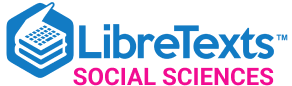
- school Campus Bookshelves
- menu_book Bookshelves
- perm_media Learning Objects
- login Login
- how_to_reg Request Instructor Account
- hub Instructor Commons

Margin Size
- Download Page (PDF)
- Download Full Book (PDF)
- Periodic Table
- Physics Constants
- Scientific Calculator
- Reference & Cite
- Tools expand_more
- Readability
selected template will load here
This action is not available.
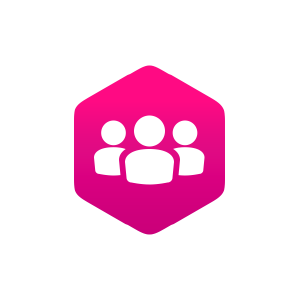
11.4: Research Methods in Social Psychology
- Last updated
- Save as PDF
- Page ID 10665
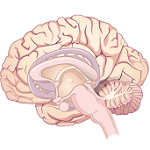
- https://nobaproject.com/ via The Noba Project
\( \newcommand{\vecs}[1]{\overset { \scriptstyle \rightharpoonup} {\mathbf{#1}} } \)
\( \newcommand{\vecd}[1]{\overset{-\!-\!\rightharpoonup}{\vphantom{a}\smash {#1}}} \)
\( \newcommand{\id}{\mathrm{id}}\) \( \newcommand{\Span}{\mathrm{span}}\)
( \newcommand{\kernel}{\mathrm{null}\,}\) \( \newcommand{\range}{\mathrm{range}\,}\)
\( \newcommand{\RealPart}{\mathrm{Re}}\) \( \newcommand{\ImaginaryPart}{\mathrm{Im}}\)
\( \newcommand{\Argument}{\mathrm{Arg}}\) \( \newcommand{\norm}[1]{\| #1 \|}\)
\( \newcommand{\inner}[2]{\langle #1, #2 \rangle}\)
\( \newcommand{\Span}{\mathrm{span}}\)
\( \newcommand{\id}{\mathrm{id}}\)
\( \newcommand{\kernel}{\mathrm{null}\,}\)
\( \newcommand{\range}{\mathrm{range}\,}\)
\( \newcommand{\RealPart}{\mathrm{Re}}\)
\( \newcommand{\ImaginaryPart}{\mathrm{Im}}\)
\( \newcommand{\Argument}{\mathrm{Arg}}\)
\( \newcommand{\norm}[1]{\| #1 \|}\)
\( \newcommand{\Span}{\mathrm{span}}\) \( \newcommand{\AA}{\unicode[.8,0]{x212B}}\)
\( \newcommand{\vectorA}[1]{\vec{#1}} % arrow\)
\( \newcommand{\vectorAt}[1]{\vec{\text{#1}}} % arrow\)
\( \newcommand{\vectorB}[1]{\overset { \scriptstyle \rightharpoonup} {\mathbf{#1}} } \)
\( \newcommand{\vectorC}[1]{\textbf{#1}} \)
\( \newcommand{\vectorD}[1]{\overrightarrow{#1}} \)
\( \newcommand{\vectorDt}[1]{\overrightarrow{\text{#1}}} \)
\( \newcommand{\vectE}[1]{\overset{-\!-\!\rightharpoonup}{\vphantom{a}\smash{\mathbf {#1}}}} \)
Kwantlen Polytechnic University
Social psychologists are interested in the ways that other people affect thought, emotion, and behavior. To explore these concepts requires special research methods. Following a brief overview of traditional research designs, this module introduces how complex experimental designs, field experiments, naturalistic observation, experience sampling techniques, survey research, subtle and nonconscious techniques such as priming, and archival research and the use of big data may each be adapted to address social psychological questions. This module also discusses the importance of obtaining a representative sample along with some ethical considerations that social psychologists face.
learning objectives
- Describe the key features of basic and complex experimental designs.
- Describe the key features of field experiments, naturalistic observation, and experience sampling techniques.
- Describe survey research and explain the importance of obtaining a representative sample.
- Describe the implicit association test and the use of priming.
- Describe use of archival research techniques.
- Explain five principles of ethical research that most concern social psychologists.
Introduction
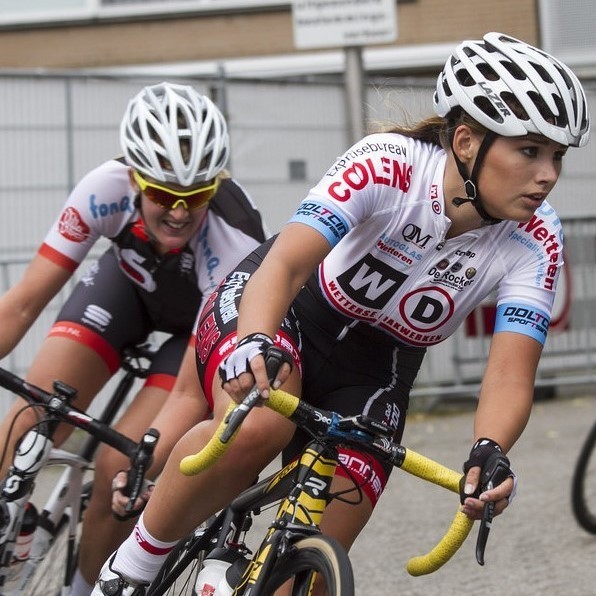
Are you passionate about cycling? Norman Triplett certainly was. At the turn of last century he studied the lap times of cycling races and noticed a striking fact: riding in competitive races appeared to improve riders’ times by about 20-30 seconds every mile compared to when they rode the same courses alone. Triplett suspected that the riders’ enhanced performance could not be explained simply by the slipstream caused by other cyclists blocking the wind. To test his hunch, he designed what is widely described as the first experimental study in social psychology (published in 1898!)—in this case, having children reel in a length of fishing line as fast as they could. The children were tested alone, then again when paired with another child. The results? The children who performed the task in the presence of others out-reeled those that did so alone.
Although Triplett’s research fell short of contemporary standards of scientific rigor (e.g., he eyeballed the data instead of measuring performance precisely; Stroebe, 2012), we now know that this effect, referred to as “ social facilitation ,” is reliable—performance on simple or well-rehearsed tasks tends to be enhanced when we are in the presence of others (even when we are not competing against them). To put it another way, the next time you think about showing off your pool-playing skills on a date, the odds are you’ll play better than when you practice by yourself. (If you haven’t practiced, maybe you should watch a movie instead!)
Research Methods in Social Psychology
One of the things Triplett’s early experiment illustrated is scientists’ reliance on systematic observation over opinion, or anecdotal evidence . The scientific method usually begins with observing the world around us (e.g., results of cycling competitions) and thinking of an interesting question (e.g., Why do cyclists perform better in groups?). The next step involves generating a specific testable prediction, or hypothesis (e.g., performance on simple tasks is enhanced in the presence of others). Next, scientists must operationalize the variables they are studying. This means they must figure out a way to define and measure abstract concepts. For example, the phrase “perform better” could mean different things in different situations; in Triplett’s experiment it referred to the amount of time (measured with a stopwatch) it took to wind a fishing reel. Similarly, “in the presence of others” in this case was operationalized as another child winding a fishing reel at the same time in the same room. Creating specific operational definitions like this allows scientists to precisely manipulate the independent variable , or “cause” (the presence of others), and to measure the dependent variable , or “effect” (performance)—in other words, to collect data. Clearly described operational definitions also help reveal possible limitations to studies (e.g., Triplett’s study did not investigate the impact of another child in the room who was not also winding a fishing reel) and help later researchers replicate them precisely.
Laboratory Research
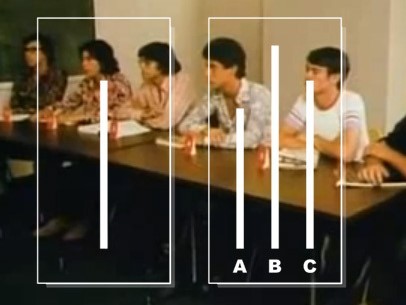
As you can see, social psychologists have always relied on carefully designed laboratory environments to run experiments where they can closely control situations and manipulate variables (see the NOBA module on Research Designs for an overview of traditional methods). However, in the decades since Triplett discovered social facilitation, a wide range of methods and techniques have been devised, uniquely suited to demystifying the mechanics of how we relate to and influence one another. This module provides an introduction to the use of complex laboratory experiments, field experiments, naturalistic observation, survey research, nonconscious techniques, and archival research, as well as more recent methods that harness the power of technology and large data sets, to study the broad range of topics that fall within the domain of social psychology. At the end of this module we will also consider some of the key ethical principles that govern research in this diverse field.
The use of complex experimental designs , with multiple independent and/or dependent variables, has grown increasingly popular because they permit researchers to study both the individual and joint effects of several factors on a range of related situations. Moreover, thanks to technological advancements and the growth of social neuroscience , an increasing number of researchers now integrate biological markers (e.g., hormones) or use neuroimaging techniques (e.g., fMRI) in their research designs to better understand the biological mechanisms that underlie social processes.
We can dissect the fascinating research of Dov Cohen and his colleagues (1996) on “culture of honor” to provide insights into complex lab studies. A culture of honor is one that emphasizes personal or family reputation. In a series of lab studies, the Cohen research team invited dozens of university students into the lab to see how they responded to aggression. Half were from the Southern United States (a culture of honor) and half were from the Northern United States (not a culture of honor; this type of setup constitutes a participant variable of two levels). Region of origin was independent variable #1. Participants also provided a saliva sample immediately upon arriving at the lab; (they were given a cover story about how their blood sugar levels would be monitored over a series of tasks).
The participants completed a brief questionnaire and were then sent down a narrow corridor to drop it off on a table. En route, they encountered a confederate at an open file cabinet who pushed the drawer in to let them pass. When the participant returned a few seconds later, the confederate, who had re-opened the file drawer, slammed it shut and bumped into the participant with his shoulder, muttering “asshole” before walking away. In a manipulation of an independent variable—in this case, the insult—some of the participants were insulted publicly (in view of two other confederates pretending to be doing homework) while others were insulted privately (no one else was around). In a third condition—the control group—participants experienced a modified procedure in which they were not insulted at all.
Although this is a fairly elaborate procedure on its face, what is particularly impressive is the number of dependent variables the researchers were able to measure. First, in the public insult condition, the two additional confederates (who observed the interaction, pretending to do homework) rated the participants’ emotional reaction (e.g., anger, amusement, etc.) to being bumped into and insulted. Second, upon returning to the lab, participants in all three conditions were told they would later undergo electric shocks as part of a stress test, and were asked how much of a shock they would be willing to receive (between 10 volts and 250 volts). This decision was made in front of two confederates who had already chosen shock levels of 75 and 25 volts, presumably providing an opportunity for participants to publicly demonstrate their toughness. Third, across all conditions, the participants rated the likelihood of a variety of ambiguously provocative scenarios (e.g., one driver cutting another driver off) escalating into a fight or verbal argument. And fourth, in one of the studies, participants provided saliva samples, one right after returning to the lab, and a final one after completing the questionnaire with the ambiguous scenarios. Later, all three saliva samples were tested for levels of cortisol (a hormone associated with stress) and testosterone (a hormone associated with aggression).
The results showed that people from the Northern United States were far more likely to laugh off the incident (only 35% having anger ratings as high as or higher than amusement ratings), whereas the opposite was true for people from the South (85% of whom had anger ratings as high as or higher than amusement ratings). Also, only those from the South experienced significant increases in cortisol and testosterone following the insult (with no difference between the public and private insult conditions). Finally, no regional differences emerged in the interpretation of the ambiguous scenarios; however, the participants from the South were more likely to choose to receive a greater shock in the presence of the two confederates.
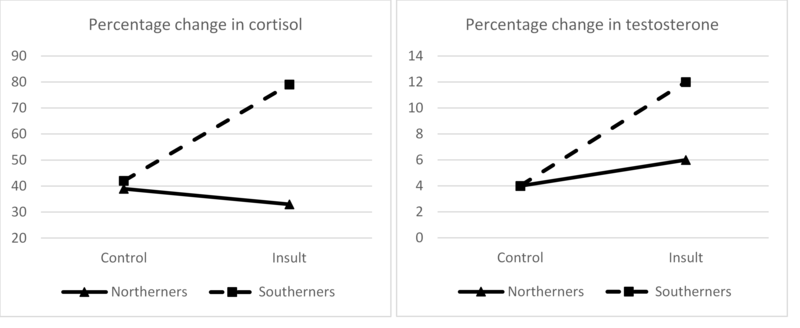
Field Research
Because social psychology is primarily focused on the social context—groups, families, cultures—researchers commonly leave the laboratory to collect data on life as it is actually lived. To do so, they use a variation of the laboratory experiment, called a field experiment . A field experiment is similar to a lab experiment except it uses real-world situations, such as people shopping at a grocery store. One of the major differences between field experiments and laboratory experiments is that the people in field experiments do not know they are participating in research, so—in theory—they will act more naturally. In a classic example from 1972, Alice Isen and Paula Levin wanted to explore the ways emotions affect helping behavior. To investigate this they observed the behavior of people at pay phones (I know! Pay phones! ). Half of the unsuspecting participants (determined by random assignment ) found a dime planted by researchers (I know! A dime! ) in the coin slot, while the other half did not. Presumably, finding a dime felt surprising and lucky and gave people a small jolt of happiness. Immediately after the unsuspecting participant left the phone booth, a confederate walked by and dropped a stack of papers. Almost 100% of those who found a dime helped to pick up the papers. And what about those who didn’t find a dime? Only 1 out 25 of them bothered to help.
In cases where it’s not practical or ethical to randomly assign participants to different experimental conditions, we can use naturalistic observation —unobtrusively watching people as they go about their lives. Consider, for example, a classic demonstration of the “ basking in reflected glory ” phenomenon: Robert Cialdini and his colleagues used naturalistic observation at seven universities to confirm that students are significantly more likely to wear clothing bearing the school name or logo on days following wins (vs. draws or losses) by the school’s varsity football team (Cialdini et al., 1976). In another study, by Jenny Radesky and her colleagues (2014), 40 out of 55 observations of caregivers eating at fast food restaurants with children involved a caregiver using a mobile device. The researchers also noted that caregivers who were most absorbed in their device tended to ignore the children’s behavior, followed by scolding, issuing repeated instructions, or using physical responses, such as kicking the children’s feet or pushing away their hands.
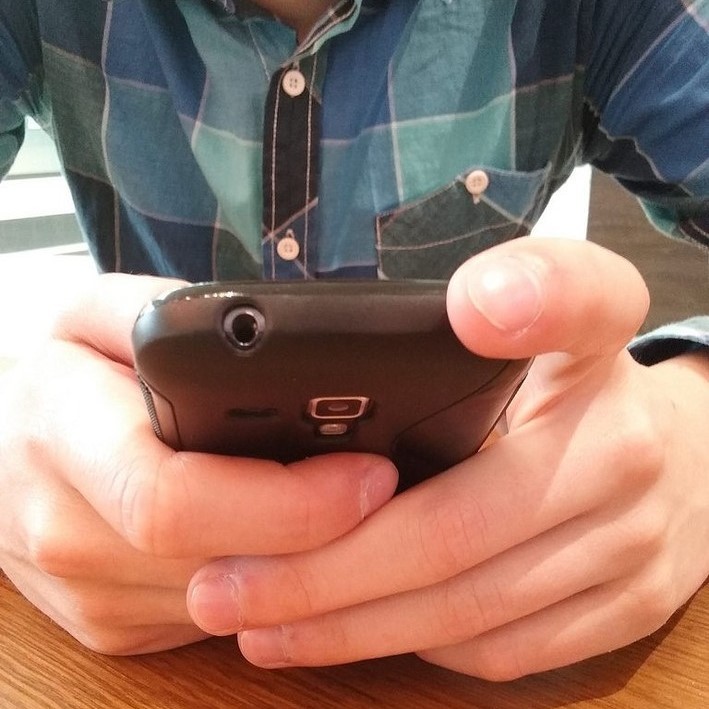
A group of techniques collectively referred to as experience sampling methods represent yet another way of conducting naturalistic observation, often by harnessing the power of technology. In some cases, participants are notified several times during the day by a pager, wristwatch, or a smartphone app to record data (e.g., by responding to a brief survey or scale on their smartphone, or in a diary). For example, in a study by Reed Larson and his colleagues (1994), mothers and fathers carried pagers for one week and reported their emotional states when beeped at random times during their daily activities at work or at home. The results showed that mothers reported experiencing more positive emotional states when away from home (including at work), whereas fathers showed the reverse pattern. A more recently developed technique, known as the electronically activated recorder , or EAR, does not even require participants to stop what they are doing to record their thoughts or feelings; instead, a small portable audio recorder or smartphone app is used to automatically record brief snippets of participants’ conversations throughout the day for later coding and analysis. For a more in-depth description of the EAR technique and other experience-sampling methods, see the NOBA module on Conducting Psychology Research in the Real World.
Survey Research
In this diverse world, survey research offers itself as an invaluable tool for social psychologists to study individual and group differences in people’s feelings, attitudes, or behaviors. For example, the World Values Survey II was based on large representative samples of 19 countries and allowed researchers to determine that the relationship between income and subjective well-being was stronger in poorer countries (Diener & Oishi, 2000). In other words, an increase in income has a much larger impact on your life satisfaction if you live in Nigeria than if you live in Canada. In another example, a nationally-representative survey in Germany with 16,000 respondents revealed that holding cynical beliefs is related to lower income (e.g., between 2003-2012 the income of the least cynical individuals increased by $300 per month, whereas the income of the most cynical individuals did not increase at all). Furthermore, survey data collected from 41 countries revealed that this negative correlation between cynicism and income is especially strong in countries where people in general engage in more altruistic behavior and tend not to be very cynical (Stavrova & Ehlebracht, 2016).
Of course, obtaining large, cross-cultural, and representative samples has become far easier since the advent of the internet and the proliferation of web-based survey platforms—such as Qualtrics—and participant recruitment platforms—such as Amazon’s Mechanical Turk. And although some researchers harbor doubts about the representativeness of online samples, studies have shown that internet samples are in many ways more diverse and representative than samples recruited from human subject pools (e.g., with respect to gender; Gosling et al., 2004). Online samples also compare favorably with traditional samples on attentiveness while completing the survey, reliability of data, and proportion of non-respondents (Paolacci et al., 2010).
Subtle/Nonconscious Research Methods
The methods we have considered thus far—field experiments, naturalistic observation, and surveys—work well when the thoughts, feelings, or behaviors being investigated are conscious and directly or indirectly observable. However, social psychologists often wish to measure or manipulate elements that are involuntary or nonconscious, such as when studying prejudicial attitudes people may be unaware of or embarrassed by. A good example of a technique that was developed to measure people’s nonconscious (and often ugly) attitudes is known as the implicit association test (IAT) (Greenwald et al., 1998). This computer-based task requires participants to sort a series of stimuli (as rapidly and accurately as possible) into simple and combined categories while their reaction time is measured (in milliseconds). For example, an IAT might begin with participants sorting the names of relatives (such as “Niece” or “Grandfather”) into the categories “Male” and “Female,” followed by a round of sorting the names of disciplines (such as “Chemistry” or “English”) into the categories “Arts” and “Science.” A third round might combine the earlier two by requiring participants to sort stimuli into either “Male or Science” or “Female and Arts” before the fourth round switches the combinations to “Female or Science” and “Male and Arts.” If across all of the trials a person is quicker at accurately sorting incoming stimuli into the compound category “Male or Science” than into “Female or Science,” the authors of the IAT suggest that the participant likely has a stronger association between males and science than between females and science. Incredibly, this specific gender-science IAT has been completed by more than half a million participants across 34 countries, about 70% of whom show an implicit stereotype associating science with males more than with females (Nosek et al., 2009). What’s more, when the data are grouped by country, national differences in implicit stereotypes predict national differences in the achievement gap between boys and girls in science and math. Our automatic associations, apparently, carry serious societal consequences.
Another nonconscious technique, known as priming , is often used to subtly manipulate behavior by activating or making more accessible certain concepts or beliefs. Consider the fascinating example of terror management theory (TMT) , whose authors believe that human beings are (unconsciously) terrified of their mortality (i.e., the fact that, some day, we will all die; Pyszczynski et al., 2003). According to TMT, in order to cope with this unpleasant reality (and the possibility that our lives are ultimately essentially meaningless), we cling firmly to systems of cultural and religious beliefs that give our lives meaning and purpose. If this hypothesis is correct, one straightforward prediction would be that people should cling even more firmly to their cultural beliefs when they are subtly reminded of their own mortality.
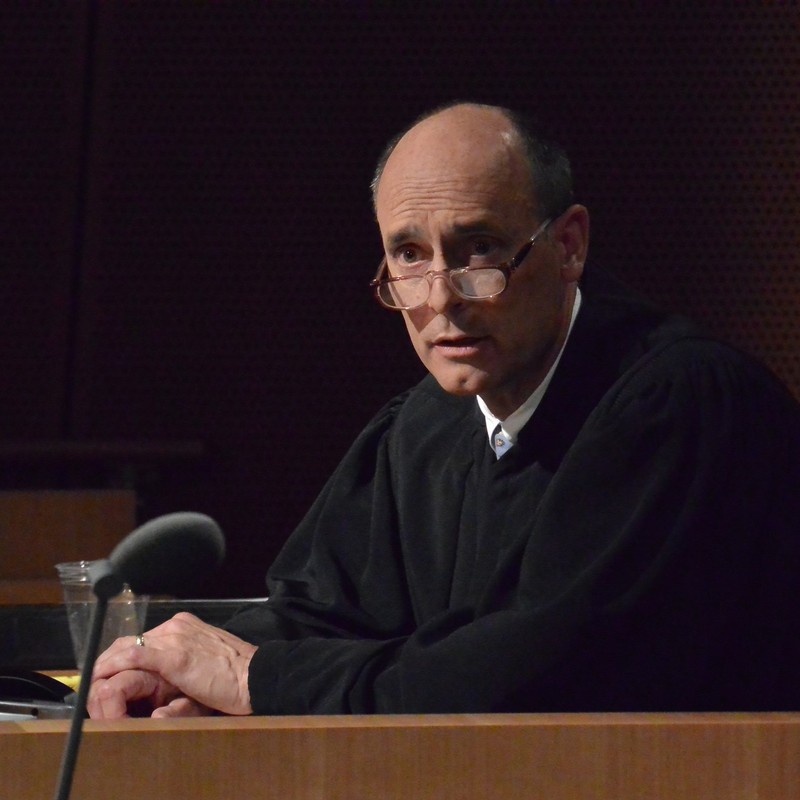
In one of the earliest tests of this hypothesis, actual municipal court judges in Arizona were asked to set a bond for an alleged prostitute immediately after completing a brief questionnaire. For half of the judges the questionnaire ended with questions about their thoughts and feelings regarding the prospect of their own death. Incredibly, judges in the experimental group that were primed with thoughts about their mortality set a significantly higher bond than those in the control group ($455 vs. $50!)—presumably because they were especially motivated to defend their belief system in the face of a violation of the law (Rosenblatt et al., 1989). Although the judges consciously completed the survey, what makes this a study of priming is that the second task (sentencing) was unrelated, so any influence of the survey on their later judgments would have been nonconscious. Similar results have been found in TMT studies in which participants were primed to think about death even more subtly, such as by having them complete questionnaires just before or after they passed a funeral home (Pyszczynski et al., 1996).
To verify that the subtle manipulation (e.g., questions about one’s death) has the intended effect (activating death-related thoughts), priming studies like these often include a manipulation check following the introduction of a prime. For example, right after being primed, participants in a TMT study might be given a word fragment task in which they have to complete words such as COFF_ _ or SK _ _ L. As you might imagine, participants in the mortality-primed experimental group typically complete these fragments as COFFIN and SKULL, whereas participants in the control group complete them as COFFEE and SKILL.
The use of priming to unwittingly influence behavior, known as social or behavioral priming (Ferguson & Mann, 2014), has been at the center of the recent “replication crisis” in Psychology (see the NOBA module on replication). Whereas earlier studies showed, for example, that priming people to think about old age makes them walk slower (Bargh, Chen, & Burrows, 1996), that priming them to think about a university professor boosts performance on a trivia game (Dijksterhuis & van Knippenberg, 1998), and that reminding them of mating motives (e.g., sex) makes them more willing to engage in risky behavior (Greitemeyer, Kastenmüller, & Fischer, 2013), several recent efforts to replicate these findings have failed (e.g., Harris et al., 2013; Shanks et al., 2013). Such failures to replicate findings highlight the need to ensure that both the original studies and replications are carefully designed, have adequate sample sizes, and that researchers pre-register their hypotheses and openly share their results—whether these support the initial hypothesis or not.
Archival Research
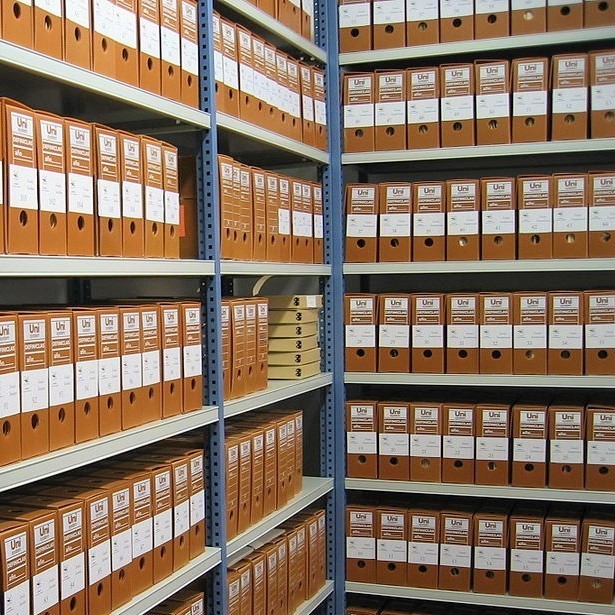
Imagine that a researcher wants to investigate how the presence of passengers in a car affects drivers’ performance. She could ask research participants to respond to questions about their own driving habits. Alternately, she might be able to access police records of the number of speeding tickets issued by automatic camera devices, then count the number of solo drivers versus those with passengers. This would be an example of archival research . The examination of archives, statistics, and other records such as speeches, letters, or even tweets, provides yet another window into social psychology. Although this method is typically used as a type of correlational research design—due to the lack of control over the relevant variables—archival research shares the higher ecological validity of naturalistic observation. That is, the observations are conducted outside the laboratory and represent real world behaviors. Moreover, because the archives being examined can be collected at any time and from many sources, this technique is especially flexible and often involves less expenditure of time and other resources during data collection.
Social psychologists have used archival research to test a wide variety of hypotheses using real-world data. For example, analyses of major league baseball games played during the 1986, 1987, and 1988 seasons showed that baseball pitchers were more likely to hit batters with a pitch on hot days (Reifman et al., 1991). Another study compared records of race-based lynching in the United States between 1882-1930 to the inflation-adjusted price of cotton during that time (a key indicator of the Deep South’s economic health), demonstrating a significant negative correlation between these variables. Simply put, there were significantly more lynchings when the price of cotton stayed flat, and fewer lynchings when the price of cotton rose (Beck & Tolnay, 1990; Hovland & Sears, 1940). This suggests that race-based violence is associated with the health of the economy.
More recently, analyses of social media posts have provided social psychologists with extremely large sets of data (“ big data ”) to test creative hypotheses. In an example of research on attitudes about vaccinations, Mitra and her colleagues (2016) collected over 3 million tweets sent by more than 32 thousand users over four years. Interestingly, they found that those who held (and tweeted) anti-vaccination attitudes were also more likely to tweet about their mistrust of government and beliefs in government conspiracies. Similarly, Eichstaedt and his colleagues (2015) used the language of 826 million tweets to predict community-level mortality rates from heart disease. That’s right: more anger-related words and fewer positive-emotion words in tweets predicted higher rates of heart disease.
In a more controversial example, researchers at Facebook attempted to test whether emotional contagion—the transfer of emotional states from one person to another—would occur if Facebook manipulated the content that showed up in its users’ News Feed (Kramer et al., 2014). And it did. When friends’ posts with positive expressions were concealed, users wrote slightly fewer positive posts (e.g., “Loving my new phone!”). Conversely, when posts with negative expressions were hidden, users wrote slightly fewer negative posts (e.g., “Got to go to work. Ugh.”). This suggests that people’s positivity or negativity can impact their social circles.
The controversial part of this study—which included 689,003 Facebook users and involved the analysis of over 3 million posts made over just one week—was the fact that Facebook did not explicitly request permission from users to participate. Instead, Facebook relied on the fine print in their data-use policy. And, although academic researchers who collaborated with Facebook on this study applied for ethical approval from their institutional review board (IRB), they apparently only did so after data collection was complete, raising further questions about the ethicality of the study and highlighting concerns about the ability of large, profit-driven corporations to subtly manipulate people’s social lives and choices.
Research Issues in Social Psychology
The question of representativeness.
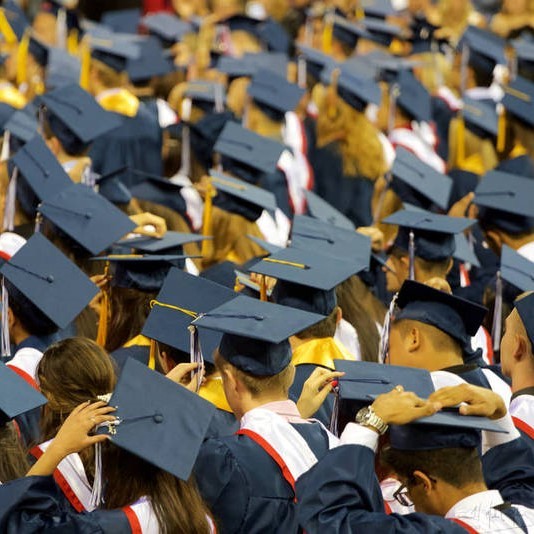
Along with our counterparts in the other areas of psychology, social psychologists have been guilty of largely recruiting samples of convenience from the thin slice of humanity—students—found at universities and colleges (Sears, 1986). This presents a problem when trying to assess the social mechanics of the public at large. Aside from being an overrepresentation of young, middle-class Caucasians, college students may also be more compliant and more susceptible to attitude change, have less stable personality traits and interpersonal relationships, and possess stronger cognitive skills than samples reflecting a wider range of age and experience (Peterson & Merunka, 2014; Visser, Krosnick, & Lavrakas, 2000). Put simply, these traditional samples (college students) may not be sufficiently representative of the broader population. Furthermore, considering that 96% of participants in psychology studies come from western, educated, industrialized, rich, and democratic countries (so-called WEIRD cultures ; Henrich, Heine, & Norenzayan, 2010), and that the majority of these are also psychology students , the question of non-representativeness becomes even more serious.
Of course, when studying a basic cognitive process (like working memory capacity) or an aspect of social behavior that appears to be fairly universal (e.g., even cockroaches exhibit social facilitation!), a non-representative sample may not be a big deal. However, over time research has repeatedly demonstrated the important role that individual differences (e.g., personality traits, cognitive abilities, etc.) and culture (e.g., individualism vs. collectivism) play in shaping social behavior. For instance, even if we only consider a tiny sample of research on aggression, we know that narcissists are more likely to respond to criticism with aggression (Bushman & Baumeister, 1998); conservatives, who have a low tolerance for uncertainty, are more likely to prefer aggressive actions against those considered to be “outsiders” (de Zavala et al., 2010); countries where men hold the bulk of power in society have higher rates of physical aggression directed against female partners (Archer, 2006); and males from the southern part of the United States are more likely to react with aggression following an insult (Cohen et al., 1996).
Ethics in Social Psychological Research
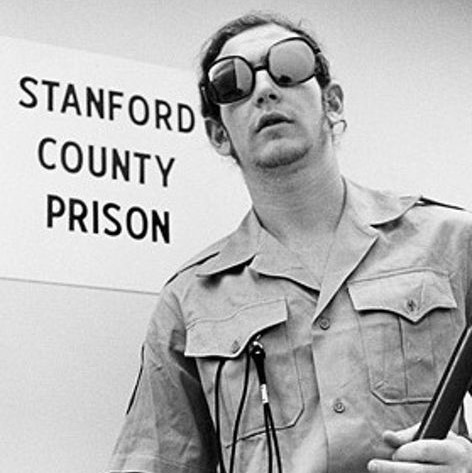
For better or worse (but probably for worse), when we think about the most unethical studies in psychology, we think about social psychology. Imagine, for example, encouraging people to deliver what they believe to be a dangerous electric shock to a stranger (with bloodcurdling screams for added effect!). This is considered a “classic” study in social psychology. Or, how about having students play the role of prison guards, deliberately and sadistically abusing other students in the role of prison inmates. Yep, social psychology too. Of course, both Stanley Milgram’s (1963) experiments on obedience to authority and the Stanford prison study (Haney et al., 1973) would be considered unethical by today’s standards, which have progressed with our understanding of the field. Today, we follow a series of guidelines and receive prior approval from our institutional research boards before beginning such experiments. Among the most important principles are the following:
- Informed consent: In general, people should know when they are involved in research, and understand what will happen to them during the study (at least in general terms that do not give away the hypothesis). They are then given the choice to participate, along with the freedom to withdraw from the study at any time. This is precisely why the Facebook emotional contagion study discussed earlier is considered ethically questionable. Still, it’s important to note that certain kinds of methods—such as naturalistic observation in public spaces, or archival research based on public records—do not require obtaining informed consent.
- Privacy: Although it is permissible to observe people’s actions in public—even without them knowing—researchers cannot violate their privacy by observing them in restrooms or other private spaces without their knowledge and consent. Researchers also may not identify individual participants in their research reports (we typically report only group means and other statistics). With online data collection becoming increasingly popular, researchers also have to be mindful that they follow local data privacy laws, collect only the data that they really need (e.g., avoiding including unnecessary questions in surveys), strictly restrict access to the raw data, and have a plan in place to securely destroy the data after it is no longer needed.
- Risks and Benefits: People who participate in psychological studies should be exposed to risk only if they fully understand the risks and only if the likely benefits clearly outweigh those risks. The Stanford prison study is a notorious example of a failure to meet this obligation. It was planned to run for two weeks but had to be shut down after only six days because of the abuse suffered by the “prison inmates.” But even less extreme cases, such as researchers wishing to investigate implicit prejudice using the IAT, need to be considerate of the consequences of providing feedback to participants about their nonconscious biases. Similarly, any manipulations that could potentially provoke serious emotional reactions (e.g., the culture of honor study described above) or relatively permanent changes in people’s beliefs or behaviors (e.g., attitudes towards recycling) need to be carefully reviewed by the IRB.
- Deception: Social psychologists sometimes need to deceive participants (e.g., using a cover story) to avoid demand characteristics by hiding the true nature of the study. This is typically done to prevent participants from modifying their behavior in unnatural ways, especially in laboratory or field experiments. For example, when Milgram recruited participants for his experiments on obedience to authority, he described it as being a study of the effects of punishment on memory! Deception is typically only permitted (a) when the benefits of the study outweigh the risks, (b) participants are not reasonably expected to be harmed, (c) the research question cannot be answered without the use of deception, and (d) participants are informed about the deception as soon as possible, usually through debriefing.
- Debriefing: This is the process of informing research participants as soon as possible of the purpose of the study, revealing any deceptions, and correcting any misconceptions they might have as a result of participating. Debriefing also involves minimizing harm that might have occurred. For example, an experiment examining the effects of sad moods on charitable behavior might involve inducing a sad mood in participants by having them think sad thoughts, watch a sad video, or listen to sad music. Debriefing would therefore be the time to return participants’ moods to normal by having them think happy thoughts, watch a happy video, or listen to happy music.
As an immensely social species, we affect and influence each other in many ways, particularly through our interactions and cultural expectations, both conscious and nonconscious. The study of social psychology examines much of the business of our everyday lives, including our thoughts, feelings, and behaviors we are unaware or ashamed of. The desire to carefully and precisely study these topics, together with advances in technology, has led to the development of many creative techniques that allow researchers to explore the mechanics of how we relate to one another. Consider this your invitation to join the investigation.
Outside Resources
Discussion questions.
- What are some pros and cons of experimental research, field research, and archival research?
- How would you feel if you learned that you had been a participant in a naturalistic observation study (without explicitly providing your consent)? How would you feel if you learned during a debriefing procedure that you have a stronger association between the concept of violence and members of visible minorities? Can you think of other examples of when following principles of ethical research create challenging situations?
- Can you think of an attitude (other than those related to prejudice) that would be difficult or impossible to measure by asking people directly?
- What do you think is the difference between a manipulation check and a dependent variable?
- Archer, J. (2006). Cross-cultural differences in physical aggression between partners: A social-role analysis. Personality and Social Psychology Review , 10(2), 133-153. doi: 10.1207/s15327957pspr1002_3
- Bargh, J. A., Chen, M., & Burrows, L. (1996). Automaticity of social behavior: Direct effects of trait construct and stereotype activation on action. Journal of Personality and Social Psychology , 71(2), 230-244. http://dx.doi.org/10.1037/0022-3514.71.2.230
- Beck, E. M., & Tolnay, S. E. (1990). The killing fields of the Deep South: The market for cotton and the lynching of Blacks, 1882-1930. American Sociological Review , 55(4), 526-539.
- Bushman, B. J., & Baumeister, R. F. (1998). Threatened egotism, narcissism, self-esteem, and direct and displaced aggression: does self-love or self-hate lead to violence? Journal of Personality and Social Psychology , 75(1), 219-229. http://dx.doi.org/10.1037/0022-3514.75.1.219
- Cialdini, R. B., Borden, R. J., Thorne, A., Walker, M. R., Freeman, S., & Sloan, L. R. (1976). Basking in reflected glory: Three (football) field studies. Journal of Personality and Social Psychology , 34(3), 366-375. http://dx.doi.org/10.1037/0022-3514.34.3.366
- Cohen, D., Nisbett, R. E., Bowdle, B. F. & Schwarz, N. (1996). Insult, aggression, and the southern culture of honor: An "experimental ethnography." Journal of Personality and Social Psychology , 70(5), 945-960. http://dx.doi.org/10.1037/0022-3514.70.5.945
- Diener, E., & Oishi, S. (2000). Money and happiness: Income and subjective well-being across nations. In E. Diener & E. M. Suh (Eds.), Culture and subjective well-being (pp. 185-218). Cambridge, MA: MIT Press.
- Dijksterhuis, A., & van Knippenberg, A. (1998). The relation between perception and behavior, or how to win a game of trivial pursuit. Journal of Personality and Social Psychology , 74(4), 865-877. http://dx.doi.org/10.1037/0022-3514.74.4.865
- Eichstaedt, J. C., Schwartz, H. A., Kern, M. L., Park, G., Labarthe, D. R., Merchant, R. M., & Sap, M. (2015). Psychological language on twitter predicts county-level heart disease mortality. Psychological Science , 26(2), 159–169. doi: 10.1177/0956797614557867
- Ferguson, M. J., & Mann, T. C. (2014). Effects of evaluation: An example of robust “social” priming. Social Cognition , 32, 33-46. doi: 10.1521/soco.2014.32.supp.33
- Gosling, S. D., Vazire, S., Srivastava, S., & John, O. P. (2004). Should we trust web-based studies? A comparative analysis of six preconceptions about internet questionnaires. American Psychologist , 59(2), 93-104. http://dx.doi.org/10.1037/0003-066X.59.2.93
- Greenwald, A. G., McGhee, D. E., & Schwartz, J. L. K. (1998). Measuring individual differences in implicit cognition: The implicit association test. Journal of Personality and Social Psychology , 74(6), 1464-1480. http://dx.doi.org/10.1037/0022-3514.74.6.1464
- Greitemeyer, T., Kastenmüller, A., & Fischer, P. (2013). Romantic motives and risk-taking: An evolutionary approach. Journal of Risk Research , 16, 19-38. doi: 10.1080/13669877.2012.713388
- Haney, C., Banks, C., & Zimbardo, P. (1973). Interpersonal dynamics in a simulated prison. International Journal of Criminology and Penology, 1, 69-97.
- Harris, C. R., Coburn, N., Rohrer, D., & Pashler, H. (2013). Two failures to replicate high-performance-goal priming effects. PLoS ONE , 8(8): e72467. doi:10.1371/journal.pone.0072467
- Henrich, J., Heine, S., & Norenzayan, A. (2010). The weirdest people in the world? Behavioral and Brain Sciences , 33(2-3), 61-83. http://dx.doi.org/10.1017/S0140525X0999152X
- Hovland, C. I., & Sears, R. R. (1940). Minor studies of aggression: VI. Correlation of lynchings with economic indices. The Journal of Psychology , 9(2), 301-310. doi: 10.1080/00223980.1940.9917696
- Isen, A. M., & Levin, P. F. (1972). Effect of feeling good on helping: Cookies and kindness. Journal of Personality and Social Psychology , 21(3), 384-388. http://dx.doi.org/10.1037/h0032317
- Kramer, A. D. I., Guillory, J. E., & Hancock, J. T. (2014). Experimental evidence of massive-scale emotional contagion through social networks. Proceedings of the National Academy of Sciences , 111(24), 8788-8790. doi: 10.1073/pnas.1320040111
- Larson, R. W., Richards, M. H., & Perry-Jenkins, M. (1994). Divergent worlds: the daily emotional experience of mothers and fathers in the domestic and public spheres. Journal of Personality and Social Psychology , 67(6), 1034-1046.
- Milgram, S. (1963). Behavioral study of obedience. Journal of Abnormal and Social Psychology , 67(4), 371–378. doi: 10.1037/h0040525
- Mitra, T., Counts, S., & Pennebaker, J. W. (2016). Understanding anti-vaccination attitudes in social media. Presentation at the Tenth International AAAI Conference on Web and Social Media . Retrieved from comp.social.gatech.edu/papers...cine.mitra.pdf
- Nosek, B. A., Smyth, F. L., Sriram, N., Lindner, N. M., Devos, T., Ayala, A., ... & Kesebir, S. (2009). National differences in gender–science stereotypes predict national sex differences in science and math achievement. Proceedings of the National Academy of Sciences , 106(26), 10593-10597. doi: 10.1073/pnas.0809921106
- Paolacci, G., Chandler, J., & Ipeirotis, P. G. (2010). Running experiments on Amazon Mechanical Turk. Judgment and Decision Making , 51(5), 411-419.
- Peterson, R. A., & Merunka, D. R. (2014). Convenience samples of college students and research reproducibility. Journal of Business Research , 67(5), 1035-1041. doi: 10.1016/j.jbusres.2013.08.010
- Pyszczynski, T., Solomon, S., & Greenberg, J. (2003). In the wake of 9/11: The psychology of terror . Washington, DC: American Psychological Association.
- Pyszczynski, T., Wicklund, R. A., Floresku, S., Koch, H., Gauch, G., Solomon, S., & Greenberg, J. (1996). Whistling in the dark: Exaggerated consensus estimates in response to incidental reminders of mortality. Psychological Science , 7(6), 332-336. doi: 10.111/j.1467-9280.1996.tb00384.x
- Radesky, J. S., Kistin, C. J., Zuckerman, B., Nitzberg, K., Gross, J., Kaplan-Sanoff, M., Augustyn, M., & Silverstein, M. (2014). Patterns of mobile device use by caregivers and children during meals in fast food restaurants. Pediatrics , 133(4), e843-849. doi: 10.1542/peds.2013-3703
- Reifman, A. S., Larrick, R. P., & Fein, S. (1991). Temper and temperature on the diamond: The heat-aggression relationship in major league baseball. Personality and Social Psychology Bulletin , 17(5), 580-585. http://dx.doi.org/10.1177/0146167291175013
- Rosenblatt, A., Greenberg, J., Solomon, S., Pyszczynski. T, & Lyon, D. (1989). Evidence for terror management theory I: The effects of mortality salience on reactions to those who violate or uphold cultural values. Journal of Personality and Social Psychology , 57(4), 681-690. http://dx.doi.org/10.1037/0022-3514.57.4.681
- Sears, D. O. (1986). College sophomores in the laboratory: Influences of a narrow data base on social psychology’s view of human nature. Journal of Personality and Social Psychology , 51(3), 515-530. http://dx.doi.org/10.1037/0022-3514.51.3.515
- Shanks, D. R., Newell, B. R., Lee, E. H., Balakrishnan, D., Ekelund L., Cenac Z., … Moore, C. (2013). Priming intelligent behavior: An elusive phenomenon. PLoS ONE , 8(4): e56515. doi:10.1371/journal.pone.0056515
- Stavrova, O., & Ehlebracht, D. (2016). Cynical beliefs about human nature and income: Longitudinal and cross-cultural analyses. Journal of Personality and Social Psychology , 110(1), 116-132. http://dx.doi.org/10.1037/pspp0000050
- Stroebe, W. (2012). The truth about Triplett (1898), but nobody seems to care. Perspectives on Psychological Science , 7(1), 54-57. doi: 10.1177/1745691611427306
- Triplett, N. (1898). The dynamogenic factors in pacemaking and competition. American Journal of Psychology , 9, 507-533.
- Visser, P. S., Krosnick, J. A., & Lavrakas, P. (2000). Survey research. In H. T. Reis & C. M. Judd (Eds.), Handbook of research methods in social psychology (pp. 223-252). New York: Cambridge University Press.
- de Zavala, A. G., Cislak, A., & Wesolowska, E. (2010). Political conservatism, need for cognitive closure, and intergroup hostility. Political Psychology , 31(4), 521-541. doi: 10.1111/j.1467-9221.2010.00767.x
Experimental Method In Psychology
Saul Mcleod, PhD
Editor-in-Chief for Simply Psychology
BSc (Hons) Psychology, MRes, PhD, University of Manchester
Saul Mcleod, PhD., is a qualified psychology teacher with over 18 years of experience in further and higher education. He has been published in peer-reviewed journals, including the Journal of Clinical Psychology.
Learn about our Editorial Process
Olivia Guy-Evans, MSc
Associate Editor for Simply Psychology
BSc (Hons) Psychology, MSc Psychology of Education
Olivia Guy-Evans is a writer and associate editor for Simply Psychology. She has previously worked in healthcare and educational sectors.
On This Page:
The experimental method involves the manipulation of variables to establish cause-and-effect relationships. The key features are controlled methods and the random allocation of participants into controlled and experimental groups .
What is an Experiment?
An experiment is an investigation in which a hypothesis is scientifically tested. An independent variable (the cause) is manipulated in an experiment, and the dependent variable (the effect) is measured; any extraneous variables are controlled.
An advantage is that experiments should be objective. The researcher’s views and opinions should not affect a study’s results. This is good as it makes the data more valid and less biased.
There are three types of experiments you need to know:
1. Lab Experiment
A laboratory experiment in psychology is a research method in which the experimenter manipulates one or more independent variables and measures the effects on the dependent variable under controlled conditions.
A laboratory experiment is conducted under highly controlled conditions (not necessarily a laboratory) where accurate measurements are possible.
The researcher uses a standardized procedure to determine where the experiment will take place, at what time, with which participants, and in what circumstances.
Participants are randomly allocated to each independent variable group.
Examples are Milgram’s experiment on obedience and Loftus and Palmer’s car crash study .
- Strength : It is easier to replicate (i.e., copy) a laboratory experiment. This is because a standardized procedure is used.
- Strength : They allow for precise control of extraneous and independent variables. This allows a cause-and-effect relationship to be established.
- Limitation : The artificiality of the setting may produce unnatural behavior that does not reflect real life, i.e., low ecological validity. This means it would not be possible to generalize the findings to a real-life setting.
- Limitation : Demand characteristics or experimenter effects may bias the results and become confounding variables .
2. Field Experiment
A field experiment is a research method in psychology that takes place in a natural, real-world setting. It is similar to a laboratory experiment in that the experimenter manipulates one or more independent variables and measures the effects on the dependent variable.
However, in a field experiment, the participants are unaware they are being studied, and the experimenter has less control over the extraneous variables .
Field experiments are often used to study social phenomena, such as altruism, obedience, and persuasion. They are also used to test the effectiveness of interventions in real-world settings, such as educational programs and public health campaigns.
An example is Holfing’s hospital study on obedience .
- Strength : behavior in a field experiment is more likely to reflect real life because of its natural setting, i.e., higher ecological validity than a lab experiment.
- Strength : Demand characteristics are less likely to affect the results, as participants may not know they are being studied. This occurs when the study is covert.
- Limitation : There is less control over extraneous variables that might bias the results. This makes it difficult for another researcher to replicate the study in exactly the same way.
3. Natural Experiment
A natural experiment in psychology is a research method in which the experimenter observes the effects of a naturally occurring event or situation on the dependent variable without manipulating any variables.
Natural experiments are conducted in the day (i.e., real life) environment of the participants, but here, the experimenter has no control over the independent variable as it occurs naturally in real life.
Natural experiments are often used to study psychological phenomena that would be difficult or unethical to study in a laboratory setting, such as the effects of natural disasters, policy changes, or social movements.
For example, Hodges and Tizard’s attachment research (1989) compared the long-term development of children who have been adopted, fostered, or returned to their mothers with a control group of children who had spent all their lives in their biological families.
Here is a fictional example of a natural experiment in psychology:
Researchers might compare academic achievement rates among students born before and after a major policy change that increased funding for education.
In this case, the independent variable is the timing of the policy change, and the dependent variable is academic achievement. The researchers would not be able to manipulate the independent variable, but they could observe its effects on the dependent variable.
- Strength : behavior in a natural experiment is more likely to reflect real life because of its natural setting, i.e., very high ecological validity.
- Strength : Demand characteristics are less likely to affect the results, as participants may not know they are being studied.
- Strength : It can be used in situations in which it would be ethically unacceptable to manipulate the independent variable, e.g., researching stress .
- Limitation : They may be more expensive and time-consuming than lab experiments.
- Limitation : There is no control over extraneous variables that might bias the results. This makes it difficult for another researcher to replicate the study in exactly the same way.
Key Terminology
Ecological validity.
The degree to which an investigation represents real-life experiences.
Experimenter effects
These are the ways that the experimenter can accidentally influence the participant through their appearance or behavior.
Demand characteristics
The clues in an experiment lead the participants to think they know what the researcher is looking for (e.g., the experimenter’s body language).
Independent variable (IV)
The variable the experimenter manipulates (i.e., changes) is assumed to have a direct effect on the dependent variable.
Dependent variable (DV)
Variable the experimenter measures. This is the outcome (i.e., the result) of a study.
Extraneous variables (EV)
All variables which are not independent variables but could affect the results (DV) of the experiment. EVs should be controlled where possible.
Confounding variables
Variable(s) that have affected the results (DV), apart from the IV. A confounding variable could be an extraneous variable that has not been controlled.
Random Allocation
Randomly allocating participants to independent variable conditions means that all participants should have an equal chance of participating in each condition.
The principle of random allocation is to avoid bias in how the experiment is carried out and limit the effects of participant variables.
Order effects
Changes in participants’ performance due to their repeating the same or similar test more than once. Examples of order effects include:
(i) practice effect: an improvement in performance on a task due to repetition, for example, because of familiarity with the task;
(ii) fatigue effect: a decrease in performance of a task due to repetition, for example, because of boredom or tiredness.

Related Articles
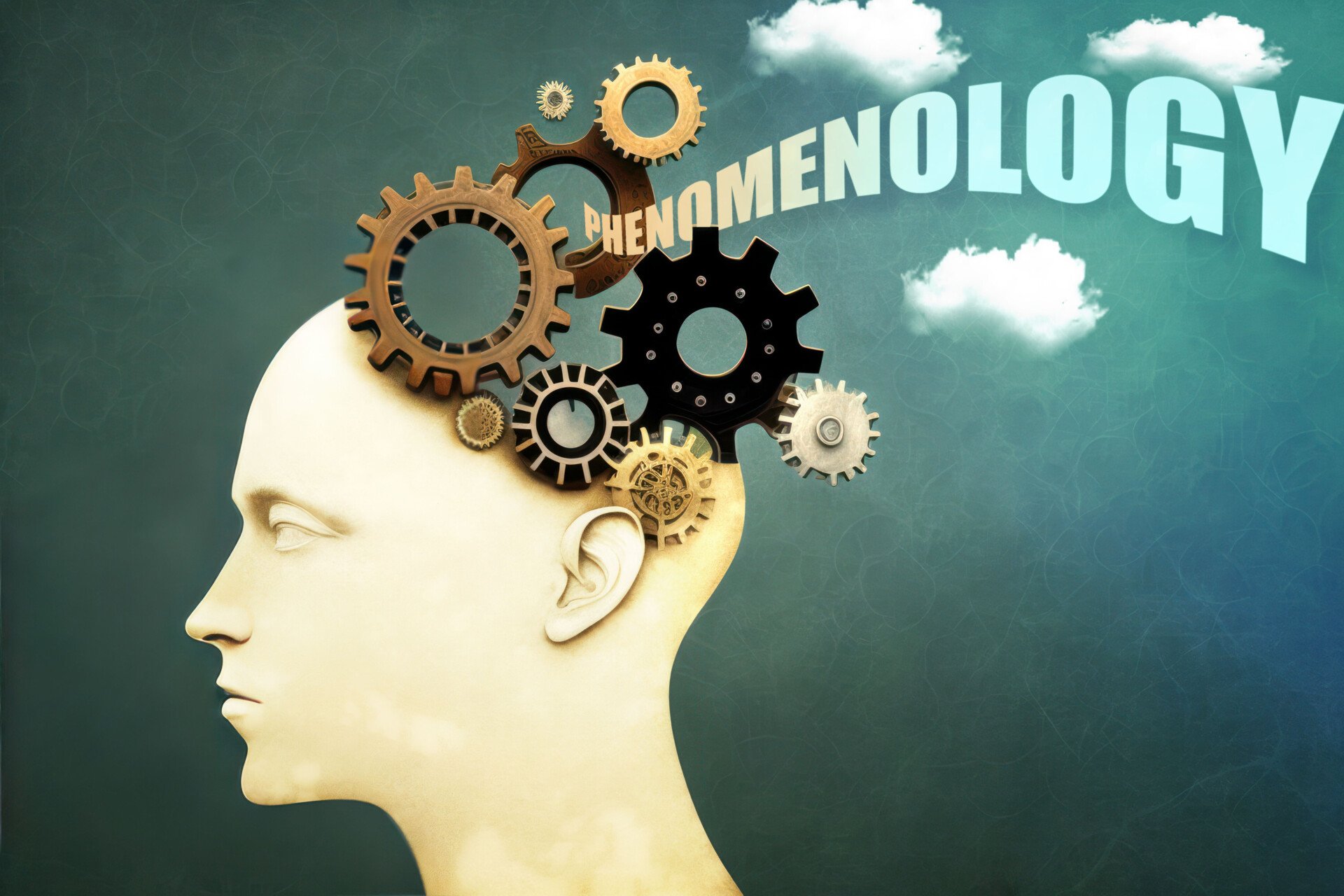
Research Methodology
Phenomenology In Qualitative Research
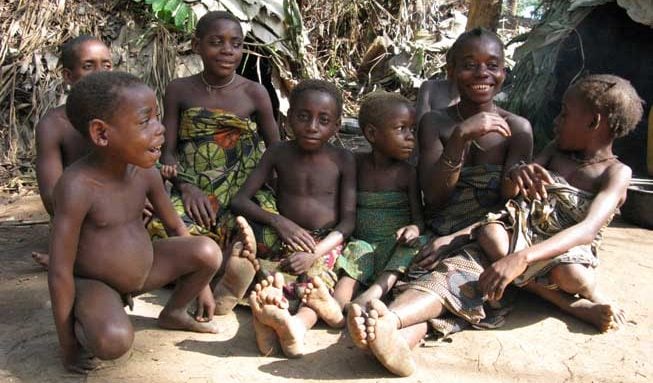
Ethnography In Qualitative Research
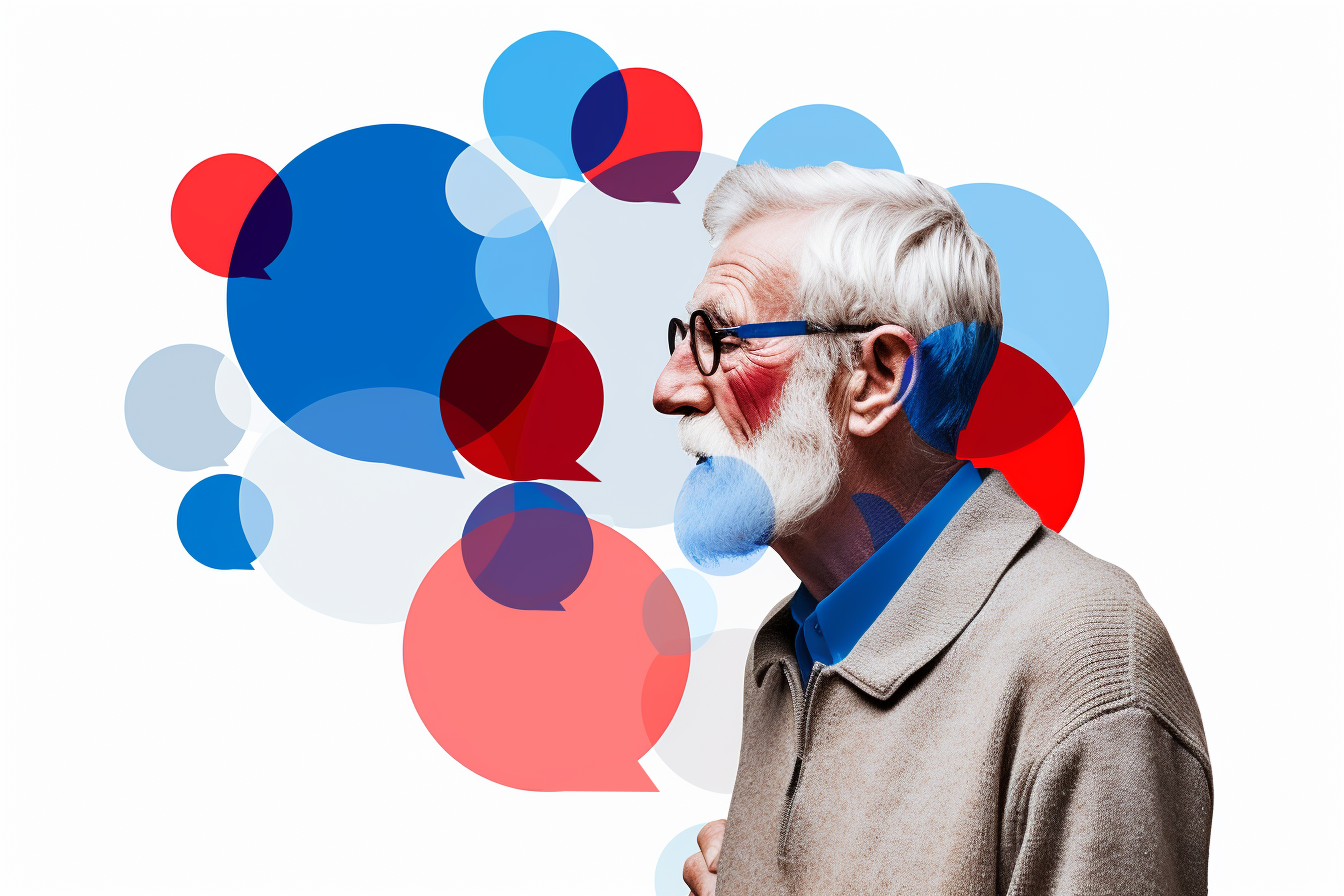
Narrative Analysis In Qualitative Research
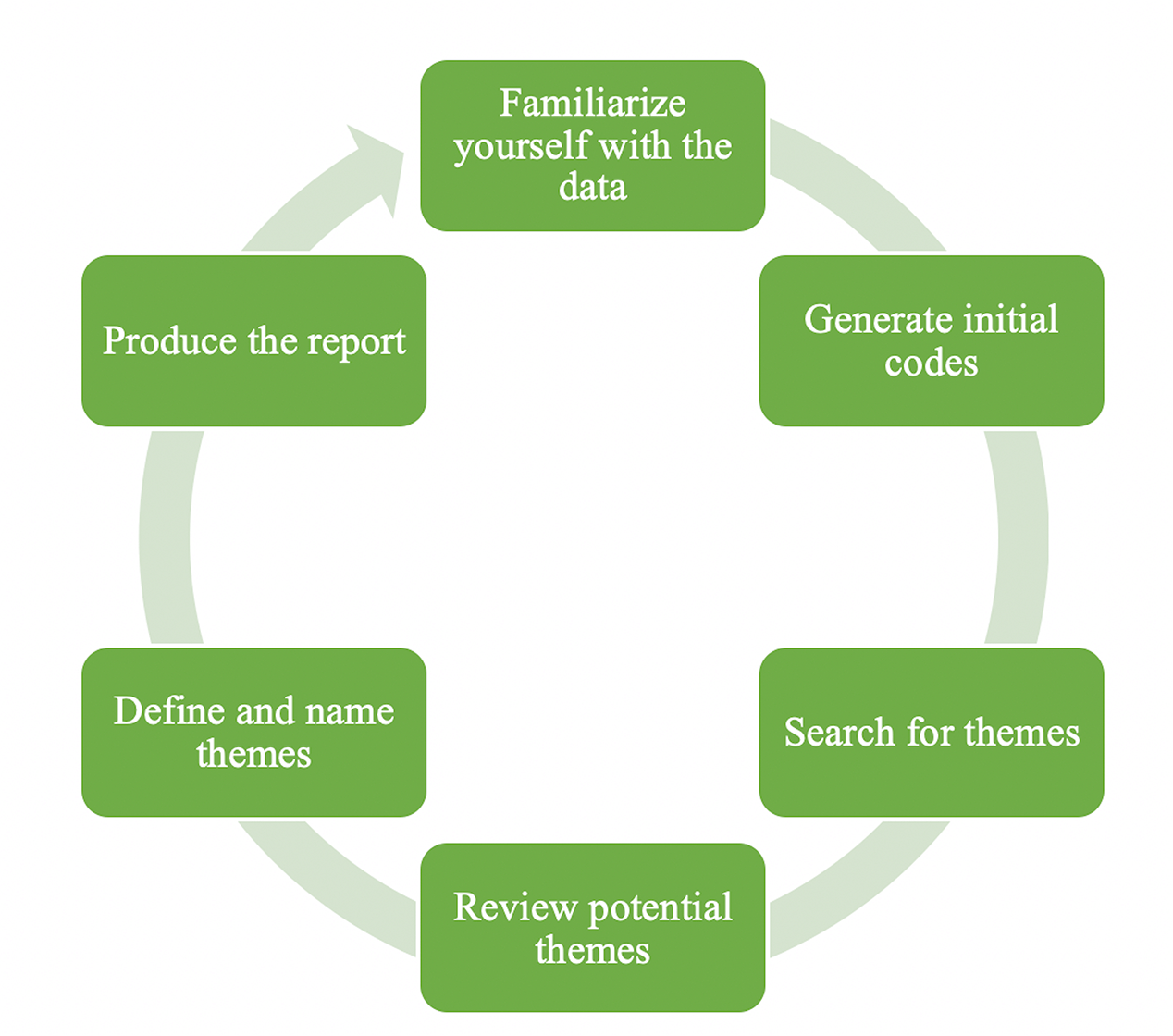
Thematic Analysis: A Step by Step Guide
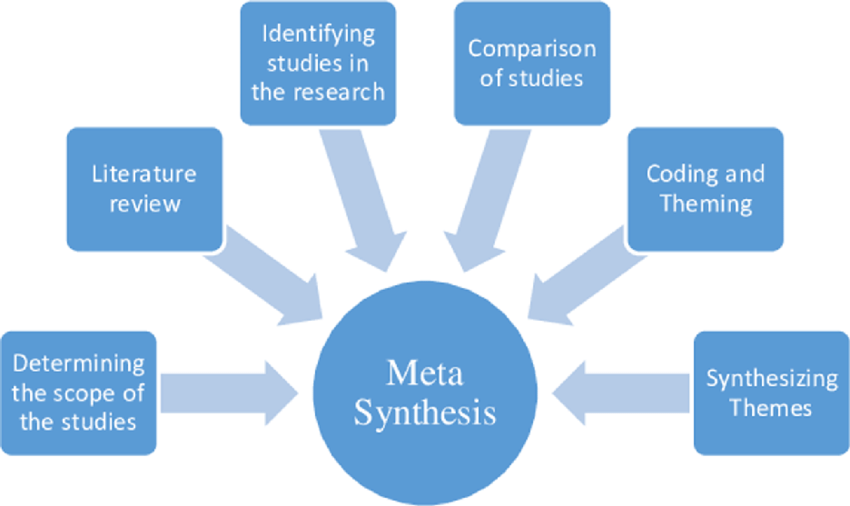
Metasynthesis Of Qualitative Research
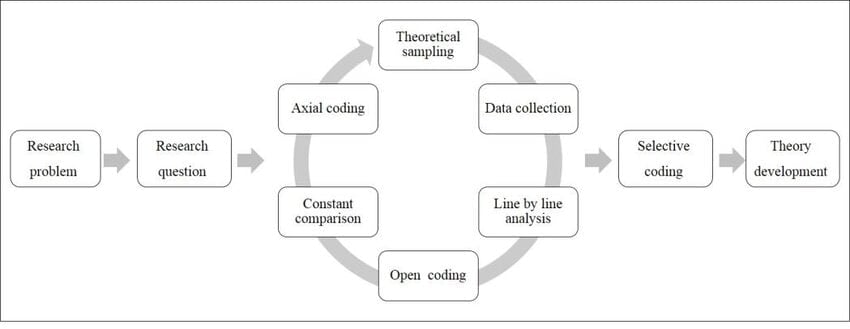
Grounded Theory In Qualitative Research: A Practical Guide
10 Things to Know About Survey Experiments
Survey experiments are widely used by social scientists to study individual preferences. This guide discusses the functions and considerations of survey experiments.
1 What is a survey experiment
A survey experiment is an experiment conducted within a survey. In an experiment, a researcher randomly assigns participants to at least two experimental conditions. The researcher then treats each condition differently. Because of random assignment, any differences between the experimental conditions would result from the treatment. In a survey experiment, the randomization and treatment occur within a questionnaire.
2 Why do a survey experiment
Survey experiments are useful when researchers want to learn about individual perceptions, attitudes, or behaviors. They are especially useful when a regular survey, without experimentation, may generate biased or even nonsensical responses. For example, if researchers are interested in studying the effects of policy information on individual preferences for a policy, directly asking each survey respondent “how does this information affect your attitudes toward the policy?” may raise concerns about the accuracy and truthfulness of the responses. Rather, researchers may find it useful to provide the policy information to a randomized subset of respondents, followed by comparing the policy preferences between those who are subject to the policy information and those who are not.
More generally, survey experiments help to measure individual preferences. For example, when the preferences of interest are multidimensional, regular surveys may not be able to reliably measure such complex preferences through individual self-reports. Other preferences, such as racist attitudes and illegal behaviors, may be sensitive — preferences with which respondents do not want to be publicly associated. Direct questioning techniques may thus understate the prevalence of these preferences. In these cases, survey experiments, compared to regular surveys, can be useful to address these measurement challenges.
There are various types of survey experiments. Five of them — conjoint experiments, priming experiments, endorsement experiments, list experiments, and randomized response — are covered in the following sections.
3 Conjoint experiments
Conjoint experiments are useful when researchers aim to measure multidimensional preferences (i.e., preferences that are characterized by more than one attribute). In a typical conjoint experiment, researchers repeatedly ask respondents to choose between two distinct options and randomly vary the characteristics of these two options. 1 Researchers may also ask respondents to rate each option on a scale. In both cases, respondents express their preferences toward a large number of pairings with randomized attributes.
Hainmueller, Hopkins, and Yamamoto ( 2014 ) demonstrate the use of conjoint experiments in a study about support for immigration. The authors showed respondents two immigrant profiles and asked (a) which immigrant the respondent would prefer be admitted to the Unites States and (b) how the respondent rated each immigrant on a scale from 1-7. The authors randomly varied nine attributes of the immigrants (gender, education, employment plans, job experience, profession, language skills, country of origin, reasons for applying, and prior trips to the United States), yielding thousands of unique immigrant profiles. This process was repeated five times so that each respondents saw and rated five pairs of immigrants. Through this procedure, the authors assessed how these randomly varied components influence support for the immigrant.
Respondents saw:

The conjoint experiment thus allows us to measure how multiple immigrant characteristics, such as their gender or country of origin, shape respondents’ attitudes toward the immigrants. Another advantage of this survey experiment, compared to a non-experimental survey, is that it preempts the need for respondents to directly express sensitive preferences; instead, they indirectly reveal their preferences. For example, while respondents who hold sexist attitudes may be less willing to openly express preferences for male immigrants due to social desirability bias, they may find it more comfortable to choose — and therefore reveal their preferences for — male immigrant profiles in this less direct setting. 2 Given these advantages, the use of conjoint experiments is not confined to the measurement of immigrant preferences; researchers have also applied conjoint experiments to study other multidimensional preferences, such as candidate choice and policy packages.
4 Priming experiments
In a priming experiment, researchers expose respondents in the treatment group to a stimulus representing topic X in order to influence their considerations at the top of their head when responding to a survey question about topic Y . The control group, however, is not exposed to the stimulus. Therefore, the difference in expressed preferences regarding Y between the treatment and control groups is due to exposure to the treatment stimulus.
Priming experiments are a broad class and include any experiment that makes a specific topic salient in the mind of the respondent. One common method of priming is the use of images. For example, Brader, Valentino, and Suhay ( 2008 ) used images as a priming instrument to estimate the role of race in shaping immigration preferences. The researchers showed subjects a positive or negative news article about immigration paired with a picture of a European immigrant or an Hispanic immigrant. Subjects expressed negative attitudes about immigration when the negative news article was paired with the Hispanic immigrant picture but not in other conditions. The picture primed people to think about Hispanic immigrants, and thinking about Hispanic immigrants reduced support for immigration compared to thinking about European immigrants.
More broadly, priming experiments can be useful when researchers are interested in learning about the influence of context. By making a specific context of interest salient to a randomized subset of respondents, researchers can gauge the impact of this primed context on the measured outcome of interest.
5 Endorsement experiments
Endorsement experiments measure attitudes toward a sensitive object, usually a controversial political actor or group. In a typical endorsement experiment, respondents are asked how much they support a policy. In the treatment condition, the policy is said to be endorsed by an actor or a group. In the control condition, however, this endorsement information is omitted. The average difference in support between the endorsed and unendorsed policy represents the change in support for the policy because of the endorsement of the controversial figure.
For example, Nicholson ( 2012 ) used an endorsement experiment to study partisan bias in the United States during the 2008 Presidential campaign. The researchers asked respondents about policies, varying whether the policy was endorsed by the Presidential candidates of the two main political parties, Barack Obama (Democrat) and John McCain (Republican). Respondents were told:
As you know, there has been a lot of talk about immigration reform policy in the news. One proposal [ backed by Barack Obama / backed by John McCain ] provided legal status and a path to legal citizenship for the approximately 12 million illegal immigrants currently residing in the United States. What is your view of this immigration reform policy?
On one hand, the difference between the control condition and the Obama (McCain) condition for Democrats (Republicans) indicates in-party bias. On the other, the difference between the control condition and the Obama (McCain) condition for Republicans (Democrats) indicates out-party bias. This experiment helps researchers gauge the favorability toward the potentially sensitive item (i.e., the political actor), as other well-designed endorsement experiments also do. Because endorsement experiments preempt the need for respondents to self-report their support for a controversial object, they are especially useful in politically sensitive contexts. For example, they have been used to measure public support for militant groups (e.g., Bullock, Imai, and Shapiro ( 2011 ) ; Lyall, Blair, and Imai ( 2013 ) ).
6 List Experiments
List experiments (also known as the item count technique) measure a sensitive attitude or behavior when researchers expect respondents to falsify it if it is solicited using a direct question. For example, respondents may be reluctant to admit that they hold racially conservative views ( Kuklinski et al. 1997 ) or engage in illegal behaviors ( García-Sánchez and Queirolo 2021 ) even after being assured of the survey’s anonymity.
In a list experiment, the researcher randomly assigns respondents to a control or treatment condition. The control condition presents respondents with a list of items; the treatment condition presents respondents with the same list plus a treatment item measuring the attitude or behavior of interest. Respondents are then asked how many of these items apply to them. The average difference between the treatment and control conditions represents the percentage of respondents for whom the treatment item applies. A list experiment does not tell the researcher about the attitude or behavior of any individual respondent, but it tells her about the prevalence of the sensitive attitude in her sample population. Answers to this question are anonymous because the respondent’s attitude toward each item cannot be determined unless the respondent answers that all or none of the items apply to them.
For example, Kuklinski et al. ( 1997 ) studied racial animus with a list experiment. They told respondents:
Now I am going to read you three things that sometimes make people angry or upset. After I read all three, just tell me HOW MANY of them upset you. I don’t want to know which ones, just HOW MANY. (1) the federal government increasing the tax on gasoline (2) professional athletes getting million-dollar contracts (3) large corporations polluting the environment (4) a black family moving in next door
In the above example, the fourth item was withheld from the control condition. The authors found that the mean number of items chosen in the treatment group was 2.37, compared to 1.95 in the control group. The difference of 0.42 between treatment and control suggests that 42% of respondents would be upset by a black family moving in next door.
Despite the anonymity provided by a list experiment, respondents may still worry that their response reflects their attitudes about the sensitive item. When respondents worry about a lack of anonymity, they may increase or decrease their response to portray themselves in the best light possible, rather than answer honestly ( Leary and Kowalski 1990 ) . Given this limitation, researchers have developed other types of list experiments, including double list experiments and placebo-controlled list experiments . Interested readers may consult Glynn ( 2013 ) and Riambau and Ostwald ( 2019 ) for detailed discussions about their implementation, as well as how they help to overcome some of the potential pitfalls of simple list experiments.
7 Randomized Response
The randomized response technique is also used to measure a sensitive attitude or behavior when the researcher expects respondents to lie about it if asked a direct question. 3 In the most common version of the randomized response technique, respondents are directly asked a yes or no question about a sensitive topic. The respondent is also given some randomization device, such as a coin or die. The respondent is told to answer the question when the randomization device takes on a certain value (e.g., tails) or to simply say “yes” when the randomization device takes a different value (e.g., heads). Researchers assume that respondents will believe their anonymity is protected because the researcher cannot know whether a “yes” resulted from agreement with the sensitive item or the randomization device.
For example, Blair, Imai, and Zhou ( 2015 ) studied support for militants in Nigeria with the randomized response technique. They gave respondents a die and had the respondent practice throwing it. They then told respondents:
For this question, I want you to answer yes or no. But I want you to consider the number of your dice throw. If 1 shows on the dice, tell me no. If 6 shows, tell me yes. But if another number, like 2 or 3 or 4 or 5 shows, tell me your opinion about the question that I will ask you after you throw the dice. [ENUMERATOR TURN AWAY FROM THE RESPONDENT] Now throw the dice so that I cannot see what comes out. Please do not forget the number that comes out. [ENUMERATOR WAIT TO TURN AROUND UNTIL RESPONDENT SAYS YES TO]: Have you thrown the dice? Have you picked it up? Now, during the height of the conflict in 2007 and 2008, did you know any militants, like a family member, a friend, or someone you talked to on a regular basis? Please, before you answer, take note of the number you rolled on the dice.
In expectation, one-sixth of respondents answer “yes” due to the die throw. The researcher can thus determine what percentage of respondents engaged in the sensitive behavior. Here, however, respondents might not feel that their answers to randomized response questions were truly anonymous. This is because if a respondent answered yes, the answer could have been dictated by the randomization device, but it could also signal agreement with the sensitive item. 4 Indeed, there are other types of randomized response techniques that address this limitation, including the repeated randomized response technique and the crosswise model . We refer interested readers to Azfar and Murrell ( 2009 ) and Jann, Jerke, and Krumpal ( 2011 ) for the logic and implementation of these techniques.
8 Implementation
To implement survey experiments, researchers need to write up multiple versions of a survey: at least one for the control condition(s) and at least one for the treatment condition(s). Then, researchers need a randomization device that allows them to randomize the survey version shown to the respondents. There are many platforms that facilitate the implementation of survey experiments, with Qualtrics being one of the most popular tools among survey researchers.
While the treatment is typically imposed through text, the treatment stimulus can also be of other forms, including images and videos. The key is to map the treatment directly onto the theoretical variable of interest. That is, if the researcher is interested in studying the effect of X on Y , the text, image, or video (or any of their combination) should induce a change in X and not in other confounding variables. 5 Visual aids, if carefully provided, can be helpful in different settings. For example, researchers have used photos as experimental stimuli to investigate the impact of candidate appearance on vote choice ( Douglas et al. 2017 ) and the effects of gender and racial diversity in shaping the legitimacy of international organizations ( Chow and Han 2023 ) .
9 Considerations
Survey experiments can be an effective tool for researchers to measure sensitive attitudes and learn about causal relationships. Not only can they be done quickly and iteratively, but they may also be included on mass online surveys because they do not require in-person contact to implement. This means that a researcher can plan a sequence of online survey experiments, changing the intervention and measured outcomes from one experiment to the next to learn about the mechanisms behind the treatment effect very quickly ( Sniderman 2018 ) .
But researchers need to be careful about survey satisficing , which occurs when respondents put in minimal effort to understand and answer a survey question. 6 In the presence of satisficing behavior, the treatment embedded in the survey experiments may not be received by respondents as intended. As such, the measured preferences will be unreliable. Given this concern, researchers should always design survey experiments that are easy to understand. The length and complexity of the survey and experimental stimuli should also be kept at a minimum level, whenever possible. A related consideration is respondent attentiveness , an issue that is extensively discussed by Alvarez et al. ( 2019 ) .
Researchers also need to consider the strength of their treatment. Sometimes the experimental stimulus is unable to generate a meaningful change in the subsequently measured attitude or behavior not because the treatment is unrelated to the outcome variable of interest, but because the treatment itself is too weak. For example, for an information provision experiment where the experimental stimulus is some factual information related to topic Y , the treatment may fail to change views on Y not because the information plays no role in shaping individual attitudes toward Y , but because respondents have already been exposed to this information in the real world. 7 More generally, researchers need to watch out for preatreatment effects ( Druckman and Leeper 2012 ) . If respondents, before participating in the survey experiment, have already encountered the experimental stimulus, there may be no measured difference between treatment and control groups because all respondents were “pretreated” with the stimulus, including those in the control group.
When designing survey experiments, researchers should pay attention to the question wording and ordering . Some terms, for example, may be unfamiliar to certain respondents or interpreted by different respondents in different ways. As such, measurement invariance may set in, such that the same construct is measured differently for different groups of individuals. In other cases, the question ordering itself may bias how individuals provide their responses ( Gaines, Kuklinski, and Quirk 2007 ) . These considerations are all important to bear in mind when researchers design their survey experiments, since they fundamentally shape the inferences one can draw from the data.
10 Limitations
While survey experiments offer a fruitful way to measure individual preferences, researchers are often more concerned about real-world outcomes. When preferences are measured — and treatments are delivered — in a survey setting, there is no guarantee that the survey-experimental findings will translate into the real world. Therefore, researchers should be cautious when they extrapolate from survey experiments ( Barabas and Jerit 2010 ) . For more discussions on the strengths and limitations of survey experiments, see:
- Mutz ( 2011 ) “Population-Based Survey Experiments.”
- Sniderman ( 2018 ) “Some Advances in the Design of Survey Experiments” in the Annual Review of Political Science .
- Lavrakas et al. ( 2019 ) “Experimental Methods in Survey Research: Techniques that Combine Random Sampling with Random Assignment.”
- Diaz, Grady, and Kuklinski ( 2020 ) “Survey Experiments and the Quest for Valid Interpretation” in the Sage Handbook of Research Methods in Political Science and International Relations .
11 References
See Hainmueller, Hopkins, and Yamamoto ( 2014 ) and Green and Rao ( 1971 ) . ↩︎
See Horiuchi, Markovich, and Yamamoto ( 2022 ) . ↩︎
See Warner ( 1965 ) , Boruch ( 1971 ) , D. Gingerich ( 2015 ) , and D. W. Gingerich ( 2010 ) . ↩︎
See Edgell, Himmelfarb, and Duchan ( 1982 ) and Yu, Tian, and Tang ( 2008 ) . ↩︎
See Dafoe, Zhang, and Caughey ( 2018 ) on information equivalence. ↩︎
See Krosnick ( 1991 ) and Simon and March ( 2006 ) . ↩︎
See Haaland, Roth, and Johannes ( 2023 ) for a review of information provision experiments. ↩︎
Have a language expert improve your writing
Run a free plagiarism check in 10 minutes, generate accurate citations for free.
- Knowledge Base
Methodology
- Guide to Experimental Design | Overview, Steps, & Examples
Guide to Experimental Design | Overview, 5 steps & Examples
Published on December 3, 2019 by Rebecca Bevans . Revised on June 21, 2023.
Experiments are used to study causal relationships . You manipulate one or more independent variables and measure their effect on one or more dependent variables.
Experimental design create a set of procedures to systematically test a hypothesis . A good experimental design requires a strong understanding of the system you are studying.
There are five key steps in designing an experiment:
- Consider your variables and how they are related
- Write a specific, testable hypothesis
- Design experimental treatments to manipulate your independent variable
- Assign subjects to groups, either between-subjects or within-subjects
- Plan how you will measure your dependent variable
For valid conclusions, you also need to select a representative sample and control any extraneous variables that might influence your results. If random assignment of participants to control and treatment groups is impossible, unethical, or highly difficult, consider an observational study instead. This minimizes several types of research bias, particularly sampling bias , survivorship bias , and attrition bias as time passes.
Table of contents
Step 1: define your variables, step 2: write your hypothesis, step 3: design your experimental treatments, step 4: assign your subjects to treatment groups, step 5: measure your dependent variable, other interesting articles, frequently asked questions about experiments.
You should begin with a specific research question . We will work with two research question examples, one from health sciences and one from ecology:
To translate your research question into an experimental hypothesis, you need to define the main variables and make predictions about how they are related.
Start by simply listing the independent and dependent variables .
Research question | Independent variable | Dependent variable |
---|---|---|
Phone use and sleep | Minutes of phone use before sleep | Hours of sleep per night |
Temperature and soil respiration | Air temperature just above the soil surface | CO2 respired from soil |
Then you need to think about possible extraneous and confounding variables and consider how you might control them in your experiment.
Extraneous variable | How to control | |
---|---|---|
Phone use and sleep | in sleep patterns among individuals. | measure the average difference between sleep with phone use and sleep without phone use rather than the average amount of sleep per treatment group. |
Temperature and soil respiration | also affects respiration, and moisture can decrease with increasing temperature. | monitor soil moisture and add water to make sure that soil moisture is consistent across all treatment plots. |
Finally, you can put these variables together into a diagram. Use arrows to show the possible relationships between variables and include signs to show the expected direction of the relationships.
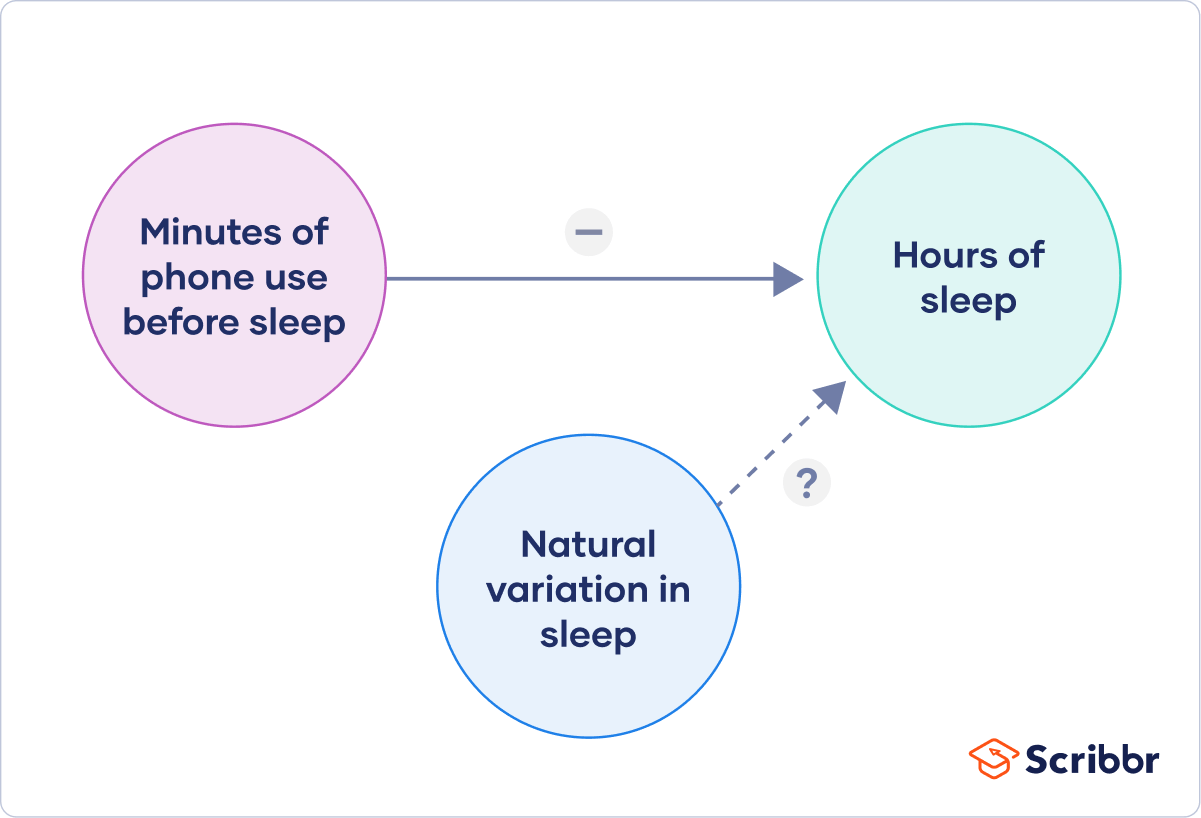
Here we predict that increasing temperature will increase soil respiration and decrease soil moisture, while decreasing soil moisture will lead to decreased soil respiration.
Receive feedback on language, structure, and formatting
Professional editors proofread and edit your paper by focusing on:
- Academic style
- Vague sentences
- Style consistency
See an example
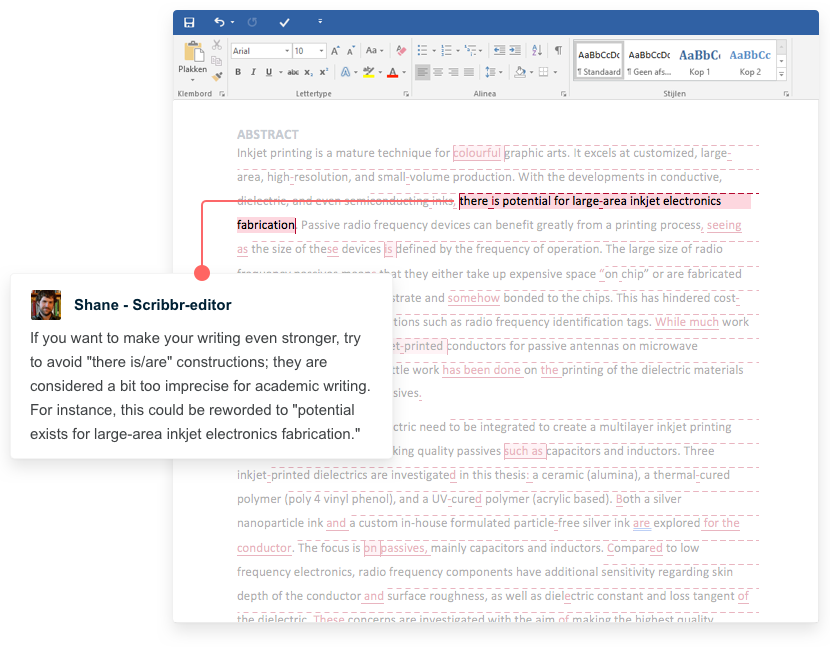
Now that you have a strong conceptual understanding of the system you are studying, you should be able to write a specific, testable hypothesis that addresses your research question.
Null hypothesis (H ) | Alternate hypothesis (H ) | |
---|---|---|
Phone use and sleep | Phone use before sleep does not correlate with the amount of sleep a person gets. | Increasing phone use before sleep leads to a decrease in sleep. |
Temperature and soil respiration | Air temperature does not correlate with soil respiration. | Increased air temperature leads to increased soil respiration. |
The next steps will describe how to design a controlled experiment . In a controlled experiment, you must be able to:
- Systematically and precisely manipulate the independent variable(s).
- Precisely measure the dependent variable(s).
- Control any potential confounding variables.
If your study system doesn’t match these criteria, there are other types of research you can use to answer your research question.
How you manipulate the independent variable can affect the experiment’s external validity – that is, the extent to which the results can be generalized and applied to the broader world.
First, you may need to decide how widely to vary your independent variable.
- just slightly above the natural range for your study region.
- over a wider range of temperatures to mimic future warming.
- over an extreme range that is beyond any possible natural variation.
Second, you may need to choose how finely to vary your independent variable. Sometimes this choice is made for you by your experimental system, but often you will need to decide, and this will affect how much you can infer from your results.
- a categorical variable : either as binary (yes/no) or as levels of a factor (no phone use, low phone use, high phone use).
- a continuous variable (minutes of phone use measured every night).
How you apply your experimental treatments to your test subjects is crucial for obtaining valid and reliable results.
First, you need to consider the study size : how many individuals will be included in the experiment? In general, the more subjects you include, the greater your experiment’s statistical power , which determines how much confidence you can have in your results.
Then you need to randomly assign your subjects to treatment groups . Each group receives a different level of the treatment (e.g. no phone use, low phone use, high phone use).
You should also include a control group , which receives no treatment. The control group tells us what would have happened to your test subjects without any experimental intervention.
When assigning your subjects to groups, there are two main choices you need to make:
- A completely randomized design vs a randomized block design .
- A between-subjects design vs a within-subjects design .
Randomization
An experiment can be completely randomized or randomized within blocks (aka strata):
- In a completely randomized design , every subject is assigned to a treatment group at random.
- In a randomized block design (aka stratified random design), subjects are first grouped according to a characteristic they share, and then randomly assigned to treatments within those groups.
Completely randomized design | Randomized block design | |
---|---|---|
Phone use and sleep | Subjects are all randomly assigned a level of phone use using a random number generator. | Subjects are first grouped by age, and then phone use treatments are randomly assigned within these groups. |
Temperature and soil respiration | Warming treatments are assigned to soil plots at random by using a number generator to generate map coordinates within the study area. | Soils are first grouped by average rainfall, and then treatment plots are randomly assigned within these groups. |
Sometimes randomization isn’t practical or ethical , so researchers create partially-random or even non-random designs. An experimental design where treatments aren’t randomly assigned is called a quasi-experimental design .
Between-subjects vs. within-subjects
In a between-subjects design (also known as an independent measures design or classic ANOVA design), individuals receive only one of the possible levels of an experimental treatment.
In medical or social research, you might also use matched pairs within your between-subjects design to make sure that each treatment group contains the same variety of test subjects in the same proportions.
In a within-subjects design (also known as a repeated measures design), every individual receives each of the experimental treatments consecutively, and their responses to each treatment are measured.
Within-subjects or repeated measures can also refer to an experimental design where an effect emerges over time, and individual responses are measured over time in order to measure this effect as it emerges.
Counterbalancing (randomizing or reversing the order of treatments among subjects) is often used in within-subjects designs to ensure that the order of treatment application doesn’t influence the results of the experiment.
Between-subjects (independent measures) design | Within-subjects (repeated measures) design | |
---|---|---|
Phone use and sleep | Subjects are randomly assigned a level of phone use (none, low, or high) and follow that level of phone use throughout the experiment. | Subjects are assigned consecutively to zero, low, and high levels of phone use throughout the experiment, and the order in which they follow these treatments is randomized. |
Temperature and soil respiration | Warming treatments are assigned to soil plots at random and the soils are kept at this temperature throughout the experiment. | Every plot receives each warming treatment (1, 3, 5, 8, and 10C above ambient temperatures) consecutively over the course of the experiment, and the order in which they receive these treatments is randomized. |
Here's why students love Scribbr's proofreading services
Discover proofreading & editing
Finally, you need to decide how you’ll collect data on your dependent variable outcomes. You should aim for reliable and valid measurements that minimize research bias or error.
Some variables, like temperature, can be objectively measured with scientific instruments. Others may need to be operationalized to turn them into measurable observations.
- Ask participants to record what time they go to sleep and get up each day.
- Ask participants to wear a sleep tracker.
How precisely you measure your dependent variable also affects the kinds of statistical analysis you can use on your data.
Experiments are always context-dependent, and a good experimental design will take into account all of the unique considerations of your study system to produce information that is both valid and relevant to your research question.
If you want to know more about statistics , methodology , or research bias , make sure to check out some of our other articles with explanations and examples.
- Student’s t -distribution
- Normal distribution
- Null and Alternative Hypotheses
- Chi square tests
- Confidence interval
- Cluster sampling
- Stratified sampling
- Data cleansing
- Reproducibility vs Replicability
- Peer review
- Likert scale
Research bias
- Implicit bias
- Framing effect
- Cognitive bias
- Placebo effect
- Hawthorne effect
- Hindsight bias
- Affect heuristic
Experimental design means planning a set of procedures to investigate a relationship between variables . To design a controlled experiment, you need:
- A testable hypothesis
- At least one independent variable that can be precisely manipulated
- At least one dependent variable that can be precisely measured
When designing the experiment, you decide:
- How you will manipulate the variable(s)
- How you will control for any potential confounding variables
- How many subjects or samples will be included in the study
- How subjects will be assigned to treatment levels
Experimental design is essential to the internal and external validity of your experiment.
The key difference between observational studies and experimental designs is that a well-done observational study does not influence the responses of participants, while experiments do have some sort of treatment condition applied to at least some participants by random assignment .
A confounding variable , also called a confounder or confounding factor, is a third variable in a study examining a potential cause-and-effect relationship.
A confounding variable is related to both the supposed cause and the supposed effect of the study. It can be difficult to separate the true effect of the independent variable from the effect of the confounding variable.
In your research design , it’s important to identify potential confounding variables and plan how you will reduce their impact.
In a between-subjects design , every participant experiences only one condition, and researchers assess group differences between participants in various conditions.
In a within-subjects design , each participant experiences all conditions, and researchers test the same participants repeatedly for differences between conditions.
The word “between” means that you’re comparing different conditions between groups, while the word “within” means you’re comparing different conditions within the same group.
An experimental group, also known as a treatment group, receives the treatment whose effect researchers wish to study, whereas a control group does not. They should be identical in all other ways.
Cite this Scribbr article
If you want to cite this source, you can copy and paste the citation or click the “Cite this Scribbr article” button to automatically add the citation to our free Citation Generator.
Bevans, R. (2023, June 21). Guide to Experimental Design | Overview, 5 steps & Examples. Scribbr. Retrieved June 18, 2024, from https://www.scribbr.com/methodology/experimental-design/
Is this article helpful?
Rebecca Bevans
Other students also liked, random assignment in experiments | introduction & examples, quasi-experimental design | definition, types & examples, how to write a lab report, get unlimited documents corrected.
✔ Free APA citation check included ✔ Unlimited document corrections ✔ Specialized in correcting academic texts
7.1 Overview of Survey Research
Learning objectives.
- Define what survey research is, including its two important characteristics.
- Describe several different ways that survey research can be used and give some examples.
What Is Survey Research?
Survey research is a quantitative and qualitative method with two important characteristics. First, the variables of interest are measured using self-reports (using questionnaires or interviews). In essence, survey researchers ask their participants (who are often called respondents in survey research) to report directly on their own thoughts, feelings, and behaviors. Second, considerable attention is paid to the issue of sampling. In particular, survey researchers have a strong preference for large random samples because they provide the most accurate estimates of what is true in the population. In fact, survey research may be the only approach in psychology in which random sampling is routinely used. Beyond these two characteristics, almost anything goes in survey research. Surveys can be long or short. They can be conducted in person, by telephone, through the mail, or over the Internet. They can be about voting intentions, consumer preferences, social attitudes, health, or anything else that it is possible to ask people about and receive meaningful answers. Although survey data are often analyzed using statistics, there are many questions that lend themselves to more qualitative analysis.
Most survey research is non-experimental. It is used to describe single variables (e.g., the percentage of voters who prefer one presidential candidate or another, the prevalence of schizophrenia in the general population) and also to assess statistical relationships between variables (e.g., the relationship between income and health). But surveys can also be experimental. The study by Lerner and her colleagues is a good example. Their use of self-report measures and a large national sample identifies their work as survey research. But their manipulation of an independent variable (anger vs. fear) to assess its effect on a dependent variable (risk judgments) also identifies their work as experimental.
History and Uses of Survey Research
Survey research may have its roots in English and American “social surveys” conducted around the turn of the 20th century by researchers and reformers who wanted to document the extent of social problems such as poverty (Converse, 1987) [1] . By the 1930s, the US government was conducting surveys to document economic and social conditions in the country. The need to draw conclusions about the entire population helped spur advances in sampling procedures. At about the same time, several researchers who had already made a name for themselves in market research, studying consumer preferences for American businesses, turned their attention to election polling. A watershed event was the presidential election of 1936 between Alf Landon and Franklin Roosevelt. A magazine called Literary Digest conducted a survey by sending ballots (which were also subscription requests) to millions of Americans. Based on this “straw poll,” the editors predicted that Landon would win in a landslide. At the same time, the new pollsters were using scientific methods with much smaller samples to predict just the opposite—that Roosevelt would win in a landslide. In fact, one of them, George Gallup, publicly criticized the methods of Literary Digest before the election and all but guaranteed that his prediction would be correct. And of course, it was. (We will consider the reasons that Gallup was right later in this chapter.) Interest in surveying around election times has led to several long-term projects, notably the Canadian Election Studies which has measured opinions of Canadian voters around federal elections since 1965. Anyone can access the data and read about the results of the experiments in these studies (see http://ces-eec.arts.ubc.ca/ )
From market research and election polling, survey research made its way into several academic fields, including political science, sociology, and public health—where it continues to be one of the primary approaches to collecting new data. Beginning in the 1930s, psychologists made important advances in questionnaire design, including techniques that are still used today, such as the Likert scale. (See “What Is a Likert Scale?” in Section 7.2 “Constructing Survey Questionnaires” .) Survey research has a strong historical association with the social psychological study of attitudes, stereotypes, and prejudice. Early attitude researchers were also among the first psychologists to seek larger and more diverse samples than the convenience samples of university students that were routinely used in psychology (and still are).
Survey research continues to be important in psychology today. For example, survey data have been instrumental in estimating the prevalence of various mental disorders and identifying statistical relationships among those disorders and with various other factors. The National Comorbidity Survey is a large-scale mental health survey conducted in the United States (see http://www.hcp.med.harvard.edu/ncs ). In just one part of this survey, nearly 10,000 adults were given a structured mental health interview in their homes in 2002 and 2003. Table 7.1 presents results on the lifetime prevalence of some anxiety, mood, and substance use disorders. (Lifetime prevalence is the percentage of the population that develops the problem sometime in their lifetime.) Obviously, this kind of information can be of great use both to basic researchers seeking to understand the causes and correlates of mental disorders as well as to clinicians and policymakers who need to understand exactly how common these disorders are.
Generalized anxiety disorder | 5.7 | 7.1 | 4.2 |
Obsessive-compulsive disorder | 2.3 | 3.1 | 1.6 |
Major depressive disorder | 16.9 | 20.2 | 13.2 |
Bipolar disorder | 4.4 | 4.5 | 4.3 |
Alcohol abuse | 13.2 | 7.5 | 19.6 |
Drug abuse | 8.0 | 4.8 | 11.6 |
And as the opening example makes clear, survey research can even be used to conduct experiments to test specific hypotheses about causal relationships between variables. Such studies, when conducted on large and diverse samples, can be a useful supplement to laboratory studies conducted on university students. Although this approach is not a typical use of survey research, it certainly illustrates the flexibility of this method.
Key Takeaways
- Survey research features the use of self-report measures on carefully selected samples. It is a flexible approach that can be used to study a wide variety of basic and applied research questions.
- Survey research has its roots in applied social research, market research, and election polling. It has since become an important approach in many academic disciplines, including political science, sociology, public health, and, of course, psychology.
- a social psychologist
- an educational researcher
- a market researcher who works for a supermarket chain
- the mayor of a large city
- the head of a university police force
- Converse, J. M. (1987). Survey research in the United States: Roots and emergence, 1890–1960 . Berkeley, CA: University of California Press. ↵

Share This Book
- Increase Font Size
- Prospective students
- International students
- Companies and organizations
- Research Areas
- Research Projects
- Expertise & Consulting
- Methods Schools
- Seminars and webinars
- Conferences
- LAB Services
- Publications
- RECSM Working Paper Series
- RECSM Members
- External Collaborators
- Former Members
- Visiting Researchers
RECSM Research and Expertise Centre for Survey Methodology
Survey experiments.
Survey experiments have emerged as one of the most powerful methodological tools in the social sciences. By combining experimental design that provides clear causal inference with the flexibility of the survey context as a site for behavioral research, survey experiments can be used in almost any field to study almost any question. Conducting survey experiments can appear fairly simple but doing them well is hard.
This course will use published examples of experimental research to demonstrate a variety of ways to leverage survey experiments for testing social science theories. The course will teach participants how to use different survey experimental designs and how to address challenges related to sampling, survey mode, ethics, effect heterogeneity, and more. Students leave the course with a thorough understanding of how survey experiments can provide useful causal inferences, knowledge of how to design and analyze simple and complex experiments, and the ability to evaluate experimental research and apply these methods in their own research.
Students interested in discussing their own research are welcome to make appointments to meet with the instructor outside of class time.
A tentative outline of the course is given below.
Session 1: Survey Experiments in Context (July 4, 9:00-11:00)
- 9:00-9:30 - Introductions and Course Overview
- 9:30-9:55 - History of the Survey Experiment (and Experiments, generally)
- 10:30-11:00 - Potential Outcomes Framework of Causality
- Druckman, J. N., Green, D. P., Kuklinski, J. H., and Lupia, A. 2006. "The Growth and Development of Experimental Research in Political Science." American Political Science Review 100: 627-635.
- Kuklinski, J. H. and Hurley, N. L. 1994. "On Hearing and Interpreting Political Messages: A Cautionary Tale of Citizen Cue-Taking" The Journal of Politics 56: 729-751.
- Holland, P. W. 1986. "Statistics and Causal Inference." Journal of the American Statistical Association 81: 945-960.
Session 2: Examples and Paradigms (July 5, 9:00-11:00)
- 9:00-9:20 - Translating Theories into Experiments
- 9:20-9:55 - Question Wording and Vignettes
- 10:05-10:30 - Measuring Sensitive Items
- Pre-post designs
- Unexpected quasi-experiments
- Measuring effects of field interventions
- Tversky, A. and Kahneman, D. 1981. "The Framing of Decisions and the Psychology of Choice." Science 211: 453-458.
- Schuldt, J. P., Konrath, S. H., and Schwarz, N. 2011. "'Global Warming' or 'Climate Change'?: Whether the Planet is Warming Depends on Question Wording." Public Opinion Quarterly 75: 115-124.
- Glynn, A. N. 2013. "What Can We Learn with Statistical Truth Serum?: Design and Analysis of the List Experiment." Public Opinion Quarterly 77: 159-172.
- Albertson, B. L. and Lawrence, A. 2009. "After the Credits Roll: The Long-Term Effects of Educational Television on Public Knowledge and Attitudes." American Politics Research 37: 275-300.
Session 3: External Validity (July 6, 9:00-11:00)
- 9:00-9:30 - External Validity
- 9:30-9:55 - Pretreatment Dynamics
- 10:05-10:25 - Measuring Behaviors and Behavioral Intentions
- 10:25-11:00 - Sampling, Respondents, and Representativeness
- Gaines, B. J., Kuklinski, J. H., and Quirk, P. J. 2007. "The Logic of the Survey Experiment Reexamined." Political Analysis 15: 1-20.
- Druckman, J. N. and Leeper, T. J. 2012. "Learning More from Political Communication Experiments: Pretreatment and Its Effects." American Journal of Political Science 56: 875-896.
- Bolsen, T. 2013. "A Light Bulb Goes On: Norms, Rhetoric, and Actions for the Public Good." Political Behavior 35: 1-20.
- Mullinix, K. J., Leeper, T. J., Druckman, J. N., and Freese, J. 2015. "The Generalizability of Survey Experiments." Journal of Experimental Political Science : In press.
Session 4: Sources of Heterogeneity (July 7, 9:00-11:00)
- 9:00-9:30 - Attention and Satisficing
- 9:30-9:55 - Effect Heterogeneity, Moderators, and Blocking
- 10:05-11:00 - Factorial Designs, Confounding, and Conjoint Designs
- Clifford, S. and Jerit, J. 2015. "Do Attempts to Improve Respondent Attention Increase Social Desirability Bias?" Public Opinion Quarterly 79: 790-802.
- Green, D. P. and Kern, H. L. 2012. "Modeling Heterogeneous Treatment Effects in Survey Experiments with Bayesian Additive Regression Trees." Public Opinion Quarterly 76: 491-511.
- Hainmueller, J., Hangartner, D., and Yamamoto, T. 2015. "Validating Vignette and Conjoint Survey Experiments Against Real-World Behavior." Proceedings of the National Academy of Sciences : In press.
Session 5: Lingering Issues (July 8, 9:00-11:00)
- Recruitment and Attrition
- Conditioning
- 9:30-9:55 - Treatment Self-Selection
- 10:05-11:00 - Ethics
- Recruitment
- Randomization
- Publication Bias
- Warren, J. R. and Halpern-Manners, A. 2012. "Panel Conditioning in Longitudinal Social Science Surveys." Sociological Research & Methods 41: 491-534.
- Leeper, T. J. "The Role of Media Choice and Media Effects in Political Knowledge Gaps." Working paper, London School of Economics and Political Science.
- Hertwig, R. and Ortmann, A. 2008. "Deception in Experiments: Revisiting the Arguments in Its Defense." Ethics & Behavior 18: 59-92.
Further Reading
Though not assigned for the course, the following texts may serve as useful background reading or places for further inspiration in the design and analysis of survey experiments.
- Mutz, D. C. 2011. Population-Based Survey Experiments . Princeton, NJ: Princeton University Press.
- Gerber, A. S. and Green, D. P. 2012. Field Experiments: Design, Analysis, and Interpretation . New York: W.W. Norton.
- Schuman, H. and Presser, S. 1981. Questions and Answers in Attitude Surveys: Experiments on Question Form, Wording, and Context . SAGE Publications.
- Groves, R. M., Fowler, F. J., Couper, M. P., Lepkowski, J. M., Singer, E., and Tourangeau, R. 2009. Survey Methodology . Wiley-Interscience.
Methodology:
- Blair, G. and Imai, K. 2012. "Statistical Analysis of List Experiments." Political Analysis 20: 47-77.
- Jamieson, J. P. and Harkins, S. G. 2011. "The Intervening Task Method: Implications for Measuring Mediation." Personality & Social Psychology Bulletin 37: 652-661.
- Green, D. P., Ha, S. E., and Bullock, J. G. 2009. "Enough Already about 'Black Box' Experiments: Studying Mediation is More Difficult than Most Scholars Suppose." The ANNALS of the American Academy of Political and Social Science 628: 200-208.
- Wang, W., Rothschild, D., Goel, S., and Gelman, A. 2015. "Forecasting Elections with Non-representative Polls." International Journal of Forecasting : In press.
- Chandler, J., Paolacci, G., Peer, E., Mueller, P., and Ratliff, K. A. 2015. "Using Nonnaive Participants Can Reduce Effect Sizes." Psychological Science : In press.
- Banducci, S. and Stevens, D. 2015. "Surveys in Context: How Timing in the Electoral Cycle Influences Response Propensity and Satisficing." Public Opinion Quarterly 79: 214-243.
- Hainmueller, J., Hopkins, D. J., and Yamamoto, T. 2014. "Causal Inference in Conjoint Analysis: Understanding Multi-Dimensional Choices via Stated Preference Experiments." Political Analysis 22: 1-30.
- Tourangeau, R. and Smith, T. W. 1996. "Asking Sensitive Questions: The Impact of Data Collection Mode, Question Format, and Question Context." Public Opinion Quarterly 60: 275-304.
- Kreuter, F., Presser, S., and Tourangeau, R. 2009. "Social Desirability Bias in CATI, IVR, and Web Surveys: The Effects of Mode and Question Sensitivity." Public Opinion Quarterly 72: 847-865.
- Hovland, C. I. 1959. "Reconciling Conflicting Results Derived from Experimental and Survey Studies of Attitude Change." American Psychologist 14: 8-17.
- Sterling, T. D., Rosenbaum, W. L., and Weinkam, J. 1995. "Publication Decisions Revisited: The Effect of the Outcome of Statistical Tests on the Decision to Publish and Vice Versa." The American Statistician 49: 108-112.
- Franco, A., Malhotra, N., and Simonovits, G. 2015. "Underreporting in Political Science Survey Experiments: Comparing Questionnaires to Published Results." Political Analysis 23: 306-312.
Instructor Bio
Thomas J. Leeper is an Assistant Professor in Political Behaviour in the Department of Government at the London School of Economics and Political Science .
Thomas J. Leeper, assistant professor in Political Behaviour at the London School of Economics and Political Science, studies public opinion dynamics using survey and experimental methods, with a focus on citizens' information acquisition, elite issue framing, and party endorsements within the United States and Western Europe. His research has been published in leading journals, including American Political Science Review , American Journal of Political Science , Public Opinion Quarterly , and Political Psychology among others.
- Architecture and Design
- Asian and Pacific Studies
- Business and Economics
- Classical and Ancient Near Eastern Studies
- Computer Sciences
- Cultural Studies
- Engineering
- General Interest
- Geosciences
- Industrial Chemistry
- Islamic and Middle Eastern Studies
- Jewish Studies
- Library and Information Science, Book Studies
- Life Sciences
- Linguistics and Semiotics
- Literary Studies
- Materials Sciences
- Mathematics
- Social Sciences
- Sports and Recreation
- Theology and Religion
- Publish your article
- The role of authors
- Promoting your article
- Abstracting & indexing
- Publishing Ethics
- Why publish with De Gruyter
- How to publish with De Gruyter
- Our book series
- Our subject areas
- Your digital product at De Gruyter
- Contribute to our reference works
- Product information
- Tools & resources
- Product Information
- Promotional Materials
- Orders and Inquiries
- FAQ for Library Suppliers and Book Sellers
- Repository Policy
- Free access policy
- Open Access agreements
- Database portals
- For Authors
- Customer service
- People + Culture
- Journal Management
- How to join us
- Working at De Gruyter
- Mission & Vision
- De Gruyter Foundation
- De Gruyter Ebound
- Our Responsibility
- Partner publishers

Your purchase has been completed. Your documents are now available to view.
Population-Based Survey Experiments
- Diana C. Mutz
- X / Twitter
Please login or register with De Gruyter to order this product.
- Language: English
- Publisher: Princeton University Press
- Copyright year: 2011
- Edition: Course Book
- Audience: Professional and scholarly;College/higher education;
- Main content: 200
- Other: 5 tables.
- Keywords: population-based survey ; survey experiments ; random samples ; external validity ; internal validity ; population-based survey experiments ; traditional experiments ; traditional surveys ; hybrid methodology ; research design ; population average ; measurement ; split-ballot approach ; hypotheses ; item count technique ; inferential process ; anchoring ; population-based experiments ; direct treatments ; indirect treatments ; researchers ; card sort techniques ; false feedback ; social science laboratories ; Internet ; vignette treatments ; factorial designs ; hypothetical people ; complex theories ; direct treatment ; game-based treatments ; online experiments ; gaming ; economic games ; random population samples ; realism ; population-based experiment ; Institutional Review Board ; war stories ; independent variable ; ethics ; human subjects ; analysis stage ; randomization checks ; survey weights ; covariates ; observational methods ; generalizability ; experimentalists ; observational studies ; surveys ; real world settings ; particularistic research ; cause ; effect ; social science theories ; research
- Published: July 5, 2011
- ISBN: 9781400840489
Online ordering is currently unavailable due to technical issues. We apologise for any delays responding to customers while we resolve this. For further updates please visit our website: https://www.cambridge.org/news-and-insights/technical-incident
We use cookies to distinguish you from other users and to provide you with a better experience on our websites. Close this message to accept cookies or find out how to manage your cookie settings .
Login Alert

- > Journals
- > Italian Political Science Review / Rivista Italiana di Scienza Politica
- > Volume 51 Special Issue 2: Capturing Causation: Is...
- > From the lab to the poll: The use of survey experiments...
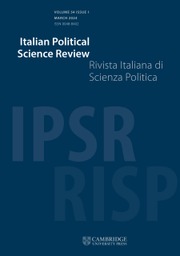
Article contents
Introduction, a primer to experiments, survey experiments in political research, a factorial experiment on preferences towards asylum seekers, a conjoint analysis on candidate preference, conclusions, from the lab to the poll: the use of survey experiments in political research.
Published online by Cambridge University Press: 28 May 2021
- Supplementary materials
The article offers an overview of the use of survey experiments in political research by relying on available examples, bibliographic data and a content analysis of experimental manuscripts published in leading academic journals over the last two decades. After a short primer to the experimental approach, we discuss the development, applications and potential problems to internal and external validity in survey experimentation. The article also provides original examples, contrasting a traditional factorial and a more innovative conjoint design, to show how survey experiments can be used to test theory on relevant political topics. The main challenges and possibilities encountered in envisaging, planning and implementing survey experiments are examined. The article outlines the merits, limits and implications of the use of the experimental method in political research.
Randomised-controlled experiments represent the gold standard for ascertaining causation. This is not to say that experiments are free of limitations and, as will be clarified, some of them contributed to a certain reluctance towards the usage of experimental methods in political science. While acknowledging the merit of experimentation in the empirical investigation of causal claims, political scientists long lagged behind psychologists and economists in the use of the experimental approach. It was only with the development of (population-based) survey experiments, facilitated by new advancements in survey techniques, that some of these limitations, and in particular the scarce generalisability of the results from an experimental sample to a population of interest, were mitigated, unveiling the virtues of the experimental design to a wider audience of social and political scientists.
This article provides an overview of the use of survey experiments in political research and an illustration of how experimental data can be modelled and interpreted. Then, it describes some key concepts for the understanding and application of experiments in the discipline, clarifying the basic theoretical issues tied to causal inference and the most common experimental designs to achieve it. In the following section, we focus on survey experiments and their ambition to combine internal and external validity. By presenting the results of a content analysis of experimental articles published by leading academic journals over the last two decades, we show a lag in the use of experimentation in European research as compared to the American one, but also an increasing interest in survey experiments from European scholars. Based on this and with the purpose of introducing the main challenges and possibilities encountered in designing, planning and implementing survey experiments, we then contrast a traditional factorial and a more innovative conjoint design, considering how treatments are usually formulated and assigned. In this regard, we illustrate how survey experimental data can be analysed to estimate average treatment effects and conditional treatment effects across subgroups of subjects by means of two original examples on attitudes towards asylum seekers and preference towards political candidates, respectively. We conclude with some considerations on the merits, limits and implications of experimental designs in political research.
Green ( Reference Green, Lewis-Beck, Bryman and Futing Liao 2004 ) points to two main characteristics to distinguish the experimental design from other methods of social investigation: a planned intervention and a random assignment. The first refers to the treatment administered to the units of analysis and whose impact on the outcome variable the researcher is interested to estimate. The treatment represents the independent variable and, in controlled experiments, is manipulated under the direct control of the researcher. The second characteristic, instead, has to do with the process through which subjects are allocated to one (or more) treatment group(s). Randomisation ensures that all groups are balanced across potential covariates – an assumption that the experimental analyst should nonetheless demonstrate – and that no systemic relationships exist between the treatment factor(s) and other observed or unobserved variables.
Because of these two key features, experimental studies are considered to be better equipped to address causal questions than observational studies. Contrary to observational research, where the researcher usually relies on statistical modelling to avoid problems of endogeneity and unobserved heterogeneity, in an experimental setting confounding variables are controlled by design, resulting in unbiased causal inference if randomisation has been properly implemented. Since in an experimental study each subject has the same probability to be assigned to a treatment condition, the final outcome will solely depend on the stimulus received, while the effect of possible confounding factors will be balanced across all the considered groups. Footnote 1
Experiments usually achieve superior internal validity – the possibility of establishing a causal effect (McDermott, Reference McDermott, Druckman, Green, Kuklinski and Lupia 2011 ) – through a ‘between-subject’ design in which the subject is assigned either to a treatment condition (i.e., the one that receives the intended manipulation) or to a control condition (i.e., the one that is used as a counterfactual to estimate what would have happened if the intervention had not been administered).
However, experiments also allow to vary treatments while holding subjects constant and controlling for subject-specific effects. This is the case of ‘within-subject’ designs, in which each participant receives one or more treatments (or controls) and causal estimates are obtained by comparing the same subjects' behaviour across the different conditions over the experiment duration, generally before and after each treatment. Within-subject designs, which require independence of exposure to multiple treatments, have greater statistical power than between-subject designs, as more data points are offered for the same subjects. Moreover, they are more adequate in environments where an individual faces more than one choice during a sequence of events (e.g., as in bargaining and collective action research). Still, ‘within-subject’ designs may introduce possible confounds by exposing the same subject to multiple interventions and more likely produce spurious results due to a ‘demand effect’, i.e., participants understand the experimenter's intention and behave accordingly to satisfy her or his expectations (Charness et al ., Reference Charness, Gneezy and Kuhnc 2012 ).
The standard procedure to estimate the treatment effect is to compare the differences for the outcome of interest across the different groups or experimental conditions, also known as the average treatment effect (ATE). However, experimenters may also decide to test for possible moderators and baseline covariates that might affect the relationship between the treatment and the outcome, thus assessing possible heterogeneity of a treatment effect depending on another treatment or one or more characteristics of the participants. This is commonly done using a regression model with interaction terms (moderators) to estimate the conditional average treatment effect (CATE).
Across disciplines, experiments have been more concerned with the identification of treatment effects than the generalisability of findings across different subjects and groups – the external validity (McDermott, Reference McDermott, Druckman, Green, Kuklinski and Lupia 2011 ). This is specifically the case of ‘laboratory’ experiments, which generally occur in a more artificial but highly controlled environment. Laboratory experiments have the advantage of flexibility, as they can easily be conducted at low costs with convenience samples, including students and volunteers. However, participants in such experiments are generally viewed as unrepresentative of any target population (Iyengar, Reference Iyengar, Druckman, Green, Kuklinski and Lupia 2011 ). Moreover, laboratory experiments imply some sort of interaction between participants and researchers, so that results may be biased by demand effects (Zizzo, Reference Zizzo 2010 ). Last, in spite of better control, the artificial nature of the setting may prevent results to be extended to real-world situations. It is worth mentioning, however, that this kind of experiments can be moved from a typical university laboratory to a more naturalistic one (township, households), so to have a hybrid ‘lab-in-the-field’ design (Morton and Williams, Reference Morton and Williams 2010 ).
When treatments are randomly administered in a naturalistic setting under the direct control of the researcher, these are classified as ‘field’ experiments. By evaluating the effect of the treatment in a real-world setting, the analyst has the possibility to make unbiased and externally valid causal claims. One can argue that estimates derived from one setting at a given time cannot be applied easily to another context or time period. Still, as Green and Gerber ( Reference Green and Gerber 2003 : 101) pointed out, ‘extrapolation from one field setting to another involves less uncertainty than the jump from lab to field or from non-experimental correlations to causation’. When researchers take advantage of random assignments that occur naturally but not under their direct planned intervention (e.g., use of a lottery to allocate resources, policies or duties), instead, we are in the presence of a randomised ‘natural’ experiment (Dunning, Reference Dunning 2012 ). Footnote 2
Last, experiments may be embedded in a survey through the manipulation of different elements of a questionnaire (Gaines et al ., Reference Gaines, Kuklinski and Quirk 2007 ). These experimental settings combine treatment manipulation and random assignment with survey sampling, ensuring a broader variation of the pool of subjects being considered and helping bring experimental research outside of the lab. Survey experiments may be conducted with either non-probability or probability samples of participants; when administered to a randomly selected, representative sample of a target population, they are referred to as ‘population-based survey experiments’ (henceforth PBSE) and allow the researcher to make population inferences about causal relationships drawn from experimental findings (Mutz, Reference Mutz 2011 ).
Development of survey experiments
Experiments for long remained an almost uncharted territory for political scientists, given their interest in the generalisability of findings to target populations, the asserted artificiality of the laboratory setting and unrepresentativeness of the experimental subjects (Iyengar, Reference Iyengar, Druckman, Green, Kuklinski and Lupia 2011 ). It was only in the 1970s, with the emergence of political psychology as an interdisciplinary field, that a certain interest in the experimental approach started to develop (McGraw and Hoekstra, Reference McGraw, Hoekstra, Delli Carpini, Huddy and Shapiro 1994 ).
Nevertheless, we had to wait until the 1990s to observe a real growth in the number of experimental studies in political science, especially in the US. As Druckman and colleagues have reported, more than half of the experimental articles appeared in the American Political Science Review (henceforth APSR) between its foundation in 1906 and the late 2000s was published after 1992 (Druckman et al ., Reference Druckman, Green, Kuklinski and Lupia 2006 , Reference Druckman, Green, Kuklinski, Lupia, Druckman, Green, Kuklinski and Lupia 2011 ). Political scientists' increasing reliance on experimental methods was later confirmed by Dunning and Rosenblatt ( Reference Dunning and Rosenblatt 2016 ), who found a further increase in the number of APSR articles reporting experimental research in the early 2010s. This was the result of technological improvement connected to computer assisted telephone interview as well as ambitious projects, such as the Multi-Investigator Study and the following Time-Sharing Experiments for the Social Sciences, which allowed researchers to administer complex randomised experiments to large probability samples of participants (Sniderman and Grob, Reference Sniderman and Grob 1996 ; Mutz Reference Mutz 2011 ).
Building on Druckman and colleagues' criteria to classify research articles presenting ‘primary data from a random assignment study with participants’ (Druckman et al ., Reference Druckman, Green, Kuklinski and Lupia 2006 : 628–629), we can observe a remarkable increase (33.3%) in the number of APSR articles using randomised experiments in the last five years as compared to the 2010–2014 period ( Figure 1 ), with a peak between 2018 and 2019 ( N = 21). Indeed, the percentage of experimental manuscripts over the total number of articles published in an issue of this journal has gradually grown over the last 15 years, so that experimental articles accounted for about one-fifth of APSR manuscripts in 2019. Footnote 3
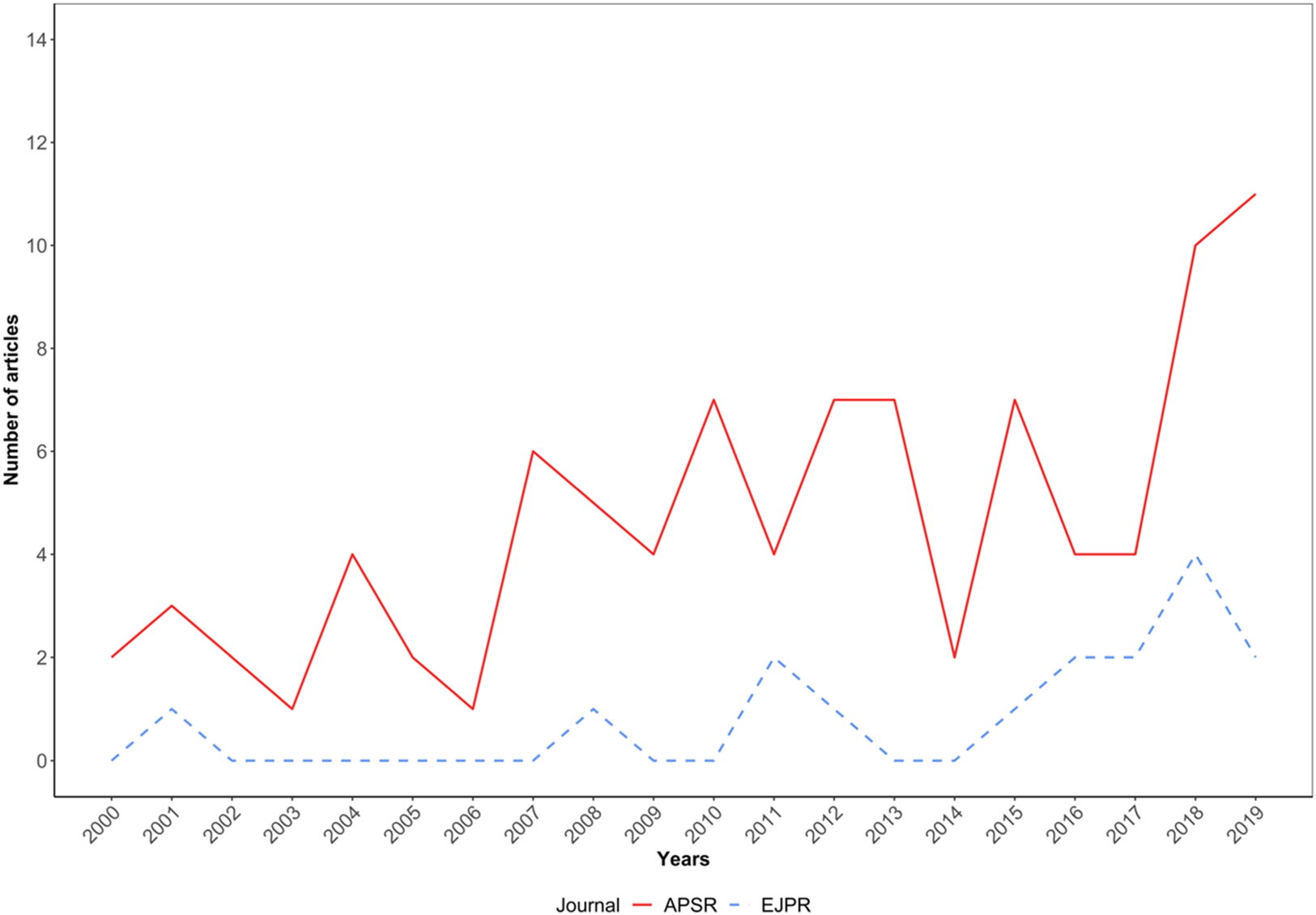
Figure 1. Number of experimental articles in APSR and EJPR, 2000–2019.
A similar upward trend, albeit of different magnitude, is observed in European political research. Using the European Journal of Political Research (henceforth EJPR) as a benchmark to assess whether and to what extent experimentation has also gained acceptance in Europe, Footnote 4 we found that about two-thirds (63%) of the articles making use of randomised experiments between 2000 and 2019 have been published in the last four years. The volume of experimental manuscripts, however, has remained quite low if compared with APSR. Experimental articles, on average, still account for only 5.1% of total manuscripts published each year by EJPR, signalling that the use of experimental methods is not only relatively newer to European scholars but also less prominent than in the American context.
European political scientists' hesitation with the use of experimental methods is also confirmed by a content analysis of the manuscripts. While the typology of experiments in APSR articles is quite various, with more than one-third of the cases (37.6%) making use of a survey experiment, followed by laboratory (32.3%), field (30.1%) and natural experiments (3.2%), almost the totality of EJPR experimental articles (93.8%) relied on survey experiments. Only in a few instances field (12.5%) and laboratory experiments (6.3%) appeared in the journal, whereas natural experiments have not been published in a volume of EJPR over the last two decades. Yet, these figures also highlight a large interest towards survey experiments and their primacy over other type of settings. In this respect, it is interesting to note that PBSE account for 62.9% and 40% of APSR and EJPR survey experiments published in the 2000–2019 period, respectively.
Survey experiments in practice: vignette factorial and conjoint designs
Initially, the use of survey experiments was mostly limited to address measurement issues through the manipulation of the presence, wording or order of questionnaire items and their random allocation to interviewees. In one of the earliest examples of the ‘split ballot’ experiment, for instance, Rugg ( Reference Rugg 1941 ) found that Americans were more likely to support freedom of speech against democracy when asked whether the US should ‘forbid’ (46% of the interviewees answered ‘yes’) rather than ‘allow’ (62% of the sample answered ‘no’) public speeches against democracy. Over the years, survey experiments based on question wording have then been employed to address more substantive issues, testing, for instance, how framing may affect citizens' opinions about public policies (e.g., Kinder and Sanders, Reference Kinder and Sanders 1996 ).
However, the most common paradigm to formulate treatments has been the use of vignettes, in which a short text describes a situation, a policy proposal or a political stance often in combination with pictures and/or videos. Vignette experiments are very well-suited to determine the extent to which multiple factors contribute to attitude formation or the occurring of certain behaviours. Overcoming the main weakness of the simple ‘split ballot’, in which levels (values) of different factors (attributes) may vary only one at a time, ‘vignette factorial designs’ allow to estimate the joint effect of multiple attributes at their different levels (Mutz, Reference Mutz 2011 ), with the number of treatment groups being determined by the number of combinations of factor levels.
The first advantage of this design is that factors are randomly assigned and orthogonal to each other, thus allowing a researcher to estimate the effect of each single treatment while ignoring the others if these are shown to be insignificant. Otherwise, s/he needs to take into account that the average effect of one factor is weighted across the levels of the others (Gerber and Green, Reference Gerber and Green 2012 ). Second, factorial designs can be used to estimate not only how two or more treatments interact among them, but also the extent to which a given factor (or the combination of some factors) hinges on a third characteristic of the respondent, which is uncorrelated by randomisation. In this respect, a ‘full factorial design’, in which all the combinations of the factor levels are examined, has to be distinguished from a ‘fractional factorial design’, in which only a subset of these combinations is considered either because their number is too large or because resources for testing them all are not available. This last consideration leads us to one of the disadvantages of factorial designs, which is the trade-off between the number of conditions to be considered and the sample size. Researchers may increase the number of experimental factors only at the cost of efficiency. The number of conditions has, in fact, to be taken into account in relation with the number of subjects per experimental group and statistical power, that is, the probability of being able to reject the null hypothesis of no treatment effect (Gerber and Green, Reference Gerber and Green 2012 ).
Such a disadvantage can be avoided in ‘conjoint analysis’, a prime technique of preference elicitation introduced at the beginning of the 1980s (Alves and Rossi, Reference Alves and Rossi 1978 ) and commonly used in marketing research, whose recent formalisation by Hainmueller et al . ( Reference Hainmueller, Hopkins and Yamamoto 2013 ) has contributed to its popularity among political scientists. In conjoint experiments, respondents are requested to choose (discrete-choice conjoint analysis) and/or to rate (rating-based conjoint analysis) sets of possible alternatives (e.g., candidates to vote for, policy proposal to pass) resulting from the random variation of an indefinite number of factors, orthogonal among each other, that can assume multiple values. The analysis consists of estimating the simultaneous independent causal effects – average marginal component effect (AMCE) – of many features of multidimensional objects on the respondent's decision.
Contrary to common survey research, conjoint analysis starts from the assumption that social and political phenomena come in different facets that individuals are likely to simultaneously evaluate in real-world situations. Asking the respondent to judge a given object by introducing trade-off costs among different aspects helps reduce the problem of social desirability and the artificiality of the task while increasing the level of external validity (Hainmueller et al ., Reference Hainmueller, Hangartner and Yamamoto 2015 ).
Conjoint experiments are not exempt from criticism. First, they are cognitively demanding, as conducting such complex experiments with many attributes evaluated at once requires the repetition of the designed task. In this respect, there is no agreement on the ideal number of attributes to consider, nor on the number of tasks to be implemented. Researchers need to balance among the theoretical aspects to investigate, the respondents' fatigue, as well as sample size and statistical power (Bansak et al ., Reference Bansak, Hainmueller, Hopkins and Yamamoto 2018 , Reference Bansak, Hainmueller, Hopkins and Yamamoto 2021 ). A second criticism is that conjoint analysis may lead scholars to less formalised and more inductive forms of research, posing less restrictions with respect to the number of factors under observation. As some have argued (e.g., Sniderman Reference Sniderman 2018 ), however, this would represent more a pro than a con, since the whole scope of conducting an experiment is actually to evaluate countervailing explanations.
Remarkably, more than one-third of survey experiments appeared in APSR (5 out of 14) and EJPR (3 out of 10) between 2015 and 2019 were based on a conjoint approach, with the remaining two-thirds largely relying on a traditional factorial design. Given the widespread use of these two techniques in contemporary political research and in order to offer a valuable help to scholars interested in modelling this kind of data, in the next section we present the experimental protocol and results of a full factorial experiment on respondents' evaluation of asylum applications, moving then to a conjoint experiment on preferences towards ideal political candidates. First, however, we describe some problems to internal and external validity a researcher may face when conducting survey experiments. These problems will then be addressed in the analyses of our case studies.
Potential problems in survey experiments
When conducting survey experiments researchers need to address some challenges. One of the most relevant has to do with ‘noncompliance’ and its impact on internal validity (Druckman et al ., Reference Druckman, Green, Kuklinski, Lupia, Druckman, Green, Kuklinski and Lupia 2011 ). This problem occurs when the subjects assigned to a certain treatment (including control) do not receive it. This might take place as a result of either the respondent's active behaviour (e.g., not accomplishing a given task or dropping out during the survey) or an involuntary action (e.g., the participant receives a different treatment from that to which s/he was assigned or eventually s/he is not exposed to any stimulus).
To tackle active noncompliance, a researcher may evaluate participants' level of attention via the recorded duration of the experiment or the use of screening questions posed during the interview and asking respondents to select a certain response option to check for their cooperation (Berinsky et al ., Reference Berinsky, Margolis and Sances 2014 ). Lower attention, however, does not necessarily imply no treatment. An alternative to address this problem is to include ‘manipulation checks’, that is, additional questions placed at the end of the experiment to evaluate whether or not the subject received the treatment as intended. Overall, there is still a debate on the utility of these types of questions since the post-treatment exclusion of subjects with low levels of attention or failing to pass manipulation checks may add a bias rather than help the analyst establish the treatment's causal effect (Gerber et al ., Reference Gerber, Arceneaux, Boudreau, Dowling, Hillygus, Palfrey, Biggers and Hendry 2014 ; Mutz and Pemantle, Reference Mutz and Pemantle 2015 ).
When noncompliance is passive and related to failure in random assignment, the researcher should carefully discuss the number of subjects initially eligible for the study, the size of groups assigned to a certain treatment, how many did not receive the planned intervention, how the statistical analysis was handled and if any subject was excluded after the experiment. Ideally, researchers should provide intent-to-treat analysis of outcome variables considering all subjects assigned to a group regardless of whether the treatment was assigned or not (Gerber et al ., Reference Gerber, Arceneaux, Boudreau, Dowling, Hillygus, Palfrey, Biggers and Hendry 2014 ).
Another important challenge to internal validity has to do with ‘treatment spill-over effects’ (Transue et al ., Reference Transue, Lee and Aldrich 2009 ), namely the possible contamination between treatments pertaining to different experiments included in the same survey. Given the greater complexity of modern surveys, it is always a good practice to randomise the order of experiments (if more than one is included) and evaluate a possible order effect when analysing data.
Turning to external validity, the first challenge deals with ‘sampling issues’. Available studies have not detected significant differences between experimental treatment effects (both ATE and CATE) obtained through non-probabilistic and representative population samples (e.g., Mullinix et al., Reference Mullinix, Leeper, Druckman and Freese 2015 ; Coppock et al ., Reference Coppock, Leeper and Mullinix 2018 ). Still, it should be emphasised that PBSE are one of the most effective tools to combine causal inference and external validity. In this case, it is always recommended to report sample characteristics (Gerber et al ., Reference Gerber, Arceneaux, Boudreau, Dowling, Hillygus, Palfrey, Biggers and Hendry 2014 ) and, if applied, the employed weighting scheme.
Ultimately, external validity has also to do with the extent to which outcomes observed in an experiment resemble real-world situations. One common criticism raised towards laboratory and survey experiments concerns the lack of realism as they offer a stylised setting and treatments that are often deemed to mirror complex everyday situations only imperfectly (Sniderman, Reference Sniderman 2018 ). In a survey context, moreover, the stimuli might be more easily received than in the real world where competing frames are present (Barabas and Jerit, Reference Barabas and Jerit 2010 ). Thus, researchers should provide a justification of the stimuli and settings used in the experiment and carefully evaluate the results. Eventually, they might also try to validate them with similar situations in real-world environments (Hainmueller et al ., Reference Hainmueller, Hangartner and Yamamoto 2015 ). That said, and although not being exempt from limitations, survey experiments represent a useful tool to test theories about a broad range of political phenomena. As in the case of any other research endeavour, drafting, conducting and analysing an experiment is a difficult task of which each step should be discussed in detail and in accordance with shared standards (Gerber et al ., Reference Gerber, Arceneaux, Boudreau, Dowling, Hillygus, Palfrey, Biggers and Hendry 2014 ; Mutz and Pemantle, Reference Mutz and Pemantle 2015 ).
Following the so-called refugee crisis, the issue of immigration has become increasingly relevant in Europe, contributing to the development of a climate of insecurity and cultural threat among the European publics (Basile and Olmastroni, Reference Basile and Olmastroni 2020 ). Given its proximity to the Libyan coasts, the crisis has been even harsher in Italy. In the last few years, anti-immigrant sentiments have spread among citizens, with populist and right-wing parties often resorting to anti-Muslim rhetoric and capitalising on people's resentment (Guidi and Martini, Reference Guidi and Martini 2019 ).
Yet, while Western citizens may tend to oppose more open immigration policies, some experimental studies show that they are far less reluctant to admit individual immigrants. This person-positivity bias seems to vary according to the immigrant's profile, with preferences over asylum-seekers structured by economic, humanitarian and ethnic concerns. Specifically, asylum seekers with a high-skill background (i.e., a better occupational standing) are more likely to be accepted than those with low-skill profiles (Iyengar et al ., Reference Iyengar, Jackman, Messing, Valentino, Aalberg, Duch, Hahn, Soroka, Harell and Kobayashi 2013 ). Immigrants who fear political prosecution are more likely to be favoured than those who move for economic reasons (Bansak et al ., Reference Bansak, Hainmueller and Hangartner 2016 ), whereas individuals from Muslim-majority countries would have less chances to see their asylum request accepted (Valentino et al ., Reference Valentino, Soroka, Iyengar, Aalberg, Duch, Fraile, Hahn, Hansen, Harell, Helbling, Jackman and Kobayashi 2019 ). Interestingly, leftists seem to be more sensitive to humanitarian vis-à-vis instrumental reasons and less concerned about immigrants' religious identity than their right-wing fellow citizens (Bansak et al ., Reference Bansak, Hainmueller and Hangartner 2016 ). Coming to the Italian context, experiments covering this topic are rare, with exceptions showing a link between ideology and party alignments, on the one hand, and partisan cue-taking and ethnic prejudice, on the other (Barisione, Reference Barisione 2020 ).
Thus, one factorial experiment might help disentangle what factors matter the most for asylum-seeker acceptance, whether there is an interplay among instrumental, humanitarian and ethnic considerations, and to what extent, if any, political ideology moderates their relationships. Although the following example is merely illustrative, available research (Bansak et al ., Reference Bansak, Hainmueller and Hangartner 2016 ; Barisione, Reference Barisione 2020 ) leads us to believe that Italians would look more favourably at asylum applications pursued by skilled immigrants rather than low-skilled ones (h1); by those escaping war as compared to those coming for economic opportunities (h2); or by subjects from Christian-majority countries against Muslim-majority ones (h3).
We also can assume that the applicant's skills and ethnicity might moderate the differential effect of the motivation for migrating, so that the gap in the approval of an asylum application presented by an individual escaping from war and one looking for better economic conditions would be reduced when the subject is skilled and from a Christian-majority country (h4).
Last, we might expect instrumental, humanitarian and ethnic concerns to hinge on the ideology of the respondent, such that the approval gap between skilled and non-skilled migrants will be larger among right-wing participants than among left-wing ones (h5a); the approval gap between migrants coming for economic and humanitarian reasons will be smaller among right-wing voters compared to left-wing ones (h5b); and the approval gap between migrants coming from Christian-majority vis-à-vis Muslim-majority countries will be larger among right-wing respondents compared to left-wing ones (h5c).
The experiment we consider in this section was embedded in the second wave of the EUENGAGE online panel survey, conducted between 6 July and 6 October 2017. Respondents were approached through an opt-in online panel provided by Research Now using quota sampling to reflect the general population's characteristics (see Appendix A). While the whole sample includes individuals from 10 EU member states, our analysis is limited to the Italian sample ( n = 1278). Since weighting non-probability samples may be a problematic task (Mullinix et al ., Reference Mullinix, Leeper, Druckman and Freese 2015 ), we decided to present analysis on unweighted data, thus focusing on causal relationships without any claim of representativeness or generalisability.
Respondents began the experiment by reading a short introduction about an asylum applicant interested in migrating to Italy. Then, each interviewee was invited to examine his background along with a picture of the applicant. While the picture remained constant, subjects were randomly assigned to one of the eight different experimental groups resulting from the combination of three treatments. Figure 2 shows one possible vignette generated through the random assignment of the examined conditions (the full protocol is in Appendix B).
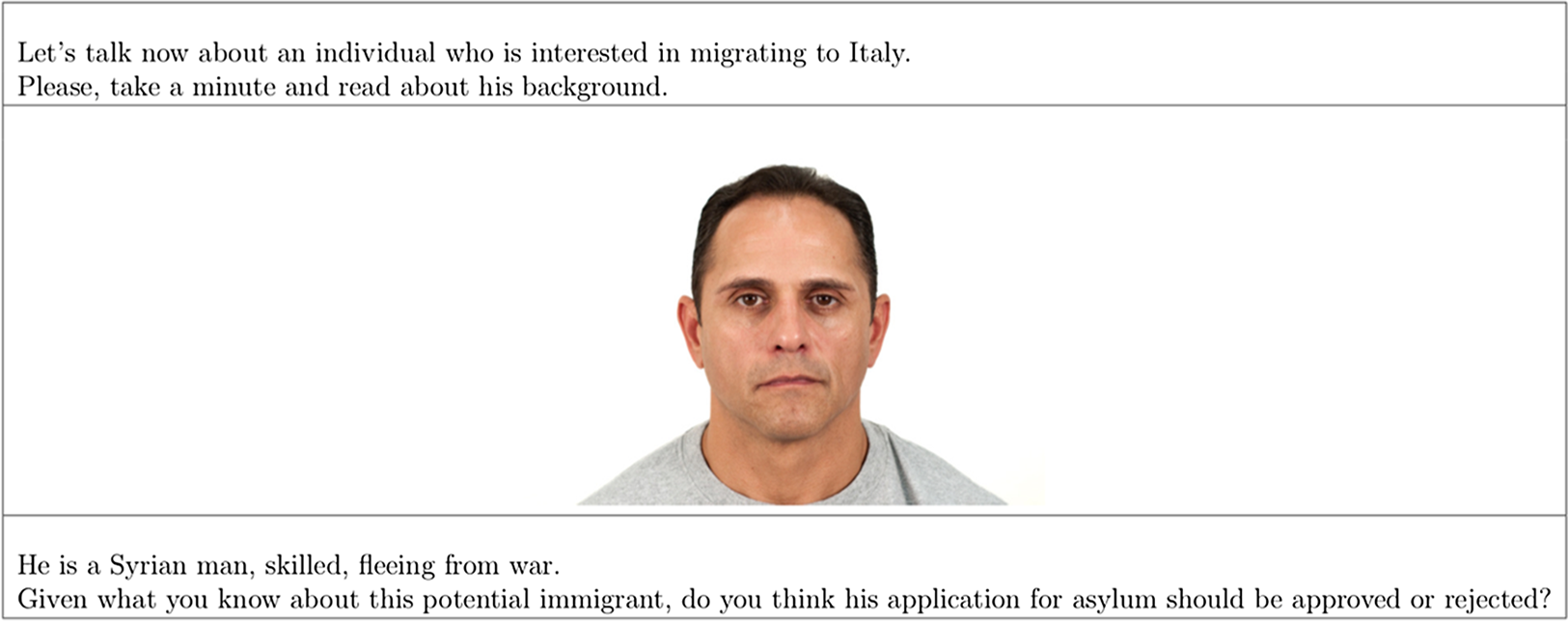
Figure 2. Stimuli: an illustration of the factorial experiment.
The picture comes from the Chicago Face Database (Ma et al ., Reference Ma, Correll and Wittenbrink 2015 ), a free source of high-resolution pictures standardised and validated via subjective evaluations and objective physical measurements. In our case, the results of subjective ratings classify the picture as a man, rated between Latino and white ethnic origin, aged around 43 years, with a neutral expression. The use of a fixed picture contributed to increase the credibility of the task while holding a broad range of conditions (gender, age and emotional facial expressions) constant across all respondents.
The experiment manipulated three conditions, providing us with a 2 × 2 × 2 full factorial design in which each hypothetical scenario presented the respondents with varying information about the migrant's qualification (low skilled or skilled), the reasons for leaving his country (looking for job or fleeing from war) and his ethnic origins (Syrian or Ukrainian). Differently from Iyengar et al . ( Reference Iyengar, Jackman, Messing, Valentino, Aalberg, Duch, Hahn, Soroka, Harell and Kobayashi 2013 ) and Valentino et al . ( Reference Valentino, Soroka, Iyengar, Aalberg, Duch, Fraile, Hahn, Hansen, Harell, Helbling, Jackman and Kobayashi 2019 ), the vignette did not specify what types of skills the applicant had, while it introduced the reason for migrating. When it comes to the country of origin, the vignette included two ethnic groups among which we might have asylum applicants, Syria and Ukraine being two contexts of conflict at the time of fieldwork. Moreover, the former constitutes a Muslim-majority country while the latter a Christian-majority one. Last, Ukrainians are a more familiar type of foreign immigrants in Italy, being the 5th most represented group out of 169 nationalities present in the country, than Syrians, who rank 69th (ISTAT 2017 ). This should elicit different degrees of cultural contrast between the two groups (higher for Syrians and lower for Ukrainians). Table 1 summarises all the experimental conditions and lists the number of respondents assigned to each group. Finally, after reading the scenario, the respondents had to express whether the migrant's application for asylum ought to be approved or rejected, so answers were collected in a dichotomous format, mimicking a real-world choice by a public official.
Table 1. Randomly assigned conditions in the factorial experiment
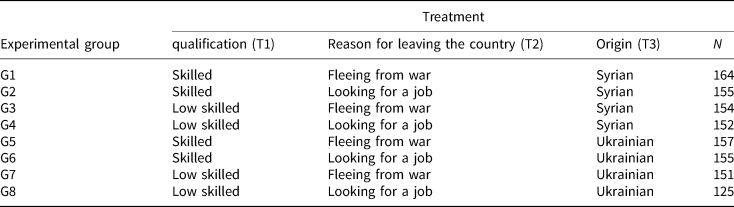
To check for the robustness of the random assignment, we performed balance tests by multinomial regression, regressing assignment to a certain experimental group on a set of socio-demographic characteristics (gender, age, educational attainment). Moreover, since our experiment involves the respondents' reaction to the admission of asylum seekers, we also checked for balance in ideology, party identity and attitudes towards immigration. The results confirm that the random procedure was correct with no variable being statistically different across the treatment groups (see Appendix C). Hence, any difference between conditions should be attributed to treatment manipulation only and not to other confounding factors.
It has to be noticed that this was not the only experiment included in the survey. In fact, other two experiments on the topics of the economy and globalisation were present, implying potential spill-overs among the three. However, the experiments were presented in a randomised order, a procedure that, as discussed above, can alleviate this type of bias.
Empirical analysis
To begin, it is worth mentioning that the general approval rate of the proposed asylum application is fairly high, with around 63% of respondents willing to accept the assigned request. This result aligns with studies conducted in other countries (Iyengar et al ., Reference Iyengar, Jackman, Messing, Valentino, Aalberg, Duch, Hahn, Soroka, Harell and Kobayashi 2013 ; Valentino et al ., Reference Valentino, Soroka, Iyengar, Aalberg, Duch, Fraile, Hahn, Hansen, Harell, Helbling, Jackman and Kobayashi 2019 ), though we take it with caution since it comes from a non-representative sample.
Given that our dependent variable is dichotomous in format, we estimated a logistic regression model. By reason of the experimental setting, we do not need to build a complex model with a battery of control variables. Rather, we identify the effects of our treatments by plugging dummy variables in as well as their interactions. For the sake of simplicity, we display results showing predicted probabilities of approval of asylum applications as a function of our covariates. To get the ATEs, we computed average marginal effects and performed Wald tests (formal notations and full models are reported in Appendix D).
We start by considering the main effect of our three treatments as they stand alone. It should be noted that approval clearly depends on profile features, confirming our first expectation (h1). Specifically, skilled applicants have 18% more probability to get accepted than non-skilled applicants ( χ 2 = 45.45; P < 0.001). Similarly, people fleeing from war have 17% more probability to see their application approved than individuals coming to Italy to find a job, thus corroborating our second anticipation (h2) ( χ 2 = 43.37; P < 0.001). Last, contrary to our third expectation (h3), a Syrian applicant has higher chances to be granted asylum, though the difference is small and non-significant (5% difference; χ 2 = 3.38; P = 0.065) (for a graphical representation, see Appendix D).
However, each of these values constitutes the effect of a single treatment on the dependent variable averaged across the levels of the other experimental conditions. The second step of our analysis is to explore the interplay among our three independent variables. The idea, here, is to test whether the gap in the approval rate between applicants escaping from war and those looking for better economic conditions is reduced when the applicant is skilled and from a Christian-majority country. Figure 3 shows the results of our model plotting the effects of two conditions – the level of qualification and the reason for leaving the country – and split them into two panels, depending on whether the potential refugee is from Syria (left panel) or from Ukraine (right panel).
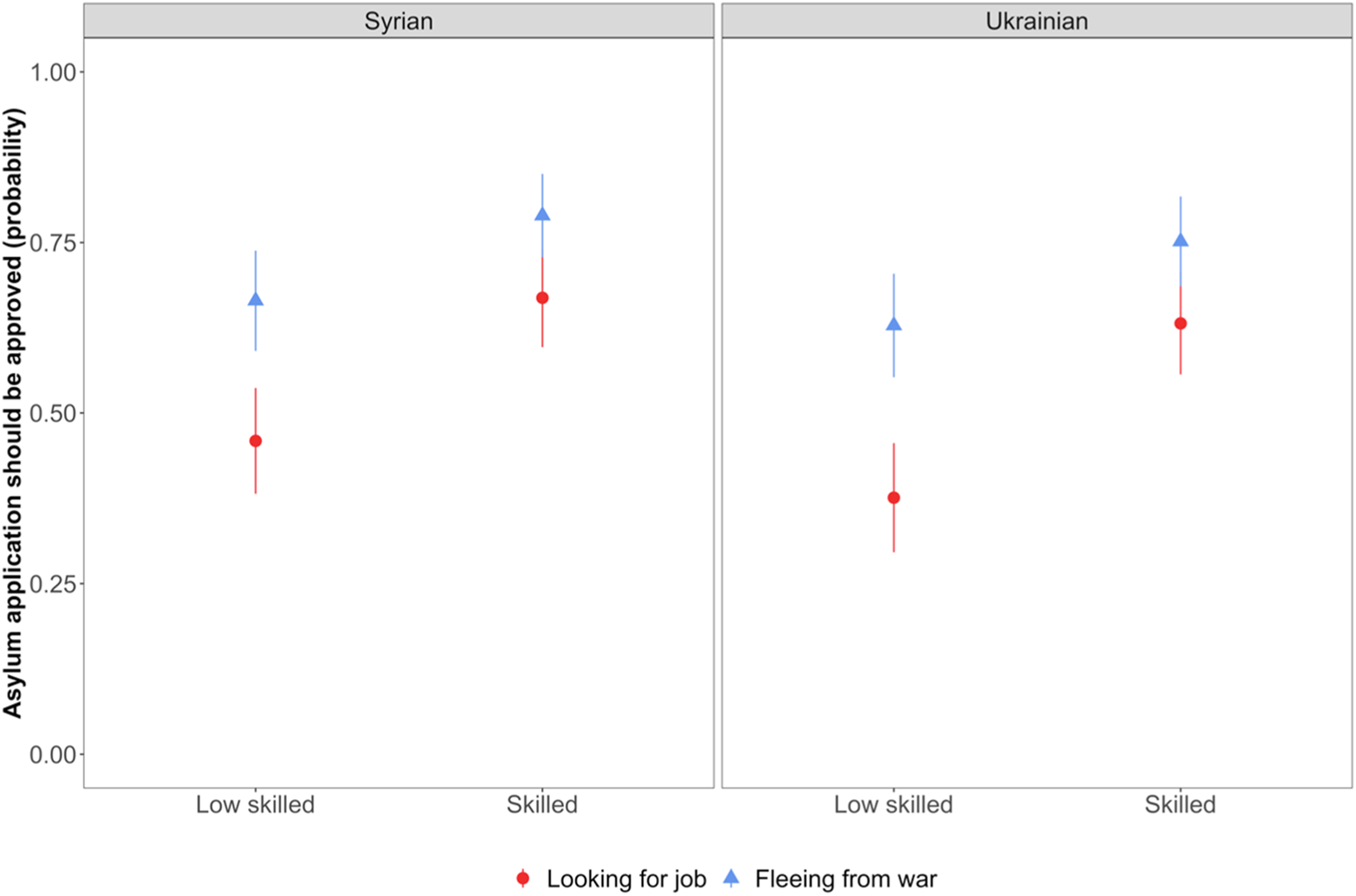
Figure 3. The effect of skills and reasons for leaving the country on the probability to accept asylum applications by immigrant's ethnic group.
Note : Graph shows predicted probabilities based on logit regression. Lines on both sides of the points represent 95% confidence intervals.
In the case of a Syrian applicant, we do not find a statistically significant interaction effect between skills and motivation for migrating. A detailed examination of the predicted probabilities from the combination of treatment conditions reveals that approval rates are always higher when the applicant has a humanitarian reason for requesting asylum (fleeing from war) compared to those who come for economic opportunities (looking for job). This is so for an unskilled applicant escaping from a war context, who has 20 percentage points ( χ 2 = 14.20; P < 0.001) more probability to get a final approval than a similar unskilled applicant looking for a new job position. Similarly, skilled applicants fleeing from a conflict have more chances of being approved than skilled individuals who are job seeking (12% difference; χ 2 = 6.26; P < 0.05). Moreover, if we keep the reason of moving for economic opportunities as a reference and compare low skilled and skilled individuals, this increases the probability of the latter of being accepted (21% higher for skilled refugees; χ 2 = 15.04; P < 0.001); yet, not enough to close the gap with skilled subjects coming for political reasons.
Turning to the case of an Ukrainian applicant, we find the same pattern, meaning that we do not find an interaction between qualification and reason for leaving the country, a result that also extends to the ethnic origins. Footnote 5 Shortly, in contrast with our fourth expectation (h4), we might say that skills temper the effect of migrating for economic opportunities; however, this effect is not strong enough to significantly reduce the gap in the acceptance rate of skilled applicants moving for humanitarian reasons.
We conclude by examining whether the effects of the applicant's skills, reason for migrating and ethnicity are moderated by the ideology of the respondent. Figure 4 displays the results of a two-way interaction model between our treatments and the ideological positioning of the respondent. As we can see, although left-wing participants tend to express higher levels of approval than right-wing respondents, we do not find different patterns for ideology. In fact, when the applicant is either skilled or migrates for humanitarian reasons, approval rates improve to the same degree across ideological groups, so we do not detect any larger or smaller gap in these conditions depending on ideology, rejecting our expectations (h5a, h5b). When it comes to the ethnicity of the applicant, in contrast with our last hypothesis (h5c), rightists do not seem to be more sensitive to the Muslim−Christian divide as far as the two selected national groups are concerned. Footnote 6
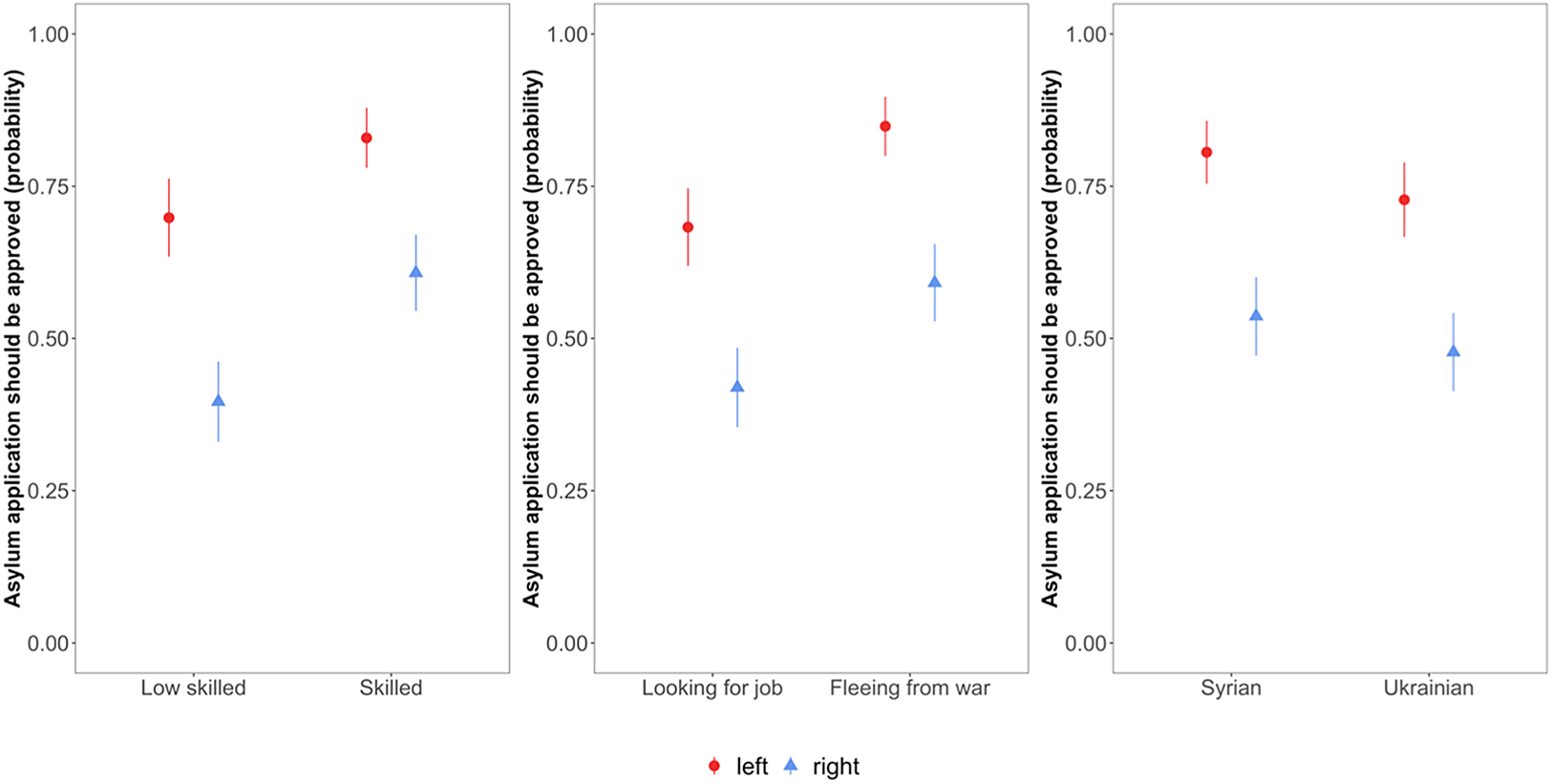
Figure 4. The effect of skills and reasons for leaving the country on the probability to accept asylum applications by respondent's ideology.
To sum up, in line with previous studies (Iyengar et al ., Reference Iyengar, Jackman, Messing, Valentino, Aalberg, Duch, Hahn, Soroka, Harell and Kobayashi 2013 ; Bansak et al ., Reference Bansak, Hainmueller and Hangartner 2016 ; Valentino et al ., Reference Valentino, Soroka, Iyengar, Aalberg, Duch, Fraile, Hahn, Hansen, Harell, Helbling, Jackman and Kobayashi 2019 ), Italians take into account both instrumental and humanitarian motives when asked about specific individual applications for asylum. However, these factors do not seem to interact among each other, neither they do with the migrant's ethnic origins, which, at least in our experiment, turn out being irrelevant. Nor we do find an interaction between ideology and reasons behind approving a hypothetical application for asylum, so future research should be conducted on this link. Last, our results hold to some robustness checks (e.g., removal of speeders, weighting, controlling for possible spill-over effects among experiments in the same survey; see Appendix D).
Personalisation of politics, that is, the gradual shift in the electorate's attention from political parties and issues to specific candidate features, has been the result of broad underlying social and political processes, including the individualisation of social life, the de-freezing of traditional cleavages and the emergence of parties as campaign organisations in a new media environment (Costa Lobo and Curtice, Reference Costa Lobo and Curtice 2015 ). These patterns have prompted observational research to look at the role of candidate features to explain voting choices, unravelling the importance of some basic traits, among which: competence (being intelligent and knowledgeable), leadership (being inspiring), integrity (being honest) and empathy (being compassionate and caring) (Pancer et al ., Reference Pancer, Brown and Barr 1999 ).
The topic has become even more relevant with the success of (neo-)populist parties and leaders. In the ideal type, populist voters would attribute larger importance to valence issues (e.g., corruption) (Curini, Reference Curini 2018 ), oppose professional politicians (Akkerman et al ., Reference Akkerman, Mudde and Zaslove 2014 ), favour candidates who act as ‘delegates’ (who care only about the interests of her/his electorate) rather than ‘trustees’ (who are independent and care about the interests of the nation), and, finally, advocate strong leadership (Caramani, Reference Caramani 2017 ).
However, existing research needs to address some relevant problems. First, standard survey measures are prone to social desirability bias, with respondents likely to score as important all the above-mentioned personality traits. Second, interviewees are usually asked to rate each trait individually and not to evaluate candidate profiles characterised by both more and less positive features. In fact, politicians' profiles are multidimensional in nature and that explains the proliferation of conjoint analysis on candidate preferences (e.g., Teele et al ., Reference Teele, Kalla and Rosenbluth 2018 ; Franchino and Zucchini, Reference Franchino and Zucchini 2015 ; for a critical view, see Incerti, Reference Incerti 2020 ).
Still, none of the available studies have analysed extensively the role of personality or valence traits suggested by observational research. Footnote 7 Therefore, one possible conjoint experiment on the topic could explore what personality traits make a politician a good candidate in the eyes of the citizens and whether the importance of these traits vary across subgroups of voters based on their populist attitudes. Drawing on the available theoretical and empirical research, we can develop some expectations.
First, we might expect Italians to rate more favourably candidates who have higher levels of competence than those who have less (h1); who show higher moral integrity than apparently dishonest candidates (h2); who are more compassionate than cold and distant (h3). Second, we anticipate populist attitudes to moderate the importance of some traits on candidate favourability. Specifically, we hypothesise populist citizens to dislike professional politicians more than non-populists (h4a). Moreover, as compared to non-populist voters, populists will more likely favour candidates with high moral integrity (h4b), strong leadership (h4c) and acting as delegates (h4d).
Our data come from a panel survey carried out by the Department of Social, Political and Cognitive Sciences at the University of Siena and collected on a sample of the Italian population aged 14 years or older selected within a probability panel held and managed by GfK Italy. The first wave of the survey ( n = 3411) was conducted between 6 and 25 May, 2019, right before the last European elections and included the key covariate used in this example, that is, a scale eliciting populist attitudes. The second wave ( n = 3179) was administered in the post-electoral period, from 28 May to 26 June 2019, and contained both the populist scale and the conjoint experiment. We restrict our analysis to subjects aged 18 years or over at the time of the interview ( n = 3096). When conducting sub-group analysis on populism, we primarily use the populist scale included in the second wave, conducting some robustness tests with the first-wave measure and concentrating on the adult respondents who participated in both waves. Since we have a probability sample resembling the general population on several socio-demographic features – albeit more skewed towards the highly educated (see Appendix C) – we have decided to run models on weighted data.
To minimise the effect of response fatigue on the quality of our experimental data and eliminate potential spill-over effects, the conjoint experiment was embedded at the beginning of the questionnaire after a few introductory questions. This is a forced, paired-choice, fully randomised conjoint with discrete and rating choices. Respondents began the experiment by reading a short introduction in which they were invited to reflect about ‘the characteristics a candidate should have to enter politics at the European level’, and then informed that they would have been provided ‘with several pieces of information about people who might have run for the European elections’. Then, for each pair of hypothetical politicians, participants were asked ‘which of the two candidates would you personally have preferred to win a seat in the European Parliament’. The experiment builds on the one proposed by Hainmueller et al . ( Reference Hainmueller, Hopkins and Yamamoto 2013 ). Figure 5 shows one possible conjoint vignette generated through random assignment to the considered macro-traits and relative attributes (for the full stimuli, see Appendix B).
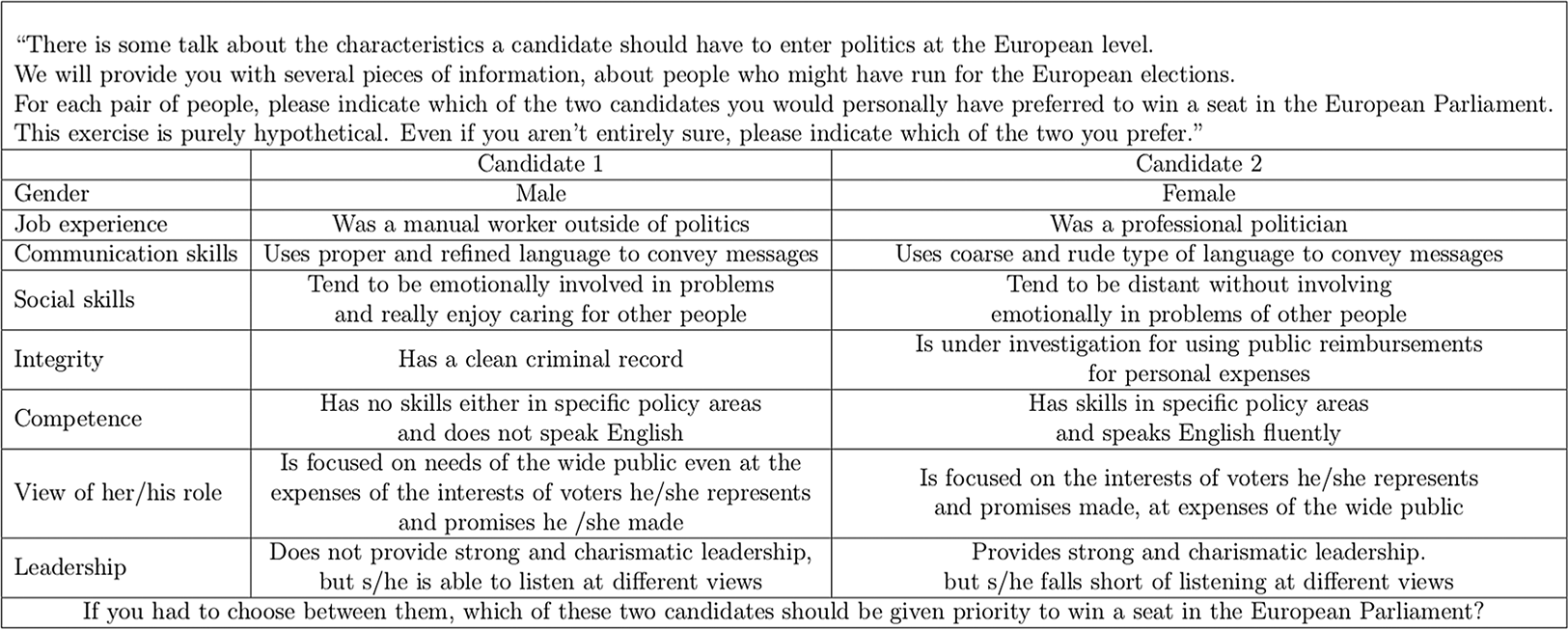
Figure 5. Stimuli: an illustration of the conjoint experiment.
Since respondents had to choose either one or the other candidate, the outcome variable is dichotomous, coming close to a real-world situation in European elections, at least in Italy, in which voters can express a preference among a list of pre-selected candidates. After this choice, participants were also asked to rate each profile on a 7-point favourability scale.
Overall, we manipulated eight macro-traits, implying that our hypotheses are tested in a broad context of candidate features. Moreover, each respondent was exposed to two pairs of candidates, therefore facing the same task twice. Two traits elicited basic sociodemographic characteristics, such as the role of gender and job position. Five traits considered personality features and skills, namely: communication skills, social skills, integrity, competence and leadership. The remaining trait elicited view of role (see Appendix B for the full list of attributes).
Profiles were generated so that the order of macro-traits was randomised and fixed across the two pairings to minimise recency effect and priming. The assignment of attributes, instead, followed an independent fully randomised approach, meaning that all attributes were randomly assigned without restrictions on their possible combination. To check for the correct implementation of the experiment, we first analysed the distribution of considered attributes in the sample – results ensure fair distribution – and then tested for balance in our main covariate (the populist scale) – results suggest that imbalance should not be a matter of concern (see Appendix B).
In our experiment, 2676 respondents rated 10,704 profiles (5352 pairings), with a design yielding 1536 possible profile combinations. In conjoint analysis, as mentioned above, the causal quantity of interest is the average marginal component effect (henceforth AMCE). In our example, this corresponds to the average difference in the probability effect of being preferred for winning a seat in the European Parliament when comparing two different attribute levels – e.g., a candidate with a ‘clean criminal record’ versus a candidate being ‘under investigation’ – while keeping all other attributes constant. Since attributes are randomised, profiles with a ‘clean criminal record’ will have, on average, the same distribution on all other attributes as compared to profiles ‘under investigation’ (as we positively tested). In the subsequent analysis, the dependent variable will be a dichotomous variable measuring people's choice.
Following Hainmueller et al . ( Reference Hainmueller, Hopkins and Yamamoto 2013 ), we estimated a linear probability model to assess the role of the different profile traits and the relative assigned attributes on people's candidate choice. In this case, the explanatory variables are a series of dummies for each of the attributes of the macro-traits under consideration. Since each participant carried out two different tasks, observations are not independent, so we clustered standard errors by respondent. As said, AMCE conveys information on the marginal causal effect of an attribute against a reference category. Following Leeper et al . ( Reference Leeper, Hobolt and Tilley 2019 ), we also computed unadjusted marginal means (henceforth MM) to give a more detailed description of the respondents' preference for all feature levels. Again, we will only show graphical results to ease interpretation (see Appendix D for full models).
Which of the considered traits does meet the favourability of the respondents, increasing the probability of choosing a certain candidate? Figure 6 shows the AMCE of each attribute against the baseline, so that when the point estimate and confidence intervals cross the zero line, the attribute has no effect. When the coefficient leans towards the right, taking positive values, it produces a positive change in the probability of choosing a certain candidate. On the contrary, when the coefficient leans towards the left, taking negative values, it yields a negative change in the probability. As can be seen, being a woman does not exert an effect compared to being a man. Conversely, many job positions outside politics – manual worker ( + 6%, P < 0.01), engineer ( + 5%, P < 0.01), university professor ( + 4%, P < 0.01) – increase the probability of being chosen if compared with a professional politician. This would confirm a negative bias towards a long-term political career.
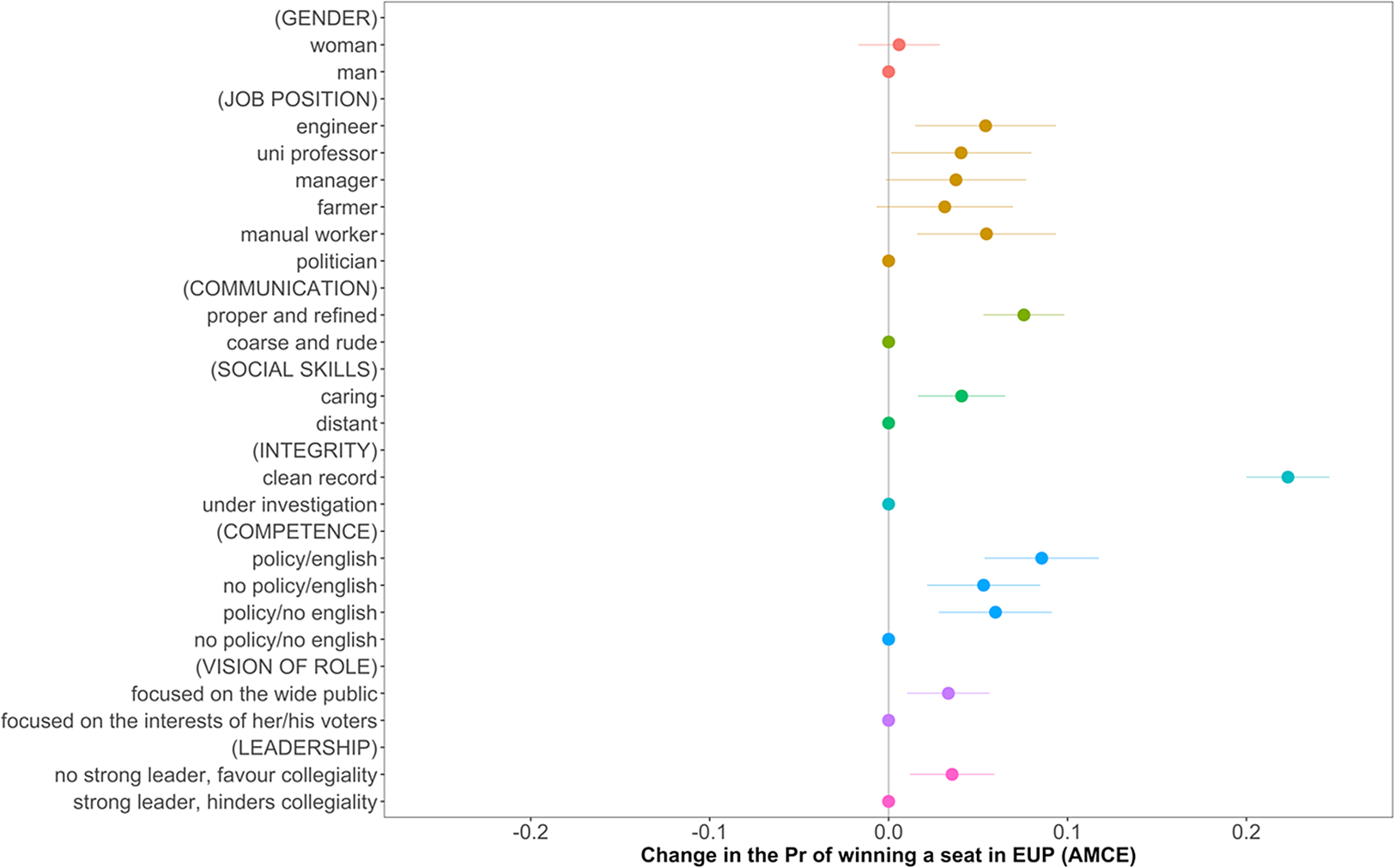
Figure 6. Average marginal component effect: effects of candidate traits on preference for election.
Note : Lines on both sides of the points represent 95% confidence intervals. The points without horizontal bars denote the attribute value used as a reference category.
Considering the way a candidate might conceive her/his role, being a trustee, who is focused on the national interest, seems to be favoured over a candidate who interprets the role as a delegate focused on the mere interest of her/his voters ( + 3%, P < 0.01). Similarly, a candidate who favours collegiality is preferred over a strong leader ( + 4%, P < 0.01), and, reasonably, proper and refined communication skills exert a positive effect on candidate selection ( + 8%, P < 0.001).
Coming to the traits on which our attention is focused, competence increases the probability of being favoured as compared to less expertise in specific policies or fluency in English ( + 6%, P < 0.001). Moreover, caring about the problems of other people and being emotionally involved exert a positive effect on candidate selection as compared to the baseline category for this trait ( + 4%, P < 0.01). Still, the most important trait by far is integrity, with candidates showing a clean criminal record being supported much more than those under investigation. The increase in probability is equal to 22 percentage points, a strong and statistically significant effect ( P < 0.001). Overall, our first three expectations (h1, h2, h3) are corroborated, albeit with differences in the magnitude of effects. All these conclusions are largely substantiated by MM results (see Appendix D).
Now, are these results conditioned by individuals' populist attitudes? To evaluate this, we first need to distinguish our respondents according to their level of populism. To do this, we rely on a scale developed by Akkerman et al . ( Reference Akkerman, Mudde and Zaslove 2014 ) and derived from a six-item battery aimed at capturing a latent attitudinal dimension characterised by three main aspects: people-centrism, anti-elitism and Manichaeism. We tested it via factor analysis and computed factor scores to get a synthetic measure of populism (full results are reported in Appendix D). Then, we ran separate models for respondents who were either above or below the median value of the resulting populist score to gauge whether the effect of attributes changed according to their level of populism.
Figure 7 summarises the results in three panels. Moving from left to right, it displays the AMCEs of attributes when populism is high, low and the difference between the two, thus allowing to detect any subgroup difference. The first thing we can notice is that, if compared with non-populist respondents, populists seem to favour candidates with some job experience (especially farmers, manual workers) over professional politicians. Looking at MMs (Appendix D), however, this is not the product of a strong preference for working-class positions or manual jobs, but of a general disapproval of professional politicians. Specifically, populists tend to punish this type of candidates 7% more than non-populists ( P < 0.01), confirming our hypothesis (h4a). Moreover, populists appear to be more sensitive to moral integrity, with a 7% increase in the probability of choosing a candidate with a clean criminal record over a candidate under investigation, compared to non-populists. Therefore, also in this case, our expectation is corroborated (h4b). On the other hand, against popular accounts (h4c and h4d), we do not find populists to prefer strong leaders or candidates who act as delegates.
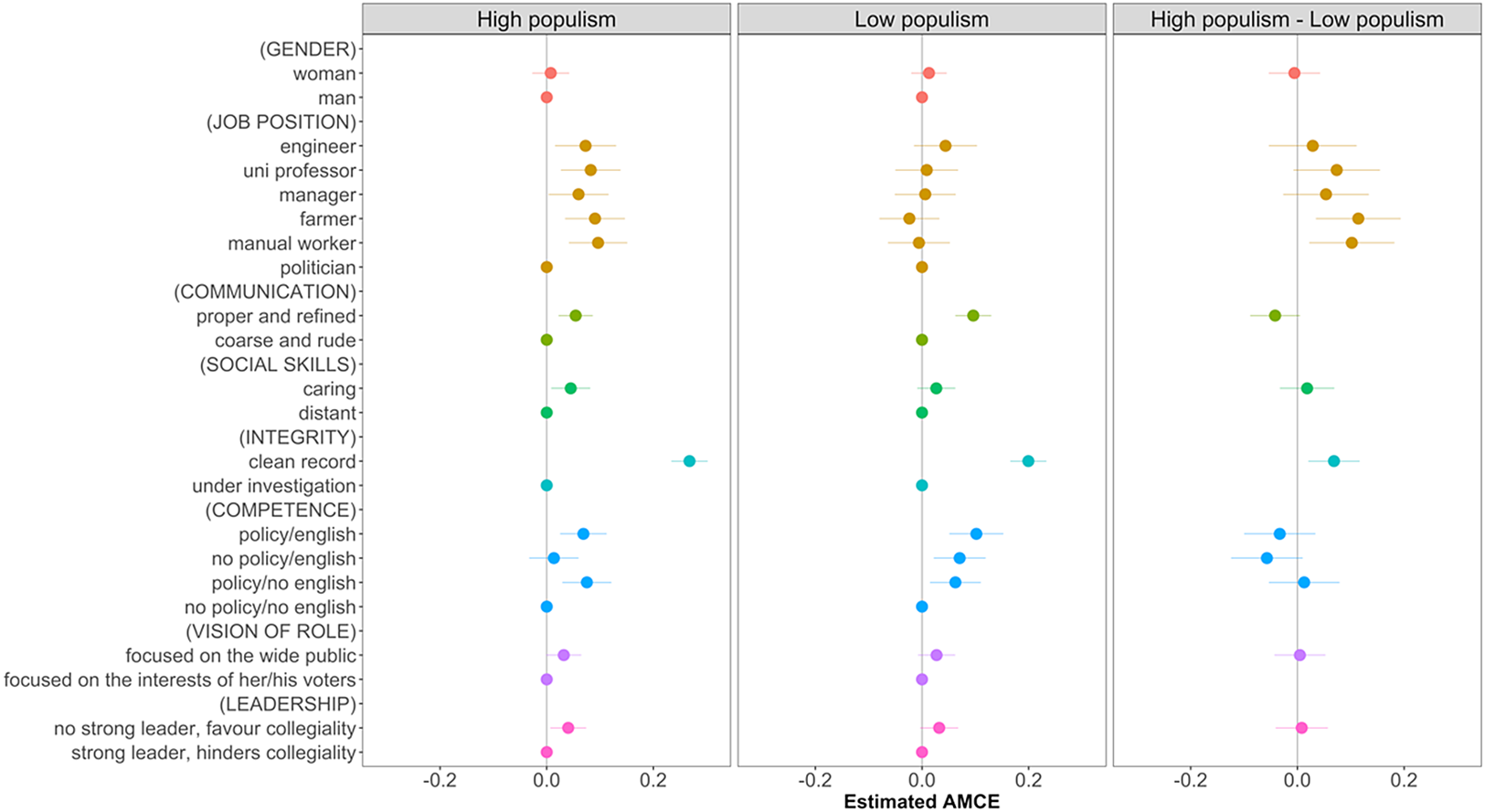
Figure 7. Average marginal component effect for populists and non-populists.
We might conclude that citizens take into account personality traits eliciting valence features when evaluating candidate fit for elections. These have to do with communication and social skills, view of the role and leadership. There is an opposition towards professional politicians and, in line with previous research (Franchino and Zucchini, Reference Franchino and Zucchini 2015 ), moral integrity is by far the most important aspect, with both results more pronounced in the case of populist respondents. A series of robustness checks confirm the reliability of our results (i.e., changing the way we measure the dependent variable, removing subjects depending on their level of attention during the survey, performing sub-group analysis using a measure of populism from the first survey wave, handling randomisation problems; see Appendix D).
The experimental approach constitutes the prime method in the quest for causality. Nowadays, political scientists willing to embark in experimental research may take advantage of a growing number of studies and choose the strategy that best fits their objectives among a wide menu of designs and settings. Of course, none of these options is free of limitation, so that doing experiments needs a good deal of creativity together with a deep awareness of the potential trade-offs between the control of the experimental setting and the external validity of the results.
This article has tried to give an overview of the basic concepts underneath the experimental method, highlighting the amount of scholarly interest in the field and possible designs to use. It has addressed the main differences among various experimental settings and discussed the main applications and potential problems in the use of survey experiments in particular. When combining randomised assignment and representative samples, survey experiments allow the researcher to make population inferences about causal relationships between variables of interest. Yet, survey experiments are not necessarily the final remedy for the study of causality and researchers should problematise each single step in their design, planning and implementation. For practical guidance, we have presented the full protocol for a traditional factorial design on individuals' attitudes towards migrants and a more innovative conjoint experiment on candidate preferences, including a set of research questions, the experimental stimuli used to address them, and the way to analyse experimental data.
The exposure and acquaintance of political scientists to experimental methods have gradually fuelled experimental publications, with a rapid spread in the number and influence of these manuscripts in the last few decades. We hope that this study, besides offering a general overview on the merit, success and use of experimental designs in international scholarly literature, will contribute to stimulate an increasing interest and usage of the experimental method among the Italian political science community. Bearing in mind the limitations that we have outlined, we can now take advantage of the possibility of experimentation in political research and shook off the idea that the study of politics is only an observational science.
Supplementary material
The supplementary material for this article can be found at https://doi.org/10.1017/ipo.2021.20
This research received financial support from the project ‘Bridging the gap between public opinion and European leadership: Engaging a dialogue on the future path of Europe EUENGAGE’ (H2020-EURO-2014-2015/H2020-EURO-SOCIETY-2014, Grant no. 649281) funded by the European Union's Horizon 2020 research and innovation programme ( www.euengage.eu ) and the Department of Excellence 2018-2022 (2272-2018-IA-PROFCMIUR001) ( https://interdispoc.unisi.it/en/ ). No conflict of interest.
The replication dataset is available at http://thedata.harvard.edu/dvn/dv/ipsr-risp
Acknowledgments
We thank James N. Druckman for having shared with us the list of experimental articles published by the American Political Science Review over the last six decades and for his encouragement and support in our comparative project on experimental research in Europe and the United States. We also thank Mattia Guidi and Thomas Leeper for their feedback on the analysis. Usual disclaimers apply.
1 This way, experiments address the three requirements for causal inference: (1) identifying a statistically significant association between two conditions; (2) establishing a precise temporal order between cause and effect; (3) avoiding the observed relationship to be confounded by third variables (Mutz Reference Mutz 2011 ). On the potential outcome approach and the Neyman−Rubin casual model, see Druckman et al. ( Reference Druckman, Green, Kuklinski, Lupia, Druckman, Green, Kuklinski and Lupia 2011 ).
2 Trials in which units are not randomly assigned, but where the ‘researcher can credibly claim that treatment is as good as randomized’ (Dunning, Reference Dunning 2012 : 16), are referred as ‘as-if’ randomised natural experiments. These quasi-natural designs are not usually considered ‘true’ experiments (Druckman et al., Reference Druckman, Green, Kuklinski and Lupia 2006 ).
3 For further details on the criteria to select published manuscripts, see Appendix A.
4 Similarly to what Druckman et al . ( Reference Druckman, Green, Kuklinski and Lupia 2006 , Reference Druckman, Green, Kuklinski, Lupia, Druckman, Green, Kuklinski and Lupia 2011 ) did for the American case, we selected the official, longest-running publication of the leading scholarly society for political scientists in Europe (European Consortium for Political Research) under the assumption that if experiments are being published in EJPR, experimental methods are also being accepted by other specialty journals; see McGraw and Hoekstra ( Reference McGraw, Hoekstra, Delli Carpini, Huddy and Shapiro 1994 ) on this point.
5 For the right-hand panel (Ukrainian), the differences are: unskilled looking for job vs. fleeing from war = 25% ( χ 2 = 20.14; P < 0.001); skilled looking for job vs. fleeing from war = 12% ( χ 2 = 5.59; P < 0.05); unskilled looking for job vs. skilled looking for job = 26% ( χ 2 = 20.91; P < 0.001).
6 The differences between skilled and unskilled is 13% for leftists and 21% for rightists, though the contrast is not statistically significant ( χ 2 = 1.69; P = 0.19); the difference between looking for job vs. fleeing from war is 16.6% for leftists and 17.2% for rightists ( χ 2 = 0.01; P = 0.92); the difference between Syrian vs. Ukrainian fleeing from war is −0.08% for leftists and −0.05% rightists ( χ 2 = 0.10; P = 0.76).
7 For a factorial experiment on candidate favourability in Italy, see Iyengar and Barisione ( Reference Iyengar and Barisione 2015 ).
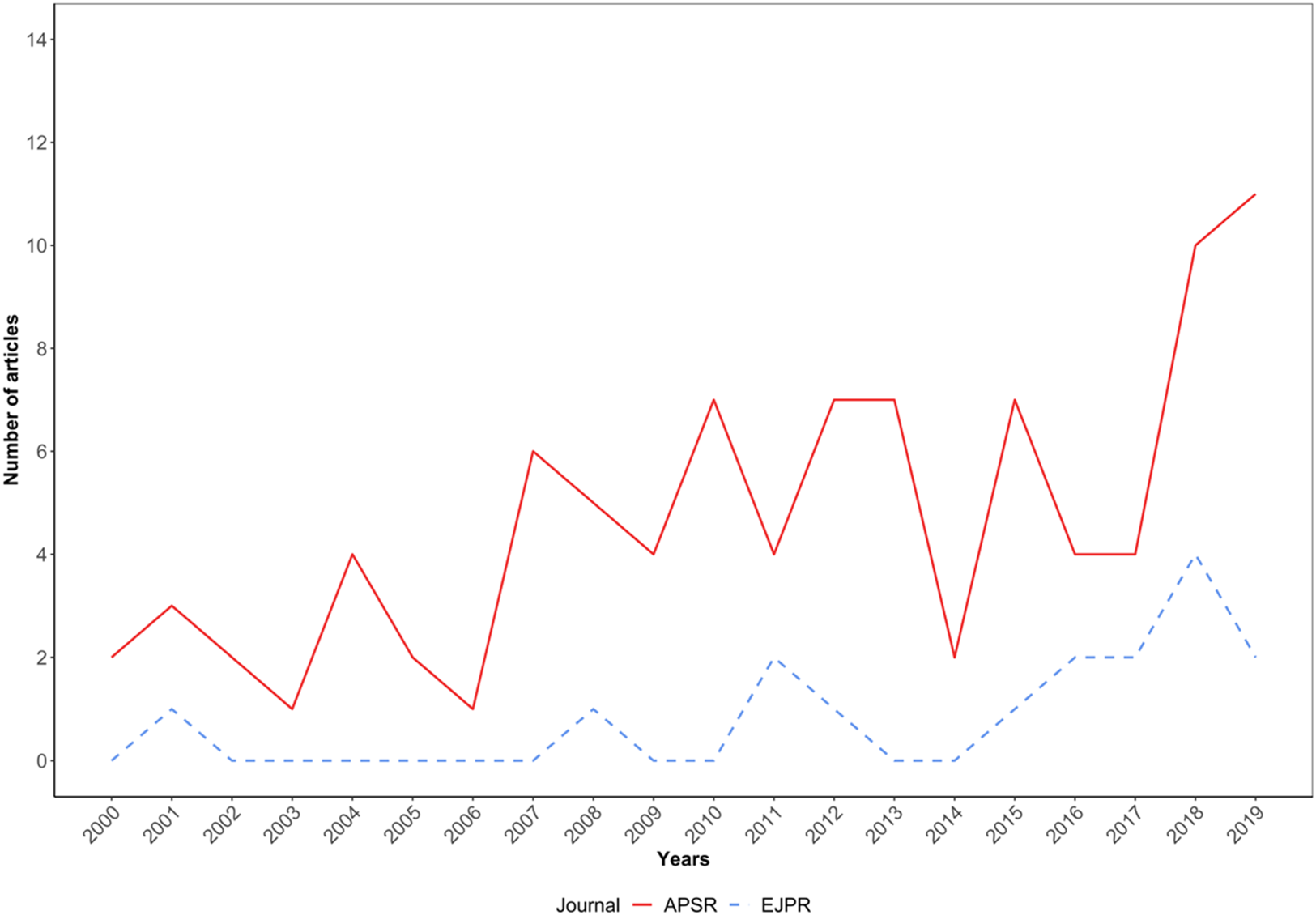
Figure 3. The effect of skills and reasons for leaving the country on the probability to accept asylum applications by immigrant's ethnic group. Note : Graph shows predicted probabilities based on logit regression. Lines on both sides of the points represent 95% confidence intervals.
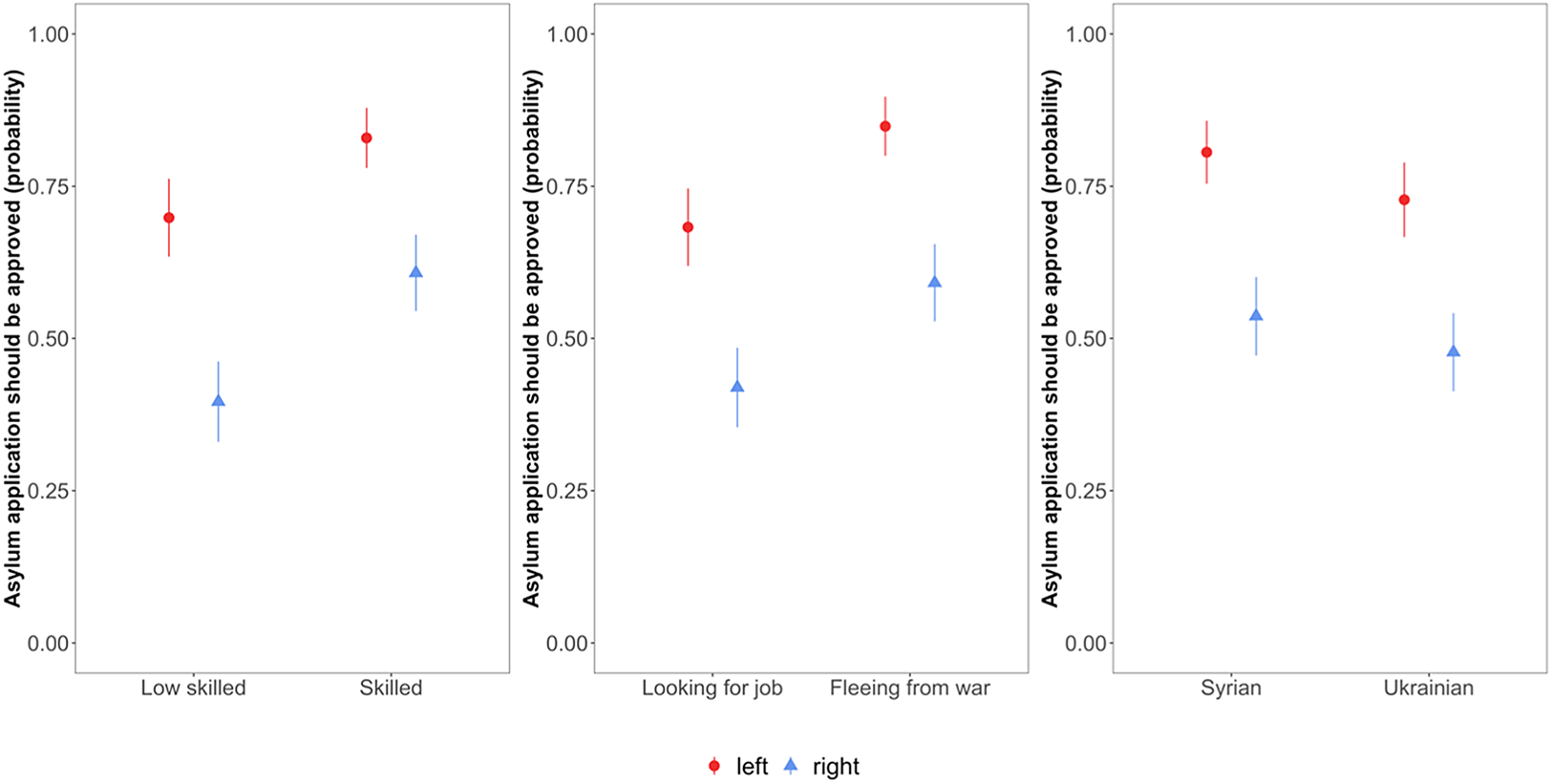
Figure 4. The effect of skills and reasons for leaving the country on the probability to accept asylum applications by respondent's ideology. Note : Graph shows predicted probabilities based on logit regression. Lines on both sides of the points represent 95% confidence intervals.
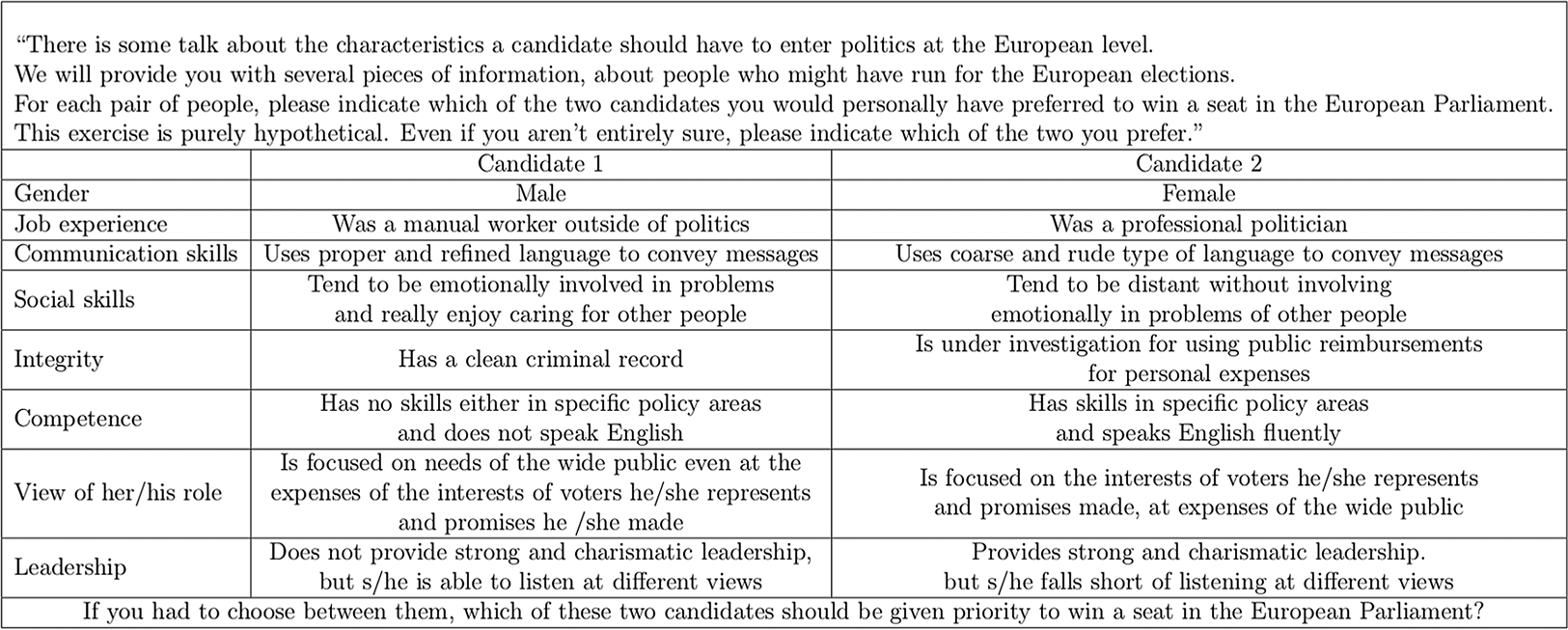
Figure 6. Average marginal component effect: effects of candidate traits on preference for election. Note : Lines on both sides of the points represent 95% confidence intervals. The points without horizontal bars denote the attribute value used as a reference category.
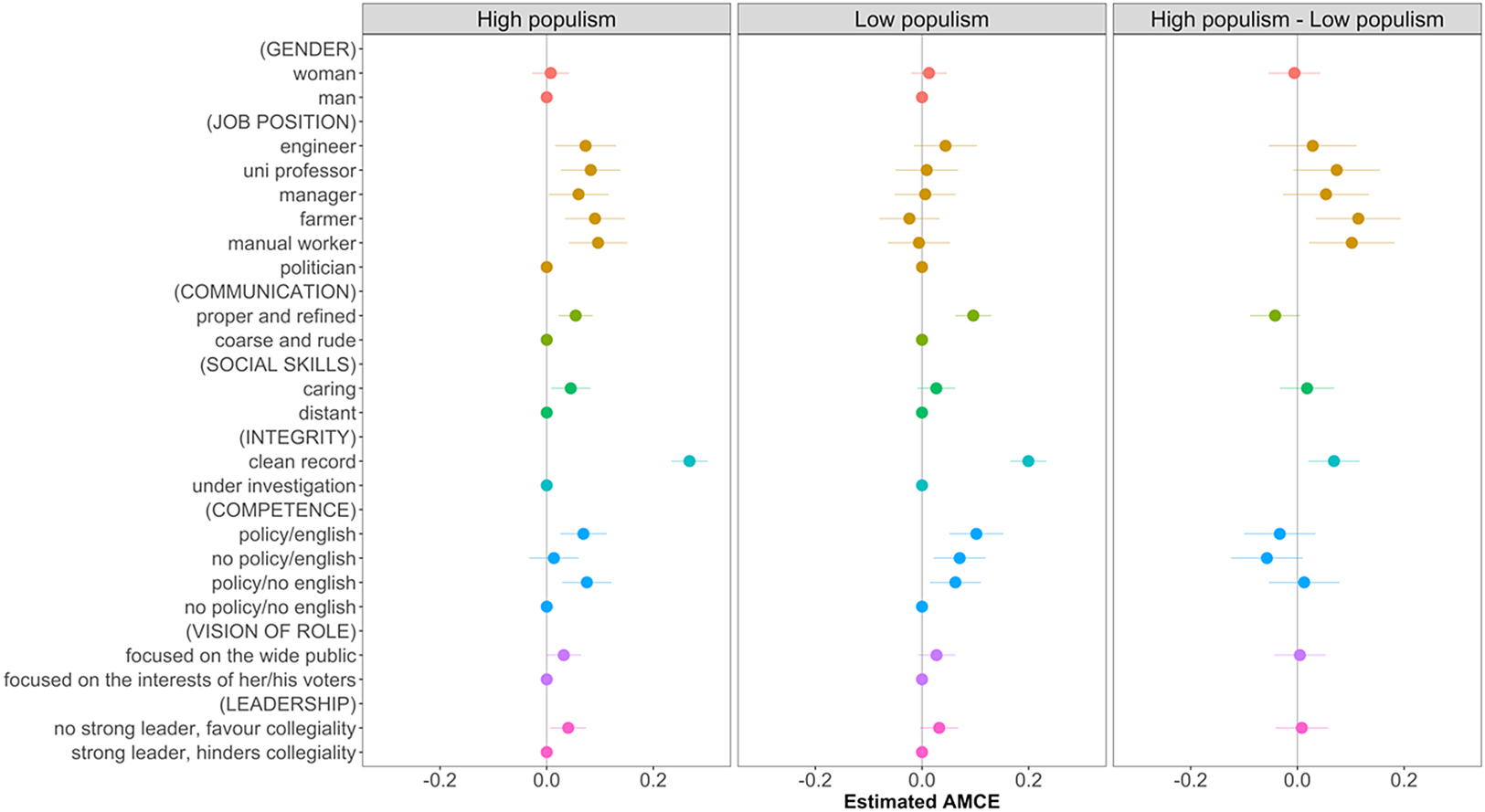
Figure 7. Average marginal component effect for populists and non-populists. Note : Lines on both sides of the points represent 95% confidence intervals. The points without horizontal bars denote the attribute value used as a reference category.
Martini and Olmastroni Dataset
Martini and Olmastroni supplementary material
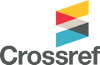
This article has been cited by the following publications. This list is generated based on data provided by Crossref .
- Google Scholar
View all Google Scholar citations for this article.
Save article to Kindle
To save this article to your Kindle, first ensure [email protected] is added to your Approved Personal Document E-mail List under your Personal Document Settings on the Manage Your Content and Devices page of your Amazon account. Then enter the ‘name’ part of your Kindle email address below. Find out more about saving to your Kindle .
Note you can select to save to either the @free.kindle.com or @kindle.com variations. ‘@free.kindle.com’ emails are free but can only be saved to your device when it is connected to wi-fi. ‘@kindle.com’ emails can be delivered even when you are not connected to wi-fi, but note that service fees apply.
Find out more about the Kindle Personal Document Service.
- Volume 51, Special Issue 2
- Sergio Martini (a1) and Francesco Olmastroni (a1)
- DOI: https://doi.org/10.1017/ipo.2021.20
Save article to Dropbox
To save this article to your Dropbox account, please select one or more formats and confirm that you agree to abide by our usage policies. If this is the first time you used this feature, you will be asked to authorise Cambridge Core to connect with your Dropbox account. Find out more about saving content to Dropbox .
Save article to Google Drive
To save this article to your Google Drive account, please select one or more formats and confirm that you agree to abide by our usage policies. If this is the first time you used this feature, you will be asked to authorise Cambridge Core to connect with your Google Drive account. Find out more about saving content to Google Drive .
Reply to: Submit a response
- No HTML tags allowed - Web page URLs will display as text only - Lines and paragraphs break automatically - Attachments, images or tables are not permitted
Your details
Your email address will be used in order to notify you when your comment has been reviewed by the moderator and in case the author(s) of the article or the moderator need to contact you directly.
You have entered the maximum number of contributors
Conflicting interests.
Please list any fees and grants from, employment by, consultancy for, shared ownership in or any close relationship with, at any time over the preceding 36 months, any organisation whose interests may be affected by the publication of the response. Please also list any non-financial associations or interests (personal, professional, political, institutional, religious or other) that a reasonable reader would want to know about in relation to the submitted work. This pertains to all the authors of the piece, their spouses or partners.
- Key Differences
Know the Differences & Comparisons
Difference Between Survey and Experiment
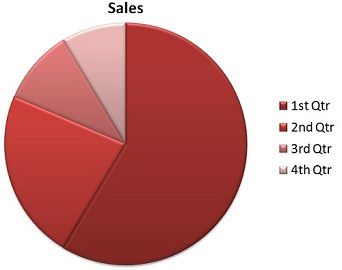
While surveys collected data, provided by the informants, experiments test various premises by trial and error method. This article attempts to shed light on the difference between survey and experiment, have a look.
Content: Survey Vs Experiment
Comparison chart.
Basis for Comparison | Survey | Experiment |
---|---|---|
Meaning | Survey refers to a technique of gathering information regarding a variable under study, from the respondents of the population. | Experiment implies a scientific procedure wherein the factor under study is isolated to test hypothesis. |
Used in | Descriptive Research | Experimental Research |
Samples | Large | Relatively small |
Suitable for | Social and Behavioral sciences | Physical and natural sciences |
Example of | Field research | Laboratory research |
Data collection | Observation, interview, questionnaire, case study etc. | Through several readings of experiment. |
Definition of Survey
By the term survey, we mean a method of securing information relating to the variable under study from all or a specified number of respondents of the universe. It may be a sample survey or a census survey. This method relies on the questioning of the informants on a specific subject. Survey follows structured form of data collection, in which a formal questionnaire is prepared, and the questions are asked in a predefined order.
Informants are asked questions concerning their behaviour, attitude, motivation, demographic, lifestyle characteristics, etc. through observation, direct communication with them over telephone/mail or personal interview. Questions are asked verbally to the respondents, i.e. in writing or by way of computer. The answer of the respondents is obtained in the same form.
Definition of Experiment
The term experiment means a systematic and logical scientific procedure in which one or more independent variables under test are manipulated, and any change on one or more dependent variable is measured while controlling for the effect of the extraneous variable. Here extraneous variable is an independent variable which is not associated with the objective of study but may affect the response of test units.
In an experiment, the investigator attempts to observe the outcome of the experiment conducted by him intentionally, to test the hypothesis or to discover something or to demonstrate a known fact. An experiment aims at drawing conclusions concerning the factor on the study group and making inferences from sample to larger population of interest.
Key Differences Between Survey and Experiment
The differences between survey and experiment can be drawn clearly on the following grounds:
- A technique of gathering information regarding a variable under study, from the respondents of the population, is called survey. A scientific procedure wherein the factor under study is isolated to test hypothesis is called an experiment.
- Surveys are performed when the research is of descriptive nature, whereas in the case of experiments are conducted in experimental research.
- The survey samples are large as the response rate is low, especially when the survey is conducted through mailed questionnaire. On the other hand, samples required in the case of experiments is relatively small.
- Surveys are considered suitable for social and behavioural science. As against this, experiments are an important characteristic of physical and natural sciences.
- Field research refers to the research conducted outside the laboratory or workplace. Surveys are the best example of field research. On the contrary, Experiment is an example of laboratory research. A laboratory research is nothing but research carried on inside the room equipped with scientific tools and equipment.
- In surveys, the data collection methods employed can either be observation, interview, questionnaire, or case study. As opposed to experiment, the data is obtained through several readings of the experiment.
While survey studies the possible relationship between data and unknown variable, experiments determine the relationship. Further, Correlation analysis is vital in surveys, as in social and business surveys, the interest of the researcher rests in understanding and controlling relationships between variables. Unlike experiments, where casual analysis is significant.
You Might Also Like:
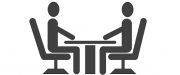
sanjay kumar yadav says
November 17, 2016 at 1:08 am
Ishika says
September 9, 2017 at 9:30 pm
The article was quite helpful… Thank you.
May 21, 2018 at 3:26 pm
Can you develop your Application for Android
Surbhi S says
May 21, 2018 at 4:21 pm
Yeah, we will develop android app soon.
October 31, 2018 at 12:32 am
If I was doing an experiment with Poverty and Education level, which do you think would be more appropriate for me?
Thanks, Chris
Ndaware M.M says
January 7, 2021 at 2:29 am
So interested,
Victoria Addington says
May 18, 2023 at 5:31 pm
Thank you for explaining the topic
Leave a Reply Cancel reply
Your email address will not be published. Required fields are marked *
Save my name, email, and website in this browser for the next time I comment.
- Tools and Resources
- Customer Services
- Contentious Politics and Political Violence
- Governance/Political Change
- Groups and Identities
- History and Politics
- International Political Economy
- Policy, Administration, and Bureaucracy
- Political Anthropology
- Political Behavior
- Political Communication
- Political Economy
- Political Institutions
- Political Philosophy
- Political Psychology
- Political Sociology
- Political Values, Beliefs, and Ideologies
- Politics, Law, Judiciary
- Post Modern/Critical Politics
- Public Opinion
- Qualitative Political Methodology
- Quantitative Political Methodology
- World Politics
- Back to results
- Share This Facebook LinkedIn Twitter
Article contents
The progress and pitfalls of using survey experiments in political science.
- Diana C. Mutz Diana C. Mutz Department of Political Science, University of Pennsylvania
- and Eunji Kim Eunji Kim Department of Political Science, University of Pennsylvania
- https://doi.org/10.1093/acrefore/9780190228637.013.929
- Published online: 28 February 2020
Survey experiments are now quite common in political science. A recent analysis of the number of mentions of this term in political science journal articles demonstrates a dramatic increase from 2000 to 2013. In addition, the term survey experiment has been picked up by many other disciplines, by researchers in a variety of different countries. Given the large number of survey experiments already published, the goal here is not to review the numerous excellent studies using this methodology, because there are far too many, spanning too many different topics. Instead, this juncture—marked by both progress and the proliferation of this method—is used to highlight some of the issues that have arisen as this methodological approach has come of age. How might research using this methodology improve in political science? What are the greatest weaknesses of survey experimental studies in this discipline to date?
The explosive growth of survey experiments in political science speaks to their popularity as a means of establishing causal inference. In his reflection on the origins of survey experiments, Paul Sniderman has suggested that their quick rise in popularity was due to two factors: a) their ability to meet expected standards of external validity within the discipline without sacrificing internal validity, and b) the lower marginal cost per study relative to studies that were representative national surveys. Collaborative data collection efforts such as the Multi-Investigator Project and Time-sharing Experiments for the Social Sciences (TESS) made it possible for more scholars to execute population-based survey experiments at a lower cost per study than traditional surveys. Using shared platforms, researchers can execute many experiments for the price of one representative survey.
These explanations make perfect sense in the context of a field such as political science, where external validity traditionally has been valued more highly than internal validity. It may be surprising to younger colleagues to learn that, not all that long ago, experiments were deemed completely inappropriate within the discipline of political science, unless they were field experiments executed in the real world. Experiments involving interventions in naturally occurring political environments were deemed tolerable, but only political psychologists were likely to find experimentation more broadly acceptable due to their strong ties to psychology. In political science, survey experiments were a means of promoting experimental methods in an external-validity-oriented discipline. Survey experiments freed political scientists from college sophomores as subjects and promised that external validity need not be sacrificed for strong causal inference.
Times have obviously changed, and political scientists now embrace a much broader array of methodologies including both observational and experimental methods. This occasion provides an opportunity to re-evaluate the strengths and weaknesses of this innovative method, in theory and in practice.
- survey experiment
- manipulation check
- confounding
- balance test
- crowd-sourced samples
- generalizability
- internal validity
- external validity
- political decision making
You do not currently have access to this article
Please login to access the full content.
Access to the full content requires a subscription
Printed from Oxford Research Encyclopedias, Politics. Under the terms of the licence agreement, an individual user may print out a single article for personal use (for details see Privacy Policy and Legal Notice).
date: 18 June 2024
- Cookie Policy
- Privacy Policy
- Legal Notice
- Accessibility
- [195.158.225.230]
- 195.158.225.230
Character limit 500 /500
- Organizations
- Planning & Activities
- Product & Services
- Structure & Systems
- Career & Education
- Entertainment
- Fashion & Beauty
- Political Institutions
- SmartPhones
- Protocols & Formats
- Communication
- Web Applications
- Household Equipments
- Career and Certifications
- Diet & Fitness
- Mathematics & Statistics
- Processed Foods
- Vegetables & Fruits
Difference Between Experiment and Survey
• Categorized under Mathematics & Statistics , Psychology , Science , Words | Difference Between Experiment and Survey
Experiment and survey methods are highly important in data gathering. Both can be utilized to test hypotheses and come up with conclusions. Research through experiments involves the manipulation of an independent variable and measuring its effect on a dependent variable. On the other hand, conducting surveys often entails the use of questionnaires and/or interviews. The following paragraphs further delve into such differences.
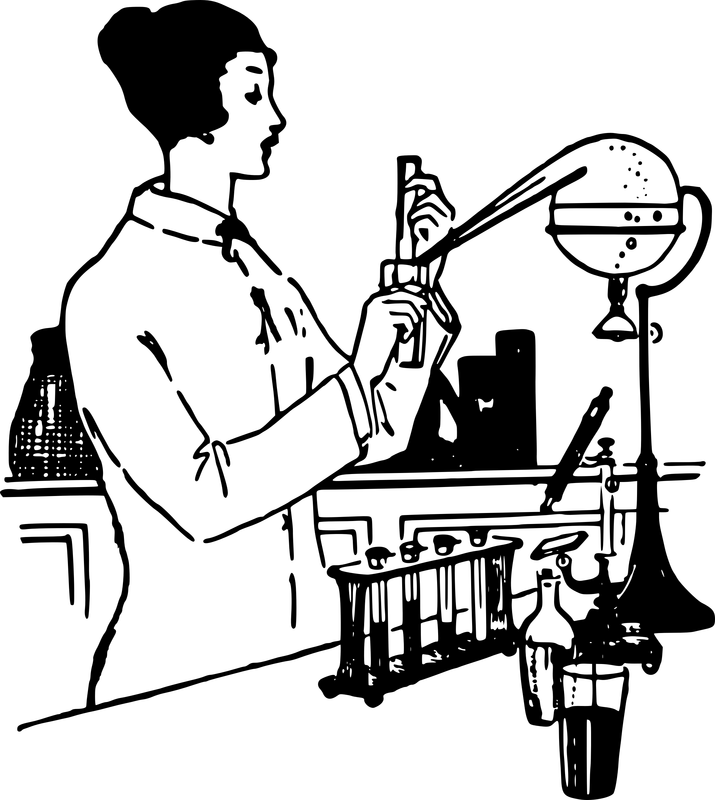
What is an Experiment?
From the Latin word, “experior” which means “to attempt” or “to experience”, experiment is defined as testing a hypothesis by carrying out a procedure under highly controlled conditions. This makes the method ideal in studying primary data. By manipulating a certain independent variable, its effect on a dependent variable can be measured. A cause and effect relationship is verified by exposing participants to certain treatments. For instance, researchers can measure how water intake can affect people’s metabolism by letting the experimental group drink 8 glasses of water each day while the control group will only have 4 glasses. Their metabolism rates will then be compared after a week and statistical treatments like T-test will be employed to validate the results.
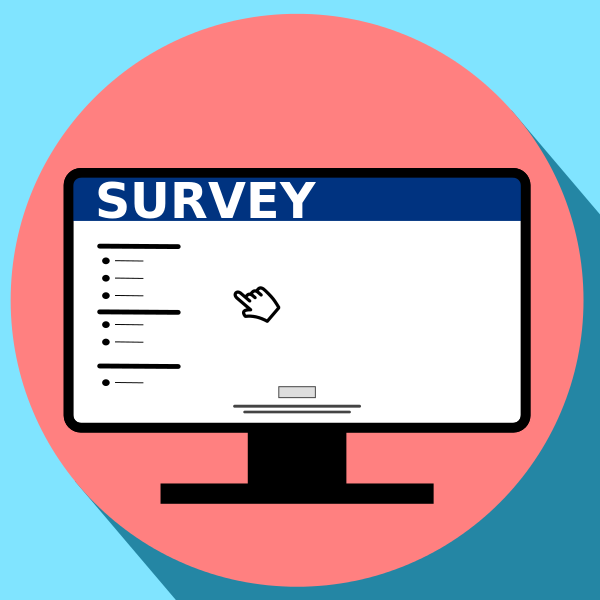
What is a Survey?
From the medieval Latin word, “supervidere” which means “to see”, survey is defined as having a comprehensive view of certain topics. Survey studies are largely conducted to look into people’s opinions, feelings, and thoughts. It is best suited for descriptive research which seeks to answer “what” questions regarding the respondents. Questionnaires are ideal in collecting information from a big population as they can be simultaneously administered to different groups and individuals. Survey questions can be sent to numerous respondents in both online and offline settings. For instance, researchers who are studying happiness levels among millennials floated questionnaires, made phone calls, and sent e-mails regarding the participants’ perceived emotional states. The data were then collated and statistical treatment such as getting the weighted mean was utilized to analyze the responses.
Difference between Experiment and Survey
Etymology of experiment and survey.
Experiment came from the Latin word “experior” which means “to attempt” or “to experience” while survey came from the Latin word “supervidere” which means “to see”.
Source of Information of Experiment and Survey
Conducting an experiment enables the researchers to gather data from the result of the experimental treatment. On the other hand, surveys get information from the selected population.
Experiments mainly deal with primary data while surveys can gather secondary data which are in line with descriptive research.
Research involved in Experiment and Survey
While survey is employed in descriptive research, the experimental method is noticeably used for experimental research.
Sample Sizes for Experiment and Survey
As compared to surveys, the sample sizes used in experiments are usually smaller. Since questionnaires can easily reach a number of people in various places, surveys can cover larger samples.
Many social and behavioral fields use the survey method in establishing facts while those in the physical and natural sciences basically employ experiments.
Laboratory Research for Experiment and Survey
Laboratory research usually makes use of experiments whereas field research largely profits from surveys.
Equipment needed for Experiment vs Survey
Experiments often use various equipment in facilitating treatments and in observing responses while surveys do not need such elaborate tools.
Correlational analysis is crucial in surveys while causal analysis is vital in experiments.
Regarding surveys, it is usually difficult to study in-depth and genuine responses as the questions are already set for all respondents and some of them may not actually reveal their true opinions. On the other hand, one common challenge in experiments is ascertaining if the change of behavior observed was really caused by the manipulation of the independent variable or other factors.
Cost for Experiment vs Survey
Conducting surveys is usually lest costly as compared to experiments as it is generally concerned with the sources in making questionnaires. As for experiments, researches need resources such as laboratories, equipment, and software.
Manipulation
Experiments involve the manipulation of the independent variable by giving different treatments to the control and experimental groups. As for surveys, the research participants are merely asked questions and this is done when manipulations are not possible.
Relationships
Experiments tests causal relationships by verifying if the independent variable significantly impacts the dependent variable. As for surveys, they usually assess naturally occurring and enduring variables.
Topic Range in Experiment vs Survey
As compared to experiments, surveys can be employed to look into a wider range of topics since the questions can be subdivided into different factors.
Randomization
Randomization practice is extremely crucial in establishing validity in experiments while such technique may or may not be employed in surveys.
Experiment vs Survey: Comparison table
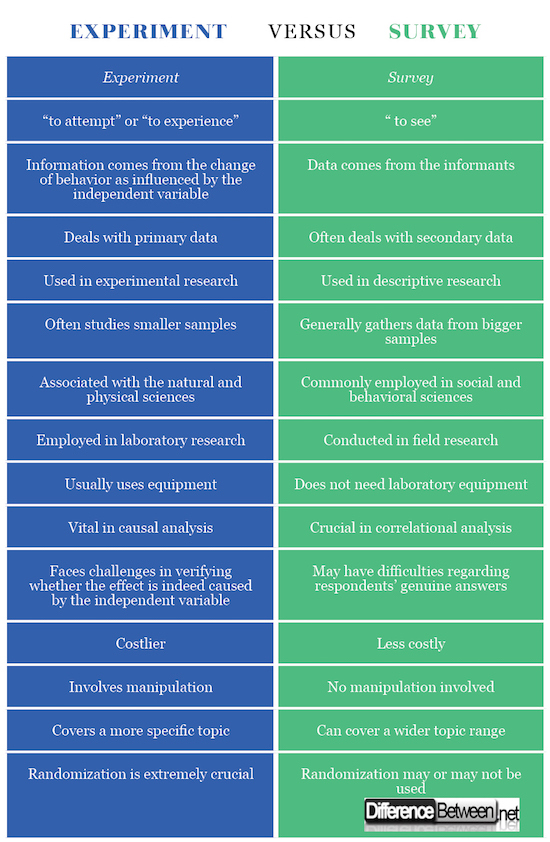
Summary of Experiment Vs Survey
- Both experiment and survey methods are vital in collecting data.
- Experiment came from the Latin word “experior” which means “to attempt” or “to experience” while survey came from Latin word “supervidere” which means “to see”.
- Experiment mainly deals with primary data while surveys can cover both primary and secondary data.
- While experiments are often done with smaller samples, surveys can be effective with larger samples.
- Experiments are often concerned with laboratory research and causal analysis while surveys are mostly associated with field research and correlational analysis.
- As compared to surveys, conducting experiments is usually costlier due to the equipment and highly controlled conditions.
- Experiments cover more specific topics while surveys can assess a wider range of interests.
- Recent Posts
- Difference Between Hematoma and Melanoma - February 9, 2023
- Difference Between Bruising and Necrosis - February 8, 2023
- Difference Between Brain Hematoma and Brain Hemorrhage - February 8, 2023
Sharing is caring!
- Pinterest 8
Search DifferenceBetween.net :

- Difference Between Study and Experiment
- Difference Between Questionnaires and Surveys
- Difference Between Polls and Surveys
- Difference Between Constant and Control
- Difference Between Dependent Variables and Independent Variables
Cite APA 7 Brown, g. (2018, May 31). Difference Between Experiment and Survey. Difference Between Similar Terms and Objects. http://www.differencebetween.net/science/difference-between-experiment-and-survey/. MLA 8 Brown, gene. "Difference Between Experiment and Survey." Difference Between Similar Terms and Objects, 31 May, 2018, http://www.differencebetween.net/science/difference-between-experiment-and-survey/.
Leave a Response
Name ( required )
Email ( required )
Please note: comment moderation is enabled and may delay your comment. There is no need to resubmit your comment.
Notify me of followup comments via e-mail
References :
Advertisments, more in 'mathematics & statistics'.
- Difference Between Horizontal and Vertical Asymptote
- Difference Between Leading and Lagging Power Factor
- Difference Between Commutative and Associative
- Difference Between Systematic Error and Random Error
- Difference Between Grounded Theory and Ethnography
More in 'Psychology'
- Difference Between INTP and INFP
- Difference Between Aversion Therapy and Flooding
- Difference Between Availability Heuristic and Representative Heuristic
- Difference Between Anchoring Heuristic and Adjustment Heuristic
- Difference Between Akinetic Mutism and Locked-In Syndrome
More in 'Science'
- Difference Between Rumination and Regurgitation
- Difference Between Pyelectasis and Hydronephrosis
- Difference Between Cellulitis and Erysipelas
- Difference Between Suicide and Euthanasia
- Difference Between Vitamin D and Vitamin D3
More in 'Words'
- Difference Between Center and Centre
- Difference Between Lodge and Resort
- Difference Between Authoritarian and Fascism
- Difference Between Advocate and Barrister
- Difference Between Advocacy and Lobbying
Top Difference Betweens
Get new comparisons in your inbox:, most emailed comparisons, editor's picks.
- Difference Between MAC and IP Address
- Difference Between Platinum and White Gold
- Difference Between Civil and Criminal Law
- Difference Between GRE and GMAT
- Difference Between Immigrants and Refugees
- Difference Between DNS and DHCP
- Difference Between Computer Engineering and Computer Science
- Difference Between Men and Women
- Difference Between Book value and Market value
- Difference Between Red and White wine
- Difference Between Depreciation and Amortization
- Difference Between Bank and Credit Union
- Difference Between White Eggs and Brown Eggs
Do you want to create free survey about:
Laboratory experiences?
Or maybe something else?
or use this template:
Laboratory experiences
Discover the intricate world of laboratory experiences through our engaging survey. Share your insights, challenges, and feedback to drive positive changes in the scientific community.
Would you like to work on this survey?
Startquestion is a free survey platform which allows you to create, send and analyse survey results.
Exploring the World of Laboratory Experiences: A Deep Dive into Research and Feedback
Welcome to our insightful survey on laboratory experiences! This questionnaire delves into the realm of scientific experimentation, uncovering valuable insights that can shape the future of laboratory practices. Whether you're a seasoned researcher or a curious novice, this survey offers a unique opportunity to reflect on your laboratory journey and provide feedback that can drive positive changes in the scientific community. From evaluating the frequency of lab experiments to identifying key challenges faced in the laboratory setting, participants will have the chance to share their perspectives on various aspects of laboratory work. Additionally, the survey explores the familiarity with different laboratory techniques, such as PCR and chromatography, shedding light on the diverse skills possessed by individuals in the scientific field. By offering both single-choice and multiple-choice questions, the survey aims to capture a comprehensive view of respondents' experiences and preferences when it comes to laboratory work. Participants are also encouraged to share their thoughts on the resources and safety measures that play a crucial role in enhancing the laboratory experience. From suggesting improvements to highlighting the motivations behind pursuing a career in laboratory work, this survey encompasses a wide range of topics that are pertinent to individuals engaged in scientific research. So, whether you're passionate about conducting experiments in the lab or simply interested in exploring the world of scientific discovery, join us on this exciting journey through the realm of laboratory experiences. Your feedback matters, your opinions count, and your voice will shape the future of scientific research.
- Software Engineering Tutorial
- Software Development Life Cycle
- Waterfall Model
- Software Requirements
- Software Measurement and Metrics
- Software Design Process
- System configuration management
- Software Maintenance
- Software Development Tutorial
- Software Testing Tutorial
- Product Management Tutorial
- Project Management Tutorial
- Agile Methodology
- Selenium Basics
- Share Your Experience
Difference between Survey and Experiment
- Difference Between Correlational and Experimental-Research
- Difference between Science and Technology
- Difference between Descriptive Research and Experimental Research
- Difference between CRD and RBD
- Difference Between Stratified and Cluster Sampling
- Difference between Data Science and Operations Research
- Difference between Raster Scan and Random Scan
- Difference between Component and Unit Testing
- Differences between Interface and Integration Testing
- Difference between Unit Testing and Integration Testing
- Difference between Pilot Testing and Beta Testing
- Difference between Trial and Inquiry
- Difference between Questionnaire and Schedule
- Difference Between Investigation and Inquiry
- What is the Difference Between Theoretical and Experimental Probability?
- Difference Between Pandas Head, Tail And Sample
- Difference between Permutations and Combinations
- Differences between API Testing and Unit Testing
- Differences Between two-sample, t-test and paired t-test
- Coding for Everyone Course
1. Survey : Survey refers to the way of gathering information regarding a variable under study from all or a specified number of respondents of the universe. Surveys are carried out by maintaining a structured form of data collection, through interview, questionnaire, case study etc. In surveys prepared questions are asked from the prepared formal questionnaire set and the output is collected in the same form.
For example – Survey among the students about the new education policy of India.
2. Experiment : Experiments refers to the way of experimenting something practically with the help of scientific procedure/approach and the outcome is observed. Experiments are carried out by performing the experiments by following scientific procedure or scientific approach. In experiments the investigator/examiner performs tests or experiments based on various factors and observes the outcome of the experiment.
For example – Experiment in the chemistry laboratory by a group of students and faculties specific to a topic.
Difference between Survey and Experiment :
S.No. | SURVEY | EXPERIMENT |
---|---|---|
01. | It refers to a way of gathering information regarding a variable under study from people. | It refers to the way of experimenting something practically with the help of scientific procedure/approach and the outcome is observed. |
02. | Surveys are conducted in case of descriptive research. | Experiments are conducted in case of experimental research. |
03. | Surveys are carried out to see something. | Experiments are carried out to experience something. |
04. | These studies usually have larger samples. | These studies usually have smaller samples. |
05. | The surveyor does not manipulate the variable or arrange for events to happen. | The researcher may manipulate the variable or arrange for events to happen. |
06. | It is appropriate in case of social or behavioral science. | It is appropriate in case of physical and natural science. |
07. | It comes under field research. | It comes under laboratory research. |
08. | Possible relationship between the data and the unknowns in the universe can be studied through surveys. | Experiments are meant to determine such relationships. |
09. | Surveys can be performed in less cost than a experiments. | Experiments costs higher than the surveys. |
10. | Surveys often deals with secondary data. | Experiments deal with primary data. |
11. | In surveys there is no requirement of laboratory equipment or there is a very small requirement of equipment just to collect any sample of data. | In experiments usually laboratory equipment are used in various activities during the experiment process. |
12. | It is vital in co-relational analysis. | It is vital in casual analysis. |
13. | No manipulation is involved in surveys. | Manipulation is involved in experiments. |
14. | In surveys data is collected through interview, questionnaire, case study etc. | In experiments data is collected through several readings of experiment. |
15. | Surveys can focus on broad topics. | Experiments focuses on specific topic. |
Please Login to comment...
Similar reads.
- Difference Between
- Software Engineering
Improve your Coding Skills with Practice
What kind of Experience do you want to share?
- Grades 6-12
- School Leaders
100 Last-Day-of-School Activities Your Students Will Love!
72 Easy Science Experiments Using Materials You Already Have On Hand
Because science doesn’t have to be complicated.
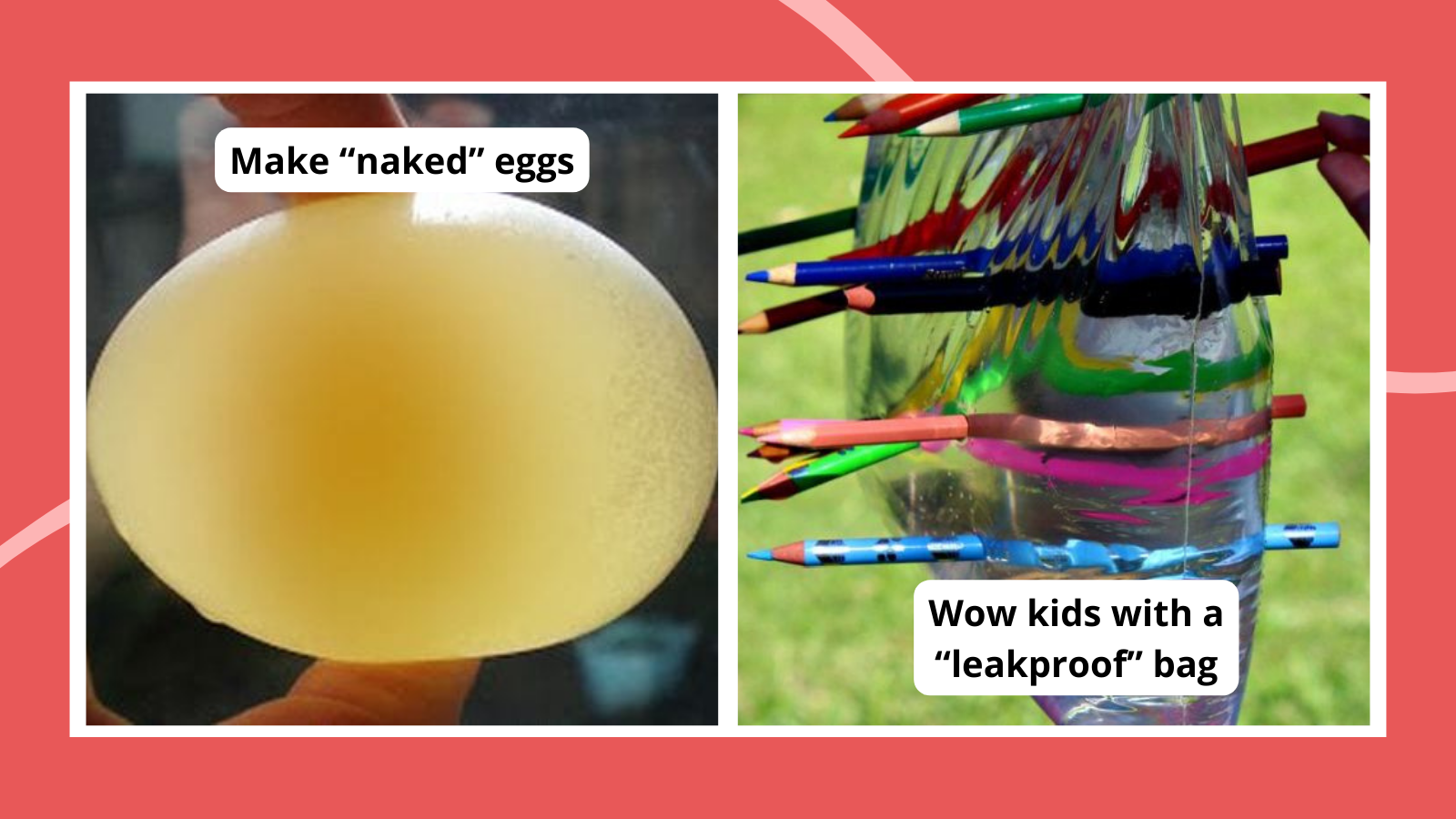
If there is one thing that is guaranteed to get your students excited, it’s a good science experiment! While some experiments require expensive lab equipment or dangerous chemicals, there are plenty of cool projects you can do with regular household items. We’ve rounded up a big collection of easy science experiments that anybody can try, and kids are going to love them!
Easy Chemistry Science Experiments
Easy physics science experiments, easy biology and environmental science experiments, easy engineering experiments and stem challenges.
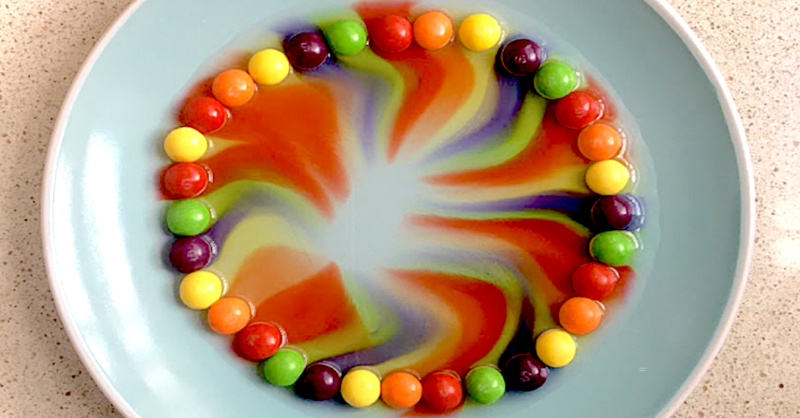
1. Taste the Rainbow
Teach your students about diffusion while creating a beautiful and tasty rainbow! Tip: Have extra Skittles on hand so your class can eat a few!
Learn more: Skittles Diffusion
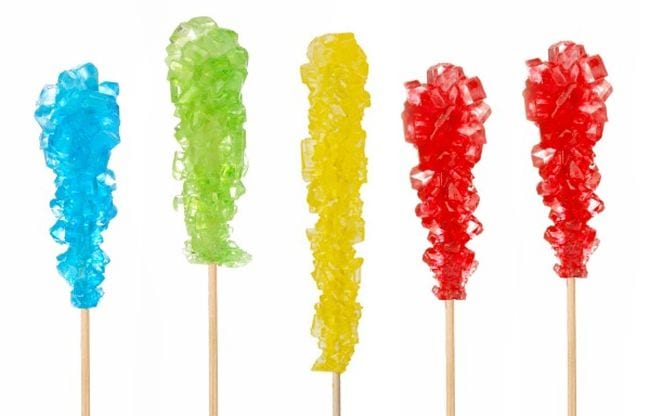
2. Crystallize sweet treats
Crystal science experiments teach kids about supersaturated solutions. This one is easy to do at home, and the results are absolutely delicious!
Learn more: Candy Crystals
3. Make a volcano erupt
This classic experiment demonstrates a chemical reaction between baking soda (sodium bicarbonate) and vinegar (acetic acid), which produces carbon dioxide gas, water, and sodium acetate.
Learn more: Best Volcano Experiments

4. Make elephant toothpaste
This fun project uses yeast and a hydrogen peroxide solution to create overflowing “elephant toothpaste.” Tip: Add an extra fun layer by having kids create toothpaste wrappers for plastic bottles.
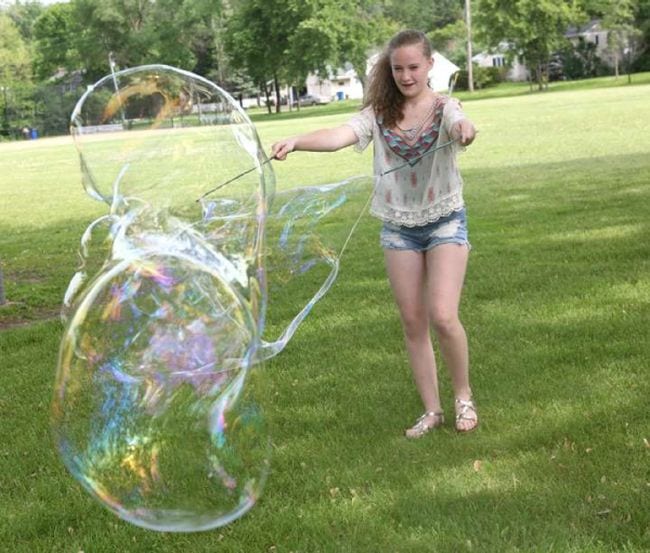
5. Blow the biggest bubbles you can
Add a few simple ingredients to dish soap solution to create the largest bubbles you’ve ever seen! Kids learn about surface tension as they engineer these bubble-blowing wands.
Learn more: Giant Soap Bubbles
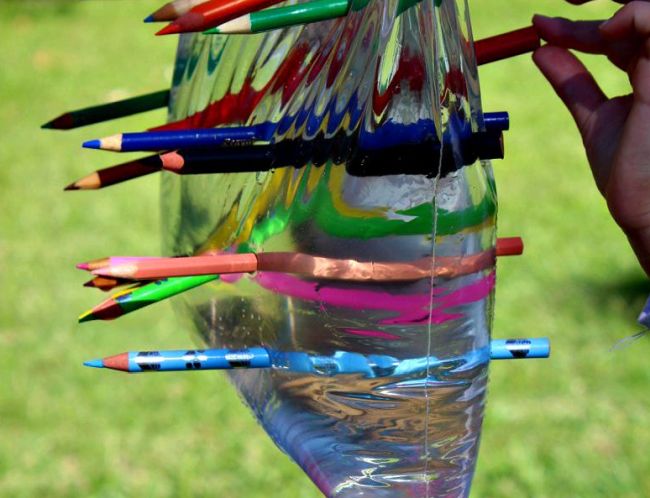
6. Demonstrate the “magic” leakproof bag
All you need is a zip-top plastic bag, sharp pencils, and water to blow your kids’ minds. Once they’re suitably impressed, teach them how the “trick” works by explaining the chemistry of polymers.
Learn more: Leakproof Bag
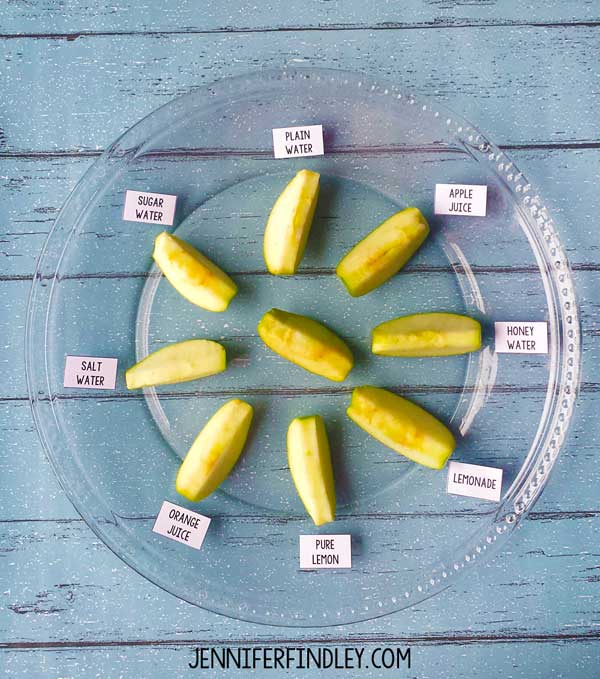
7. Use apple slices to learn about oxidation
Have students make predictions about what will happen to apple slices when immersed in different liquids, then put those predictions to the test. Have them record their observations.
Learn more: Apple Oxidation
8. Float a marker man
Their eyes will pop out of their heads when you “levitate” a stick figure right off the table! This experiment works due to the insolubility of dry-erase marker ink in water, combined with the lighter density of the ink.
Learn more: Floating Marker Man
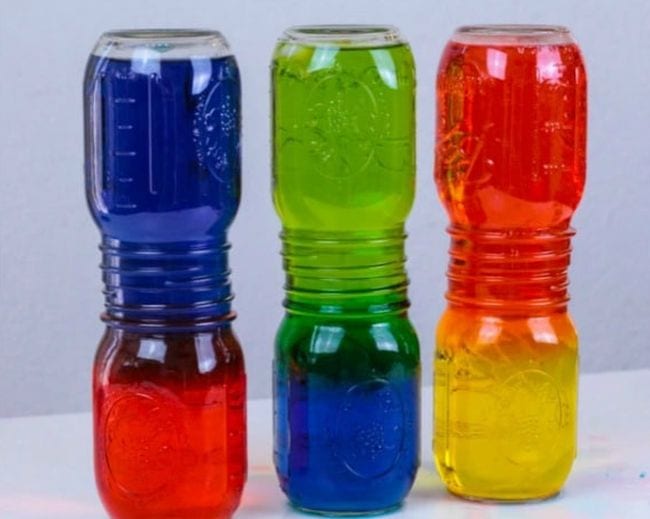
9. Discover density with hot and cold water
There are a lot of easy science experiments you can do with density. This one is extremely simple, involving only hot and cold water and food coloring, but the visuals make it appealing and fun.
Learn more: Layered Water
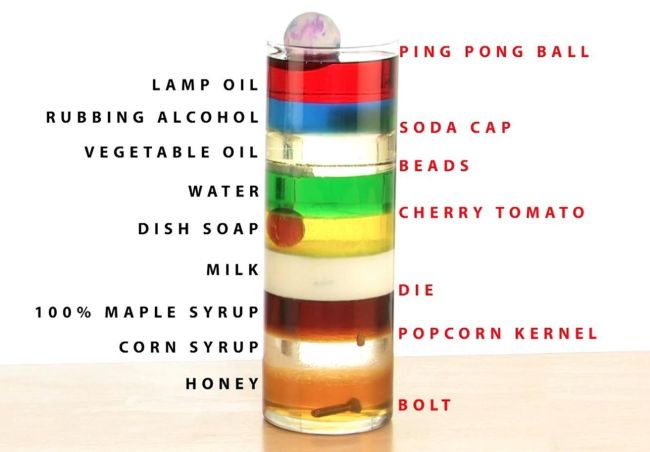
10. Layer more liquids
This density demo is a little more complicated, but the effects are spectacular. Slowly layer liquids like honey, dish soap, water, and rubbing alcohol in a glass. Kids will be amazed when the liquids float one on top of the other like magic (except it is really science).
Learn more: Layered Liquids
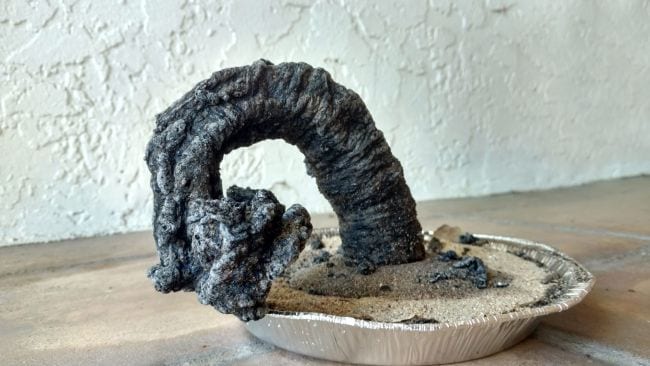
11. Grow a carbon sugar snake
Easy science experiments can still have impressive results! This eye-popping chemical reaction demonstration only requires simple supplies like sugar, baking soda, and sand.
Learn more: Carbon Sugar Snake
12. Mix up some slime
Tell kids you’re going to make slime at home, and watch their eyes light up! There are a variety of ways to make slime, so try a few different recipes to find the one you like best.
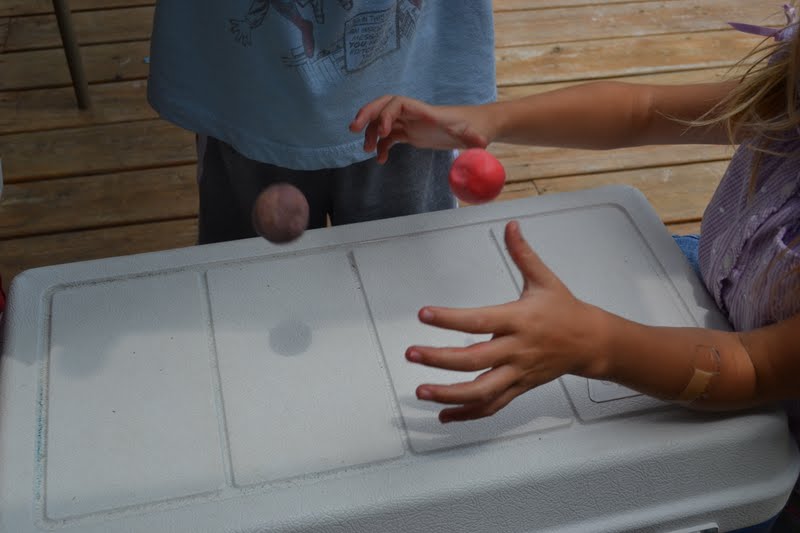
13. Make homemade bouncy balls
These homemade bouncy balls are easy to make since all you need is glue, food coloring, borax powder, cornstarch, and warm water. You’ll want to store them inside a container like a plastic egg because they will flatten out over time.
Learn more: Make Your Own Bouncy Balls
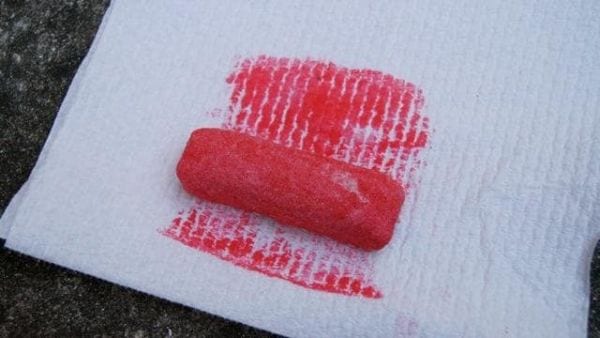
14. Create eggshell chalk
Eggshells contain calcium, the same material that makes chalk. Grind them up and mix them with flour, water, and food coloring to make your very own sidewalk chalk.
Learn more: Eggshell Chalk
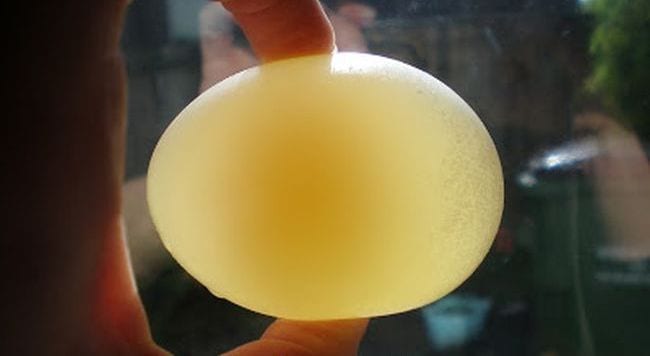
15. Make naked eggs
This is so cool! Use vinegar to dissolve the calcium carbonate in an eggshell to discover the membrane underneath that holds the egg together. Then, use the “naked” egg for another easy science experiment that demonstrates osmosis .
Learn more: Naked Egg Experiment
16. Turn milk into plastic
This sounds a lot more complicated than it is, but don’t be afraid to give it a try. Use simple kitchen supplies to create plastic polymers from plain old milk. Sculpt them into cool shapes when you’re done!
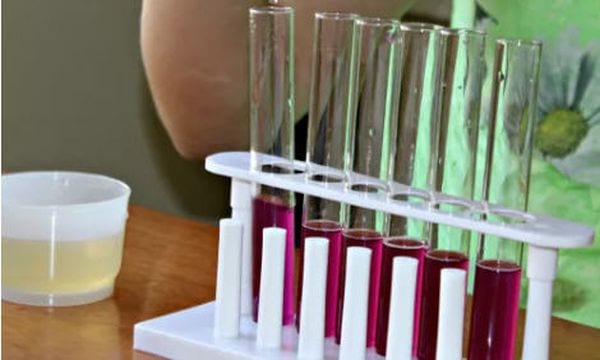
17. Test pH using cabbage
Teach kids about acids and bases without needing pH test strips! Simply boil some red cabbage and use the resulting water to test various substances—acids turn red and bases turn green.
Learn more: Cabbage pH
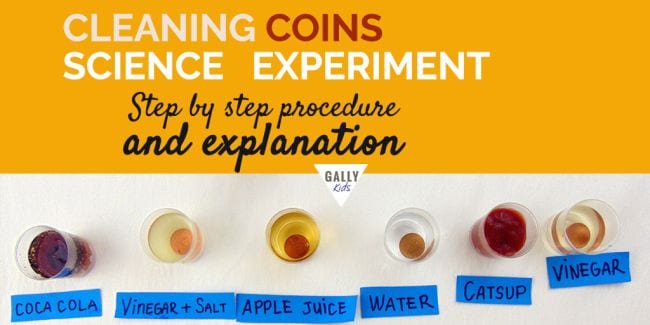
18. Clean some old coins
Use common household items to make old oxidized coins clean and shiny again in this simple chemistry experiment. Ask kids to predict (hypothesize) which will work best, then expand the learning by doing some research to explain the results.
Learn more: Cleaning Coins
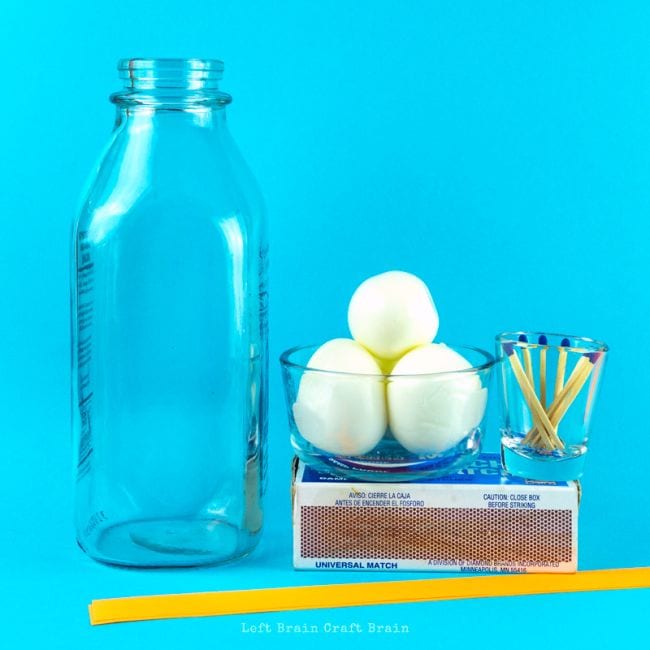
19. Pull an egg into a bottle
This classic easy science experiment never fails to delight. Use the power of air pressure to suck a hard-boiled egg into a jar, no hands required.
Learn more: Egg in a Bottle
20. Blow up a balloon (without blowing)
Chances are good you probably did easy science experiments like this when you were in school. The baking soda and vinegar balloon experiment demonstrates the reactions between acids and bases when you fill a bottle with vinegar and a balloon with baking soda.
21 Assemble a DIY lava lamp
This 1970s trend is back—as an easy science experiment! This activity combines acid-base reactions with density for a totally groovy result.
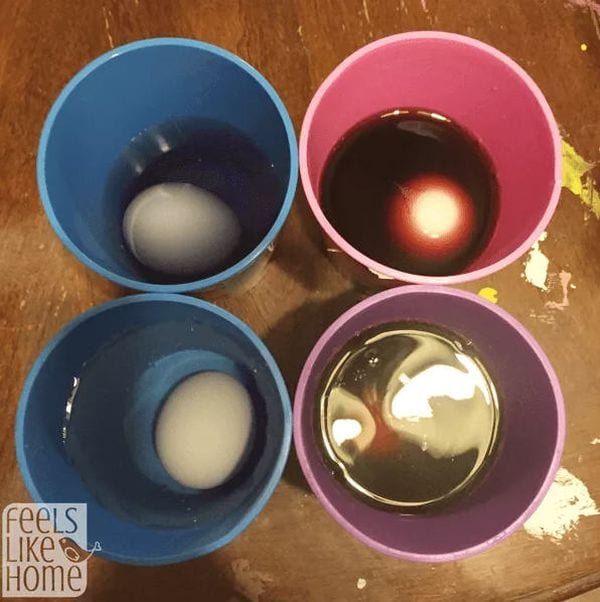
22. Explore how sugary drinks affect teeth
The calcium content of eggshells makes them a great stand-in for teeth. Use eggs to explore how soda and juice can stain teeth and wear down the enamel. Expand your learning by trying different toothpaste-and-toothbrush combinations to see how effective they are.
Learn more: Sugar and Teeth Experiment
23. Mummify a hot dog
If your kids are fascinated by the Egyptians, they’ll love learning to mummify a hot dog! No need for canopic jars , just grab some baking soda and get started.
24. Extinguish flames with carbon dioxide
This is a fiery twist on acid-base experiments. Light a candle and talk about what fire needs in order to survive. Then, create an acid-base reaction and “pour” the carbon dioxide to extinguish the flame. The CO2 gas acts like a liquid, suffocating the fire.
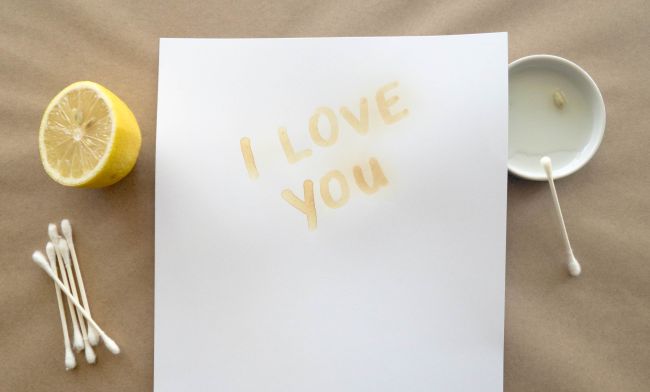
25. Send secret messages with invisible ink
Turn your kids into secret agents! Write messages with a paintbrush dipped in lemon juice, then hold the paper over a heat source and watch the invisible become visible as oxidation goes to work.
Learn more: Invisible Ink
26. Create dancing popcorn
This is a fun version of the classic baking soda and vinegar experiment, perfect for the younger crowd. The bubbly mixture causes popcorn to dance around in the water.
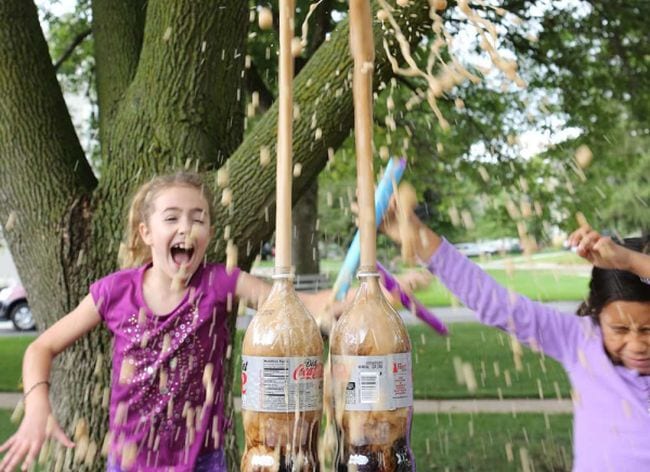
27. Shoot a soda geyser sky-high
You’ve always wondered if this really works, so it’s time to find out for yourself! Kids will marvel at the chemical reaction that sends diet soda shooting high in the air when Mentos are added.
Learn more: Soda Explosion
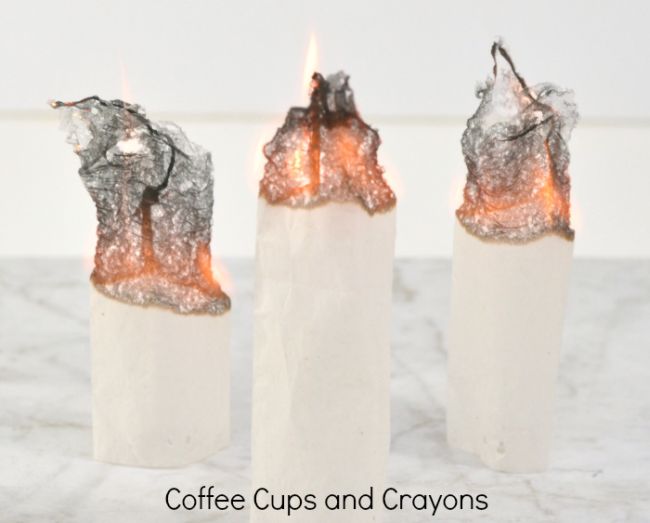
28. Send a teabag flying
Hot air rises, and this experiment can prove it! You’ll want to supervise kids with fire, of course. For more safety, try this one outside.
Learn more: Flying Tea Bags
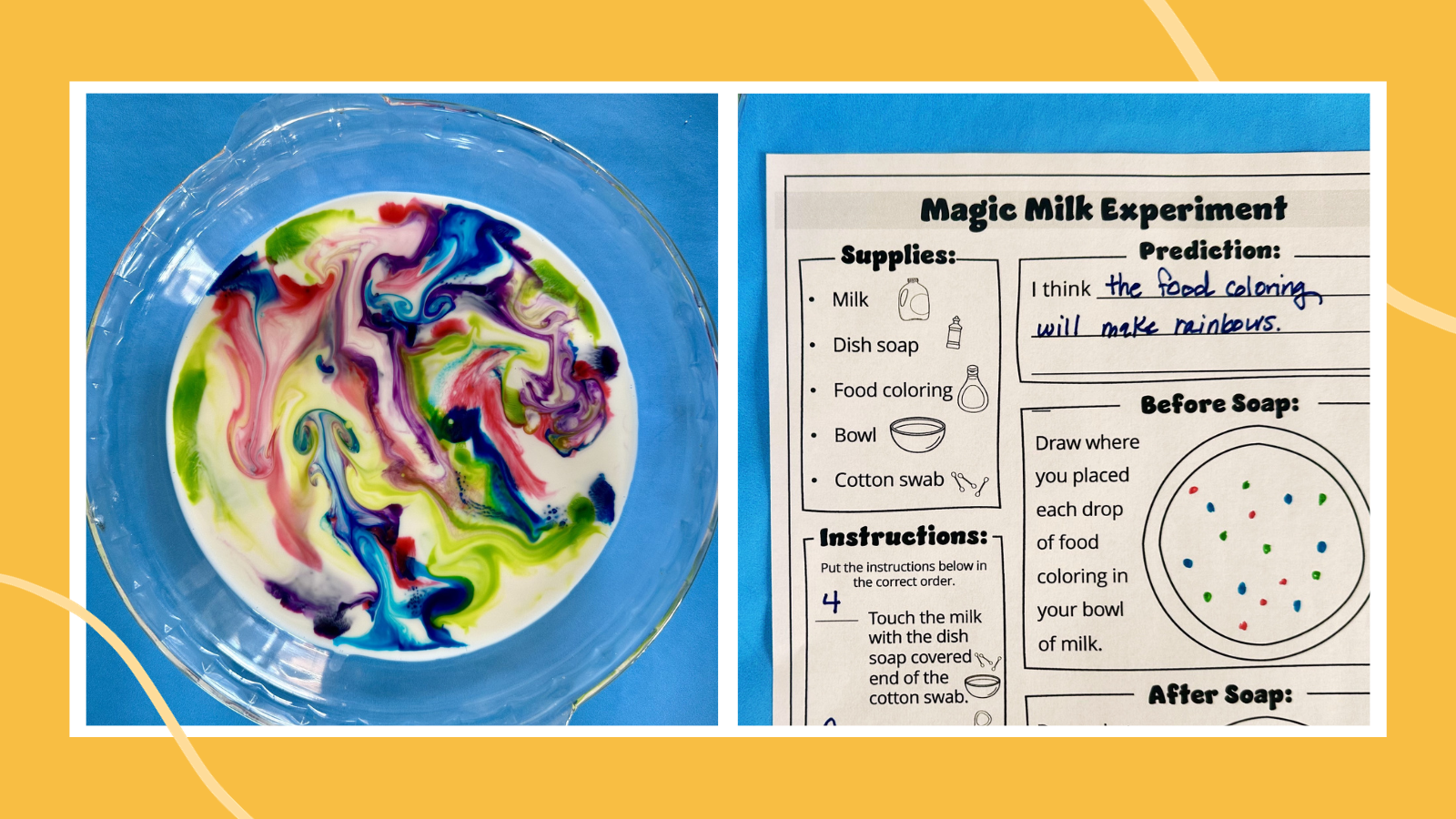
29. Create magic milk
This fun and easy science experiment demonstrates principles related to surface tension, molecular interactions, and fluid dynamics.
Learn more: Magic Milk Experiment
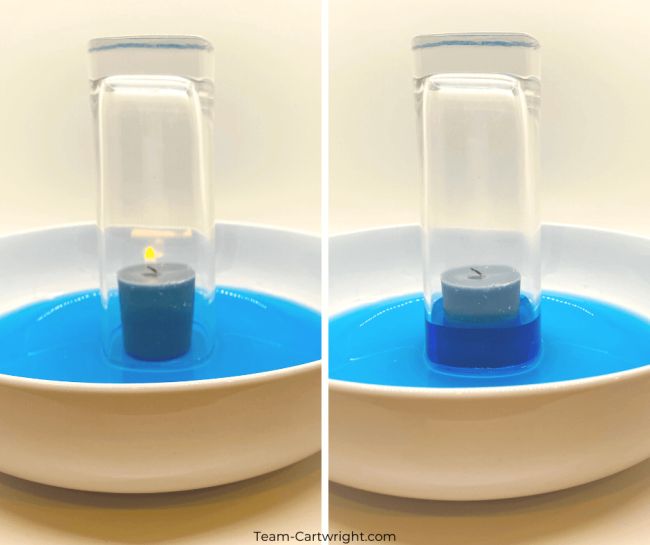
30. Watch the water rise
Learn about Charles’s Law with this simple experiment. As the candle burns, using up oxygen and heating the air in the glass, the water rises as if by magic.
Learn more: Rising Water
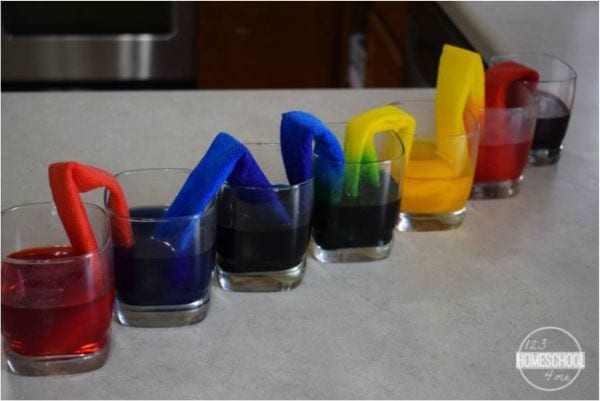
31. Learn about capillary action
Kids will be amazed as they watch the colored water move from glass to glass, and you’ll love the easy and inexpensive setup. Gather some water, paper towels, and food coloring to teach the scientific magic of capillary action.
Learn more: Capillary Action
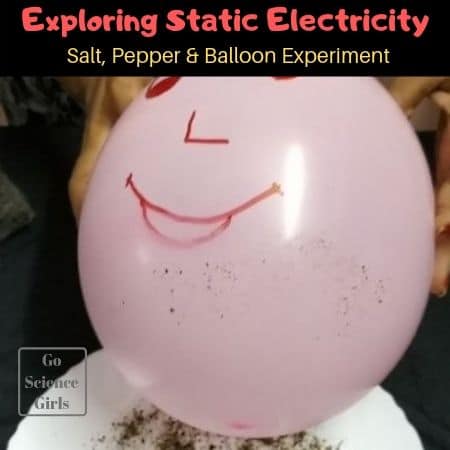
32. Give a balloon a beard
Equally educational and fun, this experiment will teach kids about static electricity using everyday materials. Kids will undoubtedly get a kick out of creating beards on their balloon person!
Learn more: Static Electricity
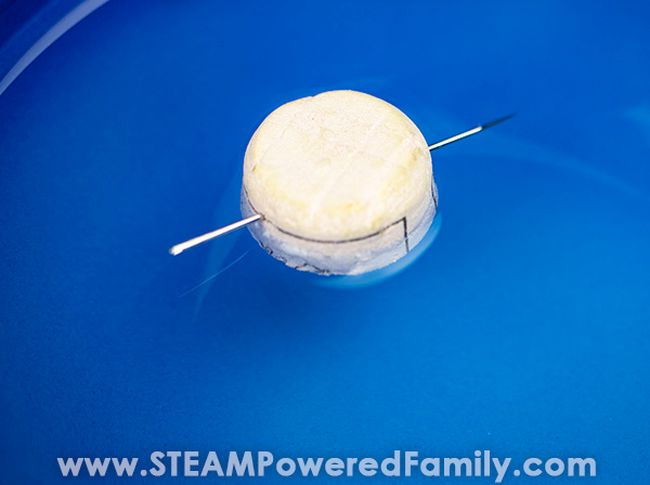
33. Find your way with a DIY compass
Here’s an old classic that never fails to impress. Magnetize a needle, float it on the water’s surface, and it will always point north.
Learn more: DIY Compass
34. Crush a can using air pressure
Sure, it’s easy to crush a soda can with your bare hands, but what if you could do it without touching it at all? That’s the power of air pressure!
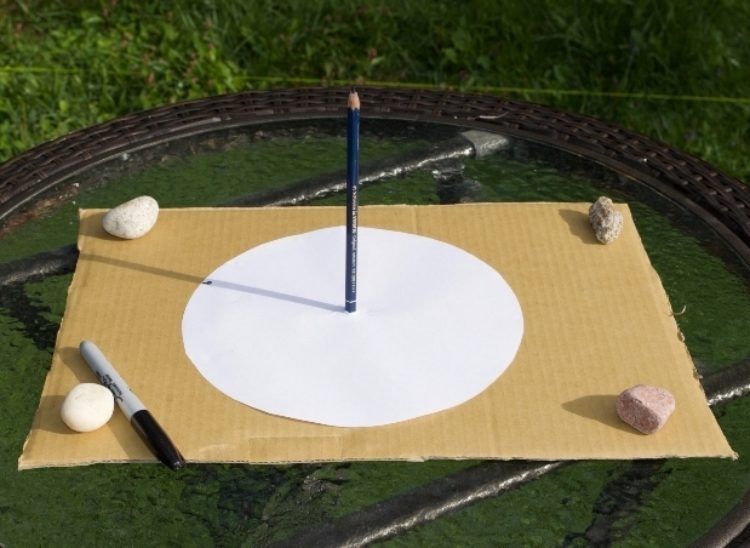
35. Tell time using the sun
While people use clocks or even phones to tell time today, there was a time when a sundial was the best means to do that. Kids will certainly get a kick out of creating their own sundials using everyday materials like cardboard and pencils.
Learn more: Make Your Own Sundial
36. Launch a balloon rocket
Grab balloons, string, straws, and tape, and launch rockets to learn about the laws of motion.
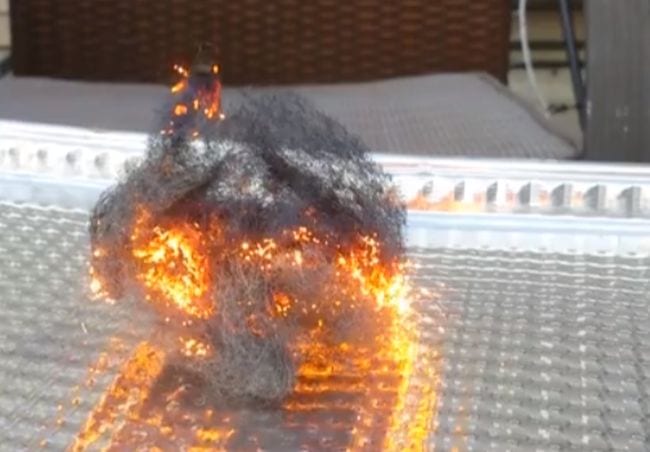
37. Make sparks with steel wool
All you need is steel wool and a 9-volt battery to perform this science demo that’s bound to make their eyes light up! Kids learn about chain reactions, chemical changes, and more.
Learn more: Steel Wool Electricity
38. Levitate a Ping-Pong ball
Kids will get a kick out of this experiment, which is really all about Bernoulli’s principle. You only need plastic bottles, bendy straws, and Ping-Pong balls to make the science magic happen.
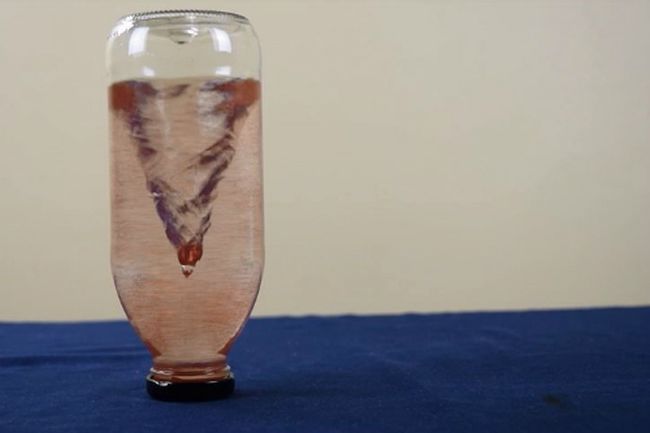
39. Whip up a tornado in a bottle
There are plenty of versions of this classic experiment out there, but we love this one because it sparkles! Kids learn about a vortex and what it takes to create one.
Learn more: Tornado in a Bottle
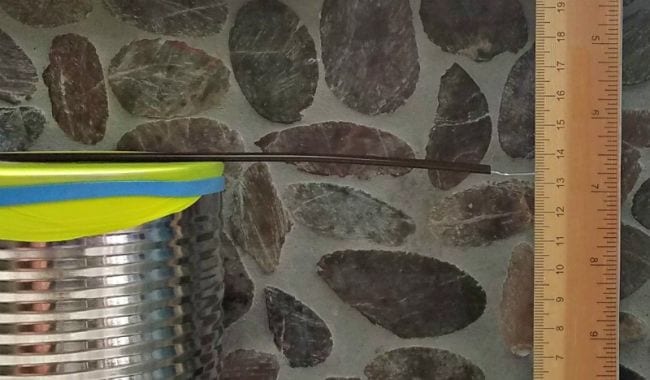
40. Monitor air pressure with a DIY barometer
This simple but effective DIY science project teaches kids about air pressure and meteorology. They’ll have fun tracking and predicting the weather with their very own barometer.
Learn more: DIY Barometer
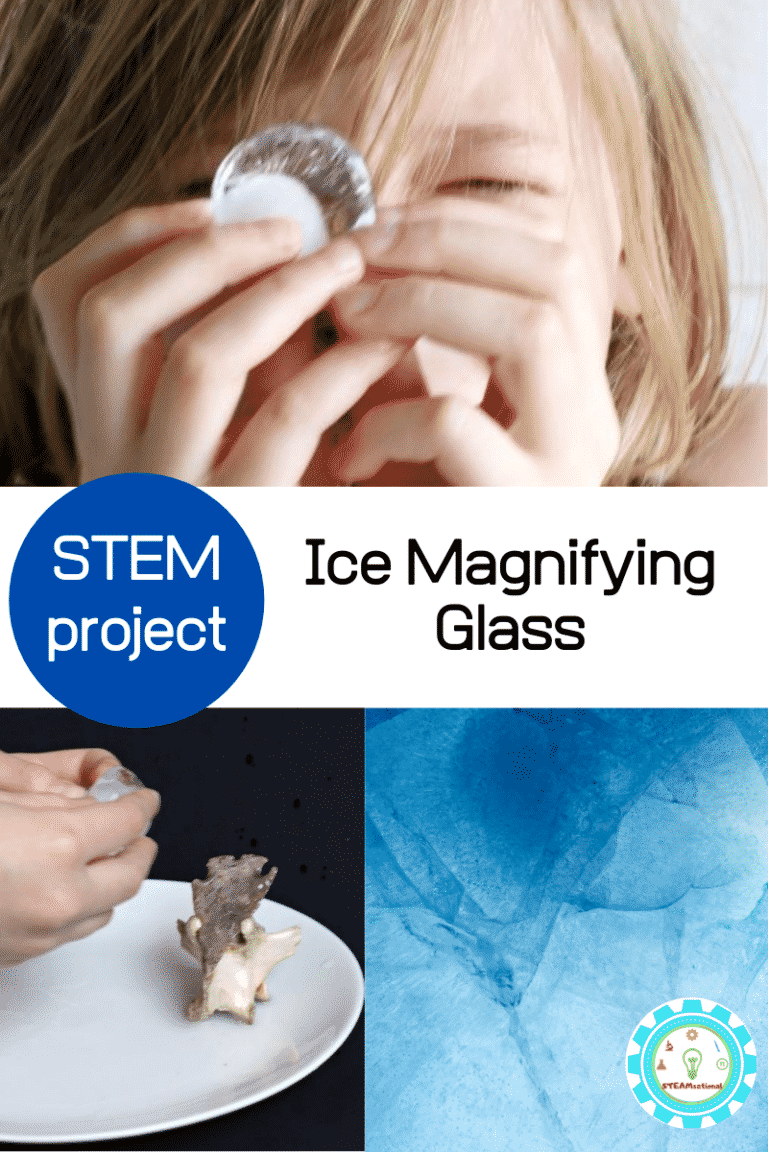
41. Peer through an ice magnifying glass
Students will certainly get a thrill out of seeing how an everyday object like a piece of ice can be used as a magnifying glass. Be sure to use purified or distilled water since tap water will have impurities in it that will cause distortion.
Learn more: Ice Magnifying Glass
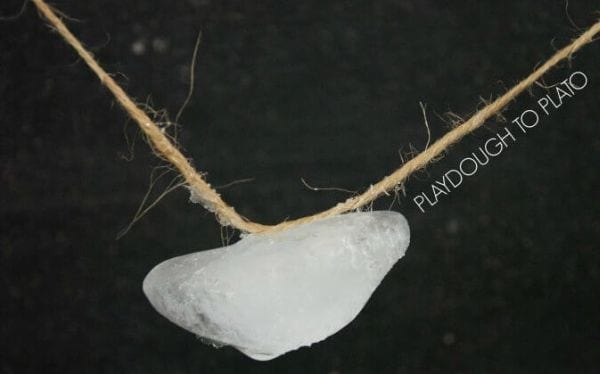
42. String up some sticky ice
Can you lift an ice cube using just a piece of string? This quick experiment teaches you how. Use a little salt to melt the ice and then refreeze the ice with the string attached.
Learn more: Sticky Ice
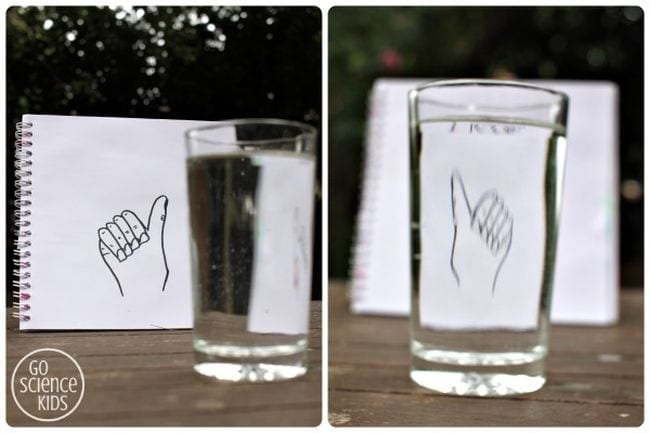
43. “Flip” a drawing with water
Light refraction causes some really cool effects, and there are multiple easy science experiments you can do with it. This one uses refraction to “flip” a drawing; you can also try the famous “disappearing penny” trick .
Learn more: Light Refraction With Water
44. Color some flowers
We love how simple this project is to re-create since all you’ll need are some white carnations, food coloring, glasses, and water. The end result is just so beautiful!
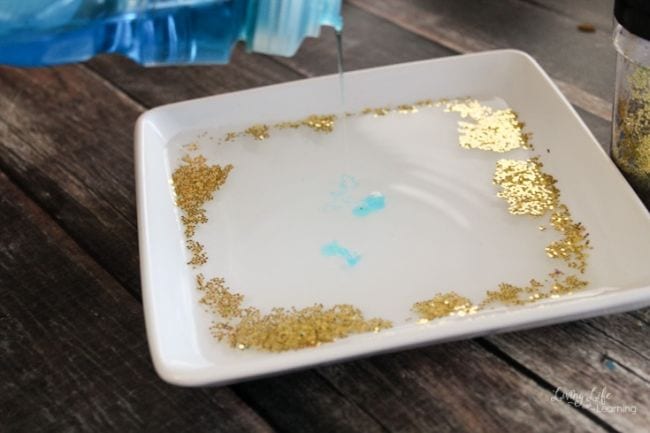
45. Use glitter to fight germs
Everyone knows that glitter is just like germs—it gets everywhere and is so hard to get rid of! Use that to your advantage and show kids how soap fights glitter and germs.
Learn more: Glitter Germs
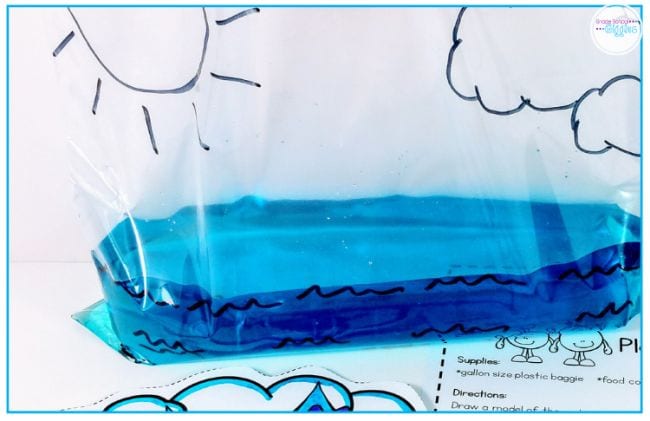
46. Re-create the water cycle in a bag
You can do so many easy science experiments with a simple zip-top bag. Fill one partway with water and set it on a sunny windowsill to see how the water evaporates up and eventually “rains” down.
Learn more: Water Cycle
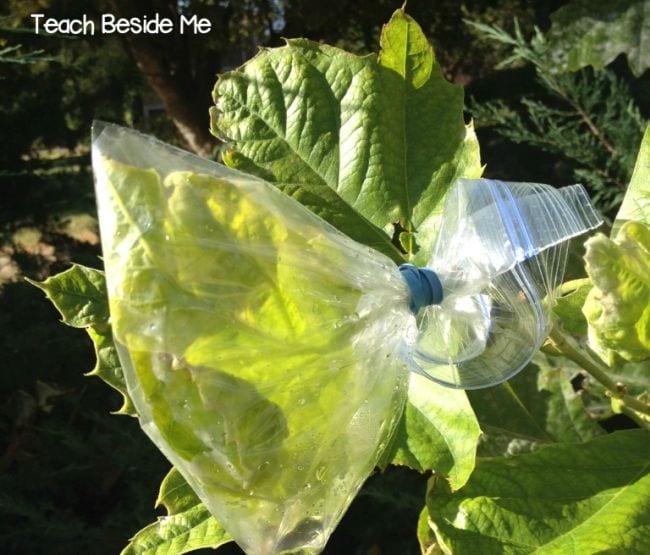
47. Learn about plant transpiration
Your backyard is a terrific place for easy science experiments. Grab a plastic bag and rubber band to learn how plants get rid of excess water they don’t need, a process known as transpiration.
Learn more: Plant Transpiration
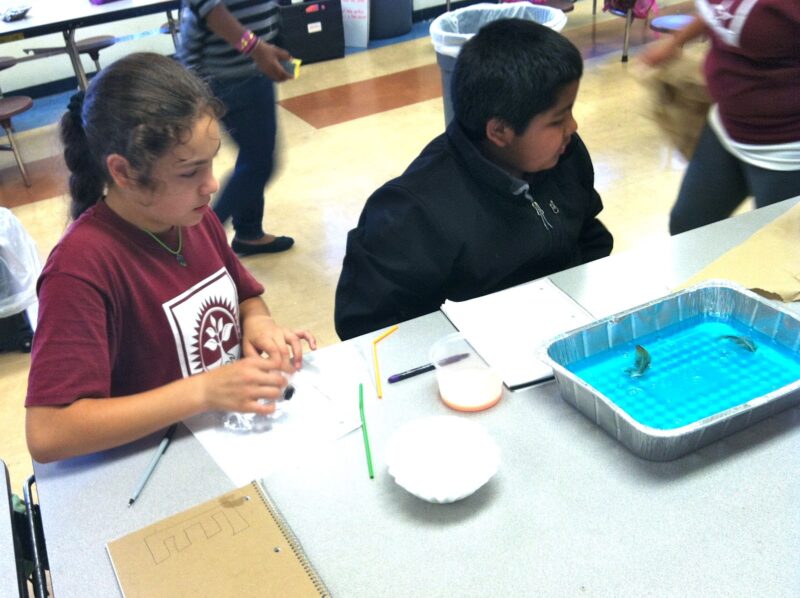
48. Clean up an oil spill
Before conducting this experiment, teach your students about engineers who solve environmental problems like oil spills. Then, have your students use provided materials to clean the oil spill from their oceans.
Learn more: Oil Spill
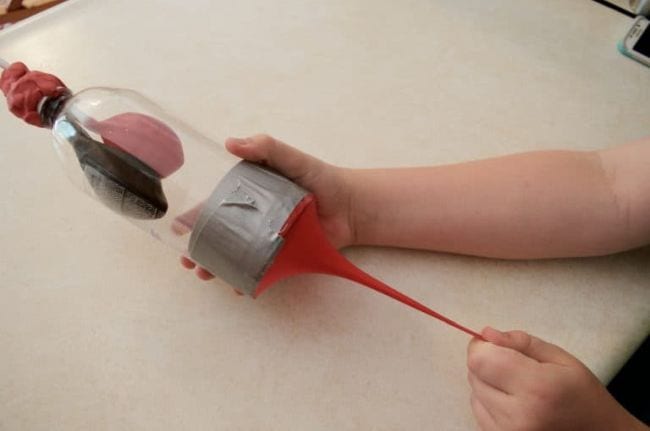
49. Construct a pair of model lungs
Kids get a better understanding of the respiratory system when they build model lungs using a plastic water bottle and some balloons. You can modify the experiment to demonstrate the effects of smoking too.
Learn more: Model Lungs
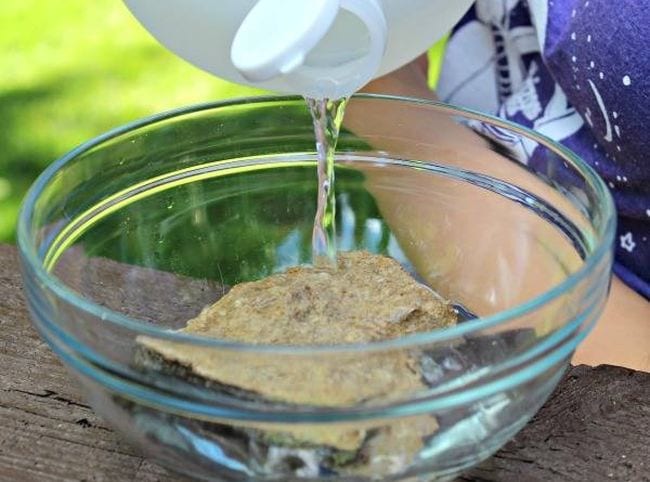
50. Experiment with limestone rocks
Kids love to collect rocks, and there are plenty of easy science experiments you can do with them. In this one, pour vinegar over a rock to see if it bubbles. If it does, you’ve found limestone!
Learn more: Limestone Experiments
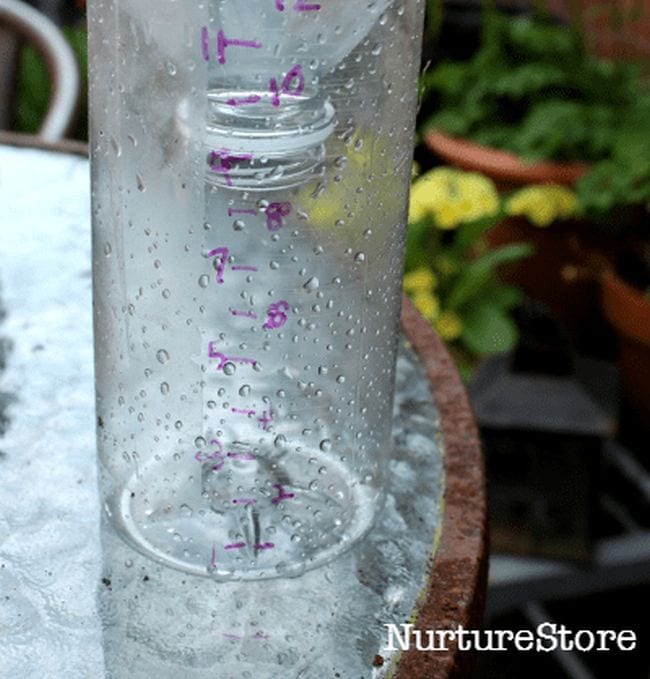
51. Turn a bottle into a rain gauge
All you need is a plastic bottle, a ruler, and a permanent marker to make your own rain gauge. Monitor your measurements and see how they stack up against meteorology reports in your area.
Learn more: DIY Rain Gauge
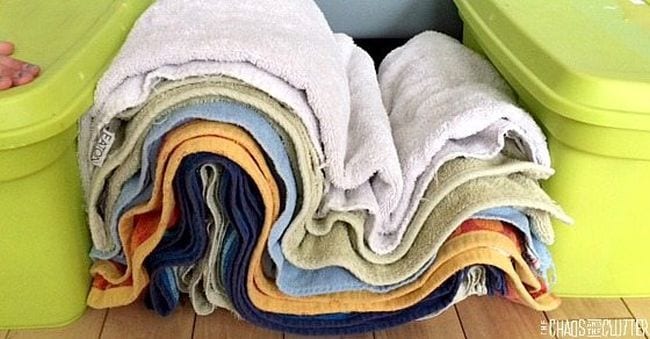
52. Build up towel mountains
This clever demonstration helps kids understand how some landforms are created. Use layers of towels to represent rock layers and boxes for continents. Then pu-u-u-sh and see what happens!
Learn more: Towel Mountains
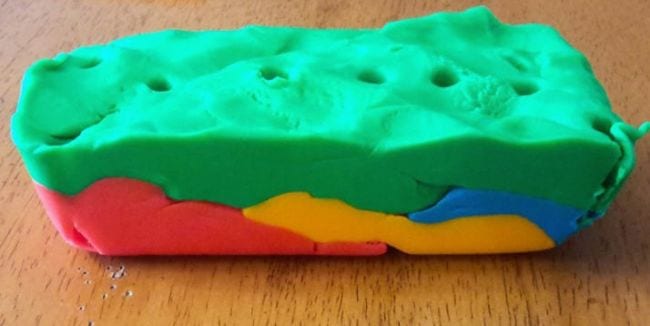
53. Take a play dough core sample
Learn about the layers of the earth by building them out of Play-Doh, then take a core sample with a straw. ( Love Play-Doh? Get more learning ideas here. )
Learn more: Play Dough Core Sampling
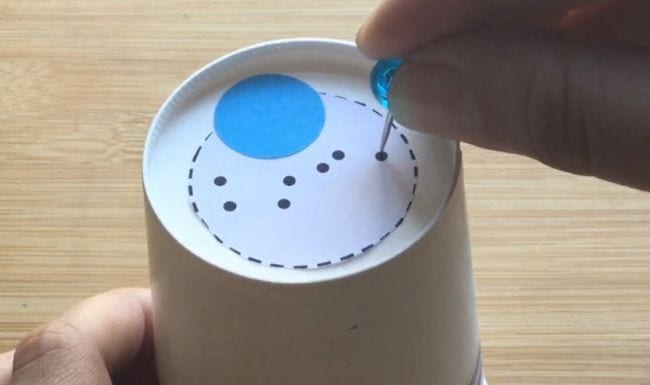
54. Project the stars on your ceiling
Use the video lesson in the link below to learn why stars are only visible at night. Then create a DIY star projector to explore the concept hands-on.
Learn more: DIY Star Projector
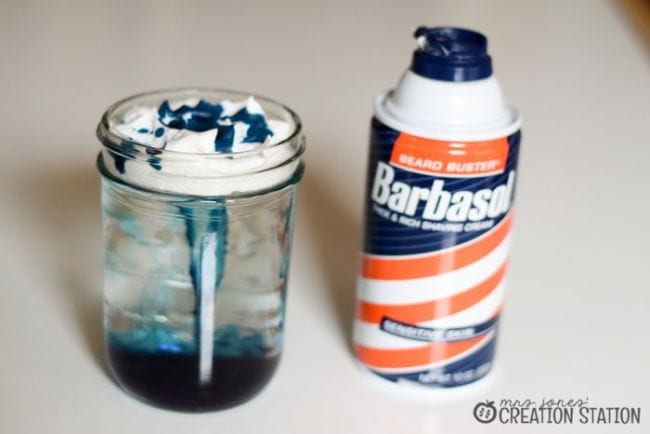
55. Make it rain
Use shaving cream and food coloring to simulate clouds and rain. This is an easy science experiment little ones will beg to do over and over.
Learn more: Shaving Cream Rain
56. Blow up your fingerprint
This is such a cool (and easy!) way to look at fingerprint patterns. Inflate a balloon a bit, use some ink to put a fingerprint on it, then blow it up big to see your fingerprint in detail.
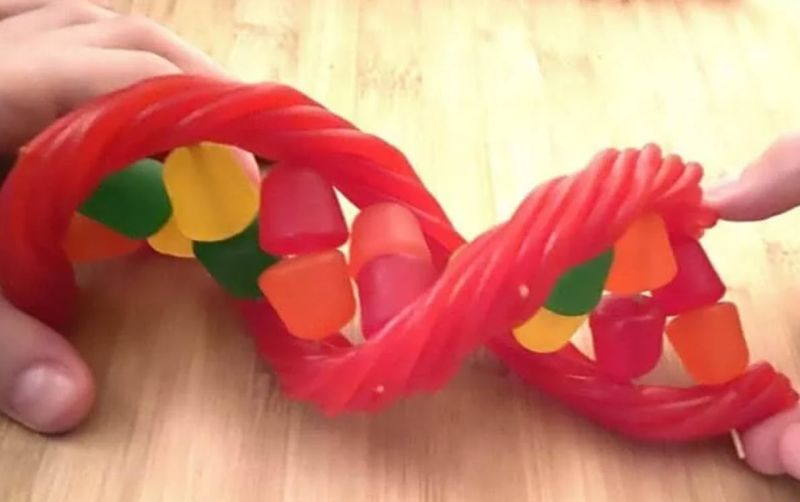
57. Snack on a DNA model
Twizzlers, gumdrops, and a few toothpicks are all you need to make this super-fun (and yummy!) DNA model.
Learn more: Edible DNA Model
58. Dissect a flower
Take a nature walk and find a flower or two. Then bring them home and take them apart to discover all the different parts of flowers.
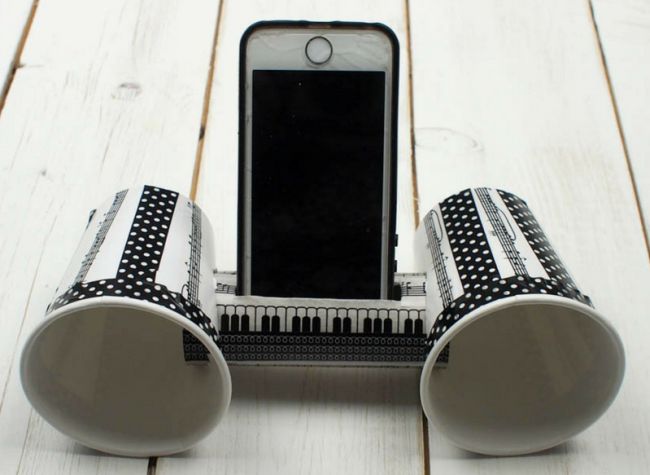
59. Craft smartphone speakers
No Bluetooth speaker? No problem! Put together your own from paper cups and toilet paper tubes.
Learn more: Smartphone Speakers
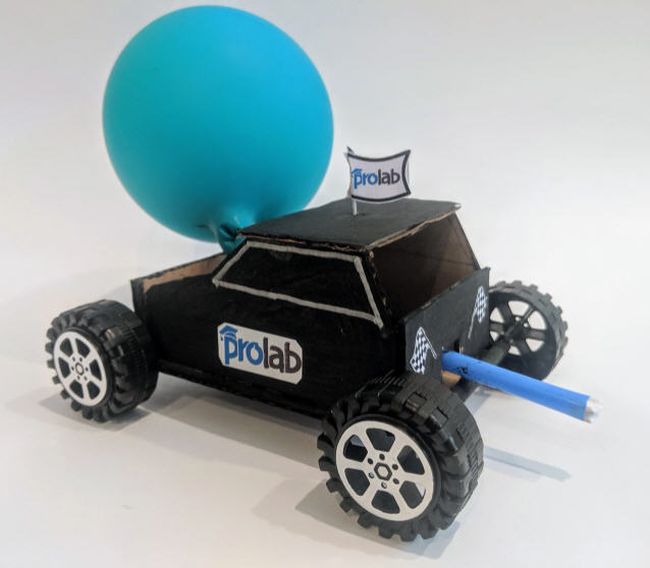
60. Race a balloon-powered car
Kids will be amazed when they learn they can put together this awesome racer using cardboard and bottle-cap wheels. The balloon-powered “engine” is so much fun too.
Learn more: Balloon-Powered Car
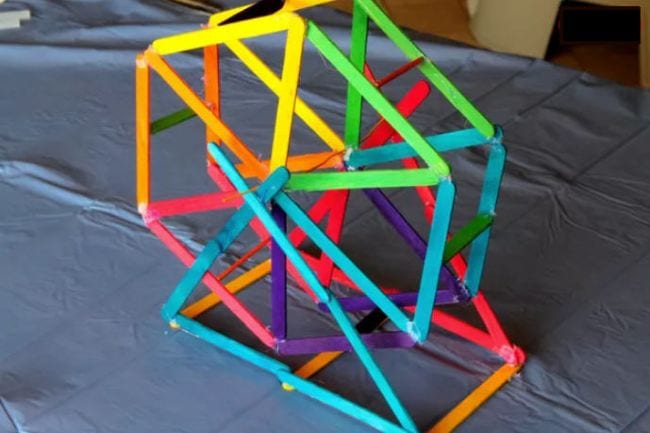
61. Build a Ferris wheel
You’ve probably ridden on a Ferris wheel, but can you build one? Stock up on wood craft sticks and find out! Play around with different designs to see which one works best.
Learn more: Craft Stick Ferris Wheel
62. Design a phone stand
There are lots of ways to craft a DIY phone stand, which makes this a perfect creative-thinking STEM challenge.
63. Conduct an egg drop
Put all their engineering skills to the test with an egg drop! Challenge kids to build a container from stuff they find around the house that will protect an egg from a long fall (this is especially fun to do from upper-story windows).
Learn more: Egg Drop Challenge Ideas
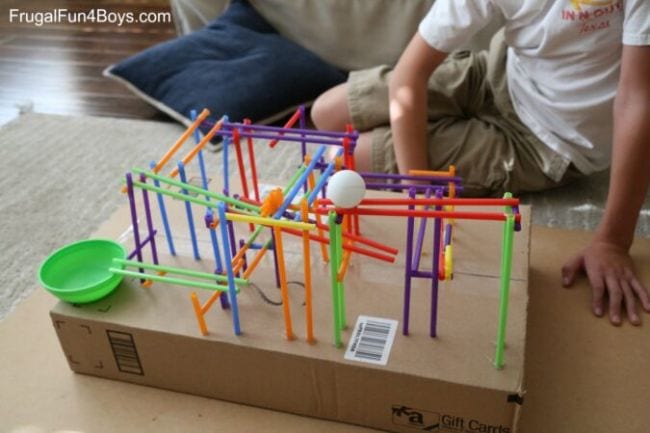
64. Engineer a drinking-straw roller coaster
STEM challenges are always a hit with kids. We love this one, which only requires basic supplies like drinking straws.
Learn more: Straw Roller Coaster
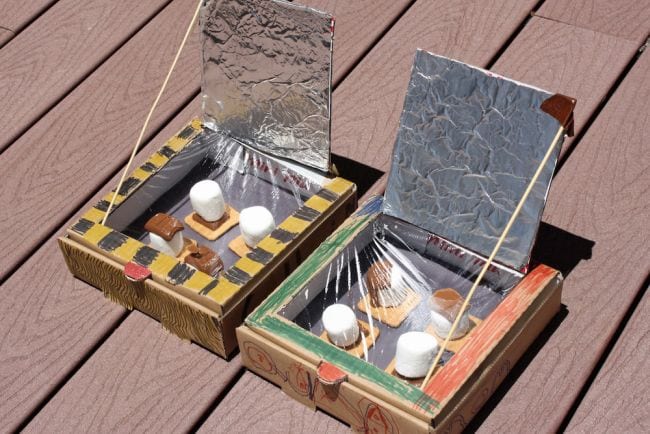
65. Build a solar oven
Explore the power of the sun when you build your own solar ovens and use them to cook some yummy treats. This experiment takes a little more time and effort, but the results are always impressive. The link below has complete instructions.
Learn more: Solar Oven
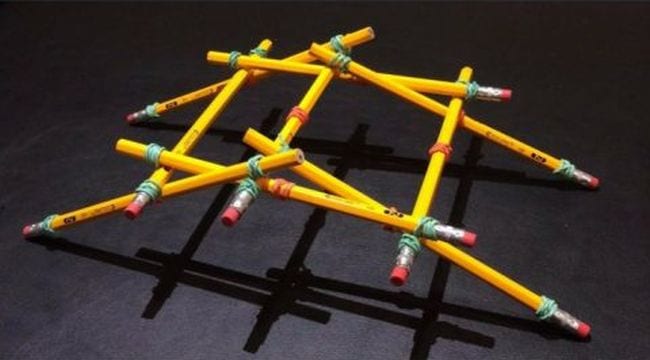
66. Build a Da Vinci bridge
There are plenty of bridge-building experiments out there, but this one is unique. It’s inspired by Leonardo da Vinci’s 500-year-old self-supporting wooden bridge. Learn how to build it at the link, and expand your learning by exploring more about Da Vinci himself.
Learn more: Da Vinci Bridge
67. Step through an index card
This is one easy science experiment that never fails to astonish. With carefully placed scissor cuts on an index card, you can make a loop large enough to fit a (small) human body through! Kids will be wowed as they learn about surface area.
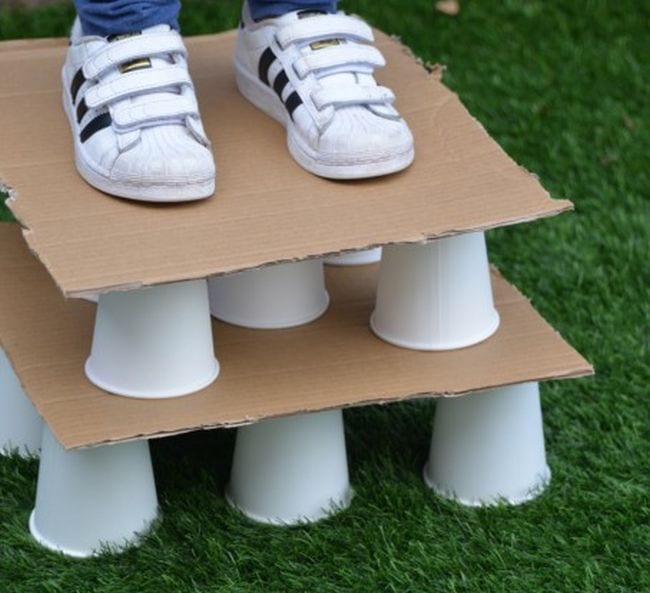
68. Stand on a pile of paper cups
Combine physics and engineering and challenge kids to create a paper cup structure that can support their weight. This is a cool project for aspiring architects.
Learn more: Paper Cup Stack
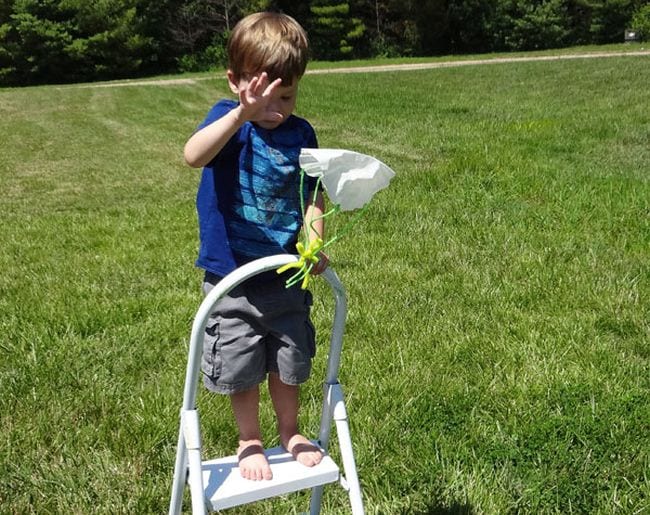
69. Test out parachutes
Gather a variety of materials (try tissues, handkerchiefs, plastic bags, etc.) and see which ones make the best parachutes. You can also find out how they’re affected by windy days or find out which ones work in the rain.
Learn more: Parachute Drop
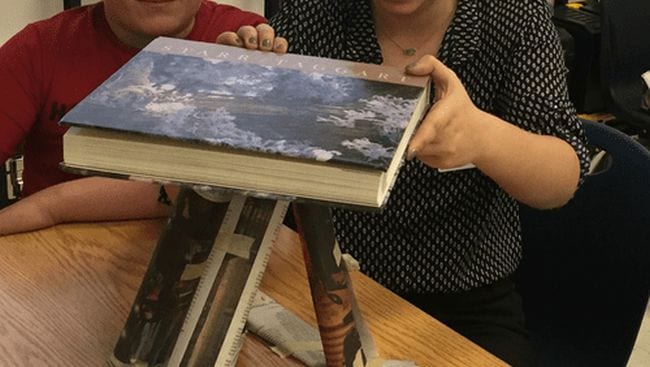
70. Recycle newspapers into an engineering challenge
It’s amazing how a stack of newspapers can spark such creative engineering. Challenge kids to build a tower, support a book, or even build a chair using only newspaper and tape!
Learn more: Newspaper STEM Challenge
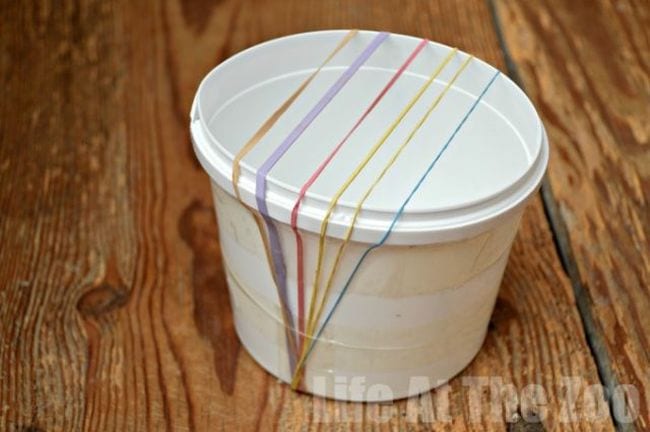
71. Use rubber bands to sound out acoustics
Explore the ways that sound waves are affected by what’s around them using a simple rubber band “guitar.” (Kids absolutely love playing with these!)
Learn more: Rubber Band Guitar
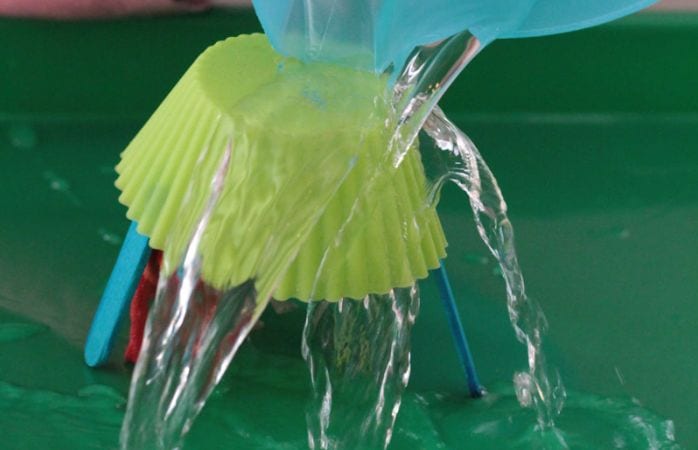
72. Assemble a better umbrella
Challenge students to engineer the best possible umbrella from various household supplies. Encourage them to plan, draw blueprints, and test their creations using the scientific method.
Learn more: Umbrella STEM Challenge
Plus, sign up for our newsletters to get all the latest learning ideas straight to your inbox.
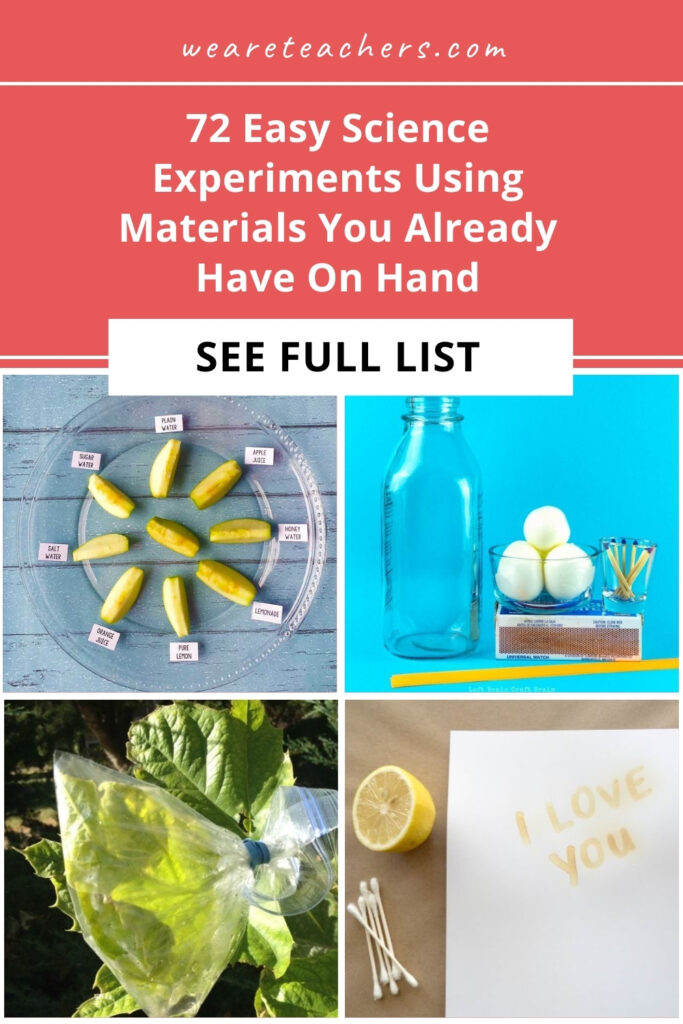
You Might Also Like
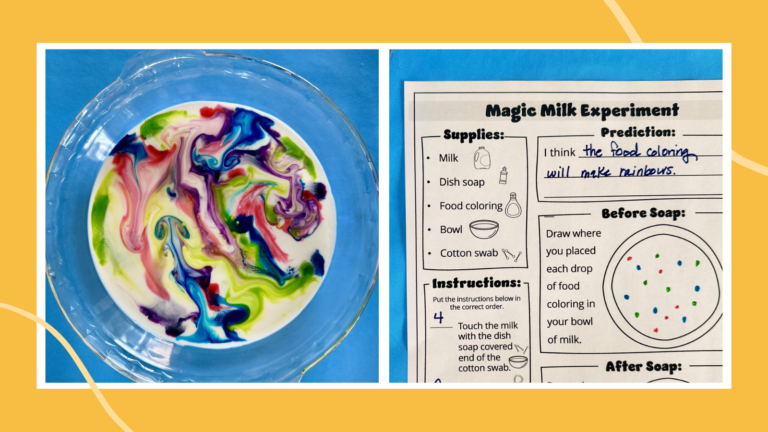
Magic Milk Experiment: How-To Plus Free Worksheet
This classic experiment teaches kids about basic chemistry and physics. Continue Reading
Copyright © 2024. All rights reserved. 5335 Gate Parkway, Jacksonville, FL 32256
Academia.edu no longer supports Internet Explorer.
To browse Academia.edu and the wider internet faster and more securely, please take a few seconds to upgrade your browser .
Enter the email address you signed up with and we'll email you a reset link.
- We're Hiring!
- Help Center
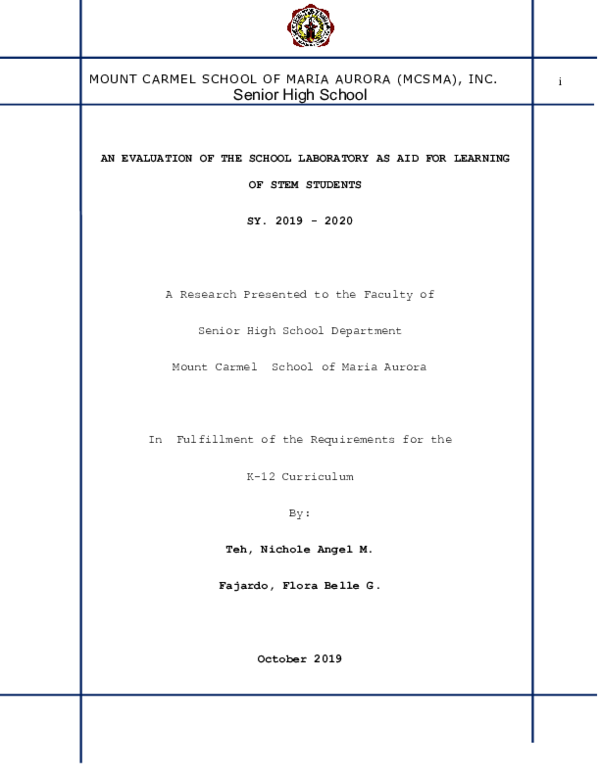
An Evaluation on the Science Laboratory as Learning Aid for STEM Students

This research paper was done by Nichole Angel M. Teh and Flora Bell Fajardo, students from the Senior High School Department (2019) under the strand of Science, Mathematics, Engineering and Technology(STEM). This is a quantitative research titled the evaluation of the science laboratory as learning aid for the STEM students. This quantitative research was conducted to evaluate the science laboratory as learning aid for STEM students in Mount Carmel School of Maria Aurora(MCSMA), Inc. The objective of this study was to discover if the students are helped by the science laboratory in their studies and if they learn more through experiments in a science laboratory. To gather the data they conducted a survey with a questionnaire that contained 20 questions that was answered by STEM students from grade 12 and 11 who were randomly chosen. There was a total of 79 respondents. The data gathered was analyzed using the percentage formula ( f/nx100). The data collected have been analyzed and interpreted by the researchers and so they came up with a positive result regarding the use of the science laboratory as learning aid for STEM students in Mount Carmel School of Maria Aurora(MCSMA),Inc.
Related Papers
PEDAGOGIK: Jurnal Pendidikan
Alif Mardiana
The science that studies nature and its processes are called Science. When learning about Science, the laboratory has an essential role in growing students' experimentation abilities and increasing students' enthusiasm for learning. This study aims to describe and determine the effectiveness of the use of laboratories in science learning at SMPN 2 Lumajang. This study uses an approach with the interview method. This research was carried out at SMPN 2 Lumajang, which involved subject science teachers at the SMPN. The results showed that the science laboratory at SMPN 2 Lumajang had two rooms: a laboratory room and a storage room. Judging from the aspect of the laboratory space, the science laboratory of SMPN 2 Lumajang still needs to be improved a bit, such as a space to store tools and materials, and there is no preparation room to make preparations before the practicum begins. The existing facilities and infrastructure influence the effectiveness of the use of laboratories ...
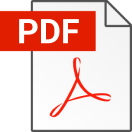
Universal Journal of Educational Research
Winda Kuncorowati
Dr. Mool Raj
4th International Scientific Conference on Philosophy of Mind and Cognitive Modelling in Education
Vincentas Lamanauskas
There are some trends currently being monitored in the country: interest in science studies and related professions is decreasing; unsatisfactory results of international student achievement research (PISA, TIMSS). In order to make students more interested in natural sciences and to motivate them to relate their life to STEAM activities, it is appropriate to encourage students to engage in independent research and to discover the joy of discovery. One of the ways to solve this problem is the students' practical experimental activity in the laboratories of University. In this way, students not only get to know the laws of science, new technologies, but also carry out experiments, research and projects. The research analyses the usage of STEAM program “Cognition of Energy and Thermal Processes” for students of ninth (1st Gymnasium) grades in order to deepen and broaden the knowledge of natural science education, develop practical abilities of students and their scientific researcher's competence. Students are advised to do five experimental works in this field. The program engages a basic educational method – inquiry-based learning. The results of the pedagogical experiment and the questionnaire survey are discussed. It can be stated that educational experimental activities are necessary and useful for students. By using these experimental activities, students can be provided with educational material in an attractive form, which stimulates the interest in the subject. Program participants have deepened and expanded their knowledge of energy and thermal processes in nature. Students improved their competence in natural science research. They learned how to plan and perform experiments, acquired the ability to formulate hypotheses, to make assumptions, to analyse and explain results, and to formulate reasoned conclusions. Students acquired practical skills to work properly and safely with devices and tools (computer systems Nova 5000 and Xplorer GLX, temperature, humidity sensors, caliper, scales, etc.) Students liked to be young researchers; they felt the joy of discovery by practically experimenting and independently exploring natural phenomena.
IOER International Multidisciplinary Research Journal
IOER International Multidisciplinary Research Journal ( IIMRJ)
The Philippine K to 12 science curriculum is a learner-centered and inquiry-based discipline that requires learners to utilize learning materials and learning spaces needed for a meaningful understanding of the scientific concepts and for developing their scientific literacy. This is anchored to the constructivism theory that supports 'learning by doing.' A laboratory is an essential place for active learning and science teaching that would provide students with opportunities to think creatively and critically to solve real-world problems. This study assessed the current status of the science laboratory facilities in two public junior high schools in the province of Lanao del Sur. This is to assess the current condition and availability of laboratory facilities and to identify the challenges faced by science teachers. This study employed descriptive case study method, in which the participants were from two selected schools in Lanao del Sur. A researcher-made checklist of laboratory facilities and semi-structured interviews were used to gather the data. Frequency was used as a statistical tool for quantifying the number of available laboratory facilities and equipment. Based on the findings, both schools have inadequate laboratory facilities that hinder the performance of the activities in the science module designed by the Department of Education. The lack of a laboratory room, the inadequacy of laboratory facilities and science equipment, defective laboratory equipment, the inadequacy of learning materials, lack of water supply, lack of electricity are common issues in both schools. Teacher-respondents of this study have difficulty in teaching some science concepts and are not fully equipped on how to use some science equipment. Addressing the identified challenges is recommended to achieve quality education for all.
recsam.edu.my
Lilia Halim
Hakim cerdas
School Science and Mathematics
Lynn Stewart
Loading Preview
Sorry, preview is currently unavailable. You can download the paper by clicking the button above.
RELATED PAPERS
Science Education International
RAM BABU PAREEK
Irish Journal of Academic Practice
Julie Dunne
Mujib Ubaidillah
Shanlax International Journal of Education
Mustafa Hamalosmanoğlu
Jurnal Pijar Mipa
ASTRID S . W . SUMAH
Laurinda Leite Leite
International Journal on New Trends in Education and Their Implications
HARUN ÇELİK , Orhan Karamustafaoğlu
East Asian Journal of Multidisciplinary Research
John Mart Elesio
Procedia - Social and Behavioral Sciences
Sevgül Çalış
EURASIA Journal of Mathematics, Science and Technology Education
manuel vidal
Science Educator
Todd Campbell
Journal of Research in Science Teaching
John Penick
Jurnal Ilmiah Pendidikan Fisika Al-Biruni
mulia rahmi
Bekele Oljira Negero
Ozlem Afacan
Journal of Turkish Science Education
Sulaiman Al-Balushi , Abdullah Ambusaidi
Arief Muliandi
Journal Of European Education
Bayram Akarsu
Unnes Science Education Journal
Rumoh Nektu
University College of Education Osmania University
Learning Environments Research
Christine Luketic , Erin Dolan
ATBU Journal of Science, Technology and Education
Afees Amuda
Aykut Emre Bozdogan
Open Access Publishing Group
- We're Hiring!
- Help Center
- Find new research papers in:
- Health Sciences
- Earth Sciences
- Cognitive Science
- Mathematics
- Computer Science
- Academia ©2024
Numerical and laboratory experiments on the toppling behavior of a massive single block: a case study of the Furnas Reservoir, Brazil
- Original Paper
- Published: 10 June 2024
Cite this article
- Shu-wei Sun ORCID: orcid.org/0000-0003-0326-8531 1 ,
- Qiang Wen 1 ,
- Maria do Carmo Reis Cavalcanti 2 ,
- Xiao-rui Yang 1 &
- Jia-qi Wang 1
107 Accesses
7 Altmetric
Explore all metrics
A massive toppling failure occurred at the edge of the Furnas Reservoir at 12:30 (UTC-03) on 8 January 2022, in Brazil. The toppling belongs to single-block toppling with a volume of about 3.32 × 10 2 m 3 and caused 10 deaths and 32 injuries. Field investigation, numerical analysis, and base friction tests were performed to explore the failure characteristics and mechanism of the toppling. A conceptual model of the toppling mechanism was constructed and the toppling process was divided into four stages: foundation erosion and weakening stage, crack propagation and dislocation stage, opening up and rotation stage, and disintegration and collapse stage. A series of real three-dimensional numerical simulations was performed to clarify the toppling evolution and related triggering mechanism using the finite difference program FLAC 3D . Two different alternatives of triggering mechanism for the toppling were comparatively analyzed, the first with a reduction in the shear strength of the weak foundation layer believed to represent the foundation weakening mechanism, and the second with removal of the weak layer believed to represent the foundation erosion mechanism. We found that the foundation weakening of the weak layer resulted in a sliding mechanism of the block, while the foundation erosion resulted in a clear toppling mechanism of the block. The base friction test was conducted to investigate the toppling process and to verify the numerical results over a limited time span. The experimental evidence demonstrated a good agreement with the numerical results as well as those observations in the field. We concluded that the slope was in a critical state due to the foundation erosion of the weak layer, while the heavy rainfall triggered the toppling. It is emphasized that the undermining of the slope foundation and/or existed cavities induced by the foundation erosion played a vital role in the formation of the toppling. Moreover, a produced vertical crack or the propagation of an existed crack in the rear part of the slope may be signs of movements and thought of precursors of the single-block toppling. Dealing with the eroded cavities was suggested to be an effective way to prevent the toppling in the Furnas Reservoir, such as backfilling the eroded cavities with masonry rubble and/or grouting. The understanding of the toppling characteristics and mechanism may offer a reference for single-block toppling issues, such as its movement characteristics and failure mechanism, and may be used for stability analysis and disaster identification of potential failures.
This is a preview of subscription content, log in via an institution to check access.
Access this article
Price includes VAT (Russian Federation)
Instant access to the full article PDF.
Rent this article via DeepDyve
Institutional subscriptions
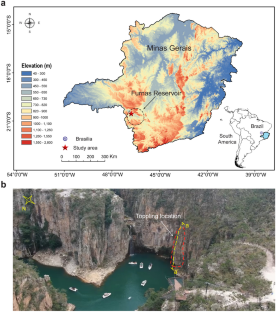
Similar content being viewed by others
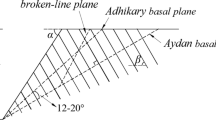
Evolution mechanism and deformation stability analysis of rock slope block toppling for early warnings
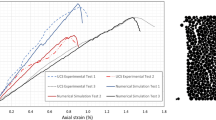
A discrete element method-based simulation of block-flexural toppling failure
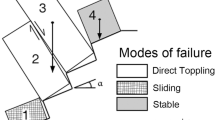
Investigation and modeling of direct toppling using a three-dimensional distinct element approach with incorporation of point cloud geometry
Availability of data and material.
All data and material used to support the findings of this study are included in the article.
Code accessibility
All codes used to support the findings of this study are included in the article.
Abramson LW, Lee TS, Sharma S, Boyce GM (2002) Slope stability and stabilization methods, 2nd edn. Wiley, New York, pp 462–591
Google Scholar
Adhikary DP, Dyskin AV (2007) Modelling of progressive and instantaneous failures of foliated rock slopes. Rock Mech Rock Eng 40(4):349–362
Article Google Scholar
Adhikary DP, Dyskin AV, Jewell RJ, Stewart DP (1997) A study of the mechanism of fiexural toppling failure of rock slopes. Rock Mech Rock Engng 30(2):75–93
Alejano LR, Go´mez-Ma´rquez I, Martı´nez-Alegrı´a R (2010) Analysis of a complex toppling-circular slope failure. Eng Geol 114:93–104
Alejano LR, Carranza-Torres C, Giani GP, Arzúa J (2015) Study of the stability against toppling of rock blocks with rounded edges based on analytical and experimental approaches. Eng Geol 195:172–184
Alzo’ubi AK, Martin CD, Cruden DM (2010) Influence of tensile strength on toppling failure in centrifuge tests. Int J Rock Mech Min Sci 47(6):974–982
Amini M, Majdi A, Veshadi MA (2012) Stability analysis of rock slopes against block-flexure toppling failure. Rock Mech Rock Eng 45(4):519–532
An Y, Wu Q, Shi C, Liu Q (2016) Three-dimensional smoothed-particle hydrodynamics simulation of deformation characteristics in slope failure. Geotechnique 66(8):670–680
Ashby J (1971) Sliding and toppling modes of failure in models and jointed rock slopes. M sci thesis London University Imperial College
Aydan Ö, Kawamoto T (1992) The stability of slopes and underground openings against flexural toppling and their stabilisation. Rock Mech Rock Eng 25:143–165
Borgatti L, Guerra C, Nesci O, Romeo RW, Veneri F, Landuzzi A, Benedetti G, Marchi G, Lucente CC (2015) The 27 February 2014 San Leo landslide (northern Italy). Landslides 12(2):387–394
Bozzano F, Mazzanti P, Prestininzi A (2008) A radar platform for continuous monitoring of a landslide interacting with an under-construction infrastructure. Ital J Eng Geol Environ 2:71–87
Bray JW, Goodman RE (1981) The theory of base friction models. Int J Rock Mech Min Sci & Geomech Abstr 18:453–468
Brideau MA, Stead D (2010) Controls on block toppling using a three-dimensional distinct element approach. Rock Mech Rock Eng 43(3):241–260
Brideau MA, Stead D (2012) Evaluating kinematic controls on planar translational slope failure mechanisms using three-dimensional distinct element modelling. Geotech Geol Eng 30:991–101
Casagli N (1994) Fenomeni di insatbilità in ammassi rocciosi sovrastanti un substrato deformabile: analisi di alcuni esempi nell’Appennino Settentrionale. Geol Romana 30:607–618
Chen YL, Liu GY, Li N, Du X, Wang SR, Azzam R (2020) Stability evaluation of slope subjected to seismic effect combined with consequent rainfall. Eng Geol 266:105461
Corgosinho PHC, Pinto-Coelho RM (2006) Zooplankton biomass, abundance and allometric patterns along an eutrophic gradient at Furnas Reservoir (Minas Gerais, Brazil). Acta Limnologica Brasileira 18(2):213–224
D’Ambra S, Giglio G, Lembo-Fazio A (2004) Arrangement and stabilization of the San Leo cliff. International Symposium Interpraevent 2004:103–114
De Freitas MH, Watters RJ (1973) Some field examples of toppling failure. Géotechnique 23(4):495–513
Evans RS (1981) An analysis of secondary toppling rock failures-the stress redistribution method. Q J Eng Geol Hydrogeol 14:77–86
Goodman RE, Bray JW (1976) Toppling of rock slopes. Proceedings of the Specialty Conference on Rock Engineering for Foundations and Slopes 2:201–234
Gu DM, Huang D (2016) A complex rock topple-rock slide failure of an anaclinal rock slope in the Wu Gorge, Yangtze River, China. Eng Geol 208:165–180
Haghgouei H, Kargar AR, Amini M, Esmaeili K (2020) An analytical solution for analysis of toppling-slumping failure in rock slopes. Eng Geol 265:105396
Hammah RE, Yacoub TE, Corkum BC, Curran JH (2005) The shear strength reduction method for the generalized Hoek-Brown criterion. Proceedings of the American Rock Mechanics Association
Hoek E, Bray JM (1974) Rock slope engineering. Institute of Mining and Metallurgy
Hoek E, Carranza-Torres CT, Corkum B (2002) Hoek-Brown failure criterion. Edition Proc. NARMS-TAC Conference, Toronto 1:267–273
Huang D, Ma H, Huang RQ (2022) Deep-seated toppling deformations of rock slopes in western China. Landslides 19(4):809–827
Itasca Consulting Group (2017) FLAC3D user’s and theory manuals, version 6.0, Minneapolis
Li Z, Wang JA, Li L, Wang LX, Liang RY (2015) A case study integrating numerical simulation and GB-InSAR monitoring to analyze flexural toppling of an anti-dip slope in Fushun open pit. Eng Geol 197:20–32
Li B, Feng Z, Wang GZ, Wang WP (2016) Processes and behaviors of block topple avalanches resulting from carbonate slope failures due to underground mining. Environ Earth Sci 75(8):694
Lin P, Liu X, Hu S, Li P (2016) Large deformation analysis of a high steep slope relating to the Laxiwa Reservoir. China Rock Mech Rock Eng 49(6):2253–2276
Lin F, Wu LZ, Huang RQ, Zhang H (2018) Formation and characteristics of the Xiaoba landslide in Fuquan, Guizhou. China Landslides 15(4):669–681
Mohtarami E, Jafari A, Amini M (2014) Stability analysis of slopes against combined circular–toppling failure. Int J Rock Mech Min Sci 67:43–56
Muller L (1968) New considerations on the Vajont slide. Felsmechanik and Ingenieurgeolo 6(1):1–91
National Standard of the People’s Republic of China (2013) Standard for test methods of engineering rock mass (GB/T 50266–2013). China Planning Press, Beijing (in Chinese)
Ning Y, Tang H, Wang F, Zhang G (2019) Sensitivity analysis of toppling deformation for interbedded anti-inclined rock slopes based on the Grey relation method. Bull Eng Geol Environ 78(8):6017–6032
Pasuto A, Soldati M (2013) Lateral spreading. Treatise on Geomorphology. Elsevier:239–24
Pérez-Rey I, Muñiz-Menéndez M, González J, Vagnon F, Walton G, Alejano LR (2021) Laboratory physical modelling of block toppling instability by means of tilt tests. Eng Geol 282:105994
Picarelli L, Urciuoli G, Mandolini A, Ramondini M (2006) Softening and instability of natural slopes in highly fissured plastic clay shales. Nat Hazards Earth Syst Sci 6:529–539
Pinheiro AL, Lana MS, Sobreira FG (2015) Use of the distinct element method to study flexural toppling at the Pico Mine. Brazil Bull Eng Geol Environ 74(4):1177–1186
Pritchard MA, Savigny KW, Evans SG (1988) Deep -seated slope movements in the Beaver River Valley, Glacier National Park, British Columbia. Environmental Science Geology Geography
Pritchard MA, Savigny KW (1991) The Heather Hill landslide: an example of a large scale toppling failure in a natural slope. Can Geotech J 28(3):410–422
Rocscience Inc. (2004) RocData user’s guide, version 3.0, Toronto
Robert McNeel and Associates (2020) Rhino 6 for Windows. Robert McNeel and Associates, Seattle
Sagaseta C (1986) On the modes of instability of a rigid block. Rock Mech Rock Eng 19(2):261–266
Sagaseta C, Sanchez JM, Canizal J (2001) A general analytical solution for the required anchor force in rock slopes with toppling failure. Int J Rock Mech Min Sci 38(3):421–435
Santos RM, Negreiros NF, Silva LC, Rocha O, Santos-Wisniewski MJ (2010) Biomass and production of cladocera in furnas reservoir, minas gerais, brazil. Braz J Biol 70(3 Suppl):879–887
Article CAS Google Scholar
Spreafico MC, Francioni M, Cervi F, Stead D, Bitelli G, Ghirotti M, Girelli VA, Lucente CC, Tini MA, Borgatti L (2015) Back analysis of the 2014 san leo landslide using combined terrestrial laser scanning and 3d distinct element modelling. Rock Mech Rock Eng 49(6):2235–2251
Spreafico MC, Cervi F, Francioni M, Stead D, Borgatti L (2017) An investigation into the development of toppling at the edge of fractured rock plateaux using a numerical modelling approach. Geomorphology 288:83–98
Sun SW, Fu Z, Zhang K (2016) Stability of slopes reinforced with truncated piles. Adv Mater Sci Eng 2016:1570983
Sun SW, Pang B, Hu JB, Yang ZX, Zhong XY (2021) Characteristics and mechanism of a landslide at Anqian iron mine. China Landslides 18(7):2593–2607
Sun SW, Liu L, Hu JB, Ding H (2022) Failure characteristics and mechanism of a rain-triggered landslide in the northern longwall of Fushun west open pit. China Landslides 19(10):2439–2458
Sun SW, Liu L, Yang ZX, Fu XY (2023) Toward a sound understanding of a large-scale landslide at a mine waste dump, Anshan, China. Landslides 20:2583–2602
Tamrakar NK, Yokota S, Osaka O (2002) A toppled structure with sliding in the Siwalik Hills, midwestern Nepal. Eng Geo 64(4):339–350
Tannant DD, Giordan D, Morgenroth J (2017) Characterization and analysis of a translational rockslide on a stepped-planar slip surface. Eng Geol 220:144–151
Tommasi P (1996) Stabilità di versanti naturali ed artificiali soggetti a fenomeni diribaltamento. Riv Ital di Geotec 4
Tu X, Dai F, Lu X, Zhong H (2007) Toppling and stabilization of the intake slope for the Fengtan Hydropower Station enlargement project. Mid-South China Eng Geol 91(2–4):152–167
Vanneschi C, Eyre M, Venn A, Coggan JS (2019) Investigation and modeling of direct toppling using a three-dimensional distinct element approach with incorporation of point cloud geometry. Landslides 16:1453–2146
Vlcko J (2004) Extremely slow slope movements influencing the stability of Spis Castle, UNESCO site. Landslides 1:67–77
Wang SJ (1981) On the mechanism and process of slope deformation in an open pit mine. Rock Mech 13(3):145–156
Wang YK, Sun SW, Pang B, Liu L (2020) Base friction test on unloading deformation mechanism of soft foundation waste dump under gravity. Measurement 163:108054
Wen BP, Aydin A (2005) Mechanism of a rainfall-induced slide-debris flow: constraints from microstructure of its slip zone. Eng Geol 78(1–2):69–88
Weng MC, Chang CY, Jeng FS, Li HH (2020) Evaluating the stability of anti-dip slate slope using an innovative failure criterion for foliation. Eng Geol 275:105737
Wong RHC, Chiu M (2001) A study on failure mechanism of block-flexural toppling by physical modelling testing. DC Rocks 2001, The 38th U.S. Symposium on Rock Mechanics (USRMS):ARMA-01–0989
Wyllie DC (1980) Toppling rock slope failures examples of analysis and stabilization. Rock Mech Felsmechanik Mécanique Roches 13:89–98
Wyllie DC (2018) Rock slope engineering: civil applications, 5th edn. Taylor & Francis Group, Boca Raton
Wyllie DC, Mah C (2004) Rock slope engineering. CRC Press
Yeung MR, Wong KL (2007) Three-dimensional kinematic conditions for toppling. In: 1st Canada-U.S. Rock Mechanics Symposium. American Rock Mechanics Association Vancouver Canada
Yin YP, Sun P, Zhang M, Li B (2011) Mechanism on apparent dip sliding of oblique inclined bedding rockslide at Jiweishan, Chongqing, China. Landslides 8:49–65
Zábranová E, Matyska C, Stemberk J, M´alek, (2020) Eigenoscillations and stability of rocking stones: the case study of “The Hus Pulpit” in the Central Bohemian Pluton. Pure Appl Geophys 177:1907–2191
Zanbak C (1983) Design vharts for rock slopes susceptible to toppling. J Geo Eng 109:1039–1061
Zhang JH, Chen ZY, Wang XG (2007) Centrifuge modeling of rock slopes susceptible to block toppling. Rock Mech Rock Eng 40(4):363–382
Zhang G, Wang F, Zhang H, Tang H, Li X, Zhong Y (2018) New stability calculation method for rock slopes subject to flexural toppling failure. Int J Rock Mech Min Sci 106:319–328
Zheng Y, Chen C, Liu T, Xia K, Liu X (2018) Stability analysis of rock slopes against sliding or flexural-toppling failure. Bull Eng Geol Environ 77(4):1383–1403
Zheng Y, Chen C, Liu T, Zhang H, Sun C (2019) Theoretical and numerical study on the block-flexure toppling failure of rock slopes. Eng Geol 263:105309
Download references
Acknowledgements
We would like to express our gratitude to the editors and reviewers for their constructive and helpful comments.
Shu-wei Sun was supported by the National Key Research and Development Plan (No. 2017YFC1503103) and the National Natural Science Fund of China (No. 51574245) and the Fundamental Research Funds for the Central Universities (2021YJSNY16).
Author information
Authors and affiliations.
School of Energy and Mining Engineering, China University of Mining and Technology (Beijing), Beijing, 100083, China
Shu-wei Sun, Qiang Wen, Xiao-rui Yang & Jia-qi Wang
Department of Civil Construction, Federal University of Rio de Janeiro, Rio de Janeiro, 21941901, Brazil
Maria do Carmo Reis Cavalcanti
You can also search for this author in PubMed Google Scholar
Contributions
All authors contributed to this study. Shu-wei Sun designed the research study. Shu-wei Sun and Qiang Wen analyzed the field data. Maria do Carmo Reis Cavalcanti supported some field data. Shu-wei Sun and Qiang Wen wrote this paper. Xiao-rui Yang conducted the base friction model test. Jia-qi Wang reviewed the manuscript. All authors gave final approval for publication.
Corresponding author
Correspondence to Shu-wei Sun .
Ethics declarations
Conflict of interest.
The authors declare no competing interests.
Rights and permissions
Springer Nature or its licensor (e.g. a society or other partner) holds exclusive rights to this article under a publishing agreement with the author(s) or other rightsholder(s); author self-archiving of the accepted manuscript version of this article is solely governed by the terms of such publishing agreement and applicable law.
Reprints and permissions
About this article
Sun, Sw., Wen, Q., do Carmo Reis Cavalcanti, M. et al. Numerical and laboratory experiments on the toppling behavior of a massive single block: a case study of the Furnas Reservoir, Brazil. Landslides (2024). https://doi.org/10.1007/s10346-024-02288-8
Download citation
Received : 04 December 2023
Accepted : 20 May 2024
Published : 10 June 2024
DOI : https://doi.org/10.1007/s10346-024-02288-8
Share this article
Anyone you share the following link with will be able to read this content:
Sorry, a shareable link is not currently available for this article.
Provided by the Springer Nature SharedIt content-sharing initiative
- Single-block toppling
- Failure mechanism
- Foundation erosion
- 3D numerical analysis
- Base friction test
- Find a journal
- Publish with us
- Track your research

- Facts and Figures
- Undergraduate Admissions
- Graduate Admissions
- Non-traditional Admissions
- Pay Deposit
- Undergraduate Majors
- Graduate Programs
- Honors College
- Study Abroad
- Professional & Continuing
- Online Programs
- Career Planning
- Living on Campus
- Clubs & Organizations
- Spirit & Traditions
- About Harrisonburg
- Pay Your Deposit
- Office of Financial Aid
- Freshman Scholarships

- James Madison University -->
- Department of Sociology and Anthropology
- Inclusive Excellence
- Student Research Opportunities
- Gender And Science Ethnography Lab
- JMU Archaeology Research Lab
- Student Resources
- Student Award Winners
- AWARDS AND SCHOLARSHIPS
- Field Programs

The JMU Archaeology Research Laboratory is located in the Frye building. The laboratory is directed by Dr. Dennis Blanton , Dr. Di Hu , and Dr. Julie Solometo . To find out more about some of the recent work we are doing with students, click below.

Shared artifact processing, lab analysis, and storage space

Classroom space

Reference Collection

American Southwest Lab
American southeast lab, experimental archaeology and geoarchaeology lab.
Back to Top

- Expenditures
- Accessibility
- Social Media

An official website of the United States government
Here's how you know
Official websites use .gov A .gov website belongs to an official government organization in the United States.
Secure .gov websites use HTTPS A lock ( ) or https:// means you’ve safely connected to the .gov website. Share sensitive information only on official, secure websites.
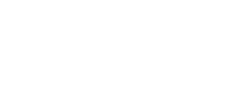
- Digg
Latest Earthquakes | Chat Share Social Media
Behavioral Toxicology Core Technology Team
Attractance behavior of asian carp when food stimulus is introduced, a rack of eight aquaria used for behavior modification studies, video recording setup from an experimental swimming behavior assay.
- Publications
About the Research.
The Environmental Health Program supports scientists in the Behavioral Toxicology Core Technology Team (CTT) at the Columbia Environmental Research Center. The scientists identify how contaminants alter the behavior of organisms and what implication those changes may have on individuals, populations, and communities.
Behavioral methodology is becoming increasingly important in assessing the health and viability of natural populations to understand the challenges posed by natural stressors and to support conservation and recovery efforts.
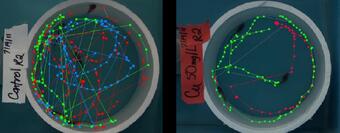
Sublethal toxicity testing and sensitive whole organism endpoints, like behavior and neuromotor function, are of emerging importance in the field of ecotoxicology. Behavioral responses are mediated through the integration of neural, neuroendocrine and neuromuscular signals, contributing to complex and highly variable inter-individual responses in exposed organisms. These overarching themes in the field of animal behavior can be universally applied across toxicological agents, model organisms, organism life stage and endpoints.
The Behavioral Toxicology CTT has utilized its behavioral expertise and facilities to study a wide range of questions related to behavior directly related to contaminants - providing tools to better understand the effects of contaminants on behavior and how they might translate to effects on growth, reproduction, and population size.
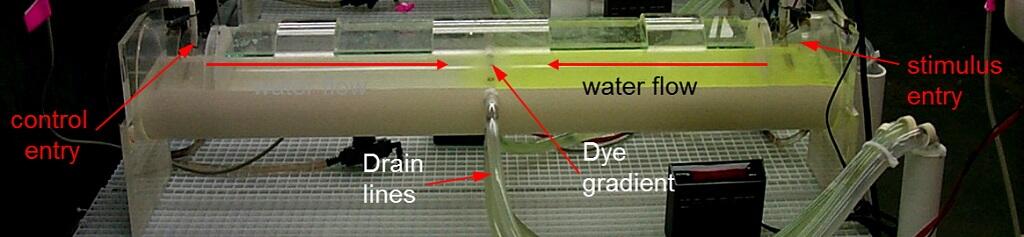
Key Instrumentation and Capabilities
- Laboratory dedicated to behavior research
- Two proportional diluters equipped with high-definition cameras for quantifying swimming activity across chemical concentration gradients
- Five (3 small and 2 large) respirometers for measuring swimming performance
- Five countercurrent avoidance chambers for characterizing avoidance or attractance to stimuli
- Electro-olfactogram recordings (olfactory cues)
- High-throughput chamber for tracking behavior of small organisms
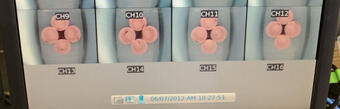
Current Science Questions and Activities
- International collaboration to standardize the zebrafish dark/light transition test.
- What effects do algal toxins have on fish health including sublethal and behavioral endpoints?
- What effects does thiamine deficiency have on fish behavior and can these effects be reversed with supplementation?
- Does carbamazepine, an anticonvulsant pharmaceutical, alter fish eye development and behavior?
Environmental Health Integrated Science Team Collaborators
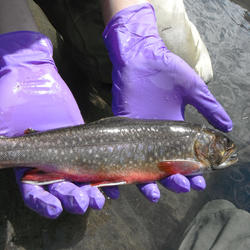
Fishing and Hunting Integrated Science Team
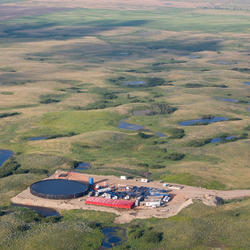
Energy Integrated Science Team
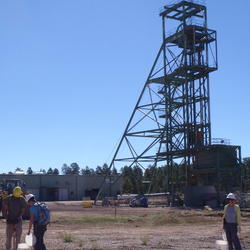
Minerals Science Team
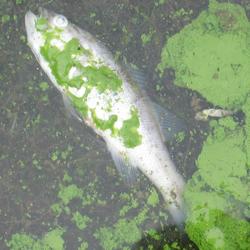
Toxins and Harmful Algal Blooms Science Team
Science activities related to the Behavioral Toxicology Core Technology Team can be found below.
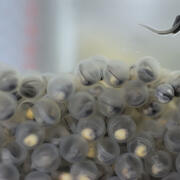
Do Trace Metal Concentrations in the Upper Columbia River Affect Early Life Stage White Sturgeon?
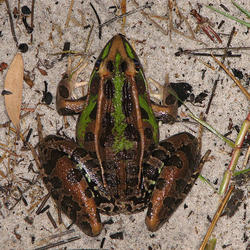
Clothianidin Exposure Associated with Changes in Tadpole Behavior
Data related to the Behavioral Toxicology Core Technology Team can be found below.
Behavioral Effects of Copper on Larval White Sturgeon
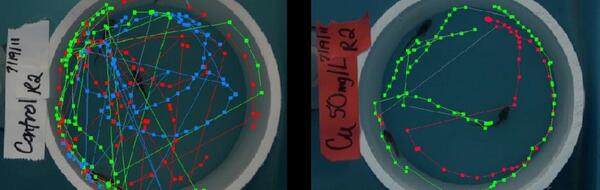
Behavioral Toxicology Laboratory -- Columbia, Missouri
Behavioral Toxicology Laboratory -- Columbia, Missouri. Swimming paths of control (left) and copper (right) exposed fish
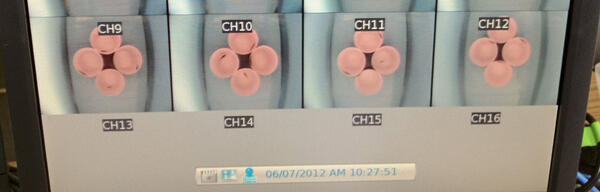
Video recording setup from a swimming behavior assay system
Behavioral Toxicology Laboratory — Columbia, Missouri. Screen shot of a computer monitor from a video recording setup from a swimming behavior experimental swimming behavior assay system. U.S. Geological Survey (USGS) scientists can record swimming activity of multiple treatments simultaneously in the swimming behavior assay.
Scientific publications related to the Behavioral Toxicology Core Technology Team can be found below.
Ammonia and aquatic ecosystems – A review of global sources, biogeochemical cycling, and effects on fish
Copper concentrations in the upper columbia river as a limiting factor in white sturgeon recruitment and recovery, effects of the neonicotinoid insecticide clothianidin on southern leopard frog (rana sphenocephala) tadpole behavior, behavioral effects of copper on larval white sturgeon, potential toxicity of dissolved metal mixtures (cd, cu, pb, zn) to early life stage white sturgeon (acipenser transmontanus) in the upper columbia river, washington, united states, sensitivity of lake sturgeon ( acipenser fulvescens ) early life stages to 2,3,7,8-tetrachlorodibenzo- p -dioxin and 3,3′,4,4′,5-pentachlorobiphenyl, quantifying fish swimming behavior in response to acute exposure of aqueous copper using computer assisted video and digital image analysis, acute sensitivity of white sturgeon ( acipenser transmontanus ) and rainbow trout ( oncorhynchus mykiss ) to copper, cadmium, or zinc in water-only laboratory exposures.
The 5-Day Friendship Challenge
Strengthen your bonds and find out what kind of friend you are with this weeklong friendship tuneup.
- Share full article

By Catherine Pearson
Leer en español
Welcome to Well’s 5-Day Friendship Challenge!
This week, we’re bringing you five science-backed strategies to help revive fizzling friendships and to deepen your close ties. Start by taking our quiz to discover your friendship style, then strengthen your bonds with each day’s exercise.
Day 1: Text a friend

I’m Catherine Pearson, and I cover families and relationships for The New York Times. Today, I’m making the case for something many of us have a love-hate relationship with: texting.
Recently, I was having a lousy day. My husband was out of town, and the kids were fighting nonstop. Just as I was about to threaten my 6- and 9-year-old boys with boarding school, a text popped up on my phone. It was from Miranda, a high-school friend whom I catch up with only a couple of times a year. She had texted simply to tell me she’d been thinking about me — it probably took her 30 seconds to write, and it took me even less time to read. But her message lifted me right out of my funk.
Ample research shows that social connection is crucial to our physical and mental health and longevity . It is good for our brains and hearts, and helps protect us against stress. One oft-quoted 2010 study concluded that lacking social connection might be comparable to smoking up to 15 cigarettes a day.
Friendship is a very specific and valuable form of social connection, said Julianne Holt-Lunstad, the lead author on the cigarette study and director of the Social Connection and Health Lab at Brigham Young University. “It’s difficult to be choosy about your neighbors or co-workers. You’re born into your family,” she explained. “Friendships are chosen and, because of that, we need to intentionally make time for them.”
Putting in the effort to maintain friendships may feel like a heavy lift, and to a certain extent it is. Research suggests people need to spend around 200 hours hanging out together in order to forge a close friendship . Unfortunately, the amount of time Americans spend engaged with friends every day has declined over the past two decades .
The good news? Research also shows that smaller efforts can help established friendships flourish. A 2022 study found that when you casually check in with a friend — the way Miranda did with that text — it’s more welcome than many of us realize.
Peggy Liu, one of the authors of that study, often writes to friends out of the blue to say, “I just thought I would say ‘hi’ and see how you’re doing.” Liu, an associate professor of business administration at the University of Pittsburgh, told me that even if it sometimes felt awkward, the practice had helped her reconnect with old friends.
Friendship Challenge Day 1: Text a friend.
Today’s challenge is a light lift — simply pick up your phone and shoot off a text. Maybe it’s for someone you’ve lost touch with. Maybe it’s for someone you’re missing. Or maybe it’s for someone you actually see quite often but want to check in with “just because.” You can use this text-message template or come up with something on your own.
Hi! Just texting to see how you're doing.
You’re not alone if reaching out feels uncomfortable. Just keep in mind what Jeffrey Hall, a professor of communication studies at the University of Kansas, told me: “It’s typically the case that when people are out of touch for a while, it’s not because they dislike each other or don’t want to hear what is going on in each other’s lives. It’s just that they have fallen into a routine of not keeping in touch.”
Discover your friendship style
Advertisement
Day 2: Repot a friendship

This is Day 2 of the 5-Day Friendship Challenge. To start at the beginning, click here .
We’ve all got them: work friends, college buddies, playground dads. Whatever you call them, they’re the discrete groups of friends from different facets of our lives. Even our “weak ties” seem to exist only in certain settings, like the neighbors you nod at while walking the dog, or the barista who has memorized your coffee order.
But there is value in decompartmentalizing such friendships, said Marisa G. Franco, a psychologist and the author of “Platonic,” a book about making and keeping friends. Research has found that connecting in different settings or contexts can help bring friends closer, she added.
Friendship Challenge Day 2: ‘Repot’ a friendship.
“Repot” is a term coined by Ryan Hubbard, who heads up Hinterland, a social lab that has generated reports on friendship. And it’s simple: Think of friends you tend to interact with in one setting. Then invite them to join you for something else.
Ask a colleague you usually gossip with on Slack to sneak out to a matinee with you. Ask a friend you normally meet for dinner to join you for a walk through a museum. Or maybe raise the stakes a bit and invite a friend on an overnight trip — you really get to know someone once you’ve hung out together in your PJs, Dr. Franco said — or to try something totally new to you both. ( Clown cardio , anyone?)
Dr. Franco pointed to research showing that sharing unusual or extraordinary experiences can sometimes help bring people together. And researchers who study romantic love have long known that novelty can nourish relationships. But it’s not all about finding activities that are unconventional or adventurous.
You can repot a relationship by asking a friend for help, Dr. Franco said, or ask if that person wants to meet your family, something we do naturally all the time as kids. You can also “integrate” your friendships, inviting people who don’t know each other to meet up.
Whatever you settle on, your overarching goal should be to “challenge the norms” of your friendship, Dr. Franco said. If you feel unsure of whom to reach out to, she recommends simply asking yourself: Is there someone I would like to feel closer to in some way?
Repotting has risks. Your friend might screech at the idea of taking a beginner’s trapeze class with you, rather than meeting for your usual glass of wine. But the only way to know is to ask, Dr. Franco said. You might also discover that you don’t like spending time with your friend in another context, which can be valuable information as well, she added.
When it works, repotting can lead to a greater sense of ease and comfort with friends, Dr. Franco said, because you are each getting a more complete picture of the other person. “Every setting,” she said, “brings out a different side of us.”
Day 3: Put a friendship on autopilot

This is Day 3 of the 5-Day Friendship Challenge. To start at the beginning, click here .
One of my favorite running middle-aged jokes on TikTok and Instagram involves two busy parent friends trying to make plans.
You know the script: “Are you free next week?” one mom shouts into her earbuds while driving car pool.
“No, I have four dance recitals, two block parties and 67 soccer games to attend,” the other mom answers, stirring a pot of chili while answering a work email.
“Next month?”
“No, we’re finally taking that vacation we’ve put off for 10 years.”
And on it goes, until they finally settle on a date in late 2026.
Making plans to socialize with friends can be challenging, no matter what stage of life you are in, said Kasley Killam, a social scientist and the author of the forthcoming book “The Art and Science of Connection.” That is why she believes that one of the best things you can do to prioritize your social health is put your friendships on autopilot by scheduling regular opportunities for connection.
“It’s about automating the logistical sides of our friendships so that we can just be present,” she said. “It ties into the fact that friendships — and all of our relationships — blossom the most when there are consistent touch points.”
Friendship Challenge Day 3: Put a friendship on autopilot.
Here are a few ways to do it:
A standing dinner date . Ask a handful of friends over to your home for an easy meal on the same day of the week every month. Add the date to your calendars, making sure it repeats each month, and whoever can make it will make it. There may be specific benefits to meeting up in real life, said Eric Kim, an assistant professor of psychology at the University of British Columbia.
Dr. Kim worked on a recent study that found having frequent face-to-face contact with friends was associated with better mental and physical health. And he’s putting what he learned into practice: Every time Dr. Kim meets up with his three closest friends, he ends the get-together by putting their next date on the calendar. Efficient!
“The more you have a routine of interacting with somebody, the less you have to work at it,” said Jeffrey Hall, a professor of communication studies at the University of Kansas. “It also gives you something to look forward to.” For example, perhaps you and a friend get together every summer to have a barbecue, or every winter when you’re back in your hometown, you visit the same friend, he said.
A weekly call or text. OK, nothing beats in-person connection. But as we already established this week , it is also true that even a brief text exchange can feel meaningful. So here comes that calendar reminder again: A pop-up might prompt you to ping the same person every week, or maybe it suggests someone new. The point is to reach out.
Break out the Post-its. A low-tech option is to place a note somewhere you are apt to see it, such as a bathroom vanity, reminding you to reach out to a friend. Or, while you are writing out your to-do list for the week, make a “to-love” list, Ms. Killam suggested. Corny? Sure. But a list like this can help you prioritize your friendships, she said.
“It’s about having these reminders and rituals so that it becomes habitual,” Ms. Killam said. “It’s so easy for our connections to just be the last thing on our to-do list.”
Day 4: Revisit old photos with a friend

This is Day 4 of the 5-Day Friendship Challenge. To start at the beginning, click here .
If you’re like me, you have a staggering number of photos saved to your phone. Does that speak to an unhealthy tendency to obsessively document even the most mundane moments? Perhaps. But today’s objective is to put your photo library to good use.
Nostalgia can be beneficial. It can curb stress and help combat feelings of loneliness. And looking back on old memories with a friend instantly makes you feel more connected, said Marisa G. Franco, a psychologist and the author of “Platonic,” a book about maintaining friendships. Something as simple as looking at an old photo of you and a friend may remind you of the depth of that bond, she said.
In fact, Dr. Franco said, one of the easiest ways to make new friends in adulthood is to simply reconnect with old ones. Revisiting cherished memories can give a fizzled friendship a much-needed jolt.
Friendship Challenge Day 4: Reminisce with a friend.
Text or email a photo or video. This is the quickest option, Dr. Franco said. You might work some details into an accompanying message, such as “I’m thinking about this moment we had together, and this is what it meant to me.”
Dig up some old photo prints. Those 8 x 10s and 5 x 7s that are just gathering dust in storage? Upload them digitally and send them to a group chat. Or have a pal come over and comb through them together while eating snacks.
Ask: “What do you remember?” Simply chatting about your shared experiences can clue you into a friend’s perspective, said Eric Kim, an assistant professor of psychology at the University of British Columbia. When recalling that camping trip you both went on years ago, you may only remember the mosquitoes and restless sleep. But talking to your friend could remind you of the beautiful waterfall you saw and the s’mores you ate.
You get new insights into a shared memory, Dr. Kim said.
Yes, reminiscing can be bittersweet. You might find yourself remembering friends who are no longer alive, or staring at a photo from a more carefree time. But you can also feel gratitude for the time you’ve shared. (And small, daily doses of gratitude have known benefits .)
“Part of reminiscing might be saying, ‘I’m so glad we had that experience together,’” said Julianne Holt-Lunstad, the director of the Social Connection and Health Lab at Brigham Young University. “Or, ‘I’m so grateful we were able to do that.’”
Day 5: Take an emotional risk

This is the final day of the 5-Day Friendship Challenge. To start at the beginning, click here .
The friendship experts I interviewed for this challenge all mentioned, in one form or another, how important vulnerability is to forming close connections. If you want big, deep platonic love in your life, you must be willing to put yourself out there emotionally.
Those therapists and researchers also acknowledged that the very idea of vulnerability makes a lot of us squirm.
“You risk rejection, exposure, judgment,” said Hope Kelaher, a licensed clinical social worker in private practice in New York City and the author of “Here to Make Friends.” “But it is the core component of any deep emotional intimacy.”
Friendship Challenge Day 5: Be vulnerable with a friend.
“Expose myself emotionally” probably wasn’t on your to-do list when you woke up, so here are a few ideas to help you start.
Ask a probing question (or 36 of them). Nearly a decade ago, The New York Times ran the article “ The 36 Questions That Lead to Love ” — which included a set of, yes, 36 questions that could help accelerate intimacy.
The questions had been generated for a study by researchers including Arthur Aron, a professor of psychology at Stony Brook University. Dr. Aron told me that he and his team had developed the questions to test whether they could create closeness between strangers, but there is growing evidence they can increase closeness between friends and romantic partners, too. Running through the full set takes about 45 minutes, and the questions get progressively deeper. Answer them with a friend to help foster mutual vulnerability.
Confide in someone new. One simple strategy is to think about who you typically talk to about thorny issues at home or work, said Marisa G. Franco, a psychologist and the author of “Platonic.” Instead of going to that person, talk to another friend you’d like to bond with. You might share something you are struggling with, she suggested, though she acknowledged that was a high-risk (and high-reward!) proposition. If you need a confidence boost, keep the “ beautiful mess effect ” in mind: Research suggests that though we tend to worry being vulnerable will make us seem weak or flawed, others tend to see it as courageous and authentic.
Offer a sincere compliment. Going deeper with a friend does not necessarily mean you must unburden yourself emotionally. Jeffrey Hall, a professor of communication studies at the University of Kansas, has worked on research showing that offering a sincere compliment to a friend can increase your own happiness and lower stress levels over the course of a day. Though telling a friend what you appreciate about him or her might feel awkward, it will probably be more welcome than you would expect .
OK, maybe it’s just me, but after spending the week together working through this challenge, I feel like we’re best friends now? I’ll keep up with your feedback in the comment sections , so please post there and let me — and your fellow readers — know how the exercises turned out for you. If you’d like to suggest other forms of connecting, drop them there, too.
I hope these exercises have been a reminder to make time for friendship. Investing in our social connections is like investing in a 401(k), as Ms. Kelaher told me. It’s a way of planning for our future stability — and well-being.

IMAGES
VIDEO
COMMENTS
3. Examples of - and best practices for - survey experiments a. Key concepts in experimental design b. Best practices for experimental design 4. Data sources 5. Analyzing survey experimental data a. Not the same as analyzing a lab experiment; not the same as analyzing a correlational survey 6.
This module provides an introduction to the use of complex laboratory experiments, field experiments, naturalistic observation, survey research, nonconscious techniques, and archival research, as well as more recent methods that harness the power of technology and large data sets, to study the broad range of topics that fall within the domain ...
Typically, scholars distinguish among laboratory experiments, field experiments, and survey experiments (Druckman et al. 2011). 5 Laboratory experiments are conducted in controlled environments, in which nearly every part of a participant's experience is (to the extent possible) created by the researcher.
1. Lab Experiment. A laboratory experiment in psychology is a research method in which the experimenter manipulates one or more independent variables and measures the effects on the dependent variable under controlled conditions. A laboratory experiment is conducted under highly controlled conditions (not necessarily a laboratory) where ...
Survey experiments are widely used by social scientists to study individual preferences. This guide discusses the functions and considerations of survey experiments. 1 What is a survey experiment. A survey experiment is an experiment conducted within a survey. In an experiment, a researcher randomly assigns participants to at least two ...
Table of contents. Step 1: Define your variables. Step 2: Write your hypothesis. Step 3: Design your experimental treatments. Step 4: Assign your subjects to treatment groups. Step 5: Measure your dependent variable. Other interesting articles. Frequently asked questions about experiments.
And as the opening example makes clear, survey research can even be used to conduct experiments to test specific hypotheses about causal relationships between variables. Such studies, when conducted on large and diverse samples, can be a useful supplement to laboratory studies conducted on university students.
Overview. Survey experiments have emerged as one of the most powerful methodological tools in the social sciences. By combining experimental design that provides clear causal inference with the flexibility of the survey context as a site for behavioral research, survey experiments can be used in almost any field to study almost any question.
A thorough and comprehensive guide to the theoretical, practical, and methodological approaches used in survey experiments across disciplines such as political science, health sciences, sociology, economics, psychology, and marketing This book explores and explains the broad range of experimental designs embedded in surveys that use both probability and non-probability samples. It approaches ...
6 Laboratory Experiments in Political Science; 7 Experiments and Game Theory's Value to Political Science; 8 The Logic and Design of the Survey Experiment; ... The modern survey experiment is the biggest change in survey research in a half century. There is some interest in how it came about, I am told. So I begin by telling how I got the idea ...
Fig. 3 separates out experimental publications in the top social science journals by type: behavioral lab study, survey experiment, or field experiment (including audit studies). The number of laboratory experiments has held steady while representation of survey and field experiments has increased from almost zero to now representing around ...
Survey experimentS for CauSal inferenCe Survey experiments for causal inference are experiments that happen to be embedded in a survey instrument. Respondents are ran-domly assigned to different versions of a treatment, and then they answer one or more outcome questions.5 Much like in field and laboratory experiments, the researcher can
Population-based survey experiments have become an invaluable tool for social scientists struggling to generalize laboratory-based results, and for survey researchers besieged by uncertainties about causality. Thanks to technological advances in recent years, experiments can now be administered to random samples of the population to which a theory applies.
These experimental settings combine treatment manipulation and random assignment with survey sampling, ensuring a broader variation of the pool of subjects being considered and helping bring experimental research outside of the lab. Survey experiments may be conducted with either non-probability or probability samples of participants; when ...
The Harvard Digital Lab for the Social Sciences (DLABSS) allows you to contribute to Harvard social science research, right from your computer. As an online community of researchers and volunteers committed to advancing social science research, our projects explore important and fascinating issues in society.
Field research refers to the research conducted outside the laboratory or workplace. Surveys are the best example of field research. On the contrary, Experiment is an example of laboratory research. A laboratory research is nothing but research carried on inside the room equipped with scientific tools and equipment.
Experimental realism is a benefit distinguishing field experiments from other methods with internal validity, such as survey experiments and lab experiments (Baekgaard et al. 2015). Field experiments might become central to new research agendas in public administration.
Survey experiments are now quite common in political science. A recent analysis of the number of mentions of this term in political science journal articles demonstrates a dramatic increase from 2000 to 2013. In addition, the term survey experiment has been picked up by many other disciplines, by researchers in a variety of different countries. ...
Laboratory Research for Experiment and Survey; Laboratory research usually makes use of experiments whereas field research largely profits from surveys. Equipment needed for Experiment vs Survey; Experiments often use various equipment in facilitating treatments and in observing responses while surveys do not need such elaborate tools.
A laboratory experiment, as the name implies, takes place in a laboratory environment under controlled conditions. The scientist performing the experiment chooses the conditions—place, time, other participants—and follows the scientific method to the letter. ... Meanwhile, a psychologist may conduct informal surveys of different groups of ...
laboratory experiments and those who partic ipate in field markets. People self-select into field markets, but are often recruited to par ticipate in surveys or experiments. A number of recent papers have provided detailed dis cussions on factors affecting the divergence in laboratory and field behavior, focusing on
By offering both single-choice and multiple-choice questions, the survey aims to capture. a comprehensive view of respondents' experiences and preferences when it comes to laboratory work. Participants are also encouraged to. share their thoughts on the resources and safety measures that play a crucial role in enhancing the laboratory experience.
In experiments usually laboratory equipment are used in various activities during the experiment process. 12. It is vital in co-relational analysis. It is vital in casual analysis. 13. No manipulation is involved in surveys. Manipulation is involved in experiments. 14. In surveys data is collected through interview, questionnaire, case study etc.
While some experiments require expensive lab equipment or dangerous chemicals, there are plenty of cool projects you can do with regular household items. We've rounded up a big collection of easy science experiments that anybody can try, and kids are going to love them! Jump to: Easy Chemistry Science Experiments; Easy Physics Science Experiments
MOUNT CARMEL SCHOOL OF MARIA AURORA (MCSMA), INC. Senior High School AN EVALUATION OF THE SCHOOL LABORATORY AS AID FOR LEARNING OF STEM STUDENTS SY. 2019 - 2020 A Research Presented to the Faculty of Senior High School Department Mount Carmel In School of Maria Aurora Fulfillment of the Requirements for the K-12 Curriculum By: Teh, Nichole Angel M. Fajardo, Flora Belle G. October 2019 i MOUNT ...
The DEM data were obtained from a UAV aerial survey conducted by the Furnas Centrais Eletricas. The data, which have a 1-m resolution, were processed using the tool of ArcGIS. ... The laboratory model experiments were designed by adopting the base friction principle to study the failure process of toppling (Bray and Goodman 1981). The ...
The lab is equipped to run controlled ancient projectile experiments. The lab also has microscopes with the capability to study microscopic remains of food residues on ancient tools and usewear on ancient tools. Access to a Niton XL5 Analyzer (pXRF) enables the analysis and characterization of geological raw source materials and igneous stone ...
Behavioral Toxicology Laboratory — Columbia, Missouri. Screen shot of a computer monitor from a video recording setup from a swimming behavior experimental swimming behavior assay system. U.S. Geological Survey (USGS) scientists can record swimming activity of multiple treatments simultaneously in the swimming behavior assay.
Friendship is a very specific and valuable form of social connection, said Julianne Holt-Lunstad, the lead author on the cigarette study and director of the Social Connection and Health Lab at ...
16 The incentive structure was a random lottery incentive mechanism (RLIM), which is commonly used as an incentive mechanism in experiments that have multiple lotteries (Cubitt, Starmer, & Sugden, Citation 1998; Cubitt et al., Citation 1998).The incentive payouts were made based on a randomly chosen round from one of the three experiments played in each session, as eluded to in footnote 5.